- 1Suisun Resource Conservation District, Suisun City, CA, United States
- 2Chapman University, Environmental Science and Policy Program, Orange, CA, United States
- 3Department of Watershed Sciences, Utah State University, Quinney College of Natural Resources, Logan, UT, United States
Introduction: Coastal estuarine wetlands provide important habitats for a variety of endemic flora and fauna but are particularly vulnerable to biological invasions. Regular monitoring of changes in these vulnerable wetlands has become increasingly important for effective management, especially considering threats from climate change effects and human disturbance. Historical analyzes of plant invasions may guide targeted management strategies to eradicate harmful species. Estimating the distribution of invasive species has never been more accessible with the improved availability of high-resolution data and innovations in remote sensing, estimating the distribution of invasive species has never been more accessible.
Methods: We assessed the spread of non-native Phragmites australis subsp. australis in Suisun Marsh on the upper San Francisco Estuary, one of the largest brackish coastal wetlands in North America. Suisun Marsh consists of managed and tidal wetlands, and efforts have been made to control invasive P. australis on the managed wetlands to support habitat values for wildlife. We used remote-sensing analyzes of publicly available, biennial color-infrared images taken by the National Agriculture Imagery Program (NAIP) to map the expansion of invasive P. australis across two decades. We generated random forest classifications of representative images to map the distribution of P. australis, then calculated a variety of metrics describing the rate and spatial extent of the P. australis spread. Additionally, we ran generalized linear models to examine factors related to the growth of P. australis.
Results: Our classifications yielded accuracies of over 90% and showed a 234% (1,084 ha) increase in P. australis between 2003 and 2018. The expansion rate of P. australis patches averaged 1.32 m/year (±0.53 SD) which is higher than most reported in the literature. We found that P. australis expansion in managed areas within levees was significantly correlated with invasion in tidal areas outside the levees on the same parcel and also related to its spread on adjacent parcels.
Discussion: Our findings suggest that despite individual landowner management efforts, P. australis has continued to expand substantially throughout Suisun Marsh. Future efforts to treat invasive P. australis may require emphasizing adaptive, collaborative management rather than individual management strategies to ensure the invasive species is eradicated on a large scale to preserve the valued ecosystem functions.
1. Introduction
The spread of non-native, invasive species poses a major threat to plant diversity, ecosystem function, and habitat quality (Vitousek et al., 1997; Mack et al., 2000; Quirion et al., 2018; Tadros et al., 2020). An estimated 5,000 invasive plant species have been introduced into the United States (Pimentel et al., 2000; Tallamy, 2004) and managing the spread of these non-native plants costs millions of dollars annually (Pimentel et al., 2000). The non-native common reed (Phragmites australis subsp. australis; hereafter P. australis) is one such plant–it is a broadly distributed grass species now found throughout the wetlands of North America. While there are some native P. australis lineages in different parts of North America (Phragmites australis subsp. americanus), the Eurasian lineage of P. australis was first introduced in the early 19th century and has since expanded throughout the continent (Saltonstall, 2002; Meyerson et al., 2010a). The Eurasian lineage has aggressively expanded into native wetlands and altered vegetation communities (Saltonstall, 2002; Meyerson et al., 2010a,b; Kettenring and Mock, 2012). It is now widely considered to be invasive in most wetland ecosystems across North America and especially in tidal marshes, brackish wetlands, salt marshes, and freshwater coastal wetlands (Meyerson et al., 2010a,b; Kettenring et al., 2012).
Invasive P. australis has been shown to cause detrimental impacts to native plant biodiversity, the quality of wildlife and fish habitat, and sedimentation rates (Lambert et al., 2010; Dibble et al., 2013; Wails et al., 2021). While in its native European range, P. australis reedbeds support a variety of specialized bird species (Poulin et al., 2002; Battisti et al., 2020), there is little evidence to suggest that invasive P. australis provides habitat for these species in North America (Benoit and Askins, 1999; Robichaud and Rooney, 2017; Tozer and Mackenzie, 2019). In addition, human development has exacerbated the spread of P. australis; its invasions are often associated with the soil denudation, nutrient enrichment, and hydrologic alteration caused by anthropogenic activities (Saltonstall, 2002; Kettenring et al., 2011; Mozdzer et al., 2013; Long et al., 2017b). For these reasons, the management of P. australis has become a priority for conservation practitioners in many wetlands (Hazelton et al., 2014; Long et al., 2017a).
Managing to control or eradicate P. australis is notoriously challenging (Hazelton et al., 2014; Long et al., 2017a). As a rhizomatous grass, P. australis can propagate both clonally as an underground network of rhizomes and via seeds resulting in rapid expansion from a local source (Kettenring et al., 2011; McCormick et al., 2016; Minchinton and Bertness, 2023). Early detection and rapid response (EDRR) efforts to control small patches (i.e., seed source patches) followed by efforts to slow expansion of increasingly larger patches is likely to lead to the most effective management across the landscape (Long et al., 2017a, Quirion et al., 2018; Rohal et al., 2019a). Numerous methods have been developed for treating P. australis with herbicides including hand-wiping, backpack spraying, and aerial spraying and with non-chemical approaches such as mowing, burning, and grazing (Hazelton et al., 2014; Samiappan et al., 2017; Volesky et al., 2017). However, while some methods may effectively control P. australis in the short term, a lack of monitoring and continuous follow-up treatments may result in reemergence of P. australis 2–3 years after the original control efforts (Lombard et al., 2012; Hazelton et al., 2014). Therefore, effectively managing P. australis to recover preferred wetland plant communities may be expensive to achieve over long time frames (Rohal et al., 2019a,b, 2023). A better understanding of the distribution of P. australis and the mechanisms that control its spread is necessary to improve management of this invasive species and to reduce its negative effects.
With advancements in the quality of aerial imagery and spatial analyzes, remote sensing has emerged as a valuable tool for studying and monitoring plant invasions (Samiappan et al., 2017; Abeysinghe et al., 2019; Paz-Kagan et al., 2019; Royimani et al., 2019). Recent images collected by satellites, aircraft, or unmanned aerial vehicles (UAVs) can be used to generate coverages for landscapes at a very high (<1-meter) resolution. Furthermore, remotely-sensed imagery provides a unique opportunity to examine historical landscapes and to detect changes, even where ground data are unavailable or costly (Andrew and Ustin, 2009). Covariates derived from remote-sensing imagery can be used to identify plant communities or particular species of interest with high accuracy (Andrew and Ustin, 2009; Samiappan et al., 2017; Abeysinghe et al., 2019; Paz-Kagan et al., 2019; Tadros et al., 2020). For example, Samiappan et al. (2017) used texture analysis to classify P. australis in the wetlands of Louisiana with an average accuracy of 85%, while in northern China invasive Spartina alterniflora was identified to a similarly high degree of accuracy (87–100%; Okoye et al., 2020). These classifications can then be used to target management efforts and reduce non-native plant invasions to improve overall ecosystem health (Abeysinghe et al., 2019; Tadros et al., 2020). Classifications of non-native plant species further provide the ability to follow EDRR principles and detect biological invasions early, providing managers with a better opportunity to eradicate or control the problem (Huang and Asner, 2009; Bradley, 2014).
While previous research has used aerial or satellite imagery to classify P. australis and other co-occurring vegetation in other regions of the United States (Rice et al., 2000; Philipp and Field, 2005; Samiappan et al., 2017; Long et al., 2017b; Abeysinghe et al., 2019), little effort has been directed at measuring the invasion of P. australis in Suisun Marsh in the upper reach of the San Francisco estuary, the largest contiguous brackish marsh on the Pacific coast of the continental U.S. Suisun Marsh is recognized as an important wetland for regional biodiversity supporting many endemic species of wildlife and plants and as an important migratory stopover for waterbirds along the Pacific Flyway (Moyle et al., 2014). A comprehensive understanding of the trajectory of the P. australis invasion in Suisun Marsh is especially crucial in light of the impending threats of sea-level rise that may further degrade natural resources in this region (Takekawa et al., 2006; Thorne et al., 2018). Native plants may struggle to tolerate increased inundation and salinity caused by sea-level rise, providing an opportunity for P. australis invasion and expansion (Patger et al., 2005; Touchette et al., 2007; Eller et al., 2017).
Our primary objective was to estimate the magnitude, distribution, and potential mechanisms of P. australis spread in Suisun Marsh. Thus, we used publicly available aerial imagery to conduct a historical analysis of the distribution and spread of P. australis in Suisun Marsh over two decades from 2003 to 2020. We used an iterative machine-learning approach (Breiman, 2001; Cutler et al., 2007) to compare patches of P. australis and non-P. australis and to generate an estimate of the changing extent of P. australis. We also compared the distributions generated with our method compared with those estimated with a manual classification approach to see if our models produced similar or higher accuracies.
2. Methods
2.1. Study area
We examined the historic spread of P. australis in the 46,950-ha brackish Suisun Marsh located on the upper reach of the San Francisco estuary in northern California, United States (38.1475 N, −122.0053 W). The region provides crucial habitat for over 200 bird species, 45 mammal species, and a wide variety of native plants (CDFW, 2023). These species include the endangered salt marsh harvest mouse (Reithrodontomys raviventris) and the endemic Suisun thistle (Cirsium hydrophilum var. hydrophilum). It also serves as an important nursery for several fishes in the San Francisco estuary, such as the critically endangered delta smelt (Hypomesus transpacificus) and endangered runs of Chinook salmon (Oncorhynchus tshawytscha). Ownership and management of wetlands involves a mix of public, private, and nonprofit landowners (CDFW, 2011).
There are two major types of wetlands in Suisun Marsh (Figure 1): managed wetlands that are surrounded by levees with water infrastructure controlling the timing and duration of applied water, and tidal wetlands that are open to the influence of the mixed diurnal tides resulting in twice-daily high and low tides differing in height by up to 2 m (CDFW, 2011). Most of the land parcels are managed wetlands with vegetated tidal berm areas on the exterior side of levees, although in the recent past, several parcels have been restored to tidal wetlands to benefit fish. Private landowners primarily oversee managed wetlands to support waterfowl habitat during the winter months, a traditional land use practice that was established in the late 1800s (Arnold, 1996). The area has been protected by state law since 1977, and recent goals for the region established in the Suisun Marsh Habitat Management, Preservation, and Restoration Plan EIR/EIS (CDFW, 2011) have included enhancing 16,000–20,000 ha of managed wetlands and restoring 2,000–3,000 ha of tidal wetlands.
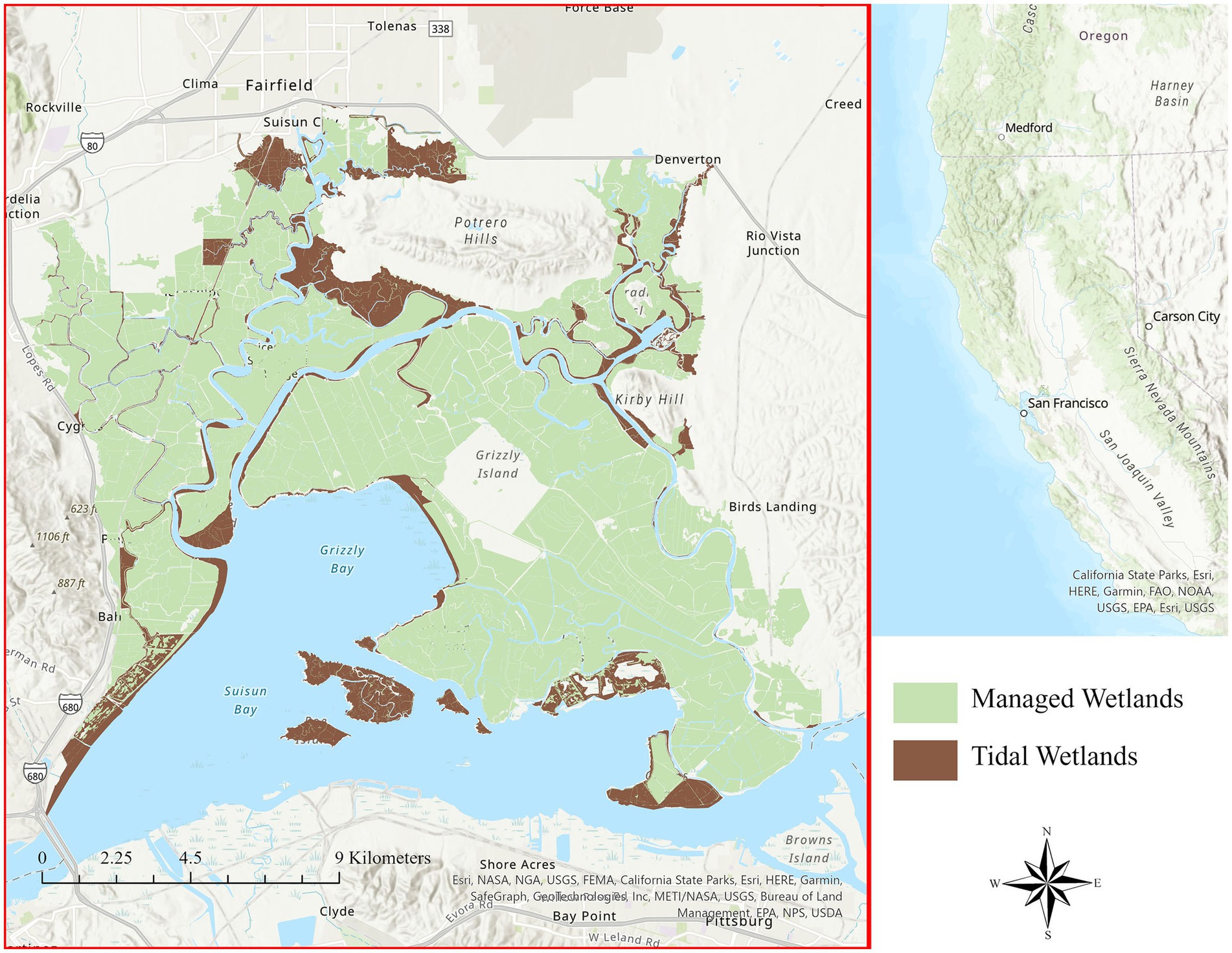
Figure 1. Suisun Marsh in the upper San Francisco estuary of northern California, United States. The location of Suisun Marsh in central California is indicated by the red square on the right inset map. Managed wetlands are shaded green, and tidal wetlands are brown. There are an estimated 20,562 hectares of managed and tidal wetlands in Suisun Marsh.
While the exact timing of the invasion is unknown, it is believed that non-native P. australis was introduced to Suisun Marsh about 50–60 years ago. Historic reports and vegetation survey results do not mention P. australis until after the 1970s, but the species was not a dominant plant reported in the region at that time (Simpson and Baruth, 1966). The invasion was still not considered to be extensive as recently as 20 years ago when P. australis was found only occasionally in brackish marshes of the region (Chambers et al., 1999). The dramatic expansion of P. australis in the estuary is therefore a recent phenomenon. Thus, while it remains a possible that some native Phragmites australis subsp. americanus are found Suisun Marsh (B. Grewell, pers. comm.), the majority of the species in the region are considered to be the invasive Eurasian lineage (Saltonstall, 2002).
2.2. Random forest classifications
We obtained aerial imagery from 2003 to 2020 taken under the National Agriculture Imagery Program (NAIP) of the U. S. Department of Agriculture. In California, these images were collected every 3 years from 2003 to 2009 and every 2 years from 2010 to the present. NAIP flights are typically conducted from early April to mid-August with most images of Suisun Marsh collected in May (2012, 2016, 2020) or June (2003, 2006, 2009, 2014). The exception to this timing was in 2018 when images were taken in July. Aerial imagery obtained just before peak growth season (late-spring to early-summer in Suisun Marsh) may allow for early detection of P. australis (Abeysinghe et al., 2019) which would be helpful to promote a rapid response (Reaser et al., 2020); however, P. australis does not reach its peak growth until the late summer (Rice et al., 2000; Engloner, 2009), and new growth may not be detected until the subsequent year’s images are taken. NAIP images from 2003 and 2006 had a 2-meter resolution and included three color bands: red, blue, and green. From 2009 onwards, NAIP images included an additional band of near infrared (NIR) and were processed at a 1-meter resolution. Therefore, our classifications were built on slightly different image characteristics pre-2009 versus post-2009; however, our preliminary analyzes indicated that these resolution differences were not substantially affecting our classifications.
We generated classifications from aerial imagery spanning eight different years over the past two decades: 2003, 2006, 2009, 2012, 2014, 2016, 2018, and 2020. For each year, representative P. australis patches were selected from the image and manually classified (Tadros et al., 2020) to use as a baseline to automate classification of the entire image. Phragmites australis often displays a unique, lime-green spectral signature and forms ovular patches (Kettenring et al., 2016; Figure 2). These patches were assumed to be homogeneous polygons of P. australis, since the species forms dense monocultures within 1–2 years of its initial invasion (Orson, 1999; Holdredge and Bertness, 2011). Separate polygons were manually selected to serve as “non-P. australis” data for the classifications; these polygons were much larger than the P. australis polygons and included a wide range of spectral values including those with signatures similar to P. australis.
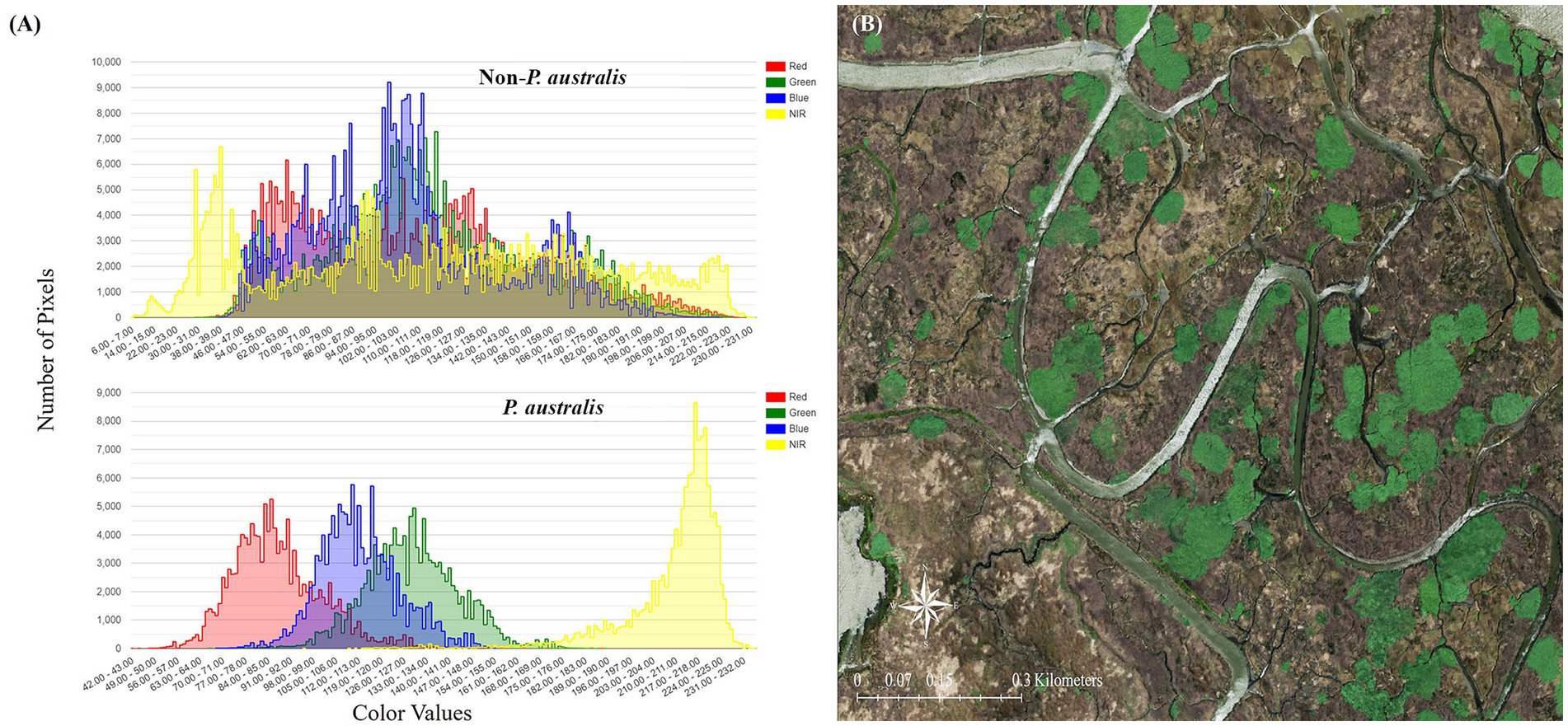
Figure 2. (A) Spectral signature of Phragmites australis subsp. australis and non-P. australis patches. Note that P. australis displays a unique signature and is visible in a narrow range of red, blue, green, and near-infrared values. (B) Distinct, green patches of P. australis in Suisun Marsh as they appear in the 2020 National Agriculture Imagery Program (NAIP) imagery.
For each classification, the P. australis and non-P. australis polygons were randomly subset into groups: 70% for training and 30% for validation (Paz-Kagan et al., 2019). Within the P. australis training polygons, 3,500 pixels were randomly selected for analysis, and within the validation polygons, 1,500 pixels were selected. Seven thousand pixels were randomly selected for analysis from the non-P. australis training polygons, and 3,000 pixels were randomly selected from the validation polygons. This process ensured that we maintained the 70:30 split between training and validation data while including substantially more non-P. australis than P. australis pixels in our models.
Classifications were built with three color bands for 2003 and 2006 and four bands (color and infrared) for 2009–2020. We also included an additional predictor variable for every year following 2003 which described the distance of every pixel from the nearest P. australis pixel classified on the previous image. Phragmites australis often spreads rapidly from a source patch (Lathrop et al., 2003; Kettenring et al., 2011, 2016); therefore, we expected that if P. australis was present in a given location in 1 year, there was a much greater probability of its presence nearby in the subsequent year. This additional predictor variable was created as a continuous raster in ArcGIS Pro (ESRI, 2019) with the “Euclidean Distance” tool from a shapefile of the previous year’s classification (Eq. 1). Covariate values were extracted at each P. australis and non-P. australis point in R Studio Version 1.2.5033 (R Core Team, 2021; R Studio Team, 2021). We generated models from covariates derived from the publicly accessible imagery to simplify the analysis and make it easier to replicate the classification for continued future monitoring of P. australis. We used the equation,
where, Phrag(t) is the distribution of P. australis at time t, red(t), blue(t), green(t), and NIR(t) refer to color and infrared values extracted from NAIP imagery at time t, and Phrag_Dist(t – 1) is the distribution of P. australis in the previous classification iteration. We used random forest classifiers (Breiman, 2001; Cutler et al., 2007) built in R to compare the covariate values of P. australis points against those of non-Phragmites points. Random forest is a machine-learning model which generates a series of decision trees that each “cast a vote” for the most popular output class based on input vectors (Breiman, 2001; Pal, 2005). We conducted preliminary testing on a variety of other model types including Mahalanobis distance, Maximum Likelihood, and Spectral Angle Mapping (SAM) on the 2020 NAIP imagery; however, random forest produced the highest accuracy metrics and was selected for our analyzes (Supplementary Table S1).
Random forest classifiers were generated using the package “randomForest” (Liaw and Wiener, 2002), and we employed 500 decision trees. To examine the accuracy of our classifiers, we compared the classes predicted by the model against the observed classes for our validation points. This process allowed us to calculate a variety of accuracy metrics including user’s accuracy, producer’s accuracy, overall accuracy, and Kappa’s statistic (Fielding and Bell, 1997; Kraemer, 2015) for all classifications. User’s accuracy is calculated for each class separately and describes the proportion of validation points that were correctly classified by the model. Producer’s accuracy is similarly calculated for each class separately and measures the proportion of points classified as a class that are truly that class. Overall accuracy describes the percentage of all validation points that were correctly classified by the model. User’s, producer’s, and overall accuracies are measured on a scale from 0.00 to 1.00 (0–100%). Lastly, Kappa’s statistic yields a metric similar to overall accuracy, while accounting for random chance in classification. Kappa’s statistic is measured from −1.00 to 1.00 with higher values indicating higher accuracies.
For each year, we ran five random forest models based on a different random selection of training and validation data and averaged the accuracy metrics. The singular random forest model which produced the best accuracy metrics was then used to create a map of predicted P. australis each year with the package “raster” in R (version 3.6–11; Hijmans and van Etten, 2012). This process produced a 2-meter (2003, 2006) or 1-meter (all other years) resolution raster with values of “1” to represent P. australis and “0” to represent non-P. australis.
2.3. Assessment of spread in Suisun Marsh
For each classification year, we examined a variety of metrics in ArcGIS Pro to better quantify the spread of P. australis. We calculated the hectares of classified P. australis for each year for all wetlands, managed wetlands and tidal wetlands, and the magnitude of change between each classification. We also quantified the amount of overlap between P. australis from one classification to the previous classification to determine the extent to which established patches persisted. This metric served to describe the spread of P. australis as expansion from existing patches compared to new emergent patches.
Since the rate of expansion is considered an important component of invasion (Kettenring et al., 2016), we calculated it on six representative patches distributed throughout the region that were present in both 2003 and 2020 (Figure 3). These patches needed to be spatially distinct from each other and unconfined by natural or anthropogenic barriers that could inhibit growth (i.e., roads or upland edges). We chose to measure perimeter expansion, rather than area expansion, following the methods described in Philipp and Field (2005). While generally ovular, P. australis patches can display irregular shapes, and we generated ellipses for each patch in each classification year to reconcile this issue. We found that the area and semi-major axis of these ellipses matched those of their respective patches as calculated in ArcGIS Pro, and a perimeter expansion rate (in meters/year) could be calculated from these ellipses by averaging the increase or decrease in both the semi-major and semi-minor axes and dividing by the number of years between classifications.
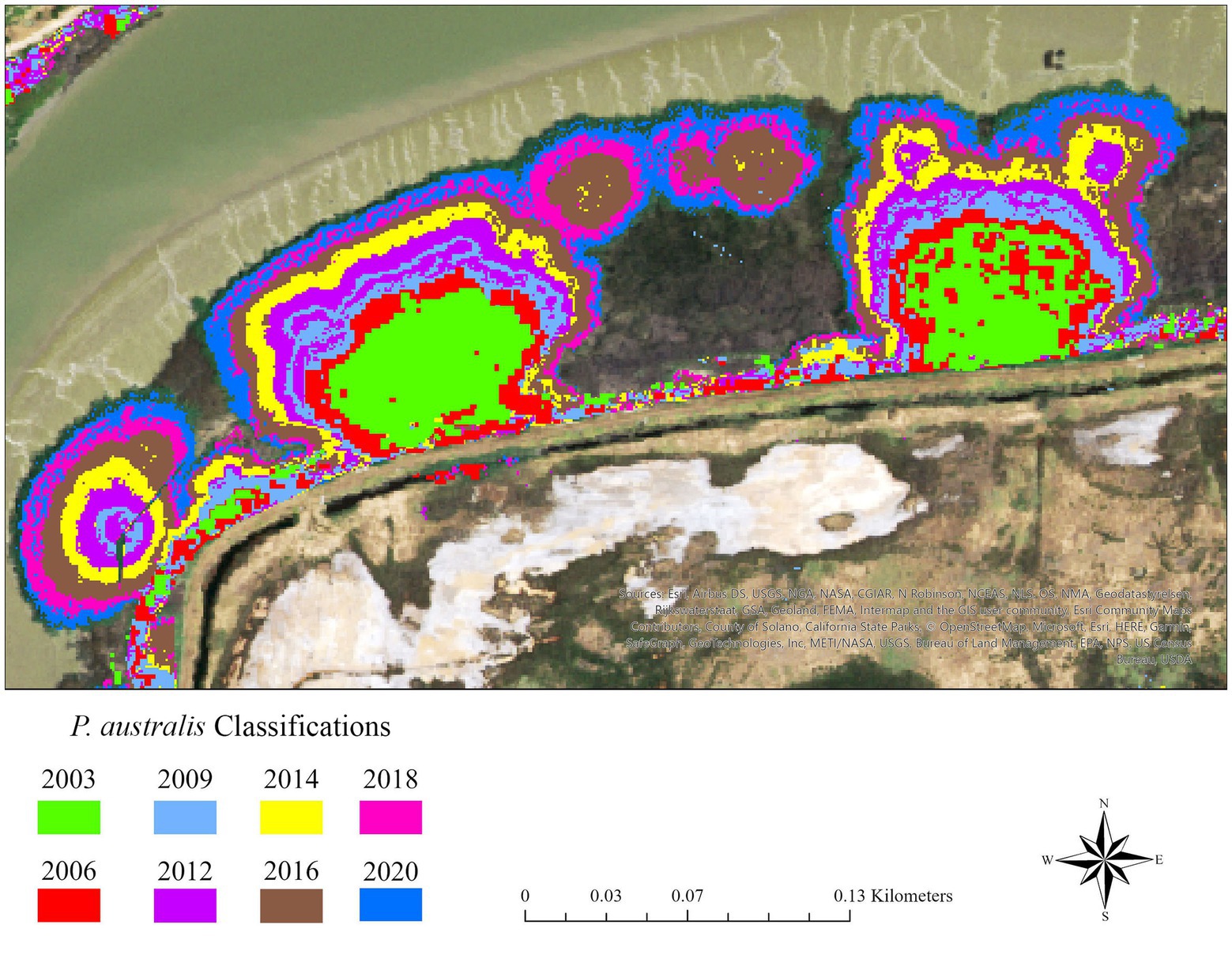
Figure 3. Expanding patches of Phragmites australis subsp. australis on Lower Joice Island, a parcel with both managed and tidal wetlands in Suisun Marsh, upper San Francisco estuary, northern California, United States. The patches are determined from random forest classifications where different colors are associated with different years. The growth in the size of existing patches is likely indicative of growth from spreading rhizomes.
2.4. Comparison to VegCAMP manual classification
We examined the efficacy of our random forest models in classifying P. australis against a manual classification of the Suisun Marsh plant communities established in 1999 to detect change in habitats of the endangered salt marsh harvest mouse (CDFW, 2011; Askim et al., 2022). The Vegetation Classification and Mapping Program (VegCAMP) was established by the California Department of Fish and Wildlife to provide a vegetation mapping standard for the state (Askim et al., 2022). Observers manually identify plant associations or species from a mosaic of true-color images collected in the year of interest to create vegetation maps of key areas in California including Suisun Marsh (Askim et al., 2022).
VegCAMP maps have been generated from aerial images of Suisun Marsh taken every 3 years since 2000, and each report documents the vegetation composition that existed 3 years prior (the 2021 VegCAMP release describes the vegetation in 2018, for example). The 3-year or longer delay in providing the vegetation maps has been caused by the extensive manual processing and has been considered to be a shortcoming of VegCAMP, as the outdated information limits its value for ongoing annual vegetation management. Furthermore, VegCAMP plant associations are produced on a relatively coarse scale with the average polygon measuring 0.69 ha and the minimum measuring 0.10 ha (Askim et al., 2022) compared to 1-square meter pixels analyzed with NAIP imagery. Therefore, VegCAMP is unable to identify small, emergent vegetation patches, while remote sensing classifications with NAIP offer a quicker, cheaper, and more accurate method for examining specific vegetation types on a local scale and to guide invasive species management.
We extracted P. australis polygons from VegCAMP classifications in ArcGIS Pro by selecting classes labeled Phragmites australis from the “NVCSName” attribute. We compared the accuracy of VegCAMP relative to our NAIP classifications by (1) measuring the percent overlap between the two classifications for each year in ArcGIS Pro, and (2) calculating user’s, producer’s, and overall accuracies from the P. australis and non-P. australis polygons that we outlined to create our random forest models. Comparisons were made for the 5 years during which VegCAMP and NAIP overlapped in 2003, 2006, 2009, 2012, and 2018.
2.5. Generalized linear mixed models
Management of P. australis in Suisun Marsh has been ongoing since 2000 (S. Chappell, pers. comm.); however, the type of management practices allowed depends on the type of wetland (managed or tidal). The Suisun Resource Conservation District (SRCD) leads a P. australis control program in Suisun Marsh that was initiated in 2000 to encourage managed wetland landowners to control P. australis supported by funds from a foundation grant to subsidize the cost of herbicides for private landowners from 2000 to 2021 (S. Chappell, pers. comm.). Treatment on managed wetlands including herbicide applications is allowed when the marsh plains are drained for maintenance work during the mid-summer (July–September). However, treating P. australis with herbicides is not allowed on tidal wetlands to protect rapid dispersal into the estuarine waters, and most non-chemical methods are restricted in tidal wetlands to protect endangered species habitat. Therefore, land parcels that include areas open to tides often have source populations of P. australis that are essentially untreatable. In addition, Suisun Marsh is comprised of a mixture of both public and private landowners who make management decisions independently (Figure 4), and P. australis management may therefore differ greatly between neighboring land parcels.
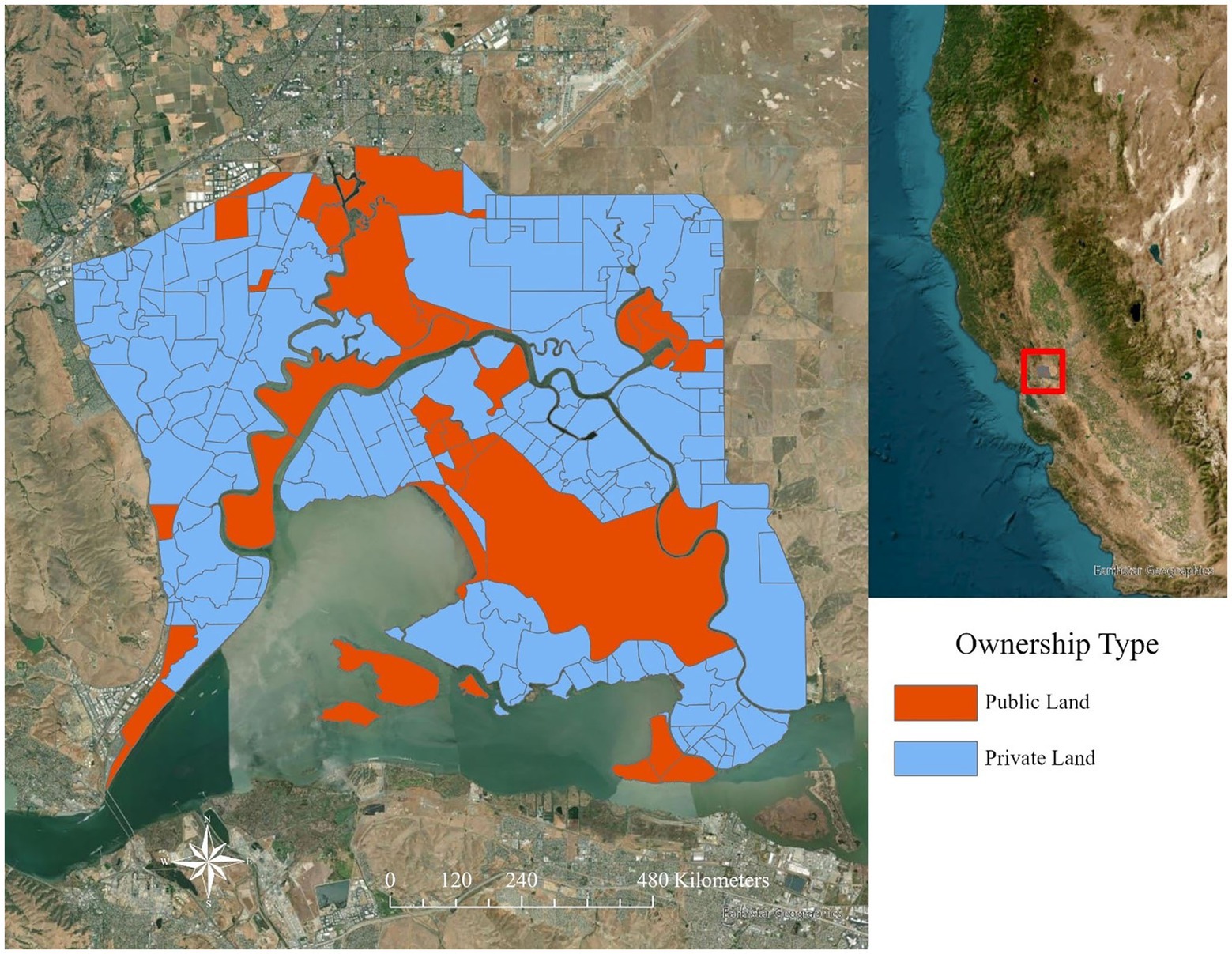
Figure 4. Ownership of land parcels in Suisun Marsh, upper San Francisco estuary in northern California, United States. Public lands are displayed in orange and private land parcels (including nonprofits) are shown in blue.
To examine the effect of these management differences for managed and tidal wetlands, we generated generalized linear mixed models in R. We assessed the relationship between P. australis growth on wetland parcels with both managed and tidal areas or on wetland parcels with neighboring parcels with P. australis. The within-parcel models were excluded if they did not contain P. australis at any point between the first and last image. We used the change in area of P. australis on managed areas for each parcel between classification iterations as a response variable, the change in area of P. australis in tidal areas between classifications and parcel size as fixed effects, and year as a random effect.
For the neighboring parcel models, we included land parcels which directly shared a border with at least one other parcel that contained P. australis between the first and last image. Also, island parcels or those separated by sloughs or other sources of permanent water were not included. We used the change in the area of P. australis in managed areas for each parcel between classifications as a response variable. We included the change in area of P. australis in managed areas and tidal areas in neighboring parcels as fixed effects. The number of neighbors was also treated as a fixed effect, while year was considered a random effect. Neighbor statistics were calculated in ArcGIS Pro using the “Neighborhood Summary Statistics” tool.
Before running any models, we tested for collinearity between covariates using Pearson’s correlation test (Benesty et al., 2009; Sedgwick, 2012; Supplementary Table S2). After running our models, we employed a likelihood ratio test (package “lmtest”; Zeileis and Hothorn, 2002) to compare the full model with all covariates against a nested model from which each fixed effect was removed to identify the influence of individual covariates on P. australis expansion in managed areas. Models were processed using the “lme4” package (Bates et al., 2015).
3. Results
3.1. Random forest classifications
Random forest classifications of the yearly images included in the analysis yielded an average overall accuracy greater than 0.90 (Table 1). For P. australis, user’s accuracy was >0.90 for all years except for 2006 (0.85); producer’s accuracy was 0.90 for all years but 2003 (0.85) and 2006 (0.73). All classifications produced user’s and producer’s accuracies for non-Phragmites that was >0.90 and consistently >0.95 (Table 1).
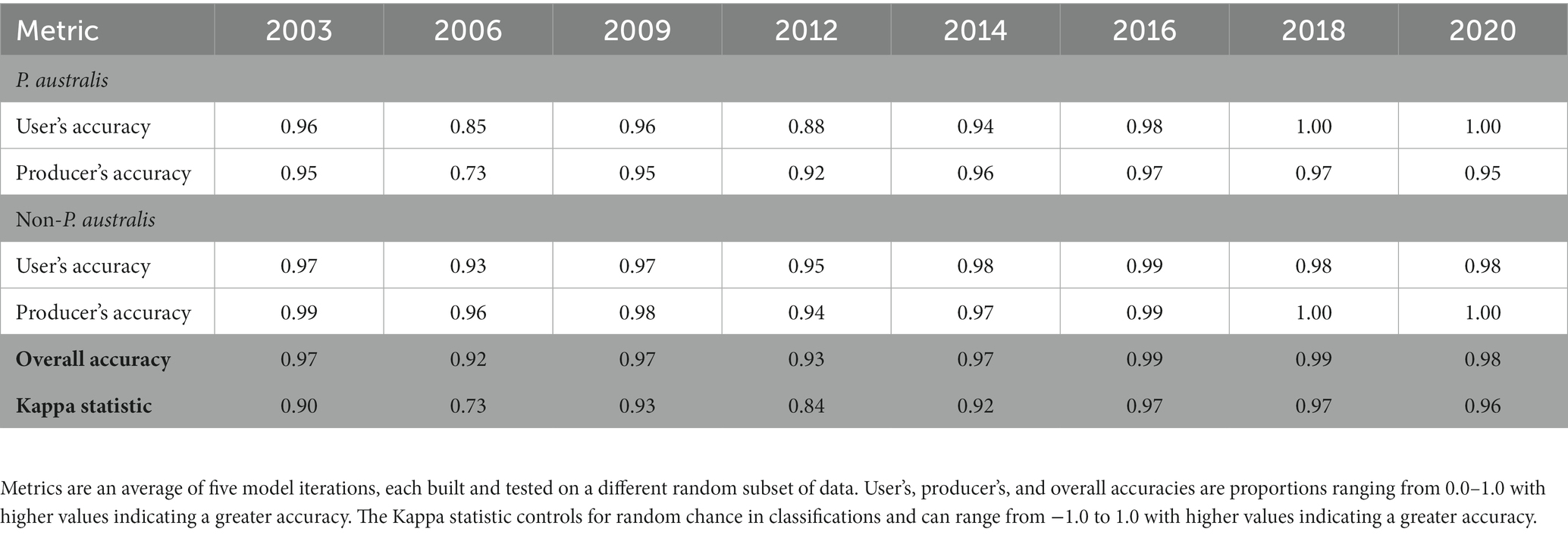
Table 1. Accuracy metrics for random forest classifications of Phragmites australis subsp. australis in Suisun Marsh.
3.2. Assessment of spread in Suisun Marsh
In 2003, 463 ha of Suisun Marsh were classified as P. australis, but by 2020, 980 ha were classified as P. australis (a 112% increase over 17 years; Figure 5). Phragmites australis peaked in 2018, with 1,547 ha classified as the invasive species (a 234% increase over 15 years; Figures 5, 6). The largest areal increase between classification years occurred between 2016 and 2018 (+392 ha); the largest percentage increase occurred between 2014 and 2016 (+35%). The areal extent of P. australis increased from the previous year except for in 2 cases: 2009 to 2012 (13% decrease) and 2018 to 2020 (37% decrease; Figure 5). With a total of about 20,560 ha of wetlands in Suisun Marsh, the 2020 classification indicated that 5% are covered by P. australis with a peak of 8% in 2018 compared with an initial estimate of 2% in 2003. We classified 271 ha of P. australis in managed wetlands and 191 ha in tidal wetlands in 2003 (Figure 6). At its peak in 2018, P. australis had expanded in managed wetlands by 721 ha (+266%), and by 364 ha in tidal wetlands (+190%).
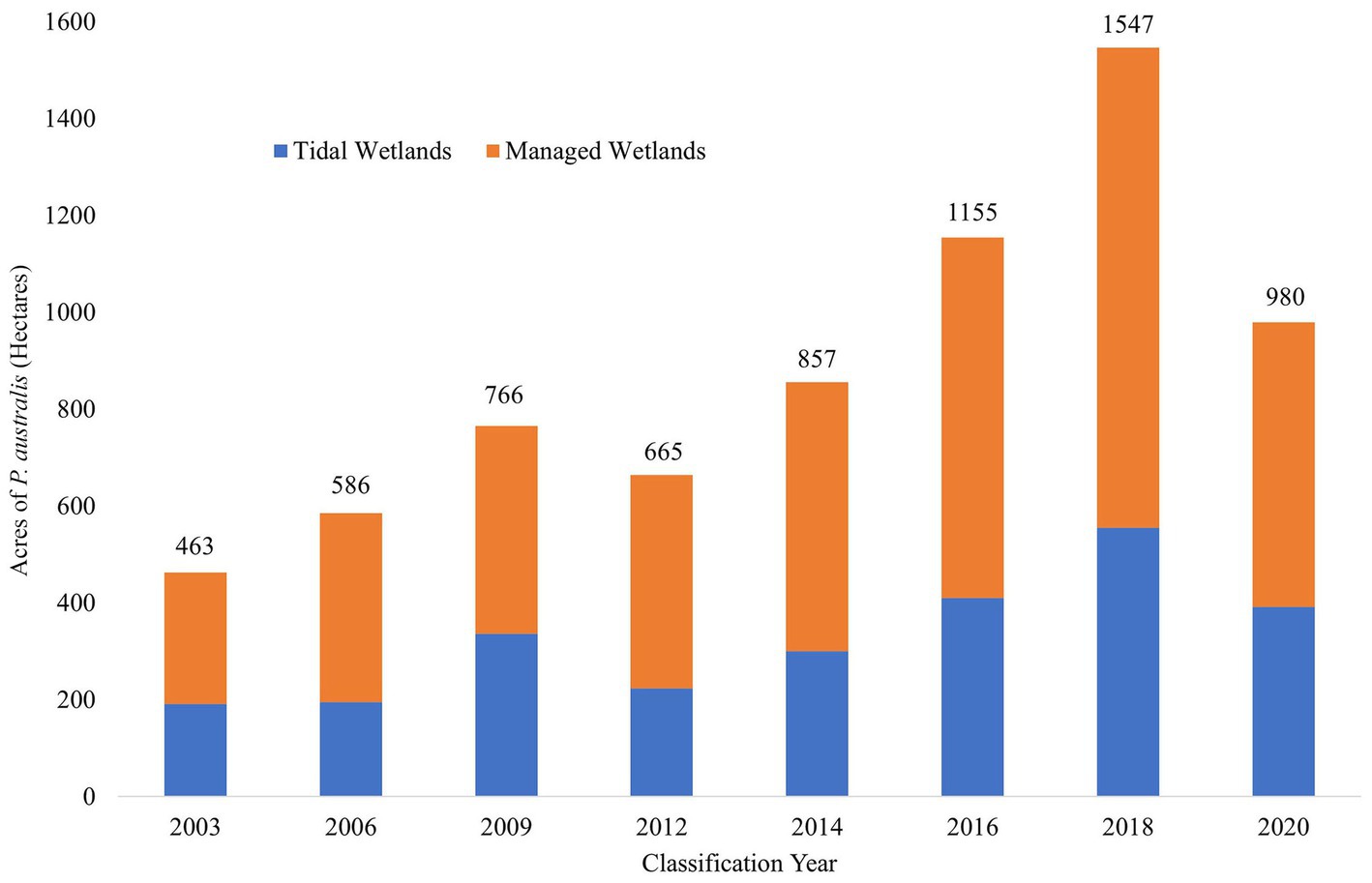
Figure 5. Estimated change in area of Phragmites australis subsp. australis between 2003 and 2020 as classified by random forest models. Columns are partitioned by wetland type: managed wetlands (orange) are surrounded by levees and have water control structures to regulate flooding and draining, while tidal wetlands (blue) are open to tidal waters which includes two mixed, daily semi-diurnal low and high tides. Managed wetlands are maintained during the summer (July–September) after wetlands are drained, while management of tidal wetlands are restricted and must be individually permitted to protect water quality and listed species. Labels above the bars indicate the total estimated area (in hectares) of P. australis in all wetlands for each classification year and show an increasing trend of P. australis over the past 2 decades.
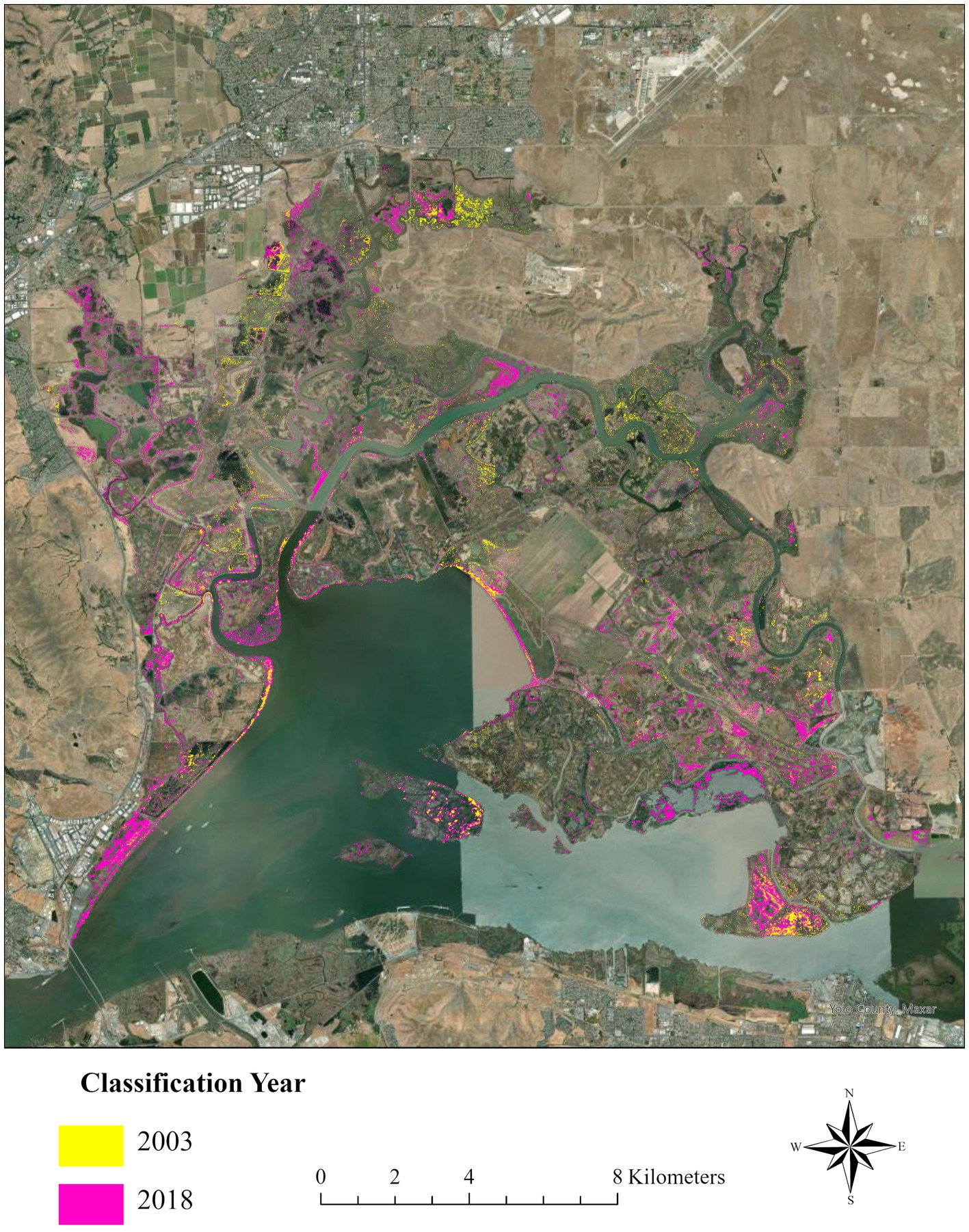
Figure 6. Expansion of Phragmites australis subsp. australis in Suisun Marsh, upper San Francisco estuary, northern California, United States from the first classification year (2003, lime green) to the most recent peak extent in 2018 (pink). We estimated 463 hectares of Phragmites in 2003, which expanded to a peak in 2018 (1,547 hectares; 234% increase).
The perimeter expansion of six representative P. australis patches averaged 1.32 m/year (± 0.53 SD) over 17 years. Expansion of patch perimeters ranged from a mean of 0.50 to 2.19 m/year Mean perimeter expansion was highest between 2012 and 2014 (2.19 ± 1.25 m/year) and lowest between 2018 and 2020 (0.61 ± 0.84 m/year; Figure 7). The largest expansion rate for a single P. australis patch between two classification years was 4.03 m/year (2012 to 2014); the lowest was −1.31 m/year (2010 to 2012; Figure 7).
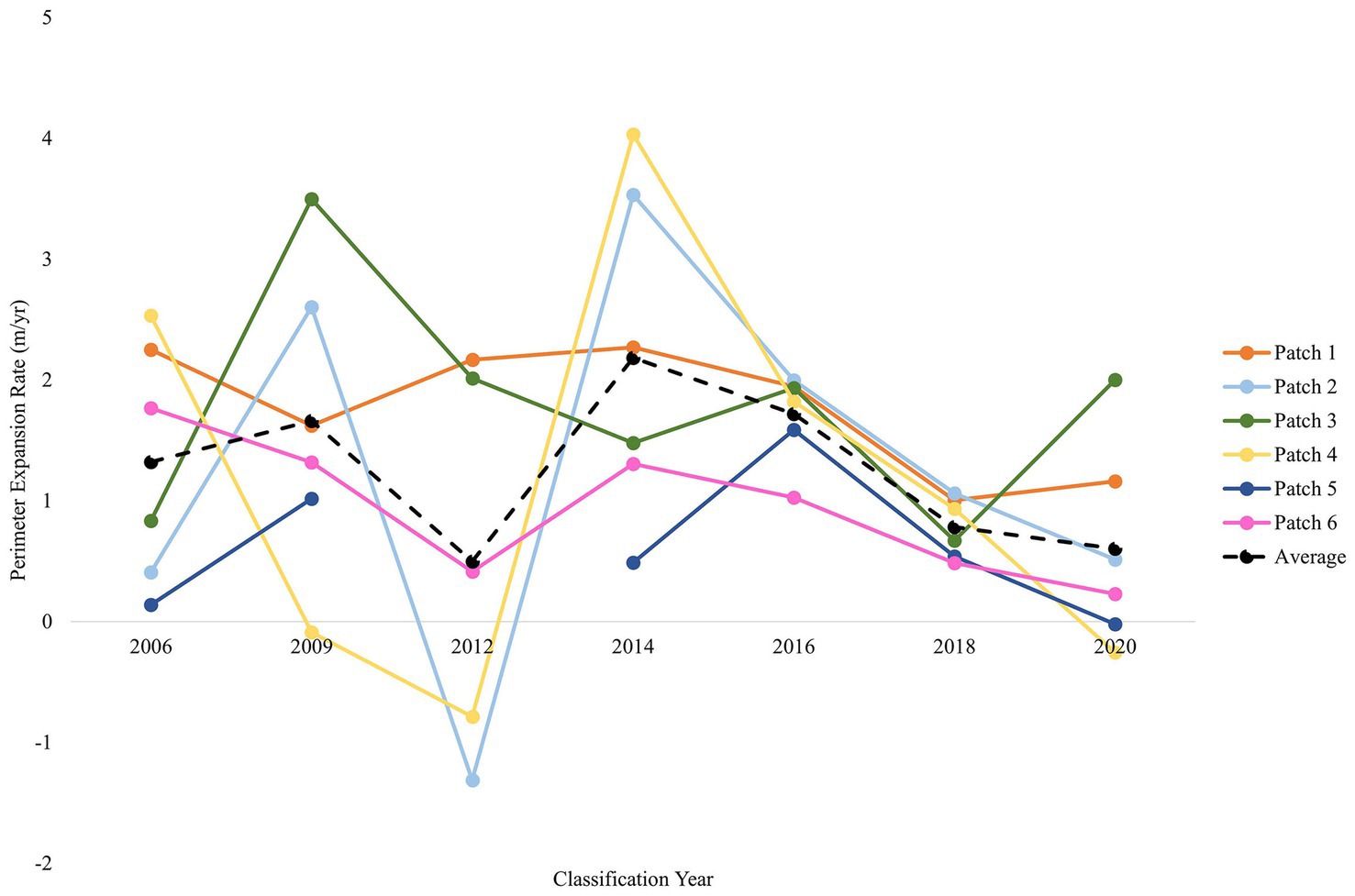
Figure 7. Perimeter expansion rates for six representative Phragmites australis subsp. australis patches distributed across Suisun Marsh shows consistent increases in the growth of patches. The perimeter expansion was calculated from 2006 to 2020 and indicated a consistent rate of linear increase that would result in an exponential increase in area. The average expansion rate of the six patches is displayed by a black dashed line. Data was unavailable for Patch 5 (dark blue) in 2012.
3.3. Comparison to VegCAMP manual classification
VegCAMP manual classifications yielded accuracy metrics >0.90 for all years except 2003 (Table 2). The percent overlap between VegCAMP and random forest classifications presented here consistently increased from a low of 19% in 2003 to a maximum of 79% in 2018 (Table 2). In 2003, VegCAMP analyzes estimated 314 ha of P. australis in Suisun Marsh while in 2018, VegCAMP analyzes estimated 1,739 ha (a 453% increase).
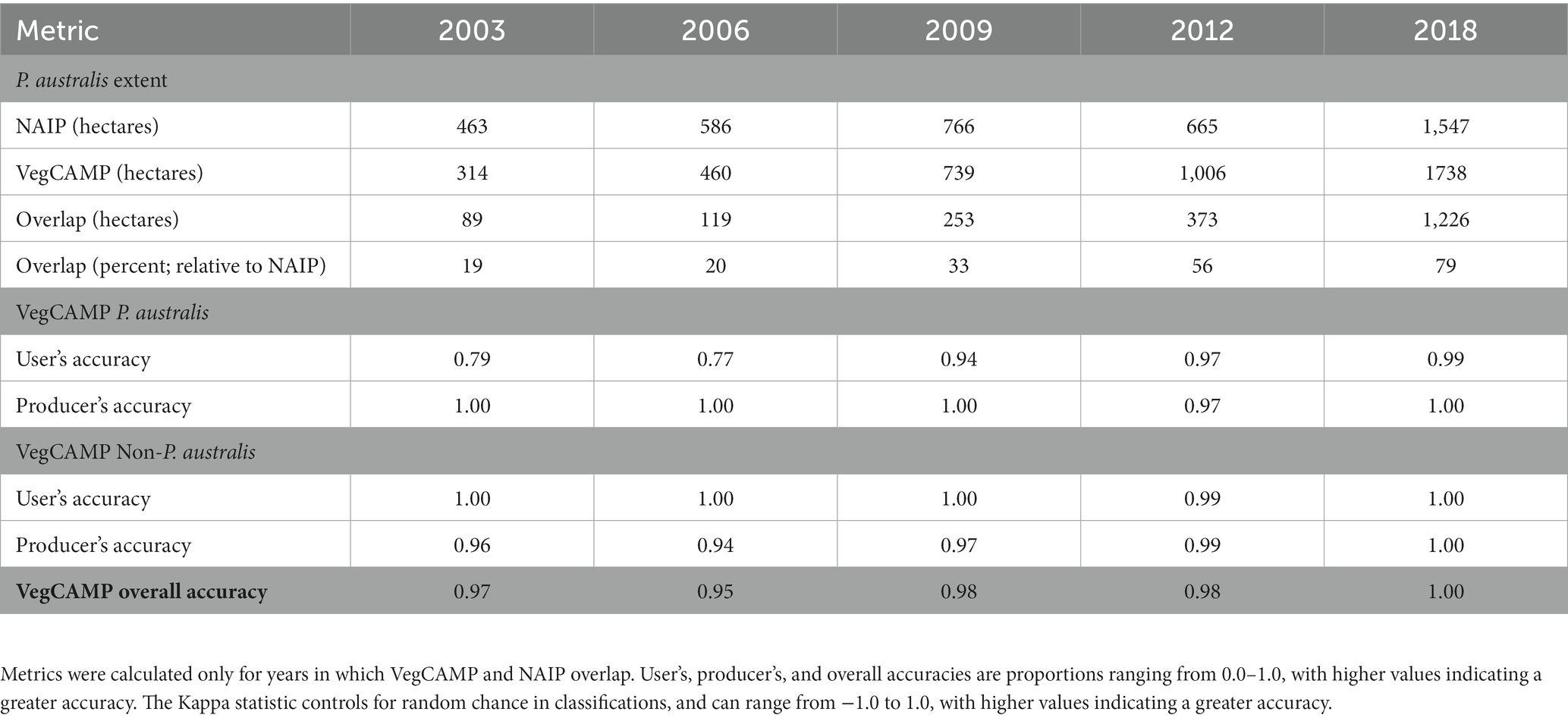
Table 2. Accuracy and comparison metrics for VegCAMP manual classifications of Phragmites australis subsp. australis.
3.4. Generalized linear mixed models
We analyzed 227 land parcels of varying size (137 ha ± 274) in Suisun Marsh, and of those, 186 had P. australis at some point during the study period. During this time frame, one third of parcels (61, 33%) showed a decrease in P. australis on their managed areas. For the within-parcel analysis, we included 167 parcels that possessed both managed and tidal areas within their boundaries. The change in area of P. australis in tidal areas within a parcel had a statistically significant effect (p < 0.01) on the change in P. australis in the managed areas of the same parcel. Change in area of P. australis increased with increasing parcel size, but the effect was not significant (p = 0.06; Table 3).
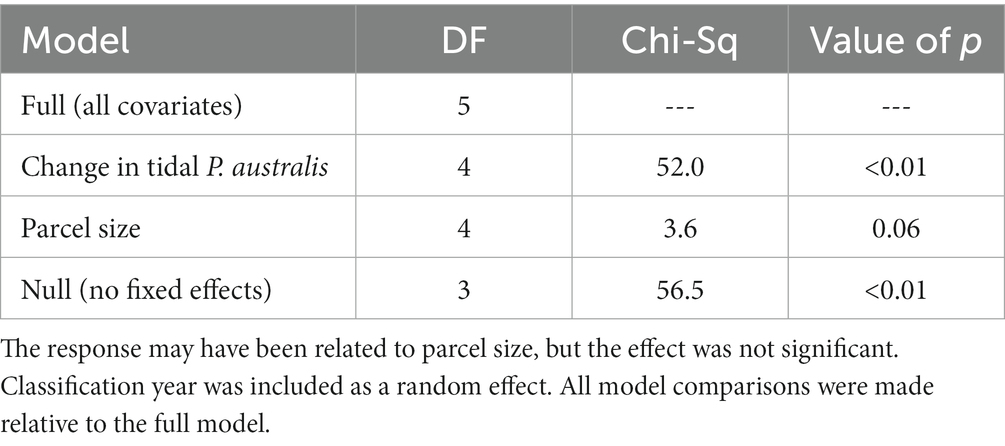
Table 3. Likelihood ratio tests indicated that increases in growth of Phragmites australis subsp. australis in managed areas were related to change in area of P. australis in tidal areas on the same land parcels between two subsequent iterations of our random forest classifications.
Our adjacent neighbor models included 178 parcels that shared a border with at least one other parcel and that had P. australis between 2003 and 2020. The number of adjacent neighbors ranged from 1 to 16, with a mean of 4.3 (± 2.2). Increasing P. australis in both tidal and managed wetlands of adjacent neighboring parcels had a significant effect associated with the change in area of P. australis in managed areas as did the number of adjacent neighbors (Table 4).
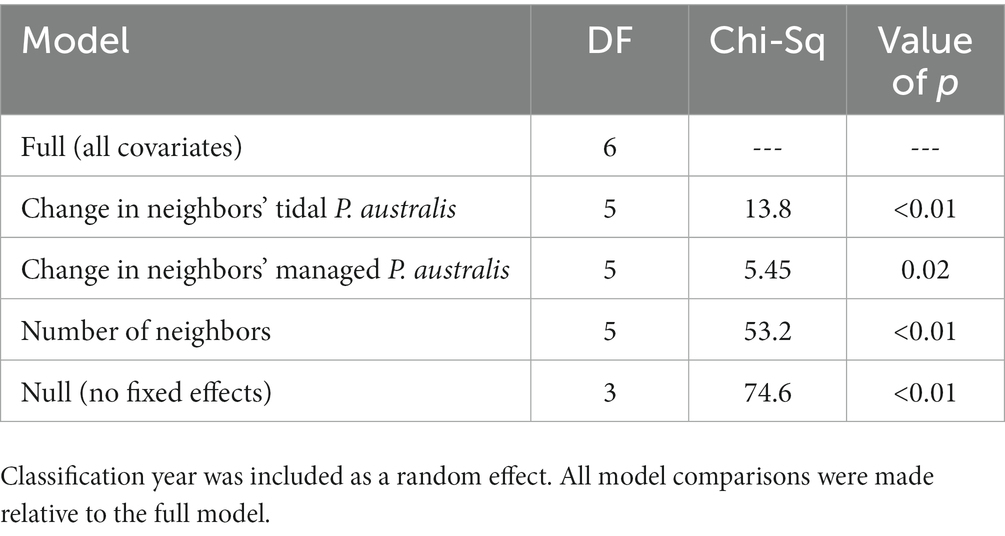
Table 4. Results of likelihood ratio tests assessing the effect of change in area of Phragmites australis subsp. australis in managed and tidal areas of adjacent neighboring land parcels on the change in area of P. australis in managed areas on a given parcel between two subsequent iterations of our random forest classifications.
4. Discussion
Understanding the historical expansion of P. australis as well as potential mechanisms that promote its growth may help landowners and conservation practitioners implement effective management plans to combat its continued spread (Meyerson et al., 2010a,b; Hazelton et al., 2014; Long et al., 2017b). We used publicly accessible NAIP imagery to create a simple, replicable, but highly accurate classification for P. australis to document the historical expansion of the invasion. Our results indicated that P. australis has expanded substantially in the region over the past two decades despite extensive management efforts to control it. We also found that P. australis expansion in managed wetland areas in Suisun Marsh may be related to the extent of invasion in tidal areas within the same parcel or in neighboring parcels of managed or tidal wetlands.
4.1. National Agriculture Imagery Program (NAIP) classifications and the expansion of Phragmites australis in Suisun Marsh
The results produced by our classification models highlighted the challenges of managing an invasive species over large temporal and geographic scales. Phragmites australis has increased substantially and consistently in Suisun Marsh over the past 2 decades, and the 234% increase in P. australis extent or 16%/year rate was greater or more rapid than results published from other ecosystems (Wilcox et al., 2003; Philipp and Field, 2005; Ji et al., 2009). In Lake Erie, a 152% increase in P. australis was reported between 1945 and 1999 (3%/year, Wilcox et al., 2003), and a 242% expansion was estimated in the Liahoe Delta of China between 1953 and 2006 (5%/year, Ji et al., 2009). Similarly, the perimeter expansion rate of P. australis in wetland ecosystems has varied considerably (Burdick et al., 2001; Philipp and Field, 2005; Fussell et al., 2015; Kettenring et al., 2016), but the mean perimeter expansion rate estimated here (1.32 m/year) was higher than most other estimates in the literature. For example, In the wetlands of Delaware and Maine, P. australis perimeter expansion was calculated at a mean of 0.91 and 0.70 m/yr (Philipp and Field, 2005; Fussell et al., 2015).
The patch expansion rate that we estimated combined with a larger and more rapid increase in total area suggests that our brackish study site may be particularly susceptible to the invasive P. australis growth and expansion. Previous studies have shown that the species is especially adept at invading disturbed areas, and its detrimental impact is aggravated by anthropogenic activities (Saltonstall, 2002; Mozdzer et al., 2013; Hazelton et al., 2014; Kettenring et al., 2015). Wide variation in annual precipitation in this Mediterranean climate region may likewise play a role in the P. australis expansion reported in this study. Average perimeter expansion of P. australis patches was highest in 2014 (2.19 m/yr) a drier period regionally, and lowest in 2012 (0.50 m/yr) during a comparatively wetter period (California Nevada River Forecast Center, National Ocean and Atmospheric Administration). Phragmites australis may therefore be able to withstand, if not thrive, in years of water deficit (Patger et al., 2005; Touchette et al., 2007), as it has been shown to rapidly expand in extreme low-water conditions elsewhere (Eller et al., 2017).
Other classification types, including object-based classification, texture analysis, and hyperspectral classification, have been used to model the distribution of P. australis (Arzandeh and Wang, 2003; Pengra et al., 2007; Samiappan et al., 2017; Abeysinghe et al., 2019). These previous efforts have consistently yielded accuracies over 0.80 but rely on more expensive methods of data collection (Abeysinghe et al., 2019). The accuracies produced from NAIP imagery were generally higher (> 0.90) and provide a more replicable and cost-effective option for wetland managers interested in monitoring P. australis distributions in the future (Walter and Mondal, 2023). Similarly, the use of publicly available imagery to create our classification will remove a barrier to continuous and long-term monitoring of the invasive species (Walter and Mondal, 2023). The ability to effectively monitor P. australis in the long term has shown to be critical in successfully managing the invasive species, but logistical and financial constraints are among the strongest deterrents to long-term monitoring (Hazelton et al., 2014; Long et al., 2017a; Quirion et al., 2018). Having a simple, replicable, and highly accurate classification model, is therefore valuable to ensuring future monitoring and successful control of P. australis. Managers interested in assessing the distribution or expansion of any invasive species should consider using publicly-available imagery like NAIP where applicable.
4.2. Remote sensing and manual classification accuracy: the potential for informing management
Our results on P. australis expansion generally agreed with results from the lengthy manual classification method conducted under VegCAMP. While VegCAMP may provide the accurate distribution of large P. australis patches, the size of the polygons analyzed limits its ability to identify small, emergent patches. This finding is further reinforced by the increased percent overlap between our random forest classifications and the VegCAMP analyzes. As P. australis patches become pervasive and more established, the overlap between the two classifications became larger (~80% in 2018).
In contrast to VegCAMP, our classifications built with remote sensing imagery can be generated in days, yield comparatively high accuracy metrics, and have the potential to effectively locate not only large patches but also emergent P. australis growth to support EDRR management (Huang and Asner, 2009; Bradley, 2014). The use of publicly available imagery also provides a much cheaper alternative to VegCAMP which costs 10 to over a hundred thousand dollars for each iteration (R. Klingonsmith, Department of Water Resources, pers. comm.). Finally, the rapid processing time of our classification allows better adaptive management to identify and target new invasions in real time (Huang and Asner, 2009; Bradley, 2014).
Despite the high accuracies produced by all of our classification iterations, certain shortcomings may be present. During its primary growth season, P. australis displays a distinct bright green signature and clusters of the invasive tend to form large, ovular patches (Kettenring et al., 2016). The classifications produced here were built upon these features; however, P. australis patches can demonstrate other visual characteristics. Before annual growth commences or toward the conclusion of its annual lifespan, P. australis patches may appear brown or beige (Figure 8). These brown marcescent patches can be difficult to distinguish and isolate given their visual similarity to a variety of other plants.
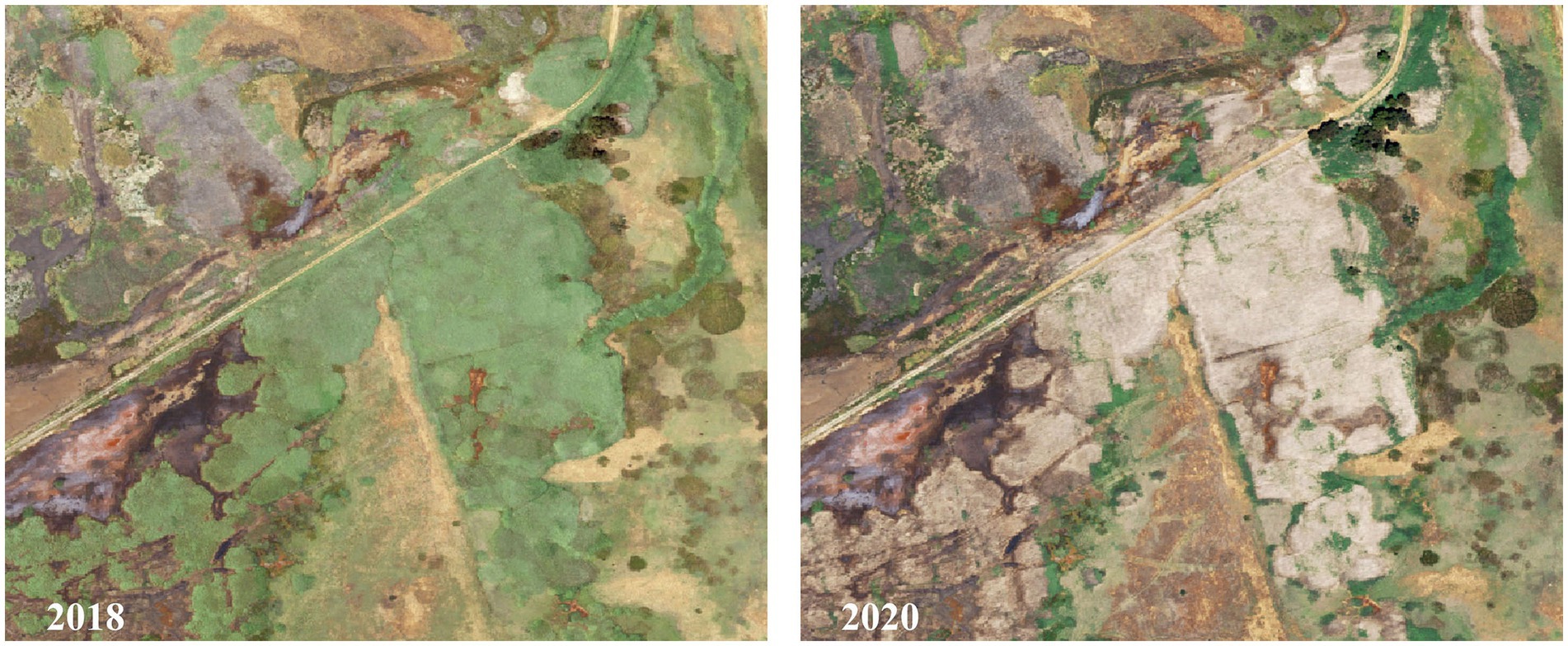
Figure 8. Effects of drought or seasonality on the visualization of Phragmites australis subsp. australis in Suisun Marsh, upper San Francisco estuary, northern California, United States. Vibrant, green patches of P. australis (2018) may appear as senesced and brown (2020) in drier years or earlier in the growing season. Our models were not trained to identify brown patches of P. australis.
Similarly, annual precipitation varies considerably in this system, and in drier years P. australis biomass may be less visibly apparent despite its continued presence. Phragmites australis in Suisun Marsh increased steadily from 2003 to 2018 before dropping almost 50% between 2018 and 2020. This dramatic decrease could be attributed to precipitation in the rainy season preceding the 2 years which affected their color rather than an actual reduction in P. australis. October 2016–April 2018 yielded 145.8 cm of rain, while October 2018–April 2020 yielded just 114.6 cm (California Nevada River Forecast Center, National Ocean and Atmospheric Administration).
Also, other variation among years could have affected the results of our classification. The 2018 NAIP imagery was collected in July (all other years were collected in May or June), and biomass of P. australis is likely greatest later in the summer (Rice et al., 2000; Engloner, 2009). Ideally, classifications targeting P. australis or plants which grow through the summer would use aerial imagery taken at the end of the peak growing season. In addition, P. australis can be affected by the annual management of water in managed wetlands (Rohal et al., 2019a). Year-to-year changes occur when landowners flood or drain their land parcels with leach cycles to reduce soil salinities on different schedules depending on the availability and water quality (salinity) of applied water. It may affect when and how long the P. australis growth season occurs and when P. australis is most visible from the air. Recognizing the limitations in classifying P. australis from aerial imagery is crucial for interpreting the distribution maps presented here and for creating management plans based on the findings.
The unique spectral signature of healthy P. australis allowed for the development of a highly successful classification based primarily on color attributes. Unfortunately, the historical nature of this study limited our ability to include ground-truthing data for the NAIP data. We encourage scientists and wetland managers to employ ground-truth data to support the remote-sensing classifications when possible. The manual selection of training and validation P. australis polygons with aerial images can introduce human error and biases, and while we are confident that the polygons selected for our classifications were P. australis, ground-truth data would confirm our assessment. With adequate planning, the polygons used for classification could be outlined in the field to provide a more accurate training and validation data set for models. Ground-truth data also is valuable to better identify very small and emergent patches of P. australis (<5 m2) that would benefit remote-sensing classifications (Huang and Asner, 2009; Bradley, 2014) and allow implementation of EDRR management practices. Ground-truth data are best collected in the field concurrently with aerial imagery flights to ensure that temporal mismatches in phenology are avoided.
4.3. Drivers of Phragmites australis expansion and management implications
Control of P. australis in Suisun Marsh has included ground and aerial herbicide spraying, burning, mowing, and disking (S. Chappell pers. comm.). While P. australis has spread dramatically over the past two decades despite these efforts, it is important to note that P. australis management has not been completely ineffective. Although we lack a database to indicate which specific wetland areas were treated, 61 land parcels showed a decrease in P. australis between 2003 and 2020 despite the 111.6% increase in P. australis overall. It is likely that without management efforts, the current distribution of P. australis would be much more expansive. Instead, our findings reinforce the difficulty in managing a pervasive and highly successful invasive species at a large scale (Kettenring et al., 2011; Hazelton et al., 2014) and the importance of consistent, multi-year treatment programs (Rohal et al., 2019a,b). Innovative solutions may be needed to help reduce the pervasive spread of P. australis. For example, advancements in drone technology have produced an effective alternative for treating plant invasions (Shahbazi et al., 2014; Roslim et al., 2021; Takekawa et al., 2023). Small survey drones can shoot high-resolution imagery of small-to-medium geographic areas relatively quickly (Koh and Wich, 2012; Cruzan et al., 2016), after which spray-drones can precisely target invasive plants to treat with herbicides (Martinez-Guanter et al., 2019; Takekawa et al., 2023).
In addition, alternative factors to individual management efforts may influence P. australis expansion. Natural processes, such as wildfire, flooding, and drought, may also influence P. australis distributions (Thompson and Shay, 1985; Patger et al., 2005; Touchette et al., 2007; Eller et al., 2017) in addition to limiting herbicide effectiveness (i.e., P. australis response to herbicide is diminished under drought conditions, Rohal et al., 2019a,b). Also, P. australis in Suisun Marsh may be developing a resistance to herbicide, which could contribute to its unrelenting spread. Little is known about P. australis and herbicide resistance (Wang et al., 2017; Blossey et al., 2020), but resistance has been demonstrated on a variety of other wetland or aquatic plants, such as Hydrilla verticillata and Agrostis stolonifera (Bollman et al., 2012; Simberloff and Leppanen, 2019). Such resistance can severely hinder management efforts by failing to kill invasive plants and creating even stronger and more pervasive strains (Peterson et al., 2018).
Our within-parcel model showed that the change in P. australis in managed areas was significantly correlated with the change in P. australis in tidal areas within the same parcel. Most managed areas are bordered by tidal areas on the exterior side of the levee; P. australis in these tidal areas are likely the primary source that spread into the managed wetlands. P. australis has been shown to spread 0.5–10 kilometers via seed transmission, and rhizome fragments can spread clonally via water drift (Fér and Hroudova, 2009; McCormick et al., 2016). Continuous monitoring of P. australis will help ensure curtail its spread, but long-term monitoring has been expensive and time-consuming (Hazelton et al., 2014; Long et al., 2017a; Quirion et al., 2018). Our classification method that uses NAIP imagery may help to reconcile these issues and provide a simpler way to promote long-term monitoring.
Varying management efforts between adjacent neighboring parcels has also inhibited the overall eradication of P. australis. Our results show that the change in P. australis over time on managed areas of a parcel was associated with the change in P. australis on the tidal and managed areas of neighboring parcels. The number of neighbors bordering a parcel was also a significant factor influencing P. australis growth. Our results highlighted the complexity of P. australis management in a diverse landscape (Epanchin-Niell et al., 2010). Co-management of invasive species has shown to be a crucial aspect of successful eradication of detrimental plants (Graham, 2019; Graham et al., 2019; Clarke et al., 2021), including P. australis management in other regions (Young and Kettenring, 2020). The different ownerships that comprise Suisun Marsh create a landscape in which collaboration is essential to protecting health of the overall ecosystem. There is a clear need for more research on the social aspects of P. australis management, including decision-making processes, in order to establish an adaptive and cooperative treatment strategy where neighboring parcels, as well as private and public entities, work together more closely to halt the spread of this disruptive invasive species (Young and Kettenring, 2020; Conrad et al., 2023). Conservation practitioners in similarly complex social-ecological landscapes will need to consider these factors when managing their invasive plants.
Data availability statement
The datasets presented in this study can be found in online repositories. The names of the repository/repositories and accession number(s) can be found at: https://drive.google.com/drive/folders/1mgzlguW8TYEFjExTUrbHVnln9_-euW75?usp=sharing.
Author contributions
JH compiled the data, conducted the statistical and spatial analyzes, and wrote the initial draft of the manuscript. JT conceived of the project, advised the analyzes, and contributed to the writing. SC contributed to developing the project and provided input to the writing. RT contributed to developing the project and writing and review. AE provided support for writing and review. KK contributed to project development and writing and review comments. All authors contributed to the article and approved the submitted version.
Funding
This project was supported by the Suisun Resource Conservation District (SRCD) with funding under a grant (DSC-21005) from the Delta Stewardship Council.
Acknowledgments
The authors appreciate the data and analysis assistance of J. Collins and T. Edmunds (SRCD) and C. Potter (Casa 2100). E. Fintel (CSU Chico) provided 2018 VegCamp analysis results, and M. Levin (Columbia University) offered valuable suggestions for the modeling process. The authors received helpful comments on the draft from Z. Ma and V. Tripuraneni (Purdue University), and V. Matzek (Santa Clara University).
Conflict of interest
The authors declare that the research was conducted in the absence of any commercial or financial relationships that could be construed as a potential conflict of interest.
Publisher’s note
All claims expressed in this article are solely those of the authors and do not necessarily represent those of their affiliated organizations, or those of the publisher, the editors and the reviewers. Any product that may be evaluated in this article, or claim that may be made by its manufacturer, is not guaranteed or endorsed by the publisher.
Supplementary material
The Supplementary material for this article can be found online at: https://www.frontiersin.org/articles/10.3389/fevo.2023.1171245/full#supplementary-material
References
Abeysinghe, T., Simic Milas, A., Arend, K., Hohman, B., Reil, P., Gregory, A., et al. (2019). Mapping invasive Phragmites australis in the old Woman Creek estuary using UAV remote sensing and machine learning classifiers. Remote Sens. 11:1380. doi: 10.3390/rs11111380
Andrew, M. E., and Ustin, S. L. (2009). Habitat suitability modelling of an invasive plant with advanced remote sensing data. Divers. Distrib. 15, 627–640. doi: 10.1111/j.1472-4642.2009.00568.x
Arnold, T. (1996). Suisun marsh history: Hunting and saving a wetland. Monterey Pacific Publishers. Marina, CA. 257.
Arzandeh, S., and Wang, J. (2003). Monitoring the change of Phragmites distribution using satellite data. Can. J. Remote. Sens. 29, 24–35. doi: 10.5589/m02-077
Askim, L., Fintel, E., Kreb, B., and Quigley, K.. (2022). 2018 vegetation map update for Suisun marsh Solano County, California. A report to the California Department of Water Resources. Prepared by Geographical Information Center California State University, Chico, p, 75.
Bates, D., Mächler, M., Bolker, B., and Walker, S. (2015). Fitting linear mixed-effects models using lme4. J. Stat. Softw. 67, 1–48. doi: 10.18637/jss.v067.i01
Battisti, C., Grosso, G., Ioni, S., Zullo, F., and Cerfolli, F. (2020). Response of specialized birds to reed-bed aging in a Mediterranean wetland: significant changes in bird biomass after two decades. Israel J. Ecol. Evol. 67, 17–22. doi: 10.1163/22244662-bja10007
Benesty, J., Chen, J., Huang, Y., and Cohen, I. (2009). “Pearson correlation coefficient” in Noise Reduction in Speech Processing (Berlin: Springer), 1–4.
Benoit, L. K., and Askins, R. A. (1999). Impact of the spread of Phragmites on the distribution of birds in Connecticut tidal marshes. Wetlands 19, 194–208. doi: 10.1007/BF03161749
Blossey, B., Endriss, S. B., Casagrande, R., Häfliger, P., Hinz, H., Dávalos, A., et al. (2020). When misconceptions impede best practices: evidence supports biological control of invasive Phragmites. Biol. Invasions 22, 873–883. doi: 10.1007/s10530-019-02166-8
Bollman, M. A., Storm, M. J., King, G. A., and Watrud, L. S. (2012). Wetland and riparian plant communities at risk of invasion by transgenic herbicide-resistant Agrostis spp. in Central Oregon. Plant Ecol. 213, 355–370. doi: 10.1007/s11258-011-0015-z
Bradley, B. A. (2014). Remote detection of invasive plants: a review of spectral, textural and phenological approaches. Biol. Invasions 16, 1411–1425. doi: 10.1007/s10530-013-0578-9
Burdick, D. M., Buchsbaum, R., and Holt, E. (2001). Variation in soil salinity associated with expansion of Phragmites australis in salt marshes. Environ. Exp. Bot. 46, 247–261. doi: 10.1016/S0098-8472(01)00099-5
CDFW. (2011). Suisun marsh habitat management, preservation, and restoration plan: Final environmental impact statement/environmental impact report. California Department of Fish and Wildlife, p. 176.
CDFW. (2023). Suisun Marsh Atlas. California Department of Fish and Wildlife. Available at: https://wildlife.ca.gov/Regions/3/Suisun-Marsh/Atlas. (Accessed January 26, 2023).
Chambers, R. M., Meyerson, L. A., and Saltonstall, K. (1999). Expansion of Phragmites australis into tidal wetlands of North America. Aquat. Bot. 64, 261–273. doi: 10.1016/S0304-3770(99)00055-8
Clarke, M., Ma, Z., Snyder, S. A., and Floress, K. (2021). Factors influencing family forest owners’ interest in community-led collective invasive plant management. Environ. Manag. 67, 1088–1099. doi: 10.1007/s00267-021-01454-1
Conrad, L. J., Thomas, M., Jetter, K., Madsen, J., Pratt, P., Moran, P., et al. (2023) Invasive aquatic vegetation in the Sacramento-san Joaquin Delta and Suisun marsh: The history and science of control efforts and recommendations for the path forward. San Francisco. San Francisco Estuary and Watershed Science.
Cruzan, M. B., Weinstein, B. G., Grasty, M. R., Kohrn, B. F., Hendrickson, E. C., Arredondo, T. M., et al. (2016). Small unmanned aerial vehicles (micro-UAVs, drones) in plant ecology. Appl Plant Sci 4:160041. doi: 10.3732/apps.1600041
Cutler, D. R., Edwards Jr, T. C., Beard, K. H., Cutler, A., Hess, K. T., Gibson, J., et al. (2007). Random forests for classification in ecology. Ecol. 88:2783–2792. doi: 10.1890/07-0539.1
Dibble, K. L., Pooler, P. S., and Meyerson, L. A. (2013). Impacts of plant invasions can be reversed through restoration: a regional meta-analysis of faunal communities. Biol. Invasions 15, 1725–1737. doi: 10.1007/s10530-012-0404-9
Eller, F., Skálová, H., Caplan, J. S., Bhattarai, G. P., Burger, M. K., Cronin, J. T., et al. (2017). Cosmopolitan species as models for ccophysiological responses to global change: the common reed Phragmites australis. Front. Plant Sci. 8:1833. doi: 10.3389/fpls.2017.01833
Engloner, A. I. (2009). Structure, growth dynamics and biomass of reed (Phragmites australis)–a review. Flora 204, 331–346. doi: 10.1016/j.flora.2008.05.001
Epanchin-Niell, R. S., Hufford, M. B., Aslan, C. E., Sexton, J. P., Port, J. D., and Waring, T. M. (2010). Controlling invasive species in complex social landscapes. Front. Ecol. Environ. 8, 210–216. doi: 10.1890/090029
Fér, T., and Hroudova, Z. (2009). Genetic diversity and dispersal of Phragmites australis in a small river system. Aquat. Bot. 90, 165–171. doi: 10.1016/j.aquabot.2008.09.001
Fielding, A. H., and Bell, J. F. (1997). A review of methods for the assessment of prediction errors in conservation presence/absence models. Environ. Conserv. 24, 38–49. doi: 10.1017/S0376892997000088
Fussell, S. B., Dionne, M. L., and Theodose, T. A. (2015). Expansion rates of Phragmites australis patches in a partially restored Maine salt marsh. Wetlands 35, 557–565. doi: 10.1007/s13157-015-0645-3
Graham, S. (2019). Coordinating invasive plant management among conservation and rural stakeholders. Land Use Policy 81, 247–255. doi: 10.1016/j.landusepol.2018.10.043
Graham, S., Metcalf, A. L., Gill, N., Niemiec, R., Moreno, C., Bach, T., et al. (2019). Opportunities for better use of collective action theory in research and governance for invasive species management. Conserv. Biol. 33, 275–287. doi: 10.1111/cobi.13266
Hazelton, E. L. G., Mozdzer, T. J., Burdick, D. M., Kettenring, K. M., and Whigham, D. F. (2014). Phragmites australis management in the United States: 40 years of methods and outcomes. AoB PLANTS 6:plu001. doi: 10.1093/aobpla/plu001
Hijmans, R. J., and Van Etten, J. (2012). Raster: Geographic Data Analysis and Modeling. Available at: http://CRAN.R-project.org/package=raster (Accessed May 31, 2022).
Holdredge, C., and Bertness, M. D. (2011). Litter legacy increases the competitive advantage of invasive Phragmites australis in New England wetlands. Biol. Invasions 13, 423–433. doi: 10.1007/s10530-010-9836-2
Huang, C., and Asner, G. P. (2009). Applications of remote sensing to alien invasive plant studies. Sensors 9, 4869–4889. doi: 10.3390/s90604869
Ji, Y., Zhou, G., Lv, G., Zhao, X., and Jia, Q. (2009). Expansion of Phragmites australis in the Liaohe Delta, north-East China. Weed Res. 49, 613–620. doi: 10.1111/j.1365-3180.2009.00727.x
Kettenring, K. M., de Blois, S., and Hauber, D. P. (2012). Moving from a regional to a continental perspective of Phragmites australis invasion in North America. AoB PLANTS 2012:pls040. doi: 10.1093/aobpla/pls040
Kettenring, K. M., McCormick, M. K., Baron, H. M., and Whigham, D. F. (2011). Mechanisms of Phragmites australis invasion: feedbacks among genetic diversity, nutrients, and sexual reproduction. J. Appl. Ecol. 48, 1305–1313. doi: 10.1111/j.1365-2664.2011.02024.x
Kettenring, K. M., and Mock, K. E. (2012). Genetic diversity, reproductive mode, and dispersal differ between the cryptic invader, Phragmites australis, and its native conspecific. Biol. Invasions 14, 2489–2504. doi: 10.1007/s10530-012-0246-5
Kettenring, K. M., Mock, K. E., Zaman, B., and McKee, M. (2016). Life on the edge: reproductive mode and rate of invasive Phragmites australis patch expansion. Biol. Invasions 18, 2475–2495. doi: 10.1007/s10530-016-1125-2
Kettenring, K. M., Whigham, D. F., Hazelton, E. L. G., Gallagher, S. K., and Baron, H. M. (2015). Biotic resistance, disturbance, and mode of colonization impact the invasion of a widespread, introduced wetland grass. Ecol. Appl. 25, 466–480. doi: 10.1890/14-0434.1
Koh, L. P., and Wich, S. A. (2012). Dawn of drone ecology: low-cost autonomous aerial vehicles for conservation. Trop. Conserv. Sci. 5, 121–132. doi: 10.1177/194008291200500202
Kraemer, H. C. (2015). “Kappa coefficient” in Wiley StatsRef: Statistics reference online (Hoboken, NJ: Wiley), 1–4. doi: 10.1002/9781118445112.stat00365.pub2
Lambert, A. M., Dudley, T. L., and Saltonstall, K. (2010). Ecology and impacts of the large-statured invasive grasses Arundo donax and Phragmites australis in North America. Invasive Plant Sci. Manage. 3, 489–494. doi: 10.1614/ipsm-d-10-00031.1
Lathrop, R. G., Windham, L., and Montesano, P. (2003). Does Phragmites expansion alter the structure and function of marsh landscapes? Patterns and processes revisited. Estuaries 26, 423–435. doi: 10.1007/BF02823719
Lombard, K. B., Tomassi, D., and Ebersole, J. (2012). Long-term management of an invasive plant: lessons from seven years of Phragmites australis control. Northeast. Nat. 19, 181–193. doi: 10.1656/045.019.s614
Long, A. L., Kettenring, K. M., Hawkins, C. P., and Neale, C. M. U. (2017b). Distribution and drivers of a widespread, invasive wetland grass, Phragmites australis, in wetlands of the great salt Lake, Utah, USA. Wetlands 37, 45–57. doi: 10.1007/s13157-016-0838-4
Long, A. L., Kettenring, K. M., and Toth, R. (2017a). Prioritizing management of the invasive grass common reed (Phragmites australis) in great salt Lake wetlands. Invasive Plant Sci. Manage. 10, 155–165. doi: 10.1017/inp.2017.20
Mack, R. N., Simberloff, D., Lonsdale, W. M., Evans, H., Clout, M., and Bazzaz, F. A. (2000). Biotic invasions: causes, epidemiology, global consequences, and control. Ecol. Appl. 10, 689–710. doi: 10.1890/1051-0761(2000)010[0689:BICEGC]2.0.CO;2
Martinez-Guanter, J., Agüera, P., Agüera, J., and Pérez-Ruiz, M. (2019). Spray and economics assessment of a UAV-based ultra-low-volume application in olive and citrus orchards. Precis. Agric. 21, 226–243. doi: 10.1007/s11119-019-09665-7
McCormick, M. K., Brooks, H. E., and Whigham, D. F. (2016). Microsatellite analysis to estimate realized dispersal distance in Phragmites australis. Biol. Invasions 18, 2497–2504. doi: 10.1007/s10530-016-1126-1
Meyerson, L. A., Lambert, A. M., and Saltonstall, K. (2010a). A tale of three lineages: expansion of common reed (Phragmites australis) in the U.S. southwest and Gulf Coast. Invasive Plant Sci. Manage. 3, 515–520. doi: 10.1614/ipsm-d-09-00052.1
Meyerson, L. A., Viola, D. V., and Brown, R. N. (2010b). Hybridization of invasive Phragmites australis with a native subspecies in North America. Biol. Invasions 12, 103–111. doi: 10.1007/s10530-009-9434-3
Minchinton, T., and Bertness, M. D. (2023). Disturbance-mediated competition and the spread of Phragmites australis in a coastal marsh. Ecol. Appl. 13, 1400–1416. doi: 10.1890/02-5136
Moyle, P. B., Manfree, A. D., and Fiedler, P. L. (2014). Suisun marsh: Ecological history and possible futures. Berkeley, CA: University of California Press.
Mozdzer, T. J., Brisson, J., and Hazelton, E. L. G. (2013). Physiological ecology and functional traits of north American native and Eurasian introduced Phragmites australis lineages. AoB Plants 5:plt048. doi: 10.1093/aobpla/plt048
Okoye, O. K., Li, H., and Gong, Z. (2020). Retraction of invasive Spartina alterniflora and its effect on the habitat loss of endangered migratory bird species and their decline in YNNR using remote sensing technology. Ecol. Evol. 10, 13810–13824. doi: 10.1002/ece3.6971
Orson, R. A. (1999). A paleoecological assessment of Phragmites australis in New England tidal marshes: changes in plant community structure during the last few millennia. Biol. Invasions 1, 149–158. doi: 10.1023/A:1010047731369
Pal, M. (2005). Random forest classifier for remote sensing classification. Int. J. Remote Sens. 26, 217–222. doi: 10.1080/01431160412331269698
Patger, M., Bragato, C., and Brix, H. (2005). Tolerance and physiological responses of Phragmites australis to water deficit. Aquat. Biol. 81, 285–299. doi: 10.1016/j.aquabot.2005.01.002
Paz-Kagan, T., Silver, M., Panov, N., and Karnieli, A. (2019). Multispectral approach for identifying invasive plant species based on flowering phenology characteristics. Remote Sens. 11:953. doi: 10.3390/rs11080953
Pengra, B. W., Johnston, C. A., and Loveland, T. R. (2007). Mapping an invasive plant, Phragmites australis, in coastal wetlands using the EO-1 Hyperion hyperspectral sensor. Remote Sens. Environ. 108, 74–81. doi: 10.1016/j.rse.2006.11.002
Peterson, M. A., Collavo, A., Ovejero, R., Shivrain, V., and Walsh, M. J. (2018). The challenge of herbicide resistance around the world: a current summary. Pest Manag. Sci. 74, 2246–2259. doi: 10.1002/ps.4821
Philipp, K. R., and Field, R. T. (2005). Phragmites australis expansion in Delaware Bay salt marshes. Ecol. Eng. 25, 275–291. doi: 10.1016/j.ecoleng.2005.04.008
Pimentel, D., Lach, L., Zuniga, R., and Morrison, D. (2000). Environmental and Economic Costs of Nonindigenous Species in the United States. Bioscience 50, 53–65. doi: 10.1641/0006-3568(2000)050[0053:EAECON]2.3.CO;2
Poulin, B., Lefebvre, G., and Mauchamp, A. (2002). Habitat requirements of passerines and reedbed management in southern France. Biol. Conserv. 107, 315–325. doi: 10.1016/S0006-3207(02)00070-8
Quirion, B., Simek, Z., Dávalos, A., and Blossey, B. (2018). Management of invasive Phragmites australis in the Adirondacks: a cautionary tale about prospects of eradication. Biol. Invasions 20, 59–73. doi: 10.1007/s10530-017-1513-2
R Core Team. (2021). R: A language and environment for statistical computing. R Foundation for Statistical Computing, Vienna, Austria. Available at: https://www.R-project.org/.
R Studio Team (2021). RStudio: Integrated development for R. RStudio, PBC, Boston, MA. Available at: http://www.rstudio.com/.
Reaser, J. K., Burgiel, S. W., Kirkey, J., Brantley, K. A., Veatch, S. D., and Burgos-Rodriguez, J. (2020). The early detection and rapid response (EDRR) to invasive species: a conceptual and federal capacities assessment. Biol. Invasions 22, 1–19. doi: 10.1007/s10530-019-02156-w
Rice, D., Rooth, J., and Stevenson, J. (2000). Colonization and expansion of Phragmites australis in upper Chesapeake Bay tidal marshes. Wetlands 20, 280–299. doi: 10.1672/0277-5212(2000)020[0280:CAEOPA]2.0.CO;2
Robichaud, C. D., and Rooney, R. C. (2017). Long-term effects of a Phragmites australis invasion on birds in a Lake Erie coastal marsh. J. Great Lakes Res. 43, 141–149. doi: 10.1016/j.jglr.2017.03.018
Rohal, C., Cranney, C., Hazelton, E., and Kettenring, K. M. (2019b). Invasive Phragmites australis management outcomes and native plant recovery are context dependent. Ecol. Evol. 9, 13835–13849. doi: 10.1002/ece3.5820
Rohal, C., Cranney, C., and Kettenring, K. M. (2019a). Abiotic and landscape factors constrain restoration outcomes across spatial scales of a widespread invasive plant. Front. Plant Sci. 10, 1–17. doi: 10.3389/fpls.2019.00481
Rohal, C. B., Hazelton, E. L. G., McFarland, E., Downard, R., McCormick, M., Whigham, D., et al. (2023). Landscape and site factors drive invasive Phragmites management and native plant recovery across Chesapeake Bay wetlands. Ecosphere 14:e4392. doi: 10.1002/ecs2.4392
Roslim, M. H. M., Juraimi, A. S., Che’Ya, N. N., Sulaiman, N., Manaf, M. N. H., Ramli, Z., et al. (2021). Using remote sensing and an unmanned aerial system for weed management in agricultural crops: a review. Agronomy 11, 1809–1824. doi: 10.3390/agronomy11091809
Royimani, L., Mutanga, O., Odindi, J., Dube, T., and Matongera, T. N. (2019). Advancements in satellite remote sensing for mapping and monitoring of alien invasive plant species (AIPs). Phys. Chem. Earth Parts A/B/C 112, 237–245. doi: 10.1016/j.pce.2018.12.004
Saltonstall, K.. (2002). Cryptic invasion by a non-native genotype of the common reed, Phragmites australis, into North America. Available at: https://www.pnas.org.
Samiappan, S., Turnage, G., Hathcock, L., Casagrande, L., Stinson, P., and Moorhead, R. (2017). Using unmanned aerial vehicles for high-resolution remote sensing to map invasive Phragmites australis in coastal wetlands. Int. J. Remote Sens. 38, 2199–2217. doi: 10.1080/01431161.2016.1239288
Shahbazi, M., Théau, J., and Ménard, P. (2014). Recent applications of unmanned aerial imagery in natural resource management. GISci. Remote Sens. 51, 339–365. doi: 10.1080/15481603.2014.926650
Simberloff, D., and Leppanen, C. (2019). Plant somatic mutations in nature conferring insect and herbicide resistance. Pest Manag. Sci. 75, 14–17. doi: 10.1002/ps.5157
Simpson, S., and Baruth, K. H.. (1966). Suisun soil Conservation District final report phase I. San Francisco, California.
Tadros, M. J., Al-Assaf, A., Othman, Y. A., Makhamreh, Z., and Taifour, H. (2020). Evaluating the effect of Prosopis juliflora, an alien invasive species, on land cover change using remote sensing approach. Sustainability 12:5887. doi: 10.3390/SU12155887
Takekawa, J. Y., Hagani, J. S., Edmunds, T. J., Collins, J. M., Chappell, S. C., Reynolds, W. H., et al. (2023). The sky is not the limit: use of a spray drone to surgically apply herbicide and control an invasive plant in managed wetlands. In prep.
Takekawa, J. Y., Woo, I., Spautz, H., Nur, N., Grenier, J. L., Malamud-Roam, K., et al. (2006). Environmental threats to tidal-marsh vertebrates of the San Francisco Bay estuary. Stud. Avian Biol. 32:176.
Tallamy, D. W. (2004). Do alien plants reduce insect biomass? Conserv. Biol. 18, 1689–1692. doi: 10.1111/j.1523-1739.2004.00512.x
Thompson, D. J., and Shay, J. M. (1985). The effects of fire on Phragmites australis in the Delta marsh, Manitoba. Can. J. Bot. 63, 1864–1869. doi: 10.1139/b85-261
Thorne, K., MacDonald, G., Guntenspergen, G., Ambrose, R., Buffington, K., Dugger, B., et al. (2018). US Pacific coastal wetland resilience and vulnerability to sea-level rise. Sci. Adv. 4:eaao3270. doi: 10.1126/sciadv.aao3270
Touchette, B. W., Iannacone, L. R., Turner, G. E., and Frank, A. R. (2007). Drought tolerance versus drought avoidance: a comparison of plant-water relations in herbaceous wetland plants subjected to water withdrawal and repletion. Wetlands 27, 656–667. doi: 10.1672/0277-5212(2007)27[656:DTVDAA]2.0.CO;2
Tozer, D. C., and Mackenzie, S. A. (2019). Control of invasive Phragmites increases marsh birds but not frogs. Can. Wildl. Biol. Manage. 8, 66–82.
Vitousek, P. M., D’antonio, C. M., Loope, L. L., Rejmánek, M., and Westbrooks, R. (1997). Introduced species: a significant component of human-caused global change. Available at: https://www.jstor.org/stable/24054520.
Volesky, J. D., Young, S. L., and Jenkins, K. H. (2017). Cattle grazing effects on Phragmites australis in Nebraska. Invasive Plant Sci. Manage. 9, 121–127. doi: 10.1614/IPSM-D-15-00056.1
Wails, C. N., Baker, K., Blackburn, R., Del Vallé, A., Heise, J., Herakovich, H., et al. (2021). Assessing changes to ecosystem structure and function following invasion by Spartina alterniflora and Phragmites australis: a meta-analysis. Biol. Invasions 23, 2695–2709. doi: 10.1007/s10530-021-02540-5
Walter, M., and Mondal, P. (2023). Mapping of Phragmites in estuarine wetlands using high-resolution aerial imagery. Environ. Monit. Assess. 195:478. doi: 10.1007/s10661-023-11071-6
Wang, Q., Li, C., Chen, C., Chen, J., Ma, X., and Que, X. (2017). Physiological responses of Phragmites australis to atrazine exposure and their relevance for tolerance. J. Agroenviron. Sci. 36, 1968–1977.
Wilcox, K. L., Petrie, S. A., Maynard, L. A., and Meyer, S. W. (2003). Historical distribution and abundance of Phragmites australis at long point, Lake Erie, Ontario. J. Great Lakes Res. 29, 664–680. doi: 10.1016/S0380-1330(03)70469-9
Young, S. L., and Kettenring, K. M. (2020). The social-ecological system driving effective invasive plant management: two case studies of non-native Phragmites. J. Environ. Manag. 267:110612. doi: 10.1016/j.jenvman.2020.110612
Keywords: Phragmites australis , invasive species, marsh, estuary, random forest classification, wetland
Citation: Hagani JS, Takekawa JY, Chappell SC, Tanner RL, Ernst AR and Kettenring KM (2023) A remote sensing approach to assess the historical invasion of Phragmites australis in a brackish coastal marsh. Front. Ecol. Evol. 11:1171245. doi: 10.3389/fevo.2023.1171245
Edited by:
Yanjie Xu, Finnish Museum of Natural History, FinlandReviewed by:
Corrado Battisti, Roma Tre University, ItalySuresh Chandra Subedi, Arkansas Tech University, United States
Copyright © 2023 Hagani, Takekawa, Chappell, Tanner, Ernst and Kettenring. This is an open-access article distributed under the terms of the Creative Commons Attribution License (CC BY). The use, distribution or reproduction in other forums is permitted, provided the original author(s) and the copyright owner(s) are credited and that the original publication in this journal is cited, in accordance with accepted academic practice. No use, distribution or reproduction is permitted which does not comply with these terms.
*Correspondence: Jason S. Hagani, jhagani@suisunrcd.org