- U.S. Geological Survey, Western Ecological Research Center, Dixon Field Station, Dixon, CA, United States
Introduction: Habitat selection analyses provide a window into the perceived value of habitats by animals and how those perceptions compare with other animals, change across time, or change in relation to availability (termed functional responses). Habitat selection analysis and functional responses can be used to develop strategies to avoid habitat limitations, guide habitat management, and set attainable conservation goals. GPS relocations of marked animals are the principal data used in habitat selection analysis. The accuracy and frequency with which tracking devices collect data are increasing and may result in non-stationary point processes that result from latent behaviors previously unidentifiable in sparse data.
Methods: We investigated non-stationary step length distributions and integrated a two-mixture model of animal movement with step selection analysis to identify patterns of activity among four species of co-occurring waterfowl that winter in the Central Valley of California, United States. We evaluated relative strength of selection and compared functional responses across a range of habitat types for two goose and two dabbling duck species.
Results: Goose species (greater white-fronted goose [Anser albifrons] and lesser snow goose [Anser caerulescens caerulescens]) used habitats similarly and displayed similar functional responses with habitat availability. Northern pintail (Anas acuta) displayed functional responses for habitats that provided primary food resources and sanctuary from hunting that were more similar to geese than to mallard (Anas platyrhynchos), which expressed a more generalist pattern of habitat selection.
Discussion: Our results define conditions where food resource competition between geese and ducks could operate, which indicate that some species may be more impacted than others. Specifically, early season food limitation may manifest more strongly in snow geese due to longer movements and stronger functional response with rice availability. Late season limitations may manifest in northern pintail, which remain reliant on rice later but may not be reflected in habitat selection patterns due to a consistent functional response with rice availability. We show that multiple movement processes present in high-resolution data can be used to obtain a variety of information about animal behavior and that subsequent step selection analyses may demonstrate unique functional responses relative to alternate habitat selection methods that warrant additional investigation.
Introduction
Habitat selection analysis is a generic term for many approaches that relate use of a habitat/resource and the availability of that habitat (Neu et al., 1974; Johnson, 1980; Manly et al., 2002; Johnson et al., 2008; Thurfjell et al., 2014). Patterns of selection and avoidance of habitats provide information on individual resource requirements (Osborn et al., 2017) and comparative indicators of habitat value (Avgar et al., 2016), inform population abundance estimates (Boyce et al., 2016), and more broadly reflect levels of fitness across individuals (Northrup et al., 2022). Habitat selection patterns may change due to changes in relative use among multiple habitat types or changes in availability of habitat types. Changes in habitat use may result from internal drivers such as changing life history needs (Groff et al., 2017) or dietary requirements (Mitchell et al., 2020). Changes in habitat availability involves external factors such as resource phenology (Resano-Mayor et al., 2019), environmental disturbance (Brussee et al., 2022), or anthropogenic change (Knopff et al., 2014). Comparisons of resource selection patterns across individuals, species, or regions can identify overlap and potential avenues for competition (Rosenzweig, 1981) and identify density-dependent processes (Morris, 1989). Some methods of quantifying habitat selection allow for the identification of individual patterns of selection (Leclerc et al., 2016; Muff et al., 2020) or inclusion of random effects (Gillies et al., 2006; Duchesne et al., 2010) to quantify individual patterns of selection and assess functional responses of use or selection relative to habitat availability (Mysterud and Ims, 1998; Holbrook et al., 2019).
Used habitat measures that comprise part of habitat selection analyses can be obtained from surveys and occupancy information but is more commonly obtained with repeated observations of the same individual(s) through time and typically using tracking devices (Manly et al., 2002). These tracking devices provide increasingly complete and accurate spatial information about animal locations (Kays et al., 2015). Increasing data frequency and accuracy are also likely to manifest animal movement patterns or activities that were not apparent with coarser data (Nathan et al., 2022). This can allow the identification of multiple behavior states or constituent activities and context-dependent analysis of resource use (Patterson et al., 2009; Bergen et al., 2022; Overton et al., 2022). It is often crucial to identify constituent behaviors within individual tracks since non-stationary processes (a time series that has statistical properties, metrics, or moments [such as mean or variance] that vary in time) resulting in imbalanced movement or habitat selection can bias selection analyses when quantifying use (Osborne et al., 2007) or identifying available habitats (Aarts et al., 2013; Pay et al., 2022). Methods to reduce the impact of non-stationary processes include data thinning (Schabenberger and Gotway, 2005), path segmentation (Patterson et al., 2009; Nathan et al., 2022), and autocorrelation weighting (Alston et al., 2023).
Across the globe, bird species often co-occur and concentrate in regions with favorable seasonal climatic conditions and where food or other resources occur predictably, and their concentrations are high. These locations, such as the Baltic Sea, may attract millions of birds during the winter, often substantial proportions of global or flyway populations, into regions where anthropogenic activities may both positively or negatively affect habitat quality, resource values, and ultimately the carrying capacity of the landscape (Skov et al., 2011; Marchowski and Leitner, 2019). Where bird concentrations are exceptionally high, such as California’s Central Valley and Poland’s Odra Estuary, understanding space use and resource selection patterns are a principal component of effective land management, setting conservation targets and developing habitat restoration and enhancement activities (Marchowski et al., 2015; Marchowski and Leitner, 2019; CVJV, 2020). Increasingly abundant goose populations (~2 million, Olson, 2022) in the Central Valley that are overpopulation targets, co-occur with 4.76 to 6.27 million dabbling ducks (Fleming et al., 2019) that are largely below conservation targets (CVJV, 2020). In this region, dabbling ducks and geese utilize similar food resources, occupy the region during the same periods, and many times restrict use to limited, often publicly owned, lands where they are not subject to hunting (sanctuary) and can roost during daylight hours without disturbance. Owing to this apparent niche overlap, habitat selection patterns to identify resource use and needs in this region are necessary for public area habitat management plans. Furthermore, comparison of selection patterns across species is needed to assess the potential for competition between geese and ducks and to parameterize landscape energetics models and estimate carrying capacity (CVJV, 2020, Miller et al., 2014; Williams et al., 2014; Petrie et al., 2016). Understanding functional responses in habitat selection patterns relative to habitat availability can guide conservation objectives and landscape planning in a region where drought is increasingly common (Diffenbaugh et al., 2015) and affects agricultural and water management practices that determine food accessibility and how the landscape provisions resources for wildlife (Petrie et al., 2016; Pathak et al., 2018).
Here, we present analyses of movement activity and resource selection for four waterfowl species that winter in the Central Valley. These include two goose species, the lesser snow goose (LSGO, Anser caerulescens caerulescens) and greater white-fronted goose (GWFG, Anser albifrons), which was represented by two sympatric subspecies, Pacific greater white-fronted goose (A. a. frontalis) and Tule goose (A. a. elegans). We also tracked two species of dabbling duck including the migratory northern pintail (NOPI, Anas acuta) and mallard (MALL, Anas platyrhynchos), which include individuals that both breed locally and migrate. Snow geese are the most abundant goose in the region, and northern pintails are the most abundant duck (Olson, 2022). Agriculture is the principal land use with rice providing a major food resource for waterfowl (Miller et al., 2010; CVJV, 2020). Corn is more limited in availability but present and may be locally abundant. Rainfall is the principal form of precipitation and occurs mostly from November to March. Following winter rains and coincident with increasing temperatures beginning in January, both goose species may switch diet from principally seeds to emerging grass (Ely and Raveling, 2011). Late in winter, ducks will also switch from principally seeds in their diet to also include aquatic invertebrates (Euliss and Harris, 1987; Miller, 1987). Persistent drought has affected the region in recent years and resulted in fallowing of agricultural lands and reduction in potential food supplies. These variable conditions allowed us to quantify resource selection patterns and compare functional responses across a variety of habitat types and among species with different ecological traits.
Materials and methods
Waterfowl were captured and fit with GPS logging transmitters using multiple methods between 2015 and 2022 at locations in California, Oregon, and within the arctic (McDuie et al., 2019; Casazza et al., 2021). Mallards were captured in the fall via rocket nets, box nets, or using dip nets from airboats while night lighting. Mallards were also captured during spring using baited swim-in traps and dip nets while incubating. Most pintails were captured via rocket nets in the fall. Some pintails were captured in the spring using rocket nets or swim-in traps. Greater white-fronted geese and lesser snow geese were captured using rocket nets in the fall. Some lesser snow geese were also caught in arctic molting regions using walk-in corral traps. Snow goose captures in the arctic were associated with breeding colonies on Wrangel Island, Russia; Banks Island, Northwest Territories, Canada; and colonies at both the Colville and Ikpikpuk River deltas on the North Slope of Alaska. Ducks were fit with various transmitter packages ranging from 10 to 25 g and affixed with backpack-style harnesses using TeflonTM ribbon or nylon-neoprene elastic harness material. Geese were fit with 35- to 42-mm-diameter collar transmitters. Transmitters collected GPS data and metadata on transmitter performance (e.g., battery, charging current, and temperature) and most also collected accelerometry data. Data collection frequency varied from every 15 min to once per day corresponding to battery voltage capacity in order to maximize the duration of tracking. All data were transmitted once or twice per day via the cellular (GSM) network when in cellular range and stored on-board when out of range. Both GPS locations and secondary data such as temperature and accelerometry were used to identify mortality events and suspend active deployment periods. Data used for analysis were limited to the Central Valley Joint Venture planning region in central California and occurred primarily in the Sacramento Valley and Sacramento–San Joaquin Delta. Telemetry data were subset to hourly GPS collection intervals with continual tracking for at least 3 days to remove any bias in selection or movement resulting from intermittent GPS collection when battery levels were low (e.g., hourly data only available during daylight hours). Data periods for analysis extended from October through April.
We conducted a step selection analysis (SSA) following the methods of Fieberg et al. (2021). We used 100 replicate movement steps for each observed movement with a circular uniform turning angle distribution. We evaluated random step length patterns using two commonly used parametric distributions, exponential and gamma, using the “amt” package (Signer et al., 2019). However, we anticipated that hourly movement data of waterfowl across long time frames would reflect a non-stationary movement process with at least two states of activity, inactive and moving. Strictly speaking, the “inactive” movement process includes multiple behaviors such as loafing, swimming, or walking that consistently reflect short and slow activity. Similarly, the “moving” state reflects both rapid and long movements indicative of flight, though also some overlapping behaviors such as directional foraging or gleaning that entail rapid, but ground-based, movements. To account for non-stationarity movement processes, we estimated step lengths using a two-mixture log-normal distribution that provides mean and variance parameters as well as mixing probabilities for two distributions of step lengths and the ability to randomly sample from estimated distributions (package “mixtools”, Benaglia et al., 2009). We estimated one two-mixture distribution using 50,000 randomly selected movements from all four species to identify inactive and moving behaviors and allow comparisons of movement activity among four waterfowl species using the same underlying model of movement. For SSA, we first estimated species-specific two-mixture movement distributions, then subset locations to those identified as moving, and finally produced random steps using the mixture component that represented movement. Inactive step distribution median step length was approximately nominal GPS location error (~20 m) and are largely non-informative for habitat selection (all 100 random steps generally were within the same habitat patch/landcover type as the observed use step). Moving step distribution, on the other hand, reflected both within-patch movements (e.g., while actively foraging) and between-patch movements (e.g., large-scale disturbance and flights from sanctuary to foraging patches).
Inactive steps were used to describe temporal patterns of mobility, the inverse of the probability of inactive movements, both within a single day and across the winter season when birds used the Central Valley (October through April). Mobility was also used to quantify the probability of moving when a location occurred within different landcover types. A linear trend through the season in mobility was calculated using logistic regression that included tests for differences in mobility and trends among species. Circadian (midnight to midnight) patterns in mobility for each species were estimated using a high-order (sixth-order) polynomial linear model to account for complex movement dynamics during crepuscular periods with significance of the trends assessed with t-statistics. Difference in patterns among species was tested using an ANOVA. Interpretation of differences was assessed graphically by contrasting trends including confidence bands among species. The log-odds of mobility within different habitats were assessed using an ANOVA separately for each species. Statistical tests were performed using Tableau Desktop software v 2022.4 (©Salesforce, San Francisco, CA).
Habitat types used in analyses were delineated for each agricultural year (May 1 through April) using the National Agricultural Statistics Service Crop Data Layer (CDL; Boryan et al., 2011). Crop and other landcover categories were condensed to six classes. Two represented primary food resources used by both ducks and geese in California (“corn” and “rice”). Two habitat types represented secondary food resources used predominantly by a single taxon in California, namely, agricultural fields and crops, including pasture, alfalfa, and idle cropland (hereafter, “PAI”) that produce grass in late winter consumed by geese, and various seasonal, permanent, and semi-permanent “wetlands” with food resources (seeds and invertebrates) consumed by ducks. CDL classification of wetlands is complicated by highly heterogenous vegetation and water extents throughout the year, which results in lower accuracy than other crop types (Lark et al., 2021). Therefore, we supplemented wetland classification using the National Wetlands Inventory (USFWS, 2018) and, within the Sacramento–San Joaquin River Delta, using the California Aquatic Resource Inventory (SFEI, 2022). This combined wetland map was produced by converting CDL raster layers to vector (polygon) representation and isolating the resulting wetland polygons. These polygons were merging to the vector layers provided in the National Wetlands Inventory or California Aquatic Resource Inventory to produce a complete wetland only polygon layer. This combined wetland-only layer was then merged with the original CDL polygons to replace wetlands that were unidentified in the CDL layers. Two non-habitat classes were also included (“urban” and “other” including various landcover and crop types). Lastly, we established a seventh habitat class (“protected” lands) to represent land parcels conferring some measure of habitat protection (GAP Status 1 and 2) using the California Protected Area Database (GreenInfo Network, 2022). Within the region used by waterfowl, this class contained state, federal, and non-governmental ownership that typically provide some measure of spatial refuge from hunting pressure but also consisted of a variety of landcover types. The final map containing seven habitat classes was extracted to points. All geospatial processing was performed in R using packages “sf”, “rgee”, and “rgdal” (Pebesma, 2018; Aybar, 2022; Bivand et al., 2022).
Step selection functions were developed for individual birds during both day and night and for two winter periods (early season, October to January 15, and late season, January 15 to April). We chose to estimate selection for each individual separately during day and night because it is known that foraging activity and, during hunting season, the need for refuge differ between taxa during both day and night (McNeil et al., 1992; McDuie et al., 2021). We divided the wintering period in half to account for seasonal diet shifts, as temperatures warm, to a greater reliance on newly emerged grasses for geese and to invertebrates for ducks (Euliss and Harris, 1987; Miller, 1987; Ely and Raveling, 2011). Although there are other factors that may influence habitat selection such as weather-induced and anthropogenic flooding, intensity of hunting pressure, or other disturbance factors (Mott, 2022), our ability to capture those impacts as habitat features was limited. Therefore, we rely on data collected across a number of years and under varying environmental conditions to provide a generalizable interpretation of resource selection across habitat types reflecting resources that are already recognized among regional conservation efforts (CVJV, 2020). Since our goal was to evaluate the functional relationships between relative importance of a habitat and the availability of that habitat, we ran step selection models for each combination of individual, photoperiod, and seasonal periods that were developed independently for each habitat type. We only considered one habitat at a time because interpretation of beta estimates for categorical covariates in step selection models is the relative selection of the covariate versus all categories not in the model. Also, including multiple covariates would incorporate habitat dependences that obscure the functional response of habitat selection with habitat availability that we were investigating. Development and interpretation of a full habitat selection model would of course benefit from a more complex model structure with consideration of statistical processes such as multicollinearity, perhaps with assessments of temporal autocorrelation of within individual random variation. Such approaches are worthwhile but appear unable to evaluate functional response for the relative strength of selection (RSS) estimates due to dependence among parameter estimates (Fieberg et al., 2021).
We compiled all RSS estimates produced for each habitat type in a conditional logit model run for each combination of individual, photoperiod, and seasonal period and contrasted the average availability of the habitat with those RSS estimates to investigate functional responses. When either use or availability of a habitat class is very low, sampling may fail to produce adequate samples for those classes resulting in highly variable and potentially biased estimates of selection (Northrup et al., 2022). For this reason, we assessed the RSS estimates, which are provided by the beta parameter estimate in each step selection model (Fieberg et al., 2021) and removed four extreme RSS estimates, each of which had large standard errors and high leverage on mean parameter estimates (Figure 1). Functional relationships between mean RSS and average availability of each habitat were investigated qualitatively using previously identified functional response patterns (Holbrook et al., 2019) but patterns were not formally subjected to statistical testing. We interpreted these functional relationship patterns as indications of how habitat value (relative to other habitats) changes as the availability of that habitat changes.
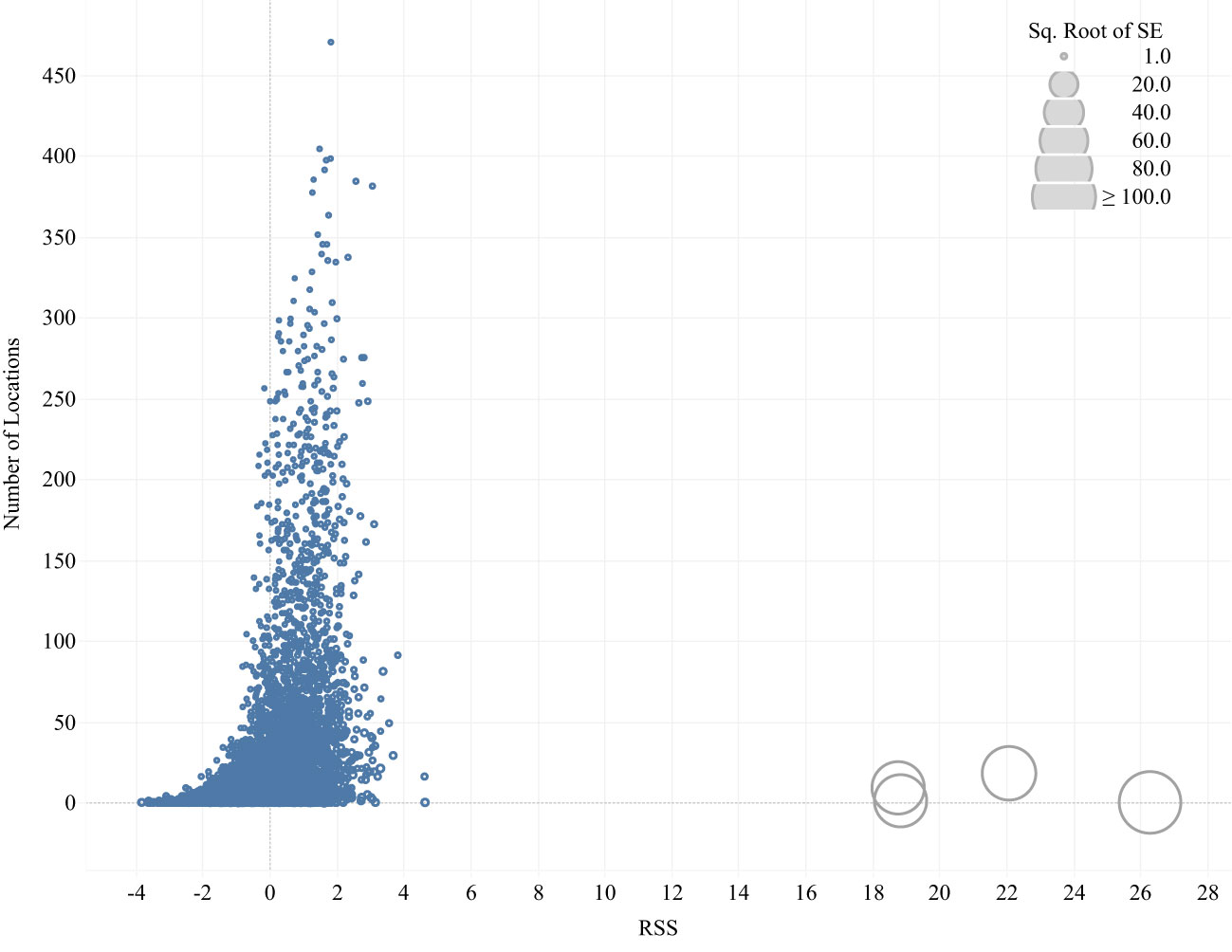
Figure 1 Distribution of relative strength of selection (RSS) estimates for seven habitat types by four species of waterfowl during day and night periods in two seasons (early and late winter). Symbol size is scaled to the square root of estimated RSS standard errors. Four RSS estimates with extreme values and high uncertainty were removed from subsequent analyses (gray symbols).
Results
A total of 691 individual waterfowl were tracked within California’s Central Valley between April 2015 and January 2023, which provided data across 971 individual bird-years and produced over 3.5 million GPS locations at intervals ranging from once per day to every minute. Our final dataset filtered GPS locations to the wintering period (October through April) and to continuous hourly intervals lasting a minimum of 3 days, resulting in 169,716 locations (Table 1; Overton and Casazza, 2023). Random step lengths estimated using both exponential and gamma distributions failed to capture the clear bi-model pattern in the empirical distribution of waterfowl movements, but the two-mixture model estimated the log-normal movement distribution accurately (Figure 2) and was used in subsequent analyses (Overton and Casazza, 2023). A step length distribution derived equally across all species (Table 2) produced estimates for one component that was approximately equal to the GPS location error among our tags (median = 21.1 m) and 69.1% of all step lengths reflected this “inactive” movement process. The remaining 30.9% of locations reflected “moving” activity with a median movement of 504 m (95% CI: 158–16,026 m). Each species demonstrated different relative proportions of these two movement activities. Each duck species indicated greater proportion of inactive movements (>80%) than geese (<70%), particularly lesser snow geese, among which just over half of movements were inactive (Table 2). Species-specific differences in movement activity were accentuated when step length distributions were calculated independently for each species, which indicated shorter median movements among geese and larger among ducks (Table 2).

Table 1 Data from greater white-fronted geese (GWFG), lesser snow geese (LSGO), mallard (MALL), and northern pintail (NOPI) fit with GPS tracking units and occurring within California between April 2015 through January 2023 including number of marked individuals, number of unique annual periods extending from May through April (bird-years), and total number of locations collected at intervals ranging from once per minute to once per day.
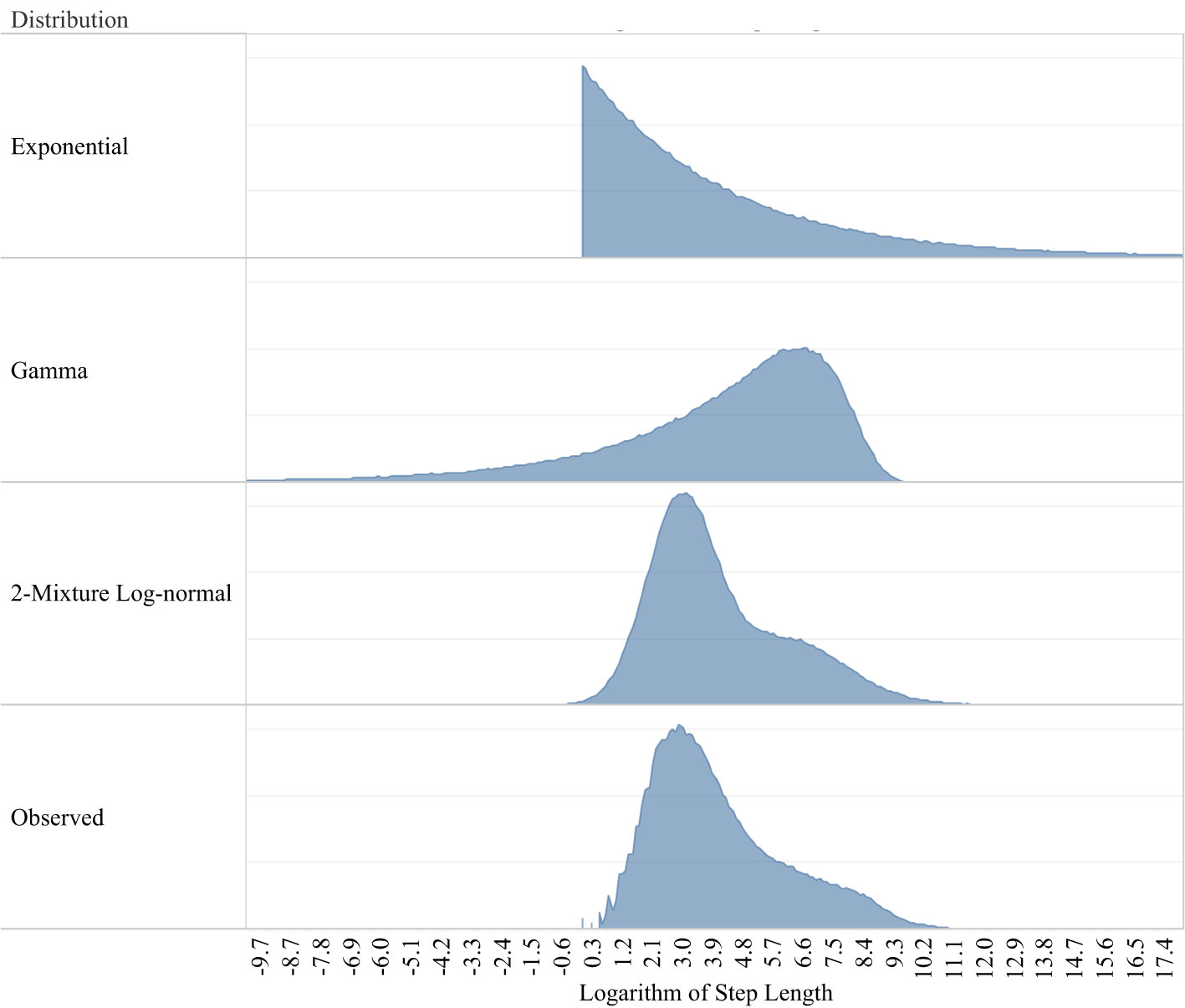
Figure 2 Two commonly used step length distributions used to define available habitat in step selection models, exponential and gamma, poorly approximated the empirical distribution of step lengths represented in waterfowl movement data. An alternative step length distribution using a two-mixture log-normal distribution produced a more accurate approximation of the observed data.
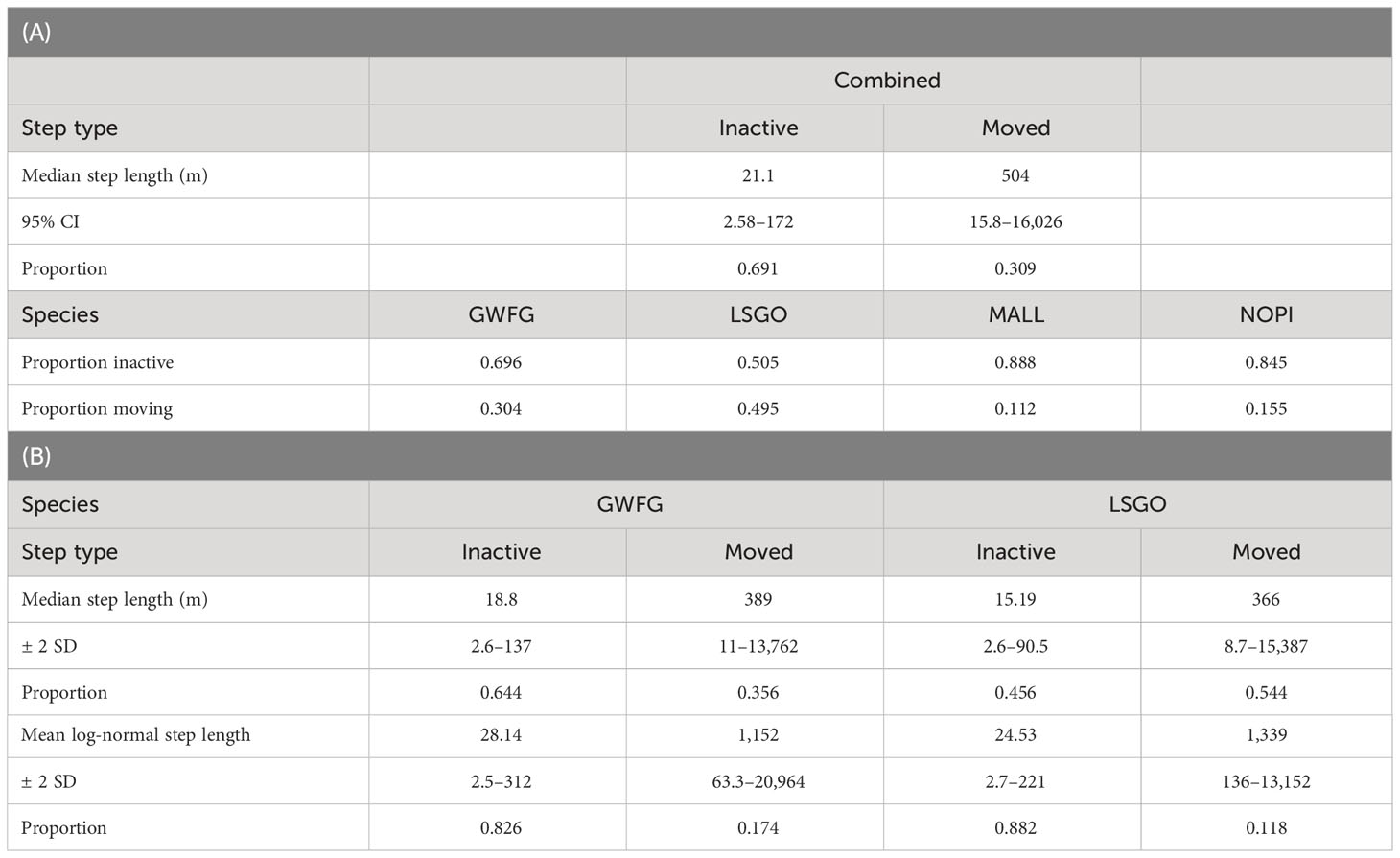
Table 2 Step length distributions were estimated using a 2-mixture log-normal distribution and a random sample of 50,000 steps across all four species (GWFG = greater white-fronted goose, LSGO = lesser snow goose, MALL = mallard, NOPI = northern pintail) of waterfowl (A) and species-specific step length distributions were also estimated using all available movements for each species (B).
Each species started the season with different rates of movement (F6,20726 = 731.35, p < 0.0001) with mallards moving the least frequently and snow geese the most. Each species’ mobility increased significantly through the season (GWFG: t6037 = 4.39, p < 0.0001; LSGO: t7052 = 5.36 p < 0.0001; MALL: t2470 = 6.075, p < 0.0001) except for Northern Pintail (t5167 = 0.515, p = 0.606), although they still maintained higher movement rates than mallard throughout the season (Figure 3). Circadian patterns also differed significantly between species (F21,8821 = 126.977, p < 0.0001). Nocturnal rates of movement were lower and diurnal rates were higher for all species, and ducks showed a stronger peak of movement activity at dawn and dusk with fewer mid-day movements than geese (Figure 4). Mobility also varied depending on the habitat occupied (Figure 5). Non-habitats (urban and other) generally had the highest rates of movement, and habitats reflecting either higher food value (rice, corn, and wetlands) or sanctuary from disturbance (protected lands) had the lowest rates of movement (Figure 5). Mallards were more inactive across all habitat types except urban, and lesser snow geese were the most mobile among all habitat types.
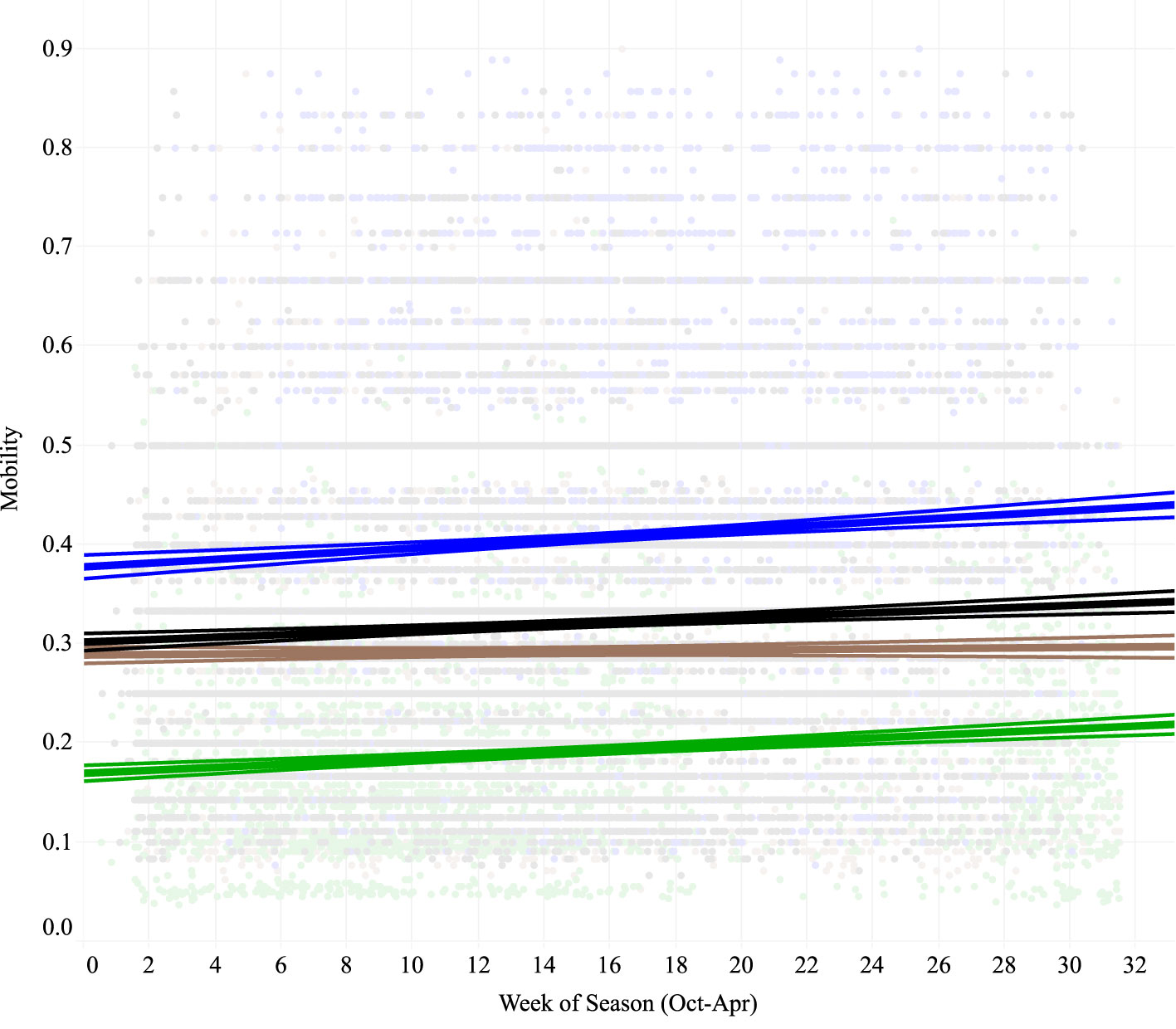
Figure 3 Movement activity increased for three of four species of waterfowl [greater white-fronted goose (black), lesser snow goose (blue), and mallard (green)] tracked in the Central Valley of California from October through April. Pintail (brown) began the season with a similar movement rate to greater white-fronted geese, but the increase in movement rate for pintail throughout the season was not significant (p = 0.42). Lesser snow geese had the highest movement rates, and mallard the lowest, throughout the season. Daily movement estimates were plotted as background points. Trend lines were plotted with 95% confidence regions.
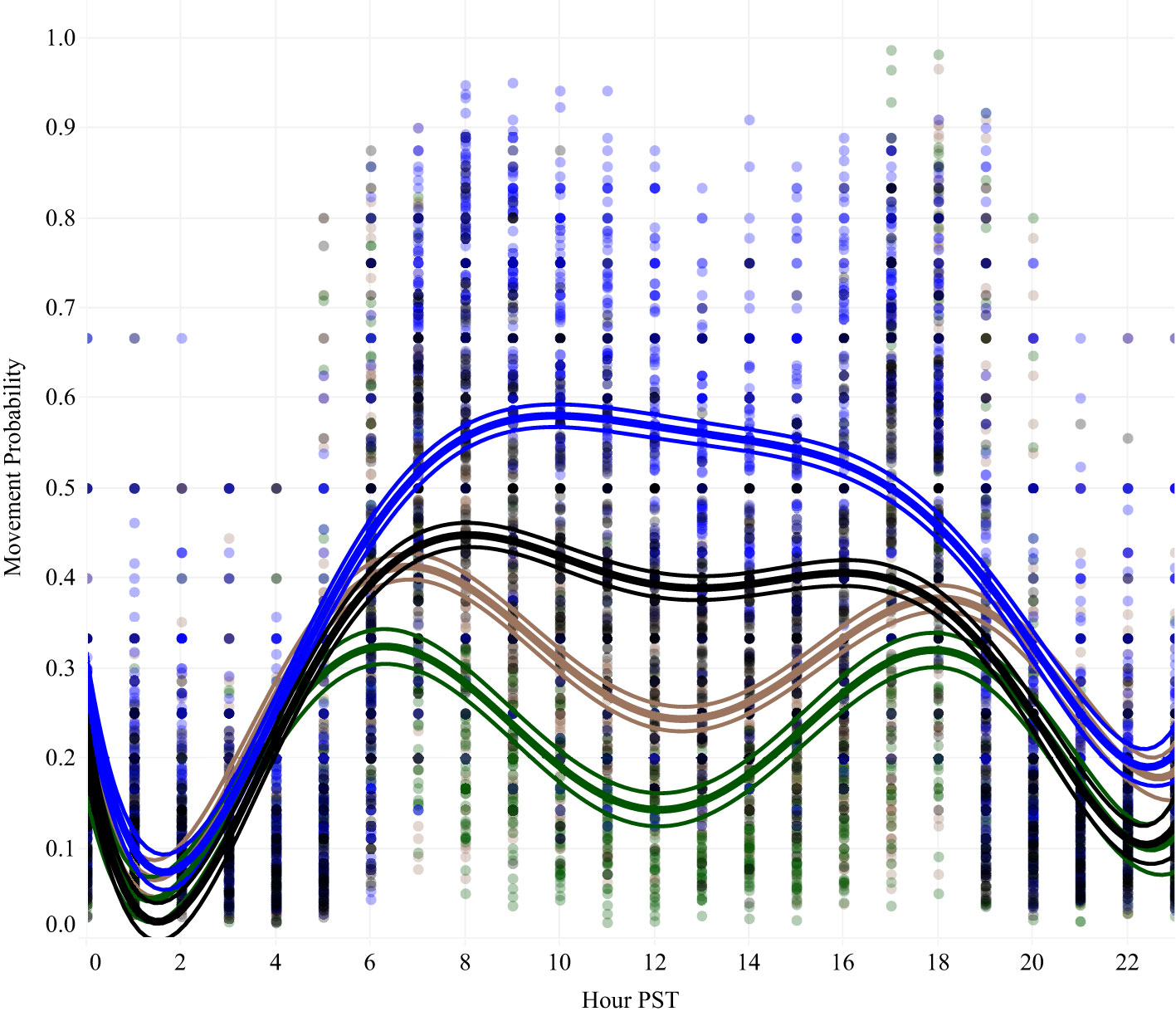
Figure 4 Circadian patterns of movement activity showed a similar pattern of reduced movement probability overnight across species. Diurnal movement activity was greater among geese, greater white-fronted goose (black) and lesser snow goose (blue), which produced a strong crepuscular peak in movement activity among ducks, mallard (green), and northern pintail (brown). Hourly estimated movement probability was plotted for each bird-year as background points. Trend lines were plotted with 95% confidence regions.
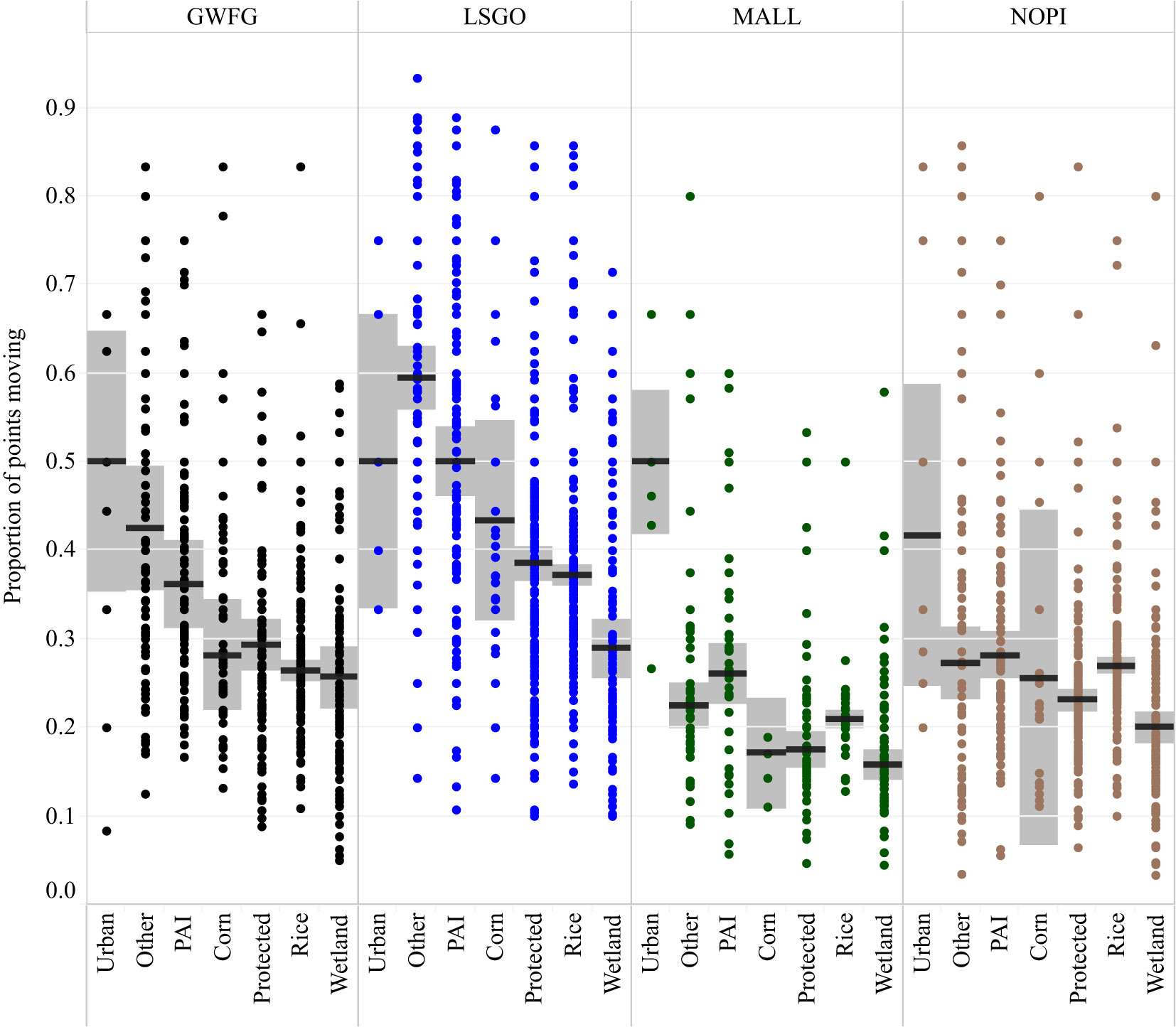
Figure 5 Movement activity among four species of waterfowl, greater white-fronted goose (black), lesser snow goose (blue), mallard (green), and northern pintail (brown), and among habitats being occupied. Points represent the proportion of movements in each habitat and by each species that best fit the “moving” component of a two-mixture log-normal step length distribution. Bar represents the median proportion of points identified as moving, and shaded regions indicate 95% confidence regions. PAI indicates pasture, alfalfa, and idle croplands.
Each species, except mallards, used rice habitats the most and rice was also the most available habitat type at normal ranges of movement. Protected lands were both the most used and the most available habitat for mallards (Table 3). The second most used habitat for species other than mallard was protected land, while mallards used PAI habitats (i.e., pasture, alfalfa, and idle cropland) the second most frequently (Table 3). All species used urban lands the least, which were not always the least available habitat within normal movement ranges (Table 3). Rice, corn, and protected lands had significantly higher selection than alternative habitats based on RSS for most species in the early winter (Figure 6). The RSS for rice declined for all species during the late winter and only pintail retained significant selection for rice after January 15. Greater white-fronted geese did not significantly select sanctuary in the early winter period and mallard did not select sanctuary in the late winter, nor did they select corn in either period (Figure 6). In the early winter, private wetlands were avoided by goose species and selected for only by mallard. In the late winter, private wetlands were significantly selected for only by snow geese (Figure 6). Urban habitats were always avoided by all species, but not significantly by mallards in the late winter. PAI habitats were avoided by all species in the early winter, but less so in the late winter when only lesser snow geese and northern pintail significantly avoided PAI relative to other habitats (Figure 6). Other non-habitat landcovers were avoided by each species in both periods (Figure 6).
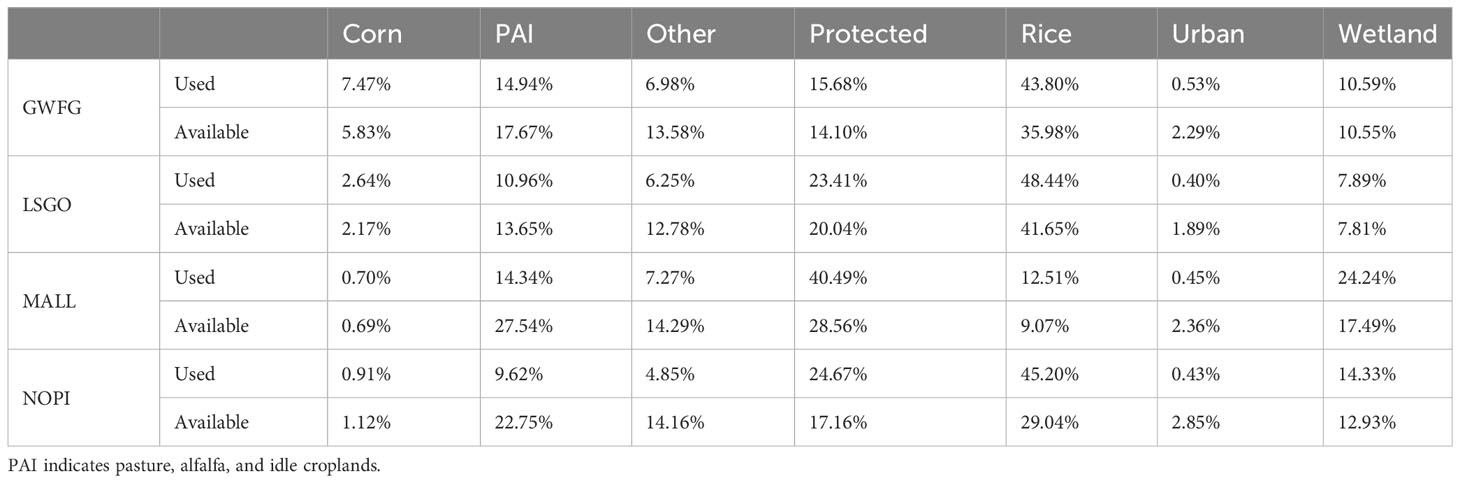
Table 3 Proportion of telemetry locations occurring within seven habitat types (“Used”) by four species (GWFG = greater white-fronted goose, LSGO = lesser snow goose, MALL = mallard, and NOPI = northern pintail) of wintering waterfowl (“Used”) in the Central Valley of California, and the average proportion of 100 random movements into each habitat type that originate from each observed location (“Available”).
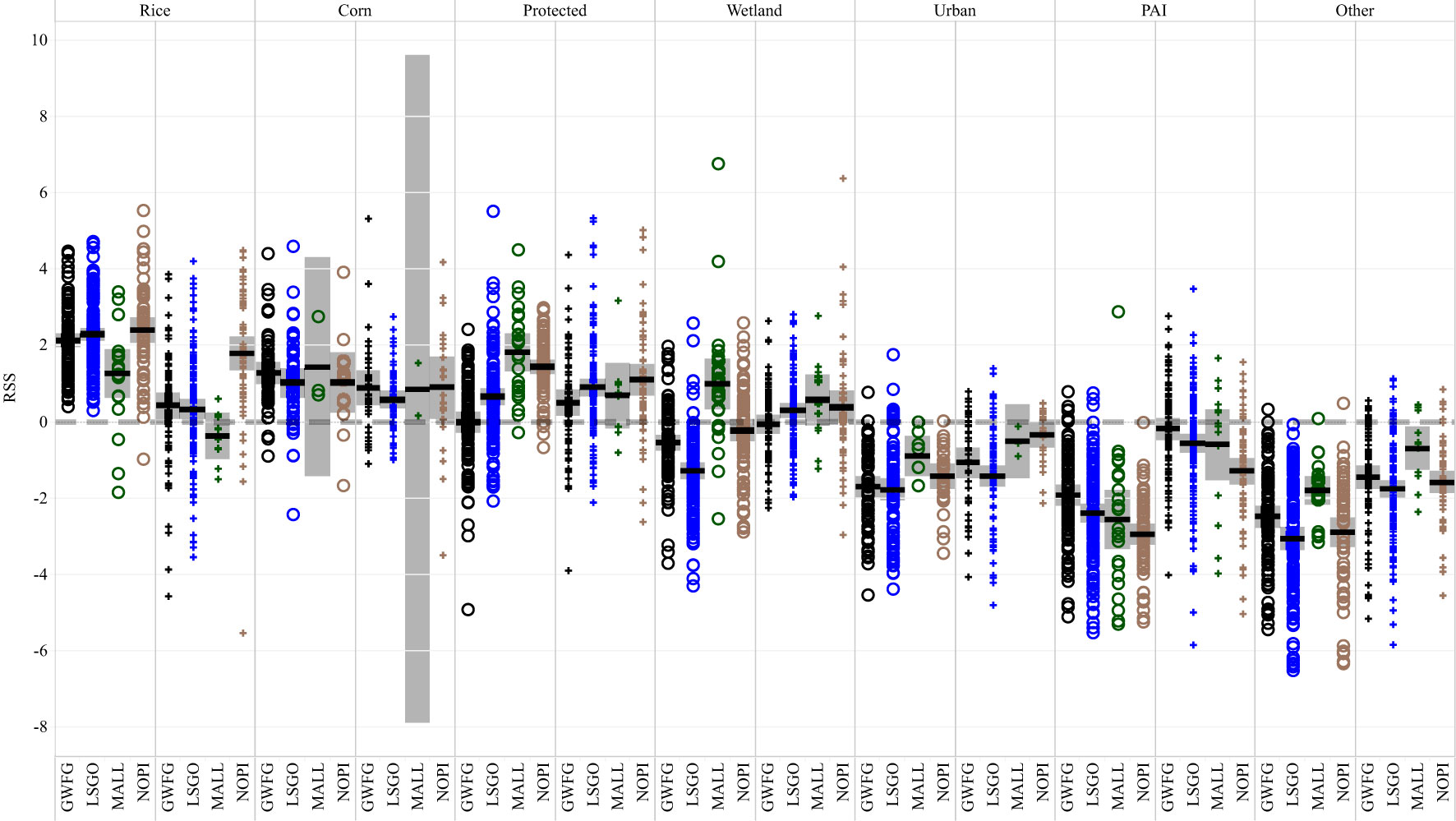
Figures 6 Relative strength of selection estimates among seven habitat classes and four species of waterfowl, greater white-fronted goose (black), lesser snow goose (blue), mallard (green), and northern pintail (brown), wintering in the Central Valley of California. Estimates were calculated for individual birds in both diurnal and nocturnal periods and within early (October to January 15; circles) and late (January 16 through April, crosses) periods. Species × habitat combinations marked with asterisks did not show a significant difference in relative use between the indicated habitat and other habitats available with that species’ movement range. All other habitats indicated significant selection or avoidance relative to other available habitats. Habitat types ranked by mean RSS across individuals from highest (left) to lowest (right) average RSS. PAI indicates pasture, alfalfa, and idle croplands.
Functional relationships describing the relative strength of selection a species had for a habitat compared with the average availability of habitat demonstrated differences in how each species used or avoided the seven habitat types. For each species and habitat, these patterns did not differ between early and late winter periods, so both periods were combined for subsequent interpretations. Differences in functional response were greatest between geese and ducks, and most similar between snow geese and greater white-fronted geese (Figure 7). Rice, which was selected by all species, had increasing RSS with availability among geese and consistent RSS across availability for ducks (Figure 7). Corn was available in limited quantities in most individuals, and though selected for by most species, the RSS did not change across availability for either goose species, nor for mallards, and the RSS for corn declined with increasing availability for pintail (Figure 7). Protected lands were also generally selected for by most individuals, and all species except mallards showed increasing RSS with availability; mallards’ RSS was constant across all availabilities of protected lands (Figure 7). Although the wetlands were avoided relative to other habitats for geese, and used equally as other habitats by pintail, a significant increase in the RSS of wetlands existed for all species except for mallard, for which RSS was constant across availability (Figure 7). Each species avoided urban habitats and showed constant RSS among the limited amount of urban land that was available. Although PAI habitats were generally avoided throughout the winter, the RSS increased with availability for each species except mallard, for which it remained constant (Figure 7). Among geese, the estimated increase in RSS for PAI as availability increased suggests that geese will select for PAI habitats when more than 45% of available habitat within their movement range is PAI (Figure 7). Other, mostly non-habitat, landcover classes were also avoided by each species and RSS increased with availability.
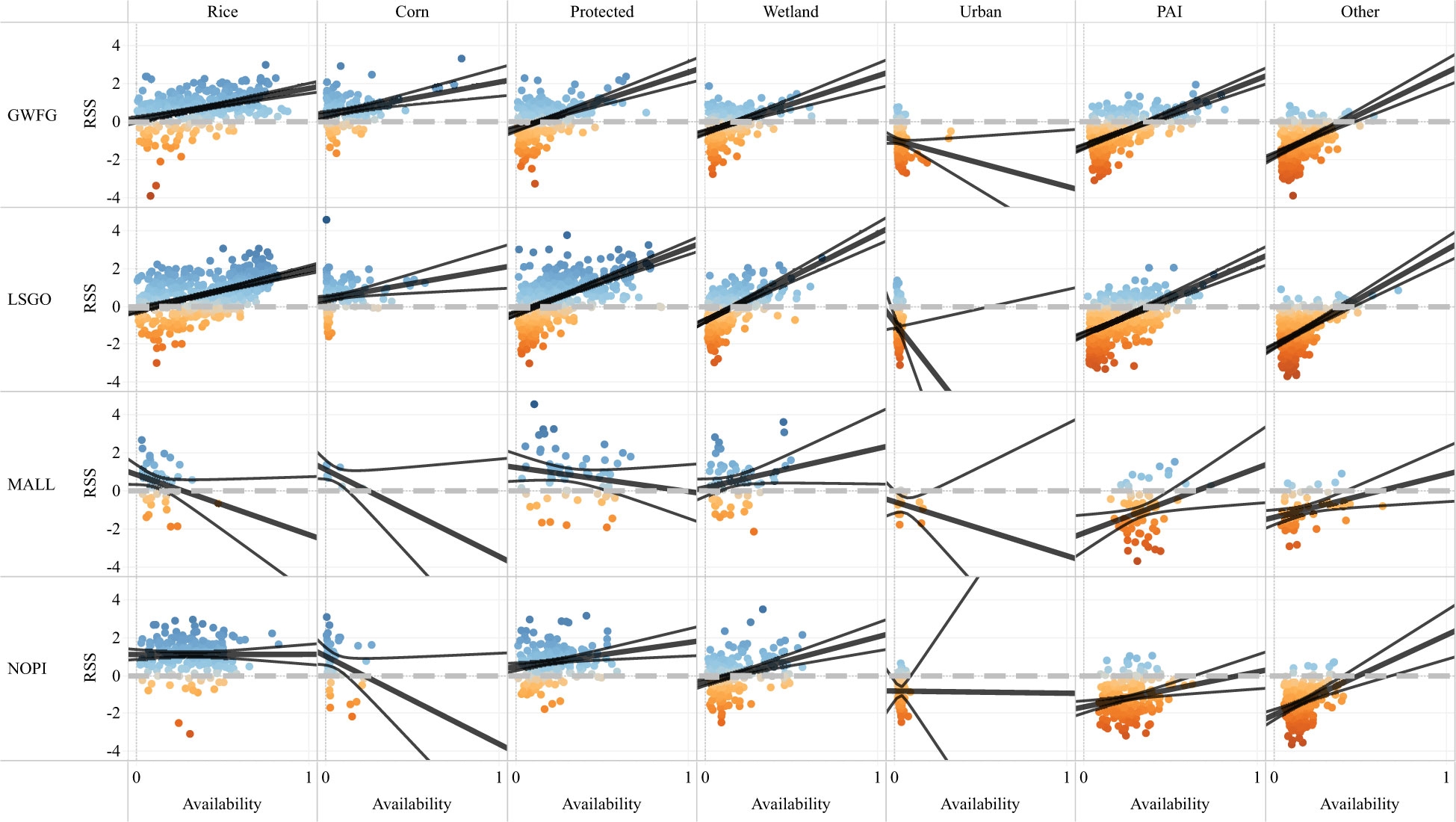
Figure 7 Functional response in the relative strength of selection (RSS) for seven habitats among four species of wintering waterfowl (GWFG = greater white-fronted goose, LSGO = lesser snow goose, MALL = mallard, and NOPI = northern pintail) using the Central Valley in California from October through April. Points represent RSS estimates from step selection analyses for each species during both diurnal and nocturnal periods and early (October to January 15) and late (January 16 through April) seasons. Color of points represents the RSS (blue = positive/selection, brown = negative/avoidance). Linear functional response (thick black line) relates RSS with average habitat availability and includes 95% confidence region (thin black lines). Reference line (dashed gray) indicates equal selection ratio between the indicated habitat and other habitats available within the normal movement range of the species. Habitat types ranked by mean RSS across individuals from highest (left) to lowest (right) average RSS. PAI indicates pasture, alfalfa, and idle croplands.
Discussion
Step lengths derived from hourly movements of wintering waterfowl exhibited a high degree of non-stationarity that resulted from multiple types of movement occurring at that temporal scale. Standard, single-component, step length distributions were not able to accurately replicate the empirical pattern of movement, but a mixture model did produce similar step length distributions. The two-mixture log-normal distribution we used should improve calculation of integrated step selection analysis (iSSA; Avgar et al., 2016). Incorporating multimodal parametric movement distributions extends the utility of iSSA to efforts that currently require empirical distributions of observed step lengths (Fortin et al., 2005; Forester et al., 2009), track segmentation (Patterson et al., 2009), or destructive sampling (Schabenberger and Gotway, 2005) to accurately reflect the available landscape.
The ability to distinguish between inactivity and movement in each birds’ track also allowed effective comparisons of movement patterns among our wintering waterfowl species. The circadian pattern in waterfowl activity described by waterfowl movements is well documented among individual species (Paulus, 1988) but has yet to be described among multiple co-occurring taxa and provides valuable estimates for agent-based modeling (Miller et al., 2014) and more accurate scenario planning in energetics models (CVJV, 2020). The seasonal increase in movement frequency observed among most waterfowl warrants closer attention. There are a variety of reasons why increased movement may occur later in the winter ranging from increased foraging effort resulting from exploitative competition (Legagneux et al., 2009; Davis and Afton, 2010) to seasonal behavioral and physiological changes related to breeding activity (McKinney, 1965). However, prior work indicates that increased frequency of movement may not correspond to increased movement distance, which was not shown to broadly increase across species or sexes of dabbling duck in the Central Valley (McDuie et al., 2019), and the increased probability of movement we estimated was small (~4% increase in April compared to October for most species, <2% for northern pintail). This suggests that increased movement frequency is not related to lower food supplies and is more likely related to social interactions associated with courtship behavior and the reduction of disturbance following the completion of hunting seasons.
A major concern in the wintering ranges of many animal species is the potential for food resource competition or other limitations (Marchowski et al., 2015; Petrie et al., 2016). Similarities in food resources across taxa, habitat dynamics that increase or decrease food accessibility, overabundant co-occurring species, reliance on variable water supplies during extended droughts, and the dependence on agricultural practices to provide many of the food resources animals depend on the increased potential that, under some conditions, food resource competition could affect the fitness of individuals and prevent achievement of conservation goals (CVJV, 2020, Williams et al., 2014; Petrie et al., 2016). Habitat types we assumed a priori to provide the most, or highest-quality, resources (e.g., rice, corn, protected lands, and wetlands, CVJV, 2020, Miller, 1987; Petrie et al., 2016) were the habitats with the lowest rates of mobility, indicating that birds remained localized in those habitats more than alternatives. Additionally, movements among geese were more common than among ducks, indicating potentially higher energetic demand. Within-taxa differences were also apparent as mallards were only 75% as mobile as northern pintail, and snow geese was nearly 40% more mobile than greater white-fronted geese. These differences can be used to parameterize species-specific demand in landscape energetics models (e.g., CVJV, 2020, Petrie et al, 2016) or agent-based models (Miller et al., 2014; Williams et al., 2014) and for scenario planning and establishing conservation objectives. They may also indicate which species are likely to demonstrate impacts of food limitations first. Based on our assessment, snow geese are likely to demonstrate physiological or behavioral response to food limitation first, if foods are limited prior to diet shifts during the late winter, due to their high movement rate, higher relative use of rice, and stronger functional response with rice availability. Food limitations occurring later in the season are likely to manifest in pintail first since they remain reliant on rice; however, such limitation may not reflect change in resource selection due to the constant functional response with respect to availability for this species.
Relative habitat value as indicated by step selection functions matched our a priori expectations; specifically, habitats providing key food resources and habitats providing sanctuary during the hunting season were selected more than the alternative habitats available to individuals. However, habitat use patterns also indicated important differences between taxa. Mallards were the only species that show significantly stronger selection for wetlands than alternate habitats and were also the species with the strongest RSS for protected areas (e.g., National Wildlife Refuges, State Wildlife Areas, and conservation easements), which, in the Central Valley, are often specifically managed to provide seasonal or semi-permanent wetlands (CVJV, 2020). Compared to the other species, mallard appear to benefit particularly from the management of both public and private emergent seasonal wetlands. The consequence is that mallard may be insulated from any future changes in agricultural practices that reduce the value of primary waterfowl food crops such as conversion from rice or reduction in winter flooding due to drought. In addition, mallard may be a better indicator than other species of the benefits of conservation actions such as wetland enhancements or costs of practices that reduce wetland function such as changes in timing or quantity of water delivery.
Variability in functional responses between RSS and habitat availability existed across both habitat classes and between species and illustrated important behavioral and ecological differences between them. Specifically, pintail RSS functional responses were more different from mallards than from geese for four of the seven habitat types. In particular, they were different for three of four habitats with the highest average RSS (rice, protected, and wetland). This indicates that among resources shared by multiple species, such as primary food sources, plasticity in individual’s response to availability may limit competition. That is, northern pintail may be more likely to experience competition with greater white-fronted and snow geese because their functional responses were more similar to those two species. Mallards, which showed a more constant functional response with availability of selected habitats (Holbrook et al., 2019), are, by comparison, a habitat generalist. Differences in RSS and in functional relationships between goose species were minor for all habitats and indicated similar patterns of reliance on Central Valley habitats. However, seasonal diet shifts, particularly by geese that transition in the late winter from grains to grazing on emerging grasses in pasture, idle cropland, and other formerly bare ground, may affect the relationship between RSS and habitat availability. Increase in relative use of PAI coincided with decreases in RSS for rice for all species except pintail. This supports the idea that many species transition diet from grains to grasses and/or invertebrates prior to the breeding season (Euliss and Harris, 1987; Miller, 1987; Ely and Raveling, 2011). Only pintail maintain strong selection for rice over other habitats. Because none of the late winter RSS values for non-rice habitats indicate both selection for the habitat and a significant increase from the early winter, northern pintail may be particularly impacted by changes to the timing or patterns of agricultural activities related to rice such as drying fields to prepare them for planting in the spring.
Prior work that has interpretated behavior patterns related to different functional responses have assessed a variety of habitat selection analysis methods (Holbrook et al., 2019). Many behaviors (e.g., proportional use, trade-offs, and avoidance) result in different mathematical relationships between selection metrics (e.g., use, use:availability ratios, and RSF coefficients) and habitat availability (Holbrook et al., 2019). RSS metrics resulting from SSA have not yet been similarly analyzed. SSA and iSSA (Avgar et al., 2016) identify habitat availability based not only on the composition and configuration of the landscape but also on the cognitive/behavioral process that governs movements and navigation (Nathan et al., 2022). Availability, in SSA, is defined at each location by the joint distributions of behaviorally mediated movement processes (step lengths and turning angles) and the configuration and composition of habitats in a spatially explicit landscape. This means functional response can then be estimated within individuals, which allows investigation of individual plasticity in resource selection (Muff et al., 2020; Northrup et al., 2022). However, the functional relationships between RSS metrics and estimates of resource availability derived using SSA likely have different mathematical forms compared to other habitat selection metrics because SSA may describe habitat at different (and multiple) scales and orders of selection (Johnson, 1980; Thurfjell et al., 2014) compared to some alternate habitat selection methods. Our results indicate that among several categorical habitat types, the relationship between the relative strength of selection and habitat availability indicates avoidance at low availability and selection at high availability, which did not match any previously described selection behaviors (Holbrook et al., 2019). This might be expected in a landscape of patchy or clustered resources when availability is determined to be greater near an animal’s current location. Spatial and temporal autocorrelation in habitat use and availability are likely to create difficult computational and methodological issues to resolve where it is appropriate to do so (Alston et al., 2023). Our segmentation of the movement process into “inactive” and “moving” modalities was a partial solution to reduce autocorrelation. Newer approaches such as autocorrelation weighting may both increase precision of functional responses and reduce bias in selection functions (Alston et al., 2023).
Our use of path segmentation to develop behavior-specific step selection functions (i.e., by separating inactive movement periods from the step length distribution) may have made resulting functions more accurate and/or improved computational efficiencies and is one of the multiple approaches to separate tracks into specific behavior states (Roever et al., 2014; Mott, 2022; Pay et al., 2022). Without segmenting and removal of the inactive step lengths, 45% to 89% of our sample of available habitat would likely be at functionally the same location as the observed step and require a greater sample of available steps to obtain representation of other habitat types. This would reduce the magnitude of RSS for occupied habitats since, at each location, the used habitat class would be identical to a greater portion of available habitat samples. This may be a particular problem for flighted birds that often have a greater disparity in movement capacity between movement activity modes, which result in bi- or multi-modal distributions of step lengths that are not accurately reflected in single-component distributions.
We developed species-specific step length distributions for our SSA because we anticipated individual species to have different biologically relevant movement processes such as period of feeding (diurnal vs. nocturnal), food acquisition methods (rotational feeding vs. dabbling), and susceptibility to disturbance. Although we used the same temporal scale (hourly) for each species, it is possible that the non-stationary movement patterns may reflect different behaviors for ducks and geese, for example, differences in foraging behavior. Evidence for this lies in that median step length when “moving” was nearly four times further in ducks than geese. Mallard and northern pintail generally feed by dabbling, tipping to reach submerged food, in flooded fields. Geese will feed in flooded fields, but also forage in dry or puddled fields where they both walk and periodically fly to the leading edge of foraging flocks where food resources are gained at the quickest rate (Black et al., 1992). Therefore, the “moving” steps for geese may reflect a greater proportion of intra-patch movements related to foraging while “moving” ducks tend to be moving between habitat patches. Our results are likely robust to this potential disparity in movement activity, because most habitat patches in the Central Valley are either smaller in scale than the median movements of geese (e.g., wetlands) or larger in extent than median movements of ducks (e.g., rice). Thus, both taxa would have been affected in approximately the same manner by the spatial structure of habitats.
Conclusion
Co-occurring species that seasonally concentrate and utilize similar food or other resources may show differences in functional responses between relative strengths of habitat selection and availability of habitats due to niche differentiation or they may show similarities that could indicate interspecific competition. Wintering waterfowl’s movements and selection of habitats in California’s Central Valley show differences between taxonomic groups (geese versus ducks), differences within taxonomic groups (mallard versus northern pintail), seasonal patterns, and different functional responses. These complex patterns reflect well understood behavioral differences (patterns of foraging activity), resource needs (preference for sanctuary areas during the hunting season), and species phenology (diet shifts). Functional responses also suggest that for most habitats, the relative importance of the habitat increases with the relative abundance of that habitat within the normal range of a single movement. This likely reflects waterfowl’s (and birds in general) tendency for limited movements within patches while foraging and their ability to move long distances between patches quickly. Habitat importance across all habitats for mallards was least affected by availability, suggesting that they are more of a generalist species. Pintails were additively selecting (Holbrook et al., 2019) for their principal food resource, rice, indicating that rice was equally important regardless of availability whereas the importance of rice to geese increased as more rice was available, implying that geese may be focusing their space use at higher scales (second order) where rice is more available to them (Holbrook et al., 2019). Our results identify the habitat selection patterns, species, and habitat types that may be most likely to reflect impacts of competition and food resource limitation. Conservation and scenario planners may use these predictions to develop landscape energy budgets and management responses to environmental limitations such as drought or changing agricultural practices during periods when food resources may become limited.
Data availability statement
The datasets presented in this study can be found in online repositories. The names of the repository/repositories and accession number(s) can be found below: U.S. Geological Survey ScienceBase Digital Repository (https://www.sciencebase.gov/catalog/), https://doi.org/10.5066/P9ELSUHN.
Ethics statement
The animal study was approved by the U.S. Geological Survey Western Ecological Research Center Institutional Animal Use and Care Committee. The study was conducted in accordance with the local legislation and institutional requirements.
Author contributions
MC conceived the original idea and design. CO conducted analyses and authored the manuscript with edits by MC. All authors contributed to the article and approved the submitted version.
Funding
We thank the Arctic Goose Joint Venture, California Department of Water Resources, Ducks Unlimited, and the USGS Ecosystems Mission Area for funding that enabled this work.
Acknowledgments
We wish to thank F. McDuie, E. Matchett, A. Mott, D. Mackell, and many technicians from the U.S. Geological Survey (USGS) Dixon Field Station; V. Patil and staff at the USGS Alaska Science Center; E. Reed and staff at the Environment and Climate Change Canada–Yellowknife; and the California Waterfowl Association for conducting the field work to collect and complete quality control for the data used in this manuscript. We thank personnel at Grizzly Island Wildlife Area, Upper Butte Basin Wildlife Area, Summer Lake Wildlife Area, and the Sacramento National Wildlife Refuge for land access and logistical support.
Conflict of interest
The authors declare that the research was conducted in the absence of any commercial or financial relationships that could be construed as a potential conflict of interest.
Publisher’s note
All claims expressed in this article are solely those of the authors and do not necessarily represent those of their affiliated organizations, or those of the publisher, the editors and the reviewers. Any product that may be evaluated in this article, or claim that may be made by its manufacturer, is not guaranteed or endorsed by the publisher.
Author disclaimer
Any use of trade, firm, or product names is for descriptive purposes only and does not imply endorsement by the U.S. Government.
References
Aarts G., Fieberg J., Brasseur S., Matthiopoulos J. (2013). Quantifying the effect of habitat availability on species distributions. J. Anim. Ecol. 82, 1135–1145. doi: 10.1111/1365-2656.12061
Alston J. M., Fleming C. H., Kays R., Streicher J. P., Downs C. T., Ramesh T., et al. (2023). Mitigating pseudoreplication and bias in resource selection functions with autocorrelation-informed weighting. Methods Ecol. Evol. 14, 643–654. doi: 10.1111/2041-210X.14025
Avgar T., Potts J. R., Lewis M. A., Boyce M. S. (2016). Integrated step selection analysis: bridging the gap between resource selection and animal movement. Methods Ecol. Evol. 7, 619–630. doi: 10.1111/2041-210X.12528
Aybar C. (2022). “Rgee: R bindings for calling the ‘Earth Engine’ API,” in R package version 1.1.5. Available at: https://CRAN.R-project.org/package=rgee.
Benaglia T., Chauveau D., Hunter D. R., Young D. (2009). mixtools: an R package for analyzing finite mixture models. J. Stat. Soft 32, 1-29. doi: 10.18637/jss.v032.i06
Bergen S., Huso M. M., Duerr A. E., Braham M. A., Katzner T. E., Schmuecker S., et al. (2022). Classifying behavior from short-interval biologging data: An example with GPS tracking of birds. Ecol. Evol. 12, e08395. doi: 10.1002/ece3.8395
Bivand R., Keitt T., Rowlingson B. (2022). “rgdal: bindings for the 'Geospatial' Data abstraction library,” in R package version 1. Available at: https://CRAN.R-project.org/package=rgdal.
Black J. M., Carbone C., Wells R. L., and Owen M.. (1992). Foraging dynamics in goose flocks: The cost of living on the edge. Animal Behav. 44 (1), 41–50.. doi: 10.1016/S0003-3472(05)80752-3
Boryan C., Yang Z., Mueller R., Craig M. (2011). Monitoring US agriculture: the US department of agriculture, national agricultural statistics service, cropland data layer program. Geocarto Int. 26, 341–358. doi: 10.1080/10106049.2011.562309
Boyce M. S., Johnson C. J., Merrill E. H., Nielsen S. E., Solberg E. J., Van Moorter B. (2016). REVIEW: Can habitat selection predict abundance? J. Anim. Ecol. 85, 11–20. doi: 10.1111/1365-2656.12359
Brussee B. E., Coates P. S., O’Neil S. T., Casazza M. L., Espinosa S. P., Boone J. D., et al. (2022). Invasion of annual grasses following wildfire corresponds to maladaptive habitat selection by a sagebrush ecosystem indicator species. Global Ecol. Conserv. 37, e02147. doi: 10.1016/j.gecco.2022.e02147
Casazza M. L., McDuie F., Jones S., Lorenz A. A., Overton C. T., Yee J., et al. (2021). Waterfowl use of wetland habitats informs wetland restoration designs for multi-species benefits. J. Appl. Ecol. 58, 1910–1920. doi: 10.1111/1365-2664.13845
CVJV (2020). Central valley joint venture 2020 implementation plan (Sacramento, CA: US Fish and Wildlife Service).
Davis B. E., Afton A. D. (2010). Movement distances and habitat switching by female mallards wintering in the lower mississippi alluvial valley. Waterbirds 33, 349–356. doi: 10.1675/063.033.0311
Diffenbaugh N. S., Swain D. L., Touma D. (2015). Anthropogenic warming has increased drought risk in California. Proc. Natl. Acad. Sci. U.S.A. 112, 3931–3936. doi: 10.1073/pnas.1422385112
Duchesne T., Fortin D., Courbin N. (2010). Mixed conditional logistic regression for habitat selection studies. J. Anim. Ecol. 79, 548–555. doi: 10.1111/j.1365-2656.2010.01670.x
Ely C. R., Raveling D. G. (2011). Seasonal variation in nutritional characteristics of the diet of greater white-fronted geese: White-Fronted Goose Diet. J. Wildlife Manage. 75, 78–91. doi: 10.1002/jwmg.13
Euliss N. H., Harris S. W. (1987). Feeding ecology of northern pintails and green-winged teal wintering in california. J. Wildlife Manage. 51, 724. doi: 10.2307/3801733
Fieberg J., Signer J., Smith B., Avgar T. (2021). A ‘How to’ guide for interpreting parameters in habitat-selection analyses. J. Anim. Ecol. 90, 1027–1043. doi: 10.1111/1365-2656.13441
Fleming K. K., Mitchell M. K., Brasher M. G., Colluccy J. M., James J. D., Petrie M. J., et al. (2019). “Derivation of regional, non-breeding duck population abundance objectives to inform conservation planning in North America. 2019 revision. North American Waterfowl Management Plan Science Support Team Technical Report 1,” in Central valley joint venture (CVJV), 2020. Central valley joint venture 2020 implementation plan (Sacramento, CA: US Fish and Wildlife Service).
Forester J. D., Im H. K., Rathouz P. J. (2009). Accounting for animal movement in estimation of resource selection functions: sampling and data analysis. Ecology 90, 3554–3565. doi: 10.1890/08-0874.1
Fortin D., Beyer H. L., Boyce M. S., Smith D. W., Duchesne T., Mao J. S. (2005). Wolves influence elk movements: Behavior shapes a trophic cascade in Yellowstone National Park. Ecology 86, 1320–1330. doi: 10.1890/04-0953
Gillies C. S., Hebblewhite M., Nielsen S. E., Krawchuk M. A., Aldridge C. L., Frair J. L., et al. (2006). Application of random effects to the study of resource selection by animals: Random effects in resource selection. J. Anim. Ecol. 75, 887–898. doi: 10.1111/j.1365-2656.2006.01106.x
GreenInfo Network (2022) California Protected Areas Database 2022b release. Available at: https://data.cnra.ca.gov/dataset/california-protected-areas-database.
Groff L. A., Calhoun A. J. K., Loftin C. S. (2017). Amphibian terrestrial habitat selection and movement patterns vary with annual life-history period. Can. J. Zool. 95, 433–442. doi: 10.1139/cjz-2016-0148
Holbrook J. D., Olson L. E., DeCesare N. J., Hebblewhite M., Squires J. R., Steenweg R. (2019). Functional responses in habitat selection: clarifying hypotheses and interpretations. Ecol. Appl. 29, e01852. doi: 10.1002/eap.1852
Johnson D. H. (1980). The comparison of usage and availability measurements for evaluating resource preference. Ecology 61, 65–71. doi: 10.2307/1937156
Johnson D. S., Thomas D. L., Ver Hoef J. M., Christ A. (2008). A general framework for the analysis of animal resource selection from telemetry data. Biometrics 64, 968–976. doi: 10.1111/j.1541-0420.2007.00943.x
Kays R., Crofoot M. C., Jetz W., Wikelski M. (2015). Terrestrial animal tracking as an eye on life and planet. Science 348, aaa2478. doi: 10.1126/science.aaa2478
Knopff A. A., Knopff K. H., Boyce M. S., St. Clair C. C. (2014). Flexible habitat selection by cougars in response to anthropogenic development. Biol. Conserv. 178, 136–145. doi: 10.1016/j.biocon.2014.07.017
Lark T. J., Schelly I. H., Gibbs H. K. (2021). Accuracy, bias, and improvements in mapping crops and cropland across the United States using the USDA cropland data layer. Remote Sens. 13, 968. doi: 10.3390/rs13050968
Leclerc M., Vander Wal E., Zedrosser A., Swenson J. E., Kindberg J., Pelletier F. (2016). Quantifying consistent individual differences in habitat selection. Oecologia 180, 697–705. doi: 10.1007/s00442-015-3500-6
Legagneux P., Blaize C., Latraube F., Gautier J., Bretagnolle V. (2009). Variation in home-range size and movements of wintering dabbling ducks. J. Ornithol 150, 183–193. doi: 10.1007/s10336-008-0333-7
Manly B. F. J., McDonald L. L., Thomas D. L., McDonald T., Erickson W. P. (2002). Resource selection by animals: statistical design and analysis for field studies. 2nd ed (Boston: Kluwer Academic Publishers, Dordrecht).
Marchowski D., Leitner M. (2019). Conservation implications of extraordinary Greater Scaup (Aythya marila) concentrations in the Odra Estuary, Poland. Condor 121, duz013. doi: 10.1093/condor/duz013
Marchowski D., Neubauer G., Ławicki Ł., Woźniczka A., Wysocki D., Guentzel S., et al. (2015). The Importance of Non-Native Prey, the Zebra Mussel Dreissena polymorpha, for the Declining Greater Scaup Aythya marila: A Case Study at a Key European Staging and Wintering Site. PloS One 10, e0145496. doi: 10.1371/journal.pone.0145496
McDuie F., Casazza M. L., Overton C. T., Herzog M. P., Hartman C. A., Peterson S. H., et al. (2019). GPS tracking data reveals daily spatio-temporal movement patterns of waterfowl. Mov Ecol. 7, 6. doi: 10.1186/s40462-019-0146-8
McDuie F., Lorenz A. A., Klinger R. C., Overton C. T., Feldheim C. L., Ackerman J. T., et al. (2021). Informing wetland management with waterfowl movement and sanctuary use responses to human-induced disturbance. J. Environ. Manage. 297, 113170. doi: 10.1016/j.jenvman.2021.113170
McNeil R., Drapeau P., Goss-Custard J. D. (1992). The occurrence and adaptive signficance of nocturnal habits in waterfowl. Biol. Rev. 67, 381–419. doi: 10.1111/j.1469-185X.1992.tb01188.x
Miller M. R. (1987). Fall and winter foods of northern pintails in the sacramento valley, california. J. Wildlife Manage. 51, 405. doi: 10.2307/3801027
Miller M. R., Garr J. D., Coates P. S. (2010). Changes in the status of harvested rice fields in the sacramento valley, california: implications for wintering waterfowl. Wetlands 30, 939–947. doi: 10.1007/s13157-010-0090-2
Miller M. L., Ringelman K. M., Schank J. C., Eadie J. M. (2014). SWAMP: An agent-based model for wetland and waterfowl conservation management. SIMULATION 90, 52–68. doi: 10.1177/0037549713511864
Mitchell L. J., Kohler T., White P. C. L., Arnold K. E. (2020). High interindividual variability in habitat selection and functional habitat relationships in European nightjars over a period of habitat change. Ecol. Evol. 10, 5932–5945. doi: 10.1002/ece3.6331
Morris D. W. (1989). Density-dependent habitat selection: Testing the theory with fitness data. Evol. Ecol. 3, 80–94. doi: 10.1007/BF02147934
Mott A. (2022). “Habitat use and distribution implications of four goose species wintering in California’s Sacramento Valley,” (Davis, CA: University of California-Davis).
Muff S., Signer J., Fieberg J. (2020). Accounting for individual-specific variation in habitat-selection studies: Efficient estimation of mixed-effects models using Bayesian or frequentist computation. J. Anim. Ecol. 89, 80–92. doi: 10.1111/1365-2656.13087
Mysterud A., Ims R. A. (1998). Functional responses in habitat use: availability influences relative use in trade-off situations. Ecol 79, 1435–1441. doi: 10.1890/0012-9658(1998)079[1435:FRIHUA]2.0.CO;2
Nathan R., Monk C. T., Arlinghaus R., Adam T., Alós J., Assaf M., et al. (2022). Big-data approaches lead to an increased understanding of the ecology of animal movement. Science 375, eabg1780. doi: 10.1126/science.abg1780
Neu C. W., Byers C. R., Peek J. M. (1974). A technique for analysis of utilization-availability data. J. Wildlife Manage. 38, 541. doi: 10.2307/3800887
Northrup J. M., Vander Wal E., Bonar M., Fieberg J., Laforge M. P., Leclerc M., et al. (2022). Conceptual and methodological advances in habitat-selection modeling: guidelines for ecology and evolution. Ecol. Appl. 32, e02470. doi: 10.1002/eap.2470
Olson S. M.2022Pacific Flyway Data Book 2022: Migratory bird abundance, harvest, and hunter activity. Helena MT: (US Fish and Wildlife Service, Division of Migratory Bird Management).
Osborn J. M., Hagy H. M., Mcclanahan M. D., Davis J. B., Gray M. J. (2017). Habitat selection and activities of dabbling ducks during non-breeding periods: Dabbling Duck Habitat Selection. Jour. Wild. Mgmt. 81, 1482–1493. doi: 10.1002/jwmg.21324
Osborne P. E., Foody G. M., Suárez-Seoane S. (2007). Non-stationarity and local approaches to modelling the distributions of wildlife. Diversity Distributions 13, 313–323. doi: 10.1111/j.1472-4642.2007.00344.x
Overton C., Casazza M., Bretz J., McDuie F., Matchett E., Mackell D., et al. (2022). Machine learned daily life history classification using low frequency tracking data and automated modelling pipelines: application to North American waterfowl. Mov Ecol. 10, 23. doi: 10.1186/s40462-022-00324-7
Overton C., Casazza M.. (2023). Movements, used habitats, and available habitats identified using step selection procedures for four species of waterfowl in California's Central Valley, 2015-2022. U.S. Geological Survey Data Release. Mov Ecol. doi: 10.5066/P9ELSUHN
Pathak T., Maskey M., Dahlberg J., Kearns F., Bali K., Zaccaria D. (2018). Climate change trends and impacts on california agriculture: A detailed review. Agronomy 8, 25. doi: 10.3390/agronomy8030025
Patterson T. A., Basson M., Bravington M. V., Gunn J. S. (2009). Classifying movement behaviour in relation to environmental conditions using hidden Markov models. J. Anim. Ecol. 78, 1113–1123. doi: 10.1111/j.1365-2656.2009.01583.x
Paulus S. L. (1988). Time-activity budgets of nonbreeding Anatidae: a review, In Waterfowl in Winter. Ed. Weller M. W. (Minneapolis, MN: University of Minnesota Press).
Pay J. M., Patterson T. A., Proft K. M., Cameron E. Z., Hawkins C. E., Koch A. J., et al. (2022). Considering behavioral state when predicting habitat use: Behavior-specific spatial models for the endangered Tasmanian wedge-tailed eagle. Biol. Conserv. 274, 109743. doi: 10.1016/j.biocon.2022.109743
Pebesma E. (2018). Simple features for R: Standardized support for spatial vector data. R J. 10, 439–446. doi: 10.32614/RJ-2018-009
Petrie M. J., Fleskes J. P., Wolder M. A., Isola C. R., Yarris G. S., Skalos D. A. (2016). Potential effects of drought on carrying capacity for wintering waterfowl in the central valley of california. J. Fish Wildlife Manage. 7, 408–422. doi: 10.3996/082015-JFWM-082
Resano-Mayor J., Korner-Nievergelt F., Vignali S., Horrenberger N., Barras A. G., Braunisch V., et al. (2019). Snow cover phenology is the main driver of foraging habitat selection for a high-alpine passerine during breeding: implications for species persistence in the face of climate change. Biodivers Conserv. 28, 2669–2685. doi: 10.1007/s10531-019-01786-9
Roever C. L., Beyer H. L., Chase M. J., and Van Aarde R. J.. (2014). The pitfalls of ignoring behaviour when quantifying habitat selection. Div. Distrib. 20 (3), 322–333. doi: 10.1111/ddi.12164
SFEI (2022) California Aquatic Resource Inventory (CARI) version 1.1. Available at: https://www.sfei.org/data/california-aquatic-resource-inventory-cari-version-11-gis-data.
Schabenberger O., Gotway C. A. (2005). Statistical methods for spatial data analysis, Texts in statistical science (Boca Raton: Chapman & Hall/CRC).
Signer J., Fieberg J., Avgar T. (2019). Animal movement tools ( amt ): R package for managing tracking data and conducting habitat selection analyses. Ecol. Evol. 9, 880–890. doi: 10.1002/ece3.4823
Skov H., Heinänen S., Žydelis R., Bellebaum J., Bzoma S., Dagys M., et al. (2011). Waterbird populations and pressures in the Baltic Sea (Copenhagen: Nordic Council of Ministers).
Thurfjell H., Ciuti S., Boyce M. S. (2014). Applications of step-selection functions in ecology and conservation. Mov Ecol. 2, 4. doi: 10.1186/2051-3933-2-4
USFWS (2018) National wetlands inventory. Available at: https://data.nal.usda.gov/dataset/national-wetlands-inventory.
Keywords: telemetry, GPS, step selection, Anas acuta, Anas platyrhynchos, Anser albifrons, Anser caerulescens
Citation: Overton CT and Casazza ML (2023) Movement behavior, habitat selection, and functional responses to habitat availability among four species of wintering waterfowl in California. Front. Ecol. Evol. 11:1232704. doi: 10.3389/fevo.2023.1232704
Received: 01 June 2023; Accepted: 02 October 2023;
Published: 23 October 2023.
Edited by:
Clare Aslan, Northern Arizona University, United StatesReviewed by:
Ryan Askren, University of Arkansas at Monticello, United StatesDominik Marchowski, Polish Academy of Sciences, Poland
Copyright © 2023 Overton and Casazza. This is an open-access article distributed under the terms of the Creative Commons Attribution License (CC BY). The use, distribution or reproduction in other forums is permitted, provided the original author(s) and the copyright owner(s) are credited and that the original publication in this journal is cited, in accordance with accepted academic practice. No use, distribution or reproduction is permitted which does not comply with these terms.
*Correspondence: Cory T. Overton, Y292ZXJ0b25AdXNncy5nb3Y=