- 1School of Economics and Management, Beijing University of Technology, Beijing, China
- 2School of Finance and Taxation, Zhongnan University of Economics and Law, Wuhan, China
- 3Institute of Technology Management, National Tsing Hua University, Hsinchu, Taiwan, China
The integrated development of various industries in China is essential for promoting long-term sustainable development and achieving carbon neutrality. In this study, we analyze panel data from 30 Chinese provinces (excluding Tibet, Hong Kong, Macao, and Taiwan) covering the period between 2005 and 2020 to investigate the impact of collaborative agglomeration between productive service and manufacturing industries on green development efficiency. We utilize a mediation effect model to examine the role of technological innovation in driving the relationship between industrial collaborative agglomeration and regional green development efficiency. Our findings reveal that the collaborative agglomeration of the productive service and manufacturing industries has a significant positive effect on improving regional green development efficiency. We also identify a non-linear relationship, indicating a double threshold effect. Technological innovation plays an important role in facilitating industrial collaborative agglomeration and promoting the efficiency of regional green development. Moreover, our results demonstrate significant regional heterogeneity in the impact of industrial collaborative agglomeration on regional green development efficiency. Based on these findings, we propose several policy recommendations to achieve high-quality regional economic development, including improving the quality of industrial synergy and agglomeration between regions, strengthening the intermediary promotion role of technological innovation, and enhancing regional green productivity.
1. Introduction
Green development is a crucial aspect of attaining high-quality economic growth, emphasizing the need to focus on energy consumption, conservation and emission reduction while promoting regional economic growth. To enhance the level of regional green development, we must consider both economic and ecological performance. Industrial agglomeration serves as a critical driving force for economic development and has a significant impact on improving the ecological environment (Peng et al., 2023). However, due to the continuous adjustment and upgrading of industrial structures, traditional single specialized agglomerations are no longer effective in reflecting the differences between production and intermediate products. We must consider not only the decline of the production advantages of traditional manufacturing but also increase the proportion of productive service industries, improve technological innovation, and enhance enterprise adaptability to external environments (Wood, 2006). Given the strong correlation between productive service and manufacturing industries, achieving coordinated development between them is crucial for promoting the green development of the economy.
The Chinese government's ambitious development goals of peaking carbon emissions by 2030 and achieving carbon neutrality by 2060 present significant challenges to the transformation and development of traditional energy-intensive industries (Tian and Sun, 2022). To achieve these goals, it is crucial to decrease investment in high-emission and high-pollution projects while increasing promotion of new energy projects. This strategy ensures that relevant enterprises can improve their capability and level of sustainable development (Wang et al., 2023). Traditional measures of economic development efficiency focus primarily on capital and labor factor input, whereas green development efficiency emphasizes resource input and environmental cost, better reflecting the potential for regional economic growth and green development strength (Ge et al., 2023). Improving the efficiency of regional green development and achieving high-quality economic growth require greater exchange and integration among different industries to provide a steady stream of impetus for regional economic development. In fact, industrial collaborative agglomeration—a special form of agglomeration economy that involves productive service industry and manufacturing industry working together—can effectively promote regional economic development and realize dual development in energy-saving and emission reduction (Chen et al., 2022).
The study aims to investigate how the synergistic agglomeration of productive services and manufacturing affects the level of regional green development and whether it can promote the transformation of regional economies from traditional to innovative growth models. Additionally, the research seeks to identify the role of technological innovation in this impact. Solving these problems is critical for promoting China's industrial restructuring and implementing the coordinated development strategy. Therefore, this study aims to shed light on these issues and provide practical insights for policy-makers.
2. Literature review
Industrial collaborative agglomeration was first proposed by Ellison and Glaeser (1997) to refer to the spatial agglomeration phenomenon of heterogeneous industries, and scholars have conducted in-depth research on this aspect. And with the development of specialization, the degree of interdependence between productive services and manufacturing industries has become increasingly close, gradually forming a mutually complementary and mutually reinforcing coordination relationship (Kelle, 2013; Liu et al., 2023).
Research on the efficiency of industrial collaborative agglomeration and green development focuses primarily on research methods, impact evaluation, and analysis of impact mechanisms. The measurement of industrial collaborative agglomeration includes various indices such as the E-G index, D-O index, Colocalization index, γ index, and θ index. Ellison et al. developed an industrial collaborative agglomeration index based on the “target model” to better reflect differences in industrial resources (Ellison and Glaeser, 1997). Duranton and Overman (2005) improved the division idea of geographical concentration by constructing the D-O index and then analyzed the agglomeration trend of the manufacturing industry. Billings and Johnson (2016) focused on industrial agglomeration within a single urban area, analyzed its influencing factors by constructing a colocalization index, and found that retail and consumer service industries were less affected by externalities.
Related scholars have used China's prefecture-level city panel data to analyze the degree of industrial collaborative agglomeration by constructing the γ index and θ index, confirming that industrial collaborative agglomeration can effectively improve urban production efficiency and industrial collaborative agglomeration (Chen et al., 2020; Zheng et al., 2022). In terms of evaluating the impact effect, researchers primarily focus on analyzing the impact of industrial collaborative agglomeration on production efficiency. Some scholars use the dynamic panel regression model to examine the impact of productive service industry and manufacturing agglomeration on manufacturing production efficiency, finding that it plays a significant role in promoting manufacturing total factor productivity (Dong et al., 2021). Other scholars have analyzed the impact of industrial agglomeration at the city level on air pollution and found an inverted “N” relationship between the two (Hao et al., 2022). Furthermore, it is observed that industrial agglomeration can reduce urban air pollution levels by influencing environmental regulation, technological progress, and industrial structure upgrading. Some scholars have also studied the impact of industrial collaborative agglomeration on urban total factor productivity and green innovation efficiency, finding a significant positive facilitative effect between the two (Hong et al., 2020; Yuan et al., 2020). In terms of the impact mechanism, some scholars have used the threshold panel regression model to identify that industrial agglomeration mainly affects regional environmental pollution by improving the industrial structure and technological innovation level. Others believe that the impact of industrial agglomeration on the efficiency of urban green development has both positive and negative externalities. Through these analyses and studies, it is confirmed that industrial agglomeration can significantly improve the level of green productivity and provide insights for further research at a theoretical level (Lanoie et al., 2008; Cieślik and Ghodsi, 2015; Xie et al., 2017).
Indeed, there are studies on the innovation effect generated in the process of regional industrial collaborative agglomeration. Researchers have analyzed knowledge exchange between different industries in China and found that there are significant correlation characteristics between cluster technology innovation and regional industrial structure, which plays an important role in promoting the improvement of regional technological innovation (Xu et al., 2022a,b). Scholars have also examined the impact and mechanism of industrial collaborative agglomeration on urban innovation from the perspective of cities and found that it can promote urban innovation by optimizing the allocation of innovation resources and increasing market scale (Wu et al., 2019). Some researchers have also investigated the spatial effect of industrial collaborative agglomeration on regional technological innovation from a spatial perspective and identified differences in the level of industrial collaborative agglomeration under different geographical locations.
While previous research primarily focused on single industrial accumulation, the relationship between productive service and manufacturing agglomeration and green development requires further investigation. Since the concept of green development was proposed, scholars have explained this concept, its strategic significance, and its development mechanism (Zeng and Zhao, 2009; Cuiyun and Chazhong, 2020). Therefore, from the perspective of technological innovation, this study integrates industrial collaborative agglomeration, technological innovation, and green development efficiency into a unified analysis framework, analyzing the impact mechanism of industrial collaborative agglomeration on regional green development efficiency to provide specific guidance for regional low-carbon economic development.
Compared to previous studies, this paper provides the following marginal contributions: Firstly, it integrates industrial synergy, technological innovation, and regional green development efficiency into a unified analysis framework. By analyzing the mediating role of technological innovation, this paper can better clarify the interaction among the three factors. Secondly, this paper considers the nonlinear impact of industrial collaborative agglomeration on regional green development efficiency and examines whether there is a threshold effect. This approach can provide a more comprehensive understanding of the relationship between industrial collaborative agglomeration and green development. Thirdly, this paper analyzes the heterogeneous impact of industrial collaborative agglomeration on regional green development efficiency. This aspect allows for the identification of differences in impact across different regions, providing insights that can be useful for policymakers.
3. Theoretical mechanism and research hypothesis
3.1. The positive externality of industrial collaborative agglomeration on the efficiency of green development
Industrial synergistic agglomeration reflects the spatial proximity of enterprises in different industries due to different complementarities and production needs. Its impact on regional green development efficiency is mainly reflected in positive externalities and negative externalities (Chertow et al., 2008).
Positive externality impact refers to the process of coordinated agglomeration of productive service industry and manufacturing industry, which promotes regional green development. On the one hand, it produces a technology spillover effect through the exchange and cooperation among different enterprises. This phenomenon is conducive to the dissemination and spillover of knowledge and technology among industrial agglomeration areas, helping enterprises master advanced production technology and methods, thereby reducing pollution control costs, improving regional pollution control capabilities, and optimizing regional emission reduction technologies (Appold, 1995).
On the other hand, coordinated agglomeration can create economies of scale. It achieves centralized consumption of resources in different regions, realizes centralized pollution control, and forms a certain development scale by attracting enterprises with similar economic activities to join. This process promotes the sharing of capital, labor, and other factor resources, optimizes the efficiency of resource element allocation, and provides a strong guarantee for local development and services (Zhang et al., 2022).
Hypothesis 1: Industrial collaborative agglomeration can exert positive externality effects, thereby promoting the improvement of regional green development efficiency.
3.2. The negative externality impact of industrial collaborative agglomeration on the efficiency of green development
The negative externalities caused by industrial collaborative agglomeration mainly refer to the process of coordinated agglomeration of productive service industries in the process of regional green development efficiency. Firstly, there is the market competition effect. The agglomeration of different industries in the same region may lead to the accumulation of a large number of production factors and not take advantage of the rational allocation and development of resources in other regions. On the other hand, it also leads to competitive effects, forcing enterprises to continuously improve their competitiveness and technological innovation level, to achieve a virtuous cycle of regional development (Martinus et al., 2020). Secondly, it creates a crowding effect. The agglomeration between industries causes excessive consumption of resources in agglomeration areas, increasing the emission of pollutants from enterprises. This phenomenon is not conducive to the proper management of the ecological environment and poses new challenges to the green development of the regional economy (Nie et al., 2021).
Hypothesis 2: Industrial collaborative agglomeration can exert negative externality effects, thereby improving the efficiency of regional green development.
3.3. The indirect mechanism of action played by technological innovation
Relevant studies show that industrial collaborative agglomeration integrates innovation factors and saves innovation costs by generating knowledge and technology spillover effects (Amiti, 2005). This integration provides a basis for improving the regional technological innovation level. The intercorrelation of inputs between different industries also helps to improve the level of human capital, which in turn generates technological innovation effects. The improvement of the technological innovation level promotes the synergistic agglomeration and development of different industries through growth and knowledge spillover effects, forming a mutually reinforcing development situation (Yang et al., 2022). Therefore, the coordinated agglomeration development of the productive service industry and manufacturing industry not only directly promotes the efficiency of regional green development but also indirectly achieves regional green development by improving the level of technological innovation (Angel, 2002). The role of technological innovation in promoting regional green development is mainly through the reform of production technology of high-emission and high-pollution enterprises, the improvement of clean production technology, the technical reduction of negative impacts on environmental protection in the process of enterprise development, the promotion of regional technological progress, and the elimination of traditional backward industries (Caniëls and Romijn, 2003). The process of industrial collaborative agglomeration promoting regional green development, by leveraging the effect of technological innovation, optimizing the efficiency of resource allocation, and ensuring the establishment of a circular economy system, will have an important impact on the high-quality development of the regional economy.
Hypothesis 3: In the process of promoting the efficiency of regional green development, industrial collaborative agglomeration may play an intermediary role in promoting technological innovation.
3.4. The nonlinear impact of industrial collaborative agglomeration on regional green development efficiency
Indeed, the improvement of the level of collaborative agglomeration between the productive service industry and the manufacturing industry requires a long-term development stage. In the early stages of development, the green development effect of technological progress may not be very significant due to the large amount of capital required to invest in innovative activities. However, with the continuous change of technology, the positive impact of green technology innovation will continue to strengthen. This phenomenon will attract the input of high-end factors, realize the rational allocation of factor resources, and help promote the long-term sustainable development of regional green development efficiency. Therefore, in the process of realizing relevant development, different industries may have a non-linear relationship with regional green development. This relationship will be affected by the constraints of relevant factors (Shijie et al., 2021). It is essential to recognize these complex relationships and understand how they interact with each other to promote regional green development efficiently. Only through coordinated efforts and long-term planning can the full potential of industrial collaborative agglomeration be realized and the goal of sustainable development achieved.
Hypothesis 4: Industrial collaborative agglomeration may have a non-linear relationship with regional green development efficiency, that is, there is a threshold effect.
4. Model setting and data description
4.1. Model settings
4.1.1. Fixed-effect model
To verify the impact of industrial collaborative agglomeration on regional green development efficiency, the following regression model can be constructed:
Among them, Greenit represents the green development efficiency of province i's t-th year, ICAit represents the collaborative agglomeration index of productive service industry and manufacturing industry in province i's t-th year, Zit is the set of control variables, and μi represents the regional fixed effect, γt represents the time fixed effect, δit is the random error term.
4.1.2. Mediation effect model
To investigate the influence mechanism of industrial collaborative agglomeration on the efficiency of green development, this paper adopts the mediation effect model and treats technological innovation as an intermediate variable. Based on the methods of previous research (Muller et al., 2005), we first examine the influence of the core explanatory variables on the dependent variable when no intermediary variables are added. We then investigate whether the core explanatory variables have a significant influence on technological innovation as an intermediary variable. Finally, we put the core explanatory variables and the technological innovation variable into the model at the same time to compare their coefficients and determine the degree of their respective contributions to explaining the variance in the dependent variable. The specific model is constructed as follows:
Among them, the tecit represents the level of technological innovation in the t year of province i, and the meaning of the remaining variables is consistent with the above.
4.1.3. Panel threshold model
To investigate the non-linear effect of industrial collaborative agglomeration on regional green development efficiency, this paper adopts the threshold regression model. The threshold regression model can capture the non-linear relationship between the independent variable and the dependent variable by dividing the sample into separate subgroups based on a specific threshold value. Drawing on Hansen's research method (Hansen, 1999), the threshold regression model can be constructed as follows:
Where β are regression parameters; q is the threshold variable; r is the size of the threshold, if there are two or more thresholds, the model structure must be expanded, this paper uses the double threshold model to analyze, taking the industrial collaborative agglomeration index as the threshold variable, the specific model form is as follows:
Where i, t region and time, μi represent the eigenvalues of the observation, I(.) As an indicative function, εit~iidN(0,δ2) is a random perturbation term, and other variables have the same meaning as above.
4.2. Variable selection and data source
4.2.1. Variable selection
1. Variable to be explained: Green Development Efficiency (Green). To measure the efficiency of regional green development, this paper adopts the method of Zhang et al. (2014) and selects capital (k), labor (l), and energy input (e). The expected output is the level of economic development (y), and the undesired output is the level of environmental pollution (b). Capital investment is measured by the fixed asset investment of the whole society (100 million yuan) in the year, labor input is measured by the total number of employees of the whole society (10,000 people) at the end of the year, and energy input is measured by the total energy consumption of the whole society (10,000 tons of standard coal). The level of economic development is measured by the per capita GDP (10,000 yuan), and the level of environmental pollution is measured by the amount of industrial wastewater, exhaust gas, and solid waste. Based on the research methods of Lin and Guan (2023) and Lin and Qiao (2023), the non-radial direction distance function of all factors and the SBM-DEA model are used to measure the efficiency of regional green development. The non-radial direction distance function can handle both desirable and undesirable outputs simultaneously, and it can effectively measure the efficiency of regional green development. The SBM-DEA model is a widely used method for evaluating the relative efficiency of decision-making units (DMUs) with multiple inputs and outputs. It can also be used to identify the best-performing DMUs and provide insights into potential areas for improvement. By using these methods to measure the efficiency of regional green development, we can obtain a comprehensive and objective assessment of the level of economic growth and environmental protection in a region. This analysis can provide a baseline for evaluating the effectiveness of policies aimed at promoting sustainable development and inform future policy decisions aimed at improving the efficiency of regional green development.
2. Core explanatory variable: To calculate the Industrial Collaborative Agglomeration Index (ICA), this paper refers to the research methods of predecessors (Ding et al., 2022) and mainly selects the relevant data of productive service industries and manufacturing industries in 30 provinces, municipalities (autonomous regions) in China from 2005 to 2020 as the basis of calculation. The specific calculation formula is as follows:
Among them, LQmi and LQm, respectively, indicate the regional entropy index of the i and j industries in the i and j regions, and ICAij indicates the degree of industrial collaborative agglomeration of i industry in the j region.
3. Mediating variable: technological innovation (TEC). To measure the level of technological innovation in each region, this paper uses the number of patents granted as a proxy for innovation output. The number of patents granted is a widely used indicator of technological innovation and can provide a quantitative measure of the level of innovation activity in a region.
4. Control variables: level of openness (FDI), measured by the ratio of foreign direct investment to GDP in each region; Human resources (HR), measured by the ratio of the number of students enrolled in regular colleges and universities in each region to the total number of students in the region; Government expenditure (GOV), measured by the ratio of regional government expenditure to regional GDP; Level of resource endowment (CAP), measured by the ratio of fixed asset investment to labor force employed in each region. The descriptive statistics for each variable are shown in Table 1.
4.2.2. Data sources
This paper utilizes panel data from 30 provinces and cities in China (excluding Tibet, Hong Kong, Macao, and Taiwan) between 2005 and 2020, obtained from official sources such as the China Statistical Yearbook, China Energy Statistical Yearbook, China Environment Statistical Yearbook, and regional statistical yearbooks. To ensure data accuracy and rationality, the following data processing methods are employed: first, the interpolation method is used to complete missing data, resulting in a complete panel dataset; second, logarithms are applied to account for dimensional differences between different sample data and large variance fluctuations. Third, constant price treatment is applied to the data related to the price index in the sample, using 2005 as the base period.
5. Analysis of empirical results
5.1. Benchmark regression analysis
Prior to conducting the benchmark regression, it is necessary to address the issue of multicollinearity. After conducting a test analysis, it was discovered that the variance expansion coefficient of the variables is markedly lower than the value typically required by experience, indicating an absence of multicollinearity issues. Furthermore, this paper selects the fixed-effect model for analyzing the impact of industrial synergy agglomeration on regional green development efficiency, with the specific regression results presented in Table 2.
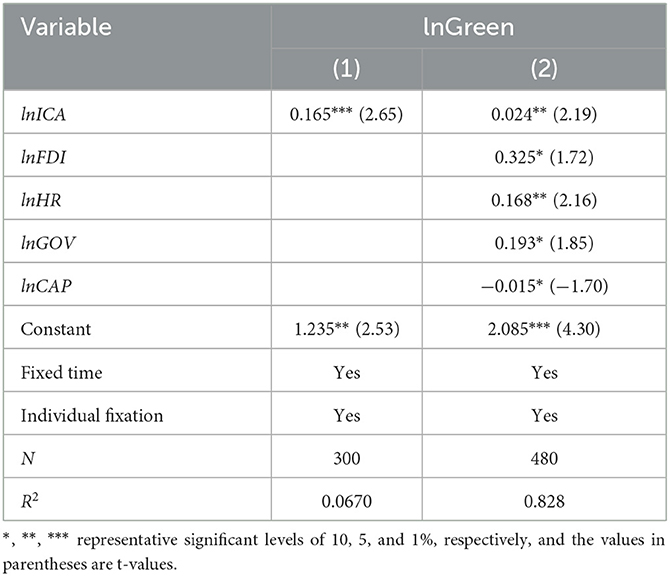
Table 2. Results of industrial collaborative agglomeration on regional green development efficiency.
Table 2 presents the regression results, indicating that in column (1) without control variables, industrial synergy agglomeration has a significantly positive effect on regional green development efficiency at a 1% significance level. Even after adding control variables in column (2), the regression coefficient of green development efficiency remains significant. This suggests that industrial synergy agglomeration can effectively facilitate the integration of different factors and significantly enhance the efficiency of regional green development during times of rapid growth.
5.2. Mediation effect estimation results
This paper employs the mediation effect model to empirically examine the impact of industrial synergy agglomeration on regional green development efficiency, as well as the role of technological innovation as an intermediary. The regression results of this analysis are presented in Table 3.
Table 3 displays the regression results of the impact of collaborative agglomeration between the productive service industry and manufacturing industry on regional green development efficiency, with technological innovation serving as the mediating variable. Model (1) focuses on the effect of industrial synergy agglomeration on green development efficiency. It is observed that an increase in industrial synergy agglomeration significantly promotes regional green development at a 5% significance level. This can be attributed to the fact that an improved industrial agglomeration level fosters closer cooperation and exchange among enterprises, leading to enhanced resource utilization efficiency and factor productivity, while also promoting technological spillover effects between industries and facilitating the adoption of green production technologies by firms.
In addition, an improved industrial agglomeration level also facilitates the internalization of pollutant emissions, reduces regional resource consumption levels, and promotes regional green development. Model (2) examines the impact of technological innovation on regional green development efficiency, which is significantly positive at a 5% level, indicating that technological innovation can serve as an effective intermediary variable. Model (3) incorporates both industrial synergy agglomeration and technological innovation into the regression model, revealing that the role of industrial synergy agglomeration in promoting green development efficiency is significantly strengthened. This provides further evidence supporting the hypothesis that technological innovation plays a mediating role in the process of industrial synergy agglomeration on regional green development efficiency.
The impact of the degree of opening up on regional green development efficiency was found to be positive, but not statistically significant. This may be attributed to the insufficient absorption and transformation capacity of foreign enterprises, leading to a less notable promotion of green development efficiency. On the other hand, the impact of human capital on green development efficiency was significantly positive. Effective accumulation of human capital and rational allocation of capital structure can optimize and adjust inter-industrial structures, transforming low-end and high-pollution industries into high-tech industries with lower energy consumption and pollution levels, hence promoting green development. Moreover, the impact of government fiscal expenditure on regional green development efficiency was also found to be significantly positive, indicating that increased fiscal expenditure can facilitate the realization and application of production technology achievements, while improving the level of regional green technology innovation. However, the level of resource endowment exerted a significant inhibiting effect on green development efficiency, further emphasizing the importance of enhancing regional resource utilization efficiency in promoting better development levels of regional green development.
5.3. Robustness test
To ensure the accuracy of the empirical results, this study employs robustness tests using the following methods: (1) inclusion of lagging variables to account for any potential lag in the impact of industrial synergy agglomeration on green development efficiency; (2) substitution of intermediary variables by using the number of patent applications in each region instead of the level of technological innovation; and (3) exclusion of samples from municipalities directly under the Central Government, given their unique economic development characteristics that may be more constrained by institutional and political factors. The regression results are presented in Table 4, which demonstrate that even with the inclusion of lagging variables, substitution of explanatory variables, or exclusion of municipality samples, the impact of industrial synergy agglomeration on regional green development efficiency remains significantly positive, largely consistent with the benchmark regression results. This further confirms the robustness of the model. Additionally, technological innovation was found to play an important mediating role in promoting green development efficiency through industrial synergy agglomeration. The regression results of the robustness tests are also presented in Table 4.
5.4. Further heterogeneity analysis
Given the differences in regional economic development levels and resource endowments, there may be variations in the impact of coordinated agglomeration of production-oriented service industries and manufacturing industries on the efficiency of green development across different regions. To further investigate this potential differentiation, the study area is divided into three regions: Eastern, Central, and Western, with the specific estimates presented in Table 5.
Table 5 presents the regression results for different regions, indicating that industrial synergy agglomeration and technological innovation significantly promote the improvement of regional green development efficiency in the eastern region. Moreover, after incorporating the mediation variable, the promotion effect is significantly enhanced across the eastern, central, and western regions, with the eastern region exhibiting a higher level of promotion than the central and western regions. This could be attributed to the relatively good economic development level and industrial structure foundation of the eastern region, which can attract significant resource agglomeration, human capital, and foreign investment, providing a solid foundation for the coordinated agglomeration and development of productive service industries and manufacturing industries, which in turn generates economies of scale and technological innovation effects, enhances resource allocation efficiency, and improves economic green development levels. On the other hand, due to the weak economic development strength and low level of industrial structure in the central and western regions, issues such as low innovation efficiency and excessive pollutant emissions from enterprises during the process of industrial synergy agglomeration are more likely to occur, leading to greater environmental destruction than economic promotion, which is not conducive to high-quality development of the regional economy.
5.5. Threshold model regression results
In the process of industrial synergy agglomeration affecting the efficiency level of regional green development, there may be a threshold effect, whereby only when the level of regional industrial synergy agglomeration reaches a certain scale does it promote the efficiency of regional green development. This paper employs an industrial synergy agglomeration threshold variable and uses a threshold regression model to investigate the threshold effect of industrial synergy agglomeration on the efficiency of regional green development. As shown in Table 6, the threshold value and threshold number of the threshold variables were first determined by the regression model. The results indicate that the single threshold effect of industrial synergy agglomeration was significant at a 1% level, while the double threshold effect was significant at a 5% level, and the triple threshold failed the significance test, indicating that the threshold number is two and the threshold values are 0.824 and 0.927, respectively, revealing a threshold effect on the impact of industrial synergy agglomeration on green development efficiency. Therefore, this paper analyzes the double-threshold model of industrial synergy agglomeration selection, with the specific regression results presented as follows.
Table 7 presents the regression results of the threshold effect model, revealing that for different levels of industrial synergy agglomeration development in various regions, when the level of regional industrial synergy agglomeration is below the first threshold of 0.824, industrial synergy agglomeration has a promoting effect on the improvement of regional green development efficiency, but the effect is not significant. When the level of regional industrial synergy agglomeration is between the first threshold of 0.824 and the second threshold of 0.927, it has a significant promoting effect on the improvement of regional green development efficiency. When the level of regional industrial synergy agglomeration is above the second threshold of 0.927, its positive effect on regional green development efficiency increases. Specifically, the impact of industrial synergy agglomeration on the efficiency of regional green development can be divided into three stages. In the early stage of industrial synergy agglomeration development, due to production factors, transaction costs, and location differences, various industries may not establish a good relationship between knowledge, technology, and economy, leading to an expansion of knowledge spillover and crowding effects generated during the process of single industrial agglomeration, which may not be utilized to improve regional green technology innovation levels. However, with the continuous improvement of the level of regional industrial synergy agglomeration, it can better play the role of positive externalities, realize centralized consumption of resources and treatment of pollutants, build a circular economy system, and promote the efficiency of regional green development. Nevertheless, excessive concentration of resources resulting from a high level of regional industrial synergy agglomeration may not be conducive to the rational allocation of resources, thereby weakening the promotion of green development efficiency.
6. Conclusions and policy recommendations
6.1. Research conclusion
In summary, this paper provides a theoretical analysis of the mechanism underlying industrial synergy agglomeration and technological innovation on the efficiency of regional green development. Using data from 30 provinces and cities in China between 2005 and 2020, we employed the non-radial distance function of all factors and the SBM-DEA model to measure regional green development efficiency. The study also used a mediation effect model to investigate the mediating role of technological innovation in promoting the impact of industrial synergy agglomeration on green development efficiency, and incorporated a threshold model to examine the non-linear relationship between the two. Results indicate that industrial synergy agglomeration has a significant positive effect on the development of regional green development efficiency, with a non-linear promotion relationship that exhibits a double threshold effect at threshold values of 0.824 and 0.927, respectively. The intermediary effect shows that technological innovation plays an important intermediary role in the process of industrial synergy agglomeration to promote regional green development efficiency. Additionally, the analysis of regional heterogeneity reveals that the intermediary promotion effect is most significant in the eastern region, with a considerable impact in the central and western regions as well.
6.2. Policy recommendations
Based on the research conclusions above, we propose the following policy recommendations:
Firstly, it is recommended to enhance the quality of collaborative agglomeration between productive service industries and manufacturing industries by promoting their deep integration. To achieve this, the government should consider the local economic development level and the industrial structure of the manufacturing industry when formulating policies, increase investment in productive service industries, adopt functional industrial policies to promote knowledge spillover and technology coupling between these industries, strengthen top-level design between them, and guide productive service industries to form regional agglomerations through the construction of industrial parks. These measures will help to strengthen the connection and cooperation between the two industries. Additionally, inter-regional exchanges should be strengthened, and inter-regional coordinated development achieved through differentiated policies and measures. Further, industrial transfer measures in the eastern region should be improved, the industrial structure level of the central region enhanced, and the high-quality development of industrial structure in the western region promoted, to improve the overall efficiency of resource allocation and realize the ascending development of industrial collaborative agglomeration.
Secondly, we recommend strengthening the intermediary promotion role of technological innovation in enhancing the positive impact of industrial collaborative agglomeration on regional green development. This can be achieved by promoting cross-industry technology exchange and cooperation during the coordinated development of productive service industries and manufacturing industries, leveraging the knowledge and technology spillover effects generated through industrial collaborative agglomeration, and improving the role of inter-industrial collaborative agglomeration in promoting technological innovation. Furthermore, we should improve the flow of factor resources among regions, promote the development and application of information technology, increase investment in knowledge-intensive production-oriented service industries such as scientific and technological research and development, and ensure that the effect of technological innovation is maximized while playing a vital role in promoting regional green development.
Thirdly, we recommend improving the efficiency of transforming scientific and technological innovation achievements to raise the level of regional green productivity. To achieve this, it is necessary to increase scientific and technological expenditure, improve the cultivation of technological innovation ability for enterprises, enhance the level of technological research and development in enterprises, reduce negative externalities caused by pollution emissions from enterprises, and improve the level of human capital to enhance the independent innovation ability of enterprises. Additionally, optimizing the allocation of resources and establishing a resource sharing mechanism among different regions can promote the integrated development of industries and facilitate the green development of industrial clusters, ultimately leading to the high-quality development of regional economies.
Data availability statement
The original contributions presented in the study are included in the article/supplementary material, further inquiries can be directed to the corresponding author.
Author contributions
JL, KZ, FW, and XZ performed the material preparation, data collection, and analysis. BL wrote the first draft of the manuscript. All authors contributed to the study conception and design, commented on previous versions of the manuscript, read, and approved the final manuscript.
Conflict of interest
The authors declare that the research was conducted in the absence of any commercial or financial relationships that could be construed as a potential conflict of interest.
Publisher's note
All claims expressed in this article are solely those of the authors and do not necessarily represent those of their affiliated organizations, or those of the publisher, the editors and the reviewers. Any product that may be evaluated in this article, or claim that may be made by its manufacturer, is not guaranteed or endorsed by the publisher.
References
Amiti, M. (2005). Location of vertically linked industries: agglomeration versus comparative advantage. Eur. Econ. Rev. 49, 809–832. doi: 10.1016/j.euroecorev.2002.12.001
Angel, D. P. (2002). Inter-firm collaboration and technology development partnerships within US manufacturing industries. Reg. Stud. 36, 333–344. doi: 10.1080/00343400220131115
Appold, S. J. (1995). Agglomeration, interorganizational networks, and competitive performance in the US metalworking sector. Econ. Geogr. 71, 27–54. doi: 10.2307/144434
Billings, S. B., and Johnson, E. B. (2016). Agglomeration within an urban area. J. Urban Econ. 91, 13–25. doi: 10.1016/j.jue.2015.11.002
Caniëls, M. C., and Romijn, H. A. (2003). Agglomeration advantages and capability building in industrial clusters: the missing link. J. Dev. Stud. 39, 129–154. doi: 10.1080/00220380412331322851
Chen, C., Sun, Y., Lan, Q., and Jiang, F. (2020). Impacts of industrial agglomeration on pollution and ecological efficiency-A spatial econometric analysis based on a big panel dataset of China's 259 cities. J. Clean. Prod. 258, 120721. doi: 10.1016/j.jclepro.2020.120721
Chen, Y., Zhu, Z., and Cheng, S. (2022). Industrial agglomeration and haze pollution: evidence from China. Sci. Total Environ. 845, 157392. doi: 10.1016/j.scitotenv.2022.157392
Chertow, M. R., Ashton, W. S., and Espinosa, J. C. (2008). Industrial symbiosis in Puerto Rico: environmentally related agglomeration economies. Reg. Stud. 42, 1299–1312. doi: 10.1080/00343400701874123
Cieślik, A., and Ghodsi, M. (2015). Agglomeration externalities, market structure and employment growth in high-tech industries: revisiting the evidence. Miscell. Geogr. 19, 76–81. doi: 10.1515/mgrsd-2015-0007
Cuiyun, C., and Chazhong, G. (2020). Green development assessment for countries along the belt and road. J. Environ. Manag. 263, 110344. doi: 10.1016/j.jenvman.2020.110344
Ding, J., Liu, B., and Shao, X. (2022). Spatial effects of industrial synergistic agglomeration and regional green development efficiency: evidence from China. Energy Econ. 112, 106156. doi: 10.1016/j.eneco.2022.106156
Dong, F., Li, Y., Zhang, X., Zhu, J., and Zheng, L. (2021). How does industrial convergence affect the energy efficiency of manufacturing in newly industrialized countries? Fresh evidence from China. J. Clean. Prod. 316, 128316. doi: 10.1016/j.jclepro.2021.128316
Duranton, G., and Overman, H. G. (2005). Testing for localization using micro-geographic data. Rev. Econ. Stud. 72, 1077–1106. doi: 10.1111/0034-6527.00362
Ellison, G., and Glaeser, E. L. (1997). Geographic concentration in US manufacturing industries: a dartboard approach. J. Polit. Econ. 105, 889–927. doi: 10.1086/262098
Ge, T., Li, C., Li, J., and Hao, X. (2023). Does neighboring green development benefit or suffer from local economic growth targets? Evidence from China. Econ. Modell. 120, 106149. doi: 10.1016/j.econmod.2022.106149
Hansen, B. E. (1999). Threshold effects in non-dynamic panels: estimation, testing, and inference. J. Econ. 93, 345–368. doi: 10.1016/S0304-4076(99)00025-1
Hao, Y., Guo, Y., Li, S., Luo, S., Jiang, X., Shen, Z., et al. (2022). Towards achieving the sustainable development goal of industry: how does industrial agglomeration affect air pollution? Innov. Green Dev. 1, 100003. doi: 10.1016/j.igd.2022.100003
Hong, Y., Lyu, X., Chen, Y., and Li, W. (2020). Industrial agglomeration externalities, local governments' competition and environmental pollution: evidence from Chinese prefecture-level cities. J. Clean. Prod. 277, 123455. doi: 10.1016/j.jclepro.2020.123455
Kelle, M. (2013). Crossing industry borders: german manufacturers as services exporters. World Econ. 36, 1494–1515. doi: 10.1111/twec.12111
Lanoie, P., Patry, M., and Lajeunesse, R. (2008). Environmental regulation and productivity: testing the porter hypothesis. J. Prod. Anal. 30, 121–128. doi: 10.1007/s11123-008-0108-4
Lin, B., and Guan, C. (2023). Evaluation and determinants of total unified efficiency of China's manufacturing sector under the carbon neutrality target. Energy Econ. 106539. doi: 10.1016/j.eneco.2023.106539
Lin, B., and Qiao, Q. (2023). Exploring the participation willingness and potential carbon emission reduction of Chinese residential green electricity market. Energy Policy 174, 113452. doi: 10.1016/j.enpol.2023.113452
Liu, Y., Ren, T., Liu, L., Ni, J., and Yin, Y. (2023). Heterogeneous industrial agglomeration, technological innovation and haze pollution. China Econ. Rev. 77, 101880. doi: 10.1016/j.chieco.2022.101880
Martinus, K., Suzuki, J., and Bossaghzadeh, S. (2020). Agglomeration economies, interregional commuting and innovation in the peripheries. Reg. Stud. 54, 776–788. doi: 10.1080/00343404.2019.1641592
Muller, D., Judd, C. M., and Yzerbyt, V. Y. (2005). When moderation is mediated and mediation is moderated. J. Pers. Soc. Psychol. 89, 852. doi: 10.1037/0022-3514.89.6.852
Nie, X., Wei, X., Xia, Q., and Zhou, M. (2021). Customers' purchase intention for hydrogen vehicle and industrial agglomeration: evidence from Jiangsu Province, China. Int. J. Hydrogen Energy 46, 18011–18019. doi: 10.1016/j.ijhydene.2020.10.055
Peng, H., Lu, Y., and Wang, Q. (2023). How does heterogeneous industrial agglomeration affect the total factor energy efficiency of China's digital economy. Energy 126654. doi: 10.1016/j.energy.2023.126654
Shijie, L., Hou, D., Jin, W., and Shahid, R. (2021). Impact of industrial agglomeration on environmental pollution from perspective of foreign direct investment—a panel threshold analysis for Chinese provinces. Environ. Sci. Pollut. Res. 28, 58592–58605. doi: 10.1007/s11356-021-14823-4
Tian, T., and Sun, S. (2022). Low-carbon transition pathways in the context of carbon-neutral: a quadrilateral evolutionary game analysis. J. Environ. Manag. 322, 116105. doi: 10.1016/j.jenvman.2022.116105
Wang, F., Wu, M., and Wang, J. (2023). Can increasing economic complexity improve China's green development efficiency? Energy Econ. 117, 106443. doi: 10.1016/j.eneco.2022.106443
Wood, P. (2006). Urban development and knowledge-intensive business services: too many unanswered questions? Growth Change 37, 335–361. doi: 10.1111/j.1468-2257.2006.00327.x
Wu, J., Wei, Y. D., Chen, W., and Yuan, F. (2019). Environmental regulations and redistribution of polluting industries in transitional China: understanding regional and industrial differences. J. Clean. Prod. 206, 142–155. doi: 10.1016/j.jclepro.2018.09.042
Xie, B. C., Duan, N., and Wang, Y. S. (2017). Environmental efficiency and abatement cost of China's industrial sectors based on a three-stage data envelopment analysis. J. Clean. Prod. 153, 626–636. doi: 10.1016/j.jclepro.2016.12.100
Xu, J., Zhao, J., She, S., and Liu, W. (2022a). Green growth, natural resources and sustainable development: evidence from BRICS economies. Resour. Policy 79, 103032. doi: 10.1016/j.resourpol.2022.103032
Xu, Y., Li, X., Tao, C., and Zhou, X. (2022b). Connected knowledge spillovers, technological cluster innovation and efficient industrial structure. J. Innov. Knowl. 7, 100195. doi: 10.1016/j.jik.2022.100195
Yang, T., Zhou, K., and Zhang, C. (2022). Spatiotemporal patterns and influencing factors of green development efficiency in China's urban agglomerations. Sust. Cities Soc. 85, 104069. doi: 10.1016/j.scs.2022.104069
Yuan, H., Feng, Y., Lee, C. C., and Cen, Y. (2020). How does manufacturing agglomeration affect green economic efficiency? Energy Econ. 92, 104944. doi: 10.1016/j.eneco.2020.104944
Zeng, D. Z., and Zhao, L. (2009). Pollution havens and industrial agglomeration. J. Environ. Econ. Manag. 58, 141–153. doi: 10.1016/j.jeem.2008.09.003
Zhang, N., Kong, F., Choi, Y., and Zhou, P. (2014). The effect of size-control policy on unified energy and carbon efficiency for Chinese fossil fuel power plants. Energy Policy 70, 193–200. doi: 10.1016/j.enpol.2014.03.031
Zhang, W., Liu, G., Gonella, F., Xu, L., and Yang, Z. (2022). Research on collaborative management and optimization of ecological risks in urban agglomeration. J. Clean. Prod. 372, 133735. doi: 10.1016/j.jclepro.2022.133735
Keywords: industrial collaborative agglomeration, technological innovation, green development efficiency, mediation effect, threshold effect
Citation: Liu B, Luo J, Zheng K, Wu F and Zhao X (2023) How will industrial collaborative agglomeration affect the efficiency of regional green development? Front. Ecol. Evol. 11:1179004. doi: 10.3389/fevo.2023.1179004
Received: 03 March 2023; Accepted: 17 April 2023;
Published: 10 May 2023.
Edited by:
Magdalena Klimczuk-Kochańska, University of Warsaw, PolandReviewed by:
Xing Li, Shanghai University of Finance and Economics, ChinaMuammer Koç, Hamad Bin Khalifa University, Qatar
Copyright © 2023 Liu, Luo, Zheng, Wu and Zhao. This is an open-access article distributed under the terms of the Creative Commons Attribution License (CC BY). The use, distribution or reproduction in other forums is permitted, provided the original author(s) and the copyright owner(s) are credited and that the original publication in this journal is cited, in accordance with accepted academic practice. No use, distribution or reproduction is permitted which does not comply with these terms.
*Correspondence: Jian Luo, luojianxishuai@163.com