- 1Heilongjiang Genuine Wild Medicinal Materials Germplasm Resources Research Center, School of Life Sciences and Technology, Harbin Normal University, Harbin, China
- 2Forest Botanical Garden of Heilongjiang Province, Harbin, China
Objective: Phellodendron amurense, a special species in Northeast Asia, is the source of the wild medicinal Phellodendri Amurensis Cortex, the second-level key protection in China. Because of its dual value of medicine and timber, it has been cut in large quantities, resulting in a sharp decline in wild resources. It has been listed as a national first-class rare tree species. Here, we aim to plan protection area and wild tending area for Chinese P. amurense in the context of climate change.
Method: In this study, based on the Biomod2 model, the main environmental factors affecting the distribution and the potentially suitable areas of the current and future were investigated. Furthermore, the correlation between the main environmental factors and the chemical components was determined by full subset regression. Habitat quality and landscape pattern change were determined by InVEST and Fragstats software based on the land use data in 1980, 2000, and 2020. Then, Zonation software was used to plan the priority protection and wild tending areas.
Results: The results showed that in the future, the potential habitat of P. amurense will gradually move to the high latitude and high altitude areas in the northwest direction, and the high suitability area will decrease sharply. Since 1980, the habitat quality of P. amurense habitat has gradually deteriorated, and the fragmentation has gradually intensified. In the future, the central part of the Greater Khingan Mountains and the forest area of the Lesser Khingan Mountains will be the long-term stable sanctuary. Fengcheng City and Tonghua County are the most suitable for the wild tending areas.
Conclusion: The existing nature reserve only contains 6.01% of the priority protection area, so we propose to expand the area to cover a larger proportion of the sanctuary and implement management and restoration plans to increase the suitability and connectivity. These results can support the protection action and provide a reference and scientific basis for further research, rational development, and utilization of P. amurense.
1. Introduction
In large-scale space, climate plays an important role in determining the spatial and temporal distribution of species, and the reason why species can only grow in an environment is that species adapt to specific climatic conditions within their geographical range (Araújo et al., 2005; Pant et al., 2021). Since the 19th century, due to the intensification of human activities, the global temperature has increased by around 1°C on average (Sommer et al., 2010; Ma et al., 2022). In response to climate change, the geographical distribution of many plants will change (Zhao et al., 2021). Compared with the past migration rate of plant species, many plants may not be able to adapt to the current rapid climate change (Huntley, 1991; Davis and Shaw, 2001; Elias et al., 2017). The average lifespan of individuals and the age at the time of reproductive maturity partly determines the adaptation potential of plant populations to climate change. Subject to other conditions, due to the length of generation and lifespan, annual plants adapt faster to climate change, while perennials adapt more slowly (Jump and Peñuelas, 2005). Rapid climate change may pose a more serious threat to some endangered woody plants’ survival.
Phellodendron amurense, a deciduous tree of the genus Phellodendron of Rutaceae (Figures 1B–D). It is an endemic species in Northeast Asia (Ma et al., 2015). And it is mainly distributed in China, the Far East of Russia, the Korean Peninsula, and Japan (Wei et al., 2019). In China, P. amurense is mainly distributed in the Northeast China and North China (Chinese Botanical Society, 1997), which is a national first-class rare tree species, second-class protected plant, and second-class key protected wild medicinal material in China. Its bark is called “Phellodendri Amurensis Cortex,” a rare traditional Chinese medicine with anticancer and antiviral effects (Zhao et al., 2013; Zhang and Zhang, 2019; National Pharmacopoeia Commission, 2020). It is also one of the three famous hardwoods in Northeast China, which has important ecological and economic value (Zhang and Wang, 2014). Since the 1980s, wild resources have been plundered and seriously damaged due to their high commercial value. In addition, the poor self-renewal ability has further exacerbated the sharp decline of wild resources (Zhang et al., 2016), leading to a serious imbalance in the supply relationship.
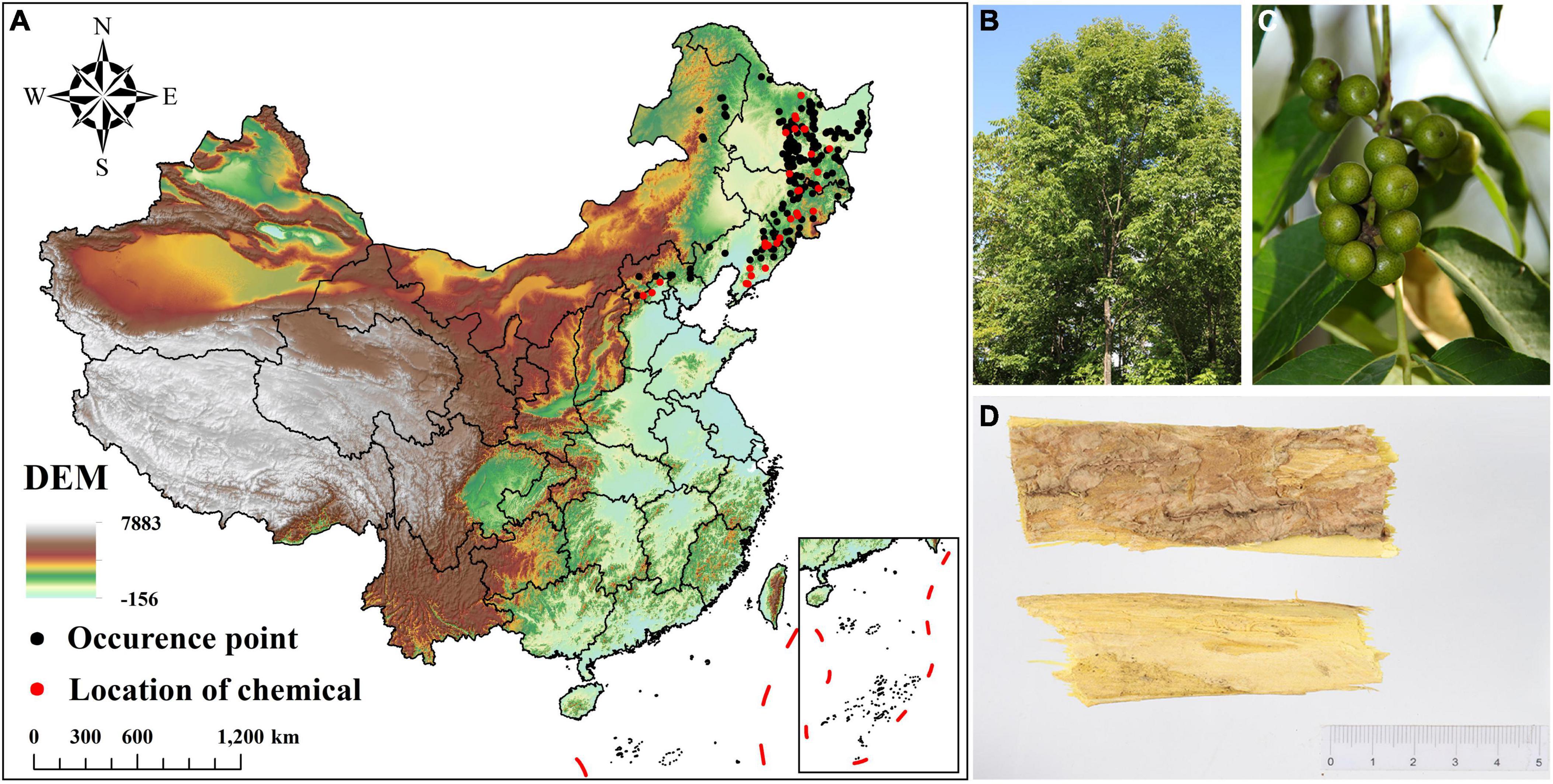
Figure 1. Occurrence data and morphological characteristics of Phellodendron amurense. (A) Occurrence data and geographical distribution of chemical components of P. amurense; (B) Plants of P. amurense; (C) Fruits of P. amurense; (D) Bark of P. amurense (medicinal material).
The conservation of rare species has shifted from focusing on the intrinsic value of species to conservation ecosystem services and the benefits provided to people (Groner et al., 2023). The establishment of nature reserves is the most effective means to slow down the rate of species extinction. However, the current nature reserves are mostly designed according to the current distribution of species, which is difficult to meet the needs for habitat protection under future climate change (Ran et al., 2019). In addition, wild tending is also another way to protect wild resources, which represents a way of artificially or naturally increasing the population size in its native or similar environment based on the growth characteristics of the target species and their requirements for ecological environment conditions, so that its resources can be collected and utilized by people and can continue to maintain community balance (Chen et al., 2004). This approach can not only solve the shortage of medical resources but also promote the recovery of the population (Chen et al., 2016). The production and change of effective components of medicinal plants have a strong correlation and correspondence with the environment (Zeng et al., 2015). Therefore, the relationship between the quality of medicinal plants and their habitat must be considered before specifying the wild tending plan (Shen et al., 2021).
This study constructed a new analytical framework. In this framework, ten species distribution models based on the Biomod2 were used to identify the main environmental factors affecting the spatial distribution of P. amurense in the Chinese region, and the correlation between the main environmental factors and chemical components content was clarified using full subset regression. Then, we combined the above analysis results with land use data to evaluate the changes in the landscape pattern of the habitat of P. amurense, and planned priority protected areas and wild tending areas. In addition, the framework takes into account the synergistic effect of habitat fragmentation and climate change, and aims to provide support for the conservation action and sustainable use of resources of P. amurense in China under the background of global climate change. Meanwhile, this framework can also provide effective reference for the management of medicinal plant resources in China. The purpose of this study is to: (1) determine the environmental factors that limit the distribution of P. amurense and their correlation with the chemical components in China; (2) explore the influence of climate change on the distribution of P. amurense in China; (3) explore the habitat quality and connectivity of the habitat of P. amurense in China; (4) plan the priority protection and wild tending areas of P. amurense, and propose protection management strategies in China; (5) provide a reference method for the protection of other rare and endangered wild species.
2. Materials and methods
2.1. Occurring data source and processing
This study obtains the distribution data of P. amurense in three ways: (1) Field investigation. From July to September 2017–2022, an extensive survey was conducted, totaling 185 geographical points; (2) Network database. The Global Biodiversity Information Facility,1 the Chinese Virtual Herbarium,2 and the National Specimen Information Infrastructure 3have collected 154 geographical occurrence points of P. amurense from 2000 to now; (3) Literature search. A total of 118 geographical data points have been collected through Google Academic, CNKI, and other platforms (e.g., Wan et al., 2014; Zhang et al., 2016). Google earth pro software (Google Earth USA) was used to eliminate the unreasonable geographical location data of P. amurense in waters and cultivated land. The “spThin” package in R 4.1.0 eliminates the geographic location data gathered within 10 km (Aiello-Lammens et al., 2015). Finally, we reserved 193 geographical data points of P. amurense for modeling (Figure 1A).
2.2. Source and processing of environmental factor data
Phellodendron amurense is a sun loving plant, and solar radiation plays an important role in its growth, and its suitability for soil is extremely strong (Tang et al., 2014). Therefore, 31 environmental factors were selected for this study, including solar radiation and biological climate factors (Supplementary Table 1). Srad01-12 were downloaded from the WorldClim Database (v2.1),4 covering 1970–2000. In order to make environmental data more representative of current climate conditions as much as possible, we downloaded monthly mean minimum temperature, monthly mean maximum temperature, and monthly mean precipitation data (2000–2018) from the WorldClim Database (v2.1). Then, we used the biovar package in R 4.1.0 to calculate 19 climate factors (2000–2018). This study used the MaxEnt3.4.4 software to establish an initial model (modeling ten times) without adjusting parameters. Environmental factors with relative contribution rates of less than 1% were removed. Moreover, the correlation function in ENMTools software was used to conduct correlation analysis on the remaining environmental factors (Warren et al., 2010). Then, we screened the two environmental factors with | r| > 0.80 and retained the environmental factors with a larger contribution rate to prevent the high correlation between environmental factors from causing an over-fitting species distribution model (Goldsmit et al., 2020; Gomes et al., 2020). Finally, eight environmental factors were retained for final modeling.
We selected CNRM-CM6-1, MIROC-ES2L, and MRI-ESM2-0 general atmospheric circulation models from the WorldClim Database (v2.1) to establish the future species distribution model. Each model includes 4 years: 2021–2040 (2030s), 2041–2060 (2050s), 2061–2080 (2070s), and 2081–2100 (2090s). Each year includes four shared socio-economic paths (SSPs): SSP1-2.6, SSP2-4.5, SSP3-7.0, and SSP5-8.5. The four selected carbon emission scenarios, SSP1-2.6 to SSP5-8.5, represent the radiative forcing scenario from low to high (Jiang et al., 2020). In order to avoid the uncertainty caused by the prediction of a single general atmospheric circulation model (GCM), we used ArcMap10.5 software to average the data of three climate models in the same year and the carbon emission scenario. Because there is no matching future data for solar radiation data, in this study, we used solar radiation as a static variable in the species distribution modeling. The spatial resolution of the above environmental data is 2.5 min.
In this study, land use change in the suitable areas of P. amurense was compared using 1980, 2000, and 2020 land use data from the Resource and Environment Science and Data Center5 with a spatial resolution of 1 km. We reclassified them into cultivated land, forest, grassland, waters, construction land, and unused land.
2.3. Construction of species distribution model
The “Biomod2” package in R 4.1.0 integrates ten common species distribution models (GLM, GBM, GAM, CTA, ANN, SRE, FD, MARS, RF, and MaxEnt). This study used this package to simulate the spatial distribution of P. amurense and analyzed the relative importance of environmental factors used in modeling (Elias et al., 2017; Xu et al., 2021). Except for the MaxEnt model, all models were built with default parameters. The “kuenm” package in R 3.6.3 was used to evaluate the performance of the MaxEnt model under different combinations of multiplicators (RM) and eigenvalues (FC). A total of 572 candidate models were constructed by combining 17 regularization multiplier values (0.10–1, interval 0.10; 2–6, interval 1; 8, 10) and five features provided by the MaxEnt model (linear feature: L, secondary feature: Q, fragmentation feature: H, product feature: P and threshold feature: T). The optimal model is selected according to the delta in the Akaike information criterion (AICc) model. When the AICc value is minimum (deltaAICc = 0), it is considered the optimal model (Cobos et al., 2019). The optimized MaxEnt3.4.4 software parameters are RM = 0.30 and FC = LQP.
In Biomod2 package, 75% of the distribution data was set as training data and the other as testing data. The division of training data and testing data was repeated five times. In order to better simulate the actual distribution and reduce the spatial deviation, 5,000 pseudoabsence points (repeated three times) were randomly created to construct the model. In the end, 150 model results were constructed in this study. The most accurate way to verify the prediction accuracy of SDMs is to combine the true skill statistic (TSS) and the area under the receiver operating characteristic curve (AUC) to evaluate the model results (Bucklin et al., 2015; Zhang et al., 2020). The TSS value ranges from −1 to 1, and the AUC value ranges from 0.5 to 1. The closer the value is to 1, the stronger the model’s prediction ability is (Freitas et al., 2019; Zhao et al., 2021). For 150 model calculation results, models with an average TSS greater than 0.80 and an average AUC greater than 0.90 were used to calculate the final species distribution layer.
2.4. Classification of suitable areas and analysis of climate characteristics
We used the maximum training sensitivity plus specificity threshold (in this study is 0.375), which can maximize the TSS value to create a binary mapping, and has been proven to produce the most accurate prediction (Jiménez-Valverde and Lobo, 2007; de Andrade et al., 2020). Three grades of the suitable area were divided: low suitability area (0.375–0.50), medium suitability area (0.50–0.70), and high suitability area (≥0.70) (Chen et al., 2022). In order to compare the changing trend of the spatial pattern of P. amurense suitable area under the current and future climate, the Distribution Changes Between Binary SDMs tool in SDMTools (ArcMap10.5) was used to calculate the area of expansion, stability, and contraction. The centroid changes (lines) tool was used to calculate the centroid migration trend (Brown, 2014).
In order to analyze the changes in environmental characteristics in the current potential area, we randomly selected 1,000 points in the current distribution area. Then, we extracted the corresponding values of the 1,000 distribution points in the different climate conditions layer corresponding to the leading environmental variables. The average value and 95% quantile of the extracted value were calculated, and the density map was drawn to intuitively understand the environmental pressure that P. amurense will face in the future (Tagliari et al., 2021).
2.5. Habitat quality assessment of habitat
In order to find out the current and past changes in the habitat quality of P. amurense habitat, the Habitat Quality Model in the InVEST model was used to quantify the habitat quality in the study area (Akbari et al., 2021). The value of the habitat quality index is between 0 and 1. The higher the value, the better the habitat quality in the region (Liu et al., 2021). Because of the distribution characteristics of the P. amurense natural population, only forest landscape was used as the evaluation object. Cultivated land, urban land, rural residential land, and construction land were considered as threat sources of the habitat. The impact weights and distances of these four threat sources were assigned (Supplementary Table 2), and the habitat suitability of different land uses and their sensitivity to threat sources were determined (Supplementary Table 3; Yang et al., 2018). Finally, the results were classified by the natural discontinuities method. By doing this, we obtained low, medium, and high-quality habitats of P. amurense.
2.6. Habitat landscape pattern analysis
The landscape index was used to quantitatively study the degree and process of fragmentation in the suitable area of P. amurense based on the land use data in 1980, 2000, and 2020. Seven landscape indexes were calculated, including the number of patches (NP), the patch density (PD), the mean patch size (AREA_MN), the area-weighted mean shape index (SHAPE_AM), the area-weighted mean patch fractal dimension (FRAC_AM), the division index (DIVISION), and the aggregation index (AI). The spatial distribution pattern of seven landscape indexes in three periods was calculated using the moving window method of FRAGSTATS 4.2 software. Moreover, the specific method is shown in the literature (Ran et al., 2019). According to the growth environment of P. amurense, the forest land use type was selected to represent the landscape fragmentation degree of the natural population.
ArcMap10.5 was used to carry out dimensionless standard normalization processing for each index, and then principal component analysis (PCA) and calculation were carried out for the seven indexes in each period. The principal component whose cumulative contribution rate was greater than 98% was selected. The weighted sum tool in ArcMap10.5 was used to perform overlay analysis on the layers with the weight of each principal component as the coefficient. Finally, the habitat fragmentation layers of P. amurense in different periods were obtained through range standardization (Supplementary Table 4; Ran et al., 2019).
2.7. Prediction of chemical composition and correlation with environmental factors
In this study, a parameter relationship model was established between the chemical composition content of Phellodendri Amurensis Cortex and environmental factors to obtain high-quality medicinal materials range of wild tending areas. In order to ensure the consistency of data, we screened the collected data and literature, used the content of 31 groups of Berberine and Palmatine in the same literature, and obtained the relevant geographical location information (Figure 1A; Zhang, 2015). These geographical location data were also used to model the species distribution of P. amurense.
Based on the MuMIn (Multi-Model Interference) package in R 4.2.1, the correlation between chemical component content and environmental factors was analyzed by full subset regression (Xu et al., 2020). The method of full subset regression is to fit all the combination models that may appear in the prediction variables in this model in turn and screen the best model under the conditions of existing variables according to a certain standard (such as R2, AIC, and SBIC) (Ren, 2022). Selected the best model by adjusting AIC and used p-value to indicate the correlation between environmental factors and the chemical content of Berberine and Palmatine. A value less than 0.10% indicates a very significant correlation, a value range of 0.10–0.50% indicates a significant correlation, and a value range of 0.50–1.00% indicates a correlation. The specific formula is as follows:
Where C is the content of chemical components, xn is the environmental variable, and βi is the regression coefficient, the results were evaluated using the coefficient of determination (R2) and F-test (Eberly, 2007).
In order to quantify the distribution of each chemical component in space, the “raster calculator” function in ArcMap10.5 was used. According to the coefficient of each environmental factor in the regression equation, the environmental factor layer of Berberine and Palmatine in the suitable area of P. amurense was standardized after the Z-Score superposition. By doing this, we obtained the chemical composition distribution layer of Berberine and Palmatine.
2.8. Planning of priority protection areas and wild tending areas
In order to serve the protection action of P. amurense under the background of climate change, the core area partitioning algorithm in Zonation 4.0 software6 was used in this study. We input layers of the current and future suitable areas, habitat quality, and landscape fragmentation in the model to plan the priority protection area. The weights of all the layers were set as 1, and other parameters were the default values of the model. We set the warping factor to “1” and removed one grid at a time to optimize the results (Moilanen, 2007). Then, we classified the output results: the top 5% were taken as the high protection areas, 5–10% as the moderate protection areas, and 10–20% as the low protection areas (Moilanen et al., 2005).
Considering that development and constructive activities are forbidden in nature reserves, the existing protection areas and planned protection areas in China were removed from the current high suitability of P. amurense. Then the spatial distribution layer of Berberine and Palmatine content and the remaining areas of the current high suitability were input in Zonation software. The parameters were the same as planning the priority protection area. The natural discontinuity method is based on the natural grouping inherent in the data, identifying the classification interval, which can most appropriately group similar values, and maximize the differences between various classes (Li and Xu, 2020). Therefore, the low, medium, and high-quality wild tending areas of P. amurense were obtained by classifying the result by the natural discontinuity method.
3. Results
3.1. Distribution of current potential distribution and landscape pattern change of P. amurense
Under the current climate conditions, the suitable habitat of P. amurense is mainly distributed in Changbai Mountain, Longgang Mountain, Zhangguangcai Mountain, and Lesser Khingan Mountains, which formed an inverted triangle. And there are also a few parts in the Yanshan Mountain and the south-central part of the Greater Khingan Mountains (Figure 2a). The total area of suitable habitat is about 55.63 × 104km2. Specifically, the high suitability area is about 29.95 × 104km2. This area is mainly distributed in the southeast of Changbai Mountain, Zhangguangcai Mountain, Longgang Mountain, and Lesser Khingan Mountains, and a small amount in the middle of the Greater Khingan Mountains, the northern branch of Changbai Mountain and the middle of Yanshan Mountain. The medium suitability area is about 15.79 × 104km2. This area is mainly distributed in the surrounding area of high suitability and with a scattered dotted distribution in the Yanshan Mountains. Moreover, the low suitability area is about 9.59 × 104km2, mainly distributed in the marginal area connecting the medium suitability and non-suitability areas (Figure 2a).
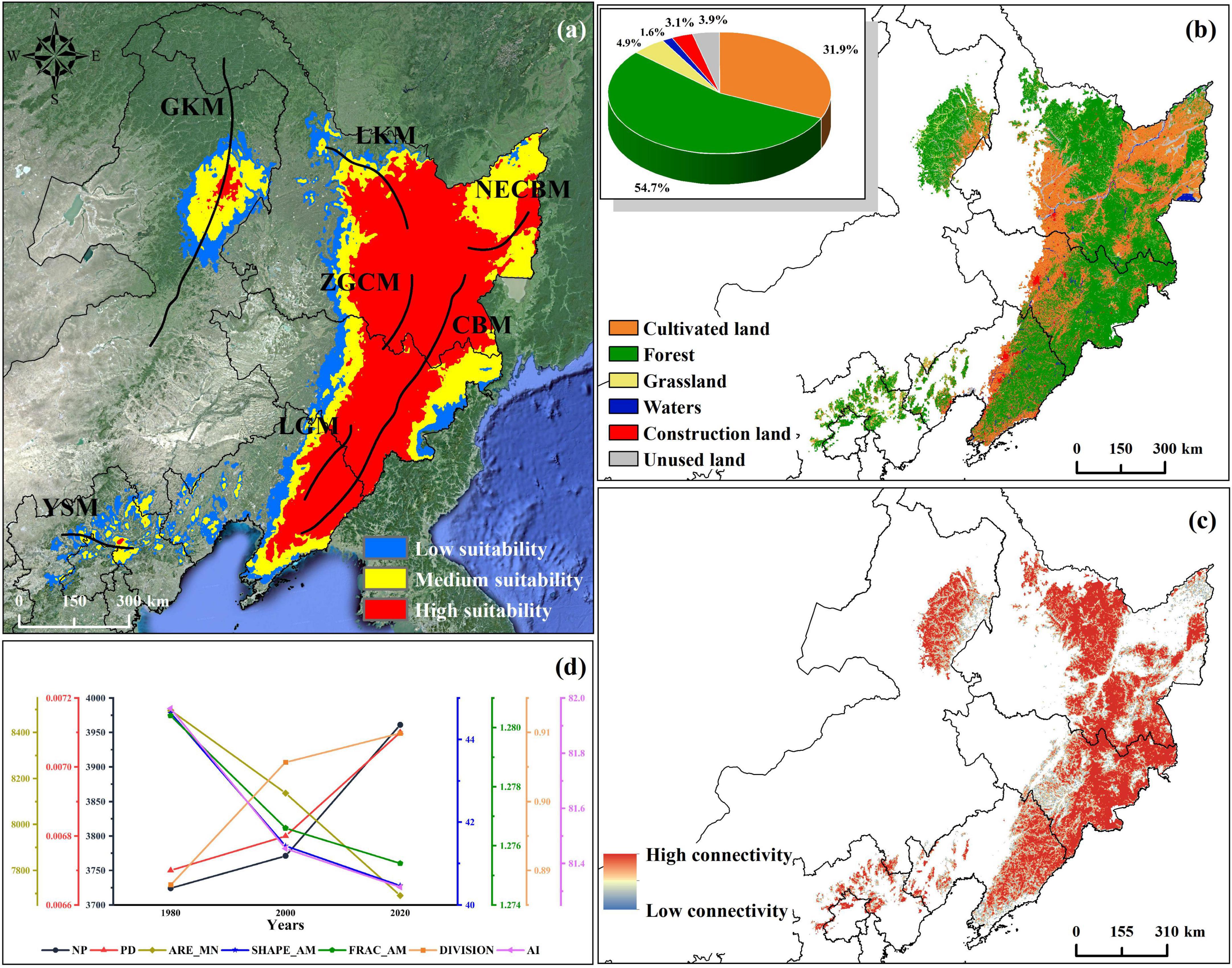
Figure 2. Distribution, land type, landscape fragmentation, and index change of suitable habitat of P. amurense under current climate conditions. (a) Distribution of suitable habitats of P. amurense under current climate conditions, of which abbreviations represents: GKM, Greater Khingan Mountains; LKM, Lesser Khingan Mountains; NECBM, North Extended Branch of Changbai Mountain; ZGCM, Zhang Guangcai Mountains; CBM, Changbai Mountains; LGM, Longgang Mountains; YSM, Yanshan Mountains. The distribution of mountain ranges has been improved from Wang et al. (2004); (b) Land use types of current potential distribution; (c) The landscape connectivity of the forests in the current potential habitat of P. amurense; (d) Change of landscape index of suitable areas in 1980, 2000, and 2020.
At present, there are six types of land use in the suitable habitat, including forest, grassland, waters, cultivated land, construction land, and unused land, of which the forest area is the largest, about 30.46 × 104km2, accounting for 31.85% of the total suitable area (Figure 2b). We used land use data to analyze the forest landscape pattern and fragmentation in the suitable habitat. A high fragmentation is found mostly at the edge of the landscape (Figure 2c). Furthermore, seven landscape pattern indexes increased or decreased year by year, showing a trend of gradual fragmentation. From 1980 to 2020, NP and PD increased by 6.36 and 5.97%; AREA_AM decreased 9.49% compared with 1980; SHAPE_AM decreased from 44.636 to 40.467; FRAC_AM decreased from 1.280 to 1.275; DIVISION increased by 0.022, 2.48% higher than that in 1980; and AI decreased by 0.648, or 0.79% (Figure 2d).
3.2. Habitat quality change of P. amurense habitat
From 1980 to 2020, the forest, grassland, waters, and unused land in the suitable habitat showed a downward trend, while cultivated and construction land showed an upward trend. The forest area decreased by 3.86% compared with 1980, mainly converted to cultivated land and grassland (Figure 3C). Notely, the conversion area gradually increased with the increase of years. Moreover, cultivated land and construction land increased by 12.80 and 36.32% compared with 1980.
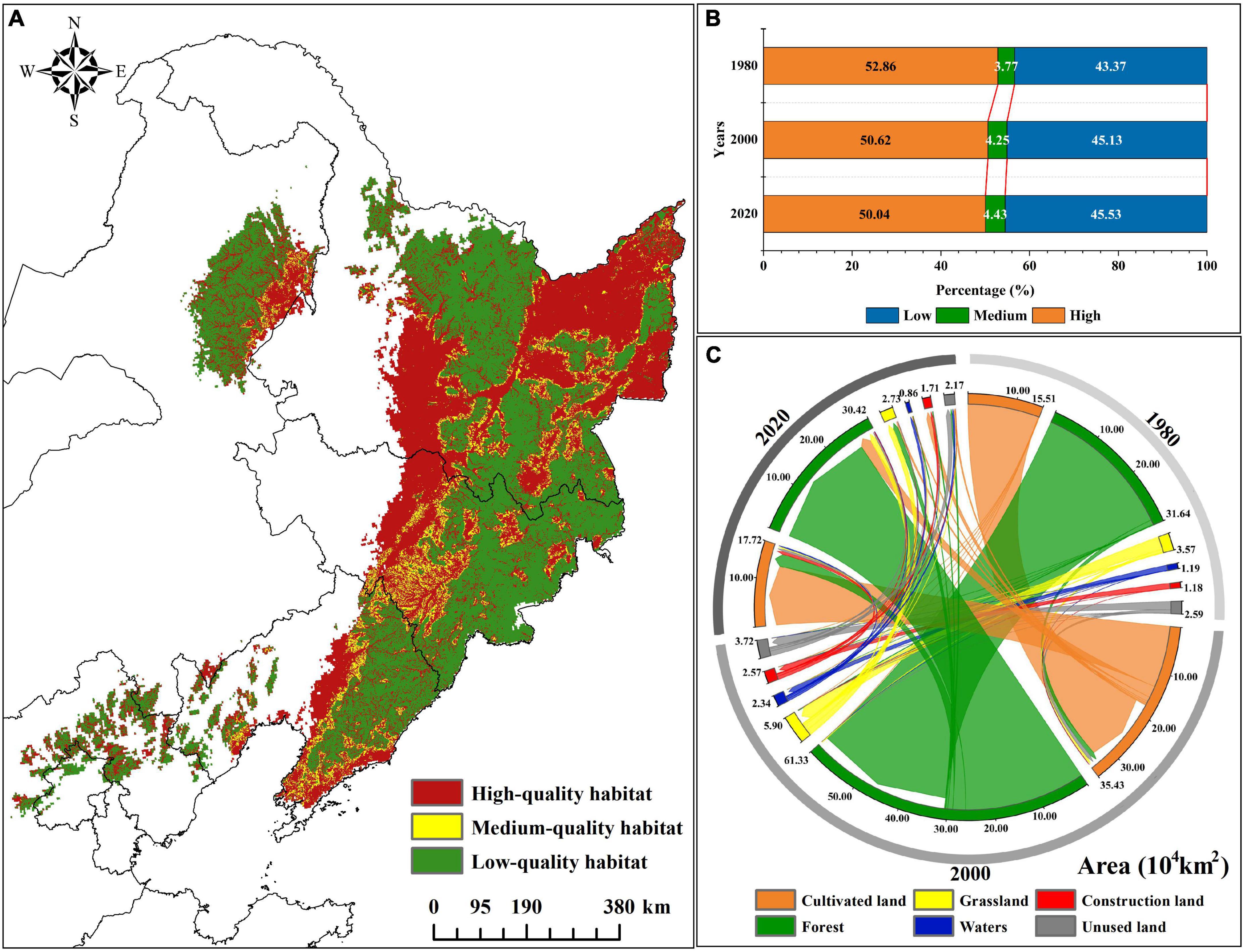
Figure 3. Habitat quality and land use change of current potential distribution of P. amurense. (A) The habitat quality of the current potential distribution; (B) Change of habitat quality area of different levels in 1980, 2000, and 2020; (C) Conversion area of land use type in 1980, 2000, and 2020.
In the forest suitable for the survival and development of population, the average value of habitat quality decreased from 0.53 in 1980 to 0.51 in 2020, showing a downward trend (Supplementary Table 5). Among them, the area of high-quality habitat showed a downward trend in the past 40 years. The area of high-quality habitat in 1980 was about 29.43 × 104 km2, and the area of high-quality habitat in 2000 and 2020 decreased by 4.23 and 5.30%. The medium and low-quality habitats area rose by 17.37 and 5.04% by 2020 (Figure 3B; Supplementary Table 5). Presently (2020), the areas with the high-quality habitat of P. amurense are mainly distributed in the central and southern parts of the Greater Khingan Mountains, the Lesser Khingan Mountains, and the forest areas of the Changbai Mountains. Moreover, the areas with medium-quality habitat are mainly concentrated in the forest landscape fringe. The areas with low-quality habitat are mainly cultivated land, grassland, construction land, rural residential land, waters, and unused land (Figure 3A).
3.3. Distribution changes under future climate conditions
Compared with the current climate conditions, the suitable area of P. amurense shows an increasing trend in the early stage of future climate change (2030s and 2050s). However, it would gradually decrease with the increase of years and carbon emissions in the 2070s and 2090s of high carbon emissions (SSP3-7.0 and SSP5-8.5). In all climate scenarios, the suitable area of P. amurense was the largest (68.25 × 104km2) in 2050s-SSP3-7.0 scenario, and in 2090s-SSP5-8.5 scenario, the total area of suitable area is the smallest (46.59 × 104km2). With carbon emission concentration increasing, the high suitability area of P. amurense would decrease gradually, while the low suitability area would gradually increase. Moreover, this trend gradually increases over the years (Supplementary Figure 1). Under 2090s-SSP5-8.5, the high suitability area would shrink to the minimum (12.21 × 104km2), which decreases by 59.22% compared with the current potential high suitability area. Moreover, under 2090s-SSP2-4.5, the low suitability area would expand to 19.49 × 104km2, with a change rate of 96.90% (Supplementary Table 6). The changing trend of the medium suitability area is the same as the total suitability area and the minimum area under 2090s-SSP5-8.5, about 17.16 × 104km2.
In the future, the suitable area would mainly expand to the northwest of the Lesser Khingan Mountains, the north of the Greater Khingan Mountains, and the Hulun Buir Plateau. The suitable area would decrease mainly in the north of Yanshan Mountains, the south of Changbai Mountains, and the North extension branch of Changbai Mountain. These trends expand gradually with the increase of years and carbon emission concentration (Figure 4; Supplementary Figure 2). Currently, the geographical coordinate of the P. amurense suitable area’s centroid is 127.04°E and 44.97°N. It is predicted that under the 2090s-SSP3-7.0, the centroid would be shifted as far as the hill region between the southern foothill of the Lesser Khingan Mountains and the Songnen Plain (Supplementary Figure 3). Among 16 future climate scenario combinations, the centroid migration direction of suitable area is generally the same, and all move to the northwest direction of high latitude (Figure 5; Supplementary Figure 3). Meanwhile, with the increase of year and carbon emission concentration, the elevation of the centroids in the suitable area also increase gradually (Figure 5).
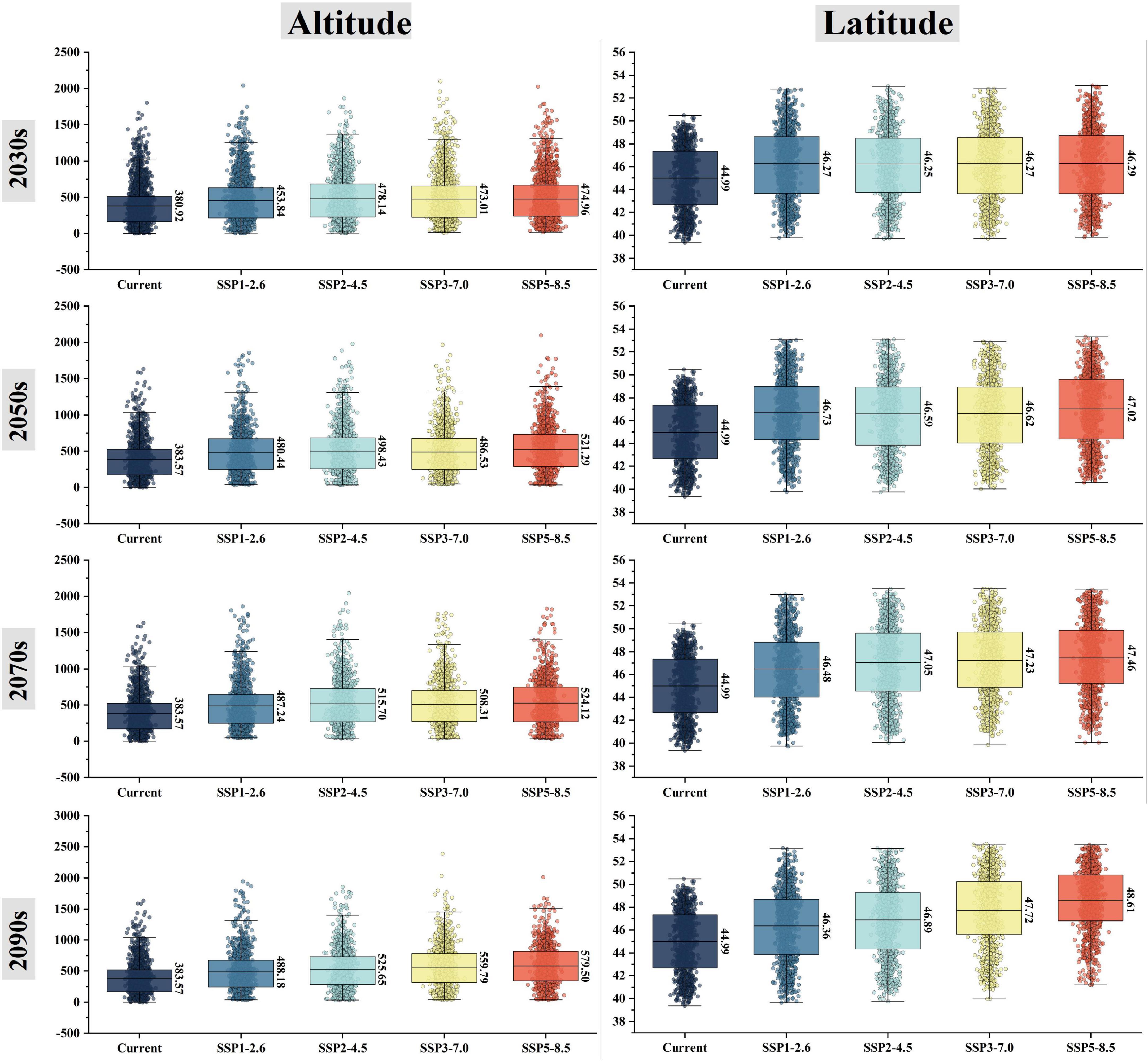
Figure 5. Spatial changes of altitude and latitude of the suitable areas for P. amurense under future climate combination.
3.4. Main environmental variables affecting the distribution of P. amurense and their correlation with chemical composition
Among the eight environmental variables, Bio04, Srad08, Bio10, Bio11, Srad06, and Bio18 are the most important environmental factors affecting the distribution of P. amurense (Supplementary Table 7). The suitable ranges of the main environmental factors in P. amurense are shown in Supplementary Table 8. Among the suitable range of Bio04 is 1168.72 to 1617.71, the range of Srad08 is 15641.80 kJ⋅m–2⋅day–1 to 18968.08 kJ⋅m–2⋅day–1, Bio10 ranges from 16.74 to 23.24°C, Bio11 ranges from −20.70 to −6.44°C, Srad06 ranges from 18276.53 kJ⋅m–2⋅day–1 to 22818.40 kJ⋅m–2⋅day–1, the lowest value of Bio18 in this range is 294.63 mm and the highest value is 563.24 mm. With the increase of year and carbon emissions, climate factors in the current potential distribution would change (Supplementary Figure 4; Supplementary Table 9). Under the 2090s-SSP5-8.5, Bio10, and Bio11 would increase significantly, and the average value would increase by 5.43 and 7.39°C, respectively. Bio18 would increase slightly (+71.30 mm). While Bio04 would decrease significantly, the average decrease from 1438.28 to 1350.45 (Figure 6).
The results showed that Berberine is positively correlated with Bio18 and Srad10 (p-value < 0.01). The relationship model between Berberine and environmental factors is established as C = 0.4055 × Bio18 + 0.6277 × Srad10; Palmatine content is positively correlated with Bio11 (p-value < 0.01), negatively correlated with Srad08 (p-value < 0.01), and negatively correlated with Srad10 (p-value < 0.05). Moreover, the relationship model between Palmatine and environmental factors is established as C = 1.9860 × Bio11-0.5745 × Srad08-1.3210 × Srad10 (Figures 7C, D; Supplementary Table 10). Currently, the spatial distribution of Berberine and Palmatine contents in the bark of P. amurense is shown in Figures 7A, B. The content of Berberine is higher in Hebei Province, northern Beijing, Liaoning Province, and southern and eastern Jilin Province. The content of Palmatine is higher in eastern and southern Heilongjiang Province, northeastern Jilin Province, and eastern and southern Liaoning Province.
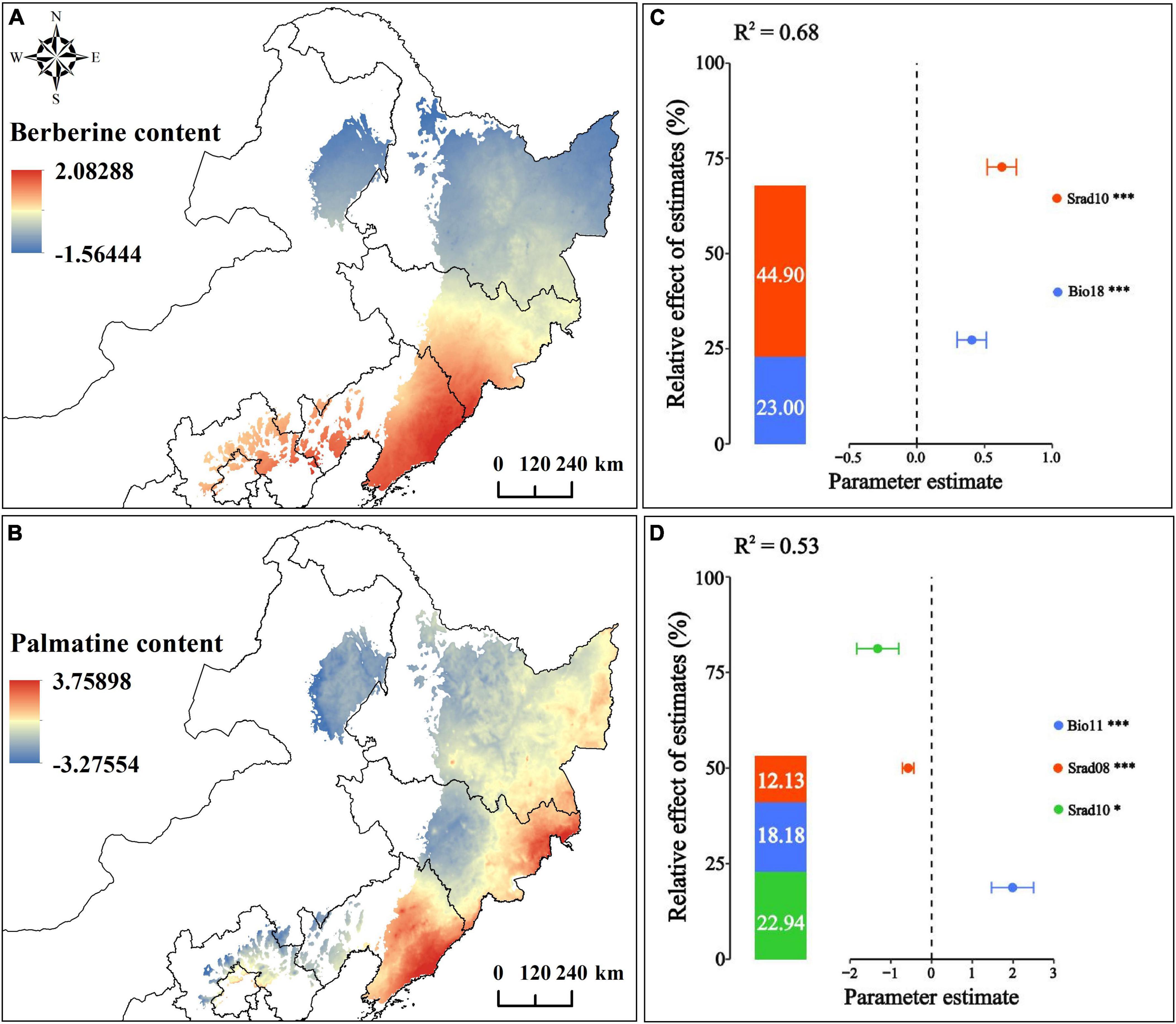
Figure 7. Correlation and spatial distribution of chemical composition and environmental factors of P. amurense; (A) Berberine spatial distribution; (B) Palmatine spatial distribution; (C) Correlation between Berberine content and environmental factors; (D) Correlation between Palmatine content and environmental factors. ***, **, and * represent the significance level of 1%, 5%, and 10% respectively.
3.5. Priority protection area and wild tending area for P. amurense
The priority protection area under the influence of future climate is about 7.66 × 104km2, accounting for 13.77% of the current potential suitable area. The high and moderate protection areas are about 1.91 × 104km2, and the high protection area is mainly distributed in the southeast of the Lesser Khingan Mountains, the middle of the Greater Khingan Mountains, and the Zhangguangcai Mountains (Figure 8A). The area of existing nature reserves in the P. amurense priority protection area is about 0.46 × 104km2, covering 6.01% of the priority protection area. Specifically, the nature reserves in the low protection area are the most, about 0.32 × 104km2. Moreover, the moderate and high protection areas are about 0.08 × 104km2 and 0.07 × 104km2 (Supplementary Table 11). Among the existing nature reserves, Red Pine Forest National Forest Park contains the largest area of moderate protection areas. Jinlongshan National Forest Park contributes the largest area of high protection areas. Furthermore, Dabane Wetland National Nature Reserve has the largest low and total protection areas (Figure 8C; Supplementary Table 12).
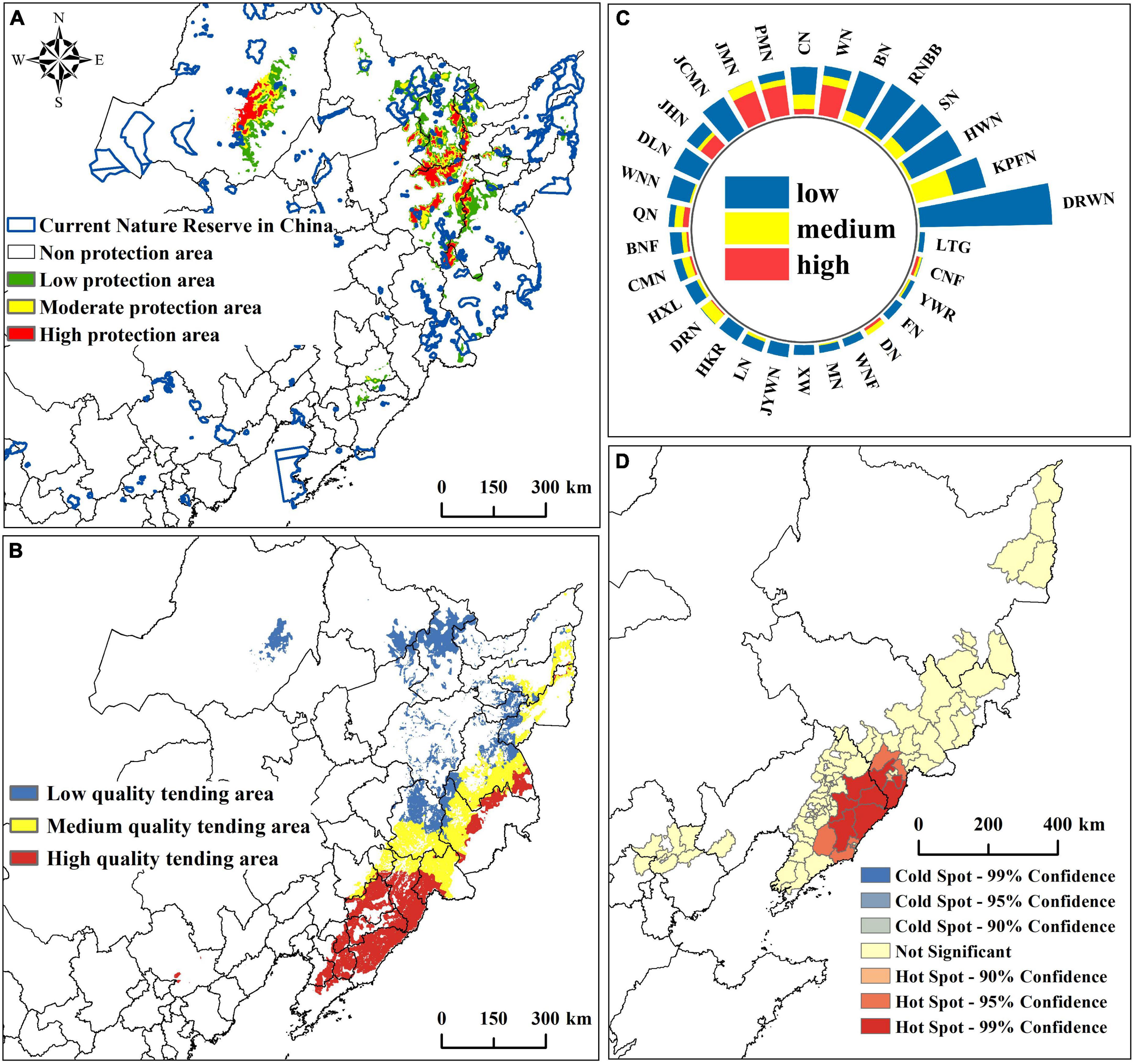
Figure 8. Prediction results of P. amurense reserve and wild tending. (A) Distribution of priority protection areas; (B) Distribution of different quality wild tending areas; (C) The area of each grade of priority protection areas within the existing nature reserve; (D) Hotspots analysis at the county level in high-quality wild tending areas.
The wild tending area of P. amurense is mainly distributed in the Changbai Mountain and Lesser Khingan Mountains, with a small amount of distribution extending from the southern of Lesser Khingan Mountain range to the southern of Changbai Mountain Range and the central and southern of Greater Khingan Mountains (Figure 8B). According to the results of the spatial distribution of chemical constituents, the high-quality wild tending area of P. amurense is mainly distributed in the south and east of the Changbai Mountain. We analyzed the hot spots of high-quality wild tending areas in the county-level administrative units in China. The results showed 13 counties suitable for planning high-quality wild tending areas, such as Fengcheng County and Tonghua County (Figure 8D; Supplementary Figure 5).
4. Discussion
4.1. Main environmental factors affecting the distribution of P. amurense and their correlation with the content of chemical components
The predictors related to temperature are the main factors affecting habitat suitability for most tree species (Liu et al., 2022). The results of this study showed that the most important environmental factor affecting the distribution of P. amurense is temperature seasonality (Bio04). Bio04 is an index to measure the temperature change in a year and can represent the stability of climate (O’donnell and Ignizio, 2012). Since the duration of sunshine and the solar incidence angle do not change significantly throughout the year, the seasonal variation of temperature in tropical and temperate regions close to the equator is narrower than that in subtropical or temperate regions, so the seasonal variation in high latitude regions is obvious (Tagliari et al., 2021). The potential habitat of P. amurense in China is mainly distributed in high latitudes, which is also the reason for the high Bio04 value (1168.72∼1617.71) in the current habitat of P. amurense.
Bio11, the mean temperature of the coldest quarter, is another important environmental factor affecting the distribution of P. amurense. In general, winter temperature affects trees’ growth rate in the following year. If the temperature is too low this season, the dehydration of protoplasm in the plant leaf cells or soil freezing will cause tree roots to freeze to death. Consequently, it will reduce photosynthesis in the next year and shorten the growth period of trees, which is not conducive to the growth of trees (Yuan and Li, 1999; Wang et al., 2003). Under climate warming, the inter-seasonal temperature difference in the suitable growth area of P. amurense gradually decreases, and the average temperature in the coldest month gradually increases, leading to the warming winter in the suitable area. The growing season of P. amurense in the next year may be extended with decreasing inter-seasonal temperature differences. Moreover, the prolonged growing season will accumulate more energy for the individual growth of the next year, which will accelerate its radial growth in the next growing season. Therefore, the rising temperature will positively affect survival and reproduction in the middle of this century. However, the seasonal temperature in the suitable habitat will decrease from 1438.28 to 1350.45, whereas the average temperature in the coldest month will increase by 7.39°C at the end of this century. It is worth noting that when the temperature in winter is too high, the respiration and metabolism of trees will be strengthened. The consumption of nutrients stored in the body for the next year’s growth will be accelerated, harming the next year’s growth and population fertility of trees (Edwards and Hanson, 1996; Cherubini et al., 1997). Therefore, the population of P. amurense will be further threatened at the end of this century due to the gradual increase of temperature increase in winter and the sharp reduction of the area of high suitability area.
Bio10 and Bio18 are also the main environmental factors affecting the distribution of P. amurense. Bio10 and Bio18 represent the mean temperature and precipitation in the warmest quarter of the year (O’donnell and Ignizio, 2012). The warmest quarter in Northeast China is from June to September, which is also the growing season of tree species in northern China (Li et al., 2017). The increasing lowest temperature in the growing season is conducive to extending the growth period of trees. During this period, appropriate precipitation will accelerate the accumulation of photosynthetic products and late growth (Liang et al., 2001). By the end of this century, the temperature and precipitation in the growing season in the suitable area will increase by 5.43 and 71.30 mm compared with the current. P. amurense needs sufficient water in the growing season, and the more adequate soil moisture, the better plant distribution (Huang et al., 2017). With the increase of temperature in the growing season, when the precipitation is appropriate, the negative impact of the decrease of forest soil water content caused by the increase of temperature on P. amurense and the positive demand of P. amurense for precipitation may reach a balance in some cases. However, when the balance is destroyed, and water stress is formed, it may limit the physiological and metabolic activities and inhibit its radial growth. Therefore, the “warm and dry” summer caused by climate warming will harm P. amurense over time.
Solar radiation profoundly impacts plant growth, development, and geographical distribution of species due to its different light intensity, light quality, and light period in different times and spaces (Li, 2017). Therefore, we included solar radiation factors in the species distribution model in this study. The results showed that these factors (Srad08, Srad10) are also important environmental variables affecting P. amurense. Since there is no matching future scenario with solar radiation data, in this study, solar radiation data exists in the species distribution model as a static variable. Some studies have pointed out that it is better or not worse to include static and dynamic variables in the species distribution model than to cover up or exclude static variables (Stanton et al., 2012). Therefore, the solar radiation data in our species distribution model improved performance for the present distribution. In conclusion, in the future climate change, the main environmental changes facing P. amurense population are warming in the cold quarter, the decrease of the temperature difference between seasons, and the warming and drying of the climate caused by the increase of temperature in the growing season.
Population chemical characterization manifests plant intra-specific diversity, which is significant for evaluating and utilizing medicinal plant germplasm (Zhang et al., 2016). The formation and accumulation of active components in P. amurense are determined by its genetic characteristics and are closely related to its surrounding ecological environment. Water and light factors have the most extensive impact on the active components of P. amurense (Zhang, 2015). The results of this study indicate that the most important environmental factor affecting the content of Berberine and Palmatine in P. amurense is solar radiation. The content of Berberine increases with the increase of solar radiation, while the content of Palmatine decreases with the increase of solar radiation. As Berberine is not the final product of secondary metabolism, it will be further converted into Palmatine and Jatrorrhizine (Gao et al., 2009; Huang et al., 2017), which makes the content of Berberine and Palmatine as well as the Jatrorrhizine present a complementary state, which may be the reason for this phenomenon. In addition to solar radiation, the environmental factor that affects the content of Berberine is precipitation, with a significant positive correlation between its content and precipitation. Another environmental factor that affects the content of Palmatine is temperature, which has a significantly positive correlation with temperature. Through constructing the spatial distribution layer of chemical components, we found that the areas with a high accumulation of active components of P. amurense are northeast Liaoning and southeast Jilin. This area is south of the current distribution, with sufficient light, warm temperature, abundant precipitation, and high annual active accumulated temperature. These results will provide a theoretical basis and important reference for the layout of production areas.
4.2. Spatial transformation characteristics of habitat of P. amurense under climate change scenario
In this study, Biomod2 is used to predict the P. amurense in China, and the results are consistent with most studies. It shows that the suitable habitat of P. amurense is mainly distributed in northeast China (Zhu, 1989; Chen, 2011). Our results showed that the Greater Khingan Mountains and the Yanshan Mountains in northern Hebei are only partially distributed, and Shandong, Shanxi, and Henan provinces are not distributed. It is worth noting that these results are not completely consistent with the results predicted by Huang et al. (2018). They were using the MaxEnt model. The Biomod2 model uses a variety of modeling techniques and integrates the results obtained by each method, which can reduce the deviation caused by the instability of a single model and can more accurately predict the range of suitable habitat of P. amurense (Araújo and New, 2007; Goldsmit et al., 2020).
In the future climate scenario combination, except for the 2090s-SSP5-8.5, the suitable area of P. amurense shows an increasing trend compared with the current. However, the increasing range of area decreased yearly with the increase of carbon emissions. Spatially, the change in the suitable area of P. amurense is consistent with most species’ migration direction (Li et al., 2019), and the general distribution area gradually moves to the northwest high latitude and high altitude area. In the future, the suitable area of P. amurense will expand to the northern forest of China, mainly located northwest of the Lesser Khingan Mountains and north of the Greater Khingan Mountains. The temperature difference between day and night in the expansion is large, and the extreme temperature can reach below −40°C (Ma, 2021), which exceeds the semi-lethal temperature of the isolated branches of P. amurense. Therefore, this region is not suitable for the growth of P. amurense. Projections of future climate scenarios indicate that northern forest temperatures will change more dramatically than the global average trend, with winter temperatures in northern forests projected to increase by 40% over the global average by 2,100 (Han and Wang, 2016). As the temperature rises, similar climatic conditions will be formed in this region as in the current P. amurense growing area. The current potential distribution of P. amurense is in the subhumid and humid regions. Under different combinations of future climate scenarios, the expansion is also in subhumid and humid regions. The soil moisture content in the expanded regions could still meet the growing demand for P. amurense. Therefore, the temperature change is the main reason affecting the dynamic change of distribution pattern of P. amurense.
The disappearance area of the suitable habitat is mainly distributed in the north of Yanshan Mountains and the south of Changbai Mountains. This region is mainly located in the subhumid region and the border region between subhumid and humid in northern China. Under future climate change, most of the humid areas in northern China will be transformed into subhumid areas, the overall humid areas will shrink, and the subhumid and semi-arid areas will expand to the east (Ma et al., 2019). The warm and dry climate will reduce the moisture of surface soil and increase evaporation, resulting in a decrease of climate moisture index and not conducive to the absorption of soil fertility by plant roots (Michaelian et al., 2011; Peng et al., 2011). The area in the subhumid and the suitable area’s boundary are no longer suitable for P. amurense due to this climate. Therefore, warming and drying caused by global warming is the main reason for reducing the suitable area of P. amurense.
In addition to temperature limiting plant growth, poor soil and nutrition also limit plant growth (Lafleur et al., 2010). In the global warming, the permafrost in the Greater Khingan Mountains and Lesser Khingan Mountains in Northeast China has experienced significant warming and degradation (Jin et al., 2009). In the 21st century, the active layer thickness of permafrost in the northern forest area may increase by 5∼30 cm every 10 years (Hayes et al., 2014). This phenomenon will accelerate the release of permafrost nutrients and promote the decomposition of soil organic matter, thus improving the barren soil and nutrient deficiency (Han and Wang, 2016). Therefore, we speculated that the increase in soil nutrients provides the basis for migration. It is worth noting that the temperature and soil temperature in the northern forest area shows contradictory responses to climate change based on the complexity of interactions between soil, landscape factors, and climate change. The sensitivity of this region to temperature is far greater than that of other biotas. With climate warming, the structure and function of the northern forest ecosystem tend to deteriorate, and its composition tends to be simple (Hennon et al., 2012). Therefore, in the future, we should strengthen the study of P. amurense population, community, landscape, and other aspects with multi-method and multi-scale coupling. At the same time, long-term field positioning observation and indoor simulation experiments are combined to increase the accuracy of model inference.
It is worth noting that the complete ecological niche models should be more reliable, because they are calibrated with a much higher number of presences and capture a much wider range of occupied environmental conditions (Pearson et al., 2004). However, without enough bias-free regional data, we cannot objectively identify the actual improvement of regional models after incorporating information from the global niche (El-Gabbas and Dormann, 2018). Through the network database and literature review, since the middle of the 20th century, the occurrence data of P. amurense outside the study area (China) is very little. And in the record of the literature, there are still areas [Korea (Democratic People’s Republic of)] did not search the occurrence data. Meanwhile, SDM that rely on regional-scale environmental variables will play a key role in forecasting species occurrence in the face of climate change (Requena-Mullor et al., 2019). Therefore, this article has conducted a regional species distribution modeling based on China as the research scope, which can still provide a reasonable explanation for the distribution pattern of suitable habitats for P. amurense in China in the context of climate change.
4.3. Impact of habitat and landscape pattern changes on the distribution of P. amurense
There are two main forms of habitat fragmentation: (1) Morphological fragmentation is caused by the reduction of habitat area and the enhancement of edge effect caused by human activities. Habitat fragmentation leads to habitat fragmentation of species. (2) Ecological function fragmentation is caused by the reduction of species’ adaptability to habitat due to climate change (Ran et al., 2019). From 1980 to 2020, the forest landscape area in the suitable area of P. amurense was gradually transferred to cultivated land, grassland, construction land, and unused land. Cultivated land was the main transfer direction, and the area increased yearly. In addition, with the decrease in forest area, the habitat quality of P. amurense decreased year by year, and fragmentation gradually intensified. It can be seen that the main reason for habitat quality and pattern changes in the past 40 years is human activities.
Interannual changes in climate are normal. Even in the absence of long-term climate change, most species tolerate such short-term climate changes by changing the functional shape of plants through climate-related genetic variation in natural populations (Fridley and Grime, 2010; Wang et al., 2020). For plants, better interspecific gene exchange can improve adaptability, increasing species and population size (Reed and Frankham, 2003; Wan et al., 2014). Habitat fragmentation will affect genetic drift, intensify inbreeding and reduce the gene flow of the population, thus posing a threat to the population, as well as affecting the number of species in the community and the ability to cope with environmental disturbances such as outbreaks of pests and diseases and extreme weather events (Jump and Peñuelas, 2005). P. amurense population has low genetic diversity and no obvious genetic pattern (Wang et al., 2014). As a traditional Chinese medicine, the bark of P. amurense has been cut down and peeled for a long time, resulting in the gradual fragmentation of its habitat, the gradual reduction of the population size, and the formation of small, isolated populations. The smaller population and lower population density will not only reduce the genetic variability of P. amurense, which may lead to more and more obvious genetic drift and inbreeding decline, but also bring inconvenience to insect pollination, resulting in small gene flow among P. amurense populations (Yan et al., 2006). The size of plant gene flow largely depends on how the plant reproduces and how the propagules move. For seed-mediated gene flow, pollen gene flow is dominant, and the dispersal and distribution mechanism of plant pollen is an important factor in determining the genetic structure between populations, among which wind-pollinated plants have higher gene flow than animal-pollinated and self-pollinated plants (Wen et al., 2010). As an insect flower, the gene flow of P. amurense is small. In addition to genetic drift and gene flow, the breeding system also greatly impacts genetic diversity. After maturity, P. amurense seeds mostly fall around the mother. However, allelopathic substances that exist in the peel and fallen leaves of mother plants can effectively inhibit seed germination (Zhang et al., 2011; Dai et al., 2012). Moreover, the plant community has high shade, many fallen leaves on the ground, and a deep dormancy mechanism. These factors restrict germination and the growth of seedlings. At the same time, the genetic information carried by seeds has a slow transmission speed and a long transmission period, which reduces the gene exchange frequency and decreases genetic diversity in the wild population (Wang et al., 2014). Therefore, the reduction of genetic diversity in the population of P. amurense may reduce the population’s tolerance and recovery ability under the interference of future climate change.
However, for both individuals and species, adaptation to environmental changes through phenotypic plasticity is limited. Moreover, changes in the distribution of species are inevitable when climate change exceeds what plants can tolerate (Bradshaw, 1965; Bradshaw and McNeilly, 1991). As the climate warms, individuals in the population may move to higher altitudes and latitudes to respond to the movement of its best climate (Jump and Peñuelas, 2005). Migration usually occurs across the species range in the form of propagules and pollen dispersal rather than simply due to the expansion and contraction of range edges (Davis and Shaw, 2001). The long-distance transmission of the seeds of P. amurense mainly depends on fruit-eating animals, but habitat fragmentation will reduce the diversity of fruit-eating animals. In the past 40 years, the forest land in the suitable habitat has been gradually fragmented. If not controlled, the suitable habitat will be seriously fragmented in the future, which will not be conducive to the spread of seeds and pose a threat to the survival of the population. Therefore, if the reduction degree and fragmentation degree of the habitat area of P. amurense is further intensified in the future, a few individuals can survive in the landscape for a long time. However, it will lose the ability to maintain a stable population, which may form the “living dead population” (Chen et al., 2022).
The main causes of species endangerment are habitat destruction, environmental pollution, habitat degradation, and overuse of biological resources (Lande, 1988). With the increase in economy, urbanization, and agricultural development, human development and utilization of the land gradually occupy a leading position. If we continue to develop the land according to the trend of land use change in the past 40 years, the habitat of P. amurense will be seriously destroyed at the end of this century. In addition, without human intervention, it is very difficult to form seedlings and increase the population of individuals since the natural habitat is not conducive to the self-renewal of the P. amurense population. Therefore, it is suggested to focus on forest vegetation restoration projects and identify priority protection areas and high-quality wild tending areas in P. amurense suitable areas. These actions can better protect the wild resources and reasonable development and utilization.
4.4. Priority conservation area and high-quality wild tending area of P. amurense
As an important tree species for traditional Chinese medicine, P. amurense is threatened due to its extensive and illegal harvest (Yu et al., 2013; Wan et al., 2014). In the past 20 years, the destruction of wild resources of P. amurense has been restrained to a certain extent due to the change in the national forest management mode and the strengthening of the protection of endangered species (Zhang et al., 2016). However, our results showed that upper climate change would seriously threaten the distribution of P. amurense in the absence of anthropogenic disturbance. Moreover, its population renewal ability is weak, which is not conducive to the improvement under threat (Zhang et al., 2016). These troubles may lead to the shortage or loss of local medicinal resources of P. amurense. Wild cultivation is an effective way to solve the gap between the supply and demand of medicinal plants. However, a major premise of medicinal plant cultivation is to ensure high-quality medicinal materials (Shen et al., 2021). Given this, it is important to integrate climate change and land use landscape patterns for planning suitable protection areas and wild tending areas of P. amurense.
Based on climate change and landscape patterns, this study determined the priority protection area of P. amurense in China. Although there are some national and provincial nature reserves within the range of P. amurense suitable area, our results showed that the existing reserves only contain 6.01% of the priority protection area under future climate change. There is still a large area that has not yet been protected. Among the existing nature reserves, Jinlong Mountain National Forest Park has the largest area of high protection areas. The climate conditions are highly suitable and will not change significantly in the next century, and the species will be less affected by climate change in the reserve. Therefore, in this century, Jinlong Mountain National Forest Park may contribute to the protection of P. amurense. We believe that the protection of P. amurense should be carried out in the southern part of the Lesser Khingan Mountains and the northern part of the Changbai Mountains. There is no potential climate pressure under future climate, and the forest is relatively concentrated, which is conducive to gene exchange between the populations.
Phellodendron amurense can be harvested after planting for 10–15 years (Yan et al., 2013). According to China’s national policy, it is forbidden to plant perennial woody medicinal materials and trees on cultivated land. Therefore, to promote the protection, recovery, and sustainable utilization of the population, it is necessary to carry out the related work of wild tending of P. amurense. The wild tending of traditional Chinese medicine achieves the coordinated development between producing medicinal materials and protecting the ecological environment. In this way, the sustainable renewal of the tending population of traditional Chinese medicine can better protect the rare and endangered medicinal materials and their biodiversity (Chen et al., 2004). In addition, the wild tending area is the original environment, with less human interference and far away from pollution sources, providing high-quality wild medicinal materials for medical treatment. At the same time, wild tending makes full use of the natural growth characteristics, reducing the cost of manual management and obtaining high returns with low investment. With wild tending being accepted and related research being carried out one after another, a new mode of ecological industry of traditional Chinese medicine will be opened.
This study plans a high-quality wild tending area based on the current forest area in high suitability of P. amurense and the distribution information of effective chemical components. In this way, we can effectively avoid the waste of investment caused by blind planting. According to the planning results, we put forward the following suggestions. (1) High-quality wild tending areas are mainly distributed in the south and east Changbai Mountain. It is important to note that most of this area is at high risk for habitat loss. Therefore, it is suggested to collect wild germplasm and evaluate its diversity. (2) The cultivation of P. amurense in the planned high-quality wild tending area should be combined with local policies, especially with the policies designated at the county level. Besides, integrate the ecological, economic, and social benefits of the mountain areas, and strengthen the establishment of the wild cultivation medicine base. These protective actions can regulate people’s habit of plundering and digging wild medicinal materials and strengthen the protection of biodiversity and the ecological environment. Furthermore, the contradiction between resources and supply-demand, population renewal, ecological protection, and biodiversity of P. amurense will be effectively solved. At the same time, it is also necessary to strengthen the research on the base management mechanism and other aspects to ensure smooth operation and achieve the purpose of nurturing. (3) Mastering the basic characteristics of the growth and development of wild traditional Chinese medicine is the basis for determining their wild tending methods, reproduction, and growth. Moreover, the development and population renewal of the tending medicinal materials are affected by various environmental factors (Chen et al., 2004). Therefore, it is necessary to strengthen the research on developmental biology, population ecology, and wild tending methods of P. amurense. In the wild tending plan of P. amurense, the environmental factors that affect the content of its chemical components and their future changes should be emphatically considered so that the planned wild tending area of P. amurense has timeliness. (4) Strengthen the research on the possibility of other organs of P. amurense to be used as medicine, and change the single way of using the medicine. It is the fundamental way that solves the contradiction between protecting and utilizing P. amurense resources.
5. Conclusion
In the analysis of the change of the distribution range of P. amurense under the future climate in China, although climate warming will gradually reduce the area of the suitable habitat and move to the northwest, there is still a climate-stable area suitable for the growth of P. amurense. In the past 40 years, the habitat quality of the suitable habitat of P. amurense has decreased year by year, and the natural habitat has shown a trend of gradual fragmentation. Based on the above reasons, we constructed a protection framework for the rare and endangered medicinal material–P. amurense. (1): The forest areas in the middle of the Greater Khingan Mountains and the Lesser Khingan Mountains are climate-stable areas. Therefore, this area is regarded as the priority protection area of P. amurense to prevent the resources from being seriously damaged, even facing the crisis of extinction by climate change and human activities. (2): Based on the correlation between the content of chemical components and environmental factors, the wild tending areas of P. amurense were planned. Wild tending not only restores and increases the population, improves the habitat environment, and enriches the genetic diversity of the population but also ensures the sustainable utilization of wild medicinal plant resources, maintains the balance between the utilization of wild medicinal plants and the protection of the ecological environment, and realizes the harmonious coexistence of human and nature. This work is a targeted proposal for the protection of P. amurense and provides a reference method for the protection and utilization of other rare and endangered wild medicinal species. In the future, we will pay more attention to the long-term monitoring of environmental factors in the high suitability of the P. amurense population. Consequently, obtaining high-quality modeling parameters can provide a more scientific and accurate theoretical basis for protecting P. amurense. We conducted in-depth biological research on P. amurense and formulated reasonable and scientific wild tending methods in line with the policy prohibiting “non-grain cultivation” of cultivated land in China. Our results can alleviate self-renewal obstacles of the P. amurense population, effectively improve its ecological and economic value, and meet the rigid growth demand for high-quality traditional Chinese medicinal materials in the era of the global health economy.
Data availability statement
The original contributions presented in this study are included in the article/Supplementary material, further inquiries can be directed to the corresponding author.
Author contributions
BZ: writing–original draft preparation, writing–review and editing, data curation, and software. HZ and XXZ: conceptualization, writing–review and editing, and methodology. BC: writing–review and editing. XYZ: investigation and validation. XK: data curation. CW: supervision and conceptualization. All authors contributed to the article and approved the submitted version.
Funding
This work was supported by the Fourth National Survey of Traditional Chinese Medicine Resources Heilongjiang Special Project (grant number: 2018Hljzypc-12).
Conflict of interest
The authors declare that the research was conducted in the absence of any commercial or financial relationships that could be construed as a potential conflict of interest.
Publisher’s note
All claims expressed in this article are solely those of the authors and do not necessarily represent those of their affiliated organizations, or those of the publisher, the editors and the reviewers. Any product that may be evaluated in this article, or claim that may be made by its manufacturer, is not guaranteed or endorsed by the publisher.
Supplementary material
The Supplementary Material for this article can be found online at: https://www.frontiersin.org/articles/10.3389/fevo.2023.1186627/full#supplementary-material
Footnotes
- ^ https://www.gbif.org
- ^ http://www.cvh.ac.cn
- ^ http://www.nsii.org.cn
- ^ www.worldclim.org
- ^ https://www.resdc.cn/
- ^ https://www.helsinki.fi/
References
Aiello-Lammens, M. E., Boria, R. A., Radosavljevic, A., Vilela, B., and Anderson, R. P. (2015). spThin: an R package for spatial thinning of species occurrence records for use in ecological niche models. Ecography 38, 541–545. doi: 10.1111/ecog.01132
Akbari, A., Pittman, J., and Feick, R. (2021). Mapping the relative habitat quality values for the burrowing owls (Athene cunicularia) of the Canadian prairies using an innovative parameterization approach in the InVEST HQ module. Environ. Manage. 68, 310–328. doi: 10.1007/s00267-021-01502-w
Araújo, M. B., and New, M. (2007). Ensemble forecasting of species distributions. Trends Ecol. Evol. 22, 42–47. doi: 10.1016/j.tree.2006.09.010
Araújo, M. B., Pearson, R. G., and Rahbek, C. (2005). Equilibrium of species’ distributions with climate. Ecography 28, 693–695.
Bradshaw, A. D. (1965). Evolutionary significance of phenotypic plasticity in plants. Adv. Genet. 13, 115–155. doi: 10.1016/S0065-2660(08)60048-6
Bradshaw, A. D., and McNeilly, T. (1991). Evolutionary response to global climatic change. Ann. Bot. 67, 5–14.
Brown, J. L. (2014). SDM toolbox: a python-based GIS toolkit for landscape genetic, biogeographic and species distribution model analyses. Methods Ecol. Evol. 5, 694–700. doi: 10.1111/2041-210X.12200
Bucklin, D. N., Basille, M., Benscoter, A. M., Brandt, L. A., Mazzotti, F. J., Romanach, S. S., et al. (2015). Comparing species distribution models constructed with different subsets of environmental predictors. Divers. Distrib. 21, 23–35. doi: 10.1111/ddi.12247
Chen, B. R., Zou, H., Zhang, B. Y., Zhang, X. Y., Jin, X. X., Wang, C., et al. (2022). Distribution pattern and change prediction of Saposhnikovia divaricata suitable area in China under climate change. Ecol. Indic. 143:109311. doi: 10.1016/j.ecolind.2022.109311
Chen, S. L. (2011). Ecological suitability zoning of Chinese medicinal material producing areas. Beijing: Science Press.
Chen, S. L., Wei, J. H., Huang, L. F., Guo, B. L., and Xiao, P. G. (2004). Probing into the theory and practice of wild medicinal materials tending. China J. Chin. Mater. Med. 29, 1123–1126.
Chen, S. L., Yu, H., Luo, H. M., Wu, Q., Li, C. F., and Steinmetz, A. (2016). Conservation and sustainable use of medicinal plants: problems, progress, and prospects. Chin. Med. 11, 1–10. doi: 10.1186/s13020-016-0108-7
Cherubini, P., Schweingruber, F. H., and Forster, T. (1997). Morphology and ecological significance of intra-annual radial cracks in living conifers. Trees 11, 216–222. doi: 10.1007/s004680050078
Cobos, M. E., Peterson, A. T., Barve, N., and Osorio-Olvera, L. (2019). kuenm: an R package for detailed development of ecological niche models using Maxent. PeerJ 7, e6281. doi: 10.7717/peerj.6281
Dai, L. C., Si, J. Y., Liu, Y. L., and Zhang, Z. (2012). Chemical Constituents from Leaves of Phellodendron amurense Rupr. Natl. Prod. Res. Dev. 24, 900–902. doi: 10.16333/j.1001-6880.2012.07.017
Davis, M. B., and Shaw, R. G. (2001). Range shifts and adaptive responses to Quaternary climate change. Science 292, 673–679. doi: 10.1126/science.292.5517.673
de Andrade, A. F. A., Velazco, S. J. E., and Júnior, P. D. M. (2020). ENMTML: An R package for a straightforward construction of complex ecological niche models. Environ. Model. Softw. 125:104615. doi: 10.1016/j.envsoft.2019.104615
Eberly, L. E. (2007). Multiple linear regression. Topics Biostat. 404, 165–187. doi: 10.1007/978-1-59745-530-5_9
Edwards, N. T., and Hanson, P. J. (1996). Stem respiration in a closed-canopy upland oak forest. Tree Physiol. 16, 433–439. doi: 10.1093/treephys/16.4.433
El-Gabbas, A., and Dormann, C. F. (2018). Wrong, but useful: regional species distribution models may not be improved by range-wide data under biased sampling. Ecol. Evol. 8, 2196–2206. doi: 10.1002/ece3.3834
Elias, M. A., Borges, F. J., Bergamini, L. L., Franceschinelli, E. V., and Sujii, E. R. (2017). Climate change threatens pollination services in tomato crops in Brazil. Agric. Ecosyst. Environ. 239, 257–264. doi: 10.1016/j.agee.2017.01.026
Freitas, G. H., Costa, L. M., Silva, P. H., Chaves, A. V., Ribeiro, L. C., and Rodrigues, M. (2019). Spatial ecology and conservation of the microendemic ovenbird Cipo Cinclodes (Cinclodes espinhacensis) from the Brazilian highlands. J. Field Ornithol. 90, 128–142. doi: 10.1111/jofo.12296
Fridley, J. D., and Grime, J. P. (2010). Community and ecosystem effects of intraspecific genetic diversity in grassland microcosms of varying species diversity. Ecology 91, 2272–2283. doi: 10.1890/09-1240.1
Gao, S., He, P., Zhang, C. P., Wang, R. B., and Zhang, Y. F. (2009). Effects of Environmental Factors on Major Medicinal Components of Coptis omeiensis C. Y. Heng. J. Southwest Univ. 31, 21–25. doi: 10.13718/j.cnki.xdzk.2009.07.029
Goldsmit, J., McKindsey, C. W., Schlegel, R. W., Stewart, D. B., Archambault, P., and Howland, K. L. (2020). What and where? Predicting invasion hotspots in the Arctic marine realm. Glob. Change Biol. 26, 4752–4771. doi: 10.1111/gcb.15159
Gomes, L. C., Bianchi, F., Cardoso, I. M., Fernandes, R. B. A., Fernandes Filho, E. I., and Schulte, R. P. O. (2020). Agroforestry systems can mitigate the impacts of climate change on coffee production: a spatially explicit assessment in Brazil. Agric. Ecosyst. Environ. 294:106858. doi: 10.1016/j.agee.2020.106858
Groner, V. P., Williams, J. J., and Pearson, R. G. (2023). Limited evidence for quantitative contribution of rare and endangered species to agricultural production. Agric. Ecosyst. Environ. 345:108326. doi: 10.1016/j.agee.2022.108326
Han, S. J., and Wang, Q. G. (2016). Response of boreal forest ecosystem to global climate change: a review. J. Beijing For. Univ. 38, 1–20. doi: 10.13332/j.1000-1522.20160046
Hayes, D. J., Kicklighter, D. W., McGuire, A. D., Chen, M., Zhuang, Q., Yuan, F., et al. (2014). The impacts of recent permafrost thaw on land–atmosphere greenhouse gas exchange. Environ. Res. Lett. 9:45005. doi: 10.1088/1748-9326/9/4/045005
Hennon, P. E., D’Amore, D. V., Schaberg, P. G., Wittwer, D. T., and Shanley, C. S. (2012). Shifting climate, altered niche, and a dynamic conservation strategy for yellow-cedar in the North Pacific coastal rainforest. BioScience 62, 147–158. doi: 10.1525/bio.2012.62.2.8
Huang, Z. H., Zhou, X., Zhang, X. R., Pu, Z., and Xing, S. H. (2017). Relationship between the distribution of Phellodendron amurense and environmental factors in the Beijing area. Plant Sci. J. 35, 56–63. doi: 10.11913/PSJ.2095-0837.2017.10056
Huang, Z. H., Zhou, X., Zhang, X. R., Pu, Z., and Xing, S. H. (2018). Suitability assessments of potential distribution areas for Amur corktree in mainland China. Acta Ecol. Sin. 38, 7459–7476. doi: 10.5846/stxb201711071997
Huntley, B. (1991). How plants respond to climate change: migration rates, individualism and the consequences for plant communities. Ann. Bot. 47, 15–22.
Jiang, T., Lv, Y. R., Huang, J. L., Wang, Y. J., Su, B. D., and Tao, H. (2020). New Scenarios of CMIP6 Model (SSP-RCP) and Its Application in the Huaihe River Basin. Adv. Meteorol. Sci. Technol. 10, 102–109. doi: 10.3969/j.issn.2095-1973.2020.05.016
Jiménez-Valverde, A., and Lobo, J. M. (2007). Threshold criteria for conversion of probability of species presence to either–or presence–absence. Acta Oecol. 31, 361–369. doi: 10.1016/j.actao.2007.02.001
Jin, H. J., Wang, S. L., Lv, L. Z., and Yu, S. P. (2009). Features of Permafrost Degradation in Hinggan Mountains, Northeastern China. Sci. Geogr. Sin. 29, 223–228.
Jump, A. S., and Peñuelas, J. (2005). Running to stand still: adaptation and the response of plants to rapid climate change. Ecol. Lett. 8, 1010–1020. doi: 10.1111/j.1461-0248.2005.00796.x
Lafleur, B., Pare, D., Munson, A. D., and Bergeron, Y. (2010). Response of northeastern North American forests to climate change: Will soil conditions constrain tree species migration? Environ. Rev. 18, 279–289. doi: 10.1139/A10-013
Lande, R. (1988). Genetics and demography in biological conservation. Science 241, 1455–1460. doi: 10.1126/science.3420403
Li, N. Q., and Xu, G. Y. (2020). Grid analysis of land use based on natural breaks (jenks) classification. Bull. Surv. Mapp. 106, 106–110. doi: 10.13474/j.cnki.11-2246.2020.0121
Li, N., Mu, C. C., Wang, B., Zhang, Y., and Ma, L. (2017). Carbon source or sink of natural forested wetland ecosystem in Xiaoxing’anling region of China. Acta Ecol. Sin. 37, 2880–2893. doi: 10.5846/stxb201601250174
Li, W. Q., Xu, Z. F., Shi, M. M., and Chen, J. H. (2019). Prediction of potential geographical distribution patterns of Salix tetrasperma Roxb. in Asia under different climate scenarios. Acta Ecol. Sin. 39, 3224–3234. doi: 10.5846/stxb201803020413
Liang, E. Y., Shao, X. M., Hu, Y. X., and Lin, J. X. (2001). Dendroclimatic evaluation of climate-growth relationships of Meyer spruce (Picea meyeri) on a sandy substrate in semi-arid grassland, north China. Trees 15, 230–235. doi: 10.1007/s004680100097
Liu, D., Lei, X. D., Gao, W. Q., Guo, H., Xie, Y. S., Fu, L. Y., et al. (2022). Mapping the potential distribution suitability of 16 tree species under climate change in northeastern China using Maxent modelling. J. For. Res. 33, 1739–1750. doi: 10.1007/s11676-022-01459-4
Liu, M. Z., Zhang, H. J., Wang, Y. F., and Pei, H. W. (2021). Characteristics of habitat quality in the agro-pastoral Ecotone of Northern China based on land uses. Res. Soil Water Conserv. 28, 156–162. doi: 10.13869/j.cnki.rswc.2021.03.018
Ma, D. Y., Yin, Y. H., Wu, S. H., and Zheng, D. (2019). Sensitivity of arid/humid patterns in China to future climate change under high emission scenario. Acta Geogr. Sin. 74, 857–874. doi: 10.11821/dlxb201905002
Ma, H. M. (2021). Distribution, protection and utilization of wild plant resources in the primitive forest area in the north of the Great Khingan Mountains. Spec. Econ. Anim. Plants 83–84.
Ma, L., Li, J., and Hu, Y. (2015). Determination of berberine in Phellodendron amurense from different sites of Changbai Mountain. J. For. Res. 26, 201–207. doi: 10.3390/ijerph192417082
Ma, Z. Y., Ren, J. X., Chen, H. T., Jiang, H., Gao, Q. X., Liu, S. Y., et al. (2022). Analysis and recommendations of IPCC working group I assessment report. Res. Environ. Sci. 35, 2550–2558. doi: 10.13198/j.issn.1001-6929.2022.08.08
Michaelian, M., Hogg, E. H., Hall, R. J., and Arsenault, E. (2011). Massive mortality of aspen following severe drought along the southern edge of the Canadian boreal forest. Glob. Change Biol. 17, 2084–2094. doi: 10.1111/j.1365-2486.2010.02357.x
Moilanen, A. (2007). Landscape zonation, benefit functions and target-based planning: unifying reserve selection strategies. Biol. Conserv. 134, 571–579. doi: 10.1016/j.biocon.2006.09.008
Moilanen, A., Franco, A. M., Early, R. I., Fox, R., Wintle, B., and Thomas, C. D. (2005). Prioritizing multiple-use landscapes for conservation: methods for large multi-species planning problems. Proc. R. Soc. B Biol. Sci. 272, 1885–1891. doi: 10.1098/rspb.2005.3164
National Pharmacopoeia Commission (2020). Pharmacopoeia of the People’s Republic of China 2020. Beijing: China Medical Science and Technology Press.
O’donnell, M. S., and Ignizio, D. A. (2012). Bioclimatic predictors for supporting ecological applications in the conterminous United States. US Geol. Survey Data Ser. 691, 4–9.
Pant, G., Maraseni, T., Apan, A., and Allen, B. L. (2021). Predicted declines in suitable habitat for greater one-horned rhinoceros (Rhinoceros unicornis) under future climate and land use change scenarios. Ecol. Evol. 11, 18288–18304. doi: 10.1002/ece3.8421
Pearson, R. G., Dawson, T. P., and Liu, C. (2004). Modelling species distributions in Britain: a hierarchical integration of climate and land-cover data. Ecography 27, 285–298. doi: 10.1111/j.0906-7590.2004.03740.x
Peng, C. H., Ma, Z. H., Lei, X. D., Zhu, Q. A., Chen, H., Wang, W. F., et al. (2011). A drought-induced pervasive increase in tree mortality across Canada’s boreal forests. Nat. Clim. Change 1, 467–471. doi: 10.1038/NCLIMATE1293
Ran, Q., Wei, H. Y., Zhao, Z. F., Zhang, Q. Z., Liu, J., and Gu, W. (2019). Impact of climate change on the potential distribution and habitat fragmentation of the relict plant Cathaya argyrophylla Chun et Kuang. Acta Ecol. Sin. 39, 2481–2493. doi: 10.5846/stxb201802010282
Reed, D. H., and Frankham, R. (2003). Correlation between fitness and genetic diversity. Conserv. Biol. 17, 230–237. doi: 10.1046/j.1523-1739.2003.01236.x
Ren, J. Y. (2022). Extreme Climate Change and its impact on vegetation in The Mongolian Plateau. Master’s thesis. Inner Mongolia: Inner Mongolia Normal University.
Requena-Mullor, J. M., Maguire, K. C., Shinneman, D. J., and Caughlin, T. T. (2019). Integrating anthropogenic factors into regional-scale species distribution models—A novel application in the imperiled sagebrush biome. Glob. Change Biol. 25, 3844–3858. doi: 10.1111/gcb.14728
Shen, T., Yu, H., and Wang, Y. Z. (2021). Assessing the impacts of climate change and habitat suitability on the distribution and quality of medicinal plant using multiple information integration: take Gentiana rigescens as an example. Ecol. Indic. 123:107376. doi: 10.1016/j.ecolind.2021.107376
Sommer, J. H., Kreft, H., Kier, G., Jetz, W., Mutke, J., and Barthlott, W. (2010). Projected impacts of climate change on regional capacities for global plant species richness. Proc. R. Soc. B Biol. Sci. 277, 2271–2280. doi: 10.1098/rspb.2010.0120
Stanton, J. C., Pearson, R. G., Horning, N., Ersts, P., and Reşit Akçakaya, H. (2012). Combining static and dynamic variables in species distribution models under climate change. Methods Ecol. Evol. 3, 349–357. doi: 10.1111/j.2041-210X.2011.00157.x
Tagliari, M. M., Danthu, P., Leong Pock Tsy, J.-M., Cornu, C., Lenoir, J., Carvalho-Rocha, V., et al. (2021). Not all species will migrate poleward as the climate warms: The case of the seven baobab species in Madagascar. Glob. Change Biol. 27, 6071–6085. doi: 10.1111/gcb.15859
Tang, W., Guo, Y., Jin, T., and Wang, Y. (2014). The countermeasure and technical measures of population protection and development of Phellodendron amurense Rupr. J. Jiln for. Sci. Technol. 43, 26–27. doi: 10.16115/j.cnki.issn.1005-7129.2014.06.032
Wan, J. Z., Wang, C. J., Yu, J. H., Nie, S. M., Han, S. J., Zu, Y. G., et al. (2014). Model-based conservation planning of the genetic diversity of Phellodendron amurense Rupr due to climate change. Ecol. Evol. 4, 2884–2900. doi: 10.1002/ece3.1133
Wang, L. J., Bao, L., Wang, H. F., and Ge, J. P. (2014). Study on genetic diversity of Phellodendron amurense. Chin. Sci. Pap. 9, 1397–1401.
Wang, S. T., Xu, Y. Z., Yang, T., Wei, X. Z., and Jiang, M. X. (2020). Impacts of microhabitats on leaf functional traits of the wild population of Sinojackia huangmeiensis. Biodivers. Sci. 28, 277–288. doi: 10.17520/biods.2019118
Wang, T., Yu, D., Li, J. F., and Ma, K. P. (2003). Advances in Research on the Relationship Between Climatic Change and Tree-Ring Width. Acta Phytoecol. Sin. 27, 23–33. doi: 10.17521/cjpe.2003.0004
Wang, X. P., Wang, Z. H., and Fang, J. Y. (2004). Mountain ranges and peaks in China. Biodivers. Sci. 12, 206–212.
Warren, D. L., Glor, R. E., and Turelli, M. (2010). ENMTools: a toolbox for comparative studies of environmental niche models. Ecography 33, 607–611. doi: 10.1111/j.1600-0587.2009.06142.x
Wei, Y., Li, X. Y., Chen, W. J., and Bao, H. Y. (2019). Research Progress on Resources and Development and Utilization of Phellodendron Sinensis in China. Gins. Res. 31, 44–51. doi: 10.19403/j.cnki.1671-1521.2019.02.014
Wen, Y. F., Han, W. J., and Wu, S. (2010). Plant genetic diversity and its influencing factors. J. Central South Univ. For. Technol. 30, 80–87. doi: 10.1406/j.cnki.1673-923.x
Xu, N., Meng, F. Y., Zhou, G. F., Li, Y. F., Wang, B., and Lu, H. (2020). Assessing the suitable cultivation areas for Scutellaria baicalensis in China using the Maxent model and multiple linear regression. Biochem. Systemat. Ecol. 90:104052. doi: 10.1016/j.bse.2020.104052
Xu, Y. D., Huang, Y., Zhao, H. R., Yang, M. L., Zhuang, Y. Q., and Ye, X. P. (2021). Modelling the effects of climate change on the distribution of endangered Cypripedium japonicum in China. Forests 12:429. doi: 10.3390/f12040429
Yan, K., Wang, Z. Y., Sun, W. J., and Wang, X. J. (2013). Phellodendri Amurensis corter standardized planting standard operation practice. For. By Prod. Spec. Chin. 33, 43–46.
Yan, Z. F., Zhang, B. G., Zhang, Z., and Yu, J. L. (2006). Genetic diversity in wild populations of Phellodendron amurense, a rare and endangered medicinal plant, detected by AFLP. Biodivers. Sci. 14, 488–497. doi: 10.1360/biodiv.060041
Yang, Z. P., Xu, J. W., Feng, X. H., Guo, M., Jin, Y. H., and Gao, X. J. (2018). Effects of land use change on habitat based on InVEST model in Northeast China. Ecol. Sci. 37, 139–147. doi: 10.14108/j.cnki.1008-8873.2018.06.018
Yu, J. H., Chen, C. M., Han, S. J., Guo, X. R., Yuan, S. S., Wang, C. J., et al. (2013). Development and characterization of polymorphic microsatellite loci in Phellodendron amurense (Rutaceae). Applic. Plant Sci. 1:1200321. doi: 10.3732/apps.1200321
Yuan, Y. J., and Li, J. F. (1999). Reconstruction and analysis of 450 Year’ winter TemPerature series in the Urumqi rivers source of Tianshan mountains. J. Glaciol. Geocryol. 21, 64–70.
Zeng, Y., Guo, L. P., Wang, J. Y., and Huang, L. Q. (2015). Chemical ecological function of plant flavonoids and the conception of directional cultivation on Chinese Materia Medica. Modern Chin. Med. 17, 776–790. doi: 10.13313/j.issn.1673-4890.2015.8.006
Zhang, L., and Wang, C. Y. (2014). Relationship between species diversity and environmental factors of plant community at different succession stages in Ecotone of great Xing’an and Xiaoxing’an mountains. For. Eng. 30, 1–5. doi: 10.16270/j.cnki.slgc.2014.05.034
Zhang, L., and Zhang, D. L. (2019). Difference in Morphology and Physiology from Male and Female Plant of Phellodendron amurense Rupr. Bull. Bot. Res. 39, 876–882. doi: 10.7525/j.issn.1673-5102.2019.06.010
Zhang, X. Y., Wei, H. Y., Zhang, X. H., Liu, J., Zhang, Q. Z., and Gu, W. (2020). Non-pessimistic predictions of the distributions and suitability of Metasequoia glyptostroboides under climate change using a random forest model. Forests 11:62. doi: 10.3390/f11010062
Zhang, Y. (2015). Correlative study between active components of wild Phellodendron amurense Rupr. and ecological factors. Master’s thesis. Beijing: Beijing Union Medical College.
Zhang, Z. P., Zhang, Y., Zhang, Z., Qi, Y. D., Zhang, B. G., Suo, F. M., et al. (2016). Population survival status and chemical characterization of wild Phellodendron amurense Rupr. in China. Plant Sci. J. 34, 381–390. doi: 10.11913/PSJ.2095-0837.2016.30381
Zhang, Z., Xia, T. R., Tao, Y. H., Dai, L. C., Liu, Y. L., and Zhang, B. G. (2011). Study on allelopathy effect of pericarp extract of Phellodendron amurense. Chin. J. Chin. Mater. Med. 36, 285–288. doi: 10.4268/cjcmm20110312
Zhao, G., Cui, X., Sun, J., Li, T., Wang, Q. I., Ye, X., et al. (2021). Analysis of the distribution pattern of Chinese Ziziphus jujuba under climate change based on optimized Biomod2 and MaxEnt models. Ecol. Indic. 132:108256. doi: 10.1016/j.ecolind.2021.108256
Zhao, L., Yang, G. D., Lian, M. L., and Gao, R. (2013). Status and prospect of research on Phellodendron amurense Rupr. North. Hortic. 15, 212–214.
Keywords: Phellodendron amurense, climate change, ecological niche modeling, landscape pattern, habitat quality, conservation management
Citation: Zhang B, Zou H, Chen B, Zhang X, Kang X, Wang C and Zhang X (2023) Optimizing the distribution pattern of species under climate change: the protection and management of Phellodendron amurense in China. Front. Ecol. Evol. 11:1186627. doi: 10.3389/fevo.2023.1186627
Received: 15 March 2023; Accepted: 05 May 2023;
Published: 19 May 2023.
Edited by:
Ming-Chih Chiu, Chinese Academy of Sciences (CAS), ChinaReviewed by:
Jhih-Rong Liao, Tokyo Metropolitan University, JapanChang Jui Tse, National Taiwan University, Taiwan
Copyright © 2023 Zhang, Zou, Chen, Zhang, Kang, Wang and Zhang. This is an open-access article distributed under the terms of the Creative Commons Attribution License (CC BY). The use, distribution or reproduction in other forums is permitted, provided the original author(s) and the copyright owner(s) are credited and that the original publication in this journal is cited, in accordance with accepted academic practice. No use, distribution or reproduction is permitted which does not comply with these terms.
*Correspondence: Xinxin Zhang, aHNkenh4MjAyMUAxNjMuY29t