- College of Forestry, Hainan University, Haikou, China
Land-use changes have received increasing attention since the 1990s. Cultivated land is a vital land resource with both natural and economic features. Socioeconomic development profoundly affects the spatiotemporal evolution of cultivated land. In turn, the spatiotemporal change of cultivated land also reflects the socioeconomic development trend. This study took Haikou City, which has the highest degree of urbanization in Hainan Province, as the study area, analyzed the spatiotemporal evolution characteristics of its cultivated land resources during the past 20 years of rapid socioeconomic development, and predicted the future spatiotemporal evolution trends of its cultivated land resources on this basis, to provide scientific reference for the rational use and sustainable development of the cultivated land resources in Haikou. This study applied the single land-use dynamic degree (SLUDD) and land-use transfer matrix to explore the spatiotemporal evolution of cultivated land in Haikou from 2000 to 2020. The patch-generating land-use simulation (PLUS) model was introduced to predict the patterns of cultivated land under different development scenarios in 2030. The results showed that: (1) although rapid socioeconomic development has contributed to Haikou's urbanization rate over the past 20 years, the accompanying expansion of construction land has caused damage to cultivated land resources, especially in urban suburbs. Site conditions, differential land benefits, and urban economic radiation may be potential influencing factors leading to the loss of peri-urban cultivated land; (2) it was verified that the PLUS model applies to the land-use patterns prediction in Haikou; and (3) the simulation results of both natural and urban development scenarios not only predicted the continued loss of cultivated land in the original zone but also exposed new sites of cultivated land loss. Although construction land expansion is an inevitable trend, there is still hope that the expansion rate can be controlled, for example, under the cultivated land conservation scenario. The simulation results of the three scenarios have essential reference values for the future maintenance of cultivated land resource security in Haikou.
1. Introduction
From the United Nations Millennium Development Goals (UNMDGs) in 2000 to the United Nations Sustainable Development Goals (UNSDGs) in 2015, the “Zero Hunger” goal has always been among them and occupies an important position (McMichael and Schneider, 2011; Pérez-Escamilla, 2017). Among many international issues, food security is the only global issue for which the United Nations has set up three special agencies [Food and Agriculture Organization (FAO), International Fund for Agricultural Development, and World Food Programme] to solve, and this fully demonstrates the importance of food security in the global security system. Global trends such as climate change, population growth, accelerated urbanization, and the COVID-19 pandemic are increasing destabilizing factors; the global agricultural systems are facing unprecedented security challenges (Cole et al., 2018; Prosekov and Ivanova, 2018; Yadav et al., 2019; Vågsholm et al., 2020). The number of hungry people worldwide has soared since the outbreak of the COVID-19 pandemic, with approximately 130 million people expected to be affected by food security issues, according to FAO (UNICEF, 2020). Compared with COVID-19, the impact of urbanization on food security is long-term. This impact is mainly reflected in the encroachment of construction land on cultivated land at the macro level. Cultivated land is the primary bearer of food production and a vital land resource to ensure food security (Zhou et al., 2021). The expanding construction land is gradually pushing the change of cultivated land structure, which further affects the spatial pattern of food production and drives the shift of the center of gravity of food production. For example, due to urbanization, the center of gravity of food production in China has gradually shifted from south to northeast. In addition, the advance of urbanization also has an impact on agricultural producers: on the one hand, a large number of rural people are engaged in city construction, leading to a decrease in the number of agricultural producers; on the other hand, most of these rural people who migrate to the cities are young and middle-aged laborers, which causes a decline in the quality of the agricultural labor force. These factors have profoundly influenced the evolution of cultivated land resources over a long time. Since the reform and opening up in 1978, under the influence of urbanization, China's cultivated land has changed in many aspects, such as the main body of utilization and social function, and the cultivated land resources on the whole show a trend of continuous loss (Liu et al., 2014; Zhao et al., 2014; Lai et al., 2020; Wang, 2022). Moreover, this trend has attracted the attention of many scholars, some of whom have conducted extensive research on the characteristics and driving forces of the spatiotemporal evolution of cultivated land in different spatial scales. For example, Chen D. et al. (2018) analyzed the spatiotemporal evolution of cultivated land in 64 countries along the Belt and Road Initiative (a development strategy proposed by China in 2013) from 2000 to 2010. Lyu et al. (2021) took 30 provinces in China as examples to explore the spatiotemporal pattern evolution and driving factors of cultivated land system resilience (CLSR). Chen L. et al. (2018) studied the spatiotemporal distribution differences and changes in the early-warning status of cultivated land system security (CLSS) in 12 cities of Heilongjiang Province and analyzed relevant driving factors. Liu et al. (2022) studied the evolution of cultivated land fragmentation (CLF) in Jiangsu Province and its driving mechanism.
Thus far, most previous studies (Xu et al., 2019; Yang et al., 2021; Chen et al., 2022; Li D. et al., 2022) focused on the spatiotemporal evolution of cultivated land in the past period. These studies revealed the influence of some driving factors on cultivated land changes, which have some reference values for cultivated land conservation. However, since the impacts of some driving factors, such as policies and human activities, are long-term, it is necessary to predict and analyze the future spatiotemporal patterns of cultivated land under the continuous influences of these driving factors. Cellular automata (CA) models have been widely used to model spatiotemporal land-use dynamics under the effects of various driving factors (Liang et al., 2021). The future land-use simulation (FLUS) model (Liu et al., 2017), the CLUE-S model (Verburg et al., 2002), and the CA-Markov model (Subedi et al., 2013) are all CA models designed based on a pattern analysis strategy (PAS). The PAS-based CA model is superior to the transition analysis strategy (TAS) based CA model in some aspects, but it still fails to reveal how the driving factors cause land-use evolution (Gao et al., 2022). The transformation rule mining framework based on the land expansion analysis strategy (LEAS) contained in the patch-generating land-use simulation (PLUS) model proposed by Liang et al. not only solved the problems of TAS and PAS but also combined the advantages of the two; in addition, the CA model of the multitype random patch seeds (CARS) was added to improve the simulation ability of actual landscape patterns. Compared with other CA models, the PLUS model has higher simulation accuracy and is more similar to the real landscape pattern metrics (Liang et al., 2021). At present, the PLUS model has been preliminarily applied in the studies of ecosystem services (Li et al., 2021; Shi et al., 2021), urban land-use structure and dynamics (Zhai et al., 2021), risk prediction of potentially contaminated sites (PCS) (Jiang et al., 2022), and other fields.
Haikou is the capital city of Hainan Province, China's southernmost province. Under the background of accelerating the construction of the Free Trade Port and vigorously developing the tourism industry in Hainan, the urbanization rate of Haikou is much higher than that of other cities and counties in Hainan, which makes the land-use patterns drastically change all the time. In this case, cultivated land resources urgently need more attention. Up to now, there have been few studies on cultivated land in Haikou. The existing studies mainly focus on the spatiotemporal evolution of cultivated land, and the forecast and analysis of the future development trend of cultivated land resources are still very scarce. Thus, this study aims to: (1) explore the spatiotemporal evolution characteristics of cultivated land resources in Haikou during the rapid socioeconomic development in the past 20 years; and (2) introduce the PLUS model to explore the development of cultivated land resources in Haikou under different development trends in the future.
2. Materials and methods
2.1. Study area
Haikou City is located in the northern region of Hainan Province (N 19°31′32“~20°04′52”, E 110°07′22“~110°42′32”) (Figure 1). It has a tropical marine monsoon climate. The average annual precipitation is 1,696.6 mm, the annual sunshine duration is 2,000 h, and the annual temperature is 24.4 °C. Its coastline is 136.23 km and its land area is 2,296.83 km2. The land area is mainly plain and platform with an altitude of < 100 m. Its geomorphic types can be roughly divided into three parts as follows: the coastal plain, river terrace, and hill and lava platforms. The overall terrain tends to be gentle, and the low-lying northern coastal plain belt accounts for approximately 52% of the total area. The southeast and northwest are slightly higher, mainly hills and lava platforms, accounting for approximately 5% of the total area. The central Nandu River terrace belt accounts for 43% of the total area, with low levels along the coast. The city includes 8 soil types, 12 subtypes, 43 soil genera, and 110 soil species, such as paddy, lateritic, and vegetable. Haikou has jurisdiction over 21 street offices, such as Xiuying and Haixiu, and 22 towns, such as Changliu and Xixiu.
Haikou plays a significant role as the political, economic, cultural, and scientific center of Hainan Province. Compared with other places in the province, Haikou has been leading in the speed of socioeconomic development. According to Haikou City Statistical Yearbook, from 2005 to 2020, the gross regional product grew from 30.89 billion yuan to 179.16 billion yuan. The implementation of significant strategies such as Hainan International Tourism Island and Free Trade Port constructions after 2010 further promoted the socioeconomic development of Haikou. In this context, as an essential development resource involved in the market circulation, the evolution characteristics and future evolution trend of cultivated land in Haikou urgently need to be explored.
2.2. Data sources and processing
Remote sensing images (RSIs) are essential data resources for monitoring land-use changes. For example, Chen C. et al. (2022) and Chen et al. (2021) explored the changes in land-use patterns of Zhoushan Island based on RSIs. The qualities of RSIs are crucial to the accuracy of land-use change monitoring. Some scholars have explored RSIs in recent years (Lv et al., 2022a,b; Sun et al., 2022). This study adopted China's multi-period land-use cover remote sensing monitoring dataset (CNLUCC) (Xu et al., 2018) as basic data (Table 1). This dataset was jointly constructed by several Chinese Academy of Sciences research institutes, and it used the Landsat RSIs as the primary information source. It is a widely used dataset for monitoring land-use changes in China, and the spatial resolution is 30 m. This study reclassified the land-use data into six types as follows: cultivated land, forestland, grassland, water area, construction land (construction land in this study includes urban and rural construction lands), and unused land. Land-use changes are mainly driven by natural, social, and other factors. Therefore, the driving factor data are indispensable in the land-use patterns simulation. This study comprehensively considered the area's actual situation and the data availability. Based on relevant research results, we selected 12 driving factors from natural and socioeconomic factors (Table 1). Slope and aspect data were extracted from digital elevation model (DEM) data using ArcGIS software. The data of distance from water area, government station, railway, highway, and road were processed using the Euclidean Distance tool in ArcGIS software. To meet the operating conditions of the PLUS model, we used ArcGIS software to unify the projection coordinate system of all data as Albers-Conic-Equal-Area, and the spatial resolutions of all data were unified as 30 m by the Resample tool in the ArcGIS software.
2.3. Methods
2.3.1. Single land-use dynamic degree
Single land-use dynamic degree (SLUDD) represents the change speed and amplitude of a specific land-use type in a certain period in the study area, and it can reveal the change rate of a particular land-use type, such as cultivated land (Chen et al., 2019). The calculation formula is as follows:
where K refers to the dynamic degree of a certain land-use type in the study area, Uai and Ubi refer to the area of a certain land-use type i at the beginning and end of the research period, respectively, and T refers to the research period.
2.3.2. Land-use transfer matrix
Land-use transfer matrix is the application of the Markov model in land-use changes. It can visually show the conversion between different land-use types in a period and indirectly reveal the transfer rate between them. Therefore, it is often used to explore the transfer characteristics of land-use types in the research period (Yang et al., 2020). The calculation formula is as follows:
where, S refers to the land-use type area, n refers to the number of land-use types, i refers to the land-use type at the beginning of the research period, and j refers to the land-use type at the end of the research period. When i≠j, Sij refers to the area transferred from land-use type i to land-use type j. When i=j, Sij refers to the area of land-use type that remains unchanged.
2.3.3. The PLUS model
The simulation flow (Figure 2) of the patch-generating land-use simulation (PLUS) model is mainly based on two modules as follows: the land expansion analysis strategy (LEAS) and the CA model based on multi-type random patch seeds (CARS). Compared with other land-use simulation models, the PLUS model has higher accuracy in simulating the evolution of land-use patches under specific scenarios (Liang et al., 2021).
2.3.3.1. The LEAS module
The LEAS module sampled the expansion part of each land-use type in the two phases of land-use changes and then combined it with the driving factors. It used the random forest classification (RFC) algorithm to explore the relationship between multiple driving factors and land-use changes and then obtained the growth probabilities of land-use types. The calculation formula is as follows:
where refers to the probability of land-use type k appearing in cell i, the value of d is 1 or 0, x refers to the vector composed of several driving factors, function I refers to the indicator function of the decision tree set, hn(x) refers to the prediction type of the NTH decision tree of x, and M refers to the total number of decision trees.
2.3.3.2. The CARS module
The Markov chain can simulate the complex process of land-use changes (Liping et al., 2018). In the PLUS model, the Markov chain predicts the future quantity demand of each land-use type based on the transfer probability of each land-use type in a period. The CARS module allows new land-use patches to grow independently within the limits of their growth probabilities. Based on the growth probabilities of land-use types and the future land-use quantity demand, the transfer cost matrix, neighborhood factor, and other related parameters were set in the CARS module to simulate the future land-use patterns. The calculation formula of the transfer probability matrix in the Markov chain is as follows:
where n refers to the number of land-use types and Pij refers to the probability of conversion from land-use type i to land-use type j, where 0 ≤ Pij ≤ 1, and the sum of elements in each row is 1.
2.3.3.3. Multi-scenario settings
Based on the relevant studies and the actual situation of the study area, this study set up three scenarios as follows: natural development scenario, urban development scenario, and cultivated land conservation scenario. We took 2020 as the base period and set the transfer probability matrix, transfer cost matrix, and neighborhood factor of each scenario, respectively, to simulate the land-use patterns of Haikou in 2030 under these three scenarios.
2.3.3.3.1. Transfer probability matrix
The Markov chain is a mathematical, statistical model based on transfer probabilities, a common model for predicting the future based on current states and trends. This model has been integrated into the PLUS model. This study input two land-use data from 2010 to 2020 into the Markov chain to obtain the land-use quantity in 2030 and the land-use transfer probability matrix during 2010–2020 (Table 2). Based on this matrix, we adjusted the transfer probability matrix of each scenario correspondingly.
Natural development scenario: In this scenario, the land-use patterns of Haikou in 2030 will continue the current land-use changes trend, based on the transfer probability matrix of 2010–2020 (Table 2).
Urban development scenario: Haikou is at the forefront of constructing Hainan International Tourism Island and Free Trade Port. The accelerated development of the three major urban clusters (Central, Changliu, and Jiangdong) and the construction of the Jiangdong New Area will further promote the construction land expansion. Therefore, based on the natural development scenario, the transfer probabilities of cultivated land, forestland, grassland, and unused land to construction land were increased by 20%. In comparison, the transfer probabilities of construction land to other land-use types were reduced by 30% (Table 3).
Cultivated land conservation scenario: The gradual implementation of relevant policies, such as “Eight bans” on the construction of unappropriated cultivated land in rural areas, “Six bans” on cultivated land protection, the prevention of “non-agricultural” and “non-grain” cultivated land, and the management and conservation of cultivated land resources in Haikou, will be further strengthened in the future. Therefore, based on the natural development scenario, the transfer probabilities of forestland, grassland, and unused land to cultivated land were increased by 30%, the transfer probability of cultivated land to construction land was reduced by 70%, and the transfer probabilities of cultivated land to other land-use types were reduced by 60% (Table 4).
2.3.3.3.2. Transfer cost matrix
The transfer cost matrix reflects the ease of transfer of current land-use types to future ones. With the acceleration of urbanization, the difficulties of converting land-use types into construction land were decreasing, and the possibilities of conversions were increasing. In addition, the transformation of construction land into other land-use types often needs to pay high costs; according to the actual situation of Haikou, the transformation of construction land into other land-use types was scarce. Therefore, this study set construction land was not allowed to transfer to other land-use types. The conversions among other land-use types were set according to the different scenarios' features.
2.3.3.3.3. Neighborhood factor parameter
The parameter range of the neighborhood factor is [0, 1], and the closer the parameter value is to 1, the stronger the expansion capacity of the land-use type is. This study set the neighborhood factor parameter of each land-use type based on the proportion of the expanded area of each land-use type in the total land expanded area during 2010–2020.
3. Results
3.1. Spatiotemporal evolution of cultivated land
According to Table 5, the area of cultivated land in Haikou decreased from 75,628.04 ha in 2000 to 68,607.54 ha in 2020, with a total area reduction of 7,020.50 ha. Comparing the changes in cultivated land areas between 2000–2010 and 2010–2020, the loss of cultivated land area in Haixiu town, Chengxi town, and 21 street offices during 2000–2010 was more prominent, and the SLUDD of cultivated land in these three zones was −5.10, −3.21, and −3.82% respectively; while the cultivated land areas in Changliu and Lingshan towns showed slight positive growth, the SLUDD was 1.47 and 1.02%, respectively. During 2010–2020, the total SLUDD of cultivated land reached −0.70%, which tended to shrink significantly compared with the previous period. The decreases in Chengxi, Haixiu, Xixiu, Changliu towns, and 21 street offices were relatively evident, with the SLUDD of −5.91, −5.27, −4.47, −4.85, and −4.23%, respectively. From 2000 to 2020, the total SLUDD of cultivated land in Haikou was −0.46%, showing a general trend of shrinkage.
The land-use system of Haikou is a complete whole consisting of various land-use types. Other land-use types change inevitably accompanied by the reduction of the cultivated land area. Therefore, this study further explored the areas transferred between other land-use types and cultivated land by the land-use transfer matrix.
During 2000–2010, in terms of the transfer amount (Figure 3), construction land, water area, and forestland were the main targets of transfers in cultivated land area. Spatially (Figure 4), cultivated land was transferred into construction land and water in patches. Among them, the zones, where cultivated land was transferred to construction land, were concentrated at the junction of 21 street offices and surrounding towns. Cultivated land was transferred into the water areas, mainly in the eastern part of Yanfeng town, the southern part of Dongzhai port, and the coastal area of Xixiu town. Such water areas were dominated by ponds, indicating that the reclamation phenomenon was prominent in this period. During this period, forestland became one of the primary transfer objects of cultivated land area, probably related to the project of returning cultivated land to forest implemented in Hainan province in 2002. Since the conversion of cultivated land to forestland was mainly scattered in a point pattern in each town, this transfer phenomenon was not evident in space.
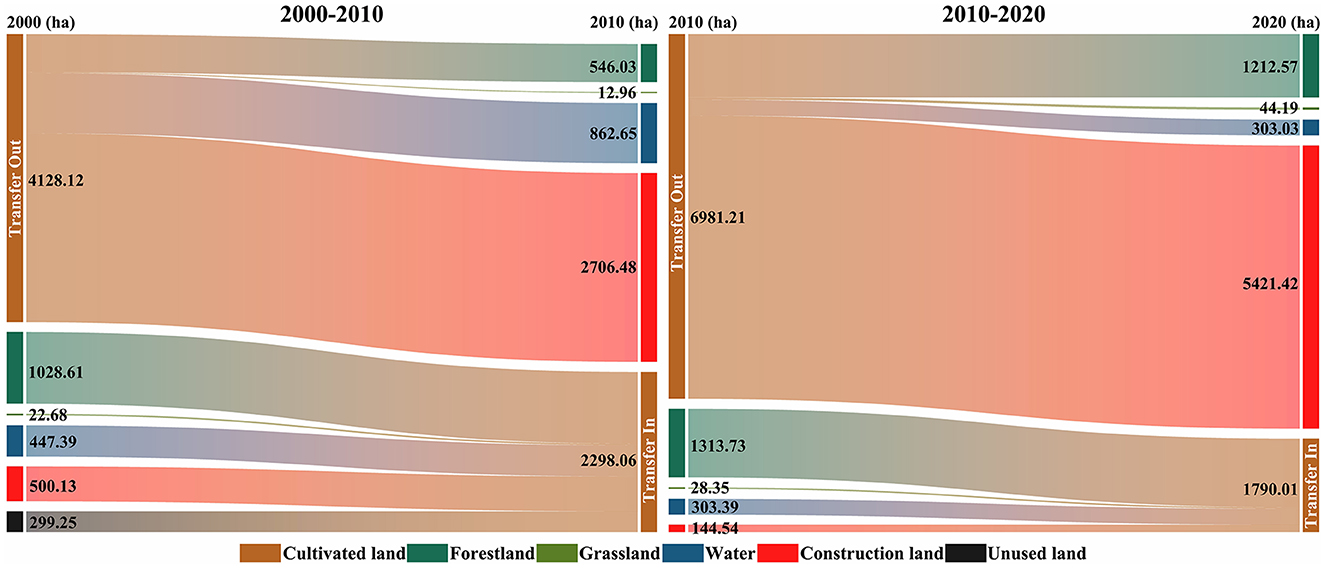
Figure 3. Transfer between other land-use types and cultivated land in Haikou during 2000–2020. The length of each land-use type node indicates the size of the area of that land-use type involved in the transfer part, the longer the node, the larger the area of that land-use type involved in the transfer part.
During 2010–2020, the areas of cultivated land transferred into other land-use types increased significantly compared with the previous period (Figure 3), especially construction land. The transfer of cultivated land into construction land accounted for 77.65% of the total transferred area. Spatially (Figure 4), the most prominent phenomenon was that cultivated land was transferred into construction land in large patches. Compared with the previous period, the cultivated land area transferred to construction land showed a trend of outward diffusion. The cultivated land in Xixiu, Changliu, and Lingshan towns was occupied by construction land in a large area. In 2010, the construction of Hainan International Tourism Island further accelerated Haikou's urbanization process. In 2011, the General Urban Planning of Haikou City (2011–2020), approved by The State Council, officially determined the construction of two new city clusters (Jiangdong and Changliu) (Figure 4). The development space of the city began to expand in both directions, and the urbanization processes of Xixiu, Changliu, and Lingshan towns in the Changliu and Jiangdong clusters were accelerated. In the same year, the westward relocation of the municipal government further promoted the development of the Changliu cluster, and the expansion trends of construction land in Xixiu and Changliu towns were further promoted. Under accelerated urbanization, the significant expansions of construction land in Xixiu, Changliu, and Lingshan towns caused substantial damage to the local cultivated land resources, resulting in a considerable loss of cultivated land area during this period.
3.2. Multi-scenario land-use patterns simulation
3.2.1. The PLUS model simulation accuracy validation
Based on the land-use data of Haikou in 2010, this study combined the growth probabilities of land-use types and the settings of relevant parameters in the CARS module to obtain the land-use patterns simulation of Haikou in 2020. The closer the value of the Kappa coefficient is to 1, the higher the simulation accuracy, and if its value reaches 0.8 or above, then the simulation accuracy of the model is satisfactory (Lin et al., 2020; Li et al., 2021). According to the simulation accuracy verification results, the overall accuracy of the simulation result was 92.28%, the Kappa coefficient was 0.879, and the FoM value was 0.275. The results indicated that the PLUS model is suitable for the land-use patterns simulation of Haikou.
3.2.2. Multi-scenario simulation results
As shown in Table 6, in the three scenarios of Haikou from 2020 to 2030, the areas of forestland, grassland, water area, and unused land all showed a decreasing trend, while the construction land area showed an increasing trend. The cultivated land areas showed a decreasing trend in both the natural and urban development scenarios but a slightly increasing trend in the cultivated land conservation scenario. The changes in cultivated land, forestland, and construction land were noticeable in these three scenarios. Compared with 2020, the construction land area increased by 9,550.89 ha, 12,544.20 ha, and 6,002.55 ha, respectively, under the natural development scenario, urban development scenario, and cultivated land conservation scenario.
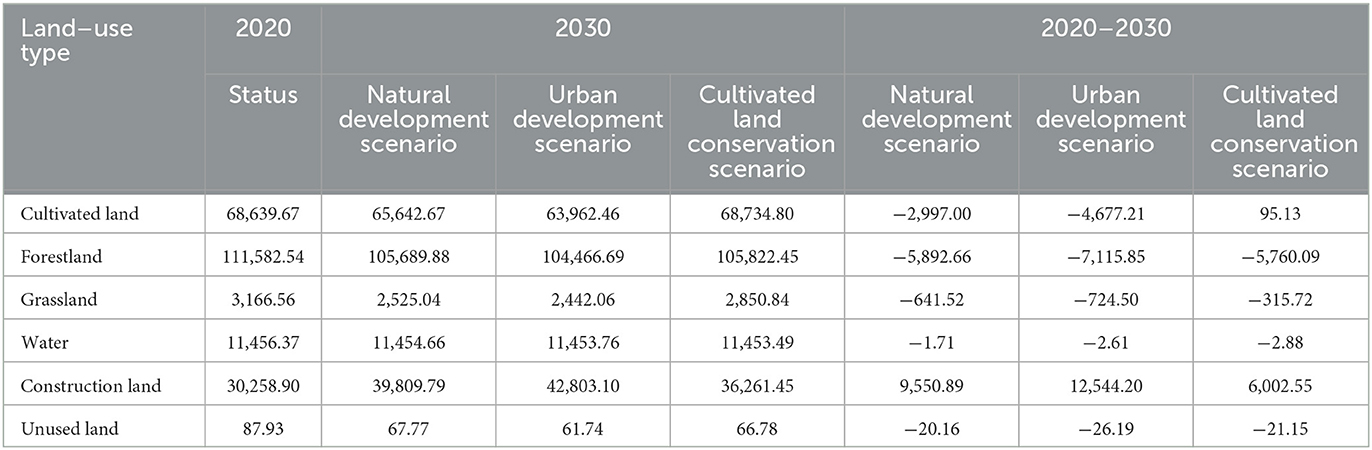
Table 6. Areas of land-use types of land-use status in 2020 and land-use simulation scenarios in 2030 (ha).
3.2.2.1. Natural development scenario
Under this scenario, the area of each land-use type, except for construction land, shrunk to different degrees, among which the shrinkages of cultivated land and forestland were more prominent, with the area of cultivated land and forestland decreasing by 2,997.00 ha and 5,892.66 ha, respectively, compared with 2020. Spatially (Figure 5), in comparison with the land-use patterns in 2020, the expansion of construction land under the natural development scenario in 2030 was noticeable, especially in Xixiu, Changliu, and Lingshan towns. Approximately 63.69% of the expansion area of construction land came from cultivated land. According to Figure 6, the places where cultivated land was transferred into construction land were mainly distributed in the Changliu and Jiangdong clusters. Compared with 2010–2020, the encroachment of cultivated land by construction land in the Jiangdong cluster tended to be evident under this scenario, mainly concentrated in the western part of Lingshan town, i.e., the eastern coast of Nandu River. In 2018, the Hainan Province Government decided to plan the construction of the Jiangdong New Area to serve as the critical area of the Free Trade Port, which will inevitably drive the expansion of construction land within the Jiangdong New Area. Combined with the simulation result, according to the current development trend, the eastern coastal area of the Nandu River in the Jiangdong cluster is likely to become a critical area for future construction and development, and a large amount of cultivated land in this area will face the risk of being encroached upon by construction land. Therefore, policymakers should pay more attention to protecting cultivated land in this area in Haikou's future urban planning process. In addition, Dongshan and Xinpo towns, where cultivated land resources are abundant, showed signs of construction land encroaching on cultivated land.
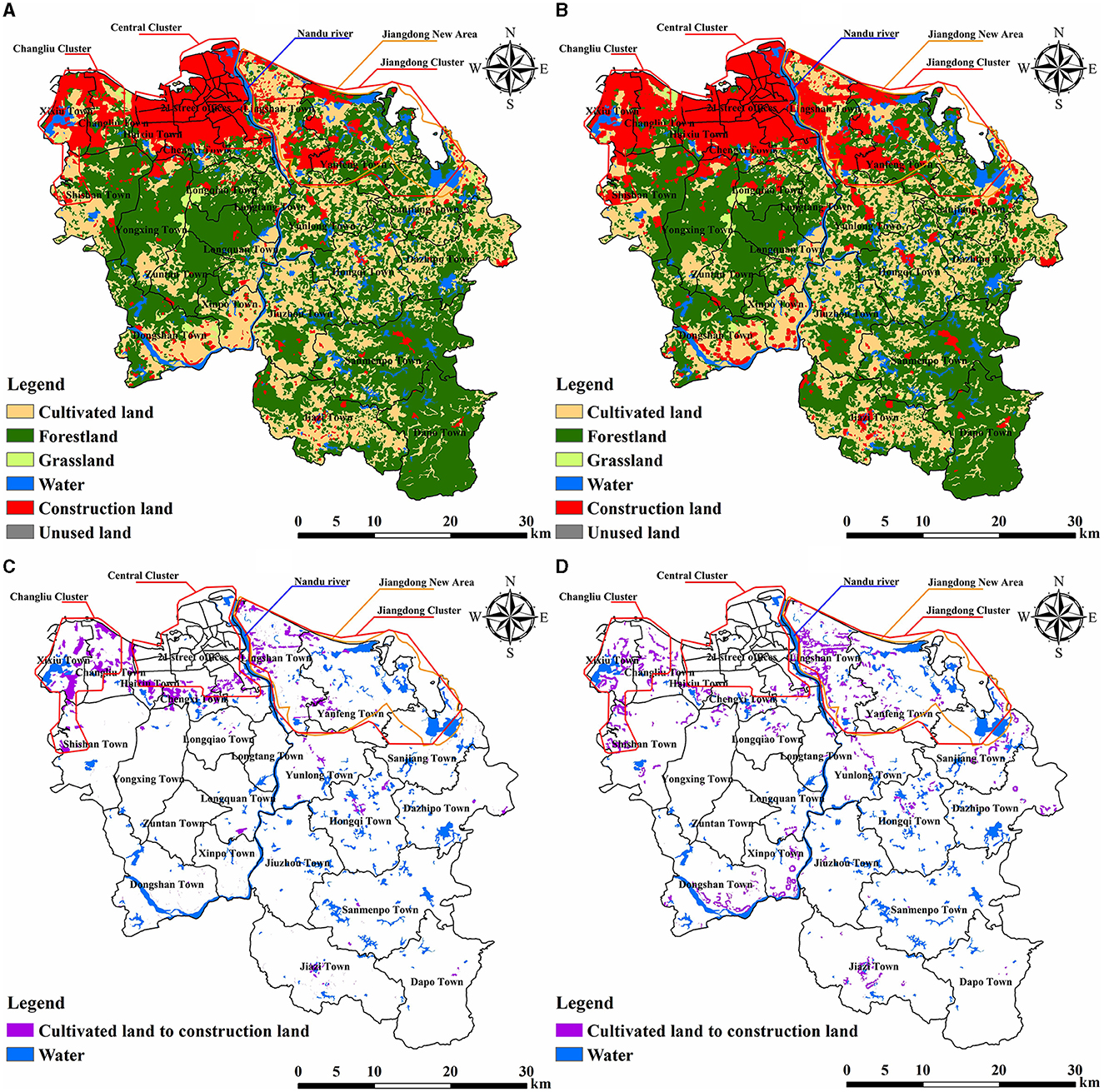
Figure 5. (A) Land-use status in 2020. (B) Natural development scenario in 2030. (C) Spatial distribution of cultivated land transferred into construction land during 2010–2020. (D) Spatial distribution of cultivated land transferred into construction land under natural development scenario during 2020–2030.
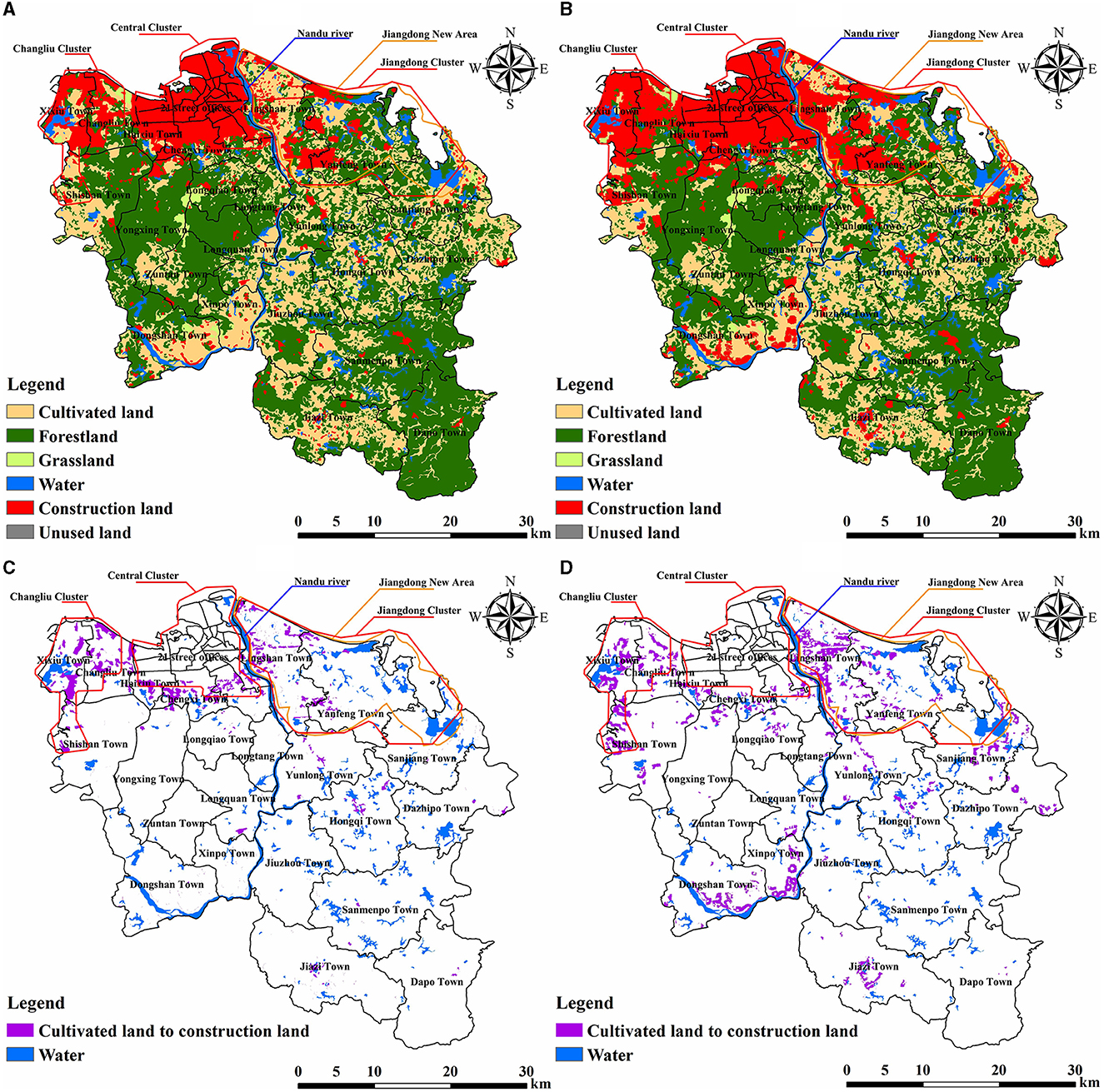
Figure 6. (A) Land-use status in 2020. (B) Urban development scenario in 2030. (C) Spatial distribution of cultivated land transferred into construction land during 2010–2020. (D) Spatial distribution of cultivated land transferred into construction land under urban development scenario during 2020–2030.
3.2.2.2. Urban development scenario
Compared with 2020, the construction land area in this scenario of 2030 increased by 12,544.20 ha, a significant expansion. In the parameters setting of this scenario, the conversion probabilities of cultivated land, forestland, grassland, and unused land to construction land were uniformly adjusted upward by 20% based on the natural development scenario. According to the simulation results, the cultivated land area transferred into construction land accounted for 71.91% of the expansion area of the construction land, indicating that cultivated land would remain the primary area source for expansion of construction land under the priority of urban development and indicated that cultivated land and construction land might share a common need for siting relative to other land-use types. This siting need drove construction land to continuously encroach on cultivated land in this scenario, thus further exacerbating the loss of cultivated land. Spatially (Figure 6), the expansion of construction land in the 2030 urban development scenario was more pronounced than in the 2020 status quo and 2030 natural development scenario. In Lingshan, Xixiu, Changliu, and Shishan towns, which are located in the northern city clusters (Jiangdong and Changliu), a large amount of cultivated land has been transferred to construction land in the form of patches; in Xinpo, Dongshan, and Jiazi towns, which are located in the southwestern part of the city, the conversion of cultivated land to construction land was also more pronounced; in the eastern part of the city, Sanjiang and Dazhipo towns also showed signs of cultivated land being encroached upon by construction land.
3.2.2.3. Cultivated land conservation scenario
In this scenario, the conversions of cultivated land to other land-use types were strictly limited. The cultivated land area under this scenario in 2030 was 68,734.80 ha, an increase of 95.13 ha compared with 2020. Compared with the other two scenarios, the loss of cultivated land resources was effectively controlled and achieved a small positive growth. The area of construction land increased by 6,002.55 ha compared with 2020, which was still growing, but the increase was reduced compared with the natural and urban development scenarios; under this scenario, the expansion of construction land was mainly satisfied by encroaching on forestland, approximately 97.59% of the expansion area came from forestland. According to Figure 7, the spatial expansion of construction land was mainly achieved by encroaching on the forestland around the built-up area in the north; comparing the land-use status in 2020 and the two scenarios of nature and urban development in 2030, the spatial expansion of construction land under the cultivated land conservation scenario slowed down significantly. In general, due to the need for socioeconomic development, the demand for construction land is bound to continue to increase, and its expansion is an inevitable trend. Under this scenario, although the construction land was still expanding, its overall expansion has been curbed to a certain extent. The threat to cultivated land resources has been dramatically reduced. However, it should be noted that, in this scenario, forestland resources became the primary source area of construction land expansion, which caused a certain degree of threat to the ecological resources of Haikou.
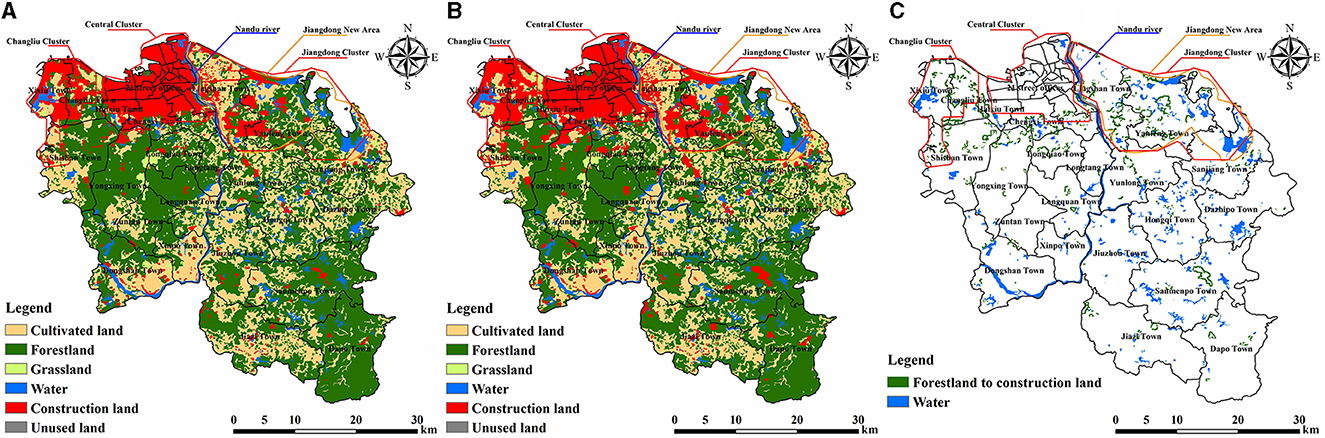
Figure 7. (A) Land-use status in 2020. (B) Cultivated land conservation scenario in 2030. (C) Spatial distribution of forestland transferred into construction land under cultivated land conservation scenario during 2020–2030.
4. Discussion
Urbanization is a significant symbol of social progress. This inexorable mainstream trend has improved people's living standards but threatens the security of particular well-being, such as food supply. Similar to the conclusion of most studies (Lu et al., 2005; Chen, 2007; Song and Deng, 2015; Yu et al., 2018), in this study, cultivated land, as a food supply service provider, was facing a significant threat from the expansion of construction land. From 1980 to 1999, the urbanization rate of Hainan Province was low. Even the rapid development of tourism during 1985–1994 only raised the rate to the national average level, it was not until late 1999, to pull Hainan out of economic recession, that the government introduced a new development strategy, and the land use began to receive more and more attention (Gu and Wall, 2007). After 2000, Hainan began to step out of the economic recession shadow, and all infrastructure constructions began to advance steadily. At the forefront of Hainan's economic development, Haikou also accelerated its urbanization process significantly (Zhou et al., 2017). This study analyzed the evolutionary trends of cultivated land resources in Haikou during 2000–2020. The results showed that the cultivated land loss in Haikou has been increasing in the two decades of 2000–2010 and 2010–2020, with the encroachment of construction land being the leading cause, which is consistent with the conclusions of Liu and Xin (2022), Li (2020), and Liu et al. (2019). Although construction land encroachment on cultivated land tended to spread to the periphery during 2010–2020, the main distribution area was still the main urban area in the north of Haikou. The concept of the main urban area was first proposed in the Overall Urban Planning of Haikou City (2011–2020), approved by The State Council in 2011. Haikou was divided into north and south districts, with the encircled expressway as the boundary. The northern district is the main urban area, the centralized urban development area. Thus, the trend of encroachment of cultivated land by construction land within the north main urban area is inevitable in the context of the continuous urbanization of Haikou. This trend was verified in the simulation results of the PLUS model. In the main urban area, compared with the Changliu cluster, the Jiangdong cluster's development started late, and “Haikou Jiangdong New Area of Hainan Free Trade Port” was officially launched in 2020. According to the natural and urban development scenarios in 2030, the encroachment of cultivated land by construction land on the east bank of the Nandu River was the most prominent phenomenon in the main urban area, which was very consistent with the future development trend of the Jiangdong area and also reflected the high simulation accuracy of the PLUS model from the side.
In both natural and urban development scenarios, Dongshan and Xinpo towns in the southwestern part of Haikou showed a more pronounced loss of cultivated land. In contrast, the SLUDDs of cultivated land in these two towns remained low until 2020. This abnormal phenomenon should cause alarm. The LEAS module calculated the growth probabilities of land-use types and the contribution degrees of driving factors to land expansions. From the contribution degrees of driving factors to construction land expansion (Figure 8A), the distances from water and road were the main driving factors for construction land expansion. We overlaid these two main driving factors with the construction land expansion area for further analysis (Figures 8B, C). It can be found that, in Dongshan and Xinpo towns, the areas where construction land encroached on cultivated land were mainly distributed along roads and water areas. Thus, the abundant water resources and convenient transportation conditions were likely to be the reasons for the expansion of construction land in these areas. The cultivated land resources in Dongshan and Xinpo towns were much more affluent than those in other city areas. With the support of the superior geographical location and other conditions, the cultivated land resources in these two towns have great potential to develop into high-quality cultivated land resources. The simulation results of the PLUS model reflected that the cultivated land resources of these two towns might face a considerable risk of loss in the future, which provided an important warning.
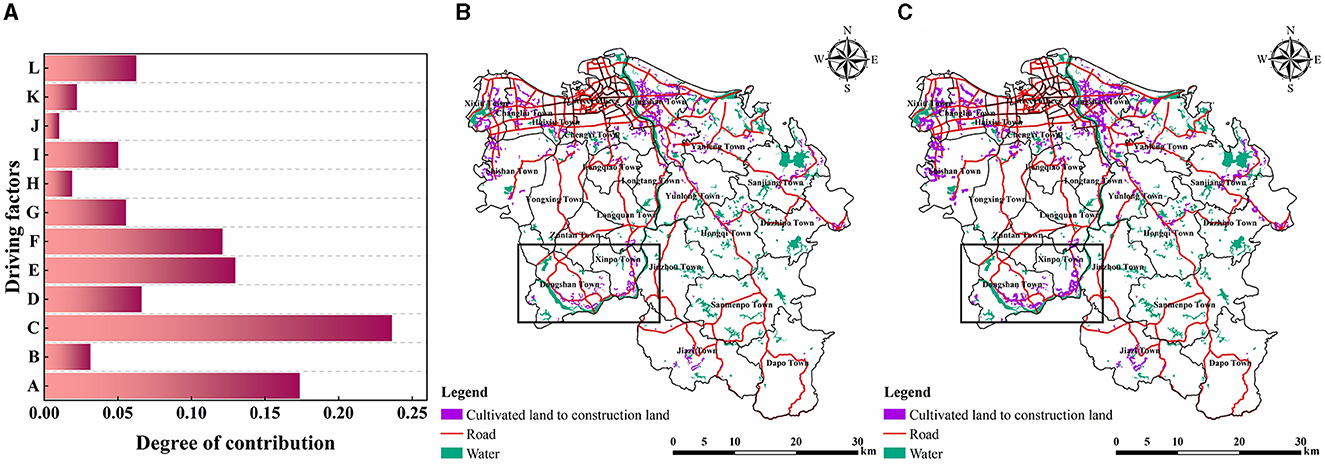
Figure 8. (A) Contribution degrees of driving factors to construction land expansion (A, Distance from road; B, DEM; C, Distance from water; D, Distance from highway; E, Distance from railway; F, GDP; G, Average annual precipitation; H, Slope; I, Population; J, Aspect; K, Average annual temperature; L, Distance from government). (B) Spatial distribution of cultivated land occupied by construction land under the natural development scenario in 2030. (C) Spatial distribution of cultivated land occupied by construction land under the urban development scenario in 2030.
This study took Haikou, a coastal city in the tropical region, as the study area and tentatively tries to apply the PLUS model, an emerging land-use simulation model, to the study of cultivated land resources in Haikou, hoping not only to fill the gap in the studies of cultivated land in Haikou but also to make some contributions to consolidate the theoretical research foundation of cultivated land resources utilization. In the future, the coupling application of the PLUS model with other algorithms or models on land-use change studies may become a new trend, for example, the GA-PLUS model (Li X. et al., 2022), SD-PLUS-InVEST model (Wang et al., 2022a), and InVEST-HFI-PLUS model (Wang et al., 2022b; Wei et al., 2022).
5. Conclusion
This study used the SLUDD and land-use transfer matrix to explore the spatiotemporal evolution of cultivated land in Haikou during 2000–2020 and introduced the PLUS model to predict the patterns of cultivated land under different scenarios in 2030. The results showed that:
(1) The demand for construction land in Haikou increased during 2000–2020, driven by rapid socioeconomic development and government policies. The rapid expansion of construction land posed a significant threat to the safety of adjacent cultivated land. During the 20 years, Haikou lost approximately 7,020.50 ha of cultivated land resources, with the most significant loss of cultivated land occurring in the suburbs. Suburban cultivated land is located in the transition zone between urban and rural areas, the most active area in urbanization. Under the influence of location conditions, differential land benefits, and urban economic radiation, cultivated land resources in this region are bound to come under more significant conservation pressure than in other regions. Therefore, this study suggested that local governments in peri-urban areas can actively develop emerging agriculture, improve farmers' agricultural income, reduce the income gap between urban and rural areas, and thus increase farmers' awareness of cultivated land use and conservation.
(2) According to the Haikou's land-use pattern in 2020 simulated by the PLUS model, the overall accuracy, Kappa coefficient, and FoM value reached 92.28%, 0.879, and 0.275, respectively, which proved that the model has good applicability for the simulation of future land-use patterns of Haikou. It should be noted that although the validation results of the simulation accuracy of the model were accurate, the prediction results may still be flawed, which were related to many factors, such as the selection of the driving factors and the data's accuracy. Therefore, future studies still need to take measures to improve the accuracy of the model simulation results.
(3) According to the predicted results of the PLUS model, the expansion of Haikou's construction land is an unstoppable trend in the future. Under the natural and urban development scenarios, in addition to the increased loss of cultivated land in the original location, the risk of loss has also been exposed in some areas with abundant cultivated land resources. Although there is a greater likelihood of continued outward expansion of construction land in the future, there is still hope that its expansion rate will be controlled. The simulation results of the cultivated land conservation scenario may provide some scientific references for curbing the uncontrolled outward expansion of construction land and protecting cultivated land in the future. The cultivated land loss is not irregular. Topography, location, and climatic conditions can all be the potential driving factors. Under the influence of these driving factors, even those areas where cultivated land remains stable year-round may be at risk of loss. The prediction results exposed new cultivated land loss sites in the city and contributed valuable information for conserving cultivated land resources.
Data availability statement
The data analyzed in this study is subject to the following licenses/restrictions: Authors are not allowed to share dataset. Requests to access these datasets should be directed to bGRzMW5mZSYjeDAwMDQwOzE2My5jb20=.
Author contributions
XL and HF: conceptualization and methodology. XL: software, formal analysis, and writing—original draft preparation. HF: writing—reviewing and editing, supervision, and funding acquisition. All the authors have read and agreed to the published version of the manuscript.
Funding
This research was funded by the Hainan Provincial Natural Science Foundation of China (Grant Nos. 421MS015 and 421QN200).
Conflict of interest
The authors declare that the research was conducted in the absence of any commercial or financial relationships that could be construed as a potential conflict of interest.
Publisher's note
All claims expressed in this article are solely those of the authors and do not necessarily represent those of their affiliated organizations, or those of the publisher, the editors and the reviewers. Any product that may be evaluated in this article, or claim that may be made by its manufacturer, is not guaranteed or endorsed by the publisher.
References
Chen, C., Liang, J., Xie, F., Hu, Z., Sun, W., Yang, G., et al. (2022). Temporal and spatial variation of coastline using remote sensing images for Zhoushan archipelago, China. Int. J. Appl. Earth Observ. Geoinform. 107, 102711. doi: 10.1016/j.jag.2022.102711
Chen, D., Yu, Q., Hu, Q., Xiang, M., Zhou, Q., and Wu, W. (2018). Cultivated land change in the Belt and Road Initiative region. J. Geograph. Sci. 28, 1580–1594. doi: 10.1007/s11442-018-1530-9
Chen, H., Chen, C., Zhang, Z., Lu, C., Wang, L., He, X., et al. (2021). Changes of the spatial and temporal characteristics of land-use landscape patterns using multi-temporal Landsat satellite data: a case study of Zhoushan Island, China. Ocean Coastal Manag. 213, 105842. doi: 10.1016/j.ocecoaman.2021.105842
Chen, J. (2007). Rapid urbanization in China: a real challenge to soil protection and food security. Catena 69, 1–15. doi: 10.1016/j.catena.2006.04.019
Chen, L., Song, G., Meadows, M. E., and Zou, C. (2018). Spatio-temporal evolution of the early-warning status of cultivated land and its driving factors: a case study of Heilongjiang Province, China. Land Use Policy 72, 280–292. doi: 10.1016/j.landusepol.2017.12.017
Chen, W., Chi, G., and Li, J. (2019). The spatial association of ecosystem services with land use and land cover change at the county level in China, 1995–2015. Sci. Total Environ. 669, 459–470. doi: 10.1016/j.scitotenv.2019.03.139
Chen, Y., Wang, S., and Wang, Y. (2022). Spatiotemporal evolution of cultivated land non-agriculturalization and its drivers in typical areas of southwest China from 2000 to 2020. Remote Sensing 14, 3211. doi: 10.3390/rs14133211
Cole, M. B., Augustin, M. A., Robertson, M. J., and Manners, J. M. (2018). The science of food security. NPJ Sci. Food 2, 1–8. doi: 10.1038/s41538-018-0021-9
Gao, L., Tao, F., Liu, R., Wang, Z., Leng, H., and Zhou, T. (2022). Multi-scenario simulation and ecological risk analysis of land use based on the PLUS model: a case study of Nanjing. Sustain. Cities Soc. 85, 104055. doi: 10.1016/j.scs.2022.104055
Gu, K., and Wall, G. (2007). Rapid urbanization in a transitional economy in China: the case of Hainan Island. Singapore J. Trop. Geography 28, 158–170. doi: 10.1111/j.1467-9493.2007.00288.x
Jiang, Y., Huang, M., Chen, X., Wang, Z., Xiao, L., Xu, K., et al. (2022). Identification and risk prediction of potentially contaminated sites in the Yangtze River Delta. Sci. Total Environ. 815, 151982. doi: 10.1016/j.scitotenv.2021.151982
Lai, Z., Chen, M., and Liu, T. (2020). Changes in and prospects for cultivated land use since the reform and opening up in China. Land Use Pol. 97, 104781. doi: 10.1016/j.landusepol.2020.104781
Li, C., Wu, Y., Gao, B., Zheng, K., Wu, Y., and Li, C. (2021). Multi-scenario simulation of ecosystem service value for optimization of land use in the Sichuan-Yunnan ecological barrier, China. Ecol. Indic. 132, 108328. doi: 10.1016/j.ecolind.2021.108328
Li, D., He, L., Qu, J., and Xu, X. (2022). Spatial evolution of cultivated land in the Heilongjiang Province in China from 1980 to 2015. Environ. Monit. Asses. 194, 1–16. doi: 10.1007/s10661-022-10119-3
Li, T. (2020). Analysis of land use dynamic change in Meilan district of Haikou city based on remote sensing images. Int. J. Sci. 7.
Li, X., Fu, J., Jiang, D., Lin, G., and Cao, C. (2022). Land use optimization in Ningbo City with a coupled GA and PLUS model. J. Clean. Prod. 375, 134004. doi: 10.1016/j.jclepro.2022.134004
Liang, X., Guan, Q., Clarke, K. C., Liu, S., Wang, B., and Yao, Y. (2021). Understanding the drivers of sustainable land expansion using a patch-generating land use simulation (PLUS) model: A case study in Wuhan, China. Comp. Environ. Urban Syst. 85, 101569. doi: 10.1016/j.compenvurbsys.2020.101569
Lin, W., Sun, Y., Nijhuis, S., and Wang, Z. (2020). Scenario-based flood risk assessment for urbanizing deltas using future land-use simulation (FLUS): Guangzhou Metropolitan Area as a case study. Sci. Total Environ. 739, 139899. doi: 10.1016/j.scitotenv.2020.139899
Liping, C., Yujun, S., and Saeed, S. (2018). Monitoring and predicting land use and land cover changes using remote sensing and GIS techniques—a case study of a hilly area, Jiangle, China. PLoS ONE 13, e0200493. doi: 10.1371/journal.pone.0200493
Liu, F., Zhang, Z., Zhao, X., Wang, X., Zuo, L., Wen, Q., et al. (2019). Chinese cropland losses due to urban expansion in the past four decades. Sci. Total Environ. 650, 847–857. doi: 10.1016/j.scitotenv.2018.09.091
Liu, J., Jin, X., Xu, W., and Zhou, Y. (2022). Evolution of cultivated land fragmentation and its driving mechanism in rural development: a case study of Jiangsu Province. J. Rural Stud. 91, 58–72. doi: 10.1016/j.jrurstud.2022.02.011
Liu, J., Kuang, W., Zhang, Z., Xu, X., Qin, Y., Ning, J., et al. (2014). Spatiotemporal characteristics, patterns, and causes of land-use changes in China since the late 1980s. J. Geograph. Sci. 24, 195–210. doi: 10.1007/s11442-014-1082-6
Liu, X., Liang, X., Li, X., Xu, X., Ou, J., Chen, Y., et al. (2017). A future land use simulation model (FLUS) for simulating multiple land use scenarios by coupling human and natural effects. Landscape Urban Plan. 168, 94–116. doi: 10.1016/j.landurbplan.2017.09.019
Liu, X., and Xin, L. (2022). Assessment of the efficiency of cultivated land occupied by urban and rural construction land in China from 1990 to 2020. Land 11, 941. doi: 10.3390/land11060941
Lu, Q., Söderlund, L., Wu, P., and Li, J. (2005). Cultivated land loss arising from the rapid urbanization in China. Söderlund, L., Sippola, J., and Kamijo-Söderlund, M., editors. In: Sustainable Agroecosystem Management and Development of Rural-Urban Interaction in Regions and Cities of China. Paper presented at the Proceedings SUSDEV-CHINA Symposium.
Lv, Z., Huang, H., Gao, L., Benediktsson, J. A., Zhao, M., and Shi, C. (2022a). Simple multiscale unet for change detection with heterogeneous remote sensing images. IEEE Geosci. Remote Sens. Let. 19, 1–5. doi: 10.1109/LGRS.2022.3173300
Lv, Z., Wang, F., Cui, G., Benediktsson, J. A., Lei, T., and Sun, W. (2022b). Spatial–spectral attention network guided with change magnitude image for land cover change detection using remote sensing images. IEEE Trans. Geosci. Remote Sens. 60, 1–12. doi: 10.1109/TGRS.2022.3197901
Lyu, X., Wang, Y., Niu, S., and Peng, W. (2021). Spatio-temporal pattern and influence mechanism of cultivated land system resilience: case from China. Land 11, 11. doi: 10.3390/land11010011
McMichael, P., and Schneider, M. (2011). Food security politics and the Millennium Development Goals. Third World Q. 32, 119–139. doi: 10.1080/01436597.2011.543818
Pérez-Escamilla, R. (2017). Food security and the 2015–2030 sustainable development goals: from human to planetary health: perspectives and opinions. Current Dev. Nutr. 1, e000513. doi: 10.3945/cdn.117.000513
Prosekov, A. Y., and Ivanova, S. A. (2018). Food security: the challenge of the present. Geoforum 91, 73–77. doi: 10.1016/j.geoforum.2018.02.030
Shi, M., Wu, H., Fan, X., Jia, H., Dong, T., He, P., et al. (2021). Trade-offs and synergies of multiple ecosystem services for different land use scenarios in the yili river valley, China. Sustainability 13, 1577. doi: 10.3390/su13031577
Song, W., and Deng, X. (2015). Effects of urbanization-induced cultivated land loss on ecosystem services in the North China Plain. Energies 8, 5678–5693. doi: 10.3390/en8065678
Subedi, P., Subedi, K., and Thapa, B. (2013). Application of a hybrid cellular automaton–Markov (CA-Markov) model in land-use change prediction: a case study of Saddle Creek Drainage Basin, Florida. Appl. Ecol. Environ. Sci. 1, 126–132. doi: 10.12691/aees-1-6-5
Sun, W., Ren, K., Meng, X., Yang, G., Xiao, C., Peng, J., et al. (2022). MLR-DBPFN: A multi-scale low rank deep back projection fusion network for anti-noise hyperspectral and multispectral image fusion. IEEE Trans. Geosci. Remote Sens. 60, 1–14. doi: 10.1109/TGRS.2022.3146296
Vågsholm, I., Arzoomand, N. S., and Boqvist, S. (2020). Food security, safety, and sustainability—getting the trade-offs right. Front. Sustain. Food Syst. 4, 16. doi: 10.3389/fsufs.2020.00016
Verburg, P. H., Soepboer, W., Veldkamp, A., Limpiada, R., Espaldon, V., and Mastura, S. S. (2002). Modeling the spatial dynamics of regional land use: the CLUE-S model. Environ. Manag. 30, 391–405. doi: 10.1007/s00267-002-2630-x
Wang, X. (2022). Changes in cultivated land loss and landscape fragmentation in China from 2000 to 2020. Land 11, 684. doi: 10.3390/land11050684
Wang, Z., Huang, C., Li, L., and Lin, Q. (2022a). Ecological zoning planing and dynamic evaluation coupled with Invest-HFI-Plus model: a case study in Bortala Mongolian Autonomous Prefecture. Acta Ecol. Sin 42, 5789–5798. doi: 10.5846/stxb202108202319
Wang, Z., Li, X., Mao, Y., Li, L., Wang, X., and Lin, Q. (2022b). Dynamic simulation of land use change and assessment of carbon storage based on climate change scenarios at the city level: A case study of Bortala, China. Ecol. Indic. 134, 108499. doi: 10.1016/j.ecolind.2021.108499
Wei, Q., Abudureheman, M., Halike, A., Yao, K., Yao, L., Tang, H., et al. (2022). Temporal and spatial variation analysis of habitat quality on the PLUS-InVEST model for Ebinur Lake Basin, China. Ecol. Indicat. 145, 109632. doi: 10.1016/j.ecolind.2022.109632
Xu, X., Hu, H., Tan, Y., Yang, G., Zhu, P., and Jiang, B. (2019). Quantifying the impacts of climate variability and human interventions on crop production and food security in the Yangtze River Basin, China, 1990–2015. Sci. Total Environ. 665, 379–389. doi: 10.1016/j.scitotenv.2019.02.118
Xu, X., Liu, J., Zhang, S., Li, R., Yan, C., and Wu, S. (2018). China's Multi-period Land Use Land Cover Remote Sensing Monitoring Data Set (CNLUCC). Beijing: Resource and Environment Data Cloud Platform.
Yadav, S. S., Hegde, V., Habibi, A. B., Dia, M., and Verma, S. (2019). Climate change, agriculture and food security. Yadav, S.S., Redden, R.J., Hatfield, J.L., editors. Food Security and Climate Change. 1st ed. Hoboken, NJ: John Wiley and Sons Ltd.
Yang, B., Wang, Z., Zou, L., Zou, L., and Zhang, H. (2021). Exploring the eco-efficiency of cultivated land utilization and its influencing factors in China's Yangtze River Economic Belt, 2001–2018. J. Environ. Manag. 294, 112939. doi: 10.1016/j.jenvman.2021.112939
Yang, Y., Bao, W., Li, Y., Wang, Y., and Chen, Z. (2020). Land use transition and its eco-environmental effects in the Beijing–Tianjin–Hebei urban agglomeration: a production–living–ecological perspective. Land 9, 285. doi: 10.3390/land9090285
Yu, D., Wang, D., Li, W., Liu, S., Zhu, Y., Wu, W., et al. (2018). Decreased landscape ecological security of peri-urban cultivated land following rapid urbanization: an impediment to sustainable agriculture. Sustainability 10, 394. doi: 10.3390/su10020394
Zhai, H., Lv, C., Liu, W., Yang, C., Fan, D., Wang, Z., et al. (2021). Understanding spatio-temporal patterns of land use/land cover change under urbanization in Wuhan, China, 2000–2019. Remote Sens. 13, 3331. doi: 10.3390/rs13163331
Zhao, X., Zhang, Z., Wang, X., Zuo, L., Liu, B., Yi, L., et al. (2014). Analysis of Chinese cultivated land′ s spatial-temporal changes and causes in recent 30 years. Trans. Chin. Soc. Agri. Eng. 30, 1–11. doi: 10.3969/j.issn.1002-6819.2014.03.001
Zhou, T., Zhao, R., and Zhou, Y. (2017). Factors influencing land development and redevelopment during China's rapid urbanization: evidence from Haikou city, 2003–2016. Sustainability 9, 2011. doi: 10.3390/su9112011
Keywords: cultivated land, spatiotemporal evolution, forecast, PLUS model, multi-scenario simulation, Haikou City
Citation: Lin X and Fu H (2023) Multi-scenario simulation analysis of cultivated land based on PLUS model—a case study of Haikou, China. Front. Ecol. Evol. 11:1197419. doi: 10.3389/fevo.2023.1197419
Received: 31 March 2023; Accepted: 08 May 2023;
Published: 02 June 2023.
Edited by:
Gary R. Sands, University of Minnesota Twin Cities, United StatesReviewed by:
Chao Chen, Suzhou University of Science and Technology, ChinaDewen Liu, Nanjing University of Posts and Telecommunications, China
Copyright © 2023 Lin and Fu. This is an open-access article distributed under the terms of the Creative Commons Attribution License (CC BY). The use, distribution or reproduction in other forums is permitted, provided the original author(s) and the copyright owner(s) are credited and that the original publication in this journal is cited, in accordance with accepted academic practice. No use, distribution or reproduction is permitted which does not comply with these terms.
*Correspondence: Hui Fu, aWZseWluZyYjeDAwMDQwOzEyNi5jb20=