- 1Wildlife Conservation Research Unit, Department of Biology, University of Oxford, Oxford, United Kingdom
- 2Kaminando Habitat Connectivity Initiative, Oakland, CA, United States
- 3Department of Biology, Northern Arizona University, Flagstaff, AZ, United States
- 4Department of Biology, Humboldt State University, Arcata, CA, United States
Aim: The goal of this study was to evaluate consistency among multiple connectivity models for jaguar and puma across Panama to evaluate the plausible current patterns of habitat connectivity for these and potentially other species in this critical biogeographic linkage zone.
Approach: We compared 72 different models of landscape connectivity for both large felids using both empirically based and expert opinion derived resistance layers. We conducted resistant kernel modeling with different dispersal abilities to reflect uncertainty in the movement potential of the two species. We applied three transformations to the resulting connectivity surfaces to account for uncertainty about the shape of the dispersal kernel function. We then evaluated the similarities and differences among these connectivity models, identifying several factors that drive their differences. We quantified the factors that drive differences in connectivity predictions using surface correlation, Mantel testing, and agglomerative hierarchical clustering.
Results: We found that the main differences among predicted connectivity surfaces were related to species and resistance modeling approach, with relatively little consistent difference related to dispersal ability and nonlinear kernel transformation. Based on the ensemble connectivity prediction across the 72 models, we identified two major core areas, corresponding to the eastern and western portions of the central mountain range, significant attenuation of connectivity in lowland and developed areas of Panama, a major breakage in connectivity in the Canal Zone spanning the width of the country, and weak but potentially critical movement routes connecting the two core areas across the Canal Zone.
Implications: This paper contributes to both a theoretical and practical understanding of the functional connectivity of large felids, confirming the strong effect of differences in source points and resistance surfaces on connectivity predictions and identifying and mapping key core areas, barriers, and potential corridors for carnivore movement across the critical Pan-American linkage of the Isthmus of Panama.
1 Introduction
In the Western Hemisphere, Beringia, and the Isthmus of Panama drove significant biogeographical changes during the Tertiary Period. The Isthmus has also played a key role in global biogeography and phylogeography. Over the last 20 million years, its periodic emergence initiated the Great American Biotic Interchange between North and South America (Woodburne, 2010) and considerably impacted marine phylogeography (O’Dea et al., 2016).
Panama now plays a similar role in maintaining population connectivity between species in Central, South, and North America. However, rapid human development, including land use change, urbanization, agricultural development, and notably the Panama Canal, along with the lakes and reservoirs created for its operation, have greatly impeded potential connectivity through the narrow passageway of the Isthmus between North and South America (Myers and Tucker, 1987; Carr et al., 2006). This linkage’s attenuation, and potential breakage, are of great conservation relevance in this era of increasing interest in broad-scale ecological networks connected by corridors and connectivity routes to maintain dynamic viable populations (Rabinowitz and Zeller, 2010; Olsoy et al., 2016).
All large terrestrial carnivores currently extant in South America colonized the continent from North America through trans-isthmian dispersal and represent the biogeographic link between North and South America. The jaguar (Panthera onca) and puma (Puma concolor) are two such species that dispersed through Central America and subsequently colonized South America (Woodburne, 2010). Today, these Neotropical felids are experiencing population declines and severe range contractions owing to an increased loss and fragmentation of habitat, prey depletion, and human conflict (De La Torre et al., 2018; Jędrzejewski et al., 2018; Guerisoli et al., 2021). As wide-ranging apex predators–sharing similar habitats and behavioral traits–they require extensive interconnected landscapes with abundant prey to maintain viable populations to persist and adapt to changing environmental conditions (Scognamillo et al., 2003; Harmsen et al., 2009; Craighead and Yacelga, 2021; Montalvo et al., 2023). In Panama, Craighead et al. (2022) identified seasonal jaguar and puma habitat selection at multiple scales, highlighting the structural connectivity among the habitats that shape the country’s landscape. While jaguars preferred primary forest and showed a negative association with human disturbance, pumas were relatively resilient to forest disturbances and ventured into secondary forest and human-altered habitats. Throughout the range of these two species, the jaguar appears to be more vulnerable to habitat loss (De La Torre et al., 2018; Jędrzejewski et al., 2018; Villalva and Palomares, 2022).
In Panama, at a national scale, there are few species connectivity assessments based on empirical habitat suitability predictions (Meyer et al. 2015; Meyer et al., 2020a; Meyer et al., 2020b). However, species connectivity predictions for Panama are imperative given its biogeographic context as a trans-hemisphere linkage, connecting Central America with the Tumbes–Chocó–Magdalena and Andean-Amazonian biogeographic zones, providing an ecological connection between Nearctic and Neotropical regions. The Tumbes–Chocó–Magdalena region extends from easternmost Panama to the Pacific coast of Colombia, and Ecuador. It is a biodiversity hotspot harboring high endemicity (Myers et al., 2000). Thus, the Isthmus of Panama serves as a critical biogeographic node facilitating dispersal and gene flow between the Americas. It is a key linkage, enabling crucial evolutionary processes between distinct biogeographic zones that shaped New World diversification.
Owing to the threat of further landscape conversion and habitat loss, and the urgent need to inform land use policy, this paper aims to predict, compare, and combine 72 different models of landscape connectivity for jaguars and pumas across Panama to evaluate the plausible current range of habitat connectivity for the species in this critical biogeographic linkage zone. There are many different approaches to modelling connectivity, as a function of landscape resistance, dispersal ability, and the distribution of source points. Frequently used approaches include resistant kernel modeling (Compton et al., 2007), factorial least cost path modeling (Cushman et al., 2009), circuit theory current flow (McRae and Beier, 2007), graph metrics of network connectivity (Saura and Torné, 2009), and least cost corridors (Singleton et al., 2002). In addition, several recent papers have used agent-based simulation modeling to evaluate the relative performance, and difference in predictions, produced by these different methods (e.g., Unnithan Kumar et al., 2022; Lumia et al., in press1). These papers have found, consistent with past empirical results (e.g., Cushman et al., 2014; Zeller et al., 2018), that under most realistic conditions and for most questions, the resistant kernel approach for connectivity modeling produces the most accurate and informative spatial predictions of functional animal connectivity. We used the resistant kernel algorithm in this study based on these results.
Our approach develops resistance surfaces for jaguars and pumas based on published empirical species distribution models transformed into resistance surfaces and expert-based land use resistance for the two species. This approach allows additional evaluation of the important question of how different predictions are between expert-opinion and habitat-suitability-based landscape resistance models, which has been an ongoing issue of high interest in landscape ecology and conservation biology (e.g., Mateo-Sánchez et al., 2015a; Mateo-Sánchez et al., 2015b; Sartor et al., 2022). Additionally, we evaluated three different dispersal abilities to reflect uncertainty in the movement potential of the two species, which is also essential both theoretically and pragmatically, given the often-large effect of dispersal ability on predictions of connectivity (e.g., Cushman et al., 2013a; Ash et al., 2020). Further, there is extensive interest in how the functional response shape of dispersal kernels affects the predictions of connectivity modeling. However, few studies have formally compared predictions made with different dispersal kernel shapes. We sought to explore this by applying three transformations to the resulting connectivity surfaces to account for uncertainty about the shape of the dispersal kernel function modeling convex linear and concave dispersal relationship.
Notably, this is one of the first connectivity studies to use a large factorial combination of parameters in an ensemble modeling approach to evaluate the consistency of connectivity predictions across a large parameter space and produce an ensemble combination of predictions to mitigate uncertainty in the parameterization of the specific component models, and benefit from the central tendency among models across the parameter space. Ensemble modeling has been widely used for species distribution modeling and climate forecasting (Araújo and New, 2007; Stott and Forest, 2007), but to our knowledge this is the first multi-species, multi-model ensemble analysis of connectivity based on underlying empirical data related to species distribution and habitat suitability.
The core research question focused on quantifying spatial variation among connectivity models based on species, dispersal ability, dispersal kernel shape, and resistance surface modeling approach to identify areas consistently predicted as core areas, corridors, and barriers across Panama. We proposed several hypotheses, including (1) models developed for the same species will be more similar than models developed for different species, although differing in other parameters, (2) models developed with the same dispersal ability will be more similar than those developed from different dispersal abilities, (3) models developed with the same kernel shape transformation will be more similar than those developed with different shape transformation, (4) models developed from habitat suitability will be more similar to each other than to models developed from expert opinion.
A further objective was to combine our predicted connectivity maps to optimize the selection of areas for conservation-restoration. The variation and congruence of multiple connectivity predictions and seeking consensus across models to guide conservation is a topic of great recent interest in connectivity science (e.g., Cushman et al., 2013a; Schoen et al., 2022). Therefore, we sought to explore the feasibility and utility of developing multi-model connectivity ensembles by proposing that areas with a high mean conductivity value and low variation of predicted connectivity value are consensus areas of high connectivity importance. Conversely, areas with low mean connectivity value and low variation are areas of consensus as movement barriers or unsuitability for large carnivore movement. Areas with high variation among models are areas of uncertainty in which modeling parameters substantially impact connectivity predictions. Further research is warranted to refine the functional relationship between large carnivore movement and landscape features, particularly in these areas of high uncertainty among model predictions, where model outcomes can have significant impacts on development and land-use policy with consequences for biodiversity and its conservation.
2 Methods
The analysis involved six components. First, we developed a suite of resistance surfaces for jaguars and pumas based on a transformation of empirically derived habitat suitability models (Mateo-Sánchez et al., 2015a; Mateo-Sánchez et al., 2015b) and expert opinion-based resistance models (Macdonald et al., 1981). Second, we generated source points for analysis probabilistically in proportion to the relative suitability of each resistance model. Third, we computed three functional response surface transformations of the resistance surfaces. Fourth, we ran resistant kernel modeling on each resistance surface, source points, and surface transformation combination across three dispersal threshold distances. Fifth, we compared the differences in connectivity predictions across the models and identified the factors that most affect spatial patterns of connectivity predictions. Sixth, we combined connectivity predictions and computed their variation to identify areas that a consensus of models identified as essential core areas, linkages, and movement barriers. Below we provide a detailed methodology for each step of the analysis.
2.1 Resistance models
We developed eight base resistance models for this analysis (Table 1). These include three for each species based on published seasonal habitat selection in Panama (Craighead et al., 2022). Specifically, Craighead et al. (2022) used multi-scale optimization in machine learning (random forest) to predict habitat selection for wet and dry seasons and annually for jaguars and pumas based on camera trap data. This analysis found that the habitat use of these species was strongly affected by landscape composition and greatly varied by season. The analysis produced six predicted probability surfaces (three for each species). Following the recommendations of Mateo-Sanchez et al. (2015a, 2015b), we used a negative exponential transformation of habitat suitability to predict resistance, given that resistance to movement is often related to habitat suitability in a nonlinear way, in which moderate and highly suitable habitats have a low resistance to movement, while resistance increases sharply for the least suitable habitat conditions (See Sartor et al., 2022). We used the exponential transformation proposed by Wan et al. (2018):
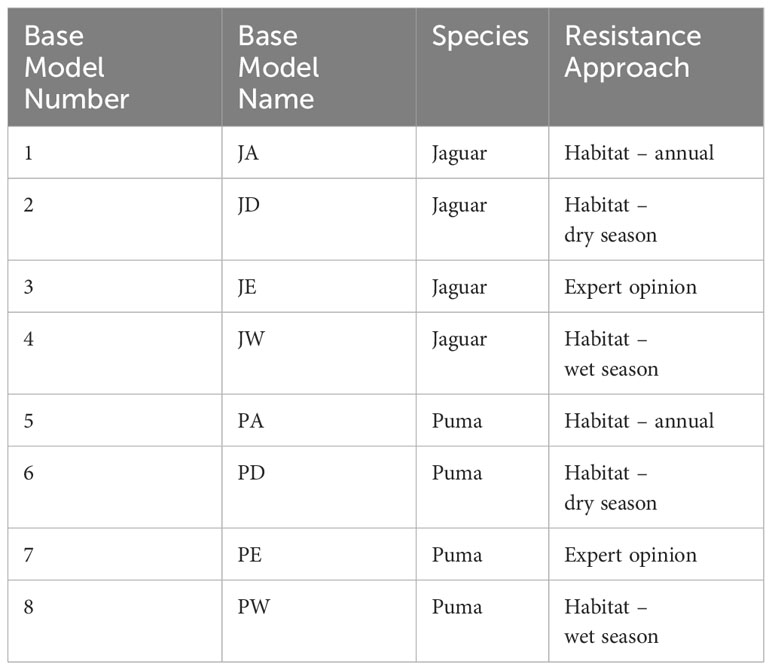
Table 1 Description of the eight base resistance models consisting of a combination of species and resistance modeling approach.
which transforms suitability into resistance using a negative exponential function and rescales it from 1 to 100. As the habitat suitability layers predict occurrence and not the effects of linear barriers on movement per se, we burned on the expected resistance effects of roads and water bodies (e.g., Zeller et al., 2021, Table 2).
We developed two additional resistance models based on the authors’ knowledge of the Panamanian landscape and the ecology of the two focal species – an approach capitalizing on field experience first fruitfully applied by Macdonald et al. (1981). We based this on reclassifying a current landcover map into resistance values (Table 3) and burning on expected effects of roads and waterbodies as movement barriers (Table 2).
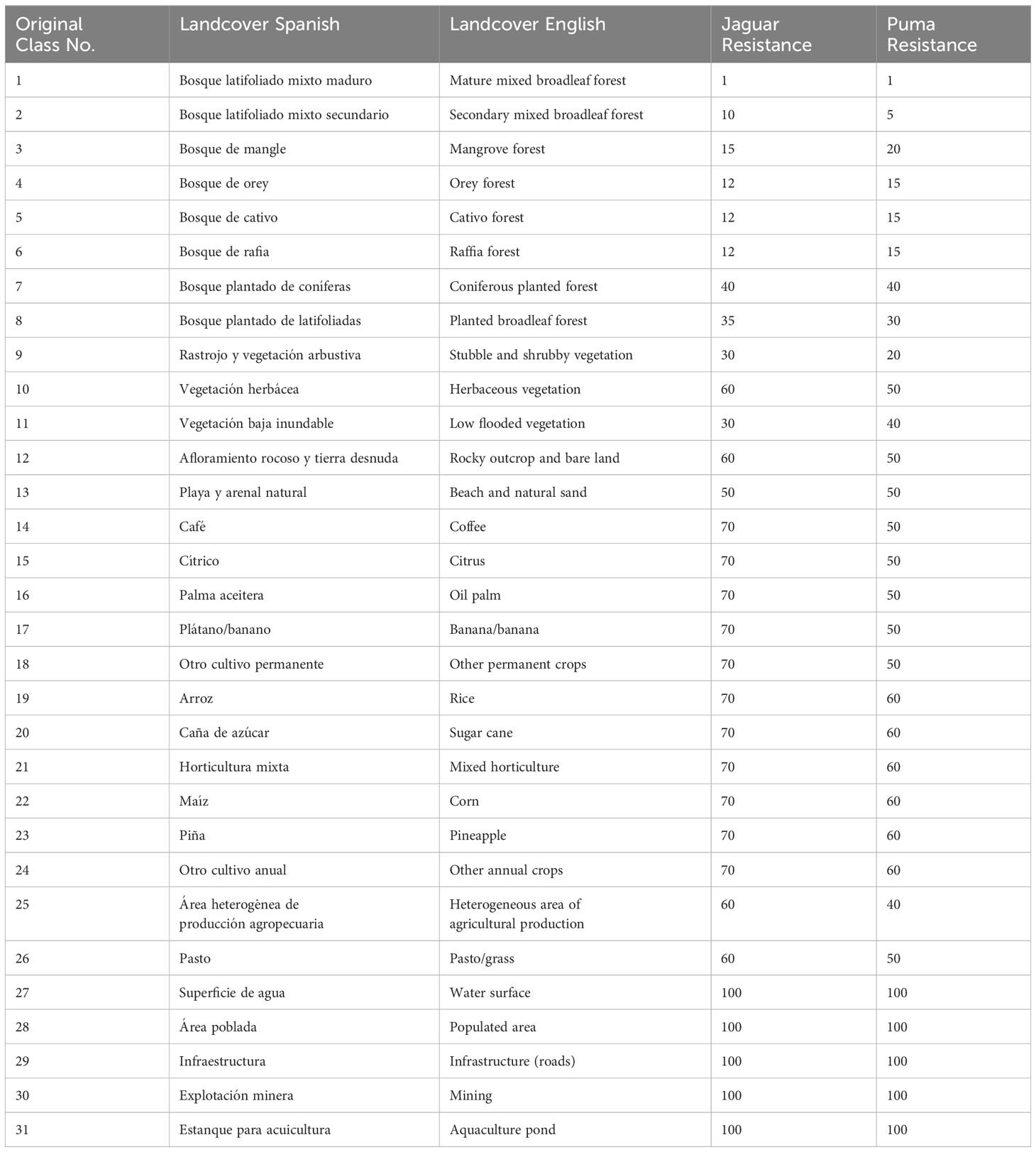
Table 3 Reclassification of the landcover map to resistance values for the jaguar and puma expert opinion resistance models.
We obtained the 2022 land cover map of Panama (Cobertura Boscosa) developed by the Ministry of Environment and elaborated with Sentinel 2-A satellite images at local scales of 1:25 000 (Table 3). We attained detailed hydrographic and roads network data from shapefiles downloaded from the Instituto Geográfico Nacional Tommy Guardia (2022) website.
We added the resistant effects of several categories of water features and roads to these eight base resistance models to incorporate the critical effects these features can have on landscape connectivity (Table 2). There is considerable uncertainty in the relative resistance and total resistance to movement related to different kinds of roads and water features. Therefore, we chose a single combination of resistance values. We did not explore how variation in these values affects the predictions given the focus of the study in comparing species (jaguar and puma) dispersal ability (200k, 400k, 600k), connectivity surface transformation (sqrt, linear, sq), and modeling approach (habitat vs expert). Given that the combination of these factors produced 75 different connectivity predictions in themselves, we felt it was infeasible to greatly expand the analysis by evaluating multiple levels of potential road and water resistance, given the factorial combinations of parameters become very large and the computational time required for spatially synoptic resistant kernel analysis is relatively high.
2.2 Source points
The density and distribution of source points can significantly impact the predictions of connectivity analysis, often to a greater extent than the influence of variation in resistance surfaces themselves (Cushman et al., 2013a; Ash et al., 2020). To simulate source points realistically and consistently for connectivity analysis, we used the probabilistic spatial sampling approach (e.g., Cushman et al., 2017; Chiaverini et al., 2022; Unnithan Kumar et al., 2021), which samples locations probabilistically with the chance of being selected as a source point equal to the value of the habitat suitability probability raster. For expert-opinion resistance models, which do not have a corresponding probability raster, an analogous raster was produced by inverting and rescaling the expert-opinion resistance layer between 0 and 1. We then applied the probabilistic sampling of source points to this transformed layer. For all eight sets of candidate source points thus produced (six based on habitat suitability and two based on expert opinion), we randomly selected 1,000 points for inclusion in the connectivity modeling to provide a consistent and comparable number between analyses and a tractable number for computation.
2.3 Resistant kernel modeling
We utilized the resistant kernel algorithm to model landscape connectivity (Compton et al., 2007). The resistant kernel model simulates organism movement from a selection of source points across a resistance landscape. The cumulative resistant kernel surface – the sum of the individual dispersal kernels from each source point – reflects the expected value of the incidence function of movement density across the landscape, in each pixel, as a function of the density and distribution of source points, the resistance surface, as well as the dispersal ability used for the analysis (e.g., Cushman et al., 2013a; Cushman et al., 2016). This approach has several advantages, including that it produces spatially synoptic predictions of movement rate and pattern in all locations of the landscape (Cushman et al., 2013a; Cushman et al., 2014), is relatively computationally efficient, and, in particular, because recent simulation studies (e.g., Unnithan Kumar and Cushman 2022; Lumia et al., in press) have found that resistant kernel analyses produce predicted connectivity surfaces that more accurately reflect the actual movement patterns of organisms across a broad range of movement parameters than several other widely used connectivity modeling algorithms.
We utilized the UNICOR (Landguth et al., 2012) software to implement resistant kernel modeling. We implemented resistant kernel modeling in UNICOR by selecting the parameter “all_paths” for “Edge Type”. We ran resistant kernels with a linear shape parameter which produces conical kernel densities surrounding each source point. We implemented three different dispersal distance thresholds, given the often-dominant importance of dispersal ability in influencing patterns of functional connectivity across landscapes (e.g., Cushman et al., 2010; Cushman et al., 2013a; Ash et al., 2020), as well as the uncertainty of the functional dispersal abilities of jaguars and pumas. To explore the sensitivity of the results to varying dispersal ability, we evaluated three different kernel radii, corresponding to 200,000, 400,000, and 600,000 cost units. The units reflect maximum dispersal abilities of 200, 400, and 600 km in ideal habitats (resistance value of 1) and distances of 2, 4, and 6 km through highly inhospitable conditions (high resistance values of 100).
Additionally, there is a need for a more formal evaluation of how the shape of the resistant kernel function affects predictions of functional connectivity. Recent simulations (e.g., Unnithan Kumar et al., 2022) found that the simulated incidence function produced by an agent-based simulation model (Unnithan Kumar et al., 2022) produced kernel densities that were highly nonlinear with high kurtosis corresponding to a power function. To explore the sensitivity of the predictions to different power function transformations of the functional kernel density surfaces, we applied three transformations to the cumulative resistant kernel surfaces produced from each combination of the other parameters (species-model x dispersal ability). These include the square, square root, and first power (no transformation). We then rescaled the output between the minimum and maximum of the untransformed connectivity surface for each of these transformations to produce models with comparable ranges differing only in functional shape. The combination of these parameters (species-model, with eight levels; dispersal ability, with three levels; surface transformation, with three levels) produced 72 connectivity surfaces (Table 4).

Table 4 Description of the 72 connectivity surfaces produced by the combination of species, model type, dispersal ability and surface transformation.
2.4 Comparison of predictions
One of the important areas of uncertainty in connectivity science is the comparability and relative performance of connectivity predictions from different algorithms and parameterizations. We implemented a series of four analyses to compare the variation in predictions across our 72 models and describe the factors that had the most substantial influence on them. First, we computed the pixel-wise correlation matrix among the 72-connectivity rasters. This matrix produces the Pearson correlation coefficient between each pair of connectivity models based on the values at each pixel in the raster map.
Second, we used this correlation as the basis for three additional analyses. First, we used polythetic agglomerative hierarchical clustering (McGarigal et al., 2000) in R, using the hclust function with Ward.D2 fusion method. We then evaluated the cluster solution to see the major structure of grouping among the 72 models and any substructure within those groups. Second, we used non-metric multidimensional scaling ordination (McGarigal et al., 2000), implemented in the vegan package in R to explore the pattern of relationship among the 72 connectivity models in an ordination framework as a comparison to the hierarchical framework provided by the clustering (as recommended by McGarigal et al., 2000). We then plotted the species-model groups on this ordination diagram to visualize the significant patterns of difference among connectivity models.
Third, we explored the four a priori hypotheses described in the Introduction using Mantel testing with model matrices (Legendre and Legendre, 1998). Mantel testing is a distance-based correlation well suited to testing hypotheses of multivariate differences. In this context, we tested whether the difference among predictions (as measured by the correlation among connectivity rasters) is significantly related to if the models are from (1) the same species, (2) the same modeling approach (habitat vs. expert opinion), (3) same dispersal ability, (4) same surface transformation, and the combination of these factors. To accomplish this, we converted each of the hypotheses into a model matrix that presents the levels of the factors in the hypothesis as 0 (reflecting match in parameters) or 1 (reflecting mismatch). The Mantel test between the correlation matrix and the model matrix is analogous to a multivariate test of differences in distance between the factors described in the hypothesis (e.g., Legendre and Legendre, 1998; Lumia et al., in press). We conducted Mantel tests in the Ecodist package in r.
2.5 Consensus of predictions and spatial prioritization
We evaluated the consensus and variation in connectivity predictions by computing the mean and coefficient of variation rasters from the stack of 72 predicted connectivity rasters. The mean raster shows the expected value of connectivity across the complete ensemble of 72 connectivity predictions. In contrast, the coefficient of variation shows the relative variation among the connectivity predictions across Panama. We evaluated the pattern of the coefficient of variation to assess the congruence of predictions and areas where agreement is highest and lowest across the 72 connectivity predictions.
We then identified core areas, barriers, and potential corridors for jaguar and puma movement across Panama based on the mean and coefficient of variation surfaces (e.g., Cushman et al., 2018; Kaszta et al., 2020). We identified important core areas as unbroken extents of continuously high kernel value. We identified barriers as areas of continuous low kernel value between identified core areas. Finally, potential corridors were identified based on relatively high kernel value in the regions between identified core areas that could provide functional routes for dispersing jaguars and pumas across potential barriers to connectivity.
3 Results
3.1 Relationships among 72 alternative resistance models
The main structure of the correlation matrix, as revealed by the hierarchical agglomerative clustering, is grouped based primarily on species, season, and expert-vs-empirical modeling approach (Figure 1). The first cluster combines landcover (expert opinion) based models from both species (jaguar and puma) clustered across all dispersal levels and transformations. The second cluster comprises all puma wet season empirical models across all scales and transformations. The third cluster is all jaguar annual empirical models. The fourth consists of all jaguar wet season empirical models, while the fifth is all jaguar dry season empirical models. Finally, the sixth combines the puma dry season and puma annual models. The variation in connectivity models was quite similar across clusters, suggesting high homoscedasticity in magnitude of variation among clusters. Overall, the clustering shows a dominant effect of a combination of species and model type (landcover-based expert vs. seasonal empirical models) in affecting connectivity predictions, with a much lower influence of dispersal ability or kernel surface transformation. This suggests that the largest driver of differences in connectivity predictions in this analysis is linked to which species and which modeling approach (empirical habitat vs expert opinion) is used for the prediction.
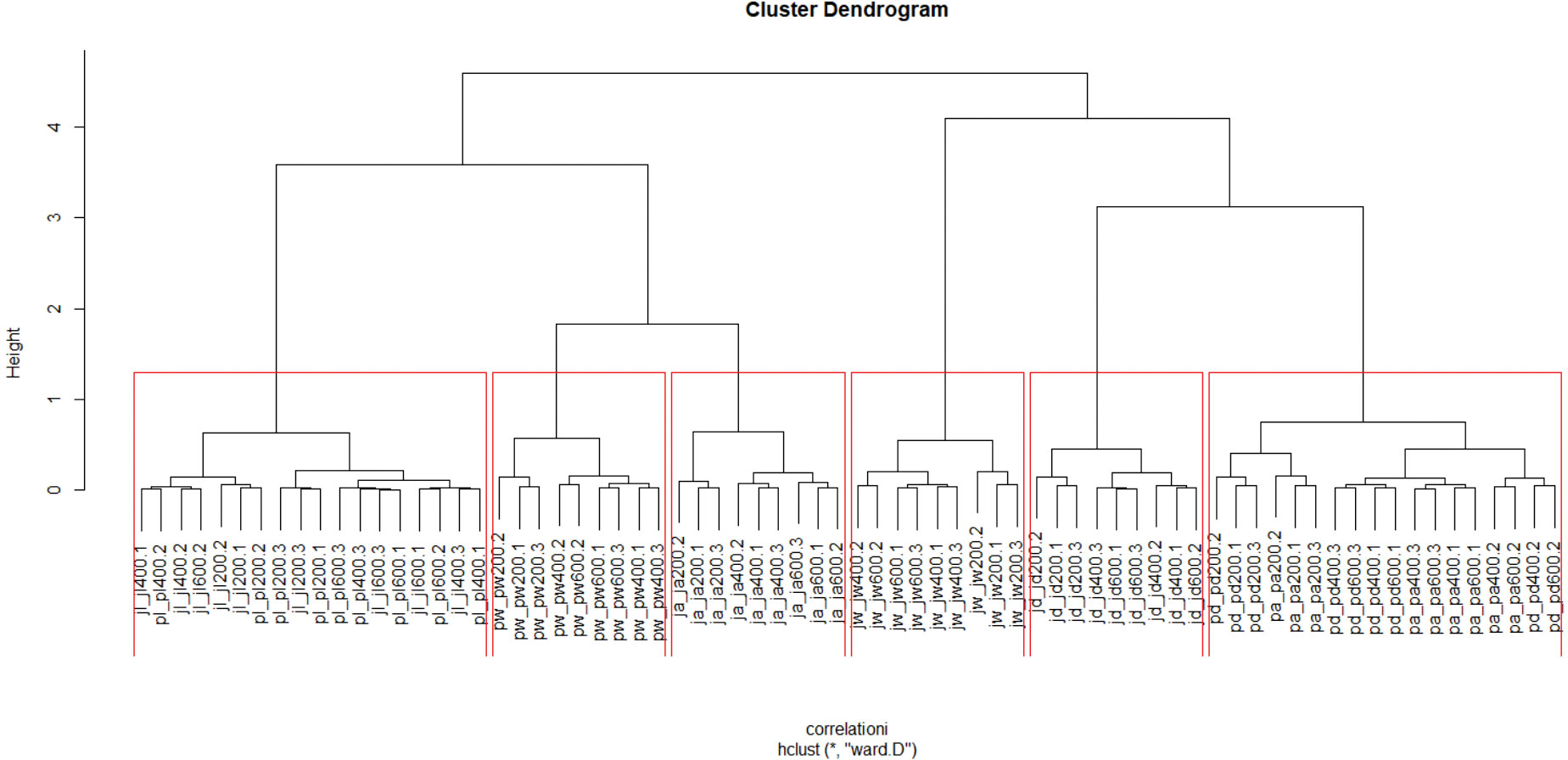
Figure 1 Hierarchical agglomerative clusters. The red boxes indicate the six main groups of structure that are interpreted in this paper.
There is also some additional substructure among clusters related to dispersal ability and surface transformation. Cluster 1 shows a strong intermixing of jaguar and puma expert opinion landcover models, as well as intermixing of the transformation functions of the connectivity surface. This cluster shows that the expert opinion model for jaguar and puma resistance are similar in terms of their predicted connectivity and that convex, linear, and concave transformations of the connectivity surfaces for the jaguar and puma landcover-based expert-opinion resistance models do not have strong effects differentiating their resistant kernel predictions. There is a more robust substructure within Cluster 1 based on dispersal distance, with the 200k dispersal predictions clustered together and the 400k and 600k dispersal distance predictions intermixed. This substructure suggests a threshold effect as a function of dispersal distance where predictions of 200k dispersal distance differ substantially from those of either 400k or 600k.
Cluster 2 shows a more robust substructure based on dispersal distance and surface transformation, again with the 200k results clustering together, and the 600k and 400k results largely clustering together in sub-association with levels of surface transformation. Again, this shows a substantial effect of dispersal distance, with 200k different than 400k and 600k, and an effect of surface transformation in discriminating connectivity predictions of the puma wet season model. Cluster 3 shows a strong substructure in which dispersal ability is discriminated in three distinct subclusters (200k, 400k, and 600k for the jaguar annual resistance model predictions), with little differentiation based on surface transformation. Cluster 4 is similar to Cluster 2, with predictions based on the jaguar wet season resistance model differentiated mainly by dispersal distance, with the 200k predictions clustered separately from the 400k and 600k predictions and little clear pattern concerning surface transformation. Cluster 5 is like Cluster 4 in that the connectivity predictions for the jaguar dry season model are discriminated based on dispersal ability, with 200k predictions clustered distinctly from those at 400k and 600k. Finally, Cluster 6 groups predictions of connectivity based on the puma dry and puma annual models, with again the main substructure related to dispersal threshold, with predictions from the 200k dispersal distance clustered together for both the annual and dry season puma models and no other clear structure discriminating predictions based on surface transformation.
The Mantel tests confirmed this pattern through distance-based analysis of model matrices (Figure 2). Figure 2 presents the Mantel testing of a priori hypotheses, ordered from highest to lowest Mantel correlation. The combination of species and model type (season and habitat vs. landcover) had a Mantel correlation of approximately -0.5, more than twice the magnitude of the subsequent strongest correlation (for the species group). Nearly all Mantel tests were significant, with main effects species, scale, and transform significantly related to similarity in connectivity predictions, with species having more than twice as large a prominent effect as the others. The results confirm that species and model type, in combination, are the dominant factors in differences among connectivity predictions.
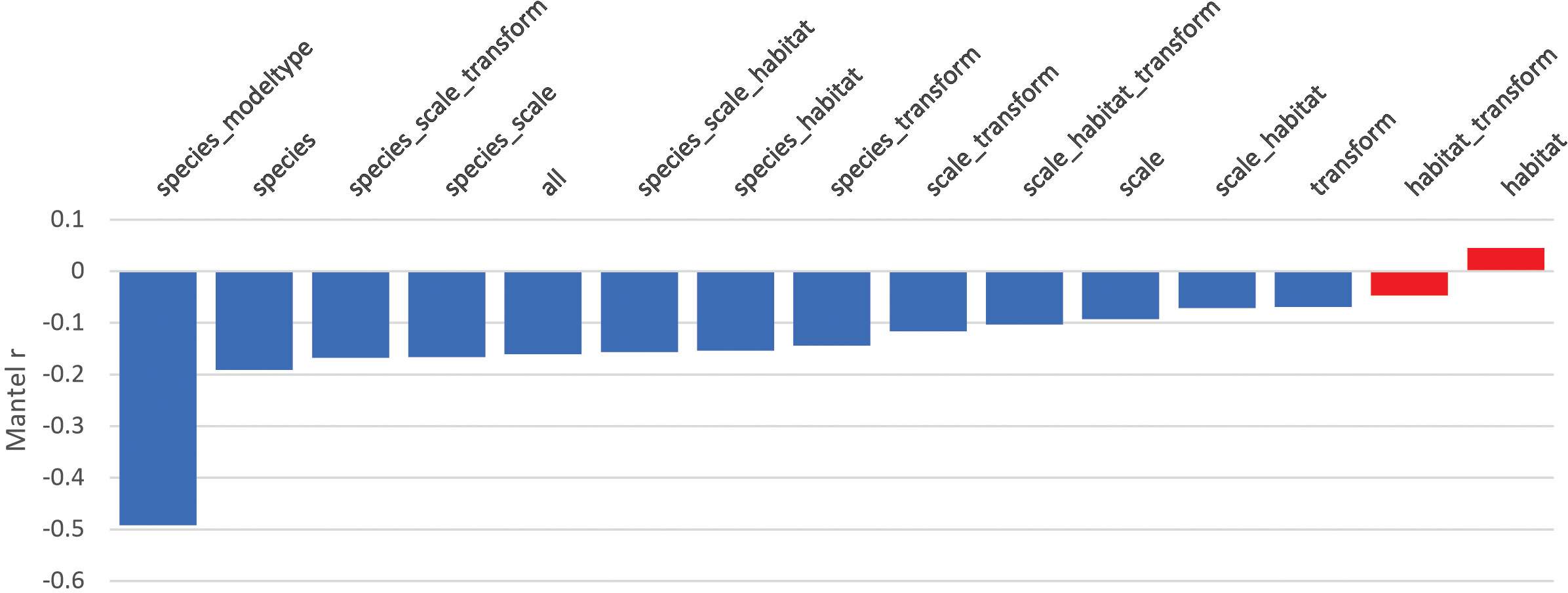
Figure 2 Mantel test on model matrices. Statistically significant tests are shown in blue and non-significant in red.
A multidimensional scaling plot confirms the same overall relationship. Figure 3 shows the first two dimensions of the NMDS, and Figure S1 provides a video of the first three dimensions, showing strong separation among the eight main species-model type categories. The groups most distinct in the first two dimensions of the NMDS are group 4 (jaguar wet season), which is substantially displaced from the other predictions to the bottom of axis two, and model 1 (Jaguar annual), which is displaced to the left of axis 1. Group 8 (puma wet season) is also relatively displaced from the other groups to the top of axis two. The remaining species-season predictions overlap in the NMDS space defined by the first two axes but show more separation in the three-axis space shown in Figure S1.
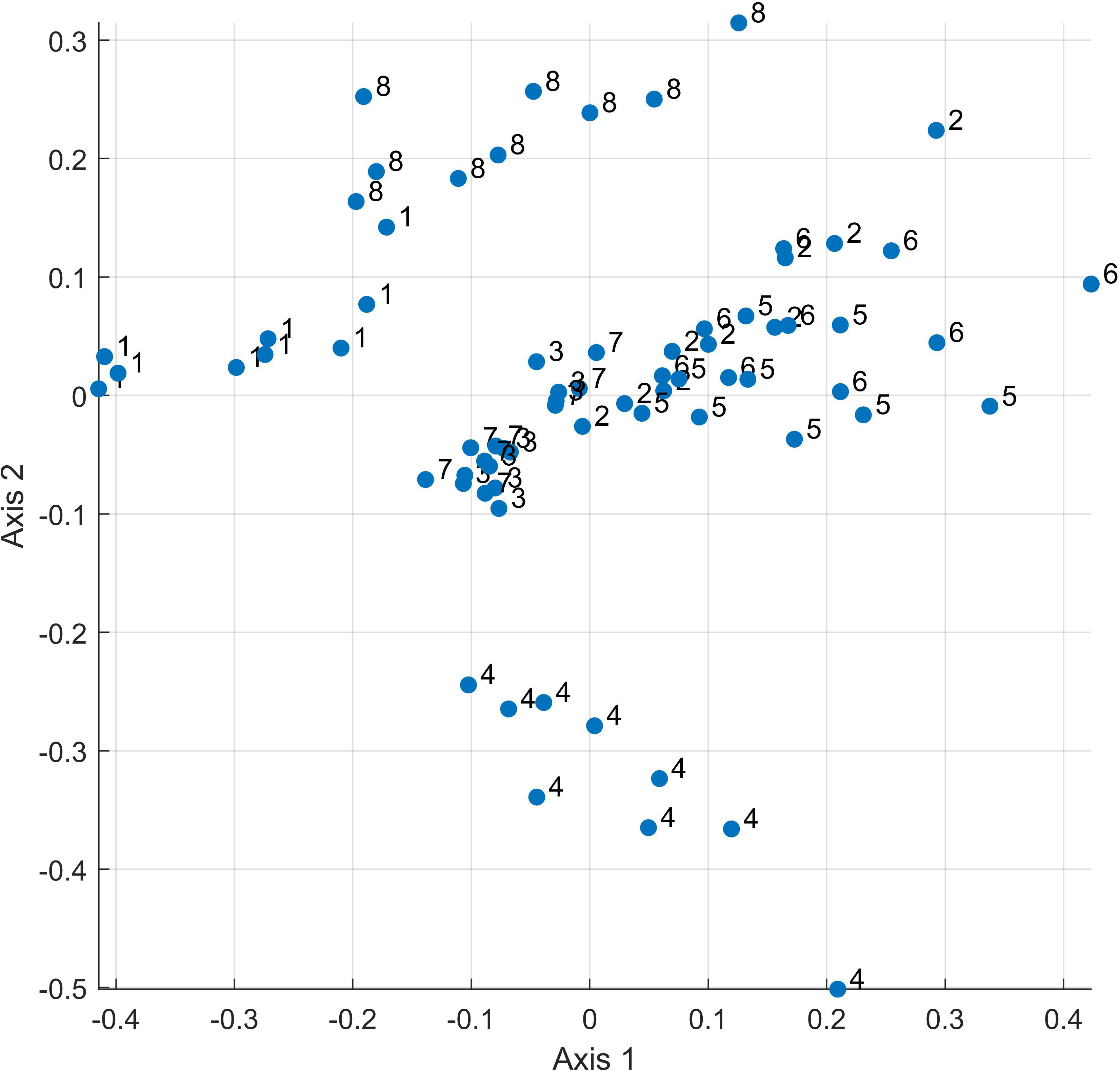
Figure 3 NMDS plot of the 72 connectivity surfaces, labeled by Species x Model-Type group. 1 – Jaguar, Annual Habitat, 2) Jaguar, Dry Season Habitat, 3) Jaguar, Landcover Expert, 4) Jaguar, Wet Season Habitat, 5) Puma, Annual Habitat, 6) Puma, Dry Season Habitat, 7) Puma, Landcover Expert, 8) Puma, Wet Season Habitat.
3.2 Prioritization based on mean and variation of the 72 predicted connectivity surfaces
Figure 4 shows the mean predicted connectivity surface across Panama. The surface shows two main core areas corresponding to the central mountain range which runs the length of the country near the Caribbean coast, with one core area to the west extending to the Costa Rican border, and another stronger core area to the east extending to the Colombian border. A quantitative assessment of the strength of these core areas shows that the eastern core is much stronger than the western, based both on area (173,497 km2 vs 55,801 km2) and sum of kernel values (indicating total predicted movement density (166,360 vs 43,307). The mean connectivity surface also shows that most of the southern part of Panama and a major breakage transverse to the axis of the country in the region of the Canal Zone is predicted to have very low functional connectivity. Importantly, the Canal Zone and approximately 100 km to the west of it is predicted to have low connectivity and may represent a functional barrier to the movement of jaguars and pumas, and thus the area where Pan-American connectivity of these species is severed. Figure S2 shows a 3-dimensional rotating plot of the predicted connectivity across the ensemble connectivity model for the entire nation and Figure S3 shows a similar plot for the Canal Zone proper.
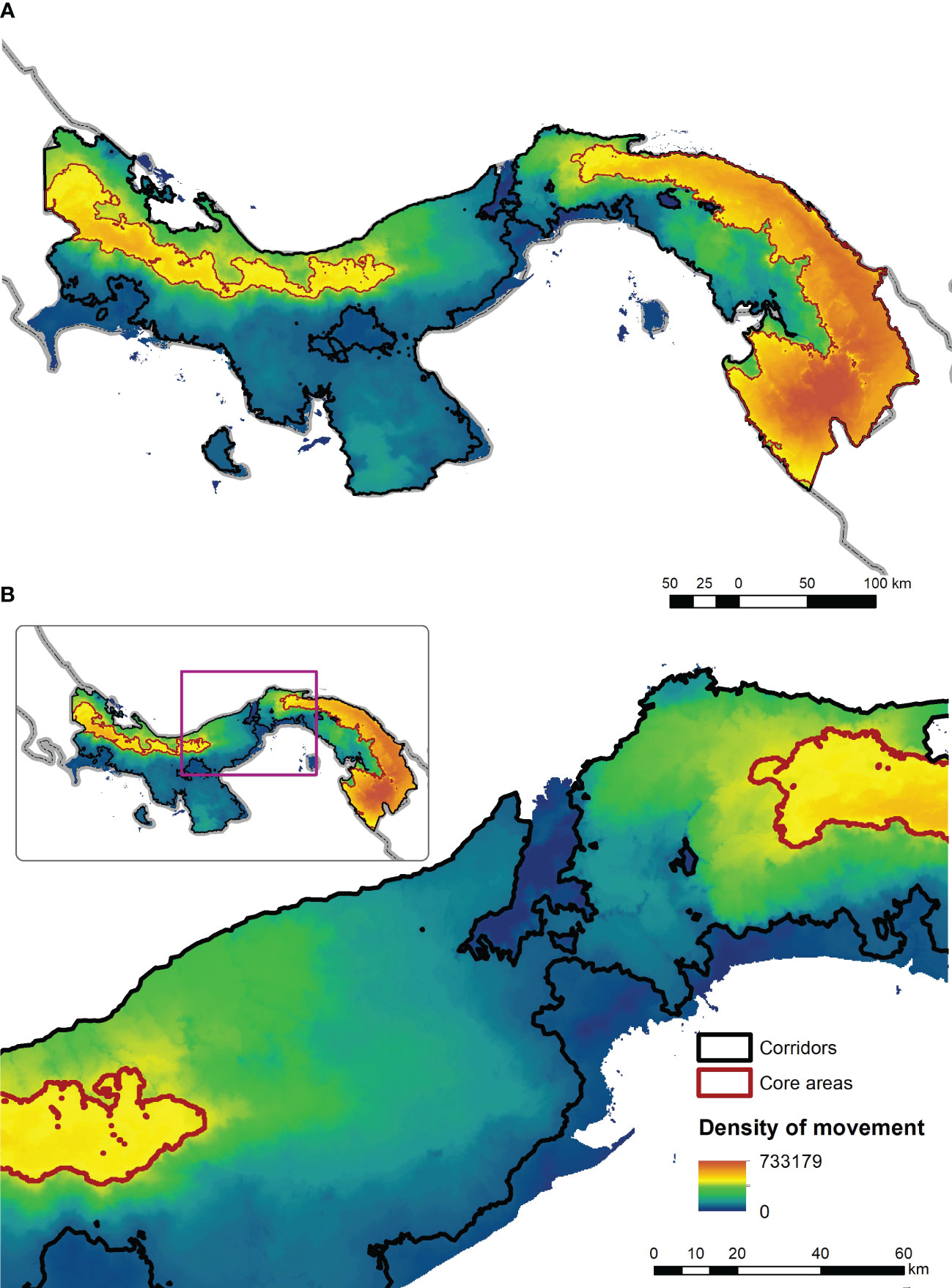
Figure 4 Plot of the ensemble mean value across the 72 resistant kernel connectivity surfaces for the full extent of Panama (A) and the Canal Zone (B). In this figure connectivity value is shown in a gradient from blue (low) to red (high) with a linear min to max stretch. The predicted core areas are shown in red outlined polygons and the predicted corridor linkage (at 10th percentile of the cumulative kernel surface) is shown in black outline.
The coefficient of variation surface of variation across the 72 connectivity surfaces shows a generally low variation (as a proportion of the mean) in the two areas predicted to be connectivity cores (Figure 5). However, the highest predicted variation is in the Canal Zone, suggesting that this critical area of a potential movement barrier is also an area where the models have a relatively low agreement. The predictions suggest that focused research on the movement of jaguars and pumas through the Canal Zone with telemetry or landscape genetics is warranted to evaluate functional connectivity through this critical area.
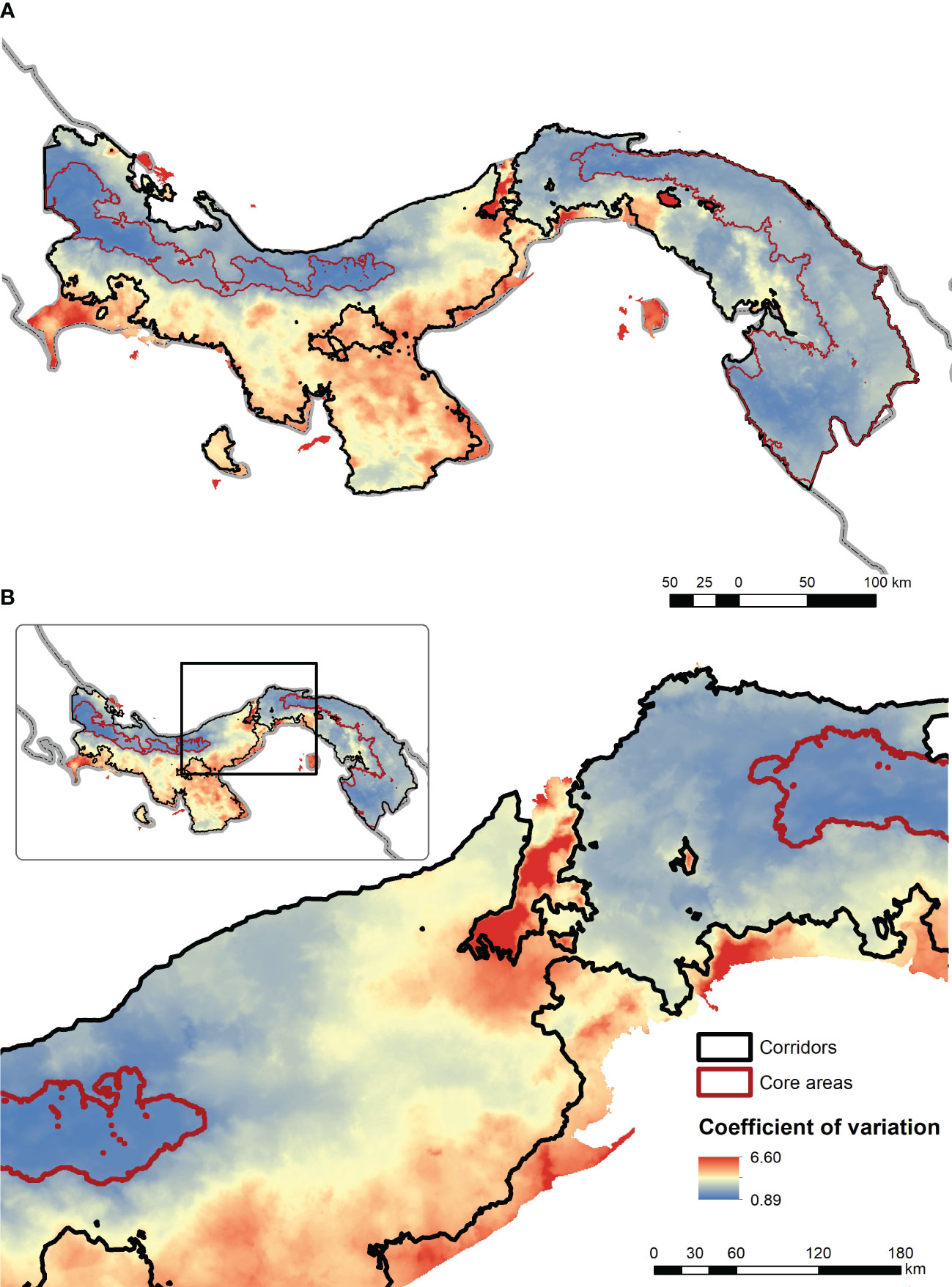
Figure 5 Coefficient of variation among the 72 resistant kernel connectivity predictions included in the ensemble connectivity model across the full extent of Panama (A) and the Canal Zone (B). In this figure the coefficient of variation is shown in a gradient from blue (low) to red (high) with a linear min to max stretch. The predicted core areas are shown in red outlined polygons and the predicted corridor linkage (at 10th percentile of the cumulative kernel surface) is shown in black outline.
Given the potentially severed Pan-American connectivity for large carnivores in the Canal Zone, we investigated potential corridor linkages across this region (Figure 6). Specifically, by overlaying the ensemble-resistant kernel surface on base maps showing settlements, roads, and other infrastructure, we identified several critical potential breakages in connectivity and three potential routes of highly attenuated but potential connectivity across the Canal Zone. Specifically, connectivity appears broken by the canal, Chagres River, Lake Gatun, and associated development along the major highways that parallel the canal on its eastern flank.
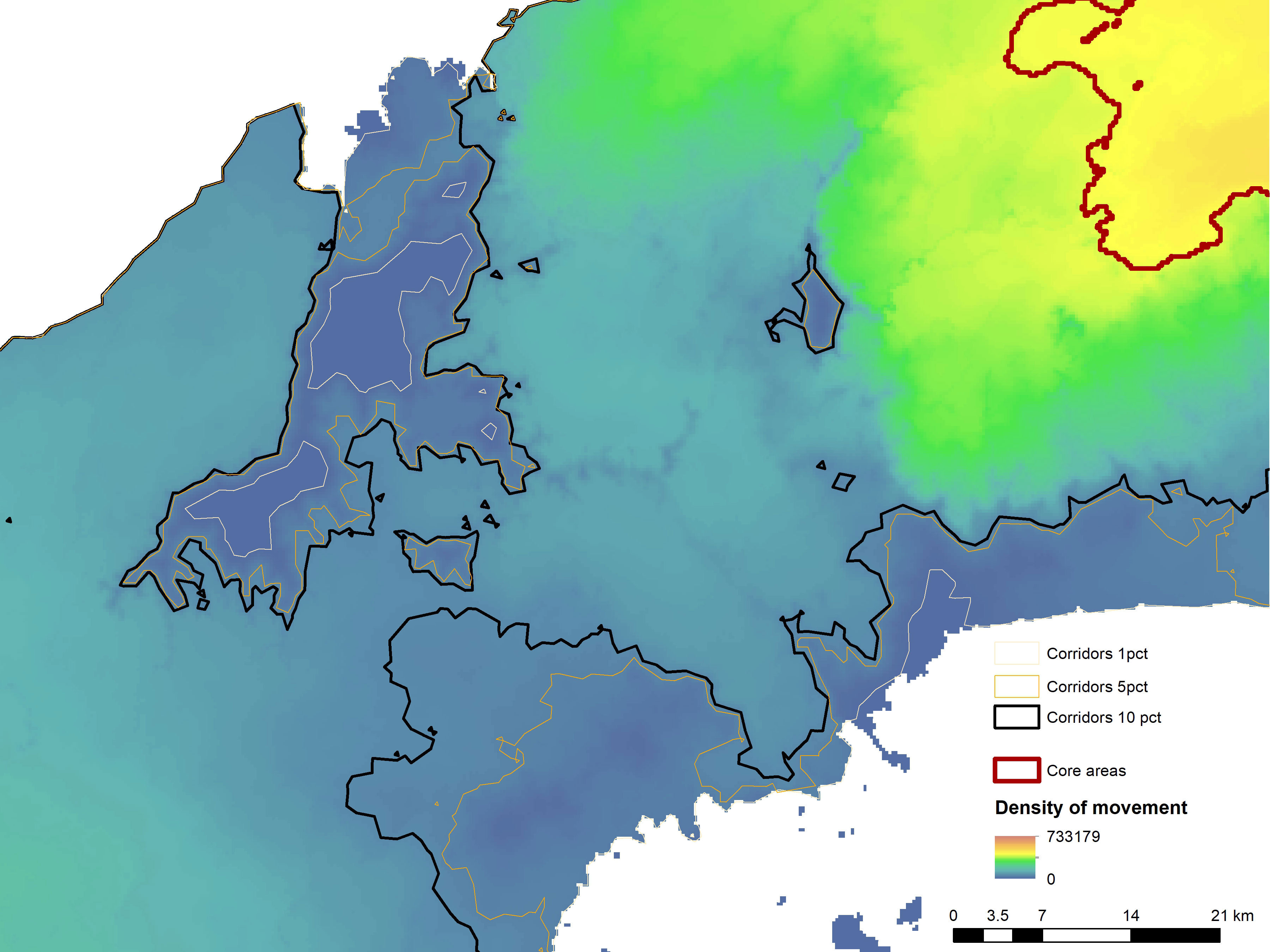
Figure 6 Identification of three potential linkage corridors across the Canal Zone shown using contours indicating different threshold dependent connectivity patterns (black – connected at the 10th percentile; orange – connected at the 5th percentile; white – connected at the 1st percentile from maximum).
A quantitative thresholding of predicted kernel density enabled us to evaluate the location and strength of potential corridor routes across the Canal Zone. This identified three potential connectivity routes. First, heading from east to west, (1) a crossing of the Chagres River (~500 m water crossing) from Isla Darien to the forested peninsula on the southern shore and then crossing ~150 km of heavily disturbed agricultural land to connect with the western core area west of Los Aguas. This is a relatively wide potential corridor (5-10 km) that is connected at a threshold of the 10th percentile (e.g., 90% of the land area of Panama has greater movement density than this corridor in the ensemble model). This shows that the strongest potential linkage across the Canal Zone is quite tenuous and weak, with a low density of predicted movement, and is located along the southern shore of Lake Gatun.
The second potential corridor identified (2) crosses the canal near the city of Gatun (~0.6 km water crossing) north of Farmazona, hugging the northern shore of Lake Gatun (along San Lorenzo Protected Area) to connect the western core area near Las Minas. This potential corridor is severely limited by the disturbance in the locks area of the canal and the nearby city of Gatun, and more so if the proposed Quebrada Ancha y María Chiquita highway is constructed, which passes a western portion of Chagres National Park (Centro de Incidencia Ambiental – Panama (CIAM), 2022). It is connected at the 5th percentile (meaning it is half as strong as the first potential corridor, which was connected at the 10th percentile).
A third (3) potential steppingstone corridor crosses Lake Gatun via Barro Colorado Island (~1 km water crossing) near Isla Buena Vista, and from the island to the mainland near Isla Maiz (~0.25 km water crossing), thence connecting to the central western core area west of Los Aguas through a ~150 km route with heavily developed agricultural land. This corridor has very low predicted movement density (connected at the 1st percentile, meaning 99% of the land area of Panama has higher predicted movement density). The two southern routes are exposed to long traverses through highly impacted agricultural landscapes, which the ensemble model predicts have low connectivity potential. All three routes have relatively long water crossings, some with multiple crossings (northern and central).
4 Discussion
This paper illuminates several issues of both theoretical and applied conservation interest. From a theoretical perspective, this is one of the first studies to apply a multi-species, multi-model, ensemble framework to predict connectivity at a national level and compare the factors that drive differences in the predictions. Specifically, we evaluated 72 different connectivity models, consisting of a factorial of species, model type (seasonal habitat, annual habitat, landcover expert), dispersal ability, and nonlinearity of the connectivity function. This combination provides a novel analysis to assess the relative influence of these factors on connectivity predictions. It also produces a consensus prediction from the ensemble of all predictions to account for uncertainty and variability in predictions.
Our results indicate that there are significant differences in predicted connectivity related to the combination of species (jaguar vs. puma) and model type (seasonal empirically based habitat vs. expert-opinion-based resistance surface) and a lesser but substantial effect of dispersal ability (with 200,000 cost unit predictions consistently different than those from 400,000 and 600,000 cost unit dispersal ability, which generally cluster together). The results show a large effect of species differences, resistance model type, and their interactions. The clustering, Mantel tests, and NMDS analysis all demonstrate the strong patterns of differences in connectivity predictions between species-model type predictions, with all connectivity models (across dispersal abilities and surface transformations) clustered together for each species-model type group. This clustering shows that predictions of connectivity are sensitive to differences in source points and resistance surfaces (as seen by Cushman et al., 2013a; Unnithan Kumar et al., 2022; Lumia et al., in press), in this case as a function of differences in species and approach used to estimate the resistance surface and source point distribution.
In contrast, the low variability (as measured by the coefficient of variation) in predictions across the 72 connectivity models overall shows that despite these differences, the interpretation of connectivity across two large carnivore species is highly consistent, with a strong consensus in predictions of core areas, barriers, and potential linkage zones or corridors between the core areas. It is encouraging that even across two different focal species, various dispersal abilities, different approaches to estimating landscape resistance and source point distribution, and nonlinearity of the connectivity kernel, the predictions were highly concordant across the 72 models. This strong congruence in results provides high confidence in the general predictions of the location and strength of core areas, corridors, and barriers and provides a convincing applied conservation message. This strong consensus is the justification for using the ensemble model, calculated as the mean across the 72-connectivity predictions, as the basis of our connectivity prioritization.
Specifically, our results show that the mountainous region of Cordillera San Blas, which runs from east of the Canal Zone to the Colombian border through the Guna Yala Indigenous territory and the Darien region, is the most robust core area for both species with by far the highest predicted movement density and continuity of predicted movements. Second, we identified an analogous but weaker core area west of the Canal Zone following the Cordillera Central Mountain range from El Valle to the Talamanca Mountains in Costa Rica. This also had high predicted movement rates throughout and strong internal connectivity. Third, we found that the lowlands along the Pacific coast along the southern half of Panama (including the Azuero Peninsula–Cerro Hoya National Park) were all predicted to provide very low connectivity, no core areas, and limited linkage potential given extensive human development and land conversion in this region. Fourth, our results indicate that the Canal Zone and Lake Gatun are potentially substantial barriers to large carnivore movement across Panama, and by extension, through the Pan-American region, given the limiting corridor represented by the Panamanian Isthmus. Given that jaguars and pumas are likely more mobile, with larger dispersal ability, and potentially greater water-crossing ability than most other species, these predictions are likely conservative for a wide range of species for which the canal and associated developments are likely complete barriers. Therefore, connectivity for other focal species, such as Baird’s tapir (Tapirus bairdii) and margay (Leopardus wiedii), for example, is likely correlated in pattern but more restricted due to lower dispersal abilities.
There are several implications of these results. Following recent work by Macdonald et al. (submitted) and the habitat area hypothesis (Fahrig, 2013), we emphasize the significant importance of maintaining the two identified major core areas for large carnivore habitat and connectivity. Population dynamics (Macdonald et al., in press2), genetic diversity (Macdonald et al., in press), and genetic differentiation (Cushman et al., 2013c) are more strongly influenced by the quality and extensiveness of habitat than its pattern and connectivity. For instance, Macdonald et al. (in press) found that simulations of population size for clouded leopard (Neofelis nebulosa) across Borneo were dominated by the extent of habitat protected, with effects of establishing corridors among patches approximately an order of magnitude smaller than those of habitat area. Macdonald et al. (in press) also found similar dominance of habitat area in predictions of clouded leopard genetic diversity across the same 28 conservation design scenarios in Borneo. Cushman et al. (2014) simulated 72 different combinations of habitat area and configuration using neutral landscape models. They found that habitat area and configuration affected the strength of predicted genetic differentiation. However, the extensiveness of habitat, as measured by several landscape metrics, had a disproportionately high effect on predicted genetic differentiation. Therefore, the conservation significance of this study includes the presence of two large carnivore core areas that, respectively, span the eastern and western halves of Panama along the two mountain ranges; and that wide-ranging carnivore conservation initiatives should prioritize these areas to maintain extensive and well-connected populations across the length of the country. These core areas are also the anchors and support for any potential connectivity across the Canal Zone. Therefore, maintaining the core areas is likely the most crucial consideration to retain any connectivity across the barrier region represented by the Canal Zone.
The analysis identified three potential linkages of uncertain and likely limited functionality across the Panama Canal watershed and Canal Zone. Probably, none of these provides extensive functional linkage for the two wide-ranging carnivores. As such, the Canal Zone and Lake Gatun are man-made dispersal barriers that potentially limit wildlife movement across Panama and the Pan-American region. Two potential corridors skirt Lake Gatun’s edges, one to the north and one to the south of the lake. Both cross the canal and several major highways (i.e., Trans-Isthmian Highway) and must traverse areas of high human development and urban sprawl. The potential corridor to the south of the lake has more than twice the functional potential as the one to the north, and more than 10 times the steppingstone corridor across Barro Colorado Island. This corridor runs through a region of less intensive human development and more space, while that in the north is through a bottleneck between the Caribbean Sea, the locks zone, adjacent urban areas, and the lake. The third potential corridor crosses the lake through the steppingstone island of Barro Colorado (BCI), from Chagres National Park to the west, where numerous villages, land conversion, and hunting pressure are evident. Following to the west is Parque Nacional Soberania (~22,000 ha), which sits astride the canal and is characterized by secondary-growth forest in various stages of regeneration and patches of old-grow forest. Our analysis indicates that this third corridor is very weak and tenuous, with a strength less than 1/5th that of the northern corridor and less than 1/10th that of the southern potential corridor. BCI consists of 15.6 km2 of semideciduous lowland moist tropical forest in Gatun Lake. It exhibits rich mammal fauna due to its protected status since 1960, but jaguars and pumas are not permanent residents (Swinkels et al., 2023).
4.1 Scope and limitations
This study has revealed jaguar and puma core areas and linkages within the Isthmus of Panama, where the canal plays a significant ecological role in disrupting species’ dispersal in the Americas. Coupling the novel ensemble connectivity modeling framework used here with jaguar and puma habitat selection models (Craighead et al., 2022) informs the magnitude of impact on species dispersal. However, as Cushman et al. (2013b) pointed out, the validation of predicted connectivity models with movement-derived data could predict specific movement decisions made by individual animals more effectively to aid in the protection of potential corridors for conservation. Several studies have found that movement data are often superior as predictors of actual animal movement as compared with habitat suitability or occupancy models (e.g., Cushman et al., 2014; Zeller et al., 2018) and that predictions are highly sensitive to movement state (Zeller et al., 2014) and demographic characteristics of the moving animals (Elliot et al., 2014). The 72 models we investigated included a broad range of human impacts, but we acknowledge that future research should evaluate a broader range of resistance hypotheses and, most importantly, validate and optimize them in relation to empirical movement and genetic data. This is particularly true in the locations of the predicted potential corridors through the Canal Zone, which are areas of high relative variability among the 72 models, indicating uncertainty in connectivity strength through these areas. We strongly advocate for future research to study the differences in functional movement abilities through the Canal Zone of male, female, and dispersing juvenile (e.g., Elliot et al., 2014) jaguars and pumas. A constraint on our study was the shortage of GPS telemetry on jaguars and pumas near the canal (i.e., from the eastern core area); acquiring such data to characterize accurately the movements of both species should be a priority. Validating our findings will be of great conservation value for the two felid species, and for other terrestrial species with similar ecological characteristics. It will also be valuable to explore other reliable approaches for establishing linkages to the two core areas, such as landscape genetics (Cushman et al., 2006; Wasserman et al., 2010; Mateo-Sanchez et al., 2015a; Mateo-Sanchez et al., 2015b; Atzeni et al., 2023). We encourage future research to determine whether the Canal Zone is permeable to jaguar and puma gene flow and what resistance patterns are estimated based on genetic data in comparison to habitat use and telemetry (e.g., Shirk et al., 2010; Wasserman et al., 2010; Mateo-Sanchez et al., 2015a; Mateo-Sanchez et al., 2015b; Sartor et al., 2022).
It is important to point out that this ecoregion might be particularly vulnerable to the increased climate variability now anticipated as a consequence of climate change. Hence, the knowledge provided here has implications for conservation practice and policy and should prompt biodiversity conservation actions by government institutions to, first, implement specific management strategies (i.e., restoration, protection, or combination of both) of the 2,500 km2 Panama Canal watershed; second, maintain the extent, strength, and integrity of the core areas highlighted in this paper; and third, optimize the linkages between the core areas indicated in our models.
5 Conclusion
Our analysis maps and assesses the functional connectivity (i.e., core areas, barriers, and potential corridors) for large, wide-ranging carnivores using a unique ensemble approach across a combination of models combining two species, two resistance modeling approaches, several dispersal abilities, and nonlinear transformations of dispersal kernel shape. It confirms the Panama Canal as a major potential barrier for wildlife movement which is highly relevant for management and conservation. These findings, coupled with previous evidence on jaguar and puma habitat use at multiple scales (Craighead et al., 2022), can potentially provide assessments of functional connectivity for these and other wide-ranging species (i.e., the endangered Baird’s tapir) in the region. Situated in one of the most biodiverse and complex sections of the Mesoamerican Biological Corridor, the Panama Canal Zone arguably represents the most important example of a conservation challenge. Furthermore, the key linkage that Panama provides between the Tumbes–Chocó–Magdalena and Andean-Amazonian Bioregions and Central America makes it a critical linkage for the maintenance of connectivity between the Nearctic and Neotropics. As Panama is a key node in this connectivity, it is important to consider the broader context when planning conservation interventions. Our analysis identified two large and intact core areas of high connectivity along the central mountain range, but which were broken by a major movement barrier spanning the width of Panama in the Canal Zone. Limited connectivity along the south shore of Lake Gatun may still exist, but is uncertain, tenuous, and must be verified by targeted movement and genetics studies for multiple species.
Data availability statement
The data analyzed in this study is subject to the following licenses/restrictions: The original data is occurrence locations of endangered species and therefore is sensitive and cannot be publicly shared. Requests to access these datasets should be directed to kaminando.kcraighead@gmail.com.
Author contributions
KC and MY designed and collected the field data. SC, KC, HW, and MY conducted the spatial modelling analyses. SC, KC, MY, HW, ZK, and DM wrote the manuscript. All authors contributed to the article and approved the submitted version.
Funding
The author(s) declare financial support was received for the research, authorship, and/or publication of this article. The field component of this research was supported by Panthera, the Shanbrom Family Foundation, Mamoni Valley Preserve, and the Laney Thornton Foundation.
Acknowledgments
We thank the Ministry of Environment (MiAmbiente) and the Guna Yala General Congress for providing research permits. Idea Wild for field equipment; Mamoni Valley Preserve for providing logistical support; and the Cocobolo Reserve for allowing access to their land and facilities. The authors are grateful to the field assistants Modesto Solis, Jonathan Solis, Gabriel Salazar, Polo Acosta, Tomas Gonzales, and the volunteers involved with data collection. We thank our reviewers for suggestions to improve this manuscript.
Conflict of interest
The authors declare that the research was conducted in the absence of any commercial or financial relationships that could be construed as a potential conflict of interest.
Publisher’s note
All claims expressed in this article are solely those of the authors and do not necessarily represent those of their affiliated organizations, or those of the publisher, the editors and the reviewers. Any product that may be evaluated in this article, or claim that may be made by its manufacturer, is not guaranteed or endorsed by the publisher.
Supplementary material
The Supplementary Material for this article can be found online at: https://www.frontiersin.org/articles/10.3389/fevo.2023.1250255/full#supplementary-material
Footnotes
- ^ Lumia, G., and Cushman, S. A. Using simulation modeling to demonstrate the performance of synoptic-resistant kernels and graph theory metrics for connectivity analysis. (In Press) J Environ Manage.
- ^ Macdonald, E. A., Cushman, S. A., and MacDonald, D. W. Do corridors mitigate the effects of habitat loss: A simulation experiment. (In Press) Ecol. Modeling.
References
Araújo M. B., New M. (2007). Ensemble forecasting of species distributions. Trends Ecol. Evol. 22 (1), 42–47. doi: 10.1016/j.tree.2006.09.010
Ash E., Cushman S. A., Macdonald D. W., Redford T., Kaszta Ż. (2020). How important are resistance, dispersal ability, population density and mortality in temporally dynamic simulations of population connectivity? A case study of tigers in southeast Asia. Land 9 (11), 415. doi: 10.3390/land9110415
Atzeni L., Wang J., Riordan P., Shi K., Cushman S. A. (2023). Landscape resistance to gene flow in a snow leopard population from Qilianshan National Park, Gansu, China. Landscape Ecol., 1–22. doi: 10.1007/s10980-023-01660-8
Carr D., Barbieri A., Pan W., Iranavi H. (2006). “Agricultural change and limits to deforestation in Central America,” in Agriculture and climate beyond 2015: A new perspective on future land use patterns, 91–107.
Centro de Incidencia Ambiental – Panama (CIAM) (2022). Available at: https://ciamPanama.org/2022/02/23/carretera-entre-quebrada-ancha-y-maria-chiquita-pone-en-riesgo-la-biodiversidad/.
Chiaverini, Hahn B., Cilimburg A., Wasserman T. N., Cushman S. A. (2022). Effects of non-representative sampling design on multi-scale habitat models: flammulated owls in the rocky mountains. Ecological.
Compton B. W., McGarigal K., Cushman S. A., Gamble L. R. (2007). A resistant-kernel model of connectivity for amphibians that breed in vernal pools. Conserv. Biol. 21 (3), 788–799. doi: 10.1111/j.1523-1739.2007.00674.x
Craighead K. A., Yacelga M. (2021). Indigenous peoples’ displacement and jaguar survival in a warming planet. Global Sustainabil. 4, e7.
Craighead K., Yacelga M., Wan H. Y., Vogt R., Cushman S. A. (2022). Scale-dependent seasonal habitat selection by jaguars (Panthera onca) and pumas (Puma concolor) in Panama. Landscape Ecol. 37 (1), 129–146.
Cushman S. A., Compton B. W., McGarigal K. (2010). “Habitat fragmentation effects depend on complex interactions between population size and dispersal ability: modeling influences of roads, agriculture and residential development across a range of life-history characteristics,” in Spatial complexity, informatics, and wildlife conservation (Tokyo: Springer Japan), 369–385.
Cushman S. A., Elliot N. B., Bauer D., Kesch K., Bahaa-El-Din L., Bothwell H., et al. (2018). Prioritizing core areas, corridors and conflict hotspots for lion conservation in southern Africa. PloS One 13 (7), e0196213.
Cushman S. A., Elliot N. B., Macdonald D. W., Loveridge A. J. (2016). A multi-scale assessment of population connectivity in African lions (Panthera leo) in response to landscape change. Landscape Ecol. 31, 1337–1353.
Cushman S. A., Landguth E. L., Flather C. H. (2013a). Evaluating population connectivity for species of conservation concern in the American Great Plains. Biodivers. Conserv. 22, 2583–2605. doi: 10.1007/s10531-013-0541-1
Cushman S. A., Lewis J. S., Landguth E. L. (2014). Why did the bear cross the road? Comparing the performance of multiple resistance surfaces and connectivity modeling methods. Diversity 6 (4), 844–854. doi: 10.3390/d6040844
Cushman S. A., Macdonald E. A., Landguth E. L., Malhi Y., Macdonald D. W. (2017). Multiple-scale prediction of forest loss risk across Borneo. Landscape Ecol. 32, 1581–1598. doi: 10.1007/s10980-017-0520-0
Cushman S. A., McKelvey K. S., Hayden J., Schwartz M. K. (2006). Gene flow in complex landscapes: testing multiple hypotheses with causal modeling. Am. Nat. 168 (4), 486–499. doi: 10.1086/506976
Cushman S. A., McKELVEY K. S. (2009). Use of empirically derived source-destination models to map regional conservation corridors. Conservation.
Cushman S. A., McRae B., Adriansen F., Beier P., Shirley M., Zeller K. A. (2013b). “Biological Corridors and Connectivity,” in Key Topics in Conservation Biology 2. Eds. Macdonald D. W., Willis K. J., 384–404.
Cushman S. A., Shirk A. J., Landguth E. L. (2013c). Landscape genetics and limiting factors. Conserv. Genet. 14, 263–274. doi: 10.1007/s10592-012-0396-0
De La Torre J. A., González-Maya J. F., Zarza H., Ceballos G., Medellín R. A. (2018). The jaguar’s spots are darker than they appear: assessing the global conservation status of the jaguar Panthera onca. Oryx 52 (2), 300–315. doi: 10.1017/S0030605316001046
Elliot N. B., Cushman S. A., Macdonald D. W., Loveridge A. J. (2014). The devil is in the dispersers: predictions of landscape connectivity change with demography. J. Appl. Ecol. 51 (5), 1169–1178. doi: 10.1111/1365-2664.12282
Fahrig L. (2013). Rethinking patch size and isolation effects: the habitat amount hypothesis. J. Biogeogr. 40 (9), 1649–1663. doi: 10.1111/jbi.12130
Guerisoli M. D. L. M., Luengos Vidal E., Caruso N., Giordano A. J., Lucherini M. (2021). Puma–livestock conflicts in the Americas: A review of the evidence. Mammal. Rev. 51 (2), 228–246. doi: 10.1111/mam.12224
Harmsen B. J., Foster R. J., Silver S. C., Ostro L. E., Doncaster C. P. (2009). Spatial and temporal interactions of sympatric jaguars (Panthera onca) and pumas (Puma concolor) in a neotropical forest. J. Mammal. 90 (3), 612–620. doi: 10.1644/08-MAMM-A-140R.1
Instituto Geográfico Nacional Tommy Guardia (2022). Available at: https://ignPanama.anati.gob.pa (Accessed February 10, 2022).
Jędrzejewski W., Robinson H. S., Abarca M., Zeller K. A., Velasquez G., Paemelaere E. A., et al. (2018). Estimating large carnivore populations at global scale based on spatial predictions of density and distribution–Application to the jaguar (Panthera onca). PloS One 13 (3), e0194719. doi: 10.1371/journal.pone.0194719
Kaszta Ż., Cushman S. A., Macdonald D. W. (2020). Prioritizing habitat core areas and corridors for a large carnivore across its range. Anim. Conserv. 23 (5), 607–616. doi: 10.1111/acv.12575
Landguth E. L., Hand B. K., Glassy J., Cushman S. A., Sawaya M. A. (2012). UNICOR: a species connectivity and corridor network simulator. Ecography 35 (1), 9–14. doi: 10.1111/j.1600-0587.2011.07149.x
Macdonald D. W., Bunce R. G. H., Bacon P. J. (1981). Fox populations, habitat characterization and rabies control. J. Biogeogr. 8 (2), 145–151. doi: 10.2307/2844557
Mateo-Sánchez M. C., Balkenhol N., Cushman S., Pérez T., Domínguez A., Saura S. (2015a). A comparative framework to infer landscape effects on population genetic structure: are habitat suitability models effective in explaining gene flow? Landscape Ecol. 30, 1405–1420. doi: 10.1007/s10980-015-0194-4
Mateo-Sánchez M. C., Balkenhol N., Cushman S., Pérez T., Domínguez A., Saura S. (2015b). Estimating effective landscape distances and movement corridors: comparison of habitat and genetic data. Ecosphere 6 (4), 1–16. doi: 10.1890/ES14-00387.1
McGarigal K., Cushman S. A., Stafford S. (2000). Multivariate statistics for wildlife and ecology research (Springer Science & Business Media).
McRae B. H., Beier P. (2007). Circuit theory predicts gene flow in plant and animal populations. Proc. Natl. Acad. Sci. 104 (50), 19885–19890. doi: 10.1073/pnas.0706568104
Meyer N. F., Esser H. J., Moreno R., van Langevelde F., Liefting Y., Oller D. R., et al. (2015). An assessment of the terrestrial mammal communities in forests of Central Panama, using camera-trap surveys. J. Nat. Conserv. 26, 28–35. doi: 10.1016/j.jnc.2015.04.003
Meyer N. F., Moreno R., Reyna-Hurtado R., Signer J., Balkenhol N. (2020b). Towards the restoration of the Mesoamerican Biological Corridor for large mammals in Panama: comparing multi-species occupancy to movement models. Movement. Ecol. 8 (1), 1–14. doi: 10.1186/s40462-019-0186-0
Meyer N. F., Moreno R., Sutherland C., de la Torre J. A., Esser H. J., Jordan C. A., et al. (2020a). Effectiveness of Panama as an intercontinental land bridge for large mammals. Conserv. Biol. 34 (1), 207–219. doi: 10.1111/cobi.13384
Montalvo V. H., Sáenz-Bolaños C., Carrillo E., Fuller T. K. (2023). A review of environmental and anthropogenic variables used to model jaguar occurrence. Neotropical. Biol. Conserv. 18 (1), 31–51. doi: 10.3897/neotropical.18.e98437
Myers N., Mittermeier R. A., Mittermeier C. G., Da Fonseca G. A., Kent J. (2000). Biodiversity hotspots for conservation priorities. Nature 403 (6772), 853–858.
Myers N., Tucker R. (1987). Deforestation in Central America: Spanish legacy and North American consumers. Environ. Rev. 11 (1), 55–71. doi: 10.2307/3984219
O’Dea A., Lessios H. A., Coates A. G., Eytan R. I., Restrepo-Moreno S. A., Cione A. L., et al. (2016). Formation of the isthmus of Panama. Sci. Adv. 2 (8), e1600883.
Olsoy P. J., Zeller K.A., Hicke J. A., Quigley H. B. (2016). Quantifying the effects of deforestation and fragmentation on a range-wide conservation plan for jaguars. Biological.
Rabinowitz A., Zeller K. A. (2010). A range-wide model of landscape connectivity and conservation for the jaguar, Panthera onca. Biol. Conserv. 143 (4), 939–945. doi: 10.1016/j.biocon.2010.01.002
Sartor C. C., Wan H. Y., Pereira J. A., Eizirik E., Trigo T. C., de Freitas T. R. O., et al. (2022). Landscape genetics outperforms habitat suitability in predicting landscape resistance for congeneric cat species. J. Biogeogr. 49 (12), 2206–2217. doi: 10.1111/jbi.14498
Saura S., Torné J. (2009). Conefor Sensinode 2.2: a software package for quantifying the importance of habitat patches for landscape connectivity. Environ. Model. Software. 24 (1), 135–139.
Schoen J. M., Neelakantan A., Cushman S. A., Dutta T., Habib B., Jhala Y. V., et al. (2022). Synthesizing habitat connectivity analyses of a globally important human-dominated tiger-conservation landscape. Conserv. Biol. 36 (4), e13909. doi: 10.1111/cobi.13909
Scognamillo D., Maxit I. E., Sunquist M., Polisar J. (2003). Coexistence of jaguar (Panthera onca) and puma (Puma concolor) in a mosaic landscape in the Venezuelan llanos. J. Zool. 259 (3), 269–279. doi: 10.1017/S0952836902003230
Shirk A. J., Wallin D. O., Cushman S. A., Rice C. G., Warheit K. I. (2010). Inferring landscape effects on gene flow: a new model selection framework. Mol. Ecol. 19 (17), 3603–3619. doi: 10.1111/j.1365-294X.2010.04745.x
Singleton P. H., Gaines W. L., Lehmkuhl J. F. (2002). Large carnivores in Washington: a geographic information system weighted-distance and least-cost corridor assessment, USDA FS PNW-RP-549.
Stott P. A., Forest C. E. (2007). Ensemble climate predictions using climate models and observational constraints. Philos. Trans. R. Soc. A.: Mathematical. Phys. Eng. Sci. 365 (1857), 2029–2052.
Swinkels C., van der Wal J. E., Stinn C., Monteza-Moreno C. M., Jansen P. A. (2023). Prey tracking and predator avoidance in a Neotropical moist forest: a camera-trapping approach. J. Mammal. 104 (1), 137–145. doi: 10.1093/jmammal/gyac091
Unnithan Kumar S., Cushman S. A. (2022). Connectivity modelling in conservation science: a comparative evaluation. Sci. Rep. 12 (1), 16680.
Unnithan Kumar S., Maini P. K., Chiaverini L., Hearn A. J., Macdonald D. W., Kaszta Ż., et al. (2021). Smoothing and the environmental manifold. Ecol. Inf. 66, 101472. doi: 10.1016/j.ecoinf.2021.101472
Unnithan Kumar S., Turnbull J., Hartman Davies O., Hodgetts T., Cushman S. A. (2022). Moving beyond landscape resistance: Considerations for the future of connectivity modelling and conservation science. Landscape Ecol. 37 (10), 2465–2480. doi: 10.1007/s10980-022-01504-x
Villalva P., Palomares F. (2022). A continental approach to jaguar extirpation: A tradeoff between anthropic and intrinsic causes. J. Nat. Conserv. 66, 126145. doi: 10.1016/j.jnc.2022.126145
Wan H. Y., Cushman S. A., Ganey J. L. (2018). Habitat fragmentation reduces genetic diversity and connectivity of the Mexican spotted owl: a simulation study using empirical resistance models. Genes 9 (8), 403. doi: 10.3390/genes9080403
Wasserman T. N., Cushman S. A., Schwartz M. K., Wallin D. O. (2010). Spatial scaling and multi-model inference in landscape genetics: Martes americana in northern Idaho. Landscape Ecol. 25, 1601–1612. doi: 10.1007/s10980-010-9525-7
Woodburne M. O. (2010). The Great American Biotic Interchange: dispersals, tectonics, climate, sea level and holding pens. J. Mamm. Evol. 17, 245–264.
Zeller K. A., Jennings M. K., Vickers T. W., Ernest H. B., Cushman S. A., Boyce W. M. (2018). Are all data types and connectivity models created equal? Validating common connectivity approaches with dispersal data. Diversity Distributions. , 24 (7), 868–879.
Zeller K. A., McGarigal K., Beier P., Cushman S. A., Vickers T. W., Boyce W. M. (2014). Sensitivity of landscape resistance estimates based on point selection functions to scale and behavioral state: pumas as a case study. Landscape Ecol. 29, 541–557.
Keywords: connectivity, multi-species, Panama, ensemble, corridor, barrier, linkage, jaguar
Citation: Cushman SA, Craighead KA, Yacelga M, Kaszta ZM, Wan HY and Macdonald DW (2023) Seventy-two models of large mammal connectivity across Panama: insights into a critical biogeographic linkage zone. Front. Ecol. Evol. 11:1250255. doi: 10.3389/fevo.2023.1250255
Received: 29 June 2023; Accepted: 15 November 2023;
Published: 05 December 2023.
Edited by:
Jose F. Gonzalez-Maya, Metropolitan Autonomous University, MexicoReviewed by:
Camilo Andrés Correa-Ayram, Pontifical Javeriana University, ColombiaMauricio Vela, Wildlife Conservation Society Colombia, Colombia
Copyright © 2023 Cushman, Craighead, Yacelga, Kaszta, Wan and Macdonald. This is an open-access article distributed under the terms of the Creative Commons Attribution License (CC BY). The use, distribution or reproduction in other forums is permitted, provided the original author(s) and the copyright owner(s) are credited and that the original publication in this journal is cited, in accordance with accepted academic practice. No use, distribution or reproduction is permitted which does not comply with these terms.
*Correspondence: Samuel A. Cushman, Samuel.cushman@biology.ox.ax.uk