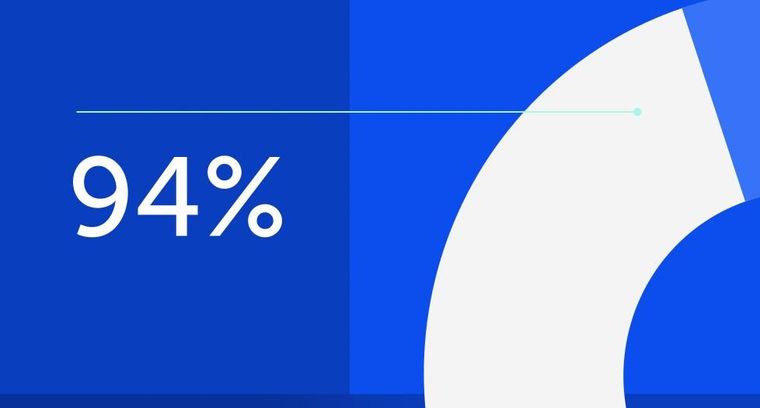
94% of researchers rate our articles as excellent or good
Learn more about the work of our research integrity team to safeguard the quality of each article we publish.
Find out more
ORIGINAL RESEARCH article
Front. Ecol. Evol., 29 September 2023
Sec. Population, Community, and Ecosystem Dynamics
Volume 11 - 2023 | https://doi.org/10.3389/fevo.2023.1199670
Tree species richness, forest structure, and seasonal fluctuations between rainy and dry seasons can strongly affect trophic interactions in forest ecosystems, but the inter- and scale dependence of these variables remains unclear. Using artificial caterpillars (~18,000 replicates), we analyzed predation pressure by arthropods, birds, and rodents along a tree species richness gradient across seasons in a subtropical tree diversity experiment (BEF-China). The aim of the study was to test if forest structure, in addition to tree species richness, has an effect on predation pressure and to further specify which structural variables are important in driving predation. We assessed the effects of tree species richness and forest structure at the plot and local neighborhood levels. We also included fine-scale placement covariates, plot size, and topographical covariates of the study site. Forest structure and tree species richness independently and interactively affected predation pressure. The spatial scale was an important determinant for tree species richness and structural effects, extending from within plot scales to the overall heterogeneity of the plots’ surrounding environment. For example, the effect of branch density in the local neighborhood depended on both surrounding tree species richness and plot-level vegetation density. Similarly, visibility-enhancing factors increased attacks by arthropods (lack of branches in close surroundings) and by birds (open area), depending on the surrounding vegetation. A comparison of structural measures showed that predation pressure can be addressed in much greater detail with multiple specific structural features than with overall forest complexity. Seasonal change also affected predation pressure, with foliage being a stronger attractant in spring, but also by presumable topography-driven study plot differences in sun exposure and humidity between rainy and dry seasons. Our study demonstrates that predation pressure is not simply a function of tree species richness or structure but is shaped by the interplay of structural elements, spatial scale, and seasonal dynamics along gradients of tree species richness and forest structure. The structural and seasonal effects are important to take into account when addressing how current and future biodiversity loss may change top‐down control of herbivory and overall ecosystem functioning.
Globally declining biodiversity is altering ecosystem functions (Hooper et al., 2012), with a loss of plant diversity leading to declining herbivore and predator populations and altered trophic structures (Haddad et al., 2009; Wan et al., 2020). Higher plant species richness can lead to higher predator abundances and top‐down suppression of arthropod herbivory, as demonstrated in agroecosystems and grasslands (Andow, 1991; Siemann et al., 1998; Barnes et al., 2020; but see, e.g., Koricheva et al., 2000; Harmon et al., 2003). This can be due to increased habitat amounts and complexity providing alternate food sources and a more stable prey supply over time, as posited by the “Enemies hypothesis” (Root, 1973). Relationships between plant species richness and higher trophic levels are generally less well explored in forests, with more evidence for the increase of predator abundance than predator activity (Stemmelen et al., 2022). This leaves the general role of tree species richness on actual predation pressure unresolved (Staab and Schuldt, 2020).
Although some studies have shown a positive effect of tree species richness on predation rates by arthropods (Leles et al., 2017) and birds (Muiruri et al., 2016; Nell et al., 2018), others have demonstrated that forest structure is a more important determinant, for example, for bird abundance and richness (Khanaposhtani et al., 2012; Mag and Ódor, 2015). Similar to the effects of increasing plant species richness, predation can increase with increasing vegetation complexity, as also shown in grasslands, through increased niche space, alternate and more abundant food sources, microclimate amelioration, and reduced intra-guild interactions among predators (Flaherty, 1969; Finke and Denno, 2002; Langellotto and Denno, 2004). However, negative effects of habitat complexity on predator and parasite efficiency can arise as well, for example via increased refuge availability for prey in more complex vegetation (Andow and Prokrym, 1990; Hawkins et al., 1993; Clark and Messina, 1998). Studies on the effect of vegetation complexity and density on predators in forests have shown, for example, positive effects on bird abundance and diversity (MacArthur and MacArthur, 1961; Khanaposhtani et al., 2012), spider abundances (Halaj et al., 1998; Halaj et al., 2000), and predation by arthropods (Schwab et al., 2021), but also negative effects on predation by birds and ants (Šipoš and Kindlmann, 2013). Notably, however, comparisons between studies of vegetation complexity tend to be difficult because of differences in the complexity measures (McCoy and Bell, 1991; Loke and Chisholm, 2022). Structural features might play a particularly important role in forest ecosystems because of the wide range of microhabitats in canopies (Wardhaugh et al., 2014), with highly promoted arthropod abundance, vegetation biomass, and structural complexity as compared to herbaceous/grassland vegetation (Lawton, 1983). The structural variation in forests can already differ within and between monocultures due to tree size, density, and species, but is generally higher in tree species mixtures because of interspecific differences in tree crown forms and diversity-driven plasticity of tree crowns (Pretzsch, 2014; Ali, 2019; Kunz et al., 2019).
The effects of plant species richness and vegetation structure on predator abundance are highly scale-dependent (Root, 1973; Russell, 1989; Staab and Schuldt, 2020), which may explain some of the variability in earlier results. Scale dependence has also been shown for the predation activity of birds (Muiruri et al., 2016) and arthropods (Schwab et al., 2021). In forests, besides the size of a plot, area effects already play an important role through tree sizes and vegetation density, as those form the plant surface area which, in turn, determines the predator–prey interaction zone (e.g., Erwin, 1983; Nakamura et al., 2017). A larger area can support greater numbers of species and higher abundances through species–area relationships brought about by an increase in resources, greater habitat variability within a larger area, and a lower likelihood of local extinctions due to larger population sizes (e.g., Connor and McCoy, 2001). Furthermore, small-scale habitat variation can then influence predation through selection of the hunting grounds based on cues from the vegetation (e.g., volatile compounds) (Heinrich and Collins, 1983; Mäntylä et al., 2008; Sam et al., 2015; see also review in Turlings and Erb, 2018) and increased visibility (Tschanz et al., 2005). The effects of vegetation structure on predation rates, in turn, have been found to be dependent on predator and prey densities (Riihimäki et al., 2006; Scheinin et al., 2012). Predators may aggregate at the highest prey densities (Hassell and May, 1974; Kareiva and Odell, 1987), and the movement of predators between habitats of varying prey densities can promote and stabilize predator populations and prevent prey resource depletion where prey densities are high but increase depletion where they are low (Holt, 1985; Pulliam, 1988; Polis et al., 1996). Thus, very low or high predator and prey abundances can make the effect of structural habitat components negligible (McCoy and Bell, 1991). The source–sink interactions between “component communities” (Root, 1973), which the structurally different patches can be seen as, are expected to become stronger the larger the predator population in the source (Pulliam, 1988), the greater the dispersal ability of the predators, and the closer the source and sink patches are to each other (Bianchi et al., 2010). Movement between patches is suggested to be the reason why the positive effects of vegetation diversity observed at small scales are negligible when they occur at larger scales (Bommarco and Banks, 2003). This negative scale dependence of plant species richness has been observed, for example, for predation by birds in forests (Muiruri et al., 2016). However, it remains largely unexplored in most studies of tree diversity–predation relationships.
A given habitat can also vary seasonally in being a source or sink for predators and prey (Pulliam, 1988). Prey abundance and predator activity are highest in early to mid-summer in temperate forests (e.g., Remmel et al., 2009). In the tropics, arthropod abundances are highest in the rainy season, or somewhat after with a delay due to development time, correlating with the flush of new leaves (Janzen, 1973; Wolda, 1978; Richards and Coley, 2007; Valtonen et al., 2013). As the foliage-eating prey are impacted by seasonal food availability (Wolda, 1978), the associated predators need to time their activity according to prey abundance (Janzen, 1973; Polis et al., 1996; Molleman et al., 2016), and as such, predation rates have been observed to peak during these periods (Richards and Windsor, 2007; Molleman et al., 2016). Caterpillar abundance was also observed to be highest in the late rainy season in temperate but seasonally rainy sub-tropical forests (in the same field site and year of the current study) (Anttonen et al., 2022). However, organisms at higher trophic levels are expected to be more susceptible to environmental conditions and able to respond to them based on higher mobility (Menge and Sutherland, 1987), and abiotic conditions can break down the positive correlation between predator and prey abundances (Richards and Windsor, 2007). The dry season can promote arthropod abundances in more humid habitats and cause mobile arthropods to shift from open sites to forests (Janzen, 1973; Richards and Windsor, 2007), where higher canopy cover, and also structurally more complex stands, have lower solar radiation, lower temperatures, and less variation in abiotic conditions (e.g., Breshears, 2006; Ehbrecht et al., 2017).
When looking into the small-scale dynamics of predation pressure, predator abundance and species richness are not necessarily the most accurate predictors due to the source–sink interactions of habitats and small-scale predator efficiency determinants, making direct measurements of predation rates necessary. Artificial caterpillars have proved an effective method for testing predation rates in various types of forests (e.g., Posa et al., 2007; Muiruri et al., 2016; Roslin et al., 2017), allowing reliable assignment of predation to broad taxonomic groups (Low et al., 2014). The method, however, does not necessarily cover the whole extent of predator taxa in the habitats due to a lack of prey movement and olfactory signals (Lövei and Ferrante, 2017; Zvereva and Kozlov, 2023). This approach, nevertheless, allows for efficient comparisons of how predation pressure is shaped by dynamics between patches of different quality, by scale dependence of tree species richness and forest structure, and by seasonal variation in abiotic conditions.
In this study, we tested how tree species richness at different spatial scales (local neighborhood vs. plot), forest structure, and season jointly affect predation pressure on artificial caterpillars in a forest biodiversity–ecosystem functioning experiment in subtropical China (BEF-China; Bruelheide et al., 2014). In this experiment, tree species richness per plot (~26 m × 26 m) was manipulated over a gradient from monoculture to 24-species mixtures. Forest structure was quantified using different approaches: measurements of basal area (BA) of focal and neighborhood trees, tree mortality-based estimates of open area, and terrestrial laser scanning (TLS). Because tree species richness is often expected to influence predation through changes in forest structure, we analyzed the data with and without TLS-derived variables. Additionally, we compared how well predation pressure is explained by the separate measures of tree species richness and forest structural variables to measures of overall vegetation complexity. Moreover, predation pressure may respond to very fine-scale features of vegetation and visibility as well as to larger-scale variables that define the area around the plot. For this reason, we included covariates of fine-scale surroundings around the artificial caterpillar (presence of leaves and branches), of topography (elevation, slope angle, and solar irradiance), and of plot grouping (as a proxy for environmental heterogeneity in the surrounding plots).
We hypothesized that: (1) increasing tree species richness and denser (and more complex) vegetation both increase attacks through a presumed increase in predator abundance and species richness; (2) predation pressure in small-scale habitats varies depending on the large-scale structure, such as visibility promoting attacks in overall denser vegetation. Also, a higher amount of branches at the immediate neighborhood scale is expected to increase attacks through predator aggregation within larger-scale areas where overall predator and prey densities are presumably lowest; (3) predation pressure is highest in the late rainy season and is promoted by new foliage in spring. Season was expected to interact further with vegetation structure and topography, with predation pressure shifting to more humid habitats during the dry season, such as those with a more closed canopy and topographically lower solar irradiance plots. Testing how the interplay between scales of forest structural variables within close neighborhoods affects predation pressure and how these variables are influenced by plant richness will aid in producing a more mechanistic view of the top‐down regulation of arthropod herbivory.
The experiment was conducted in the world’s largest biodiversity–ecosystem functioning experiment (BEF-China) in sub-tropical China (Jiangxi province, 117° 54′ E, 29° 07′ N) (Bruelheide et al., 2014). The annual precipitation in the area is 1,821 mm, and the mean annual temperature is 16.7°C (Yang et al., 2013). The experimental site (“site A” of the BEF-China experiment) was planted in 2009 in a mountainous area, ranging from 105 m to 275 m above sea level (Yang et al., 2013), with an average slope of 27.5° (Bruelheide et al., 2014). The mountain slopes encircle a narrow wetland valley, where the lowest elevation plots are situated. The field site is 14.8 ha in size and consists of 268 plots placed in random order, of which 65 were used in this experiment. Each plot is 25.8 m × 25.8 m in size, which equals the Chinese traditional area unit of 1 mu. Each tree species combination is represented by five plots, of which four are grouped into one continuous unit, so-called “super-plots” (see Bruelheide et al., 2014). The tree replicates used in this study were divided between the 1 mu and 4 mu plots. See Supplementary Figure S1 for a map and an example picture of BEF-China site A and plot setup, and Bruelheide et al. (2014) and Huang et al. (2018) for further details.
Trees within the plots were planted in 20 rows and 20 columns with a 1.29 m distance from each other, in equal amounts per species, and placed in a random design. The tree planting setup followed a “broken stick” design, which halves the tree species richness between levels while keeping the composition otherwise constant, with tree species richness levels of 1, 2, 4, 8, 16, and 24 (Bruelheide et al., 2014). The 24-species mixture is an extra mixture on top of the richness gradient and was included to increase the number and spatial spread of the highest-richness tree replicates. The 24- and 16-species mixtures were combined into a single high species richness level in the analyses and renamed as a 16-species mixture. We used all tree species per plot for predation estimation up to the 16 species mixtures. Additionally, some Castanopsis eyrei Champ. Individuals were recognized to belong to Castanopsis fargesii Franch., increasing the total number of tree species in the experiment to 17. All tree species used in the experiment are listed in Supplementary Table S1.
We analyzed the data based on individual trees, but the focal trees within each tree species richness level were selected as mono- and heterospecific tree species pairs. This design was used to follow the set-up of Trogisch et al. (2021) in order to match the predation estimation with high accuracy with the TLS data examining the structural traits of the trees, for which the paired design was also used (Hildebrand et al., 2021) (see Section 2.3). In the paired design, the trees were selected from the plots at random, but with the requirement that they have either a mono- or heterospecific pair. Both trees were then used for predation estimation. All possible tree species pair combinations were used up to four-species mixtures, and these combinations were then replicated at higher richness levels. Additionally, only tree species pairs with nonoverlapping neighborhoods were selected. The paired design was accounted for with a random effects structure in the models (see Section 2.4).
Predation was estimated in four campaigns: autumn 2018 (August–September), spring (March–April), mid-summer (June), and late summer (August) 2019. The autumn 2018 campaign was set as the last season in analyses due to the campaign being conducted later in autumn than in 2019 (the campaign started on 26 August 2018 vs. 8 August 2019), with leaf senescence starting in the first trees in 2018 but not in 2019. The 2019 spring campaign began for most trees just prior to bud burst and ended when the trees were nearly in full leaf. Spring and mid-summer campaigns were conducted during the rainy season, and late summer and autumn campaigns in the dry season.
The number of tree replicates was 375 in spring, 383 in mid-summer, 380 in late summer, and 384 in autumn, with almost all tree individuals remaining the same between sampling campaigns. The number of trees sampled in total across all 17 species was 96, 113, 50, 74, and 62 for richness levels 1, 2, 4, 8, and 16, respectively, varying between one and 10 (mean = 4.3, SD = 2.4) replicates per species per richness level per campaign. The differences in tree replicate numbers are caused by the experimental design of Trogisch et al. (2021), which focuses on tree species pair interactions and promotes tree species replicate numbers in monocultures (six replicates per species) and two-species mixtures (nine replicates per species) over higher tree species richness levels (five replicates per species), and by variability in self-thinning between tree species in different richness levels. The plot-level tree species richness used in the analyses was based on the planting design but corrected for Schima superba Gardner & Champ.- Rhus chinensis Mill. two-species combinations, where no individuals of R. chinensis were alive and were therefore treated as monocultures. Additionally, the tree species richness was estimated for each tree neighborhood based on the eight closest potential neighbors.
Production, placement, and checking of artificial caterpillars followed the general recommendations by Howe et al. (2009) and Low et al. (2014). The artificial caterpillars were made of green nontoxic and odorless modeling clay (Staedtler® Noris Club® Plasticine green), formed around an iron string, and placed by wrapping the string around branches (Supplementary Figures S2, S3). Caterpillars were ~5 cm long and ~0.5 cm wide, with small variations due to manual production. The artificial caterpillar size was within the range of real caterpillars observed at the field site. Six caterpillars were placed per tree in two groups of three caterpillars each. Caterpillars within one group were placed horizontally no more than 50 cm apart from the central caterpillar of the group, with as little vertical difference as possible. The two caterpillar groups were placed at different height levels as long as the tree height allowed, with an average lower group height of 1.4 m (SD = 0.61) and a higher group height of 2.8 m (SD = 0.85). The height of the caterpillar group was recorded with half a meter accuracy, and this distance from the ground was included in the analyses to account for potentially different movement modes between predator groups. The caterpillars were placed primarily within 20 cm of leaves on branches. If branches with leaves were not within reach, the caterpillar was placed on a leafless branch or, if no branches were within reach, on the trunk of the tree, with a preference for leaves in close proximity (tiny branches). Thus, placement on the trunk indicates increased visibility of the caterpillars due to the absence of branches on focal trees in close proximity to the caterpillars. The location (branch or trunk) and presence of leaves were marked down for each caterpillar.
Each campaign consisted of three rounds, namely placing caterpillars and checking two times. Damaged caterpillars after the first round were either replaced or fixed for the second round. Rainy weather caused delays to the checking schedule due to hazardous conditions, and water droplets on the caterpillars, making small bite marks difficult to detect. Caterpillar placement between consecutive days was divided into different parts of the field site, which ensured that no area with respect to topography was systematically checked later in the season or accumulated disproportionate rain delays. In the case of rain delays, checking was intensified and divided between the delayed and the original schedule caterpillars. In a few cases, a certain tree was accidentally ignored during the intended checking date, leading to increased checking intervals. The average checking period was 8.4 (SD = 1.1), 9.1 (SD = 1.8), 7 (SD = 0.1), and 7.8 (SD = 0.9) days in spring, mid-summer, late summer, and autumn, respectively. The exact length of the checking period for each caterpillar was included in the statistical analyses.
The attack marks were identified to the following broad taxonomic categories: arthropod (Supplementary Figure S2A, C), bird (Supplementary Figures S3A–D), mammal (Supplementary Figures S3E, F) (Low et al., 2014), and snail (Supplementary Figure S2B) (M. Volf, personal communication). The mammal category was subdivided into rodent (Supplementary Figure S3E) and insectivore (likely a bat) (Supplementary Figure S3F) categories. Arthropod attacks were subdivided into two categories by visual observation of clearly distinguishable types of damage. Piercing mandible marks of varying width were considered to have been made by predators (Supplementary Figure S2A). Possible sting marks by Hymenoptera (Low et al., 2014) or Heteroptera were challenging to reliably separate from piercing mandible marks and were, therefore, included in the arthropod predator category. Shallow scraping damage was recognized to be made by grasshoppers in the Caelifera suborder (Supplementary Figure S2C) by frequent observations of them being in contact with the caterpillars and leaving similar damage on thicker tree leaves (Supplementary Figure S2D). This damage could lead to nearly the whole caterpillar being eaten and plasticine frass pellets being excreted on the spot. Obscure bite marks were checked with ×10 and ×20 field magnifiers or photographed for later assessment. Due to checking bite marks in the field, very small arthropod bite marks requiring microscopic examination were likely not noticed (Howe et al., 2009). All caterpillars were checked by the same person, and attacks were recorded as presence–absence.
In total, we placed 20,508 artificial caterpillars. Caterpillars with unrecognizable attack marks (12), being damaged by leaves, etc., or falling from the tree (488) were removed from the data. Furthermore, 2,292 caterpillar checks were removed because of missing structural trait measurements, making the final amount used in analyses 17,728 caterpillars (note that exclusions slightly overlap). Additionally, from the caterpillars kept in the data, attacks by bats were not analyzed because of only a few observations (5). Also, attacks by grasshoppers, despite their commonness (see Section 3), and snails (19 observations), were not analyzed as they represent herbivory.
We aimed to test how forest structure affects predation probability in the immediate neighborhood and at the plot level, and we used several measures to describe the forest surroundings around the artificial caterpillars. The diameter at breast height was measured for the focal trees and the eight potential trees in the immediate neighborhood and transformed to BA. Although the neighborhood BA was estimated only from the immediate neighborhood of the focal trees, it is expected to reflect conditions of the overall plot because of the observed uniformity in low tree species richness plots and the correlation of neighborhood tree species richness on plot tree productivity at high richness levels (Fichtner et al., 2017). Based on BA, 25% of the trees reached >10 m in height at the time of the experiment (Schnabel et al., 2021). Few trees were smaller than 1.3 m, and the DBH was marked down to 0.5 cm for these trees. The open area in the neighborhood represents gaps in the canopy and was estimated by summing up the ground surface areas left open by dead trees around a focal tree. The area assigned to each dead tree was calculated on the basis of the systematic planting pattern and covers ~1.66 m2. Continuous open areas (i.e., ≥ 2 dead trees next to each other) were weighed higher to account for space filling by foliage from neighboring trees, which was achieved by dividing the total open area by the number of gaps left by dead trees.
Further information on vegetation structure was derived from terrestrial laser scanning (TLS) data of the local neighborhood of each focal tree and the overall plot, conducted in February–April 2019 (Hildebrand et al., 2021). At both spatial scales, we aimed to distinguish the effects of the density of vegetation elements and their vertical distribution by using a set of clearly distinguishable structural measures. Neighborhood branch density was estimated as the number of branch-occupied voxels (defining an observed point in three-dimensional space) with a 5 cm edge per 50 cm vertical layer in a 3 m radius for each caterpillar placement height (voxels per layer). Neighborhood canopy packing was estimated as the vertical Gini index of the canopy voxel vertical distribution in a 3 m radius, where lower values indicate a more equal distribution (Ehbrecht et al., 2017) of biomass and thus a less densely packed canopy. At the plot level, the density of vegetation elements was addressed using the mean fractal dimension (MeanFrac) index (Ehbrecht et al., 2017) as a proxy. MeanFrac is a scale-independent measure that increases with a higher perimeter-area ratio, defined to measure structural complexity, and it roughly responds to the space-filling capacity of an object independently of area (Loke and Chisholm, 2022) and, thus, to the density of vegetation elements (Ehbrecht et al., 2017; Zemp et al., 2019). As a scale-independent measure, stands with a high density of branches from small trees can gain the same or higher MeanFrac values than stands with large trees and a higher amount of open space (Ehbrecht et al., 2017; Zemp et al., 2019), which ensures that when using MeanFrac as a proxy for vegetation density, it is not driven directly by biomass. The canopy vertical stratification of the plot was quantified using the effective number of layers (ENL) index (Ehbrecht et al., 2016). For calculating ENL, the voxel point cloud was subdivided into 25 cm slices, the proportion of filled voxels in each slice was quantified, and the inverse Simpson Index was calculated between these layers. ENL values increase with increasing tree height and a more even vertical distribution of space occupation. Thus, the lowest ENL values occurred in the small amounts of plots with very small trees with practically no canopy layer, but the variation of ENL highly increased when trees were larger (Supplementary Figure S4).
Additionally, in comparison to using MeanFrac and ENL separately, we reduced the plot-level structural variables to a single measure of stand structural complexity index (SSCI), which is MeanFrac scaled by ENL (Ehbrecht et al., 2017). For clarity between measures, we refer to MeanFrac as vegetation density and SSCI as canopy complexity. MeanFrac, ENL, and SSCI were aligned relative to the slope angle (see Perles-Garcia et al., 2021). Forest structure can vary based on tree species richness (Williams et al., 2017; Kunz et al., 2019), including canopy vertical stratification, as shown at the BEF-China field site (Perles-Garcia et al., 2021). However, the correlations between tree species richness and structural variables in our study were low enough to allow them to be used in the same GLME models due to the high number of tree species with a wide range in crown forms, growth, and mortality rates. We further reduced tree species richness and all plot and neighborhood-level structural variables, i.e., neighborhood tree species richness, focal BA, neighborhood BA, open area in the neighborhood, voxels per layer, vertical Gini, MeanFrac, and ENL with principal components analysis (all variables scaled) for a measure of overall forest complexity using the function “princomp” in the package “vegan” (Oksanen et al., 2022). The scores of the first three principal components (PC), which together explained ~64% cumulative variance (Supplementary Table S2A), were selected for further analysis. The highest loadings on PC1 were ENL and neighborhood BA, but all other variables also contributed (Supplementary Table S2B). The highest loadings on PC2 were voxels per layer, MeanFrac, and vertical Gini, and on PC3 focal BA, but also with further smaller contributions by other variables.
Plot replicates were divided between 1 mu and 4 mu plots. The smaller plots had a more variable plot neighborhood, as all their eight surrounding plots had a different tree species composition compared to the focal plot, while tree species composition in a 1 mu super-plot was the same as in three of the neighboring plots. Thus, increasing plot size (1 mu vs. 4 mu) can be considered a proxy for a lower variation in tree species diversity and vegetation structure in the more distant neighborhood. Topographical variables, elevation (measured by hypsometer and interpolation from a map using GIS), slope angle (GIS), and solar irradiance (GIS, MWh/m²) (Bruelheide et al., 2013), were also included to account for the differences in the environmental conditions caused by plot placement along slope positions. Slope angle also influences canopy vertical structure, which is not completely addressed by ENL, as the canopy follows a vertical gradient along the slope irrespective of the stratification along the trunks (Lang et al., 2010).
Arthropod predator, bird, and rodent attacks were analyzed using generalized linear mixed-effects models (glmmTMB) (Brooks et al., 2017) (see Supplementary Material 2 for the analysis code). The response variable of being attacked was analyzed as binary using Bernoulli distribution with complementary log-log transformation (link function “cloglog”) in R v 4.2.2 environment (R Core Team, 2022). Random factors used in the models were focal tree species identity and focal trees nested within the plot. Tree species pair identity nested within the plot was tested in comparison to the focal tree, with the models compared by their AIC values.
Before including fixed factors, low collinearity between them was ensured by estimating their internal Spearman correlations (all variables: r < 0.7; see Supplementary Figure S5). In addition, variance inflation was estimated for all fixed factors and their interactions (only variables with vif < 5 were included in the models) with package “performance” (Lüdecke et al., 2021). Further model validations using predicted and observed estimates and residuals were done using the “DHARMa” package (Hartig, 2022). In order to focus on the most important estimates, interactions of fixed factors, but not the covariates, were reduced based on model AIC using the function “drop1”. Fixed factors in all models were season (as an ordinal variable representing shift from early to late growing season and transition from rainy to dry season), tree species richness, neighborhood- and focal-tree BA, open area in the neighborhood, plot size, plot topography, covariates describing the immediate placement conditions, and checking schedule-related covariates: checking round and the number of days the caterpillar was positioned on the tree (delays caused by rainy days). Focal and neighborhood BA were square root-transformed for increased linearity in all models. The presence of leaves, placement on a branch or trunk, and checking round were included in the models as factorials, and all other variables were scaled by subtracting the mean and dividing by the standard deviation. The effect of tree species richness was tested by comparing the AIC values of two different models, one using plot-level richness and the other using the richness of the eight closest potential neighbors. The relationship of tree species richness with structural metrics was further tested by comparing models that either included only tree species richness or additionally included the TLS-derived structural variables. Furthermore, two different models were compared that used either SSCI or MeanFrac and ENL. Finally, due to moderate collinearity between tree species richness and ENL (Spearman r = 0.47 and 0.45 with plot and neighborhood tree species richness, respectively) and between voxels per layer and MeanFrac (Spearman r = 0.6), neighborhood tree species richness and MeanFrac were removed from the reduced arthropod predator model using TLS-derived structural variables in order to see if this has strong effects on the respective moderately collinear covariates.
Furthermore, several interactions between explanatory variables were tested. After ensuring low vif values, for arthropod predator models, these were as follows:
● Interactions of tree species richness with focal and neighborhood tree sizes, voxels per layer, vertical Gini, and MeanFrac.
● Interactions of plot, neighborhood, and fine-scale placement variables. Interactions of voxels per layer and vertical Gini were included with MeanFrac, and interactions of the presence of leaves were included with voxels per layer and ENL but not with MeanFrac due to high vif values. The interaction of MeanFrac and ENL was included with focal and neighborhood tree sizes. The effect that placement on the trunk may have on visibility due to the absence of branches in close proximity to the artificial caterpillar was further tested with interaction with focal and neighborhood tree sizes, all TLS-derived plot- and neighborhood structural variables (that describe the canopy structure surrounding the patches of increased visibility), open area in the neighborhood, and placement height. The interaction of open area in the neighborhood was further included with all plot and neighborhood TLS-derived structural variables, neighborhood tree size, and placement height.
● In the comparative model using SSCI, the same interactions were included as with MeanFrac and ENL except for interactions of vertical Gini and placement on branch or trunk with SSCI and voxels per layer with the presence of leaves, due to increased vif values.
● The interactions of plot size with tree species richness, neighborhood BA, and TLS-derived plot-level structural variables were included. The plot size–tree species richness relationship was also included with neighborhood tree species richness, as it was highly correlated with the plot-level tree species richness (Spearman r = 0.86).
● Seasonal differences in attack probability were tested with tree species richness, all TLS-derived plot and neighborhood structural variables, the presence of leaves, all topographical variables, and checking round and number of days on the tree.
Due to the low number of attacks, bird and rodent models were analyzed with simplified models in order to avoid overfitting and to keep the results generalizable. All the same interactions with season and plot size were used as with arthropod predators, except in the bird model, where the interaction of season and presence of leaves was dropped due to high vif values. Otherwise, interactions were limited to testing the effects of plot-level vegetation density (MeanFrac) and canopy complexity (SSCI). These included tree species richness, focal and neighborhood tree sizes, voxels per layer, vertical Gini, open area in the neighborhood, placement height, presence of leaves, and placement on branch or trunk, except for bird models where the interaction of placement on branch or trunk with SSCI was dropped due to a high vif value.
In the models with neighborhood tree species richness and forest structure reduced to principal components, interactions with PC1, PC2, and PC3 were included with each other, plot size, and season for all predator groups. Additionally, for arthropod predators, interactions of PC1, PC2, and PC3 were included with placement on the branch or trunk, and PC1 and PC3 with the presence of leaves, but not PC2, in order to reduce vif values. Interactions between checking round, days on the tree, and topographical variables were included with season for all predator groups, similar to other models.
Overall, attacks by arthropods were much more frequent than those by any vertebrate predator. Predatory arthropod attack rates per season were 5.2% in spring, 19.8% in mid-summer, 2.4% in late summer, and 9.6% in autumn. Bird and rodent attacks were systematically low. Bird attacks increased toward the later seasons, with per-season values of 0.2% in spring, 0.7% in mid-summer, 1.8% in late summer, and 1.6% in autumn. Rodent attacks decreased toward the later seasons, with per-season values of 0.9% in spring, 1.0% in mid-summer, 0.6% in late summer, and 0.2% in autumn. Additionally, grasshopper attack rates per season were 2.0% in spring, 5.6% in mid-summer, 51.4% in late summer, and 17.6% in autumn.
The following are the results of models including neighborhood tree species richness and TLS-derived variables as fixed factors and using focal tree individuals as random factors (Table 1) for each predator group (see Section 3.5 for comparison to alternative analyses).
Table 1 Summary of generalized linear mixed effects model results for arthropod predator, bird, and rodent attacks, using neighborhood tree species richness and TLS-derived structural variables (for the model without TLS variables, see Supplementary Table S3); MeanFrac and ENL are used separately in the models instead of SSCI (for the model including SSCI, see Supplementary Table S4).
Neighborhood tree species richness did not show a direct significant relationship with any of the predator groups (after including TLS-derived variables), but arthropod predator attacks increased in larger plots with high tree species richness (Figure 1A; Table 1). In contrast, arthropod predator attacks were promoted at low vertical stratification (ENL) (Figure 1B) in 1 mu plots, and a similar, although nonsignificant (0.1 < p > 0.05) relationship was observed with vegetation density (MeanFrac) (Figure 1C). Larger plot sizes had a negative effect on rodent attacks, and attack probability was higher in smaller plots of low vegetation density (Figure 1D).
Figure 1 Attack probability as a function of plot size (1 vs. 4 mu) and (A) neighborhood tree species richness, (B) ENL, or (C, D) MeanFrac. In (D), 7 data points between y-axis values 0.175 and 0.33 were removed for visual purposes from the point column with the highest values. Points show fitted values, shaded areas the 95% confidence intervals, and histograms the amount of observed data points per x-axis levels.
Neighborhood tree species richness showed a positive effect on arthropod predator attacks in more densely branched neighborhoods (voxels per layer) (Figure 2A; Table 1). In addition, more densely branched neighborhoods also had a positive effect on arthropod predator attacks at low plot-level vegetation density and a negative effect at high vegetation density (Figure 2B). For bird attacks, no significant direct influence was observed with plot vegetation density and open area in the neighborhood, but the attacks were promoted when there was more open area in the high vegetation density neighborhoods (Figure 2C). Arthropod predator attacks were more common on larger trees (focal tree BA), and additionally, attacks were more likely when there were no branches close to the caterpillars (placement on trunk instead of branch) and the surrounding neighborhood trees were smaller, but less likely when the neighborhood trees were large (Figure 2D). Higher placement heights had a positive effect on arthropod predator attacks, whereas rodent attacks were more frequent with lower placement heights. A canopy with fewer vertical layers promoted bird attacks at the plot level. The more frequent arthropod predator attacks when leaves were present in the immediate surroundings were also further promoted in less vertically stratified plots (Figure 2E). Additionally, a more densely packed canopy at the neighborhood level (vertical Gini) had a positive effect on arthropod predator attacks.
Figure 2 Attack probability as a function of (A) voxels per layer and neighborhood tree species; (B) voxels per layer and MeanFrac; (C) MeanFrac and open area in the neighborhood; (D) placement on trunk or branch and neighborhood BA; and the presence of leaves in close proximity of caterpillars and (E) ENL or (F) season. In (A–C), colors represent the estimated change in attack probability and colors represent the estimated change in attack probability and points the observed values. In plots (D, F), points show fitted values and shaded areas the 95% confidence intervals. Histograms show the amount of observed data points per axis level.
Arthropod predator and rodent attacks were highest in spring, whereas bird attacks increased toward autumn. Arthropod predator attacks were promoted in spring when leaves were present in the immediate surroundings of the caterpillars (Figure 2). Higher canopy vertical stratification had a positive effect on arthropod predator attacks in spring, but the relationship turned negative in autumn (Figure 3A). Bird attacks were instead highest at less vertically stratified plots except in autumn, when they were systematically high across the ENL value range (Figure 3B). A lower amount of branches in the local neighborhood had a negative effect on arthropod predator attacks toward autumn (Figure 3C). Rodent attacks were promoted in denser vegetation plots in spring (Figure 3D), and a similar but nonsignificant (0.1 < p > 0.05) positive trend with vegetation density was observed with arthropod predators (Figure 2F).
Figure 3 Attack probability as a function of season and (A, B) ENL, (C) voxels per layer, or (D) MeanFrac. Colors represent the estimated change in attack probability, points the observed values, and histograms the amount of observed data points per axis level.
Plots with lower solar irradiance had a higher attack probability with all predator groups in the dry season toward autumn (Figures 4A–C), but high solar irradiance had an equally high effect on rodent attacks, with a positive trend also for arthropod predator attacks in spring. Similarly, plots at low elevation promoted arthropod predator attacks in the dry season, with a weaker positive trend in high elevation plots observed in spring (Figure 4D). In addition, lower slope angles promoted bird attacks in autumn (Figure 4E) and higher slope angles promoted rodent attacks in spring (Figure 4F).
Figure 4 Attack probability as a function of season and (A–C) solar irradiance, (D) elevation, or (E, F) slope angle. Colors represent the estimated change in attack probability, points the observed values, and histograms the amount of observed data points per axis level.
Attacks were less frequent with all predator groups in the second checking round, but this effect varied seasonally for arthropod predators, with a positive effect of the later checking round in spring turning negative toward autumn (Supplementary Figure S9A). The delays caused by rainy days led to a significant increase in attacks by arthropod predators but not by birds and rodents. However, this increase was strong only during the dry season (Supplementary Figure S9B).
Comparison of models with tree species richness at the neighborhood level instead of the plot level revealed that neighborhood tree species richness led to clearly improved models for arthropod predators and birds (ΔAIC > 2), but for rodents the improvement was small (ΔAIC = 1.1). Models without terrestrial laser scanning (TLS)-derived structural variables (Table 1) showed higher estimates of neighborhood tree species richness and neighborhood BA (Table 1; Supplementary Table S3), whereas in the models with TLS-derived variables, the ENL showed similar relationships with stronger estimates (see Supplementary Figure S6 for seasonal neighborhood tree species richness relationship for arthropod predators). Removing tree species richness from the arthropod predator model using TLS-derived structural variables showed a sign of missing covariates, with the interaction of plot size with ENL becoming weak (results not shown). Thus, because of the moderate collinearity of ENL with neighborhood tree species richness (Spearman r = 0.45; Supplementary Figure S5), which varied in strength between tree species (Supplementary Figure S7) and neighborhood BA (Spearman r = 0.54), the results of these variables need to be interpreted in comparison to each other. Instead, removing MeanFrac from the arthropod predator TLS model had fairly small effects on the estimates of voxels per layer despite their moderately high collinearity (Spearman r = 0.60), with the direct effect of voxels per layer becoming significantly positive and the interaction of voxels per layer with season becoming weaker (results not shown), due to the lack of the wider-scale vegetation density covariate with an opposite seasonal trend. The inclusion of TLS variables affected also estimates of slope angle due to slope angle and ENL demonstrating different aspects of canopy vertical structure, with only low collinearity between them (Spearman r = 0.17). Using the focal tree as a random factor instead of tree species pair identity also improved the arthropod predator and bird models (ΔAIC > 2; results not shown), but in the case of rodents, the improvement was fairly small (ΔAIC = 1).
Simplifying the plot-level forest structure to SSCI (Supplementary Table S4) instead of using MeanFrac and ENL (Table 1) separately led to reduced information value (ΔAIC > 2; see Table 1 and Supplementary Table S4 for the exact AIC scores) of arthropod predator and bird models, as estimates of SSCI and its interactions were weaker than with MeanFrac and ENL. However, in the case of rodents, where otherwise only MeanFrac from the structural measurements showed a strong relationship with the likelihood of a caterpillar being attacked, the change in AIC was marginally in favor of SSCI (ΔAIC = 0.3).
Simplifying tree species richness and forest structure to PC scores offered a robust outlook on the effects of forest overall complexity on predation, with none of the PCs explaining predation directly for arthropod predators, but PC1 having a negative effect on predation by birds and rodents, and PC3 having a nonsignificant (0.1 < p > 0.05) negative trend on birds (Supplementary Table S5). The PC1, whose loadings were affected by multiple variables but most strongly by neighborhood BA and ENL, and focal BA-driven PC3 interacted with the season in the case of arthropod predators and birds, and vegetation density measure-driven PC2 in the case of rodents. The PC1 and PC2 also had significant interaction in the case of arthropod predators. The PC-based analysis offered clearly poorer information value compared to using structural measures separately for arthropod predators and rodents (ΔAIC > 2; see Table 1 and Supplementary Table S5 for the exact AIC scores), whereas the difference was marginal for birds (ΔAIC = 0.1). Notably, interactions with plot size and very fine-scale variables of attraction and visibility (presence of leaves and branches) in PC-based models were weak and not retained in the reduced models, in contrast to models using structural measures separately.
Our results demonstrate that predation by arthropods and vertebrates is influenced interactively by tree species richness and various forest structural features at different spatial scales, from the plot level to the immediate vicinity of the artificial caterpillars. In addition, forest structure and topography affected the predation pressure seasonally. Furthermore, using separate measures of forest structure instead of overall measures of complexity provided higher information value in the GLME models. Particularly, the specific spatial-scale relatedness of variables became obscured with complexity measures. Our results, thus, emphasize that predation pressure is not a simple one-factorial function of tree species richness or any specific forest structural metric and that understanding the regulation of top-down control in forests requires closer attention to scale dependency and seasonal dynamics.
Predation by arthropods was higher than by vertebrates, as often shown in studies with the same methods (e.g., Sam et al., 2015; Leles et al., 2017; Roslin et al., 2017; but see Poch and Simonetti, 2013; Yang et al., 2018). Yet, we restrict the comparisons here to only among predator groups, as the likelihood of attacking artificial caterpillars might vary between predator taxa (Zvereva and Kozlov, 2023). Birds are well known to be important predators of caterpillars, and rodents have been reported as an important predator group of arthropods in agroecosystems (Tschumi et al., 2018) and have also been observed to attack artificial caterpillars placed on trees (e.g., Posa et al., 2007). However, it needs to be noted that the estimated attack probabilities of rodents and birds were low in our study due to overall infrequent attacks, and therefore, interpretations should only be made with caution. As we lack predator abundance or behavior-related data from the field site during the time of the experiment, we discuss here the potential mechanisms explaining the relationships between tree species richness and forest structure with predation pressure in light of other studies focusing on these relationships. The main focus is on the effects through expected changes in predator abundance, movement between patches, and close-scale visibility and attraction determinants.
Our results provided partial support for the expectation of increased predation at higher tree species richness, based on the expected increase in predator abundances as posited by the “Enemies hypothesis” (Root, 1973), but this support was dependent on predator group, spatial scale, and season. The relationship was generally positive and similar to earlier studies about arthropod predator abundances (Andow, 1991; Haddad et al., 2009), predation rates (Leles et al., 2017), and attacks by birds (Muiruri et al., 2016). However, after the inclusion of forest structural metrics, the positive effect of tree species richness was replaced altogether by structural effects for birds and remained only in larger plots and in more densely branched neighborhoods for arthropods, showing that the tree species richness effect is partially driven by accompanying changes in forest structure. The effect of higher tree species richness on predator population sizes is likely stronger in larger areas due to species–area relationships. However, different tree species can host differing predator fauna compositions (as shown for spiders, Zhang et al., 2018), and, for example, differences in leaf structure can influence predation rates (Carter et al., 1984; Grevstad and Klepetka, 1992; Coll and Ridgway, 1995; Clark and Messina, 1998). In turn, higher branch/foliage density has been shown to increase prey and arthropod predator (spider) abundances (Halaj et al., 1998; Halaj et al., 2000). Thus, denser mixtures of foliage from different neighboring tree species may locally increase predation pressure through predator richness and abundance, especially for the tree species where the efficiency of predators is otherwise lowest. The direct effect of plant species richness may, thus, be more evident in agroecosystems and grasslands than in forests (Russell, 1989; Wan et al., 2020) due to plants of different species being systematically in closer contact with each other when they are smaller.
Besides tree species richness, forest structure is an important component affecting predator–prey interactions as it shapes the physical environment that animals encounter. Our results showed that predation pressure responded to vegetation structure between spatial scales, as demonstrated in previous studies of predation by arthropods (Schwab et al., 2021). The positive effects of a more vertically stratified canopy (ENL) and vegetation density (MeanFrac) on predation by arthropods were restricted to smaller plots. Even if the higher canopy vertical stratification and vegetation density would promote arthropod predation, the higher tree species and structural variability in the plot surroundings may have overruled this effect. More variable plot neighborhoods also increased rodent attacks, but in contrast to arthropods, rodent attacks were promoted in smaller low vegetation density plots. The different responses to plot size–forest structural relationships likely demonstrate habitat size restrictions between the predator groups, as rodent abundances have been shown to respond to habitat characteristics at various spatial scales, but in areas mostly much larger compared to our study plots (Bowman et al., 2000; Silva et al., 2005).
In addition to the plot size-related effects, the response of predators to small-scale vegetation structure was shaped by the larger-scale neighborhood structure within the plots, as the intensity of arthropod predator attacks varied in relation to neighborhood branch density and plot-level vegetation density. The encounter and/or acceptance likelihood of less-appealing artificial prey might increase if natural prey is scarce, as search time can be expected to be inversely related to prey density (Andow and Prokrym, 1990). For example, higher predation rates on artificial caterpillars were observed in forest sites that actually had lower real lepidopteran density compared to those with higher density, a result that was attributed to the increased pressure on the available prey (Koh and Menge, 2006). It has also been demonstrated that seemingly prey-deficient sink habitats can encounter steady predation pressure from neighboring source populations, upholding larger predator abundances (Holt, 1985; Pulliam, 1988; Koricheva et al., 2000; Bommarco and Banks, 2003; Harmon et al., 2003). As arthropod attacks increased in the lower neighborhood branch density patches only in overall high vegetation density plots, there is a likely source effect from the surrounding vegetation making the more visible caterpillars subject to more frequent predator encounters in these simple structured component communities. In turn, higher predation pressure in densely branched neighborhoods when the plot-level vegetation is less dense might indicate aggregation of predators, either by higher prey density attracting or retaining predators in these patches (Hassell and May, 1974; Kareiva and Odell, 1987) or possibly by the dense patch itself appearing as a cue of prey presence within a surrounding area of lower quality.
Arthropod predation varied also in relation to the presence of leaves in the immediate surroundings of the caterpillars and canopy vertical layering at the plot level. The direct positive effect of a vertically more densely packed canopy was stronger for predation by arthropods in the local neighborhood (vertical Gini), but the positive effect of leaves in the immediate surroundings of caterpillars was promoted when the canopy was less vertically stratified at plot level. Predators can use cues of prey availability from herbivore-damaged leaves (Heinrich and Collins, 1983; Mäntylä et al., 2008; Sam et al., 2015), which naturally require leaves to be present, but the leaves themselves might work as an indicator of prey in stands with less foliage. This was further indicated by the increased effect of the presence of leaves when they were still scarce during budburst and by increased arthropod attacks at higher placement heights where the caterpillars were closer to the canopy. Potential prey outside the foliage zone might receive less attention from the predators, and conversely, when the canopy is more vertically stratified, the presence of leaves as a fine-scale cue may have weaker effects.
Potential visibility effects were observed with predation by birds, as attacks were more common in the plots with the smallest trees and the least stratified canopy. Birds are expected to prefer shade habitats (Perfecto et al., 1996), but they are highly mobile predators, and caterpillars in more visible environments may attract more attacks. In addition, larger open areas in the local neighborhood increased attacks on more densely vegetated plots. Denser vegetation in the canopy layer as well as in the understory is known to promote bird species richness, abundance, and the predation pressure they exert (MacArthur and MacArthur, 1961; Khanaposhtani et al., 2012; Mag and Ódor, 2015; Filloy et al., 2023). Instead, results have been more equivocal on the role of reduced habitat complexity and increased openness in increasing predation by birds due to easier finding of prey, ranging from positive (Šipoš and Kindlmann, 2013; Yang et al., 2018) to negative (Koh and Menge, 2006). It is thus likely, as suggested in earlier studies (Muiruri et al., 2016), that visibility is important for birds mainly in small spatial scales, with habitat selection being of higher importance.
Whereas vegetation biomass has been shown to increase predation rates in grasslands (Haddad et al., 2009; Ebeling et al., 2014), we did not observe positive effects of tree sizes besides the focal tree BA. Instead, lower neighborhood BA demonstrated possibly visibility-driven effects in the absence of branches on the focal trees. The effect of vegetation biomass on predation pressure in forests might, thus, be more variable due to the higher size and structural variation within the vegetation than what occurs in grasslands. However, some effects observed in this study may be additionally influenced by canopy structure-driven differences in understory vegetation, which varied to a large extent in the study plots and was clearly higher when the trees were small or had large open areas around them. For example, open areas have been observed to attract predators, presumably through higher herb/sapling and resulting higher herbivore densities (Richards and Windsor, 2007). Rodent attacks were also more common at lower placement heights, and rodent abundances are known to be increased by denser understory vegetation (Silva and Prince, 2008; Fischer and Schröder, 2014). The effects of understory were, however, not possible to quantify in our study.
Our results showed that predation pressure is not static in relation to forest structure and topography but has a seasonal dynamic across predator groups. New foliage can increase predation through the promotion of herbivore prey abundance (Richards and Coley, 2007), and the positive effect of canopy vertical stratification on predation by arthropods and more densely vegetated plots on rodents in spring is likely due to high foliage resource abundance for herbivores. However, besides the effects of foliage, our results suggest a shift in predation pressure based on abiotic effects between rainy and dry seasons. Plots with less sunny conditions (solar irradiation) had higher predation pressure in the dry season, and an additional similar shift was seen with arthropod predators along the elevational gradient toward the more humid lowland plots. Predation has been shown to decrease with increasing elevation, attributed to lower temperatures (Preszler and Boecklen, 1996; Roslin et al., 2017), but as the differences in elevation within the BEF-China sites were only small, the effects of higher elevation are more likely to indicate seasonal differences in exposure to wind and sun. Increased checking intervals due to rain also had hardly any effect on arthropod predation during the rainy season but instead promoted arthropod attacks during the dry season.
Arthropod predators and rodents additionally appeared to favor more sun-exposed plots in spring, likely due to earlier leaf production and increased herbivore prey abundance but also possibly due to benefitting from higher temperatures themselves during the cooler weather period. Topography affected predation only a little during the mid-summer rainy period, possibly due to lower difference in foliage abundance and altogether higher temperatures with presumably lower variation in humidity than during the dry season. In addition, altogether higher prey and predator densities may also reduce the effect between habitats of varying quality (McCoy and Bell, 1991), and caterpillar abundances were observed to be highest in the mid-summer rainy season in the BEF-China field site in the study year (Anttonen et al., 2022), when the arthropod predator attack intensity was also highest. Predation pressure following prey abundance also likely explains the observed higher arthropod predator attacks in the second checking round in spring and the first round in autumn. Arthropod predation pressure further increased in autumn in more densely branched neighborhoods, likely due to more shadowy microhabitats and/or aggregation on the most promising patches of prey in the season when prey abundance is lower. Bird attacks also increased toward late summer, when the predation pressure by naïve fledglings is highest (Remmel et al., 2009; Zvereva and Kozlov, 2023), but the attacks were nearly equally high even after the fledgling season in autumn, likely because of increased abundance and species richness caused by migratory birds (Van Bael et al., 2008). During this period, the preference for the plots with the smallest trees and canopy with the least vertical layers became absent, potentially due to the combined effect of reduced prey but increased bird abundance, forcing birds to search for prey across the available habitats.
The seasonal effect of canopy vertical stratification on predators may have also depended on canopy-driven microclimatic effects, besides differences in foliage abundance. ENL values decrease when the plot has a vertically more uniform canopy cover, which can drastically decrease light availability and temperature (Breshears, 2006), leading to more humid conditions. In addition to the positive effect that higher canopy vertical stratification had in spring, arthropod predator attacks also increased during the dry season in less- stratified canopy plots. Instead, no seasonal effect of BA was seen with arthropods even in the non-TLS model, demonstrating that the observed seasonal ENL–predation relationship is not driven simply by tree size differences. In addition, predation pressure by birds and rodents responded seasonally to canopy vertical structure through slope angle in a similar manner as arthropods to ENL. Altogether, the observed seasonal effects indicate that besides predation pressure following seasonal shifts in prey abundance, the predator’s avoidance of more sun-exposed areas during the dry season and a combination of young leaves (Richards and Coley, 2007), foliage abundance, and microclimatic effects in spring are important determinants shaping the predator’s response to its environment. However, specific studies on how tree phenology and seasonal fluctuations in abiotic conditions affect predation pressure would be needed, as studies on the topic are scarce (but see Richards and Coley, 2007; Molleman et al., 2016). This is especially the case for temperate, but seasonally rainy, sub-tropical forests.
Our study showed that forest structure captures a considerable proportion of the variation in predation pressure, which otherwise could be partly explained by tree species richness. Effects of forest structure have been addressed in studies of bird and arthropod predators using a variety of measures (e.g., MacArthur and MacArthur, 1961; Halaj et al., 1998; Khanaposhtani et al., 2012; Šipoš and Kindlmann, 2013; Poch and Simonetti, 2013), often related to vegetation “complexity”. The strong inconsistency between studies in terms of the value measured and the terminology used (McCoy and Bell, 1991; Tews et al., 2004; Loke and Chisholm, 2022) makes comparisons between them difficult, as there are several indices for measuring forest structure, including with TLS alone (McElhinny et al., 2005; Reich et al., 2021; Loke and Chisholm, 2022). Our results demonstrated that when using measures of overall forest complexity with principal components instead of different spatial scale-specific measures or one measure of plot-level complexity (SSCI) instead of vertical structure (ENL) or vegetation density (MeanFrac) separately, not only is the overall information value of the GLME models reduced, but also the interaction of different structural elements between spatial scales becomes challenging to define. TLS is a powerful method for measuring forest structure, making possible the estimation of multiple structural features (Calders et al., 2020) that can be further combined with other specific measures of small- and large-scale environments. However, further work is needed to clarify how the different structural variables relate to predation and animal behavior in general in different forest ecosystems.
Some specific notions of factors having an effect on the overall attack rate should be taken into consideration for future studies. The fairly long checking period in our study likely dampens the differences in predation between habitats to some extent, but this also demonstrates robustness in the results and buffers against sporadic effects caused by weather conditions. In addition, predators may learn the positions of the nonpalatable artificial caterpillars (Mäntylä et al., 2008), including eusocial insects, which likely explains the overall lower attack frequency during the second checking round of each season. Thus, longer-term experiments with artificial caterpillars would benefit from regular changes of the caterpillar’s positions. Grasshoppers have been observed to attack artificial baits in other ecosystems (Gordon and Kerr, 2022), and the sudden decline in predation by arthropods in late summer may be due in part to the masking effects of high grasshopper-induced damages. Thus, regular observations of the fauna in contact with the artificial caterpillars are advisable. Very fine-scale determinants of visibility and potential attraction to predators are important to take into account in studies of the wider forest’s structural effects, or vice versa, as their effects are not stable but interactive with each other. The effect of structural variables may also change seasonally, requiring close consideration of their role in study designs.
Our study highlights that not just tree species richness or structural complexity per se determines predation pressure in forests. Instead, scale dependence, the interplay of tree species richness and structural variables, and seasonal fluctuations in abiotic conditions and tree phenology all play a role in shaping the predation pressure on herbivores. These relationships, rarely addressed in previous studies, might explain some of the inconsistencies found in preceding analyses of tree diversity–predator relationships (Staab and Schuldt, 2020; Stemmelen et al., 2022). However, what effects predator and prey abundances, movement between habitats, and fine-scale predator efficiency determinants have on predation pressure, and how these relate to predators of varying mobility and habitat range, is still a question requiring more attention. Addressing these topics in forest ecosystems and BEF-research will aid in predicting how biodiversity loss will shape the top‐down control of herbivores, with important consequences for ecosystem functioning. Overall, our results highlight the need to build a more dynamic framework for assessing predation pressure in forests.
The raw data supporting the conclusions of this article will be made available by the authors, without undue reservation.
Conceptualization: PA, AS, and C-DZ. Methodology: PA, AS, MP-G, MK, GvO, C-DZ, HB, and K-PM. Validation: PA. Formal analysis: PA and AS. Investigation: PA, MP-G, and YL. Writing – original draft preparation: PA. Writing – review and editing: PA, AS, MP-G, MK, GvO, HB, YL, C-DZ, and K-PM. Visualization: PA. Supervision: AS and C-DZ. Project administration: AS, C-DZ, HB, and K-PM. Funding acquisition: HB, K-PM, AS, and C-DZ. All authors contributed to the article and approved the submitted version.
We gratefully acknowledge support by the International Research Training Group TreeDì jointly funded by the Deutsche Forschungsgemeinschaft (DFG, German Research Foundation) – 319936945/GRK2324, and the University of the Chinese Academy Sciences (UCAS). Further support came from the German Centre for Integrative Biodiversity Research (iDiv) Halle-Jena-Leipzig (DFG – FZT 118, 202548816). We acknowledge the financial support from the DFG under the program Open Access Publishing for the publication of this work.
We would like to thank for field assistance the student helpers Annika Müller, Clara Scholz, Felix Bach, Georg Hähn, Henriette Christel, Isabel Düring, Max Marczak, Paula Bräuer, and Tobias Wolff; and Li Fu Qi, Xiang-Yuan Wang, and Yin Guan Qi for indispensable aid in the field work, as well as Chun-King Jiang and Zhang-Rong Cheng for preparing the artificial caterpillars. We are grateful to Martin Volf for assisting in attack mark identification and to Michael Orr and Pengfei Guo for their support and assistance during field work.
The authors declare that the research was conducted in the absence of any commercial or financial relationships that could be construed as a potential conflict of interest.
All claims expressed in this article are solely those of the authors and do not necessarily represent those of their affiliated organizations, or those of the publisher, the editors and the reviewers. Any product that may be evaluated in this article, or claim that may be made by its manufacturer, is not guaranteed or endorsed by the publisher.
The Supplementary Material for this article can be found online at: https://www.frontiersin.org/articles/10.3389/fevo.2023.1199670/full#supplementary-material
Ali A. (2019). Forest stand structure and functioning: current knowledge and future challenges. Ecol. Indic. 98, 665–677. doi: 10.1016/j.ecolind.2018.11.017
Andow D. A. (1991). Vegetational diversity and arthropod population response. Annu. Rev. Entomol. 36, 561–586. doi: 10.1146/annurev.en.36.010191.003021
Andow D. A., Prokrym D. R. (1990). Plant structural complexity and host-finding by a parasitoid. Oecologia 82, 162–165. doi: 10.1007/BF00323530
Anttonen P., Li Y., Chesters D., Davrinche A., Haider S., Bruelheide H., et al. (2022). Leaf nutritional content, tree richness, and season shape the caterpillar functional trait composition hosted by trees. Insects 13, 1100. doi: 10.3390/insects13121100
Barnes A. D., Scherber C., Brose U., Borer E. T., Ebeling A., Gauzens B., et al. (2020). Biodiversity enhances the multitrophic control of arthropod herbivory. Sci. Adv. 6, 1–9. doi: 10.1126/sciadv.abb6603
Bianchi F. J. J. A., Schellhorn N. A., Buckley Y. M., Possingham H. P. (2010). Spatial variability in ecosystem services: simple rules for predator-mediated pest suppression. Ecol. Appl. 20, 2322–2333. doi: 10.1890/09-1278.1
Bommarco R., Banks J. (2003). Scale as modifier in vegetation diversity experiments: effects on herbivores and predators. Oikos 102, 440–448. doi: 10.1034/j.1600-0579.2003.12578.x
Bowman J., Forbes G., Dilworth T. (2000). The spatial scale of variability in small-mammal populations. Ecography (Cop.). 23, 328–334. doi: 10.1111/j.1600-0587.2000.tb00288.x
Breshears D. D. (2006). The grassland-forest continuum: trends in ecosystem properties for woody plant mosaics? Front. Ecol. Environ. 4, 96–104. doi: 10.1890/1540-9295(2006)004[0096:TGCTIE]2.0.CO;2
Brooks M. E., Kristensen K., Van Benthem K. J., Magnusson A., Berg C. W., Nielsen A., et al. (2017). glmmTMB balances speed and flexibility among packages for zero-inflated generalized linear mixed modeling. R J. 9, 378. doi: 10.32614/RJ-2017-066
Bruelheide H., Nadrowski K., Assmann T., Bauhus J., Both S., Buscot F., et al. (2014). Designing forest biodiversity experiments: general considerations illustrated by a new large experiment in subtropical C hina. Methods Ecol. Evol. 5, 74–89. doi: 10.1111/2041-210X.12126
Bruelheide H., Schmidt K., Seidler G., Nadrowski K. (2013) Site A plots: diversity treatments, coordinates, topography. Available at: http://china.befdata.biow.uni-leipzig.de/datasets/82.
Calders K., Adams J., Armston J., Bartholomeus H., Bauwens S., Bentley L. P., et al. (2020). Terrestrial laser scanning in forest ecology: expanding the horizon. Remote Sens. Environ. 251, 112102. doi: 10.1016/j.rse.2020.112102
Carter M. C., Sutherland D., Dixon A. F. G. (1984). Plant structure and the searching efficiency of coccinellid larvae. Oecologia 63, 394–397. doi: 10.1007/BF00390671
Clark T. L., Messina F. J. (1998). Plant architecture and the foraging success of ladybird beetles attacking the Russian wheat aphid. Entomol. Exp. Appl. 86, 153–161. doi: 10.1046/j.1570-7458.1998.00276.x
Coll M., Ridgway R. L. (1995). Functional and numerical responses of Orius insidiosus (Heteroptera: Anthocoridae) to its prey in different vegetable crops. Ann. Entomol. Soc Am. 88, 732–738. doi: 10.1093/aesa/88.6.732
Connor E. F., McCoy E. D. (2001). “Species–area relationships,” in Encyclopedia of Biodiversity (Academic Press, Amsterdam: Elsevier), 397–411. doi: 10.1016/B0-12-226865-2/00252-2
Ebeling A., Meyer S. T., Abbas M., Eisenhauer N., Hillebrand H., Lange M., et al. (2014). Plant diversity impacts decomposition and herbivory via changes in aboveground arthropods. PLoS One 9, e106529. doi: 10.1371/journal.pone.0106529
Ehbrecht M., Schall P., Ammer C., Seidel D. (2017). Quantifying stand structural complexity and its relationship with forest management, tree species diversity and microclimate. Agric. For. Meteorol. 242, 1–9. doi: 10.1016/j.agrformet.2017.04.012
Ehbrecht M., Schall P., Juchheim J., Ammer C., Seidel D. (2016). Effective number of layers: a new measure for quantifying three-dimensional stand structure based on sampling with terrestrial LiDAR. For. Ecol. Manage. 380, 212–223. doi: 10.1016/j.foreco.2016.09.003
Erwin T. L. (1983). Tropical forest canopies: the last biotic frontier. Bull. Entomol. Soc Am. 29, 14–20. doi: 10.1093/besa/29.1.14
Fichtner A., Härdtle W., Li Y., Bruelheide H., Kunz M., von Oheimb G. (2017). From competition to facilitation: how tree species respond to neighbourhood diversity. Ecol. Lett. 20, 892–900. doi: 10.1111/ele.12786
Filloy J., Oxbrough A., Oddi J. A., Ramos C. S., Ribero M. N., Santoandré S., et al. (2023). Understorey structural complexity mediated by plantation management as a driver of predation events on potential eucalypt pests. For. Ecol. Manage. 531, 1–9. doi: 10.1016/j.foreco.2023.120799
Finke D. L., Denno R. F. (2002). Intraguild predation diminished in complex-structured vegetation: implications for prey suppression. Ecology 83, 643–652. doi: 10.1890/0012-9658(2002)083[0643:IPDICS]2.0.CO;2
Fischer C., Schröder B. (2014). Predicting spatial and temporal habitat use of rodents in a highly intensive agricultural area. Agric. Ecosyst. Environ. 189, 145–153. doi: 10.1016/j.agee.2014.03.039
Flaherty D. L. (1969). Ecosystem trophic complexity and densities of the Willamette mite, Eotetranychus willamettei Ewing (Acarina: Tetranychidae). Ecology 50, 911–916. doi: 10.2307/1933710
Gordon S. C. C., Kerr J. T. (2022). Floral diversity increases butterfly diversity in a multitrophic metacommunity. Ecology 103, 1–14. doi: 10.1002/ecy.3735
Grevstad F. S., Klepetka B. W. (1992). The influence of plant architecture on the foraging efficiencies of a suite of ladybird beetles feeding on aphids. Oecologia 92, 399–404. doi: 10.1007/BF00317466
Haddad N. M., Crutsinger G. M., Gross K., Haarstad J., Knops J. M. H., Tilman D. (2009). Plant species loss decreases arthropod diversity and shifts trophic structure. Ecol. Lett. 12, 1029–1039. doi: 10.1111/j.1461-0248.2009.01356.x
Halaj J., Ross W. D., Moldenke R. A. (1998). Habitat structure and prey availability as predictors of the abundance and community organization of spiders in western Oregon forest canopies. J. Arachnol. 26, 203–220.
Halaj J., Ross D. W., Moldenke A. R. (2000). Importance of habitat structure to the arthropod food-web in Douglas-fir canopies. Oikos 90, 139–152. doi: 10.1034/j.1600-0706.2000.900114.x
Harmon J. P., Hladilek E. E., Hinton J. L., Stodola T. J., Andow D. A. (2003). Herbivore response to vegetational diversity: spatial interaction of resources and natural enemies. Popul. Ecol. 45, 75–81. doi: 10.1007/s10144-003-0146-8
Hartig F. (2022) DHARMa: Residual Diagnostics for Hierarchical (Multi-Level / Mixed) Regression Models. Available at: http://florianhartig.github.io/DHARMa/.
Hassell M. P., May R. M. (1974). Aggregation of predators and insect parasites and its effect on stability. J. Anim. Ecol. 43, 567. doi: 10.2307/3384
Hawkins B. A., Thomas M. B., Hochberg M. E. (1993). Refuge theory and biological control. Science 80, 1429–1432. doi: 10.1126/science.262.5138.1429
Heinrich B., Collins S. L. (1983). Caterpillar leaf damage, and the lame of hide-and-seek with birds. Ecology 64, 592–602. doi: 10.2307/1939978
Hildebrand M., Perles-Garcia M. D., Kunz M., Härdtle W., von Oheimb G., Fichtner A. (2021). Tree-tree interactions and crown complementarity: the role of functional diversity and branch traits for canopy packing. Basic Appl. Ecol. 50, 217–227. doi: 10.1016/j.baae.2020.12.003
Holt R. D. (1985). Population dynamics in two-patch environments: some anomalous consequences of an optimal habitat distribution. Theor. Popul. Biol. 28, 181–208. doi: 10.1016/0040-5809(85)90027-9
Hooper D. U., Adair E. C., Cardinale B. J., Byrnes J. E. K., Hungate B. A., Matulich K. L., et al. (2012). A global synthesis reveals biodiversity loss as a major driver of ecosystem change. Nature 486, 105–108. doi: 10.1038/nature11118
Howe A., Lövei G. L., Nachman G. (2009). Dummy caterpillars as a simple method to assess predation rates on invertebrates in a tropical agroecosystem. Entomol. Exp. Appl. 131, 325–329. doi: 10.1111/j.1570-7458.2009.00860.x
Huang Y., Chen Y., Castro-Izaguirre N., Baruffol M., Brezzi M., Lang A., et al. (2018). Impacts of species richness on productivity in a large-scale subtropical forest experiment. Science 80, 80–83. doi: 10.1126/science.aat6405
Janzen D. H. (1973). Sweep samples of tropical foliage insects: effects of seasons, vegetation types, elevation, time of day, and insularity. Ecology 54, 687–708. doi: 10.2307/1935359
Kareiva P., Odell G. (1987). Swarms of predators exhibit “preytaxis” if individual predators use area-restricted search. Am. Nat. 130, 233–270. doi: 10.1086/284707
Khanaposhtani M. G., Kaboli M., Karami M., Etemad V. (2012). Effect of habitat complexity on richness, abundance and distributional pattern of forest birds. Environ. Manage. 50, 296–303. doi: 10.1007/s00267-012-9877-7
Koh L. P., Menge D. N. L. (2006). Rapid assessment of lepidoptera predation rates in neotropical forest fragments. Biotropica 38, 132–134. doi: 10.1111/j.1744-7429.2006.00114.x
Koricheva J., Mulder C. P. H., Schmid B., Joshi J., Huss-Danell K. (2000). Numerical responses of different trophic groups of invertebrates to manipulations of plant diversity in grasslands. Oecologia 125, 271–282. doi: 10.1007/s004420000450
Kunz M., Fichtner A., Härdtle W., Raumonen P., Bruelheide H., Oheimb G. (2019). Neighbour species richness and local structural variability modulate aboveground allocation patterns and crown morphology of individual trees. Ecol. Lett. 22, 2130–2140. doi: 10.1111/ele.13400
Lang A. C., Härdtle W., Bruelheide H., Geißler C., Nadrowski K., Schuldt A., et al. (2010). Tree morphology responds to neighbourhood competition and slope in species-rich forests of subtropical China. For. Ecol. Manage. 260, 1708–1715. doi: 10.1016/j.foreco.2010.08.015
Langellotto G. A., Denno R. F. (2004). Responses of invertebrate natural enemies to complex-structured habitats: a meta-analytical synthesis. Oecologia 139, 1–10. doi: 10.1007/s00442-004-1497-3
Lawton J. H. (1983). Plant architecture and the diversity of phytophagous insects. Annu. Rev. Entomol. Vol. 28, 23–39. doi: 10.1146/annurev.en.28.010183.000323
Leles B., Xiao X., Pasion B. O., Nakamura A., Tomlinson K. W. (2017). Does plant diversity increase top-down control of herbivorous insects in tropical forest? Oikos 126, 1142–1149. doi: 10.1111/oik.03562
Loke L. H. L., Chisholm R. A. (2022). Measuring habitat complexity and spatial heterogeneity in ecology. Ecol. Lett. 25, 2269–2288. doi: 10.1111/ele.14084
Lövei G. L., Ferrante M. (2017). A review of the sentinel prey method as a way of quantifying invertebrate predation under field conditions. Insect Sci. 24, 528–542. doi: 10.1111/1744-7917.12405
Low P. A., Sam K., McArthur C., Posa M. R. C., Hochuli D. F. (2014). Determining predator identity from attack marks left in model caterpillars: guidelines for best practice. Entomol. Exp. Appl. 152, 120–126. doi: 10.1111/eea.12207
Lüdecke D., Ben-Shachar M., Patil I., Waggoner P., Makowski D. (2021). performance: an R package for assessment, comparison and testing of statistical models. J. Open Source Software 6, 3139. doi: 10.21105/joss.03139
MacArthur R. H., MacArthur J. W. (1961). On bird species diversity. Ecology 42, 594–598. doi: 10.2307/1932254
Mag Z., Ódor P. (2015). The effect of stand-level habitat characteristics on breeding bird assemblages in Hungarian temperate mixed forests. Community Ecol. 16, 156–166. doi: 10.1556/168.2015.16.2.3
Mäntylä E., Alessio G. A., Blande J. D., Heijari J., Holopainen J. K., Laaksonen T., et al. (2008). From plants to birds: higher avian predation rates in trees responding to insect herbivory. PLoS One 3, e2832. doi: 10.1371/journal.pone.0002832
McCoy E. D., Bell S. S. (1991). “Habitat structure: the evolution and diversification of a complex topic,” in Habitat Structure. Eds. Bell S. S., McCoy E. D., Mushinsky H. R. (Dordrecht: Springer Netherlands), 3–27. doi: 10.1007/978-94-011-3076-9_1
McElhinny C., Gibbons P., Brack C., Bauhus J. (2005). Forest and woodland stand structural complexity: Its definition and measurement. For. Ecol. Manage. 218, 1–24. doi: 10.1016/j.foreco.2005.08.034
Menge B. A., Sutherland J. P. (1987). Community regulation: variation in disturbance, competition, and predation in relation to environmental stress and recruitment. Am. Nat. 130, 730–757. doi: 10.1086/284741
Molleman F., Remmel T., Sam K. (2016). Phenology of Predation on Insects in a Tropical Forest: Temporal Variation in Attack Rate on Dummy Caterpillars. Biotropica 48, 229–236. doi: 10.1111/btp.12268
Muiruri E. W., Rainio K., Koricheva J. (2016). Do birds see the forest for the trees? Scale-dependent effects of tree diversity on avian predation of artificial larvae. Oecologia 180, 619–630. doi: 10.1007/s00442-015-3391-6
Nakamura A., Kitching R. L., Cao M., Creedy T. J., Fayle T. M., Freiberg M., et al. (2017). Forests and Their Canopies: Achievements and Horizons in Canopy Science. Trends Ecol. Evol. 32, 438–451. doi: 10.1016/j.tree.2017.02.020
Nell C. S., Abdala-Roberts L., Parra-Tabla V., Mooney K. A. (2018). Tropical tree diversity mediates foraging and predatory effects of insectivorous birds. Proc. R. Soc B Biol. Sci. 285, 20181842. doi: 10.1098/rspb.2018.1842
Oksanen J., Simpson G. L., Blanchet F. G., Kindt R., Legendre P., Minchin P. R., et al. (2022) vegan: Community Ecology Package. Available at: https://CRAN.R-project.org/package=vegan.
Perfecto I., Rice R. A., Greenberg R., van der Voort M. E. (1996). Shade Coffee: A Disappearing Refuge for Biodiversity. Bioscience 46, 598–608. doi: 10.2307/1312989
Perles-Garcia M. D., Kunz M., Fichtner A., Härdtle W., von Oheimb G. (2021). Tree species richness promotes an early increase of stand structural complexity in young subtropical plantations. J. Appl. Ecol. 58, 2305–2314. doi: 10.1111/1365-2664.13973
Poch T. J., Simonetti J. A. (2013). Insectivory in Pinus radiata plantations with different degree of structural complexity. For. Ecol. Manage. 304, 132–136. doi: 10.1016/j.foreco.2013.04.044
Polis G. A., Holt R. D., Menge B. A., Winemiller K. O. (1996). “Time, Space, and Life History: Influences on Food Webs,” in Food Webs (Boston, MA: Springer US), 435–460. doi: 10.1007/978-1-4615-7007-3_38
Posa M. R. C., Sodhi N. S., Koh L. P. (2007). Predation on artificial nests and caterpillar models across a disturbance gradient in Subic Bay, Philippines. J. Trop. Ecol. 23, 27–33. doi: 10.1017/S0266467406003671
Preszler R. W., Boecklen W. J. (1996). The influence of elevation on tri-trophic interactions: Opposing gradients of top-down and bottom-up effects on a leaf-mining moth. Ecoscience 3, 75–80. doi: 10.1080/11956860.1996.11682318
Pretzsch H. (2014). Canopy space filling and tree crown morphology in mixed-species stands compared with monocultures. For. Ecol. Manage. 327, 251–264. doi: 10.1016/j.foreco.2014.04.027
Pulliam H. R. (1988). Sources, Sinks, and Population Regulation. Am. Nat. 132, 652–661. doi: 10.1086/284880
R Core Team (2022) R: A Language and Environment for Statistical Computing. Available at: https://www.r-project.org/.
Reich K. F., Kunz M., von Oheimb G. (2021). A new index of forest structural heterogeneity using tree architectural attributes measured by terrestrial laser scanning. Ecol. Indic. 133, 108412. doi: 10.1016/j.ecolind.2021.108412
Remmel T., Tammaru T., Mägi M. (2009). Seasonal mortality trends in tree-feeding insects: a field experiment. Ecol. Entomol. 34, 98–106. doi: 10.1111/j.1365-2311.2008.01044.x
Richards L. A., Coley P. D. (2007). Seasonal and habitat differences affect the impact of food and predation on herbivores: A comparison between gaps and understory of a tropical forest. Oikos 116, 31–40. doi: 10.1111/j.2006.0030-1299.15043.x
Richards L. A., Windsor D. M. (2007). Seasonal variation of arthropod abundance in gaps and the understorey of a lowland moist forest in Panama. J. Trop. Ecol. 23, 169–176. doi: 10.1017/S0266467406003907
Riihimäki J., Vehviläinen H., Kaitaniemi P., Koricheva J. (2006). Host tree architecture mediates the effect of predators on herbivore survival. Ecol. Entomol. 31, 227–235. doi: 10.1111/j.1365-2311.2006.00784.x
Root R. B. (1973). Organization of a Plant-Arthropod Association in Simple and Diverse Habitats: The Fauna of Collards (Brassica Oleracea). Ecol. Monogr. 43, 95–124. doi: 10.2307/1942161
Roslin T., Hardwick B., Novotny V., Petry W. K., Andrew N. R., Asmus A., et al. (2017). ). Higher predation risk for insect prey at low latitudes and elevations. Science 80, 80–83. doi: 10.1126/science.aaj1631
Russell E. P. (1989). Enemies hypothesis: a review of the effect of vegetational diversity on predatory insects and parasitoids. Environ. Entomol. 18, 590–599. doi: 10.1093/ee/18.4.590
Sam K., Koane B., Novotny V. (2015). Herbivore damage increases avian and ant predation of caterpillars on trees along a complete elevational forest gradient in Papua New Guinea. Ecography (Cop.). 38, 293–300. doi: 10.1111/ecog.00979
Scheinin M., Scyphers S. B., Kauppi L., Heck K. L., Mattila J. (2012). The relationship between vegetation density and its protective value depends on the densities and traits of prey and predators. Oikos 121, 1093–1102. doi: 10.1111/j.1600-0706.2011.19941.x
Schnabel F., Liu X., Kunz M., Barry K. E., Bongers F. J., Bruelheide H., et al. (2021). Species richness stabilizes productivity via asynchrony and drought-tolerance diversity in a large-scale tree biodiversity experiment. Sci. Adv. 7, 11–13. doi: 10.1126/sciadv.abk1643
Schwab D., Wurz A., Grass I., Rakotomalala A. A. N. A., Osen K., Soazafy M. R., et al. (2021). Decreasing predation rates and shifting predator compositions along a land-use gradient in Madagascar’s vanilla landscapes. J. Appl. Ecol. 58, 360–371. doi: 10.1111/1365-2664.13766
Siemann E., Tilman D., Haarstad J., Ritchie M. (1998). ). Experimental Tests of the Dependence of Arthropod Diversity on Plant Diversity. Am. Nat. 152, 738–750. doi: 10.1086/286204
Silva M., Hartling L., Opps S. B. (2005). Small mammals in agricultural landscapes of Prince Edward Island (Canada): Effects of habitat characteristics at three different spatial scales. Biol. Conserv. 126, 556–568. doi: 10.1016/j.biocon.2005.07.007
Silva M., Prince M. E. (2008). The conservation value of hedgerows for small mammals in Prince Edward Island, Canada. Am. Midl. Nat. 159, 110–124. doi: 10.1674/0003-0031(2008)159[110:TCVOHF]2.0.CO;2
Šipoš J., Kindlmann P. (2013). Effect of the canopy complexity of trees on the rate of predation of insects. J. Appl. Entomol. 137, 445–451. doi: 10.1111/jen.12015
Staab M., Schuldt A. (2020). The influence of tree diversity on natural enemies—a review of the “enemies” hypothesis in forests. Curr. For. Rep. 6, 243–259. doi: 10.1007/s40725-020-00123-6
Stemmelen A., Jactel H., Brockerhoff E., Castagneyrol B. (2022). Meta-analysis of tree diversity effects on the abundance, diversity and activity of herbivores’ enemies. Basic Appl. Ecol. 58, 130–138. doi: 10.1016/j.baae.2021.12.003
Tews J., Brose U., Grimm V., Tielbörger K., Wichmann M. C., Schwager M., et al. (2004). Animal species diversity driven by habitat heterogeneity/diversity: the importance of keystone structures. J. Biogeogr. 31, 79–92. doi: 10.1046/j.0305-0270.2003.00994.x
Trogisch S., Liu X., Rutten G., Xue K., Bauhus J., Brose U., et al. (2021). The significance of tree-tree interactions for forest ecosystem functioning. Basic Appl. Ecol. 55, 33–52. doi: 10.1016/j.baae.2021.02.003
Tschanz B., Schmid E., Bacher S. (2005). Host plant exposure determines larval vulnerability – do prey females know? Funct. Ecol. 19, 391–395. doi: 10.1111/j.1365-2435.2005.00999.x
Tschumi M., Ekroos J., Hjort C., Smith H. G., Birkhofer K. (2018). Rodents, not birds, dominate predation-related ecosystem services and disservices in vertebrate communities of agricultural landscapes. Oecologia 188, 863–873. doi: 10.1007/s00442-018-4242-z
Turlings T. C. J., Erb M. (2018). Tritrophic interactions mediated by herbivore-induced plant volatiles: mechanisms, ecological relevance, and application potential. Annu. Rev. Entomol. 63, 433–452. doi: 10.1146/annurev-ento-020117-043507
Valtonen A., Molleman F., Chapman C. A., Carey J. R., Ayres M. P., Roininen H. (2013). Tropical phenology: bi-annual rhythms and interannual variation in an Afrotropical butterfly assemblage. Ecosphere 4, art36. doi: 10.1890/ES12-00338.1
Van Bael S. A., Philpott S. M., Greenberg R., Bichier P., Barber N. A., Mooney K. A., et al. (2008). Birds as predators in tropical agroforestry systems. Ecology 89, 928–934. doi: 10.1890/06-1976.1
Wan N., Zheng X., Fu L., Kiær L. P., Zhang Z., Chaplin-Kramer R., et al. (2020). Global synthesis of effects of plant species diversity on trophic groups and interactions. Nat. Plants 6, 503–510. doi: 10.1038/s41477-020-0654-y
Wardhaugh C. W., Stork N. E., Edwards W. (2014). Canopy invertebrate community composition on rainforest trees: Different microhabitats support very different invertebrate communities. Austral Ecol. 39, 367–377. doi: 10.1111/aec.12085
Williams L. J., Paquette A., Cavender-Bares J., Messier C., Reich P. B. (2017). Spatial complementarity in tree crowns explains overyielding in species mixtures. Nat. Ecol. Evol. 1, 63. doi: 10.1038/s41559-016-0063
Wolda H. (1978). Seasonal fluctuations in rainfall, food and abundance of tropical insects. J. Anim. Ecol. 47, 369. doi: 10.2307/3789
Yang X., Bauhus J., Both S., Fang T., Härdtle W., Kröber W., et al. (2013). Establishment success in a forest biodiversity and ecosystem functioning experiment in subtropical China (BEF-China). Eur. J. For. Res. 132, 593–606. doi: 10.1007/s10342-013-0696-z
Yang B., Li B., He Y., Zhang L., Bruelheide H., Schuldt A. (2018). Tree diversity has contrasting effects on predation rates by birds and arthropods on three broadleaved, subtropical tree species. Ecol. Res. 33, 205–212. doi: 10.1007/s11284-017-1531-7
Zemp D. C., Ehbrecht M., Seidel D., Ammer C., Craven D., Erkelenz J., et al. (2019). Mixed-species tree plantings enhance structural complexity in oil palm plantations. Agric. Ecosyst. Environ. 283, 106564. doi: 10.1016/j.agee.2019.06.003
Zhang Q., He D., Wu H., Shi W., Chen C. (2018). Local-scale determinants of arboreal spider beta diversity in a temperate forest: roles of tree architecture, spatial distance, and dispersal capacity. PeerJ 6, e5596. doi: 10.7717/peerj.5596
Keywords: artificial caterpillar, forest structure, scale-dependence, season, top-down control, topography, tree species richness, vegetation density
Citation: Anttonen P, Perles-Garcia M, Kunz M, von Oheimb G, Li Y, Bruelheide H, Ma K-P, Zhu C-D and Schuldt A (2023) Predation pressure by arthropods, birds, and rodents is interactively shaped by tree species richness, vegetation structure, and season. Front. Ecol. Evol. 11:1199670. doi: 10.3389/fevo.2023.1199670
Received: 03 April 2023; Accepted: 04 September 2023;
Published: 29 September 2023.
Edited by:
Bertrand Fournier, University of Potsdam, GermanyReviewed by:
Joan Casanelles Abella, Swiss Federal Institute for Forest, Snow and Landscape Research (WSL), SwitzerlandCopyright © 2023 Anttonen, Perles-Garcia, Kunz, von Oheimb, Li, Bruelheide, Ma, Zhu and Schuldt. This is an open-access article distributed under the terms of the Creative Commons Attribution License (CC BY). The use, distribution or reproduction in other forums is permitted, provided the original author(s) and the copyright owner(s) are credited and that the original publication in this journal is cited, in accordance with accepted academic practice. No use, distribution or reproduction is permitted which does not comply with these terms.
*Correspondence: Perttu Anttonen, cGVydHR1LnAuYW50dG9uZW5AZ21haWwuY29t
Disclaimer: All claims expressed in this article are solely those of the authors and do not necessarily represent those of their affiliated organizations, or those of the publisher, the editors and the reviewers. Any product that may be evaluated in this article or claim that may be made by its manufacturer is not guaranteed or endorsed by the publisher.
Research integrity at Frontiers
Learn more about the work of our research integrity team to safeguard the quality of each article we publish.