- 1Key Laboratory of Agro-ecological Processes in Subtropical Region, Institute of Subtropical Agriculture, Chinese Academy of Sciences, Changsha, China
- 2Guangxi Key Laboratory of Karst Ecological Processes and Services, Huanjiang Observation and Research Station for Karst Ecosystems, Huanjiang, China
- 3Guangxi Industrial Technology Research Institute for Karst Rocky Desertification Control, Nanning, China
- 4Management Center for Guangxi Mulun National Naure Reserve, Huanjiang, China
Introduction: Leaf functional traits constitute a crucial component of plant functionality, providing insights into plants’ adaptability to the environment and their regulatory capacity in complex habitats. The response of leaf traits to environmental factors at the community level has garnered significant attention. Nevertheless, an examination of the environmental factors determining the spatial distribution of leaf traits in the karst region of southwest China remains absent.
Methods: In this study, we established a 25 ha plot within a karst forest and collected leaf samples from 144 woody species. We measured 14 leaf traits, including leaf area (LA), leaf thicknes (LT), specific leaf area (SLA), leaf length to width ratio (LW), leaf tissue density (LTD), leaf carbon concentration (LC), leaf nitrogen concentration (LN), and leaf phosphorus concentration (LP), leaf potassium concentration (LK), leaf calcium concentration (LCa), leaf magnesium Concentration (LMg), leaf carbon to nitrogen ratio (C/N), leaf carbon to phosphorus ratio (C/P), and leaf nitrogen to phosphorus ratio (N/P), to investigate the spatial distribution of community-level leaf traits and the response of the leaf trait community-weighted mean (CWM) to topographic, soil, and spatial factors.
Results: Results showed that the CWM of leaf traits display different spatial patterns, first, the highest CWM values for LT, LTD, C/N, and C/P at hilltops, second, the highest CWM values for LA, SLA, LW, LC, LN, LP, and LK at depressions, and third, the highest CWM values for LCa, LMg, and N/P at slopes. The correlation analysis showed that topographic factors were more correlated with leaf trait CWM than soil factors, with elevation and slope being the strongest correlations. RDA analysis showed that topographic factors explained higher percentage of leaf trait CWM than soil factors, with the highest percentage of 19.96% being explained by elevation among topographic factors. Variance Partitioning Analysis showed that the spatial distribution of leaf traits is predominantly influenced by the combined effects of topography and spatial factors (37%-47% explained), followed by purely spatial factors (24%-36% explained).
Discussion: The results could improve our understanding of community functional traits and their influencing factors in the karst region, which will contribute to a deeper understanding of the mechanisms that shape plant communities.
1 Introduction
Functional traits encompass a range of physiological and ecological indicators that profoundly influence plant survival, growth, and mortality. These traits have gradually developed through the long evolutionary process of exotic plants, which have adopted diverse strategies for resource acquisition and allocation (Reich et al., 2003; Meng et al., 2007; Liu and Ma, 2015). Certain traits can partially reflect species’ adaptation to their environment and their impacts on ecosystem functioning (McIntyre et al., 1999), making them crucial for investigating species coexistence, community assembly, and ecosystem function (Diaz et al., 2004; Violle et al., 2012). In the context of ecosystem development, leaves play a vital role as the primary site of plant photosynthesis and a significant organ for energy acquisition (Ackerly et al., 2002). Leaf functional traits can illuminate fundamental characteristics of plants and their efficient resource utilization. The application of leaf traits in studying plants’ adaptation mechanisms to the environment represents a recent breakthrough in physiological ecology (Pierce et al., 2017; Rawat et al., 2021). Leaf traits include morphological traits (LA, LT, LTD, etc.), elemental traits (LC, LN, etc.), leaf lifespan, and photosynthetic capacity (Cornelissen et al., 2003). These leaf functional traits differ substantially in different environments and reflect the ability of leaves to acquire resources and adapt to the environment (Stahl et al., 2013). The continuous deepening of research on leaf functional traits can clarify the influence of leaf traits on ecosystem processes and improve the knowledge of community construction and ecosystem function, which has important ecological significance.
Previous studies have primarily focused on examining the variation and correlations of species traits, as well as the relationship between individual traits and the environment (Pang et al., 2021). However, our understanding of leaf traits at the community level, including their variation and driving factors, remains limited compared to individual and species levels (He et al., 2018). To examine how these plant communities can be described from a functional perspective, Garnier tested the biomass ratio hypothesis and proposed “functional markers”, or community-aggregated (i.e., weighed according to the relative abundance of species) functional leaf traits, to assess the impact of community change on ecosystem properties (Garnier et al., 2004). Nowadays, leaf community trait values are widely described by CWM, which is a weighted average of a functional trait based on the species breast area to determine the mean value of the functional trait in the community. For example, Zhang found that the CWM of C:N:P ecological stoichiometry in Chinese forests can represent ecological stoichiometry at the community level quite adequately (Zhang et al., 2018). It can effectively reflect the trait characteristics of the community, capture the selection of plant leaf functional properties to the environment, reveal the adaptation strategies of plants at the community level, and quantify the functional trait characteristics spatially. Spatial patterns manifest in both physical and biological variables in nature (Legendre et al., 2002; Tuda, 2007). Spatial autocorrelation in the distribution of individuals may arise due to biological processes (e.g., dispersal, competition), historical factors (e.g., disturbances), or spatially structured environmental factors (Ives and Zhu, 2006). While these processes may not be directly measurable, their influence can be evident in raw data or model residuals. Considering these spatial structures as contributions from biological processes can greatly aid our understanding of community assembly (Keitt and Urban, 2005). The study of the spatial distribution pattern of functional traits in communities not only helps to describe the structure of functional traits in communities more accurately (Wang et al., 2010), but also reveals the interrelationships and adaptation strategies between traits and environmental factors such as topography and soil.
Environmental factors exert control over the spatial redistribution of light, heat, water, and soil nutrients through geomorphic processes and morphological changes, significantly influencing the functional traits of plants in forest ecosystems (Bennie et al., 2008; Zhu et al., 2016). Topographic factors play a substantial role in shaping plant functional traits by indirectly affecting plant growth through their influence on the redistribution of climatic factors such as light, temperature, and precipitation (Yang et al., 2014). Key topographic factors affecting plant growth include elevation, slope, and slope direction (Zhong et al., 2019). In regions characterized by complex topography, diverse habitats are formed by various topographic factors (Fang et al., 2004), leading to complex and variable effects on plant leaf functional traits (Kikuchi and Miura, 1993; Hara et al., 1996; Yang and A., 2006). Investigating the response mechanisms of plant leaf functional traits to different topographic factors can enhance our understanding of plants’ adaptation strategies and ecological responses to the environment (Qin and Shangguan, 2019).
Soil provides nutrients and a growth environment for terrestrial plants (Pandey, 2015). There were experiments on climate and plant response in the Arctic showed that soil fertility has an effect on plant biomass, and leaf stoichiometry traits (Dormann and Woodin, 2002). The supply of nutrient elements from the surrounding environment is in relative balance with the elemental content in biological organisms, thus the elemental content of the soil affects the elemental stoichiometry characteristics of plant leaves and other organs. Nutrient content in soil factors Reflects the degree of soil fertility, and its content is a good indicator of the ecological stoichiometry of plant leaf nutrients (Yang et al., 2019). Additionally, soil pH value influences soil nutrient element content and, to some extent, plant growth (Tahmasebi Kohyani et al., 2008). Exploring the response of plant functional traits to topographic changes can reveal plants’ adaptation strategies to the environment, providing a basis for ecological restoration in areas with complex topography.
The South China Karst region is recognized as one of the world’s largest exposed carbonate rock areas, covering approximately 1.9 million km2 (Brandt et al., 2018). Karst, characterized by its unique geomorphology, represents an area with highly developed karst features and an extremely fragile ecosystem (Legrand, 1973). This region is distinguished by low environmental carrying capacity, shallow soils, sparse vegetation, and limited water storage capacity (Zeng et al., 2007). Due to the distinctive geological background and the binary hydrogeological structure leading to rapid water infiltration, plants face challenges in accessing sufficient water, resulting in slow growth (Chen and Wang, 2008). Over time, plant species in karst areas have undergone long-term natural selection and have developed characteristics such as lithogenicity, calcium preference, and tolerance to barren conditions (Wang et al., 2003), which are reflected in their leaf traits as unique adaptation mechanisms. However, only relatively few studies have investigated leaf trait analysis at the plant community level in karst areas, and the mechanisms by which plant communities respond to spatial heterogeneity and environmental change remain unclear. Quantifying leaf traits and their environmental drivers at the community level can help to explore the relationship between karst vegetation and the environment in greater depth and contribute to the restoration of degraded ecosystems. In this study, we established a 25-ha forest plot in Mulun National Natural Reserve, located in Karst region of southwestern China, focusing on 14 leaf traits of woody plants. The primary objectives were to assess the spatial distribution of functional traits at the community level, examine their associations with environmental factors. We hypothesized that the spatial pattern of leaf trait CWM is more variable under different microhabitats in karst areas and that there are discrepancies in the contributions of different environmental factors. To test this hypothesis, we measured leaf morphology and elemental characteristics of 144 species (≥25 individuals) in various microhabitats in the Karst region of southwest China. Specifically, our objectives were: (a) to evaluate the spatial distribution patterns of different leaf trait CWMs across different habitats; (b) to explore the correlation between plant leaf trait CWMs and environmental factors, (c) to quantify the contribution of environmental factors in explaining the variation in leaf traits CWM, and to analyze their main effects on functional traits of the community.
2 Materials and methods
2.1 Study site
The study site is situated in the Mulun National Nature Reserve, Huanjiang County, Guangxi Province, China (25°7’-25°12’N, 107°54’-108°5’E). The area is characterized by a subtropical monsoon climate, with a mean annual temperature (MAT) ranging of 15.0°C to 18.7°C, and a mean annual precipitation (MAP) ranging of 1530 mm to 1820 mm. Typically, precipitation occurs on 130-140 days per year, primarily between May and September. In 2014, a 25-ha (500 m × 500 m) dynamic sample plot was established in Mulun National Nature Reserve, following the standard field protocol of the Center for Tropical Forest Science (CTFS). The topographic features of the dynamic plot exhibit karst peak cluster depressions, resulting in variable terrain and complex habitats. In the sample plot, there were 108,667 individual woody plants with diameter at breast height (DBH) ≥1 cm, belonging to 227 species in 147 genera of 61 families. Dominant species within the plot include Cryptocarya macrocarpa, Itoa orientalis, Platycarya longipes, and Lindera communis (Du et al., 2017; Lu et al., 2021).
2.2 Species selection and leaf traits measure
The 144 species with a number of individuals greater than or equal to 25 in the plot were selected and investigated. To ensure representative sampling, 10-15 mature and well-grown individuals of each tree species were randomly selected from the sample site. The spatial distribution of the selected individuals covered various habitat types within the plot, providing a comprehensive representation of the forest stand’s diameter structure.
Following the guidelines from Pérez-Harguindeguy’s functional trait collection manual and the standards of the Center for Tropical Forest Science (CTFS), leaf samples were collected from the south, north, east, and west sides of the canopy where there was minimal shading. A minimum of 20 leaves were collected from each selected plant. Leaf area (LA, mm2) and leaf length-width ratio (LW) were measured using the ZhongJing SM I800 Plus scanner and the Wanshen LA-S series plant image analysis system. Leaf thickness (LT, mm) was measured using a thickness gauge, avoiding the leaf veins during measurement. The collected leaves were placed in envelopes and then dried in a laboratory oven at 60°C until a constant weight was reached (approximately 48 hours). The dry weight of the leaves was recorded. For further analysis, the dried leaf samples were ground to 100 meshes using a ball mill. The element content of the samples was determined using various methods: leaf carbon (LC) and nitrogen (LN) concentration were analyzed using an element analyzer, phosphorus content was determined using ammonium molybdate spectrophotometry, and the concentration of elements such as potassium (LK), calcium (LCa), magnesium (LMg), aluminum (LAl), iron (LFe), zinc (LZn), manganese (LMn), sodium (LNa), and sulfur (LS) were analyzed using the ICP-OES - 5110 instrument.
2.3 Environmental variables
To establish a grid system, Real-time kinematic (RTK) and total station measurements were used to measure and fix 10m × 10m grid points, resulting in a total of 2601 points. The average elevation (ELE) of each quadrat (20m×20m) was obtained by calculating the average values of the four corner points. The slope (SLO) and slope aspect (ASP) within each quadrat were measured using a compass. The concavity (CON) was calculated as the difference in elevation between the average elevation of the target quadrat and the average elevation of the surrounding eight quadrats. The rock outcrop rate (ROC) was estimated based on field observations within each quadrat. The terrain wetness index (TWI) was calculated using the system for automated geoscientific analyses (SAGA GIS).
For soil analysis, a total of 625 soil samples were collected from the center of the 20 m sample plots and the center of four smaller plots (5 points in total). The samples were taken from the 0-10 cm surface soil layer. The collected soil samples were screened using a 2 mm mesh, air-dried in a ventilation chamber, and then ground in a ball mill for chemical analysis. Several soil parameters were measured in the mixed soil samples, including soil pH, soil organic carbon (SOC), soil available nitrogen (AN), soil available phosphorus (AP), soil available potassium (AK), and the concentrations of five chemical elements (nitrogen, phosphorus, potassium, calcium, and magnesium). The soil pH was measured using the electrode potential method. SOC was determined using the potassium dichromate oxidation-external heating method. Total nitrogen (TN) was measured using the semi-micro Kelvin method-flow injection instrument method. Total phosphorus (TP) was analyzed using the NaOH melting - molybdenum-antimony anti-chromogenic - ultraviolet spectrophotometry. AN was determined using the alkali-hydrolysis-diffusion method. AP was measured using the molybdenum-antimony anti-chromogenic - ultraviolet spectrophotometry. AK was determined using atomic absorption method. Calcium and magnesium concentrations were analyzed using inductively coupled plasma emission spectrometry (ICP).
2.4 Statistical analysis
Leaf traits were analyzed at the community level to explore spatial characteristics and their responses to the environment. At the community level, CWM was used to evaluate the trait values in leaves. The CWM of the trait values were calculated for each plot j as follows:
where Wij is the weighted average of the sum of DBH of species i in plot j, and traitij is the leaf trait value of species i in plot j.
Pearson correlation analysis was used to analyze the correlation between the functional traits of the species and environmental factors. And applied a redundancy analysis (RDA) to explore the association between explanatory variables (environmental factors) and variations in leaf traits CWM. Moran’s eigenvector maps (MEM) is an improvement of the traditional principal coordinate neighbor matrix (PCNM), which is based on a truncated Euclidean distance matrix. Distance-based Moran’s eigenvector maps (dbMEM) was utilized as a spatial variables. We using Variance Partitioning Analysis (VPA) to partition the environmental sources of variance driving the differences found in leaf traits CWM, allowing testing the proportion of variation attributable to environmental effects, interaction effects, and unexplained effects.
Above analyses were conducted in the R 4.2.2 R Core Team. (2021). The calculation of CWM was calculated with the “FD” package (Laliberté et al., 2014), the dbMEM were calculated using “adespatial” package (Dray et al., 2020), the RDA analysis and VPA analysis were performed using the “vegan” package (Oksanen et al., 2015), the Hierarchical Partitioning analysis was calculated using “rdacca.hp” package (Lai et al., 2022).
3 Results
3.1 Spatial pattern of leaf traits
The distribution of trait values CWM in the dynamics plot exhibited distinct spatial patterns (Figure 1). For traits such as LA, SLA, LW, LC, LN, LP, and LK, the CWM values were higher in depression areas and lower in slope and summit areas. In contrast, traits like LT, LTD, C/N, and C/P exhibited lower values in depression areas but higher values in slope and summit areas. Additionally, the CWM values for LCa and LMg, as well as N/P, were higher in slope areas and lower in depression and summit areas.
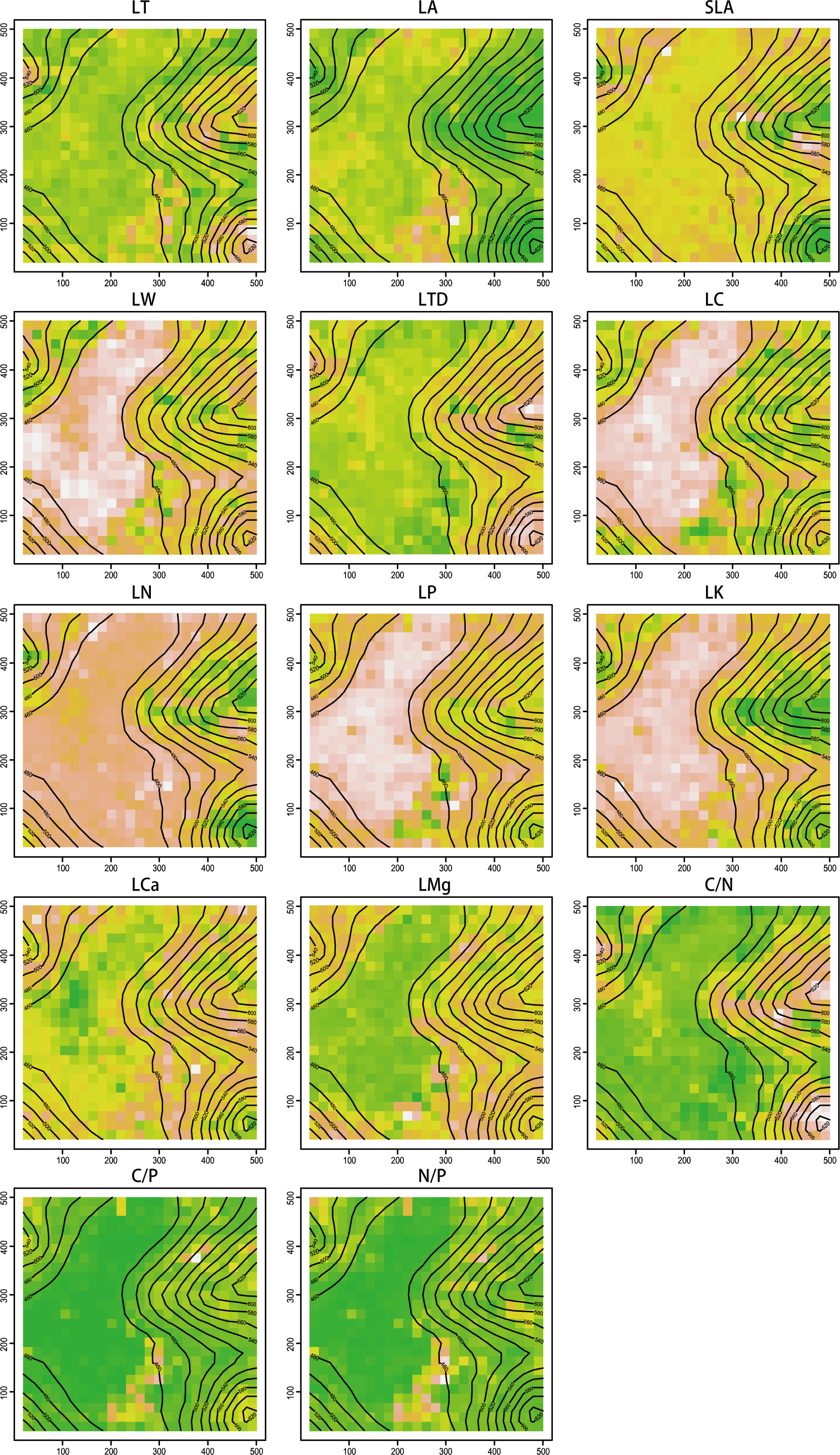
Figure 1 Spatial distribution pattern of function traits CWM. Green represents lowest content, followed by yellow, orange, pink, and the highest is white.
3.2 Correlation between traits and environment
The correlation between the CWM of traits and various environmental factors exhibited variability, with terrain factors generally showing stronger correlations compared to soil factors. Among all the factors, ELE exhibited the highest correlation with trait CWM values (Figure 2). Elevation was significantly positively correlated with LT, LTD, LCa and LMg, as well as C/N, C/P, and N/P. Conversely, elevation showed a significant negative correlation with LA, SLA, LW, as well as LC, LN, LP, and LK. Regarding other factors, SLO, ASP, CON, ROC, soil pH, SOC, and TN, as well as Mg, exhibited similar correlation trends as elevation. The TWI, TP, TK, and AP showed an opposite correlation trend compared to elevation. AK, AN, and Ca displayed a slightly different trend, mainly reflected in their correlation with LTD and C/N ratios.
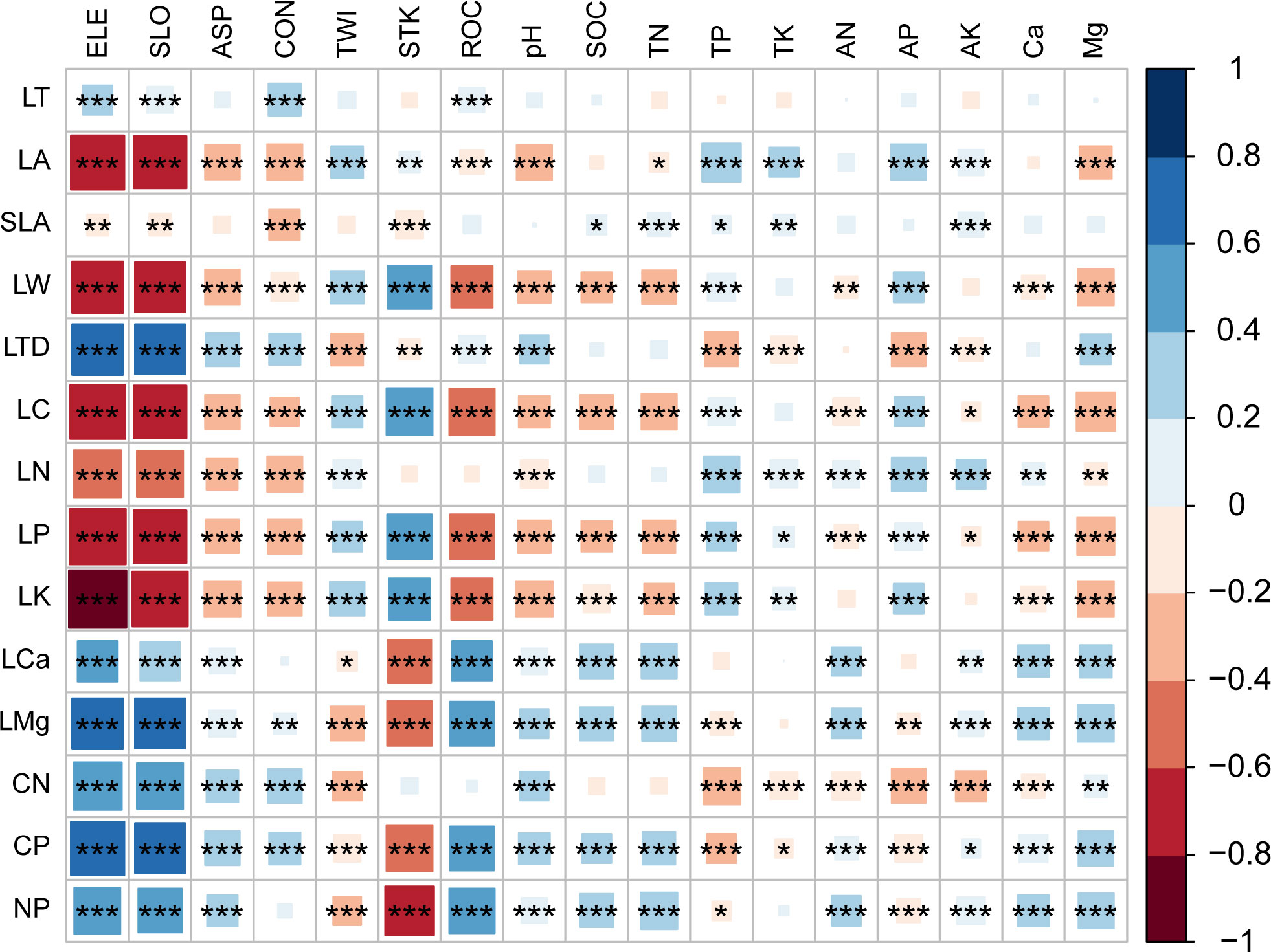
Figure 2 Correlation coefficients between environment factors and traits CWM. ELE, average elevation; SLO, slope; ASP, aspect; CON, convexity; TWI, topographic wetness index; STK, soil thick; ROC, rock outcrop ratio; pH, soil pH value; SOC, soil organic carbon; TN, soil total nitrogen; TP, soil total phosphorus; TK, soil total potassium; AN, soil available nitrogen; AP, soil available phosphorus; AK, soil available potassium; Ca, Soil calcium content; Mg, Soil magnesium content; LT, leaf thickness; LA, leaf area; SLA, specific leaf area; LW, leaf length to width ratio; LTD, leaf tissue density; LC, leaf carbon content; LN, leaf nitrogen content; LP, leaf phosphorus content; LK, leaf potassium content; LCa, leaf calcium content; LMg, leaf magnesium content; CN, leaf carbon to nitrogen ratio; CP, leaf carbon to phosphorus ratio; NP, leaf nitrogen to phosphorus ratio. * P<0.05; **P<0.01; ***P<0.001.
3.3 Effects of environmental factors on traits CWM
The topographic RDA revealed that the first and second axes explained 50.49% and 5.73% of the variance in functional traits, respectively, contributing to a cumulative explanation of 56.22% (Figure 3). On the other hand, the soil RDA accounted for 16.27% of the variance in the first axis and 2.41% in the second axis, resulting in a total contribution of 18.68%. Concerning topographic factors, the first axis explained most of the total variance and was negatively correlated with both SLO and ELE. While ROC and STK showed an opposite trend to the trait CWM values, contributing significantly to the second axis. Among all the topographic factors, elevation had the highest contribution value (19.96%), while TWI had the lowest contribution (1.29%). Additionally, the shared contribution of ELE and SLO accounted for 7.7% of the total variation (Appendix S1). Regarding soil factors, only soil pH showed a negative correlation with the second axis. TN and SOC made significant contributions to the second axis. Among all the soil factors, TP had the highest individual contribution (4.35%), while AN had the lowest (0.37%). The highest contribution of all the pure and shared environmental factors was attributed to TP at 2.9% (Appendix S1).
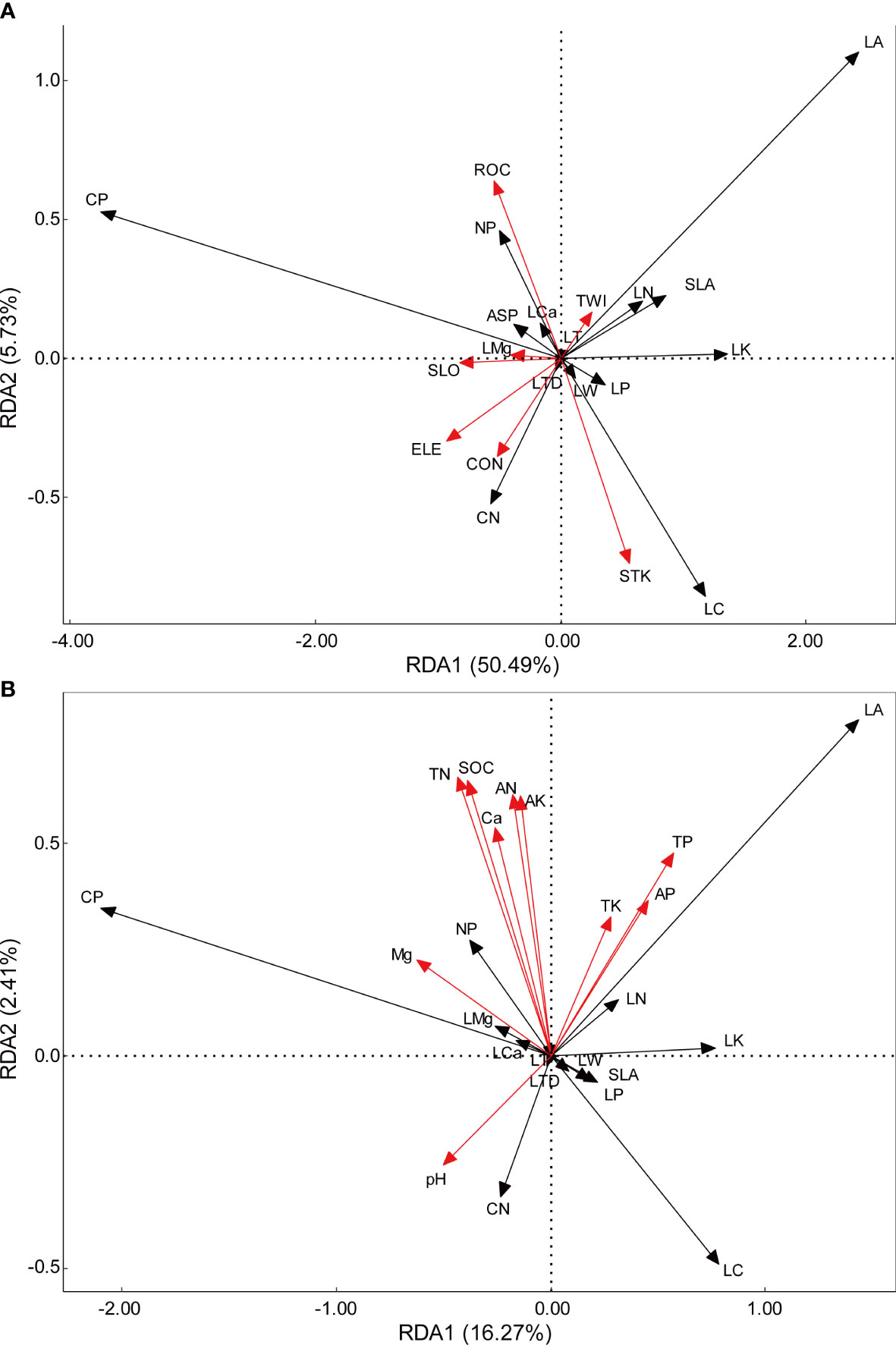
Figure 3 Redundancy analysis of the effects of topography factors (A) and soil factors (B) on leaf traits CWM. Red arrows represent environmental factors, black arrows represent leaf traits.
The Variance Partitioning Analysis was conducted to assess the proportion of variation in functional trait values explained by topography, soil, and spatial factors at the plant community level. It was found that the highest percentage of the variation in LA, LN, LP, LK, C/N, and C/P was explained by the interaction of topographic and spatial factors (Figure 4), with 37-47% of the explanation. On the other hand, purely spatial factors were the largest explanatory factors for LT, SLA, LW, LTD, LC, LCa, LMg, and N/P with 24-36% of the explanation. Overall, the combined effect of the interaction between topography factors and spatial factors, along with pure spatial factors, accounted for the majority of the observed variations in leaf traits. Interestingly, the interaction between topography factors and soil did not explain any of the observed variables across all traits.
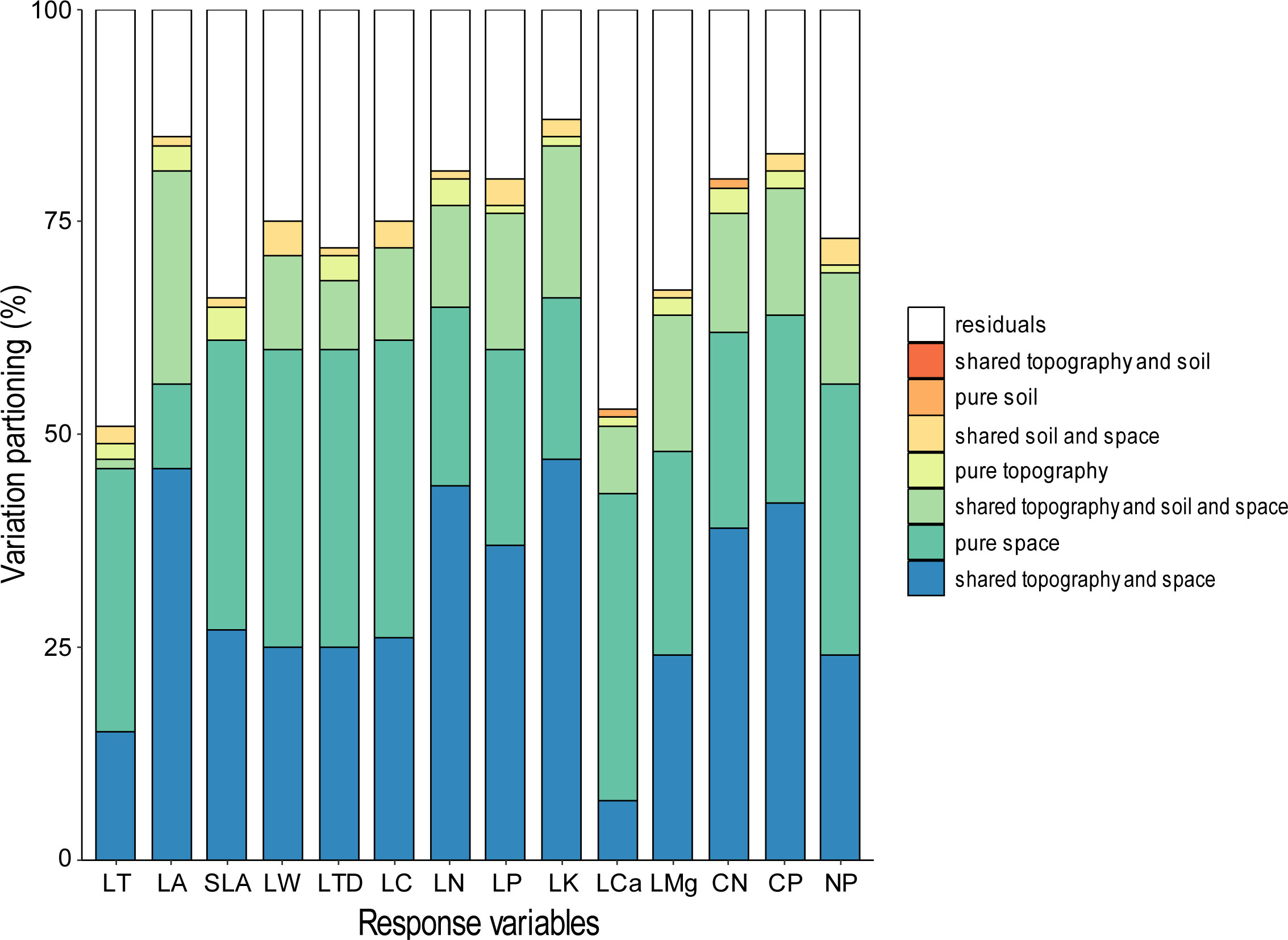
Figure 4 Individual contribution of primary parameters to the proportion (%) of variation explained in leaf traits CWM in plots.
4 Discussion
In this study, the community-level functional traits showed distinct distribution patterns within the 25 ha sample plots, and their patterns were largely influenced by the ELE. Three broad distribution patterns of leaf traits were observed: high values in depressions but low values in hillsides and hilltops, high values in hillsides and hilltops but low values in depressions, and high values in hillsides but low values in hilltops and depressions. The CWM of LA, SLA, and LW were higher in the depression areas. This may be attributed to plants allocating more resources to leaf production in environments with greater light competition, resulting in larger leaf areas to enhance their competitive advantage (Wilsey, 1996). On the other hand, LT and LTD exhibited higher values at hilltops and lower values in depressions, indicating that plants in hilltop environments adopt thicker leaf structures to cope with drier conditions and maintain better drought resistance (Poorter et al., 2009). The elemental traits of leaves are closely related to soil chemical properties. Depressions tend to have thicker soil layers and better nutrient conditions due to rainfall flushing and gravity, leading to higher LC, LN, LP, and LK concentrations in these areas (Hinsinger, 2001; Coelho et al., 2018). The high rock exposure rate in karst slope habitats accelerates the decomposition of Ca elements in limestone due to intense rainfall and weathering, resulting in higher Ca content in soils on slopes (Li et al., 2004). Vegetation cover, well-developed plant roots, and rich soil organic matter on hillsides promote karstic processes and elemental migration, leading to higher Mg content in slope soils, which positively affects leaf Ca and Mg concentrations (Li et al., 2004; Huang et al., 2016). The higher leaf C:N and C:P ratios observed at hilltops indicate that plant leaves at the summit have relatively higher carbon content. Carbon content is often associated with the construction of leaf structures, suggesting that leaves at the summit contain more carbon-based secondary compounds such as lignin and tannin (Poorter and De Jong, 1999), which is consistent with the higher leaf tissue density observed at the summit. N content was positively correlated with rock exposure, resulting in higher LN content on slopes with a higher rock outcrop rate, leading to higher leaf N:P ratios on slopes (Zhang et al., 2007). Overall, the distribution patterns of community-level functional traits in the dynamics plot are shaped by the interplay between topographic factors, soil properties, and environmental conditions. Elevation, in particular, plays a significant role in driving these patterns, influencing various leaf traits and their elemental concentrations across different habitat types within the study area.
During the long-term evolution of plants, different traits exhibit correlations with the environment, reflecting the adaptability of plant functional traits to specific ecological conditions (Reich, 2014). In this study, the functional trait values CWM showed significant correlations with various environmental factors, albeit with variations among different traits. Overall, the correlations between leaf trait CWM and environmental factors can be broadly categorized into two groups. The first group of leaf traits showed negative correlations with ELE, SLO, ASP, CON, ROC, pH, SOC, TN, AN, Ca, and Mg. These traits were negatively correlated with TWI, STK, TP, and AP. On the other hand, the second group of leaf traits exhibited opposite correlations with these environmental factors. LA and LW were negatively correlated with most topographic factors but positively correlated with TWI and STK. This suggests that plants in karst areas tend to increase leaf area in areas with abundant nutrients and better water conditions to enhance their competitiveness. LT and LTD, which are associated with drought resistance, showed positive correlations with ELE, SLO, and ASP, and negative correlations with TWI, reflecting plants’ strategies to resist leaf damage and enhance drought resistance under dry and hot conditions (Comstock and Mencuccini, 1998).
LC, LN, LP, and LK concentrations exhibited similar correlations with environmental factors, with their correlations with topographic factors coinciding with the high or low content of soil elements in different topographies. Among the soil factors, SOC, TN, and AN showed negative correlations with LC, LP, and LK. This could be attributed to plants in depressions, which have higher soil nutrient elements and tend to adopt a resource acquisition strategy, resulting in lower leaf reserve capacity and lower nutrient element content (Navas et al., 2010). LCa and LMg concentrations were positively correlated with most environmental factors, except TWI, STK, TP, and AP. Thicker soils tend to have better water storage capacity, suggesting weaker weathering of calcium and magnesium in such soils, resulting in lower soil Ca and Mg contents and consequently affecting leaf Ca and Mg concentrations. The results of this study provide insights into the correlations between plant leaf functional traits and different environmental factors. This understanding contributes to uncovering the adaptation strategies and ecological responses of plants to their environments, thereby providing a theoretical foundation for ecological restoration and biodiversity conservation in karst regions.
In this study, variation partitioning analysis revealed that a significant portion of the variation in leaf trait CWM could be explained by the combined effects of topography and spatial factors. Different topographic features, such as ELE, ASP, and STK, play important roles in driving vegetation patterns at the landscape scale (Li et al., 2013). Moreover, as the residual effects of human disturbance diminish along the vegetation succession gradient, the intensity of topographic factors increases, making them reliable predictors of natural vegetation recovery. The spatial pattern of community dynamics can be influenced by both environmental and spatial processes, as described by the neutral theory (Hubbell, 2006). According to the neutral theory, the spatial structure of species composition is primarily shaped by dispersal rather than habitat filtering (McGill et al., 2006). Some studies have shown that environmental gradients significantly affect species composition in subtropical karstic regions (Guo et al., 2017).
However, in this study, spatial processes were found to have a greater contribution to species composition, suggesting that spatial processes are the dominant drivers of community composition. It is important to note that the contribution of spatial processes may also include the effects of unmeasured environmental variables, such as sunlight and soil microbes, which strongly influence plant growth and species distribution in arid regions (Jafari et al., 2004; Yang et al., 2020). In the context of this study, most environmental processes were found to interact with spatial processes. The depressions between karst hills in the study plots created a habitat gradient with a distinct spatial pattern. This gradient likely contributes to the observed variations in species composition and community dynamics. The unique environmental conditions and spatial configuration of the depressions may create microhabitats that support specific plant species and promote species aggregation. These spatial processes, along with environmental factors, play a crucial role in shaping the community structure in the study area.
5 Conclusions
In this study, we revealed the spatial distribution of CWM for leaf traits. The differences in the distribution of different traits reflect the adaptation of plants to the special karst habitat and the results of long-term evolution. The relative contributions of environmental and spatial processes in subtropical karst forests were synthesized. It showed that the correlation between topographic factors and leaf trait CWM was higher than that of soil factors, with the strongest correlation between elevation and slope, environmental and spatial processes jointly contributed to the spatial pattern of plant leaf trait CWM. The results reveal the relationship between the relative contributions of ecological niche and spatial processes and species diversity. The results also highlight the importance of topographic moisture indices in shaping karst forest community structure, and the study of the driving mechanisms of species coexistence in karst forest ecosystems can help improve understanding of plant community construction and provide important insights for vegetation restoration.
Data availability statement
The raw data supporting the conclusions of this article will be made available by the authors, without undue reservation.
Author contributions
Conceptualization, HD and QL. Funding acquisition, FZ and HD. Investigation, QL, HD, LS, ZL, WP. Methodology, FZ, KW. Writing – original draft, QL and HD. All authors contributed to the article and approved the submitted version.
Funding
This study was supported by the National Key Research and Development Program of China (2022YFF1300703), National Natural Science Foundation of China (42071073, 42277245), Youth Innovation Promotion Association of the Chinese Academy of Sciences (2021366), Natural Science Foundation of Guangxi Province (2023GXNSFAA026109), Hechi Distinguished Expert Program to FZ.
Conflict of interest
The authors declare that the research was conducted in the absence of any commercial or financial relationships that could be construed as a potential conflict of interest.
Publisher’s note
All claims expressed in this article are solely those of the authors and do not necessarily represent those of their affiliated organizations, or those of the publisher, the editors and the reviewers. Any product that may be evaluated in this article, or claim that may be made by its manufacturer, is not guaranteed or endorsed by the publisher.
Supplementary material
The Supplementary Material for this article can be found online at: https://www.frontiersin.org/articles/10.3389/fevo.2023.1230819/full#supplementary-material
References
Ackerly D. D., Knight C. A., Weiss S. B., Barton K., Starmer K. P. (2002). Leaf size, specific leaf area and microhabitat distribution of chaparral woody plants: contrasting patterns in species level and community level analyses. Oecologia 130 (3), 449–457. doi: 10.1007/s004420100805
Bennie J., Huntley B., Wiltshire A., Hill M. O., Baxter R. (2008). Slope, aspect and climate: Spatially explicit and implicit models of topographic microclimate in chalk grassland. Ecol. Model. 216 (1), 47–59. doi: 10.1016/j.ecolmodel.2008.04.010
Brandt M., Yue Y., Wigneron J. P., Tong X., Tian F., Jepsen M. R., et al. (2018). Satellite-observed major greening and biomass increase in south China karst during recent decade. Earths. Future 6 (7), 1017–1028. doi: 10.1029/2018EF000890
Chen H., Wang K. (2008). Soil water research in karst mountain areas of southwest China. Res. Agric. Modernization. 29 (6), 734–738. doi: 10.3969/j.issn.1000-0275.2008.06.023
Coelho M. S., Carlos P. P., Pinto V. D., Meireles A., Negreiros D., Cerdeira Morellato L. P., et al. (2018). Connection between tree functional traits and environmental parameters in an archipelago of montane forests surrounded by rupestrian grasslands. Flora 238, 51–59. doi: 10.1016/j.flora.2017.04.003
Comstock J., Mencuccini M. (1998). Control of stomatal conductance by leaf water potential in Hymenoclea salsola (T & G), a desert subshrub. Plant Cell Environ. 21 (10), 1029–1038. doi: 10.1046/j.1365-3040.1998.00353.x
Cornelissen J. H. C., Lavorel S., Garnier E., Diaz S., Buchmann N., Gurvich D. E., et al. (2003). A handbook of protocols for standardised and easy measurement of plant functional traits worldwide. Aust. J. Bot. 51 (4), 335–380. doi: 10.1071/BT02124
Diaz S., Hodgson J. G., Thompson K., Cabido M., Cornelissen J. H. C., Jalili A., et al. (2004). The plant traits that drive ecosystems: Evidence from three continents. J. Vegetation. Sci. 15 (3), 295–304. doi: 10.1111/j.1654-1103.2004.tb02266.x
Dormann C. F., Woodin S. J. (2002). Climate change in the Arctic: using plant functional types in a meta-analysis of field experiments. Funct. Ecol. 16 (1), 4–17. doi: 10.1046/j.0269-8463.2001.00596.x
Dray S., Bauman D., Guillaume B., Borcard D., Clappe S., Guillaume G., et al. (2020). adespatial: Multivariate Multiscale Spatial Analysis. R package version 0.3-8. Ecol. Monogr. 82 (3), 119598. doi: 10.1890/11-1183.1
Du H., Hu F., Zeng F., Wang K., Peng W., Zhang H., et al. (2017). Spatial distribution of tree species in evergreen-deciduous broadleaf karst forests in southwest China. Sci. Rep. 7. doi: 10.1038/s41598-017-15789-5
Fang J., Shen Z., Cui H. (2004). Ecological characteristics of mountains and research issues of mountain ecology. Chin. Biodivers. 12 (1), 10–19. doi: 10.17520/biods.2004003
Garnier E., Cortez J., Billes G., Navas M. L., Roumet C., Debussche M., et al. (2004). Plant functional markers capture ecosystem properties during secondary succession. Ecology 85 (9), 2630–2637. doi: 10.1890/03-0799
Guo Y., Wang B., Mallik A. U., Huang F., Xiang W., Ding T., et al. (2017). Topographic species-habitat associations of tree species in a heterogeneous tropical karst seasonal rain forest, China. J. Plant Ecol. 10 (3), 450–460. doi: 10.1093/jpe/rtw057
Hara M., Hirata K., Fujihara M., Oono K. (1996). Vegetation structure in relation to micro-landform in an evergreen broad-leaved forest on Amami Ohshima Island, south-west Japan. Ecol. Res. 11 (3), 325–337. doi: 10.1007/BF02347790
He N., Zhang J., Liu C., Xu L., Chen Z., Liu Y., et al. (2018). Patterns and influencing factors of traits in forest ecosystems: Synthesis and perspectives on the synthetic investigation from the north-east transect of eastern China (NETEC). Acta Ecol. Sin. 38 (18), 6359–6382. doi: 10.5846/stxb201803140507
Hinsinger P. (2001). Bioavailability of soil inorganic P in the rhizosphere as affected by root-induced chemical changes: a review. Plant Soil 237 (2), 173–195. doi: 10.1023/A:1013351617532
Huang F., Ding T., Li X., Gou Y., Wang B., Xiang W., et al. (2016). Species diversity for various associations along an altitudinal gradient in the karst seasonal rainforest in Nonggang. Acta Ecol. Sin. 36 (14), 4509–4517. doi: 10.5846/stxb201411232324
Hubbell S. P. (2006). Neutral theory and the evolution of ecological equivalence. Ecology 87 (6), 1387–1398. doi: 10.1890/0012-9658(2006)87[1387:NTATEO]2.0.CO;2
Ives A. R., Zhu J. (2006). Statistics for correlated data: Phylogenies, space, and time. Ecol. Appl. 16 (1), 20–32. doi: 10.1890/04-0702
Jafari M., Chahouki M. A. Z., Tavili A., Azarnivand H., Amiri G. Z. (2004). Effective environmental factors in the distribution of vegetation types in Poshtkouh rangelands of Yazd Province (Iran). J. Arid. Environ. 56 (4), 627–641. doi: 10.1016/S0140-1963(03)00077-6
Keitt T. H., Urban D. L. (2005). Scale-specific inference using wavelets. Ecology 86 (9), 2497–2504. doi: 10.1890/04-1016
Kikuchi T., Miura O. (1993). Vegetation patterns in relation to microscale landforms in hilly land regions. Vegetatio 106 (2), 147–154. doi: 10.1007/BF00045068
Lai J., Zou Y., Zhang J. P., Peres-Neto P. (2022). Generalizing hierarchical and variation partitioning in multiple regression and canonical analysis using the rdacca.hp R package. Methods Ecol. Evol. 13 (4), 782–788. doi: 10.1111/2041-210X.13800
Laliberté E., Legendre P., Shipley B. (2014). FD: Measuring functional diversity from multiple traits, and other tools for functional ecology Vol. 1 (R package version), 0–12.
Legendre P., Dale M. R. T., Fortin M. J., Gurevitch J., Hohn M., Myers D. (2002). The consequences of spatial structure for the design and analysis of ecological field surveys. Ecography 25 (5), 601–615. doi: 10.1034/j.1600-0587.2002.250508.x
Legrand H. E. (1973). Hydrological and ecological problems of karst regions. Science 179 (4076), 859–864. doi: 10.1126/science.179.4076.859
Li E., Jiang Z., Cao J., Jiang G., Deng Y. (2004). The comparison of properties of Karst soil and Karst erosion ratio under different successional stages of Karst vegetation in Nongla, Guangxi. Acta Ecol. Sin. 24 (6), 1131–1139. doi: 10.3321/j.issn:1000-0933.2004.06.006
Li Y., Zhao C., Dong X., Hou Z., Ma X., Zhang Q. (2013). Responses of Stellera chamaejasme twig and leaf traits to slope aspect in alpine grassland of Northwest China. Chin. J. Ecol. 32 (12), 3145–3151. doi: 10.13292/j.1000-4890.2013.0483
Liu X., Ma K. (2015). Plant functional traitsConcepts, applications and future directions. Sci. Sin. Vitae. 45 (4), 325–339. doi: 10.1360/N052014-00244
Lu M., Du H., Song T., Peng W., Su L., Zhang H., et al. (2021). Drivers of tree survival in an evergreen-deciduous broadleaf karst forest in southwest China. For. Ecol. Manage. 499. doi: 10.1016/j.foreco.2021.119598
McGill B. J., Maurer B. A., Weiser M. D. (2006). Empirical evaluation of neutral theory. Ecology 87 (6), 1411–1423. doi: 10.1890/0012-9658(2006)87[1411:EEONT]2.0.CO;2
McIntyre S., Lavorel S., Landsberg J., Forbes T. D. A. (1999). Disturbance response in vegetation towards a global perspective on functional traits. J. Vegetation. Sci. 10 (5), 621–630. doi: 10.2307/3237077
Meng T., Jian N. I., Wang G. (2007). Plant functional traits, environments and ecosystem functioning. Acta Phytoecol. Sin. 31 (1), 150–165. doi: 10.17521/cjpe.2007.0019
Navas M. L., Roumet C., Bellmann A., Laurent G., Garnier E. (2010). Suites of plant traits in species from different stages of a Mediterranean secondary succession. Plant Biol. 12 (1), 183–196. doi: 10.1111/j.1438-8677.2009.00208.x
Oksanen J., Blanchet F. G., Kindt R., Legendre P., Minchin P., O'Hara B., et al. (2015). Vegan: community Ecology Package (R Package Version 2.2-1 2), 1–2.
Pandey R. (2015). Plant Biology and Biotechnology: Volume I: Plant Diversity, Organization, Function and Improvement (New Delhi: Springer India), 499–538.
Pang S., Ou Z., Shen W., He F., Lu G. (2021). Leaf function traits variations and adaptive strategies of dominant woody economic plants in karst area of Southwest Guangxi. Guihaia 41 (5), 707–714. doi: 10.11931/guihaia.gxzw202002005
Pierce S., Negreiros D., Cerabolini B. E. L., Kattge J., Diaz S., Kleyer M., et al. (2017). A global method for calculating plant CSR ecological strategies applied across biomes world-wide. Funct. Ecol. 31 (2), 444–457. doi: 10.1111/1365-2435.12722
Poorter H., De Jong R. (1999). A comparison of specific leaf area, chemical composition and leaf construction costs of field plants from 15 habitats differing in productivity. New Phytol. 143 (1), 163–176. doi: 10.1046/j.1469-8137.1999.00428.x
Poorter H., Niinemets U., Poorter L., Wright I. J., Villar R. (2009). Causes and consequences of variation in leaf mass per area (LMA): a meta-analysis. New Phytol. 182 (3), 565–588. doi: 10.1111/j.1469-8137.2009.02830.x
Qin J., Shangguan Z. (2019). Effects of forest types on leaf functional traits and their interrelationships of Pinus massoniana coniferous and broad-leaved mixed forests in the subtropical mountain, Southeastern China. Ecol. Evol. 9 (12), 6922–6932. doi: 10.1002/ece3.5259
R Core Team (2021). R: A Language and Environment for Statistical Computing. R Foundation for Statistical Computing, Vienna, Austria. URL https://www.R-project.org/.
Rawat M., Arunachalam K., Arunachalam A., Alatalo J. M., Pandey R. (2021). Assessment of leaf morphological, physiological, chemical and stoichiometry functional traits for understanding the functioning of Himalayan temperate forest ecosystem. Sci. Rep. 11 (1), 23807. doi: 10.1038/s41598-021-03235-6
Reich P. B. (2014). The world-wide 'fast-slow' plant economics spectrum: a traits manifesto. J. Ecol. 102 (2), 275–301. doi: 10.1111/1365-2745.12211
Reich P. B., Wright I. J., Cavender-Bares J., Craine J. M., Oleksyn J., Westoby M., et al. (2003). The evolution of plant functional variation: Traits, spectra, and strategies. Int. J. Plant Sci. 164 (3), S143–S164. doi: 10.1086/374368
Stahl U., Kattge J., Reu B., Voigt W., Ogle K., Dickie J., et al. (2013). Whole-plant trait spectra of North American woody plant species reflect fundamental ecological strategies. Ecosphere 4 (10), 128. doi: 10.1890/ES13-00143.1
Tahmasebi Kohyani P., Bossuyt B., Bonte D., Hoffmann M. (2008). Importance of grazing and soil acidity for plant community composition and trait characterisation in coastal dune grasslands. Appl. Vegetation. Sci. 11 (2), 179–186. doi: 10.3170/2008-7-18351
Tuda M. (2007). Understanding mechanism of spatial ecological phenomena: a preface to the special feature on "spatial statistics''. Ecol. Res. 22 (2), 183–184. doi: 10.1007/s11284-006-0328-x
Violle C., Enquist B. J., McGill B. J., Jiang L., Albert C. H., Hulshof C., et al. (2012). The return of the variance: intraspecific variability in community ecology. Trends Ecol. Evol. 27 (4), 244–252. doi: 10.1016/j.tree.2011.11.014
Wang S., Li Y., Li R. (2003). Karst rocky desertification: formation background, evolution and comprehensive taming. Quaternary. Sci. 23 (6), 657–666.
Wang X. G., Wiegand T., Hao Z. Q., Li B. H., Ye J., Lin F. (2010). Species associations in an old-growth temperate forest in north-eastern China. J. Ecol. 98 (3), 674–686. doi: 10.1111/j.1365-2745.2010.01644.x
Wilsey B. J. (1996). Urea additions and defoliation affect plant responses to elevated CO2 in a C-3 grass from Yellowstone National Park. Oecologia 108 (2), 321–327. doi: 10.1007/BF00334657
Yang Y., A. L. D. (2006). A brief review of studies on differentiation of vegetation pattern along a topographic gradient in hilly reions. Acta Phytoecol. Sin. 30 (3), 504–513. doi: 10.17521/cjpe.2006.0067
Yang J., El-Kassaby Y. A., Guan W. (2020). Multiple ecological drivers determining vegetation attributes across scales in a mountainous dry valley, southwest China. Forests 11 (11). doi: 10.3390/f11111140
Yang X., Li J., Zhao T., Mo L., Zhang J., Ren H., et al. (2019). Variation and heritability of morphological and physiological traits among Leymus chinensis genotypes under different environmental conditions. J. Arid. Land. 11 (1), 66–74. doi: 10.1007/s40333-018-0018-x
Yang S., Wen Z., Miao L., Qi D., Hua D. (2014). Responses of plant functional traits to micro-topographical changes in hilly and gully region of the Loess Plateau, China. J. Appl. Ecol. 25 (12), 3413–3419.
Zeng F., Peng W., Song T., Wang K., W.U. H., Song X., et al. (2007). Changes in vegetation after 22 years' natural restoration in the karst disturbed area in Northwest Guangxi. Acta Ecol. Sin. 27 (12), 5110–5119. doi: 10.1016/S1872-2032(08)60016-5
Zhang W., Chen H., Wang K., Su Y., Zhang J., Yi A. (2007). The heterogeneity and its influencing factors of soil nutrients in peak-cluster depression areas of karst region. Agric. Sci. China 6 (3), 322–329. doi: 10.1016/S1671-2927(07)60052-2
Zhang J., Zhao N., Liu C., Yang H., Li M., Yu G., et al. (2018). C:N:P stoichiometry in China's forests: From organs to ecosystems. Funct. Ecol. 32 (1), 50–60. doi: 10.1111/1365-2435.12979
Zhong J., Chen J., Chen Q., Ji L., Kang B. (2019). Quantitative classification of MRT,CCA ordination,and species diversity along elevation gradients of a natural secondary forest in the Qinling mountains. Acta Ecol. Sin. 39 (1), 277–285. doi: 10.5846/stxb201712282338
Keywords: leaf trait, CWM, spatial distribution, environmental factor, community assembly, karst ecosystem
Citation: Long Q, Du H, Su L, Zeng F, Lian Z, Peng W, Tan W, Sun R and Wang K (2023) Patterns in leaf traits of woody species and their environmental determinants in a humid karstic forest in southwest China. Front. Ecol. Evol. 11:1230819. doi: 10.3389/fevo.2023.1230819
Received: 29 May 2023; Accepted: 19 July 2023;
Published: 09 August 2023.
Edited by:
Shuai Ouyang, Central South University of Forestry and Technology, ChinaReviewed by:
Guo Yili, Chinese Academy of Sciences (CAS), ChinaMengmeng Gou, Chinese Academy of Forestry, China
Copyright © 2023 Long, Du, Su, Zeng, Lian, Peng, Tan, Sun and Wang. This is an open-access article distributed under the terms of the Creative Commons Attribution License (CC BY). The use, distribution or reproduction in other forums is permitted, provided the original author(s) and the copyright owner(s) are credited and that the original publication in this journal is cited, in accordance with accepted academic practice. No use, distribution or reproduction is permitted which does not comply with these terms.
*Correspondence: Hu Du, hudu@isa.ac.cn