- 1Faculty of Forensic Medicine, Zhongshan School of Medicine, Sun Yat-sen University, Guangzhou, China
- 2Guangzhou Forensic Science Institute, Guangzhou, China
- 3Institute of Rare Diseases, West China Hospital of Sichuan University, Sichuan University, Chengdu, China
- 4School of Basic Medical Sciences, North Sichuan Medical College, Nanchong, China
- 5Department of Forensic Medicine, College of Basic Medicine, Chongqing Medical University, Chongqing, China
- 6Center of Forensic Expertise, Affiliated Hospital of Zunyi Medical University, Zunyi, Guizhou, China
- 7Anti-Drug Technology Center of Guangdong Province, Guangzhou, China
The comprehensive characterization of the fine-scale genetic background of ethnolinguistically diverse populations can gain new insights into the population admixture processes, which is essential for evolutionary and medical genomic research. However, the genetic diversity and population history of southern Chinese indigenous people are underrepresented in human genetics research and their interaction with historical immigrants remains unknown. Here, we collected genome-wide SNP data from 20 Guizhou populations belonging to three primary language families [Tai-Kadai (TK), Hmong-Mien (HM), and Tibeto-Burman (TB)], including four groups newly collected here, and merged them with publicly available data from 218 modern and ancient East Asian groups to perform one comprehensive demographic and evolutionary history reconstruction. We comprehensively characterized the genetic signatures of geographically diverse populations and found language-related population stratification. We identified the unique HM genetic lineage in Southwest China and Southeast Asia as their shared ancestral component in the demographic history reconstruction. TK and TB people showed a differentiated genetic structure from HM people. Our identified admixture signals and times further supported the hypothesis that HM people originated from the Yungui Plateau and then migrated southward during the historical period. Admixture models focused on Sino-Tibetan and TK people supported their intense interaction, and these populations harbored the most extensive gene flows consistent with their shared linguistic and cultural characteristics and lifestyles. Estimates of identity-by-descent sharing and effective population size showed the extensive population stratification and gene flow events in different time scales. In short, we presented one complete landscape of the evolutionary history of ethnolinguistically different southern Chinese people and filled the gap of missing diversity in South China.
Introduction
Modern China is a linguistically diverse region with the Altaic language family (Tungusic, Mongolic, and Turkic) in the north, Sino-Tibetan (ST; Tibeto-Burman, TB; Sinitic) mainly in the central region, and four other language families (Hmong-Mien, HM; Tai-Kadai, TK; Austronesian, AN; and Austroasiatic, AA) in the south. Chinese populations underwent extensive movements and admixtures in prehistoric and historical times inferred from ancient DNA from the Amur River Basin (ARB), West Liao River (WLR) Basin, Yellow River Basin (YRB), and Guangxi and Fujian in South China (Yang et al., 2020; Mao et al., 2021; Wang et al., 2021a; Wang et al., 2021c). Molecular genetic studies based on genome-wide SNP data from modern Chinese populations revealed that East Asians could be genetically divided into five major genetic clusters or clines. These substructures were associated with different geographical divisions or linguistic affiliations, including the ARB cline in Northwest China, the Tibetan cline in the highland of East Asia, the HM cline in Southwest China, the southern Chinese cline, and the Han Chinese cline (He et al., 2021a; He et al., 2021d; He et al., 2023c; He et al., 2023d; Wang et al., 2023). Southwest China possesses ~50% of China’s ethnic minority groups, accounting for ~37% of the population in this region (Harper and Mayhew, 2007). Guizhou is the province with the most mountains in Southwest China and is located in the center of the Yungui Plateau. Guizhou harbors three linguistically diverse families, including ST, HM, and TK, and has an enormous ethnolinguistic diversity, followed by Yunnan Province. However, genetic studies focused on non-Han populations in this region are underrepresented. Thus, more genetic studies focused on ethnolinguistically different Guizhou minority indigenous populations should be conducted.
In addition to large-scale genomic cohorts being conducted to advance the understanding of genetic determinants of disease susceptibility and genetic background of Han Chinese populations, many molecular anthropological and evolutionary genetic studies have started to focus on the genetic diversity of ethnolinguistically diverse minority groups in China, such as the characterization of population history and biological adaptation of Tibetan (Yi et al., 2010; Qi et al., 2013; Huerta-Sanchez et al., 2014; Lou et al., 2015; Deng et al., 2019) and Sherpa (Zhang et al., 2017) from the Tibetan Plateau and the dissection of Eurasian admixture signature in Uyghur (Pan et al., 2022) and Hui (Ma et al., 2021). However, we should note that most previous genetic studies were conducted based on forensic-related genetic markers (short tandem repeats, insertion/deletions, micro-haplotypes, ancestry-informative SNPs, and other X- or Y-linked markers) or lower-density SNPs in the merged dataset (Mengge et al., 2020; Zhang et al., 2021b; Chen et al., 2022). Moreover, the advances in computational and statistical techniques have promoted the exploration of a more detailed and complex demographic history of worldwide populations, such as the statistical methods for reconstructing sample frequency spectrum and phased haplotype data (Bergstrom et al., 2020; Byrska-Bishop et al., 2022). However, population genetic studies focused on ethnolinguistically different Chinese populations were mainly conducted based on the sharing alleles via the f-statistics and other descriptive analyses [principal component analysis (PCA) and ADMIXTURE]. Genetic studies on exploring fine-scale population structure via sharing haplotype patterns are lacking, which can dissect fine-scale population substructures (Lawson et al., 2012).
Hence, deep population admixture modeling with more representative uncharacterized populations and state-of-the-art statistical methods should be employed in the new era of population genomic study. Thus, we collected the genome-wide data of 700K SNPs from 20 Guizhou populations, including four newly collected ones [Miao_Kaili (KLM), Dong_Kaili (KLD), Tujia_Yanhe (YHTJ), and Gelao_Daozhen (DZGL)]. According to the fifth census, Guizhou has a more than 35 million population. Miao people comprise ~12.2% of the total population; Dong, ~4.6%; Tujia, ~4.1%; and Gelao, ~1.6%. We then made a comprehensive genetic analysis based on the sharing alleles and haplotypes to illuminate the following key questions: (1) What is the fine-scale genetic landscape of our studied four Guizhou populations, and what are the patterns of the genomic diversity of Guizhou people? (2) What is the genetic similarity and differentiation between our studied populations and other Guizhou reference populations? (3) What is the genetic relationship between Guizhou people and ancient East Asian reference populations? (4) What is the demographic history of Guizhou people and their genetic interaction with surrounding and incoming populations? Our comprehensive population admixture models showed that ethnolinguistically diverse southwestern Chinese populations had differentiated genetic structures. The most significant genetic differentiation was observed between HM and others. Our results also found extensive admixture signals derived from ancestral source proximity from northern and southern East Asians, suggesting that ancient population movements and admixtures between ST and other southern Chinese indigenous populations contributed to the observed patterns of genetic and ethnolinguistic diversity in Southwest China.
Materials and methods
Sample collection, array genotyping, and reference dataset
We collected blood samples from 19 Tujia people in Yanhe, 19 Gelao people from Daozhen, 10 Miao people from Kaili, and 10 Dong people from Kaili in Guizhou Province with informed consent. We required participants to be indigenous residents in the sampling location †for at least three generations. The Ethics Committee of West China Hospital of Sichuan University and the North Sichuan Medical College has approved this project. Our work was conducted following the recommendations stated in the Helsinki Declaration of 2000. Genomic DNA was extracted using the PureLink Genomic DNA Mini Kit (Thermo Fisher Scientific) and quantified via the Applied Biosystems 7500 Real-time PCR System (Thermo Fisher Scientific). We genotyped 699,537 genome-wide SNPs, including 645,199 autosomal, 26,341 X-chromosomal, 22,512 Y-chromosomal, 1,287 pseudo-autosomal, and 4,199 mitochondrial SNPs, in newly sampled individuals using the Infinium Global Screening Array (Illumina, CA, USA) and quality-controlled via the following internal standards. We aligned raw genotype data to the human reference genome assembly GRCh37 (human_g1k_v37), and then we removed SNPs with a missing call rate exceeding 0.05 and individuals with a missing genotype rate exceeding 0.1 using PLINK (–geno: 0.05 –mind: 0.1). Additionally, we removed SNPs that failed the Hardy-Weinberg test using PLINK with the “–hwe 0.0001” option. We calculated the PI_HAT values using PLINK with the “–genome” parameter and estimated pairwise kinship coefficients using KING with the “-related –ibs” parameter. We removed one of the pairs of individuals with a PI_HAT value greater than 0.125 and kinship coefficients greater than 0.0625 (up to third-degree relationships). We retained 516,448 SNPs among 58 individuals in the following population genetic studies. We merged our data with previously published high-density SNP data that were genotyped via the Illumina array used in this study (Lu et al., 2020; Guo et al., 2021; Yao et al., 2021; Wang et al., 2021b; Zhang et al., 2021b; Chen et al., 2022; He et al., 2022; Wang et al., 2022; He et al., 2023c), previously reported Human Genome Diversity Project (HGDP) and Oceania genomic resource (Bergstrom et al., 2020; Choin et al., 2021), and Human Origins (HO) and 1240K datasets retrieved from the Allen Ancient DNA Resource (Jeong et al., 2019; Liu et al., 2020; Ning et al., 2020; Yang et al., 2020; Kutanan et al., 2021; Wang et al., 2021a; Mallick et al., 2023) to form three different population genetic analysis datasets: high-density Illumina dataset (~461K overlapping SNPs), medium-density merged 1240K dataset (~147K), and low-density merged HO dataset (~57K).
Principal component analysis and ADMIXTURE modeling
We used the smartpca software implemented in EIGENSOFT packages (Patterson et al., 2006) to conduct PCA for exploring the general population relationship among ethnolinguistically diverse modern populations and ancient people from East Asia and Southeast Asia with the default parameters. All ancient East Asians were projected onto the essential background based on the first two components. The AA-speaking Mlabri people were separated from others in the original PCA and projected onto the general background. We used modern populations from Altaic, ST, AN, AA, TK, and HM language families as the reference populations. To further explore the admixture proportion of our studied individuals, we used unsupervised model-based ADMIXTURE (Alexander et al., 2009) to dissect the ancestral composition with different numbers of ancestral sources. We set the K values ranging from 2 to 20 and used 100 bootstrap replicates (-B100) as well as 10-fold cross-validation (–cv = 10) to explore the best-fitted models.
Estimation of genetic distances
We calculated the pairwise Fst genetic distances between our studied populations and other reference populations following the approach provided by a previous study (Weir and Cockerham, 1984). We used the qp3pop program in ADMIXTOOLS (Patterson et al., 2012) with default parameters to conduct the outgroup f3-statistics in the form f3(Source1, Source2; Outgroup). We used central African Mbuti people as the outgroup. When we used four studied populations as the second source, the estimated f3-values can be used to show the genetic affinity between newly studied populations and other reference populations. All modern and ancient East Asians were used as the first source.
Identification of admixture signatures
To explore the admixture signals, we conducted admixture f3-statistics in the form f3(Source1, Source2; targeted populations) using qp3pop in ADMIXTOOLS (Patterson et al., 2012). The estimated values with Z-scores less than −3 indicate that two fitted source populations can be used as potential ancestral sources. We then conducted four population analyses in the form f4(population1, population2; population3, Mbuti) using qpDstat package in ADMIXTOOLS (Patterson et al., 2012) to explore the possible topologies with potential admixture signatures in three different ways with the parameters of f4Models: Yes. We conducted f4(studied population1, studied population2; reference populations, Mbuti) to explore the genetic homogeneity or heterogeneity between studied populations. We used f4(reference population1, reference population2; studied populations, Mbuti) to explore the genetic affinity between studied populations and one of the reference populations compared with the other reference populations. Finally, we performed f4(reference population1, studied populations; reference population2, Mbuti) to explore whether the reference populations shared more or less ancestry with our studied people compared with other reference populations.
Phylogenetic relationship reconstruction
Phylogenetic topology with gene flow events can provide much evidence to support the truth of population evolution and their corresponding admixture events. Thus, we first conducted TreeMix (Pickrell and Pritchard, 2012) analysis with the migration edges ranging from 2 to 10 based on the allele frequency distribution to explore the genetic relationship between Guizhou populations and other reference populations. We also inferred the phylogenetic relationship between Guizhou populations and East Asian reference populations using IQ-TREE2 (Minh et al., 2020) and we adopted the standard model selection (-m TEST) to determine the best-fitted model. We randomly selected 10 samples from each population, and all samples were included when the overall sample size of a population was less than 10. Afterwards, we used qpGraph with Yangtze River rice farmers and Yellow River millet farmers as the ancestral surrogates of southern and northern East Asians to illuminate the formation of our targeted populations (Maier et al., 2023).
QpAdm-based admixture coefficients
To illuminate the ancestral proportion using the formal estimated admixture models with ancestral populations as the ancestral sources, we conducted two-way and three-way admixture models using qpAdm (Patterson et al., 2012). The outgroups that were used to constrain the confidence of the estimated admixture models followed our previous studies (He et al., 2023c).
Genome-wide patterns of haplotype sharing and admixture time estimation
We used Segmented HAPlotype Estimation & Imputation Tool (SHAPEIT v.2.0) (Delaneau et al., 2011) with the parameters of “–burn 10 –prune 10 –main 30” to phase the genome-wide SNP data to obtain the haplotype data from the paternal and maternal sides. We used the fineSTRUCTURE framework (Lawson et al., 2012) to infer the fine-scale population structure based on the similarity of haplotype sharing patterns. The number of total iterations for MCMC, maximization steps when finding the best state, the minimum number of SNPs for EM estimation, and fraction of individuals used for EM estimation were set to 100,000, 50,000, 1,000 and 0.1 (-s3iters 100000 -s4iters 50000 -slminsnps 1000 -slindfrac 0.1), respectively. We then used ChromoPainterv2 (Lawson et al., 2012) with the parameter of “-s 0 -i 10 -in -iM” to paint each chromosome of recipient populations as a combination of all other sequences from donor populations. Refined-IBD was used to estimate the pairwise IBD (identity-by-descent) matrix (Browning and Browning, 2011), and ibdne.23Apr20.ae9.jar was used to estimate the effective population size changes (Browning and Browning, 2015; Browning et al., 2018). Finally, we used GLOBETROTTER (Hellenthal et al., 2014) with options of “bootstrap.date.ind: 1 and bootstrap.num: 100” to identify and date admixture events occurring in the ancestral surrogates of target populations. The constant generation time was set to 29 years. We also used sourcefindv2.R (https://github.com/hellenthal-group-UCL/sourcefindV2) to identify the IBD-based genetic contribution from different ancestral sources to our studied populations.
Results
Overview of population genetic structure
Here, we integrated genome-wide SNP data of 530K SNPs from 20 populations to explore the comprehensive fine-scale genetic structure among ethnolinguistically diverse populations in Guizhou Province, including four populations newly collected here. We conducted PCA among 1,722 individuals from 37 ancient populations (115 individuals) and 147 modern populations (1,607 individuals) to provide an overview of population structure of East Asians. We found significant genetic differentiation between northern East Asians consisting of Altaic speakers and southern East Asians and Southeast Asians comprising AN, AA, HM, and TK speakers. We also found that HM-speaking populations from Guizhou (Miao and Yao) and Mlabri Hunter-Gatherers were separated from their neighbors (Supplementary Figure 1). To further explore the fine-scale population structure within regional populations, we first projected Mlabri into a basal Asian PCA skeleton and deeply characterized the fine-scale population structure among East Asians. We found a consistent pattern of genetic differentiation among ethnolinguistically diverse populations. Generally, genetic variance inferred from the top two components localized AA-speaking Htin and AN-speaking Ede people, HM-speaking Hmong, and some Altaic-speaking people (Mongolic and Tungusic people) in the three extremes of the patterns of genetic gradient and differentiation (Figure 1A). PC3 separated TB-speaking populations (purple) from other reference populations (Supplementary Figure 2). HM-speaking people from Guizhou and Sichuan Provinces in China, Vietnam, and Thailand in Southeast Asia formed a specific genetic cline in the genetic landscape of East Asians and Southeast Asians. Newly genotyped KLM clustered with Guizhou Gejia, Dongjia, and Xijia people. Interestingly, all four newly studied populations were grouped into three clusters. TK-speaking KLD separated from Miao people and localized in the intermediated position among HM, ST, and AA/AN clines. TK-speaking DZGL also separated from KLD and clustered with YHTJ, which grouped closely with Han Chinese populations.
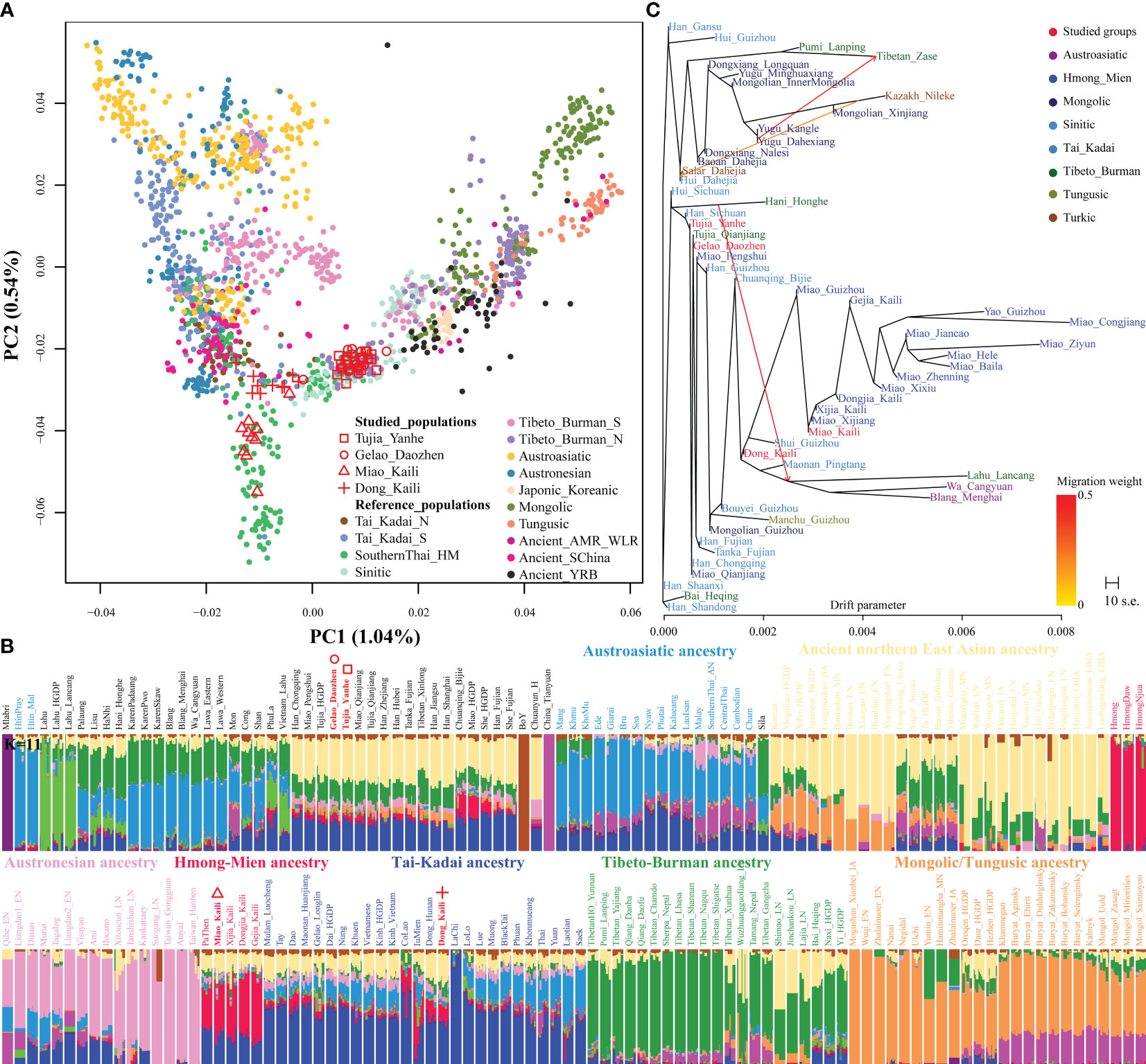
Figure 1 Patterns of the genetic structure of Guizhou populations and other East Asians. (A) Principal component analysis (PCA) of East Asians inferred from the top two components. Ancient populations and modern Mlabri were projected onto the modern genetic background. Colors indicate different language families. The detailed legend for each population is presented in Supplementary Figure 2. (B) Admixture components of included populations inferred from model-based ADMIXTURE. (C) TreeMix-based phylogenetic relationship between Guizhou populations and surrounding populations obtained based on the high-density SNP data that were genotyped via the Illumina array.
To further dissect the ancestry composition of these newly genotyped populations and explore their ancestral source surrogates, we conducted model-based ADMIXTURE analysis among modern and ancient East Asians. We assumed that 11 ancestral source populations contributed to the formation of all observed genetic variation (K = 11), which was regarded as the best-fitted model with the least estimated cross-validation error (Supplementary Figure 3). We found that some ancestry compositions were unique in some geographically isolated people, such as AA-speaking Mlabri and Htin, TK-speaking Boy and TB-speaking Lahu, or temporally specific populations (Tianyuan). These ADMIXTURE-based patterns were consistent with their relatively isolated sampling positions. Other six ancestral components contributed to the mosaic ancestry composition of modern and ancient East Asians and their geographical neighbors (Figure 1B). Four populations from Guizhou Province also showed genetic differentiations: DZGL and YHTJ people harbored their primary ancestry from ancient northern East Asians (ANEAs), modern northern TB ancestors, and TK-related southern East Asian sources, which grouped together with geographically close Tujia, Miao, and Han Chinese populations. KLM people grouped tightly with geographically close HM people and harbored the primary ancestry related to the ancestor of Hmong people and also possessed ancestry related to TK people and ANEAs from the YRB. However, KLD possessed ancestry from two significantly differentiated ancestral populations associated with the ancestor of modern TK people and ancient YRB people, and it shared a similar pattern of ancestry composition with Dong from Hunan.
We utilized the high-density Illumina dataset to reconstruct the phylogenetic relationship between Guizhou populations and other Chinese populations and phased it for further fine-scale population structure analysis. When we focused on the genetic diversity of linguistically different Chinese populations, we observed one northern genetic cline represented by Altaic and ST people and another southern genetic cline represented by HM people (Supplementary Figure 4A). Model-based ADMIXTURE analysis among these populations revealed five major ancestral components with the proportion maximized in Han Chinese, Kazakh, Tibetan, Yao, and Maonan (Supplementary Figure 4B), respectively. All five identified ancestries were observed in Guizhou populations, suggesting that multiple ancestral sources contributed to the observed genetic and linguistic diversity. Even Western Eurasian ancestry represented by Kazakh was observed in Guizhou Hui people, suggesting that the dispersal of the ancestor of Hui people contributed to the Western Eurasian-related ancestry in Guizhou populations. Yao-represented ancestry was unique and widely distributed among Guizhou HM people, consistent with the observed patterns in the TreeMix and IQ-TREE-based clustering patterns (Figure 1C; Supplementary Figure 5). Finally, we explored the genetic relationship between Guizhou TK-, HM-, and ST-speaking populations and coastal AN people by merging our data with the extracted overlapping SNP data from the HGDP (Supplementary Figures 6, 7). We observed substantial genetic differentiation between Guizhou and coastal AN populations, as well as between linguistically diverse Guizhou populations (Supplementary Figure 6). HM people generally formed a genetic cline, and newly genotyped Guizhou groups were scattered among this cline. In the ADMIXTURE results, we found that AN people significantly influenced Guizhou TK people and had a minor influence on Guizhou HM-/ST-speaking populations (Supplementary Figure 7A). We reconstructed the TreeMix-based phylogenetic relationship and confirmed differentiated genetic relatedness between linguistically diverse Guizhou populations (Supplementary Figure 7B). IBD-based clustering patterns based on the average lengths and counts also showed a relatively separated genetic relationship between Guizhou populations and AN people (Supplementary Figures 7C, D). The identified patterns of ancestral composition and phylogenetic relationship illuminated that the gene pool of Guizhou populations possessed unique HM-related ancestral lineage and also had been significantly influenced by surrounding historical populations’ migrations and admixtures, such as previously reported migration of the ancestor of Hui people and persistent southward of northern East Asians from the Paleolithic across Holocene to historical periods (Yang et al., 2020; Liu et al., 2021b).
Differentiated demographic history among linguistically diverse Guizhou populations
To formally test the genetic similarities and differences between and within Guizhou populations and surrounding people related to ST, HM, TK, AA, and AN language families, we estimated the genetic distances to illuminate the genetic affinity and conducted f-tests to explore the differentiated allele sharing between Guizhou populations and their ancestral surrogated proximities. The estimated pairwise Fst values and the results of descriptive analysis showed the different genetic structures and demographic history of Guizhou HM-, TK-, and ST-speaking populations (Supplementary Table 1). This observed pattern suggested their complex admixture landscape and served as a window of the genetic background of ethnolinguistically diverse southern Chinese people. The formal analysis focused on the allele sharing via outgroup f3(reference populations, studied populations; Mbuti) showed that KLM possessed the most substantial genetic drift with Guizhou Yao people; a similar pattern was also observed when focused on KLD people. However, DZGL and YHTJ people had the closest genetic relationship with Han Chinese populations (Supplementary Table 2). We used symmetrical f4-statistics of f4(reference population1, reference population2; studied populations, Mbuti) to explore the differentiated genetic structure between Guizhou groups and other reference populations (Figure S8). We observed the highest Z-scores in the f4(Han people, reference population; YHTJ/DZGL, Mbuti), which suggested that DZGL and YHTJ shared more alleles with northern East Asians related to Han Chinese than other reference populations (Figures S8A, B). The obtained estimates of f4(DZGL, YHTJ; reference population, Mbuti) indicated that linguistically different DZGL and YHTJ formed a genetically close clade and showed more allele sharing compared with reference populations, suggesting that these two linguistically different but geographically close studied populations had similar genetic structure (Supplementary Table 2). Interestingly, we observed the highest positive Z-scores when we focused on HM/TK-speaking populations, indicating that Miao and Dong people from Guizhou Province shared more alleles with southern indigenous people than other East Asians (Figures S8C, D). Additionally, we conducted asymmetrical f4(Geographically diverse Miao, KLM; reference populations, Mbuti) and found that HM-speaking Miao from Vietnam and TK/AN people shared more alleles with KLM compared to Songtao and Leishan Miao (Supplementary Table 3). The identified patterns of allele sharing showed the differentiated genetic structure among geographically different HM people. The estimates of negative Z-score of −7.507 in the f4(DZGL, KLD; Hmong, Mbuti) showed direct genetic differentiation between geographically diverse TK people and genetic interaction between HM and TK people, suggesting that compared to DZGL people, KLD people obtained more genetic influence from HM people. The estimated positive values in f4(YHTJ/DZGL, KLD; Tibetan/ANEAs, Mbuti) demonstrated that TK-speaking DZGL and TB-speaking YHTJ shared more ancestry related to northern East Asians than southern indigenous Dong people (Supplementary Table 3). The estimated Z-scores of f4(Guizhou populations, KLM; reference populations, Mbuti) further suggested that KLM obtained more gene flow from geographically distinct Miao people relative to geographically close KLD, and linguistically different DZGL and YHTJ shared more alleles with northern East Asians compared with KLM (Figures 2A–C). Interestingly, Xijia, an unrecognized ethnic group in Guizhou but officially classified as a part of Miao, had the closest genetic relatedness to KLM (Figure 2D). At the same time, Yaoai Yao showed more allele sharing with geographically diverse HM-speaking populations relative to linguistically close KLM (Figure 2E), further indicating the genetic differentiation between geographically diverse HM people.
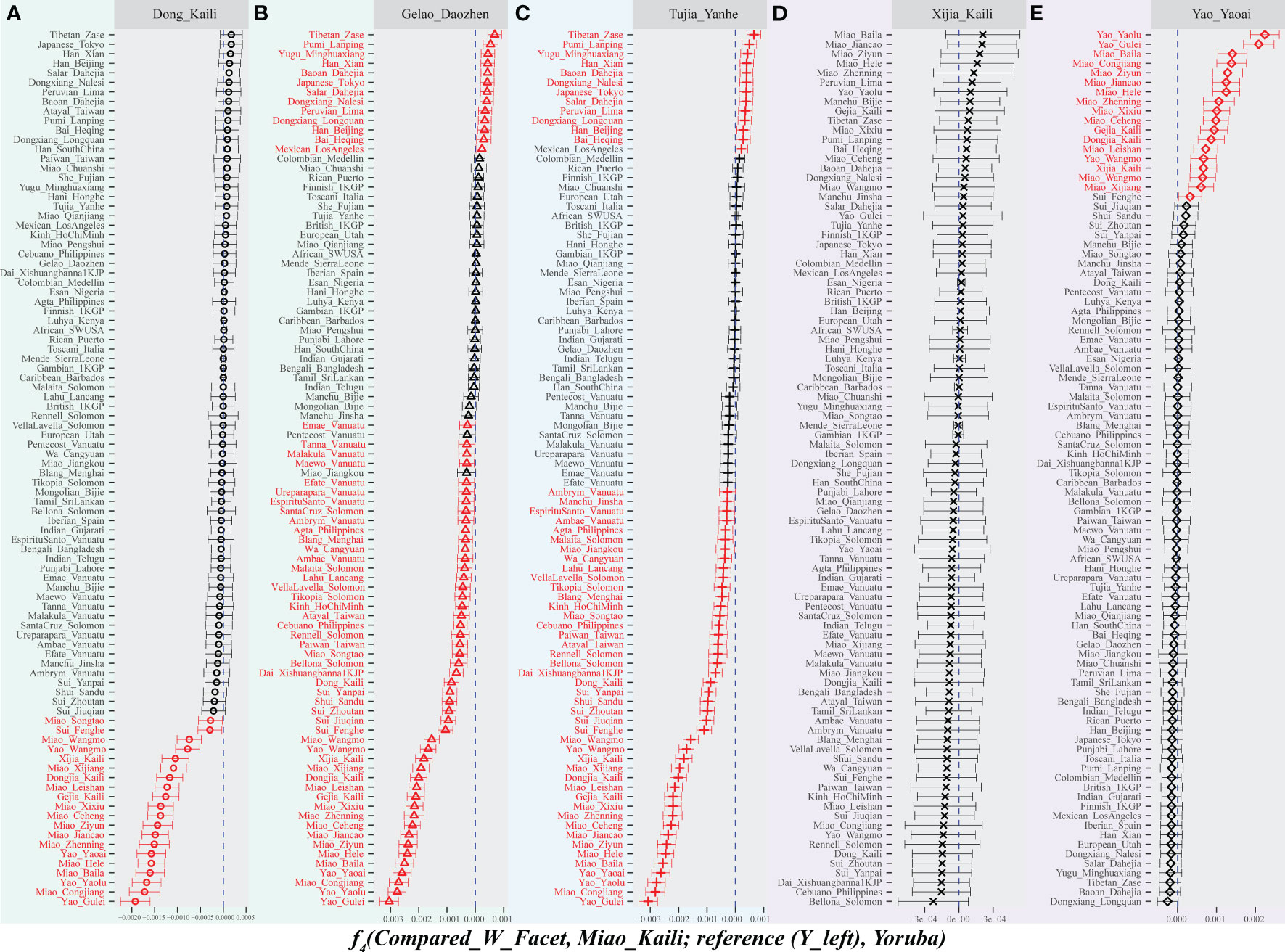
Figure 2 Differentiated sharing patterns between Guizhou Miao and other Guizhou populations compared with worldwide reference populations. Populations on the left donate worldwide reference populations, and populations on the top denote other Guizhou populations, that is, “Compared_W_Facet” in the f4-statistics. The dashed blue line indicates that the f4value is zero. The symbol marked in red on the left side of the blue dotted line refers to the reference population that has an f4value less than 0 and a Z value less than −3, and the symbol marked in red on the right side of the blue dotted line refers to the reference population that has an f4value greater than 0 and a Z value greater than 3.
Haplotype data were evidenced to have more power to dissect fine-scale genetic structure. Thus, we used phased SNP data to explore the demographic history and estimate the admixture process of four newly studied populations and other Guizhou populations. The PCA clustering pattern based on the pairwise ancestry coefficient can separate four studied people into three clusters: KLM, KLD, and overlapping YHTJ and DZGL (Supplementary Figure 9A). These observed patterns of population stratification within Guizhou populations confirmed the population differentiation inferred from the f-statistics. Heatmaps of the ancestry coincidence matrix and corresponding reconstructed population dendrogram based on the average chunk counts also showed two major separated branches (Supplementary Figures S9B–E), consistent with the observed patterns in the PCA, TreeMix, and ADMIXTURE based on the allele frequency distribution (Supplementary Figures S9F–I). We calculated and visualized the sharing IBD length and count and found that Guizhou populations shared more IBD with each other based on the total population IBD or different IBD categories (Figures 3A, B). We further focused on populations from the Yungui Plateau and found fine-scale patterns of IBD sharing among HM, ST, and TK people in different IBD lengths, which suggested continuous gene flow among them in the past 2,000 years (Figures 3C–G). Focused on the genetic diversity within Guizhou populations, clustering patterns based on admixture estimates and co-ancestry matrix showed that Miao people were separated from other Guizhou populations (Supplementary Figures 10, 11A). Based on the patterns of sharing IBD, we identified two major branches and several subbranches among Guizhou populations, including the subclusters identified among HM-speaking Miao people (Supplementary Figures 11B, C). We also found that Miao people shared the longest and the largest IBD with other HM-speaking populations. We then estimated the effective population size of four newly studied populations. Compared with DZGL people, KLD, KLM, and YHTJ people harbored a relatively low ancient population size (Figure 3H). DZGL trended to experience population declines in the Holocene period. We should also pay attention to the fact that the accurate IBD segment estimation and local ancestry calls can influence the accuracy of the estimates of historical population size (Browning and Browning, 2015; Browning et al., 2018). Furthermore, including more representative geographically different Guizhou people and analyzing whole-genome sequencing data combined with the PSMC, FitCoal, MSMC2, or SMC++ should be conducted to validate these observed patterns in the following large-scale genome sequencing projects, such as the 10K Chinese People Genomic Diversity Project (10K_CPGDP) (Schiffels and Durbin, 2014; He et al., 2023b; Hu et al., 2023).
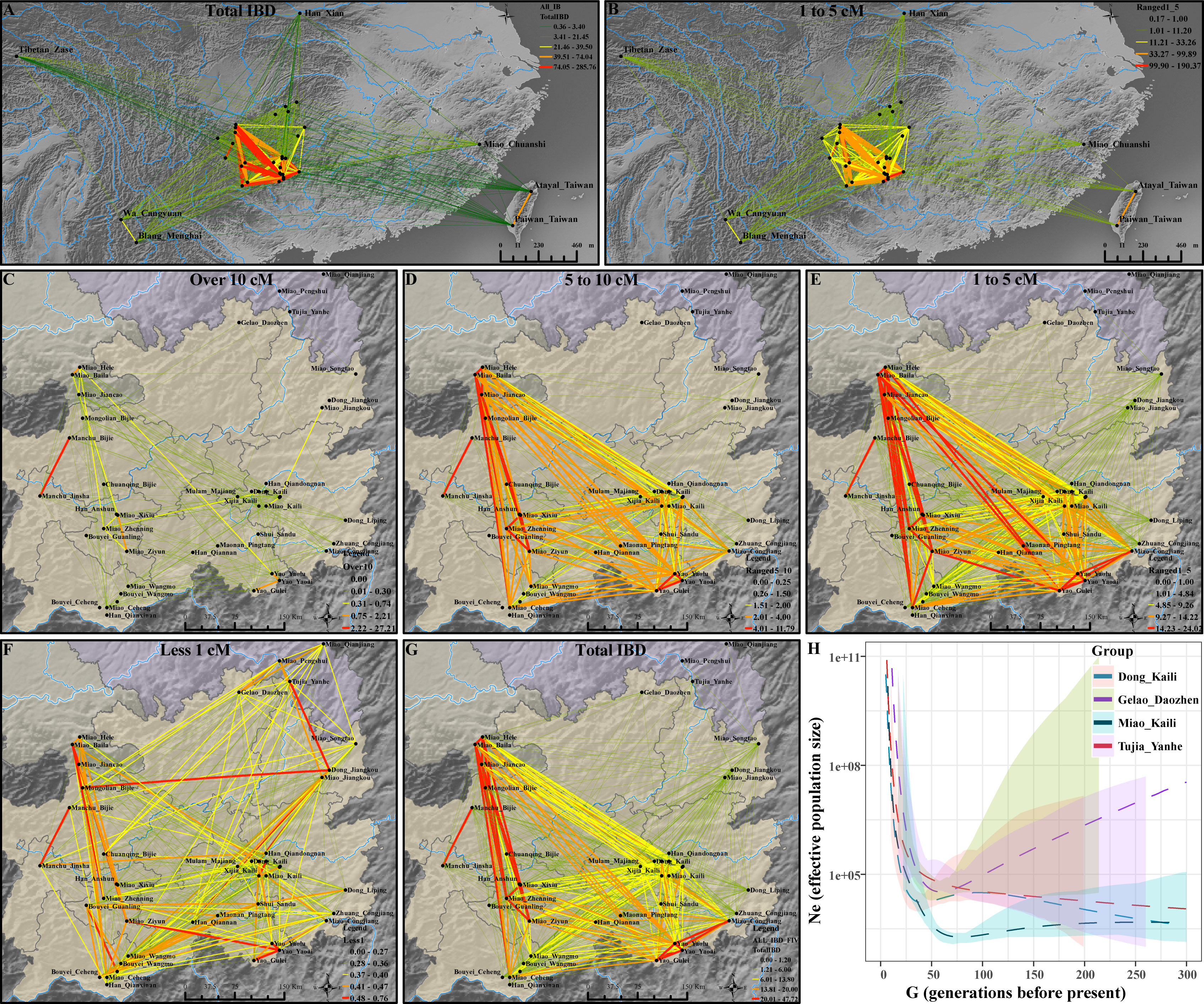
Figure 3 Extensive genetic interaction and effective population size. (A, B) IBD sharing patterns among East Asians with the total IBD length and length ranging from 1 to 5 cM. (C–G) Network visualization of population average IBD length in the range of over 10 cM, 5–10 cM, 1–5 cM, and less than 1 cM and the total length among Yungui populations. (H) The effective population size of ethnolinguistically diverse Guizhou populations over the past 150 generations estimated based on the sharing IBD length.
Estimates of admixture proportions and admixture dates
To directly explore the admixture signatures of potentially existing ancestral sources for admixture events in Guizhou populations, we conducted admixture f3-statistics in the form f3(Source1, Source2; targeted Guizhou populations). Significant negative Z-scores for KLM and KLD people were observed when we used Hmong and Han Chinese as the indigenous and northern surrogate sources (Supplementary Table 4). Focused on DZGL and YHTJ people, we observed the most negative signals when we used TK-speaking Dai as the southern source and Han Chinese as the northern ancestral source. To estimate the ancestral proportion of these identified ancestral sources, we conducted two-way qpAdm admixture models and found that KLM and KLD harbored more ancestry related to southern East Asians and DZGL and YHTJ people shared more alleles related to northern East Asians (Supplementary Table 5). We further constructed the admixture processes via three-way admixture models with ancient genomes from the YRB, coastal southeastern East Asia, and inland Southeast Asia to explain the observed gene pool of Guizhou populations (Supplementary Table 6). We found that all three ancient ancestral sources contributed to the formation of modern Guizhou populations. To directly reconstruct the phylogenetic relationship with admixture events, we reconstructed qpGraph-based demographic models to explore the formation patterns for modern Guizhou people (Figures 4A–C). The fitted models showed that northern ancestral sources related to the Middle Neolithic Xiaowu people and southern ancestral sources related to Hanben contributed to the genetic formation of four Guizhou populations with more ancestry derived from southern East Asians. We further explored the demographic history of Guizhou populations without early diverged ancient source populations using the automatic qpGraph-fitted strategies implemented in ADMIXTOOLS2 (Figure 4D), and we also confirmed that ancient northern and southern East Asians contributed to the gene pool of these ethnolinguistically diverse populations. We found that HM-speaking KLM and KLD harbored more ancestry related to ancient southern lineage, and YHTJ and DZGL received more genetic influence from ancient northern East Asians. We finally confirmed the north–south admixture pattern within newly genotyped Guizhou populations via Sourcefind-based IBD sharing patterns and identified a one-date-multiway admixture event for KLD and a one-date admixture event for YHTJ, DZGL, and KLM via the GLOBETROTTER, respectively. Estimates of admixture dates showed that the Cambodian−Han admixture in KLD occurred ~696 years ago (24 generations); the Li−Han admixture in YHTJ occurred ~1,189 years ago (41 generations); the Li−Han admixture in DZGL occurred ~986 years ago (34 generations); and the Cambodian−Yao admixture in the relatively isolated KLM occurred ~812 years ago (28 generations). Our fitted admixture models suggested that extensive genetic admixture shaped the patterns of genetic diversity and admixture landscape of Southwest Chinese people.
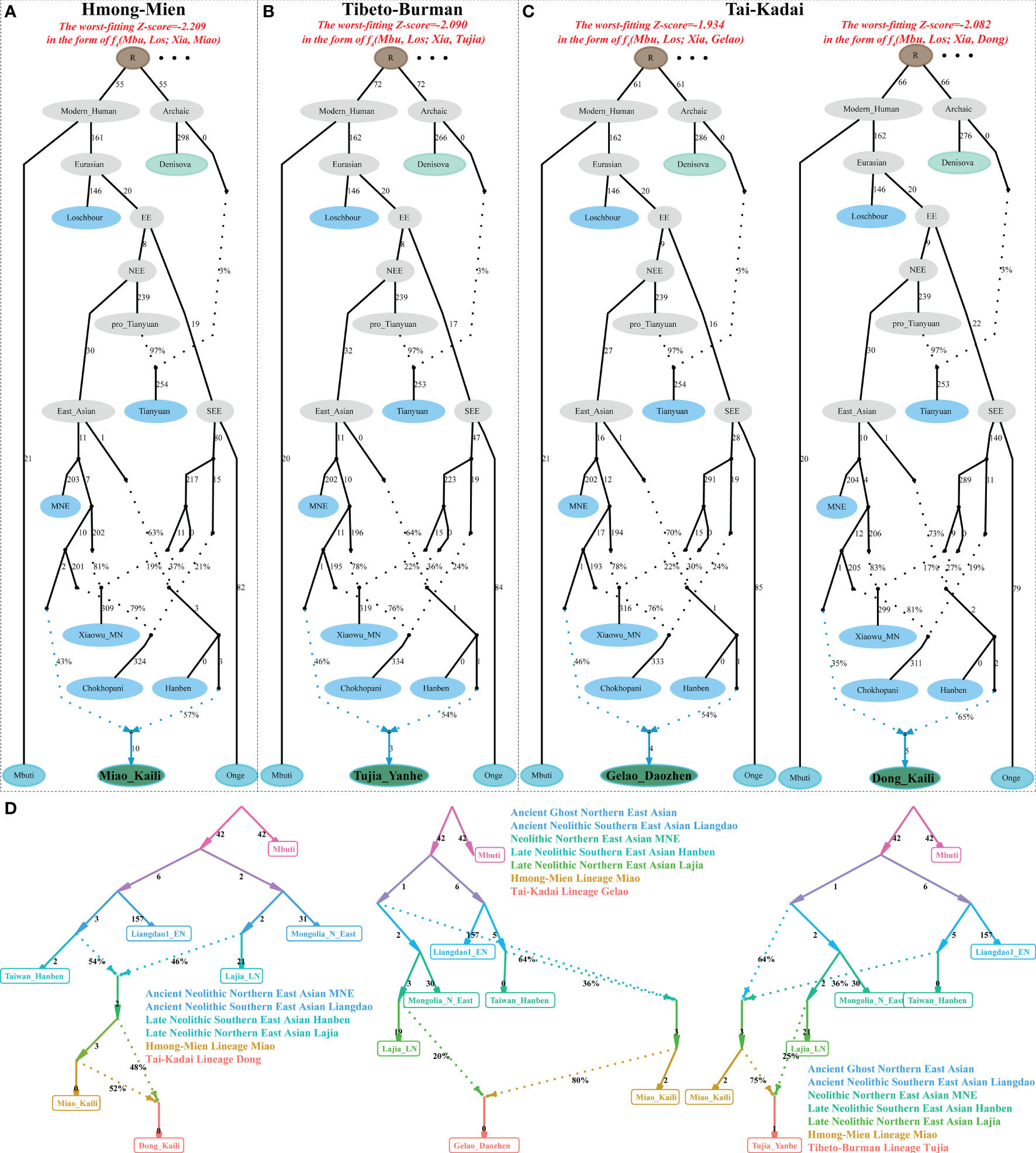
Figure 4 Demographic models of four representative populations from Guizhou inferred from qpGraph. (A–C) We started with a graph topology that fitted the data for Denisovan, Loschbour, Tianyuan, and Mbuti and one admixture event. We grafted on Mongolia_N_East, Neolithic Yellow River farmers, Nepal Chokhopani, Taiwan Hanben, KLM (A), YHTJ (B), DZGL, and KLD (C) in turn. (D) The reconstructed qpGraph-based topology with Mbuti, Early Neolithic Southern East Asian Liangdao, Mongolia_N_East, Taiwan Hanben, Neolithic Yellow River farmers, and four Guizhou populations. The dotted line indicates the admixture events with the corresponding admixture proportion. One thousand times, the branch length was labeled on each edge. Light green indicates the archaic people and dark green indicates the modern studied populations. Other modern and ancient ancestral lineages were labeled with light blue color. EE, eastern Eurasian; NEE, northern Eastern Eurasian; SEE, southern Eastern Eurasian; MNE, Mongolia Neolithic East.
Discussion
Capturing the full landscape of human genetic diversity is the initial goal of human genetics research. However, underrepresenting human genetic diversity of ethnolinguistically diverse populations hindered the comprehensive understanding of the genetic differentiation of worldwide populations, adaptation, and medical relevance. Recent genetic studies have emphasized that European bias has influenced the transferability of genetic risk-predicting models focused on European ancestry to other worldwide populations, such as East Asians and Africans (Sirugo et al., 2019; McQuillan et al., 2020). Although China harbors enriched ethnolinguistic, cultural, and ecological diversity, we also gradually noticed a Han bias in genetic studies, such as the three recently reported Chinese clinical cohort studies, including the NyuWa genome resource (Zhang et al., 2021a), the Westlake BioBank for Chinese pilot project (Cong et al., 2022), and the China Metabolic Analytics Project (Cao et al., 2020), which mainly recruited Han Chinese people in these cohorts. We have acknowledged that comprehensive characterization of the genetic diversity of ethnolinguistically diverse populations can promote advances in molecular anthropology, medical, and population evolutionary research (McQuillan et al., 2020). This work collected four populations (KLM, DZGL, KLD, and YHTJ) from Guizhou, which is one of the regions with the richest biodiversity in China. We then merged it with publicly available genome-wide data from Guizhou populations and other modern and ancient reference genomes from worldwide populations to elucidate the following topics: (1) the formation of Guizhou TK, HM, and ST people and (2) the formation and association between ethnolinguistic diversity and genetic diversity.
Previous genetic studies focused on a single population or populations from the same language family have tried to illuminate the genetic admixture model of ethnolinguistically diverse Guizhou populations (Chen et al., 2021; Chen et al., 2022). Other genetic studies focused on large sample sizes or multiple populations were conducted based on low-resolution marker systems used in forensic science, which limited the comprehensive exploration of the fine-scale genetic structure and the status of population interaction (He et al., 2019; He et al., 2021b). The combined datasets could be used to explore Guizhou Province’s fine-scale population substructure and language-related population stratification. We identified differentiated demographic history among four newly collected populations and all merged Guizhou populations based on different statistical methods. We emphasized the importance and differences between this work and previous genetic reports, including the comprehensively reconstructed admixture models based on the sharing alleles and haplotypes and the high-density SNP data. PanAsia project used a 50K SNP-based dataset from Asian populations and identified the association between genetic structure, geographic isolation and linguistic affiliation (Consortium et al., 2009). Most previous genetic studies also used the merged dataset (50K–190K) to explore the genetic relationship and admixture models of their focused populations, which has limited the use of most of the genetic diversity of missing SNPs in the HO and 1240K SNP panels (Zhang et al., 2019; He et al., 2021c; Yao et al., 2021; Wang et al., 2022). Thus, we used three different datasets (original high-density worldwide modern population dataset, and medium-density and low-density modern and ancient datasets) to characterize Guizhou populations’ genetic features comprehensively. We found that HM-speaking KLM separated from other newly genotyped Guizhou populations in the PCA and model-based ADMIXTURE results, but it clustered with other geographically different HM-speaking Miao and Yao people in Guizhou. All these HM-speaking people formed a specific genetic cline, which harbored more genetic components related to ancestral ancient southern East Asians as the estimated pairwise Fst genetic distances, outgroup f3-based shared genetic drift as well as the inferred admixture models in the ADMIXTURE, qpAdm, and qpGraph models. Most f4-results in the form f4(Guizhou populations, HM people; Southern East Asians, Mbuti) have statistically negative values, which directly supported that HM-speaking people harbored more genetic ancestry from southern indigenous people. Our reconstructed admixture models also suggested that the major ancestry of HM people originated from South China. Archaeological evidence has found wonderful Neolithic cultures from the Qujialing, Daxi, Shijiahe, and Lingjiatan sites, which suggested that Neolithic people from the middle Yangtze River had a relatively large effective population size and might be the major ancestral sources of modern HM people in this region. Multiple southward population movements from the YRB revealed that northern East Asians have significantly influenced the genetic landscape of spatiotemporally diverse southeastern East Asians and Southeast Asians (Yang et al., 2020). This work also identified the fact that northern East Asians influenced the gene pool of Guizhou Miao people, based on the modern population dataset. Some of Miao people harbored prominent ancestry from Han Chinese people, which might be the recent genetic influence from geographically close Han Chinese. A recent study on four Guizhou Miao populations confirmed that they possessed a specific genetic structure, according to our observations, and derived their major ancestry from Guangxi Gaohuahua people (He et al., 2023a). Liu et al. reported the genome-wide SNP data of 52 Sichuan Miao people and combined them with 13 geographically representative HM populations. They found obvious genetic substructures that existed in geographically distinct HM populations: one represented the HM-related cline, and the other possessed a strong affinity with Han Chinese (Liu et al., 2021a). Wang et al. comprehensively analyzed HM-speaking populations from South China and Southeast Asia and identified northern and southern HM subclades. They identified an HM-specific ancestry that was enriched in modern Hmong and found that modern HM speakers might originate from the Yungui Plateau or the surrounding regions in South China (Wang et al., 2022). In this study, we also identified this HM-specific ancestry related to the ancestor of Hmong people, and that KLM possessed a primary Hmong-related ancestry. Genetic research focused on Miao, Zhuang, and Han populations from Guangxi and Yunnan found that the proportion of HM-related ancestry decreased from west to east in HM-speaking groups, whereas the proportion of TK-related ancestry showed the opposite trend (Huang et al., 2022). Sampling more geographically diverse HM-speaking populations and conducting high-depth whole-genome sequencing are indispensable in future studies to promote our understanding of population origin, demographic processes, and adaptive history of HM speakers.
The patterns of population structure and admixture profile of TK people showed their extensively shared ancestry with HM and Han Chinese populations and revealed the genetic differentiation among ethnolinguistically different TK people. Clustering patterns inferred from PCA and ADMIXTURE showed that KLD had a closer genetic relationship with HM and other reference TK people. However, DZGL people harbored much ancestry related to Han Chinese populations. Our reconstructed admixture models also showed that the ancestral proportion of southern surrogates was higher in KLD but relatively low in DZGL. Generally, these results were consistent with our previous findings that TK‐speaking populations in northern Guizhou had more northern East Asian-related ancestry, while TK people in southern Guizhou possessed more southern East Asian-related ancestry (Wang et al., 2023). Historical records have documented that Baiyue or Minyue tribes have been widely distributed in South China and shared more cultural links with modern AN and TK people (Zhang et al., 2020). Our obtained admixture models supported that TK people experienced extensive admixture processes with incoming ancient southern East Asians. This work also identified the mixed features of TK people, which showed a strong genetic relationship between TK people and geographically close populations. These identified admixture landscapes and gene flow events suggested that Guizhou TK people have a mixed genetic landscape with ancestral sources from northern and southern ancient East Asians. Our previous study also identified the genetic differentiations among TK people from South China and Southeast Asia and between northern and southern Chinese inland TK people (Wang et al., 2023). Ren et al. genotyped six TK-speaking populations from Guizhou Province and found that Gelao and Dong people in the north of Guizhou harbored more Han-related ancestry than Dong, Zhuang, Bouyei, and Mulao people in the south. Our newly studied DZGL had a similar genetic profile to Gelao in northern Guizhou reported by Ren et al., and the newly studied KLD had a similar genetic profile to the previously reported Dong in southern Guizhou but not in northern Guizhou (Ren et al., 2022). Chen et al. sampled and genotyped Guizhou Maonan people and found their ancestry primarily from Guangxi historical people and minor ancestry from Northeast Asians (Chen et al., 2022). As observed in this study, we also identified extensive genetic admixture between TK and HM people. TB-speaking Tujia had a closer genetic relationship with geographically close Gelao people. Both apparent genetic influence received from the YRB farmers and Han Chinese was consistent with our findings observed in geographically diverse Guizhou Tujia and Han Chinese populations (He et al., 2021d; Wang et al., 2021b; Wang et al., 2023). Generally, we found three different genetic components in Guizhou populations, represented by TB, TK, and HM people, contributing to modern Guizhou people’s genetic landscape.
Conclusion
We used genome-wide SNP data from four newly collected populations and publicly available populations from TK, HM, and ST language families to characterize the genetic landscape of Guizhou populations. We identified fine-scale genetic structures in new Guizhou populations with two main different genetic profiles (Gelao/Tujia vs. Dong/Miao). The constructed admixture models showed that ethnolinguistically diverse Guizhou populations had differentiated genetic structures, suggesting that they had different ancestral sources and harbored complex admixture processes. Admixture models showed that HM-speaking KLM and TK-speaking KLD people shared more ancestry from ancestral ancient southern East Asians. DZGL and YHTJ people shared more genetic materials from ancestral northern East Asians. We also identified substantial genetic differentiations between geographically distinct TK people in northern and southern Guizhou. Generally, Guizhou populations harbored ancestry from northern sources from the YRB and southern sources from inland and island South China, reflecting multiple ancestral sources that contributed to the ethnolinguistic diversity of modern Guizhou populations.
Data availability statement
The original contributions presented in the study are publicly available. The raw allele frequency spectrum data are available at ZENODO (https://zenodo.org/) with accession number 7273078. The raw sequencing data derived from human samples have been deposited in the National Omics Data Encyclopedia (NODE, http://www.biosino.org/node) and can be accessed with accession number GVM000606. The acquisition and use of the data shall comply with the regulations of the People's Republic of China on the administration of human genetic resources. Requests for access to raw data can be directed to Guanglin He (Guanglinhescu@163.com) and Mengge Wang (Menggewang2021@163.com). Genetic analyses were conducted based on the computational codes of David Reich Lab and a public protocol to dissect population structure and migration history based on human genome variation data (Zhao et al., 2023).
Ethics statement
The Ethics Committees of the North Sichuan Medical College (No: 2021-A9) and West China Hospital of Sichuan University (2023-306) have approved this project. The patients/participants provided their written informed consent to participate in this study.
Author contributions
GH, MW, HS, PC, and CL conceived and designed the study. GH, MW, SD, QS, YL, JY, and RT made all analyses. GH and MW wrote the manuscript. HS, PC, and CL revised the paper. All authors contributed to the article and approved the submitted version.
Funding
This study was supported by the National Natural Science Foundation of China (NSFC 82202078).
Acknowledgments
We thank Prof. Etienne Patin at Institut Pasteur for sharing high-coverage whole-genome sequencing data from Taiwan Island, Island Southeast Asia and Oceania. We thank Prof. Wibhu Kutanan, Prof. Mark Stoneking, and Dr. Dang Liu for sharing genome-wide SNP data from Vietnam, Thailand, and Laos. We also thank all volunteers who participated in this project.
Conflict of interest
The authors declare that the research was conducted in the absence of any commercial or financial relationships that could be construed as a potential conflict of interest.
Publisher’s note
All claims expressed in this article are solely those of the authors and do not necessarily represent those of their affiliated organizations, or those of the publisher, the editors and the reviewers. Any product that may be evaluated in this article, or claim that may be made by its manufacturer, is not guaranteed or endorsed by the publisher.
Supplementary material
The Supplementary Material for this article can be found online at: https://www.frontiersin.org/articles/10.3389/fevo.2023.1235655/full#supplementary-material
References
Alexander D. H., Novembre J., Lange K. (2009). Fast model-based estimation of ancestry in unrelated individuals. Genome Res. 19 (9), 1655–1664. doi: 10.1101/gr.094052.109
Bergstrom A., McCarthy S. A., Hui R., Almarri M. A., Ayub Q., Danecek P., et al. (2020). Insights into human genetic variation and population history from 929 diverse genomes. Science 367 (6484), eaay5012. doi: 10.1126/science.aay5012
Browning B. L., Browning S. R. (2011). A fast, powerful method for detecting identity by descent. Am. J. Hum. Genet. 88 (2), 173–182. doi: 10.1016/j.ajhg.2011.01.010
Browning S. R., Browning B. L. (2015). Accurate non-parametric estimation of recent effective population size from segments of identity by descent. Am. J. Hum. Genet. 97 (3), 404–418. doi: 10.1016/j.ajhg.2015.07.012
Browning S. R., Browning B. L., Daviglus M. L., Durazo-Arvizu R. A., Schneiderman N., Kaplan R. C., et al. (2018). Ancestry-specific recent effective population size in the Americas. PloS Genet. 14 (5), e1007385. doi: 10.1371/journal.pgen.1007385
Byrska-Bishop M., Evani U. S., Zhao X., Basile A. O., Abel H. J., Regier A. A., et al. (2022). High-coverage whole-genome sequencing of the expanded 1000 Genomes Project cohort including 602 trios. Cell 185 (18), 3426–3440 e3419. doi: 10.1016/j.cell.2022.08.004
Cao Y., Li L., Xu M., Feng Z., Sun X., Lu J., et al. (2020). The ChinaMAP analytics of deep whole genome sequences in 10,588 individuals. Cell Res. 30 (9), 717–731. doi: 10.1038/s41422-020-0322-9
Chen J., He G., Ren Z., Wang Q., Liu Y., Zhang H., et al. (2021). Genomic insights into the admixture history of mongolic- and tungusic-speaking populations from Southwestern East Asia. Front. Genet. 12 (880). doi: 10.3389/fgene.2021.685285
Chen J., He G., Ren Z., Wang Q., Liu Y., Zhang H., et al. (2022). Fine-scale population admixture landscape of tai-kadai-speaking maonan in Southwest China inferred from genome-wide SNP data. Front. Genet. 13. doi: 10.3389/fgene.2022.815285
Choin J., Mendoza-Revilla J., Arauna L. R., Cuadros-Espinoza S., Cassar O., Larena M., et al. (2021). Genomic insights into population history and biological adaptation in Oceania. Nature 592 (7855), 583–589. doi: 10.1038/s41586-021-03236-5
Cong P. K., Bai W. Y., Li J. C., Yang M. Y., Khederzadeh S., Gai S. R., et al. (2022). Genomic analyses of 10,376 individuals in the Westlake BioBank for Chinese (WBBC) pilot project. Nat. Commun. 13 (1), 2939. doi: 10.1038/s41467-022-30526-x
Consortium H.P.-A.S., Abdulla M. A., Ahmed I., Assawamakin A., Bhak J., Brahmachari S. K., et al. (2009). Mapping human genetic diversity in Asia. Science 326 (5959), 1541–1545. doi: 10.1126/science.1177074
Delaneau O., Marchini J., Zagury J. F. (2011). A linear complexity phasing method for thousands of genomes. Nat. Methods 9 (2), 179–181. doi: 10.1038/nmeth.1785
Deng L., Zhang C., Yuan K., Gao Y., Pan Y., Ge X., et al. (2019). Prioritizing natural-selection signals from the deep-sequencing genomic data suggests multi-variant adaptation in Tibetan highlanders. Natl. Sci. Rev. 6 (6), 1201–1222. doi: 10.1093/nsr/nwz108
Guo J., Wang W., Zhao K., Li G., He G., Zhao J., et al. (2021). Genomic insights into Neolithic farming-related migrations in the junction of east and southeast Asia. Am. J. Biol. Anthropology 177 (2), 328–342. doi: 10.1002/ajpa.24434
Harper D., Mayhew B. (2007). China's South-west. Lonely Planet. Footscray, Vic. ; London. Available at: https://cir.nii.ac.jp/crid/1130282272108789632.
He G. L., Li Y. X., Wang M. G., Zou X., Yeh H. Y., Yang X. M., et al. (2021d). Fine-scale genetic structure of Tujia and central Han Chinese revealing massive genetic admixture under language borrowing. J. Systematics Evol. 59 (1), 1–20. doi: 10.1111/jse.12670
He G. L., Li Y. X., Zou X., Yeh H. Y., Tang R. K., Wang P. X., et al. (2023c). Northern gene flow into southeastern East Asians inferred from genome-wide array genotyping. J. Systematics Evol. 61 (1), 179–197. doi: 10.1111/jse.12826
He G., Liu J., Wang M., Zou X., Ming T., Zhu S., et al. (2021b). Massively parallel sequencing of 165 ancestry-informative SNPs and forensic biogeographical ancestry inference in three southern Chinese Sinitic/Tai-Kadai populations. Forensic Sci. Int. Genet. 52, 102475. doi: 10.1016/j.fsigen.2021.102475
He G. L., Wang M. G., Li Y. X., Zou X., Yeh H. Y., Tang R. K., et al. (2021a). Fine-scale north-to-south genetic admixture profile in Shaanxi Han Chinese revealed by genome-wide demographic history reconstruction. J. Systematics Evol. 60 (4), 955–972. doi: 10.1111/jse.12715
He G., Wang J., Yang L., Duan S., Sun Q., Li Y., et al. (2023a). Genome-wide allele and haplotype-sharing patterns suggested one unique Hmong-Mein-related lineage and biological adaptation history in Southwest China. Hum. Genomics 17 (1), 3. doi: 10.1186/s40246-023-00452-0
He G., Wang Z., Zou X., Wang M., Liu J., Wang S., et al. (2019). Tai-Kadai-speaking Gelao population: Forensic features, genetic diversity and population structure. Forensic Sci. Int. Genet. 40, e231–e239. doi: 10.1016/j.fsigen.2019.03.013
He G. L., Wang M. G., Zou X., Yeh H. Y., Liu C. H., Liu C., et al. (2023d). Extensive ethnolinguistic diversity at the crossroads of North China and South Siberia reflects multiple sources of genetic diversity. J. Systematics Evol. 61 (1), 230–250. doi: 10.1111/jse.12827
He G., Yao H., Sun Q., Duan S., Tang R., Chen J., et al. (2023b). Whole-genome sequencing of ethnolinguistic diverse northwestern Chinese Hexi Corridor people from the 10K_CPGDP project suggested the differentiated East-West genetic admixture along the Silk Road and their biological adaptations. bioRxiv. doi: 10.1101/2023.02.26.530053
He G., Zhang Y., Wei L.-H., Wang M., Yang X., Guo J., et al. (2021c). The genomic formation of Tanka people, an isolated “Gypsies in water” in the coastal region of Southeast China. Am. J. Biol. Anthropology. 178 (1), 154–170. doi: 10.1101/2021.07.18.452867
He G. L., Zhang Y. H., Wei L. H., Wang M. G., Yang X. M., Guo J. X., et al. (2022). The genomic formation of Tanka people, an isolated "gypsies in water" in the coastal region of Southeast China. Am. J. Biol. Anthropology 178 (1), 154–170. doi: 10.1002/ajpa.24495
Hellenthal G., Busby G. B. J., Band G., Wilson J. F., Capelli C., Falush D., et al. (2014). A genetic atlas of human admixture history. Science 343 (6172), 747–751. doi: 10.1126/science.1243518
Hu W., Hao Z., Du P., Di Vincenzo F., Manzi G., Cui J., et al. (2023). Genomic inference of a severe human bottleneck during the Early to Middle Pleistocene transition. Science 381 (6661), 979–984. doi: 10.1126/science.abq7487
Huang X. F., Xia Z. Y., Bin X. Y., He G. L., Guo J. X., Adnan A., et al. (2022). Genomic insights into the demographic history of the Southern Chinese. Front. Ecol. Evol. 10. doi: 10.3389/fevo.2022.853391
Huerta-Sanchez E., Jin X., Bianba Z., Peter B. M., Vinckenbosch N., Liang Y., et al. (2014). Altitude adaptation in Tibetans caused by introgression of Denisovan-like DNA. Nature 512 (7513), 194–197. doi: 10.1038/nature13408
Jeong C., Balanovsky O., Lukianova E., Kahbatkyzy N., Flegontov P., Zaporozhchenko V., et al. (2019). The genetic history of admixture across inner Eurasia. Nat. Ecol. Evol. 3 (6), 966–976. doi: 10.1038/s41559-019-0878-2
Kutanan W., Liu D., Kampuansai J., Srikummool M., Srithawong S., Shoocongdej R., et al. (2021). Reconstructing the human genetic history of Mainland Southeast Asia: insights from genome-wide data from Thailand and Laos. Mol. Biol. Evol. 38 (8), 3459–3477. doi: 10.1093/molbev/msab124
Lawson D. J., Hellenthal G., Myers S., Falush D. (2012). Inference of population structure using dense haplotype data. PloS Genet. 8 (1), e1002453. doi: 10.1371/journal.pgen.1002453
Liu D., Duong N. T., Ton N. D., Van Phong N., Pakendorf B., Van Hai N., et al. (2020). Extensive ethnolinguistic diversity in Vietnam reflects multiple sources of genetic diversity. Mol. Biol. Evol. 37 (9), 2503–2519. doi: 10.1093/molbev/msaa099
Liu Y., Xie J., Wang M., Liu C., Zhu J., Zou X., et al. (2021a). Genomic insights into the population history and biological adaptation of southwestern chinese hmong-mien people. Front. Genet. 12. doi: 10.3389/fgene.2021.815160
Liu Y., Yang J., Li Y., Tang R., Yuan D., Wang Y., et al. (2021b). Significant east asian affinity of the sichuan hui genomic structure suggests the predominance of the cultural diffusion model in the genetic formation process. Front. Genet. 12 (834). doi: 10.3389/fgene.2021.626710
Lou H., Lu Y., Lu D., Fu R., Wang X., Feng Q., et al. (2015). A 3.4-kb Copy-Number Deletion near EPAS1 Is Significantly Enriched in High-Altitude Tibetans but Absent from the Denisovan Sequence. Am. J. Hum. Genet. 97 (1), 54–66. doi: 10.1016/j.ajhg.2015.05.005
Lu J., Zhang H., Ren Z., Wang Q., Liu Y., Li Y., et al. (2020). Genome-wide analysis of unrecognised ethnic group Chuanqing people revealing a close affinity with Southern Han Chinese. Ann. Hum. Biol. 47 (5), 465–471. doi: 10.1080/03014460.2020.1782470
Ma X., Yang W., Gao Y., Pan Y., Lu Y., Chen H., et al. (2021). Genetic origins and sex-biased admixture of the huis. Mol. Biol. Evol. 38 (9), 3804–3819. doi: 10.1093/molbev/msab158
Maier R., Flegontov P., Flegontova O., Isildak U., Changmai P., Reich D. (2023). On the limits of fitting complex models of population history to f-statistics. Elife 12, e85492. doi: 10.7554/eLife.85492
Mallick S., Micco A., Mah M., Ringbauer H., Lazaridis I., Olalde I., et al. (2023). The Allen Ancient DNA Resource (AADR): A curated compendium of ancient human genomes. bioRxiv. doi: 10.1101/2023.04.06.535797
Mao X., Zhang H., Qiao S., Liu Y., Chang F., Xie P., et al. (2021). The deep population history of northern East Asia from the Late Pleistocene to the Holocene. Cell 184 (12), 3256–3266 e3213. doi: 10.1016/j.cell.2021.04.040
McQuillan M. A., Zhang C., Tishkoff S. A., Platt A. (2020). The importance of including ethnically diverse populations in studies of quantitative trait evolution. Curr. Opin. Genet. Dev. 62, 30–35. doi: 10.1016/j.gde.2020.05.037
Mengge W., Guanglin H., Yongdong S., Shouyu W., Xing Z., Jing L., et al. (2020). Massively parallel sequencing of mitogenome sequences reveals the forensic features and maternal diversity of tai-kadai-speaking hlai islanders. Forensic Sci. Int. Genet. 47, 102303. doi: 10.1016/j.fsigen.2020.102303
Minh B. Q., Schmidt H. A., Chernomor O., Schrempf D., Woodhams M. D., von Haeseler A., et al. (2020). IQ-TREE 2: new models and efficient methods for phylogenetic inference in the genomic era. Mol. Biol. Evol. 37 (5), 1530–1534. doi: 10.1093/molbev/msaa015
Ning C., Li T., Wang K., Zhang F., Li T., Wu X., et al. (2020). Ancient genomes from northern China suggest links between subsistence changes and human migration. Nat. Commun. 11 (1), 2700. doi: 10.1038/s41467-020-16557-2
Pan Y., Zhang C., Lu Y., Ning Z., Lu D., Gao Y., et al. (2022). Genomic diversity and post-admixture adaptation in the Uyghurs. Natl. Sci. Rev. 9 (3), nwab124. doi: 10.1093/nsr/nwab124
Patterson N., Moorjani P., Luo Y., Mallick S., Rohland N., Zhan Y., et al. (2012). Ancient admixture in human history. Genetics 192 (3), 1065–1093. doi: 10.1534/genetics.112.145037
Patterson N., Price A. L., Reich D. (2006). Population structure and eigenanalysis. PloS Genet. 2 (12), e190. doi: 10.1371/journal.pgen.0020190
Pickrell J. K., Pritchard J. K. (2012). Inference of population splits and mixtures from genome-wide allele frequency data. PloS Genet. 8 (11), e1002967. doi: 10.1371/journal.pgen.1002967
Qi X., Cui C., Peng Y., Zhang X., Yang Z., Zhong H., et al. (2013). Genetic evidence of paleolithic colonization and neolithic expansion of modern humans on the tibetan plateau. Mol. Biol. Evol. 30 (8), 1761–1778. doi: 10.1093/molbev/mst093
Ren Z., Yang M., Jin X., Wang Q., Liu Y., Zhang H., et al. (2022). Genetic substructure of Guizhou Tai-Kadai-speaking people inferred from genome-wide single nucleotide polymorphisms data. Front. Ecol. Evol. 10. doi: 10.3389/fevo.2022.995783
Schiffels S., Durbin R. (2014). Inferring human population size and separation history from multiple genome sequences. Nat. Genet. 46 (8), 919–925. doi: 10.1038/ng.3015
Sirugo G., Williams S. M., Tishkoff S. A. (2019). The missing diversity in human genetic studies. Cell 177 (1), 26–31. doi: 10.1016/j.cell.2019.02.048
Wang M. G., He G. L., Zou X., Chen P. Y., Wang Z., Tang R. K., et al. (2023). Reconstructing the genetic admixture history of Tai-Kadai and Sinitic people: Insights from genome-wide SNP data from South China. J. Systematics Evol. 61 (1), 157–178. doi: 10.1111/jse.12825
Wang T., Wang W., Xie G., Li Z., Fan X., Yang Q., et al. (2021c). Human population history at the crossroads of East and Southeast Asia since 11,000 years ago. Cell 184 (14), 3829–3841 e3821. doi: 10.1016/j.cell.2021.05.018
Wang C. C., Yeh H. Y., Popov A. N., Zhang H. Q., Matsumura H., Sirak K., et al. (2021a). Genomic insights into the formation of human populations in East Asia. Nature 591 (7850), 413–419. doi: 10.1038/s41586-021-03336-2
Wang M., Yuan D., Zou X., Wang Z., Yeh H. Y., Liu J., et al. (2021b). Fine-scale genetic structure and natural selection signatures of southwestern hans inferred from patterns of genome-wide allele, haplotype, and haplogroup lineages. Front. Genet. 12. doi: 10.3389/fgene.2021.727821
Wang Y., Zou X., Wang M., Yuan D., Yang L., Zeng Y., et al. (2022). The genomic history of southwestern Chinese populations demonstrated massive population migration and admixture among proto-Hmong-Mien speakers and incoming migrants. Mol. Genet. Genomics 297 (1), 241–262. doi: 10.1007/s00438-021-01837-3
Weir B. S., Cockerham C. C. (1984). Estimating F-statistics for the analysis of population structure. Evolution 38 (6), 1358–1370. doi: 10.1111/j.1558-5646.1984.tb05657.x
Yang M. A., Fan X., Sun B., Chen C., Lang J., Ko Y. C., et al. (2020). Ancient DNA indicates human population shifts and admixture in northern and southern China. Science 369 (6501), 282–288. doi: 10.1126/science.aba0909
Yao H., Wang M., Zou X., Li Y., Yang X., Li A., et al. (2021). New insights into the fine-scale history of western-eastern admixture of the northwestern Chinese population in the Hexi Corridor via genome-wide genetic legacy. Mol. Genet. Genomics 296 (3), 631–651. doi: 10.1007/s00438-021-01767-0
Yi X., Liang Y., Huerta-Sanchez E., Jin X., Cuo Z. X., Pool J. E., et al. (2010). Sequencing of 50 human exomes reveals adaptation to high altitude. Science 329 (5987), 75–78. doi: 10.1126/science.1190371
Zhang H., He G., Guo J., Ren Z., Zhang H., Wang Q., et al. (2019). Genetic diversity, structure and forensic characteristics of Hmong-Mien-speaking Miao revealed by autosomal insertion/deletion markers. Mol. Genet. Genomics 294 (6), 1487–1498. doi: 10.1007/s00438-019-01591-7
Zhang X., He G., Li W., Wang Y., Li X., Chen Y., et al. (2021b). Genomic insight into the population admixture history of tungusic-speaking manchu people in Northeast China. Front. Genet. 12 (1761). doi: 10.3389/fgene.2021.754492
Zhang X., Li C., Zhou Y., Huang J., Yu T., Liu X., et al. (2020). A matrilineal genetic perspective of hanging coffin custom in Southern China and Northern Thailand. iScience 23 (4), 101032. doi: 10.1016/j.isci.2020.101032
Zhang C., Lu Y., Feng Q., Wang X., Lou H., Liu J., et al. (2017). Differentiated demographic histories and local adaptations between Sherpas and Tibetans. Genome Biol. 18 (1), 115. doi: 10.1186/s13059-017-1242-y
Zhang P., Luo H., Li Y., Wang Y., Wang J., Zheng Y., et al. (2021a). NyuWa Genome resource: A deep whole-genome sequencing-based variation profile and reference panel for the Chinese population. Cell Rep. 37 (7), 110017. doi: 10.1016/j.celrep.2021.110017
Keywords: ethnolinguistic diversity, genome-wide SNP, genetic diversity, admixture events, evolutionary history
Citation: Wang M, Duan S, Sun Q, Liu Y, Tang R, Yang J, Chen P, Liu C, Sun H and He G (2023) The complex genetic landscape of southwestern Chinese populations contributed to their extensive ethnolinguistic diversity. Front. Ecol. Evol. 11:1235655. doi: 10.3389/fevo.2023.1235655
Received: 06 June 2023; Accepted: 19 September 2023;
Published: 11 October 2023.
Edited by:
Marshall Abrams, University of Alabama at Birmingham, United StatesReviewed by:
Eaaswarkhanth Muthukrishnan, Georgia Institute of Technology, United StatesDang Liu, Institut Pasteur, France
Copyright © 2023 Wang, Duan, Sun, Liu, Tang, Yang, Chen, Liu, Sun and He. This is an open-access article distributed under the terms of the Creative Commons Attribution License (CC BY). The use, distribution or reproduction in other forums is permitted, provided the original author(s) and the copyright owner(s) are credited and that the original publication in this journal is cited, in accordance with accepted academic practice. No use, distribution or reproduction is permitted which does not comply with these terms.
*Correspondence: Mengge Wang, bWVuZ2dld2FuZzIwMjFAMTYzLmNvbQ==; Chao Liu, bGl1Y2hhb2d6ZkAxNjMuY29t; Hongyu Sun, c3VuaHlAbWFpbC5zeXN1LmVkdS5jbg==; Guanglin He, R3VhbmdsaW5oZXNjdUAxNjMuY29t
†These authors have contributed equally to this work and share first authorship