- 1School of Information Engineering, China University of Geosciences, Beijing, China
- 2Technology, Innovation Center for Territory Spatial Big-Data, Ministry of Natural Resources of China, Beijing, China
- 3Beijing Fangshan Observation and Research Station of Comprehensive Exploration Technology, Ministry of Natural Resources of China, Beijing, China
- 4School of Public Administration and Policy, Renmin University of China, Beijing, China
- 5School of Economics and Finance, Huaqiao University, Quanzhou, China
With the escalating impacts of global climate change, carbon peaking and carbon neutrality have become pressing issues of mutual concern for nations worldwide. Accurately measuring carbon emissions at regional and municipal levels in order to design differentiated reduction policies and promote carbon neutrality has become a challenging problem. To tackle this challenge, we developed a 250 m resolution grid map of carbon footprint data and constructed a national-level database (comprised of 2,800 county-level units) to analyze China’s carbon footprint from multiple angles such as overall characteristics, urban agglomerations, county-level units, population density, per capita disposable income, and more. This research provides fresh insights for studying multi-scale footprint spatial pattern dynamics. Our findings indicate that (1) The 250 m resolution Carbon Footprint Database developed in this study covers the national, provincial, county, urban, and rural areas, enabling dynamic research on multi-scale footprint spatial patterns; (2) The top 5% of areas with the highest carbon footprints account for approximately 1/5 of total carbon footprints in China, and approximately 1/4 of areas account for more than 50% of total carbon footprints; (3) The potential for reducing carbon emissions in China’s rural areas has been underestimated by over 10%, while those in the western regions are growing rapidly due to poverty alleviation efforts; (4) An inverted U-shaped “environmental Kuznets curve” emerges between disposable income and emissions intensity, with different regions exhibiting different consumption patterns. This study offers a novel approach for formulating reduction policies at different levels throughout the country.
1. Introduction
The increasing frequency of natural disasters and extreme weather events has brought greater attention to climate change on a global scale. Although the UNFCCC, Kyoto Protocol, and Paris Agreement adhere to the principle of “common but differentiated responsibilities,” they overlook the historical emissions, responsibility for consumption-based emissions, and cross-border emissions flows (Mi et al., 2017; Heinonen et al., 2020). This has led to a significant overestimation of China’s carbon emissions, thereby imposing strict limits on China’s future carbon emissions. Nevertheless, China remains steadfast in implementing robust emission reduction measures to address climate change, with clearly defined targets of peaking carbon emissions by 2030 and achieving carbon neutrality by 2060 (Kanemoto et al., 2016; The State Council of China, 2022).
Although China is often regarded as a homogenous entity in the field of climate change research, it is a vast country with significant regional differences in economic development, resource endowments, population, and lifestyles. Therefore, it is crucial to target key emission reduction areas and achieve precise emissions reduction (Cai et al., 2018a; Mi et al., 2021). As the final destination of production activities, household consumption plays a crucial role in reducing carbon emissions and implementing differentiated mitigation policies (Liu et al., 2019; Mi et al., 2020). Globally (Hertwich and Peters, 2009), approximately 72% of carbon emissions are driven by household final consumption. The United States has the highest rate of household carbon emissions, accounting for over 80% (Jones et al., 2014; Mi et al., 2020), followed by the United Kingdom at 74% (Minx et al., 2013), South Korea at 52% (Park and Heo, 2007), Japan at 60% (Mi et al., 2016), and China at 30–40%, with an annual growth rate of 8.7% (Fan et al., 2013; Kanemoto et al., 2016; Zhang and Wang, 2017). With the acceleration of Chinese household income growth and the expansion of the middle-income group, household consumption contributed approximately 76.2% to GDP growth in 2018 (National Bureau of Statistics of China, 2022). Carbon footprint can be used to quantify and compare the contribution of human activities to global warming, and has the potential to prevent carbon leakage and share emission responsibilities more equitably. It has become a popular method in spatial carbon accounting (Pang et al., 2019; Heinonen et al., 2020). Consumption-based methods are suitable for different geographical ranges (such as countries, states, cities, and communities), linking commodity and service consumption to their associated embodied emissions. Therefore, input–output analysis is commonly used for evaluation. Carbon footprints are typically divided into area carbon footprints (ACF) and personal carbon footprints (PCF). ACF covers all consumption-based emissions resulting from economic activities within the study region, regardless of who caused them, while PCF covers all consumption-based emissions caused by residents within the study region, regardless of the source of emissions (Chen S. et al., 2020).
Carbon footprint assessments at different scales reveal significant potential differences between consumption and emission patterns, which have been a focal point of low-carbon emission reduction discussions in various countries and regions (Ottelin et al., 2019). Firstly, the Intergovernmental Panel on Climate Change (IPCC) rejects one-size-fits-all solutions to address carbon emissions and emphasizes the importance of regional differences (Ivanova et al., 2017). Secondly, the gap between urban and rural areas has always been a major challenge for implementing national carbon reduction plans. Existing research mainly focuses on mega-cities, regional cities, and urban areas, with little knowledge about emissions in rural areas (Wiedenhofer et al., 2017; Qian et al., 2022). Due to economic inequality, the per capita CO2 emissions of urban households in China are nearly two to three times higher than those of rural households (Hubacek et al., 2017; Shao et al., 2018). Finally, there are significant differences in emissions between different income levels. Globally, 36–45% of global emissions can be attributed to the top 10% of emitters (Ivanova et al., 2020). Therefore, quantifying household consumption carbon emissions is crucial for formulating effective and precise emission reduction policies. Calculating consumer carbon emissions is mainly based on macro and micro data and Environmental Extended Multi-Regional Input–Output Analysis (EEMRIO). which has been widely used to estimate air pollution (Yang et al., 2016), carbon emissions (Ma et al., 2022), land use (Zhao et al., 2020), biodiversity (Lenzen et al., 2012), and environmental impacts (Tukker et al., 2016) from consumption trade.
The high spatial resolution characterization of carbon emissions and their spatial heterogeneity is a crucial and topical issue in global and regional CO2 emission research. In recent years, research on carbon emissions has increasingly focused on smaller scales and more refined contents (Zhang et al., 2014; Ottelin et al., 2019). From the perspective of administrative divisions, due to data limitations, the estimated scales are mostly national (Feng et al., 2021), regional (Yang and Yang, 2020), provincial (Liu et al., 2022), and key cities (Bai et al., 2021). From the grid scale, the resolution of global carbon emissions has ranged from 5° × 5° (Ghaemi and Smith, 2020) to 1° × 1° (Cai et al., 2018b) to 0.1° × 0.1° (Fan et al., 2018), even reaching 250 m (Moran et al., 2018). Correspondingly, the spatial resolution of China’s carbon emissions has been increased from 25 km × 25 km (Liu et al., 2013) to 10 km × 10 km (Cai et al., 2018b) and has been increased to 1 km × 1 km (Gurney et al., 2019; Long et al., 2021) in some regional studies. These studies are mostly based on energy statistical data, combined with population distribution, land use, commercial and industrial point source data, and road networks, and their results play a significant role in global, regional, national, and city carbon emission research. However, due to the lack of statistical data, it has become challenging to estimate carbon emissions at the sub-provincial and grid scales that characterize specific geographical information. On the other hand, carbon emission statistics are currently at the administrative unit level, lacking regional spatial heterogeneity information, making it difficult to conduct in-depth research on international hot issues such as spatial correlations, spatial clustering features, and carbon emission differences in different regions.
Therefore, there is an urgent need for more accurate spatial explicit data to estimate carbon emissions at a finer scale. Spatial explicit data based on population data can provide strong data support for fine-scale analysis of carbon emissions in regions such as the global (Moran et al., 2018), the United States (Jones et al., 2014), Germany (Gill and Moeller, 2018), and other regions.
Therefore, creating high spatial resolution carbon emission grid maps is essential for understanding the spatial differences and details of carbon emissions at the county and urban–rural levels. Through governance at different scales, more appropriate carbon reduction responsibilities can be allocated to the overall reduction of carbon emissions, avoiding the situation of “local carbon reduction, overall increase” (Li et al., 2019).
To address the research gap in this field, we have designed an integrated model to create a high spatial resolution grid of consumption-based carbon footprint data for China. By linking detailed data at four levels of administration (national, provincial, county, and urban/rural) with an environmentally extended multi-regional input–output model, we have generated a new spatially explicit map of carbon footprint hotspots and produced maps of per capita carbon footprints at the county and urban/rural levels. We have also investigated the relationship between population density, disposable income, and carbon footprints, and expect to find a positive slope. This hypothesis is based on the fact that in China, areas with higher population density tend to have higher income and carbon footprints. This research will enrich and improve the fundamental understanding of the spatial patterns of fine-scale carbon footprint in China.
2. Data and method
2.1. Research data
Multi-regional input–output model (MRIO). The input–output tables for 30 provinces in China for the years 2015 and 2017 were obtained from the China Emission Accounts and Dataset (CEADs). representing the latest available multi-regional input–output tables. Due to data unavailability, the Tibet, Hong Kong, Macao, and Taiwan regions were excluded from the analysis. In order to correspond to household consumption categories, the 42 production sectors in the input–output tables were aggregated into 8 categories, as shown in Table 1. This analysis focuses solely on household emissions and excludes consumption by non-profit organizations, government consumption, and capital formation.
CO2 emissions data. The county-level energy-related carbon emissions data in China in 2015 and 2017 were obtained from CEADs (Chen J. et al., 2020), which is currently the county-level carbon dioxide emission data with the longest time span and the widest coverage.
The data on disposable income for urban and rural residents is sourced from various outlets, including the statistical yearbooks of 30 provinces, district and county government websites, and statistical bulletins. In 2015, there were 2,783 county-level regions, which increased to 2,794 in 2017. Income is a crucial factor in determining consumer spending and is frequently used to gauge the standard of living in a given region (Liu et al., 2018).
The high spatial resolution population grid data comes from WorldPop Population Counts UN adjusted (100 m resolution).1
The urban built-up area data was obtained from the Global Artificial Impervious Area (GAIA) data product developed by Tsinghua University, which covers the period from 1985 to 2018 with a resolution of 30 m (Gong et al., 2020), This dataset represents the longest time-series of artificial impervious surface data in the world to date and can be accessed at http://data.ess.tsinghua.edu.cn/.
According to the “Classification of Individual Consumption by Purpose (2013)” released by the National Bureau of Statistics of China, household consumption can be categorized into eight groups, namely, food, clothing, housing, household facilities and equipment, transportation and communication, education, culture and entertainment, healthcare and medical services, and miscellaneous goods and services. Correspondingly, the household carbon footprint estimated by the MRIO model is classified into eight primary consumption categories: food, clothing, housing, household facilities, transportation, education, healthcare, and other. This excludes the direct energy use emissions from rural and urban households, as the input–output model only estimates the carbon emissions indirectly emitted by economic production sectors. In this study, the targeted energy-related emissions from direct coal, natural gas, and electricity consumption by households are classified under the “housing” category, while petroleum-related emissions are allocated to the “transportation” category and included in the household carbon footprint.
2.2. Fusion model based on consumption carbon emission estimation
To effectively estimate China’s consumption CF, it is necessary to construct a set of quantitative methods for rapid and accurate estimation of national, provincial, county, and grid-level scales. However, because the primary input–output data only provide information at the national level, the current research scale is single, and it is impossible to carry out refined spatial analysis in multiple dimensions. This study uses data models at four levels of national, provincial, district and county levels, and urban and rural levels, and takes population density as a link to realize the coupling and integration of various data and construct a macro-to-micro, multi-scale China A refined consumption carbon footprint grid model to estimate China’s carbon footprint. The model diagram is as follows (Figure 1).
The grid model of China’s fine consumption carbon emission is mainly constructed in four steps. The steps are as follows:
(1) Combining the carbon emission data of China’s energy consumption in 2015 and 2017 with the multi-regional input–output model of environmental expansion, it is divided into the consumption emissions of each province through the linkage between departments and regions.
Using EE-MRIO to calculate China’s total carbon emissions, the country’s carbon emissions are divided into provinces. The eight consumption categories included in the study are shown in Table 1. The basic linear equation can be expressed as:
Using matrix representation, the technical coefficient matrix is:
is a unit matrix, is the inverse of the Leontief matrix; the final requirement is:
where , and is the technical coefficient of each sector from region to region ; is the intermediate demand from department in region to department in region ; is the output of sector in region ; indicates that sector produces in region and eventually consumes in region
To account for the environmental impact, we have introduced an emission factor. The emission factor is defined as the amount of CO2 emitted per unit of output. CO2 emissions based on consumption can be calculated as follows:
where is a vector of total CO2 emissions from goods and services used for final demand; is the matrix of emission intensity; represents the emission coefficient of sector in region ; is the CO2 emission inventory of sector in region ; as mentioned above, and represent the Leontief inverse matrix and the final demand, respectively. Notably, carbon emissions embodied in imports were not included in the study due to the high uncertainty associated with nesting the Chinese MRIO model into the global MRIO model.
(2) Break down provincial consumption emissions into counties.
In this study, we decomposed the carbon emissions of consumption based on population distribution, urban–rural differences, and residents’ income levels. To ensure data accuracy and comparability, we resampled the World Pop population data (100 m) to 250 m. Due to data limitations, we used MRIO to calculate the proportion of household consumption input–output data in the province’s total input–output data, and thereby calculated the province’s consumption-based carbon emission proportion. We then calculated the proportion for each county based on its GDP as a percentage of the province’s GDP. By multiplying this proportion with the county’s total carbon emissions, we obtained the consumption-based carbon footprint for each county.
where, for districts and countries consumption carbon emissions, for districts and counties the consumption ratio of the province m where it is located, for districts and counties total carbon emissions.
(3) According to the difference between urban and rural areas, disposable income and population.
The urban and rural population grid cells are divided into urban and rural areas of each county through the urban built-up area data (30 m) and the national county-level regional vector map. The consumption carbon emissions of urban and rural areas are obtained according to the proportion of the urban and rural disposable income of each county in the country.
(4) Decompose the population grid data and urban and rural consumption carbon emissions to calculate the per capita consumption CF.
The consumption carbon emissions of urban and rural areas are allocated to each grid through population grid data, and the per capita CF of urban and rural areas in each district and county is calculated.
where are the per capita CFs of urban and rural areas in county , respectively, and represent the urban and rural disposable income, respectively, and is the population of urban and rural areas in county
In the study, it was found that since the accuracy of the city boundaries extracted from the global urban built-up area data is 90%, the area of some county-level cities has been reduced or ignored, such as western Sichuan and northern Gansu. However, other city boundary data cannot be replaced due to time and scope reasons. In this regard, this study is based on the 2015 and 2017 China Land Cover Dataset (CLCD) (30 m) and Google earth high-precision map to proofread the urban built-up area data, and adjusts the urban boundaries of 87 county-level areas.
As an illustration, let us consider three counties: Gao Tai County in Gansu Province, Yu Cheng District in Sichuan Province, and Ying Jing County in Sichuan Province (Figure 2).
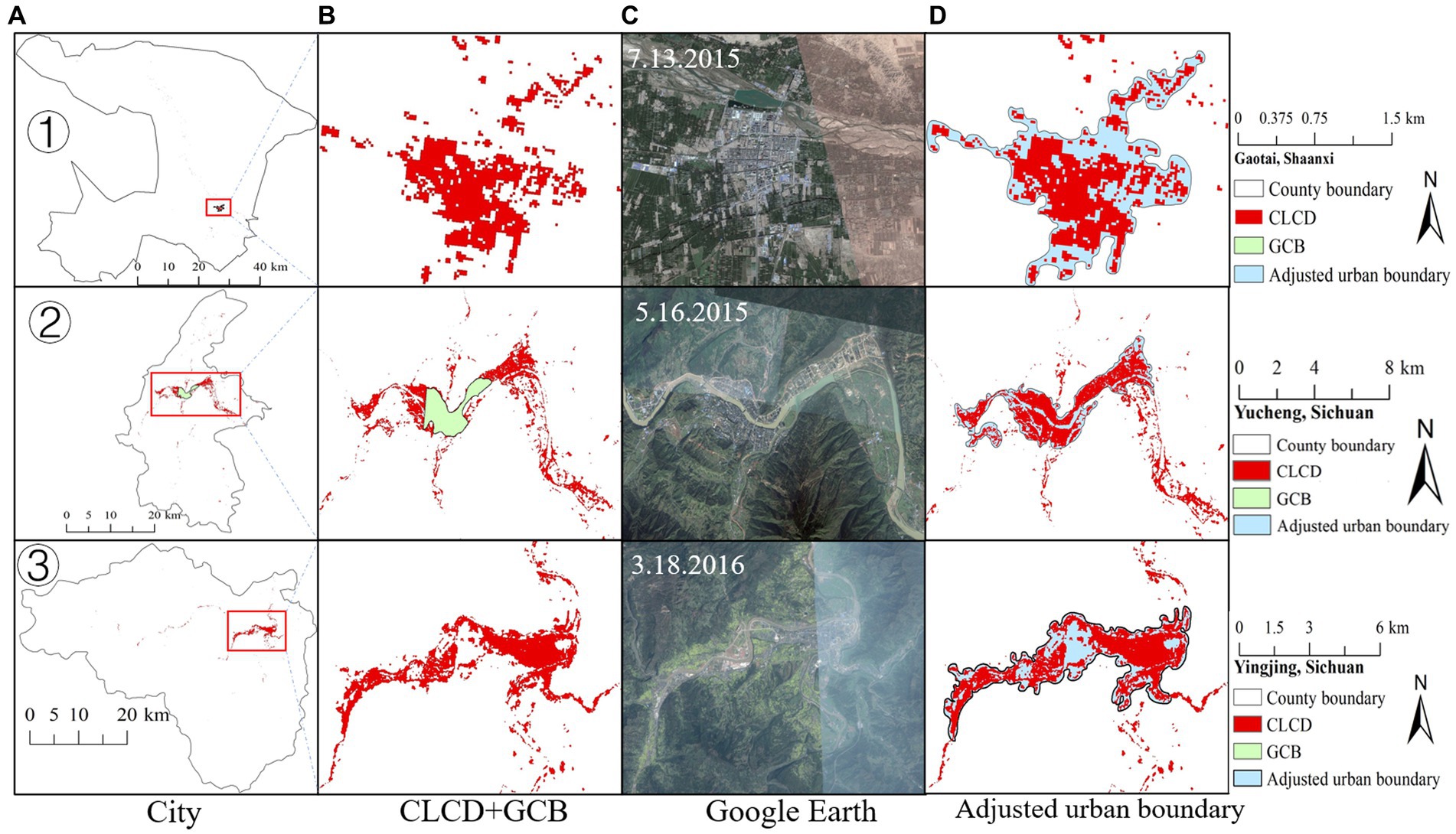
Figure 2. Adjusted urban boundaries of certain county-level areas. ① Corresponds to Gaotai County, Gansu Province. ② Corresponds to Yucheng District, Sichuan Province. ③ Corresponds to Yingjing County, Sichuan Province. (A) City. (B) CLCD + GCP. (C) Google Earth. (D) Adjusted urban boundary.
3. Results and analysis
In order to fully explore the spatial heterogeneity of China’s carbon footprint of consumption, this study has mapped the spatial distribution of China’s carbon footprint and the per capita consumption carbon footprint at the county level. Analysis was conducted on the total carbon footprint, per capita carbon footprint distribution, and data comparison verification at the levels of large regions, urban agglomerations, provinces, cities, and counties. Through a progressive and in-depth analysis at different scales, this study investigates the spatial correlation and aggregation characteristics of carbon emissions within regions, as well as the differences in carbon emissions between different regions.
3.1. Overall spatial pattern of consumption carbon emission
The 250 m high spatial resolution consumption carbon footprint grid data established in this study is a spatially explicit CF estimate data to analyze the spatial pattern. It can be used to analyze the spatial pattern and difference in the consumption CF of Chinese residents. The calculation results of China’s consumption CF are shown in Figure 3. The results show that the CF of China’s consumption has increased from 3681.19 MtC in 2015 to 3757.10 MtC in 2017, accounting for 39.6 and 41% of the nation’s total energy consumption carbon emissions, respectively. The 5% regions with the highest total CF accounted for 19.28 and 19.6% of the total national carbon, while only 22.86 and 23.8% of the regions accounted for 50% of the total national carbon. The fact that the CF is highly concentrated in some wealthy cities means that strong measures in a relatively small number of regions can have a significant impact on achieving carbon neutrality in China.
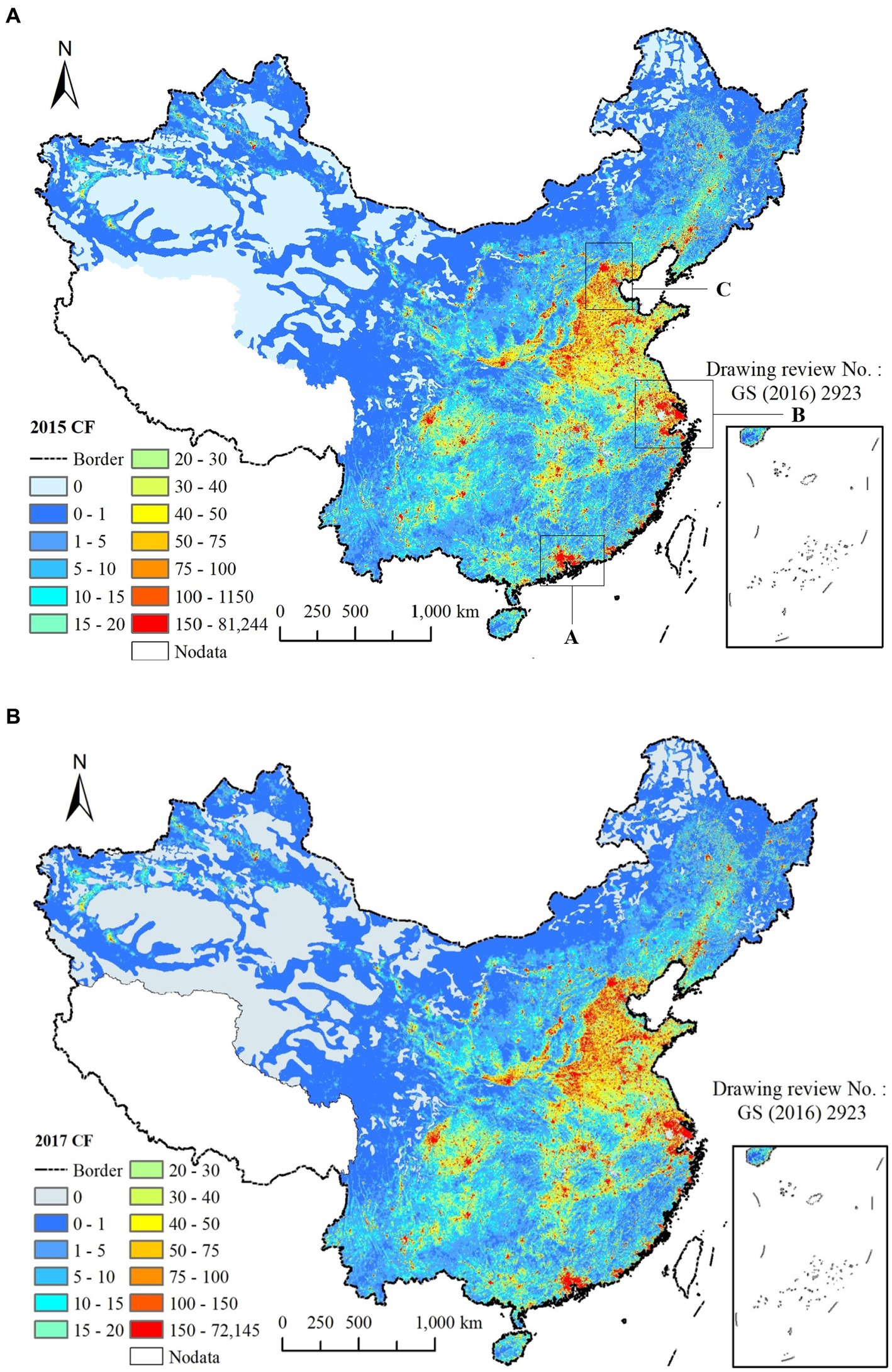
Figure 3. Grid data of consumption carbon footprint in 2015 and 2017. (A) Spatial distribution of carbon footprint in 2015. (B) Spatial distribution of carbon footprint in 2017.
However, it is worth noting that although the consumer carbon footprint in most western regions is relatively low, these regions are more likely to have a faster growth rate. This conclusion has been confirmed by Yang and Yang (2020). From the growth rate perspective, between 2015 and 2017, the western, central and eastern regions increased by 7.7, 6.8, and 4.5%, respectively. In addition, the growth rate of consumer carbon footprint in both urban and rural areas of the western region is higher than that of other regions, while the rural consumer carbon footprint in the eastern region has experienced a negative growth rate (−0.7%). The main reason for this trend is that the income level in the western region is growing faster than other regions, leading to a rapid increase in the consumer carbon footprint. For instance, the per capita disposable income growth rate in urban and rural areas of Inner Mongolia was 16.7 and 26%, respectively. In the future, the difference in consumer carbon footprint between western and eastern regions of China will further narrow, and the western region will become a potential area for China’s carbon footprint.
For urban and rural consumption CF, the proportion of total carbon of urban and rural areas in 2015 was 56.55 and 43.45%, respectively. The proportion of urban and rural areas in 2017 was 58.27 and 41.73%. In prior research, the proportion of total carbon consumption in rural areas was only 25 and 32% (Gill and Moeller, 2018; Chen et al., 2019; Liu and Zhang, 2022). They all underestimated the potential of rural areas to reduce emissions. As one of the fastest-growing regions in the urbanization and rural revitalization process, with a population of approximately 600 million (2015), household consumption is undergoing tremendous changes, especially in the transition from “poverty alleviation” to “rural revitalization,” leading to significant changes in CF and inequality.
3.2. Spatial analysis of per capita consumption carbon footprint at the county level
To address the urban–rural divide, the county-level total consumption carbon emissions can be disaggregated based on population data to obtain a per capita carbon footprint indicator that eliminates the impact of population scale. This indicator is then used to depict the per capita carbon footprint of urban and rural residents, which further reveals the urban–rural divide in per capita carbon footprint, as shown in Figure 4. Our experimental findings indicate that the per capita carbon footprint of urban residents is much higher than that of rural residents. In 2015, the per capita carbon footprint of urban residents was 3.25 tC, while that of rural residents was 1.72 tC. By 2017, the per capita carbon footprint of urban residents had risen to 3.48 tC, while that of rural residents had increased to 1.78 tC.
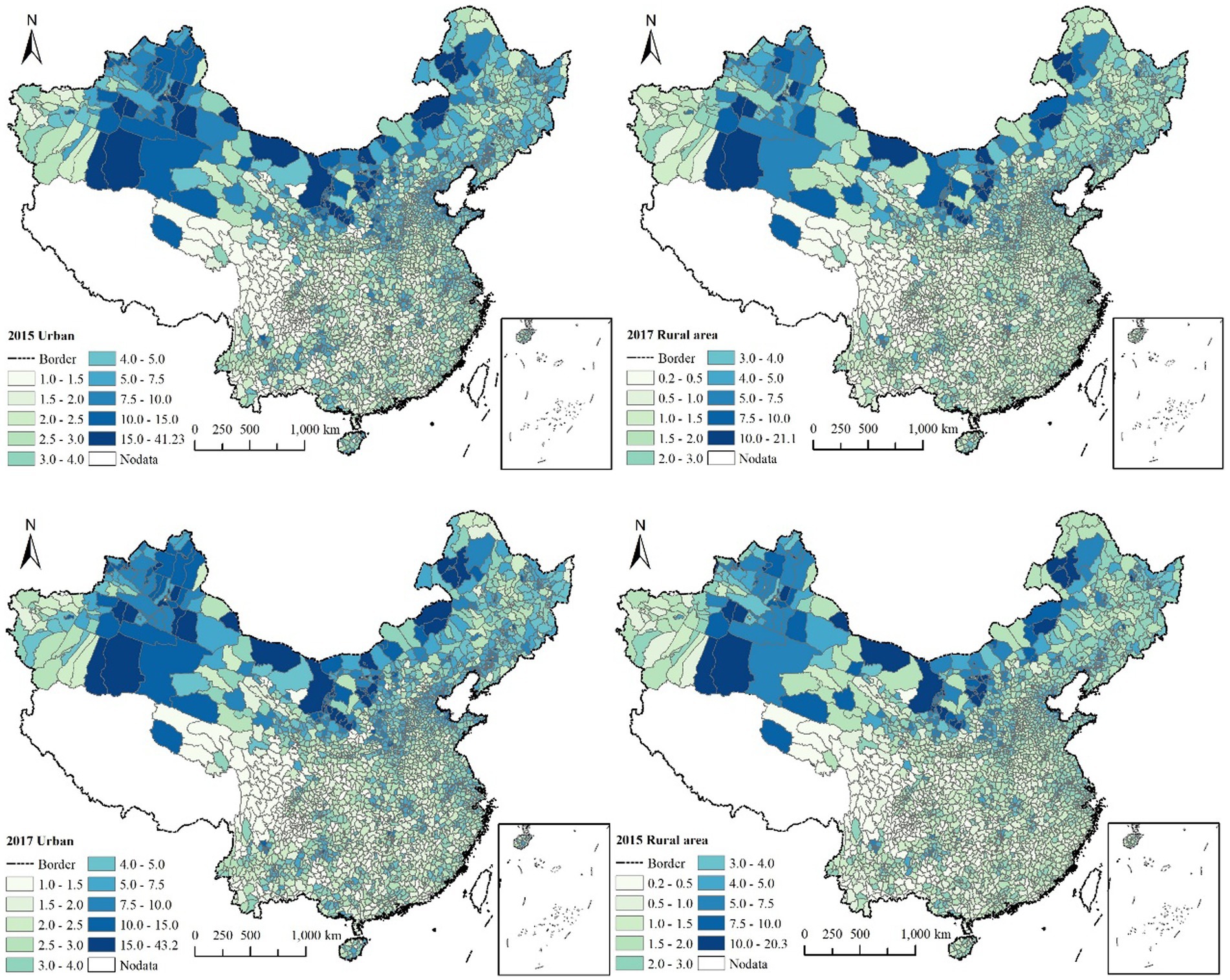
Figure 4. Distribution of per capita carbon emission in urban and rural areas in districts and counties in 2015 and 2017. The standard map is made based on the standard map service website GS (2016) 2923 of the Ministry of Natural Resources of China. The boundary of the base map is not modified.
The most obvious feature is the huge disparity in per capita carbon footprints between different regions. In China, per capita carbon footprints vary greatly among urban and rural areas, as well as among counties, as shown in Figure 4. In 2015, the highest per capita carbon footprint in urban areas was 41.23 tC in Quenger Banner, which was 40 times higher than the lowest in Yanjin County at 1.02 tC. In rural areas, the highest per capita carbon footprint was 20.31 tC in Ejina Banner, which was 98 times higher than the lowest in Weixin County at 0.204 tC. By 2017, the per capita carbon footprint in urban areas ranged from 1.03 tC in Zhenxiong to 43.23 tC in Beitun City, while in rural areas it varied from 0.23 tC in Weixin County to 21.06 tC in Shenmu County.
3.3. Multidimensional analysis of key areas
The Greater Bay Area, Beijing-Tianjin-Hebei region, and Yangtze River Delta are presently the largest sources of carbon emissions, as major metropolitan areas and urban agglomerations. Meanwhile, suburbs of major cities such as Wuhan, Hangzhou, and Chengdu are regarded as city-level scales, and areas in the west that are rapidly developing, such as Inner Mongolia and Xinjiang, are counties with high per capita carbon emissions, deserving attention. These three types of research areas are prominent sources of carbon emissions in China, and simultaneously provide a favorable analytical angle and demonstration role for high-spatial-resolution consumption carbon footprint grid data from the three scales of provinces, cities, and counties. Therefore, detailed analyses and discussions of these three types of regions are conducted, exploring the spatial correlations, spatial aggregation characteristics, and differences in carbon emissions among regions at different scales.
3.3.1. Coordinated development of urban agglomerations to promote emission reduction
The three highly developed regions in China—the Yangtze River Delta, Pearl River Delta, and Beijing-Tianjin-Hebei urban agglomerations—are not only important engines driving the country’s economic development, but also areas that attract significant attention for their carbon reduction efforts. These regions account for only 5.18% of China’s total land area, but contribute to 28% of the country’s carbon footprint (9.9% for Beijing-Tianjin-Hebei, 12.0% for the Yangtze River Delta, and 6% for the Pearl River Delta), as shown in Figure 5. However, there are also significant regional disparities within these urban agglomerations. The carbon footprint of the Guangdong-Hong Kong-Macao Greater Bay Area is highly centralized, with Guangzhou and Shenzhen as the core cities, while Foshan, Dongguan, and Huizhou also have a high carbon footprint. In the Yangtze River Delta, Shanghai stands out, while most parts of Jiangsu Province have a high carbon footprint, and only Hangzhou and Ningbo are hotspots in Zhejiang Province. In the Beijing-Tianjin-Hebei region, there is a stark north–south difference, with the carbon footprint mainly concentrated in the North China Plain. As a key area for carbon footprint reduction, there is tremendous potential for carbon reduction within urban agglomerations. How can we effectively achieve carbon reduction within these regions? Research has shown that there is a significant spatial interaction effect in the carbon reduction capacity of urban agglomerations (Wang et al., 2019; Yu et al., 2020). Specifically, for every 1% increase in population, the carbon footprint is reduced by 0.22%. Urban agglomerations can significantly promote carbon reduction through the sharing and spillover of knowledge, skills, and technology. Therefore, carbon reduction planning for urban agglomerations should not only consider individual cities, but also neighboring cities. Cross-city joint prevention and control measures should be implemented to improve the sharing and utilization of population resources, and a carbon reduction coordination mechanism should be established between cities to form a united front for carbon reduction.
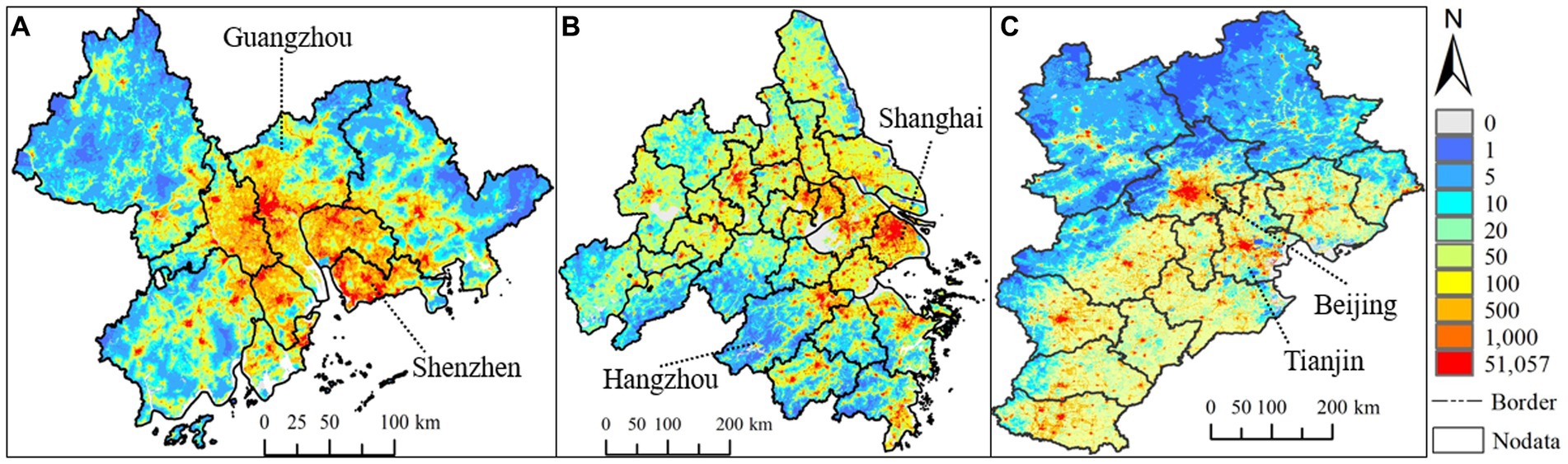
Figure 5. Carbon emission of three urban agglomerations in China (2015). Positions of A, B and C are shown in column “A” of Figure 3.
3.3.2. Metropolitan suburbs have higher per capita carbon footprints
It is worth noting that the per capita CF of the suburbs of major cities is higher than that of their central urban areas, and the per capita CF of the suburbs is 2.3–6.8 times that of the central urban areas, as shown in Figure 6.
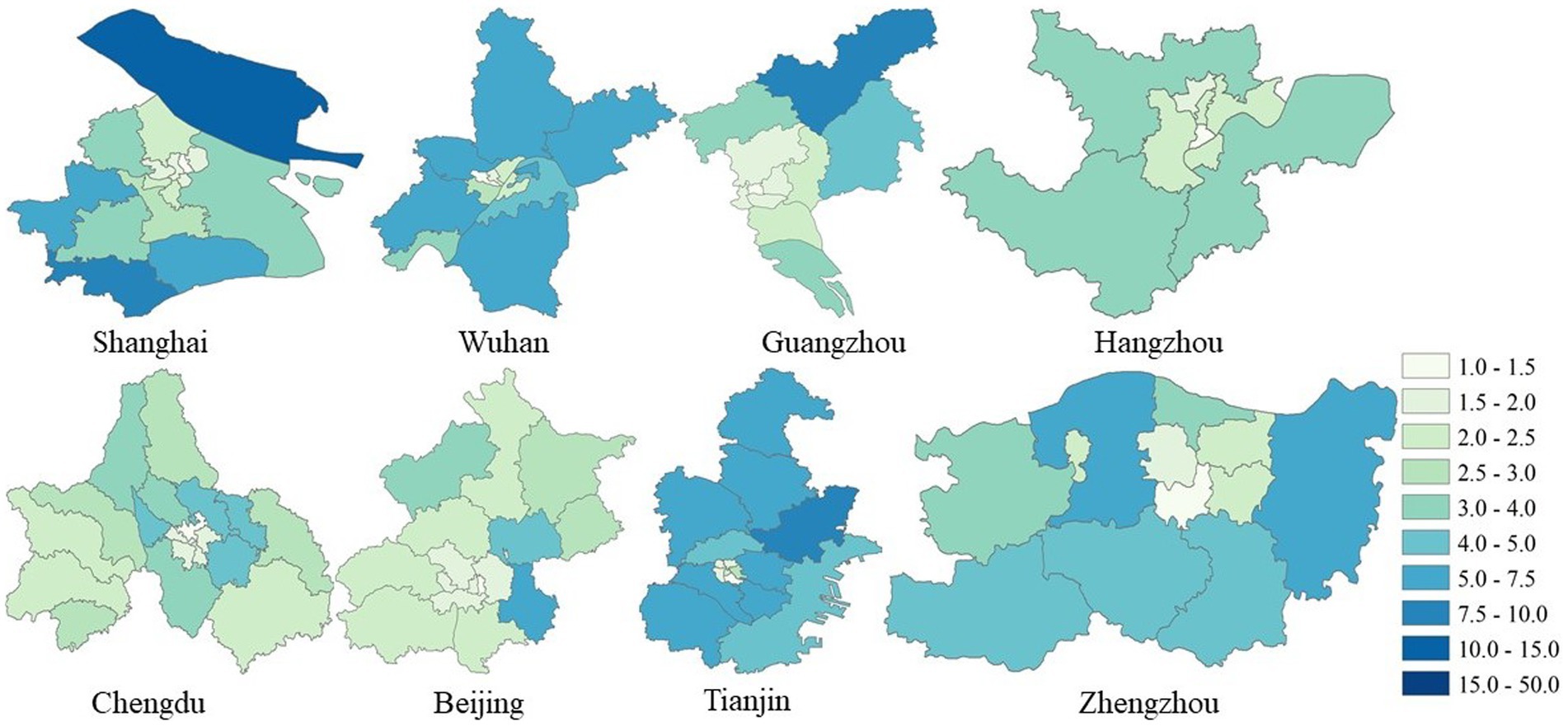
Figure 6. Distribution of per capita carbon footprints in the central and suburban areas of some large cities.
There are three main factors driving the suburbanization trend in large cities. The first is to alleviate the housing affordability crisis, air pollution, and traffic congestion in the central city, by shifting population and industries to the outskirts (Shan et al., 2018). The second factor is the density effect (Schubert et al., 2013), which refers to the benefits of high-rise buildings, shorter commuting distances, and developed public transportation systems in densely populated areas. Several studies have shown that, due to higher income, larger housing spaces, and longer private transportation distances, the carbon footprint per capita in suburbs is relatively high (Jones et al., 2014; Ghaemi and Smith, 2020). The third factor is that the overall higher income levels and higher disposable income per capita in the suburbs of major cities lead to higher carbon footprints, despite the lower population density. As China’s urbanization process continues, more people are flocking to megacities and provincial capitals, leading to an increasing concentration of carbon footprints within the cities. The agglomeration of population and industries is causing the carbon footprint in urban suburbs to rise continuously. With the ongoing urbanization of Beijing, Tianjin, Shanghai, Wuhan, and other cities, the social and structural morphology of urban areas has entered a new stage, breaking through the turning point of the Kuznets curve and entering the downward stage.
3.3.3. The per capita carbon footprint at the county level shows the characteristics of carbon emissions in the western region
Such as Ningxia, Xinjiang, and Inner Mongolia. By estimating at the county level, we have identified several disproportionately high per capita carbon footprints in certain counties. These counties are mainly located in the western region (Figure 7) of China and are characterized by extremely low total carbon emissions, but extremely high per capita carbon footprints. They possess abundant energy resources, such as coal and natural gas, but their industries are mostly high energy-consuming and high-emission sectors, resulting in high per capita carbon footprints. For example, Inner Mongolia is one of the major suppliers of energy products in China and has the largest net emissions of any province. However, due to its vast territory and sparse population, it has become the region with the highest per capita carbon footprint.
Due to differences in population size, the per capita CF in the area of class (iii) is much higher than that of the area of class (i) and (ii). Compared to previous studies, the historical period of lower per capita carbon footprints in the central and western regions, which were achieved through policies such as the Western Development strategy aimed at eliminating poverty, has already passed. Therefore, how to maintain the reduction of carbon footprints in the process of development is an issue that requires close attention in this region.
3.4. Research on the correlation between population density, disposable income, and carbon footprint
Figure 8 illustrates the relationship between population density, household income, urban–rural carbon emissions (carbon footprint), and the differences in urban–rural carbon footprints across different regions in China. Each bubble represents a city, with its size corresponding to its population density, and distinguishing between urban and rural areas. Overall, carbon emissions and footprints show a positive correlation with disposable income and population density, but the strength of this correlation varies. In urban areas, the positive correlation between disposable income, population density, and total carbon emissions is more pronounced than that with per capita carbon footprint. As population increases and incomes rise, carbon emissions in the region are expected to increase accordingly. The highest carbon emissions are observed in Dongguan (Guangdong), Pudong New Area (Shanghai), and Chaoyang (Beijing). In contrast, the relationship between population density, disposable income, and total carbon emissions in rural areas is less closely related and shows a scattered distribution. The correlation with per capita carbon footprint is slightly higher than that in urban areas.
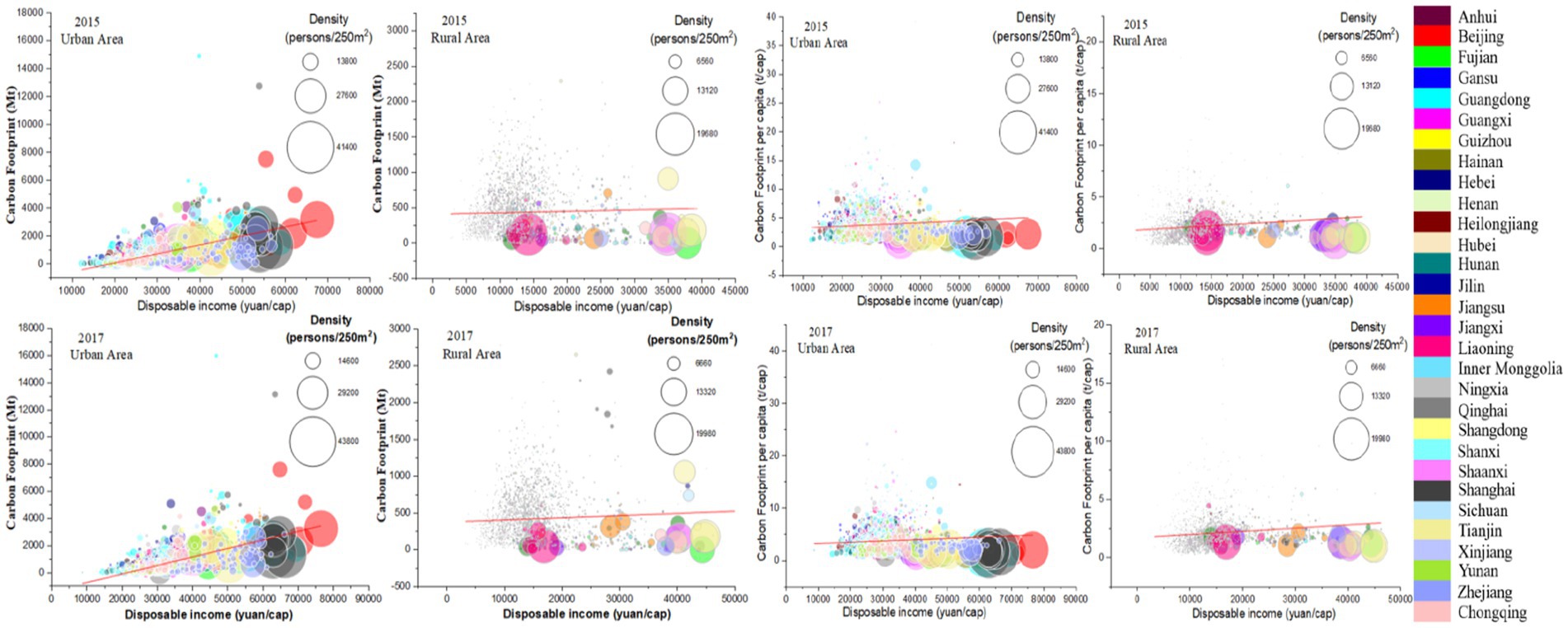
Figure 8. Bubble chart of the relationship between population density, disposable income, and total carbon (carbon emission per capita) in 2015 and 2017.
It is noteworthy that the areas with generally higher carbon emissions and footprints are those with medium incomes and population densities. Specifically, when disposable income in urban areas is between 30,000 and 50,000 RMB and in rural areas is between 10,000 and 20,000 RMB, both carbon emissions and footprints are higher than those in other income ranges. As pointed out by Ottelin et al. (2019), the relationship between population density, disposable income, and carbon footprint varies across regions. In this study, we found a moderate positive correlation between these variables, with the strength of this relationship further related to the level of poverty in the region. When per capita disposable income is below 30,000 RMB in urban areas and below 15,000 RMB in rural areas, per capita carbon footprint appears to be more strongly correlated with population density and disposable income. However, as disposable income increases further, the growth of carbon footprint reaches a turning point and enters a decreasing phase, forming an inverted U-shaped “Environmental Kuznets Curve.” This suggests that when the economy reaches a certain stage of development, carbon footprint is no longer solely determined by disposable income and population density, but is influenced by more complex factors and different regions have different consumption patterns.
3.5. Data validation and uncertainty analysis
3.5.1. Comparison with existing research
To validate the accuracy of our results, we compared them with those of other researchers. Currently, there is limited research on consumer carbon footprints. A search of keywords such as “carbon emissions,” “consumption,” and “carbon footprint” on Web of Science revealed that the vast majority of studies on consumer carbon footprints used research data from 2007 to 2012 due to limitations in MRIO data. A small number of studies used data from 2015, 2017, and 2018. Regarding the research methodology, studies using the MRIO approach for calculating consumer carbon footprints were conducted in 2007, 2012, 2015, and 2017, also due to MRIO data limitations. The method used to calculate carbon emissions in 2018 was the IPCC sectoral approach, which resulted in significant differences in results due to inconsistent methods. Therefore, some results were compared based on the ratio of consumer carbon emissions to total emissions in their respective studies. We selected some authoritative papers from different scales such as per capita consumer carbon emissions in urban and rural areas of China in 2015 and 2016, the total carbon emissions of consumption in China, as well as the carbon emissions of several municipal and county-level cities, to demonstrate the accuracy of our study. The comparison results are shown in Table 2.
Overall, the difference in results is not significant, except for an error of 33% in Wu’an City, Hebei Province in 2015. The errors in other results are less than 17%, and some are as low as 0.16%. The per capita carbon emissions error is generally lower than the total carbon emissions error. The comparison results indicate that the results of our study are consistent with those of other studies, demonstrating good reliability.
3.5.2. Comparison with ODIAC data for validation
In the current study, most CF estimation studies do not have any clear consistency. However, to ensure the validity of the data, we verified the experimental results using ODIAC data provided by the Center for Global Environmental Research (CGER), Japan (Oda et al., 2018), in collaboration with Oak Ridge National Laboratory, USA, with a spatial resolution of 1 km*1 km, covering global emissions from 1980 to 2020. As shown in Figure 9, this research is in excellent agreement with the overall spatial trend of the ODIAC data, R2 is 0.7058, and the RMSE is higher, which is 216.57, mainly due to the difference in resolution between the two. Compared to other research, our overall trends are more consistent with studies of similar scopes, such as Zhou et al. (2022).
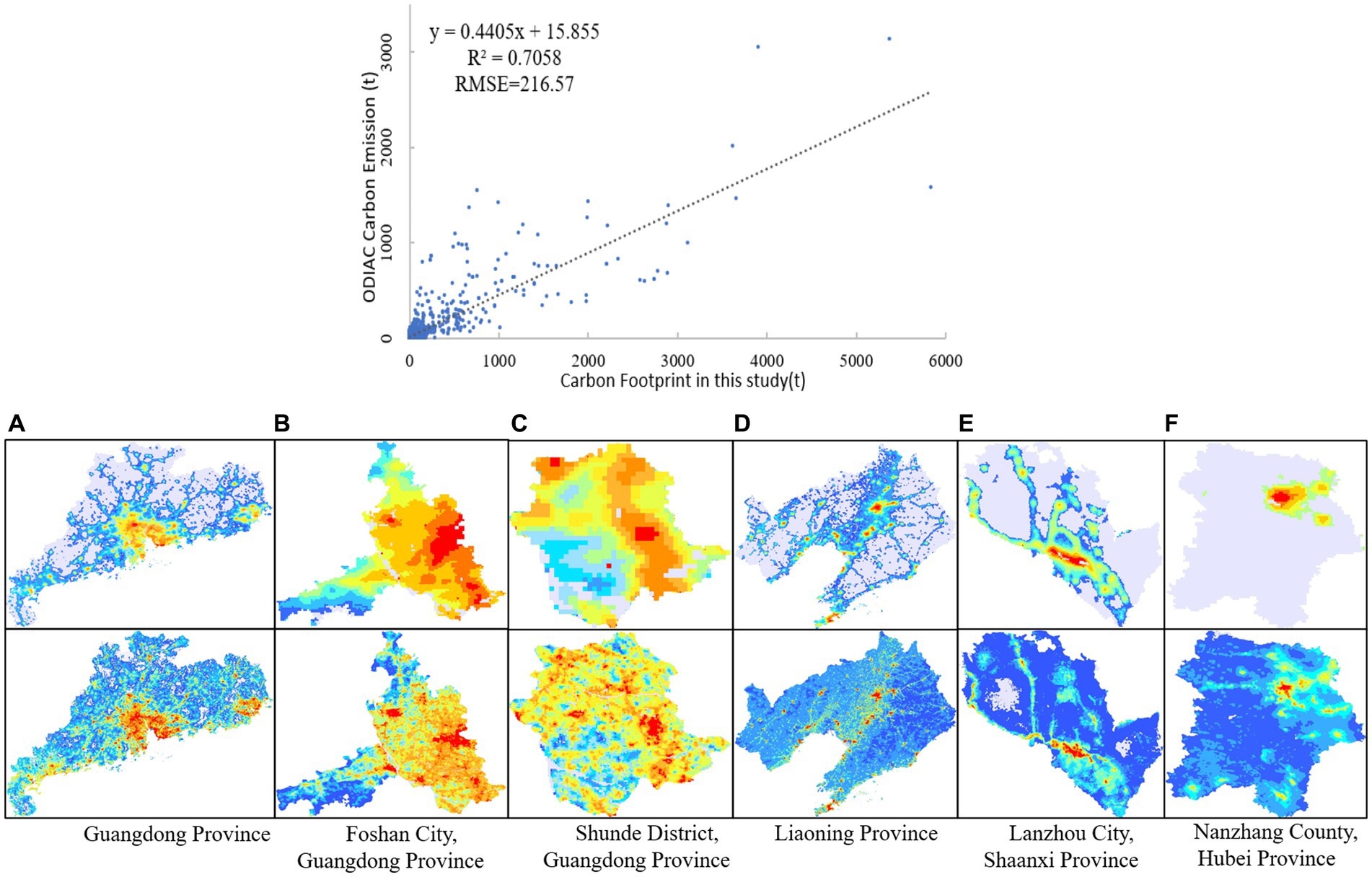
Figure 9. Comparison of this study with ODIAC data. (A) Guangdong Province. (B) Foshan City, Guangdong Province. (C) Shunde District, Guangdong Province. (D) Liaoning Province. (E) Lanzhou City, Shaanxi Province. (F) Nanzhang County, Hubei Province.
Despite the similarity of ODIAC spatial trends to our study, its spatial effect is significantly lower than the results of our study. We compared and validated our results with ODIAC data at multiple scales, including different scales within the same province of southern China, such as Guangdong Province, Foshan City, and Shunde District, as well as different provinces across China, such as Liaoning Province in northeast China, Lanzhou City in Shaanxi Province in western China, and Nanzhang County in Hubei Province in central China. This comparison reflects the effectiveness of different research scales in different regions of China and the regional differences. The validation results show that the overall trends are basically consistent, and the hotspot regions can be effectively identified. In terms of details, it can be seen that the hotspot regions in our study have better detail, clear directionality, and higher data refinement compared to ODIAC data. It is evident that in areas outside urban areas, especially at the county level (Figures 9C,F), ODIAC only displays partial urban areas, while our study can display the distribution of carbon footprints in rural areas, highlighting its advantages as a supplement to current emission inventories.
3.5.3. Uncertainty analysis
The uncertainty of the research lies in the following aspects. First, the MRIO model used to calculate regional trade consumption data is not entirely reliable. They assume that the industry is homogeneous and that all industries supply the same price. Other studies investigated the reliability of MRIO results at the worldwide level found that independent models were within the margin of error of ±5% in developed countries (Heinonen et al., 2020). Second, the uncertainty of the data. The proportion of consumption CF of county-level cities is determined based on the provincial MRIO table. Currently, there is a lack of city-level or even county-level MRIO tables, so the level of detail of the data will bring some uncertainty. In addition, due to the limitations of the global built-up area data, the 2017 CF is estimated using the 2018 built-up area, which has an uncertainty range of 1.06–3.6% depending on the annual built-up area growth rate and urbanization rate.
Monte Carlo simulations were used to calculate the uncertainty of the consumption CF of 2,800 county-level cities in China, and 20,000 simulations were carried out to analyze the uncertainty of estimated emissions in each region. We assume that both activity data and emission factors have a normal distribution. The results show that the uncertainty of all cities is less than 10%, which is within the normal range of uncertainty. Among them, the lowest uncertainty is 1.2% in Jing’an District, Shanghai, and the highest uncertainty is 9.38% in Ejina Banner, Inner Mongolia.
4. Discussion
With China’s pledge to become carbon neutral by 2060, policymakers urgently need to consider how to reduce carbon emissions. Residential consumption, as one of the main drivers of carbon emissions, must be prioritized in plans to achieve carbon neutrality. We estimate China’s total consumption-based CF as well as its per capita CF, revealing significant disparities in CFs across regions. This result has an excellent fitting result with the current international authoritative carbon emission data ODIAC.
Although our data improve the previous understanding of the scale, precision, and regional disparities of carbon emissions accounting in China, it still has a number of limitations that we hope to solve these problems in our future work. First, due to the limitations of data, 2015 and 2017 in CADEs are the latest detailed MRIO tables and cannot discuss the latest information on carbon emissions from household consumption in recent years. Owing to data limitations, only provincial-level MRIO data and county-level carbon emissions data are available. Consumption-based carbon emissions proportions at the county level remain unknown. Therefore, this study utilizes MRIO and GDP data to decompose provincial consumption proportions into county-level proportions. However, the imperfect correspondence between GDP and consumption data may potentially impact the disparities in consumption-based carbon emissions among different cities within the same province. The methodology developed in this research is based on the most comprehensive data we could find to facilitate the accuracy of data on carbon emissions from residential consumption. Secondly, we mainly study the CF analysis of county-level cities and residents and the interaction of influencing factors such as population and income, but if more specific information such as income level, consumption structure, population structure, education level, occupational information, etc. The spatial characteristics and drivers driving the continued increase in the CF of consumption will be fully understood.
Despite these limitations, this study provides a detailed analysis of the spatial pattern and differences in consumption CF in county-level regions and per capita in China, providing new spatial details and empirical results of the CF, and more generally demonstrates the contribution of high-resolution variables to the quality of analysis.
Through further checking and comparing Moran’s research (Moran et al., 2018), it is found that Moran combined national statistics on urban and rural spending patterns, regional purchasing power data, and demographic maps for different countries to estimate carbon emissions for 13,000 cities in the world. Seoul, Guangzhou, New York, Shanghai, Los Angeles, and Hong Kong are the top six, respectively. Moran claims that Guangzhou is the city with the largest CF in China, but based on the current research, there is no data to support that Guangzhou is the city with the largest CF in China. In this research, Shanghai ranks first with a CF of 96.18 Mt., and Guangzhou (32.34 Mt) ranks eighth. Therefore, we believe that when Moran defines Guangzhou, Foshan, Dongguan, Zhongshan, Jiangmen, Huizhou, Qingyuan, Shenzhen, and other Pearl River Delta urban agglomerations (a total of 127.62 Mt) as Guangzhou, it is possible to exceed Shanghai’s CF. Currently, due to the inconsistent research methods of consumption CF the lack of time-limited and accurate actual carbon emission data, and the lack of solid theoretical background, the estimation of carbon emissions is not accurate. However, this does not support the basis for Guangzhou to become the second-largest emission city in the world, which leads to the misunderstanding of the spatial pattern of carbon emissions in China by various governments and social groups.
5. Conclusion
As household consumption plays an increasingly important role in China, constructing detailed spatial grid data on consumer carbon emissions and revealing their spatial hotspots and heterogeneity is an important step in understanding the complexity of China’s carbon emission spatial patterns. This is also an important supplementary indicator for implementing China’s carbon reduction regional coordination policies. In this study, we estimated China’s 250 m high spatial resolution consumer carbon emissions grid data using a fine-grained consumer carbon emission grid model. We created a spatially clear, hotspot-rich, and closely related carbon footprint map of China and a county-level per capita carbon footprint map. Our study found that carbon footprint spatial distribution is imbalanced, with significant differences in per capita carbon emissions within regions and between urban and rural areas. Finally, we verified our experimental results using ODIAC data.
It is worth noting that several new discoveries were made during the course of this study:
(1) The 250 m high-resolution grid map of carbon emissions at the consumer level created in this study covers various spatial scales, including national, provincial, county, and urban–rural areas. This fills the gaps in existing databases and enables dynamic investigations of the spatial patterns of carbon emissions across multiple scales. This provides a new approach for regions that have difficulty in estimating carbon emissions.
(2) The study indicates that the top 5% of regions with the highest carbon emissions account for approximately 1/5 of the country’s total carbon emissions, while about 1/4 of the regions account for over 50% of the total carbon emissions. The carbon reduction potential in rural areas of China has been severely underestimated by approximately 10% or more, whereas the carbon emissions in western regions have shown the fastest growth due to poverty alleviation efforts.
(3) The per capita carbon emissions in suburban areas of large cities are higher than those in the central urban areas. This is likely due to the density effect of large cities, the trend of suburbanization, and the overall higher level of development. A “Environmental Kuznets Curve” has been formed between disposable income and carbon emissions, which shows an inverted U-shaped relationship.
(4) In our study, Shanghai had the highest residential consumption-based carbon emissions among cities in China. This contradicts the widely accepted conclusion in existing research that Guangzhou has the highest consumption-based carbon emissions. This result has prompted a reconsideration of the spatial pattern of China’s consumption-based carbon emissions.
China is facing a dilemma: on one hand, it needs to encourage consumption to ensure domestic economic growth, while on the other hand, it must slow down the rapid growth of carbon emissions to respond to international pressure and fulfill its clear commitments to reduce emissions. For the Chinese government, the priority is to guide consumer behavior and encourage individuals to change their lifestyles based on changes in their consumption habits. Additionally, the government should establish regional coordination mechanisms to develop corresponding emission reduction plans and promote cross-regional cooperation to coordinate carbon consumption and emissions. These actions can help decision-makers to reconsider current emission reduction goals and modify related policies, while considering resource endowments, historical contributions, income levels, population density, and cross-regional coordination to build a more balanced, flexible, and comprehensive policy mechanism.
Data availability statement
The raw data supporting the conclusions of this article will be made available by the authors, without undue reservation.
Author contributions
FX: formal analysis, writing—original draft, and writing—review and editing. XZ: conceptualization, methodology, software, and funding acquisition. MZ: data curation and review—revise and editing. DL: investigation, resources, and data. YM: conceptualization, methodology, and formal analysis. TW: investigation and writing—review and editing. XH: data and writing—original draft. All authors contributed to the article and approved the submitted version.
Funding
This work was supported by the National Natural Science Foundation of China (Nos. 72033005 and 42201471), Beijing Social Science Foundation (No. 2022YJC264), and the Third Xinjiang Scientific Expedition of the Key Research and Development Program by Ministry of Science and Technology of the People’s Republic of China (No. 2022xjkk1104).
Conflict of interest
The authors declare that the research was conducted in the absence of any commercial or financial relationships that could be construed as a potential conflict of interest.
Publisher’s note
All claims expressed in this article are solely those of the authors and do not necessarily represent those of their affiliated organizations, or those of the publisher, the editors and the reviewers. Any product that may be evaluated in this article, or claim that may be made by its manufacturer, is not guaranteed or endorsed by the publisher.
Footnotes
References
Bai, Y., Zheng, H., Shan, Y., Meng, J., and Li, Y. (2021). The consumption-based carbon emissions in the Jing-Jin-Ji urban agglomeration over China’s economic transition. Earth’s Future 9:e2021EF002132. doi: 10.1029/2021EF002132
Cai, B., Guo, H., Cao, L., Guan, D., and Bai, H. (2018a). Local strategies for China’s carbon mitigation: an investigation of Chinese city-level CO2 emissions. J. Clean. Prod. 178, 890–902. doi: 10.1016/j.jclepro.2018.01.054
Cai, B., Wang, X., Huang, G., Wang, J., Cao, D., Baetz, B. W., et al. (2018b). Spatiotemporal changes of China’s carbon emissions. Geophys. Res. Lett. 45, 8536–8546. doi: 10.1029/2018GL079564
Chen, J., Gao, M., Cheng, S., Hou, W., Song, M., Liu, X., et al. (2020). County-level CO2 emissions and sequestration in China during 1997–2017. Sci Data 7:391. doi: 10.1038/s41597-020-00736-3
Chen, S., Long, H., Chen, B., Feng, K., and Hubacek, K. (2020). Urban carbon footprints across scale: important considerations for choosing system boundaries. Appl. Energy 259:114201. doi: 10.1016/j.apenergy.2019.114201
Chen, G., Zhu, Y., Wiedmann, T., Yao, L., Xu, L., and Wang, Y. (2019). Urban-rural disparities of household energy requirements and influence factors in China: classification tree models. Appl. Energy 250, 1321–1335. doi: 10.1016/j.apenergy.2019.04.170
Fan, Z.-X., Fang, X.-Q., and Yun, S. (2018). Changes in global grid pattern of carbon emissions. Clim. Change Res. 14, 505–512. doi: 10.12006/j.issn.1673-1719.2018.069
Fan, J.-L., Liao, H., Liang, Q. M., Tatano, H., Liu, C. F., and Wei, Y. M. (2013). Residential carbon emission evolutions in urban–rural divided China: an end-use and behavior analysis. Appl. Energy 101, 323–332. doi: 10.1016/j.apenergy.2012.01.020
Feng, K., Hubacek, K., and Song, K. (2021). Household carbon inequality in the U.S. J. Clean. Prod. 278:123994. doi: 10.1016/j.jclepro.2020.123994
Ghaemi, Z., and Smith, A. D. (2020). A review on the quantification of life cycle greenhouse gas emissions at urban scale. J. Clean. Prod. 252:119634. doi: 10.1016/j.jclepro.2019.119634
Gill, B., and Moeller, S. (2018). GHG emissions and the rural-urban divide. A carbon footprint analysis based on the German official income and expenditure survey. Ecol. Econ. 145, 160–169. doi: 10.1016/j.ecolecon.2017.09.004
Gong, P., Li, X., Wang, J., Bai, Y., Chen, B., Hu, T., et al. (2020). Annual maps of global artificial impervious area (GAIA) between 1985 and 2018. Remote Sens. Environ. 236:111510. doi: 10.1016/j.rse.2019.111510
Gurney, K. R., Patarasuk, R., Liang, J., Song, Y., O’Keeffe, D., Rao, P., et al. (2019). The Hestia fossil fuel CO2 emissions data product for the Los Angeles megacity (Hestia-LA). Earth Syst. Sci. Data 11, 1309–1335. doi: 10.5194/essd-11-1309-2019
Heinonen, J., Ottelin, J., Ala-Mantila, S., Wiedmann, T., Clarke, J., and Junnila, S. (2020). Spatial consumption-based carbon footprint assessments - a review of recent developments in the field. J. Clean. Prod. 256:120335. doi: 10.1016/j.jclepro.2020.120335
Hertwich, E. G., and Peters, G. P. (2009). Carbon footprint of nations: a global, trade-linked analysis. Environ. Sci. Technol. 43, 6414–6420. doi: 10.1021/es803496a
Hubacek, K., Baiocchi, G., Feng, K., and Patwardhan, A. (2017). Poverty eradication in a carbon constrained world. Nat. Commun. 8:912. doi: 10.1038/s41467-017-00919-4
Ivanova, D., Barrett, J., Wiedenhofer, D., Macura, B., Callaghan, M., and Creutzig, F. (2020). Quantifying the potential for climate change mitigation of consumption options. Environ. Res. Lett. 15:093001. doi: 10.1088/1748-9326/ab8589
Ivanova, D., Vita, G., Steen-Olsen, K., Stadler, K., Melo, P. C., Wood, R., et al. (2017). Mapping the carbon footprint of EU regions. Environ. Res. Lett. 12:054013. doi: 10.1088/1748-9326/aa6da9
Jones, C., and Kammen, D. M., Spatial Distribution of U.S (2014). Household carbon footprints reveals suburbanization undermines greenhouse gas benefits of urban population density. Environ. Sci. Technol. 48, 895–902. doi: 10.1021/es4034364
Kanemoto, K., Moran, D., and Hertwich, E. G. (2016). Mapping the carbon footprint of nations. Environ. Sci. Technol. 50, 10512–10517. doi: 10.1021/acs.est.6b03227
Lenzen, M., Moran, D., Kanemoto, K., Foran, B., Lobefaro, L., and Geschke, A. (2012). International trade drives biodiversity threats in developing nations. Nature 486, 109–112. doi: 10.1038/nature11145
Li, J., Zhang, D., and Su, B. (2019). The impact of social awareness and lifestyles on household carbon emissions in China. Ecol. Econ. 160, 145–155. doi: 10.1016/j.ecolecon.2019.02.020
Liu, L., Qu, J., Zhang, Z., Zeng, J., Wang, J., Dong, L., et al. (2018). Assessment and determinants of per capita household CO2 emissions (PHCEs) based on capital city level in China. J. Geogr. Sci. 28, 1467–1484. doi: 10.1007/s11442-018-1556-z
Liu, X., Wang, X., Song, J., Wang, H., and Wang, S. (2019). Indirect carbon emissions of urban households in China: patterns, determinants and inequality. J. Clean. Prod. 241:118335. doi: 10.1016/j.jclepro.2019.118335
Liu, M., Wang, H., Wang, H., Oda, T., Zhao, Y., Yang, X., et al. (2013). Refined estimate of China’s CO2 emissions in spatiotemporal distributions. Atmos. Chem. Phys. 13, 10873–10882. doi: 10.5194/acp-13-10873-2013
Liu, G., and Zhang, F. (2022). China’s carbon inequality of households: perspectives of the aging society and urban-rural gaps. Resour. Conserv. Recycl. 185:106449. doi: 10.1016/j.resconrec.2022.106449
Liu, X., Zhang, L., Hao, Y., Yin, X., and Shi, Z. (2022). Increasing disparities in the embedded carbon emissions of provincial urban households in China. J. Environ. Manag. 302:113974. doi: 10.1016/j.jenvman.2021.113974
Long, Z., Zhang, Z., Liang, S., Chen, X., Ding, B., Wang, B., et al. (2021). Spatially explicit carbon emissions at the county scale. Resour. Conserv. Recycl. 173:105706. doi: 10.1016/j.resconrec.2021.105706
Ma, R., Zheng, X., Zhang, C., Li, J., and Ma, Y. (2022). Distribution of CO2 emissions in China’s supply chains: a sub-national MRIO analysis. J. Clean. Prod. 345:130986. doi: 10.1016/j.jclepro.2022.130986
Mi, Z., Meng, J., Guan, D., Shan, Y., Song, M., Wei, Y. M., et al. (2017). Chinese CO2 emission flows have reversed since the global financial crisis. Nat. Commun. 8:1712. doi: 10.1038/s41467-017-01820-w
Mi, Z., Zhang, Y., Guan, D., Shan, Y., Liu, Z., Cong, R., et al. (2016). Consumption-based emission accounting for Chinese cities. Appl. Energy 184, 1073–1081. doi: 10.1016/j.apenergy.2016.06.094
Mi, Z., Zheng, J., Green, F., Guan, D., Meng, J., Feng, K., et al. (2021). Decoupling without outsourcing? How China’s consumption-based CO2 emissions have plateaued. iScience 24:103130. doi: 10.1016/j.isci.2021.103130
Mi, Z., Zheng, J., Meng, J., Ou, J., Hubacek, K., Liu, Z., et al. (2020). Economic development and converging household carbon footprints in China. Nat Sustain 3, 529–537. doi: 10.1038/s41893-020-0504-y
Minx, J., Baiocchi, G., Wiedmann, T., Barrett, J., Creutzig, F., Feng, K., et al. (2013). Carbon footprints of cities and other human settlements in the UK. Environ. Res. Lett. 8:035039. doi: 10.1088/1748-9326/8/3/035039
Moran, D., Kanemoto, K., Jiborn, M., Wood, R., Többen, J., and Seto, K. C. (2018). Carbon footprints of 13\hspace0.167em000 cities. Environ. Res. Lett. 13:064041. doi: 10.1088/1748-9326/aac72a
National Bureau of Statistics of China. (2022). Information disclosure of the National Bureau of statistics. Available at: http://www.stats.gov.cn/xxgk/jd/sjjd2020/201901/t20190122_1764776.html (Accessed October 17, 2022).
Oda, T., Maksyutov, S., and Andres, R. J. (2018). The open-source data inventory for anthropogenic CO2, version 2016 (ODIAC2016): a global monthly fossil fuel CO2 gridded emissions data product for tracer transport simulations and surface flux inversions. Earth Syst. Sci. Data 10, 87–107. doi: 10.5194/essd-10-87-2018
Ottelin, J., Ala-Mantila, S., Heinonen, J., Wiedmann, T., Clarke, J., and Junnila, S. (2019). What can we learn from consumption-based carbon footprints at different spatial scales? Review of policy implications. Environ. Res. Lett. 14:093001. doi: 10.1088/1748-9326/ab2212
Pang, M., Meirelles, J., Moreau, V., and Binder, C. (2019). Urban carbon footprints: a consumption-based approach for Swiss households. Environ. Res. Commun. 2:011003. doi: 10.1088/2515-7620/ab59c5
Park, H.-C., and Heo, E. (2007). The direct and indirect household energy requirements in the Republic of Korea from 1980 to 2000—an input–output analysis. Energy Policy 35, 2839–2851. doi: 10.1016/j.enpol.2006.10.002
Qian, Y., Zheng, H., Meng, J., Shan, Y., Zhou, Y., and Guan, D. (2022). Large inter-city inequality in consumption-based CO2 emissions for China’s pearl river basin cities. Resour. Conserv. Recycl. 176:105923. doi: 10.1016/j.resconrec.2021.105923
Schubert, J., Wolbring, T., and Gill, B. (2013). Settlement structures and carbon Emissions in Germany: the effects of social and physical concentration on carbon Emissions in Rural and Urban residential areas. Environ. Policy Gov. 23, 13–29. doi: 10.1002/eet.1600
Shan, Y., Guan, D., Hubacek, K., Zheng, B., Davis, S. J., Jia, L., et al. (2018). City-level climate change mitigation in China. Science. Advances 4:eaaq0390. doi: 10.1126/sciadv.aaq0390
Shao, L., Li, Y., Feng, K., Meng, J., Shan, Y., and Guan, D. (2018). Carbon emission imbalances and the structural paths of Chinese regions. Appl. Energy 215, 396–404. doi: 10.1016/j.apenergy.2018.01.090
Sun, M., Chen, G., Xu, X., Zhang, L., Hubacek, K., and Wang, Y. (2021). Reducing carbon footprint inequality of household consumption in Rural areas: analysis from five representative provinces in China. Environ. Sci. Technol. 55, 11511–11520. doi: 10.1021/acs.est.1c01374
The State Council of China. (2022) Opinions on completely, accurately and comprehensively implementing the new development concept and doing a good job of carbon peak and carbon neutralization. Available at: http://www.gov.cn/zhengce/2021-10/24/content_5644613.htm (Accessed October 17, 2022).
Tukker, A., Bulavskaya, T., Giljum, S., de Koning, A., Lutter, S., Simas, M., et al. (2016). Environmental and resource footprints in a global context: Europe’s structural deficit in resource endowments. Glob. Environ. Chang. 40, 171–181. doi: 10.1016/j.gloenvcha.2016.07.002
Wang, S., Fang, C., Sun, L., Su, Y., Chen, X., Zhou, C., et al. (2019). Decarbonizing China’s urban agglomerations. Ann. Am. Assoc. Geogr. 109, 266–285. doi: 10.1080/24694452.2018.1484683
Wiedenhofer, D., Guan, D., Liu, Z., Meng, J., Zhang, N., and Wei, Y. M. (2017). Unequal household carbon footprints in China. Nat. Clim. Change 7, 75–80. doi: 10.1038/nclimate3165
Wu, S., Lei, Y., and Li, S. (2019). CO2 emissions from household consumption at the provincial level and interprovincial transfer in China. J. Clean. Prod. 210, 93–104. doi: 10.1016/j.jclepro.2018.10.356
Yang, S., Fath, B., and Chen, B. (2016). Ecological network analysis of embodied particulate matter 2.5 – a case study of Beijing. Appl. Energy 184, 882–888. doi: 10.1016/j.apenergy.2016.04.087
Yang, Y., and Yang, S. (2020). Are industrial carbon emissions allocations in developing regions equitable? A case study of the northwestern provinces in China. J. Environ. Manag. 265:110518. doi: 10.1016/j.jenvman.2020.110518
Yu, X., Wu, Z., Zheng, H., Li, M., and Tan, T. (2020). How urban agglomeration improve the emission efficiency? A spatial econometric analysis of the Yangtze River Delta urban agglomeration in China. J. Environ. Manag. 260:110061. doi: 10.1016/j.jenvman.2019.110061
Zhang, X., and Wang, Y. (2017). How to reduce household carbon emissions: a review of experience and policy design considerations. Energy Policy 102, 116–124. doi: 10.1016/j.enpol.2016.12.010
Zhang, Y., Wang, H., Liang, S., Xu, M., Liu, W., Li, S., et al. (2014). Temporal and spatial variations in consumption-based carbon dioxide emissions in China. Renew. Sust. Energ. Rev. 40, 60–68. doi: 10.1016/j.rser.2014.07.178
Zhao, J., Cohen, J. B., Chen, Y., Cui, W., Cao, Q., Yang, T., et al. (2020). High-resolution spatiotemporal patterns of China’s FFCO2 emissions under the impact of LUCC from 2000 to 2015. Environ. Res. Lett. 15:044007. doi: 10.1088/1748-9326/ab6edc
Keywords: consumption carbon emission, carbon emission per capita, urban and rural area, carbon neutrality, multidimensional analysis
Citation: Xu F, Zheng X, Zheng M, Liu D, Ma Y, Wang T and Han X (2023) Fine mapping and multidimensional analysis of carbon emission reduction in China. Front. Ecol. Evol. 11:1163308. doi: 10.3389/fevo.2023.1163308
Edited by:
Guojie Wang, Nanjing University of Information Science and Technology, ChinaCopyright © 2023 Xu, Zheng, Zheng, Liu, Ma, Wang and Han. This is an open-access article distributed under the terms of the Creative Commons Attribution License (CC BY). The use, distribution or reproduction in other forums is permitted, provided the original author(s) and the copyright owner(s) are credited and that the original publication in this journal is cited, in accordance with accepted academic practice. No use, distribution or reproduction is permitted which does not comply with these terms.
*Correspondence: Xinqi Zheng, emhlbmd4cUBjdWdiLmVkdS5jbg==