- 1Institute of Highland Forest Science, Chinese Academy of Forestry, Kunming, China
- 2Pu'er Forest Ecosystem Research Station, National Forestry and Grassland Administration of China, Kunming, China
Microbial communities, which are affected by soil types and climate factors, contribute to maintain the function of terrestrial ecosystems. Recent studies have shown that interdomain relationships in below–aboveground communities may contribute greatly to ecosystem functioning. However, the responses of interactions among plant, soil fungal, and bacterial communities to the change of woodland use and their effects on ecosystem multifunctionality (EMF) remain poorly understood. In this study, the plant–microbe and fungi–bacteria interdomain ecology network (IDEN) based on SparCC pairwise associations were constructed by simultaneous aboveground plant surveys and belowground microbial analyses among four different woodland use intensities (WUI) along different seasons. The effects of different seasons on these relationships were surveyed to probe into the links to EMF. With the increase of woodland use intensity, the plant–microbe network complexity decreased, while the fungus–bacteria network complexity increased. In both dry and wet seasons, ecosystem multifunctionality decreased with the increase of woodland use intensity. Some tree species are the network hubs and may play a pivotal role in the community structure stability of the forest ecosystem. During the dry season, WUI could indirectly affect EMF through plant–microbial network complexity. During the wet season, WUI had a direct effect on EMF. WUI also indirectly affected EMF through plant–microbial network complexity and fungus–bacterial network complexity. Air temperature is the main climatic factor for EMF in the dry season, while soil moisture content is the climatic factor for EMF in the wet season. Our study revealed the important role of the relationship between plants and their associated soil microbial communities (IDENs) in maintaining ecosystem processes and function. Investigating the recovery dynamics of inter-domain ecological networks after extreme disturbances is important for understanding the overall development of ecosystems.
1 Introduction
The change of woodland use is the main drivers of global biodiversity loss. Losses from global change affect humans by simultaneously affecting soil microbial communities and plant diversity, thereby affecting multiple ecosystem functions and services (Zeller et al., 2017; Klaus et al., 2018). The change of woodland use can cause disturbance by changing vegetation coverage and promote soil erosion and disturbance, all of which can affect the microbial communities’ composition (Goss-Souza et al., 2019). Previous studies indicated that different woodland use intensity (WUI) can jointly regulate soil microorganisms (bacteria and fungi) via plant species diversity and soil properties (Li et al., 2022), but the understanding of how the interdomain interactions between plant and microbial domains contribute to the overall functioning of ecosystems remains limited. In the study of ecological networks, we found that WUI could affect the ecosystem multifunctionality by influencing fungal network and bacterial network through plant and microbial diversity (Li et al., 2022). Hence, this study further studied the effect of ecological networks between interdomains (between plants and microorganisms and between fungi and bacteria) on the ecosystem multifunctionality.
In terrestrial ecosystems, the dynamics of interactions between microbes and plant hosts play a critical role in sustaining ecosystem functions. The patterns of changes in plant community composition, soil microbial community structure, and composition measures are increasingly being used to assess ecosystem responses to anthropogenic disturbances and to provide indicators of ecosystem recovery (Cui et al., 2021). In most instances, microbial communities (bacteria and fungi) are important mediators of biogeochemical processes and play essential roles in the establishment of plant communities, which makes knowledge about their recovery after extreme disturbances valuable for understanding ecosystem development (Sun and Badgley, 2019). Plant microbiomes are the most effective association group in higher organism microbiome that actively participates in balancing ecosystem functions (van der Heijden and Hartmann, 2016). The existence of multiple microbial consortiums in relation to plants contributes to increasing or restoring plant ecosystem productivity by carbon and water cycles, nutrient capture, crop production, and carbon uptake and storage, improving plant responses to various environmental stressors, and mitigating the effect of climate change by regulating ecosystem to extend soil carbon storage (Ahkami et al., 2017). Various soil microbial and plant species interact with each other to eventually form complex networks in forest ecosystems (Zhou et al., 2011; Toju et al., 2014). Ecological network analysis can clarify community composition, predict community structure stability, disclose ecological processes, and provide insights into the discovery of keystone species and the complex interactions of species (Layeghifard et al., 2016). Nevertheless, there are few studies on the coexistence of aboveground plants and belowground soil microorganisms. In order to explore the relationships among plants, fungi, and bacteria, Feng et al. (2019) used plant distribution survey data and microbial high-throughput sequencing data to construct interdomain ecological networks (IDENs) based on the abundance data set constructed by paired correlation calculation (SparCC and SPIECEASI) and utilizing correlation-based methods to derive the correlation between plants and microorganisms. Therefore, this study revealed the interaction patterns between plants and microorganisms through the interdomain ecological network and clarified the key species that affected microorganisms and the impact of the interdomain ecological network on ecosystem function under different intensities of woodland use.
Climate change (e.g., warming and wetting) is threatening ecosystem functioning and biodiversity worldwide (Walther, 2010). Monsoon evergreen broad-leaved forest is an important zonal vegetation type in subtropical areas of southern China. The study area of Yunnan Province has a warm and humid subtropical monsoon climate with distinct dry and wet seasons (Li et al., 2022). Microbial communities play an important role in regulating organic matter degradation and nutrient mobilization (Chaparro et al., 2012). An increase in precipitation and soil temperature going from the dry to the rainy season may lead to compositional changes in soil microbial community composition (Smith et al., 2015). Due to climate change, abiotic factors such as drought, high temperature, and salinity have a great impact on natural vegetation (Rezzonico et al., 2017). Elevated temperature and precipitation improve plant growth, causing more root exudation and litter input (Zhou et al., 2012; Jing et al., 2015). Plants and microorganisms form a spectrum of relationships such as mutualism, commensalism, and parasitism, which overcome the mentioned abiotic stresses and improve productivity (Rajkumar et al., 2013; Ahkami et al., 2017). However, the complex interactions network within microbial communities and plants in varying woodland use types under climate change are still unknown and difficult to predict. Therefore, this study analyzed the effects of different woodland use intensities on microorganisms and the effect of the interdomain ecological network on ecosystem multifunctionality in dry and wet seasons. Hence, comprehending the relationship between interdomain ecological networks and climate conditions in different woodland use types can predict the regulation of plant and microbial processes under global climate change and formulate vegetation restoration plans.
Urbanization and the growth of population have led to severe deforestation and degradation of the original evergreen broad-leaved forests. Therefore, with increasing human utilization, most forests have degraded into secondary forests, shrubs and grassland, or even bare ground (Song, 2007). Therefore, the objective of this study was to determine the effects of the dry and wet seasons on the ecosystem multifunctionality of interdomain plant–microbe and fungi–bacteria ecological networks under different woodland use intensities and to identify the key species. We tested the following hypotheses: (i) transformation of monsoon evergreen broad-leaved forest to other vegetation types reduces EMF in dry and wet seasons; (ii) the complexity of interdomain ecological networks (plant–microbe, microbe–microbe) has a significant impact on EMF, and in dry and wet seasons, woodland use intensity affected EMF through interdomain ecological networks; (iii) the effects of climate factors on interdomain ecological networks and EMF differ in dry and wet seasons (Figure 1).
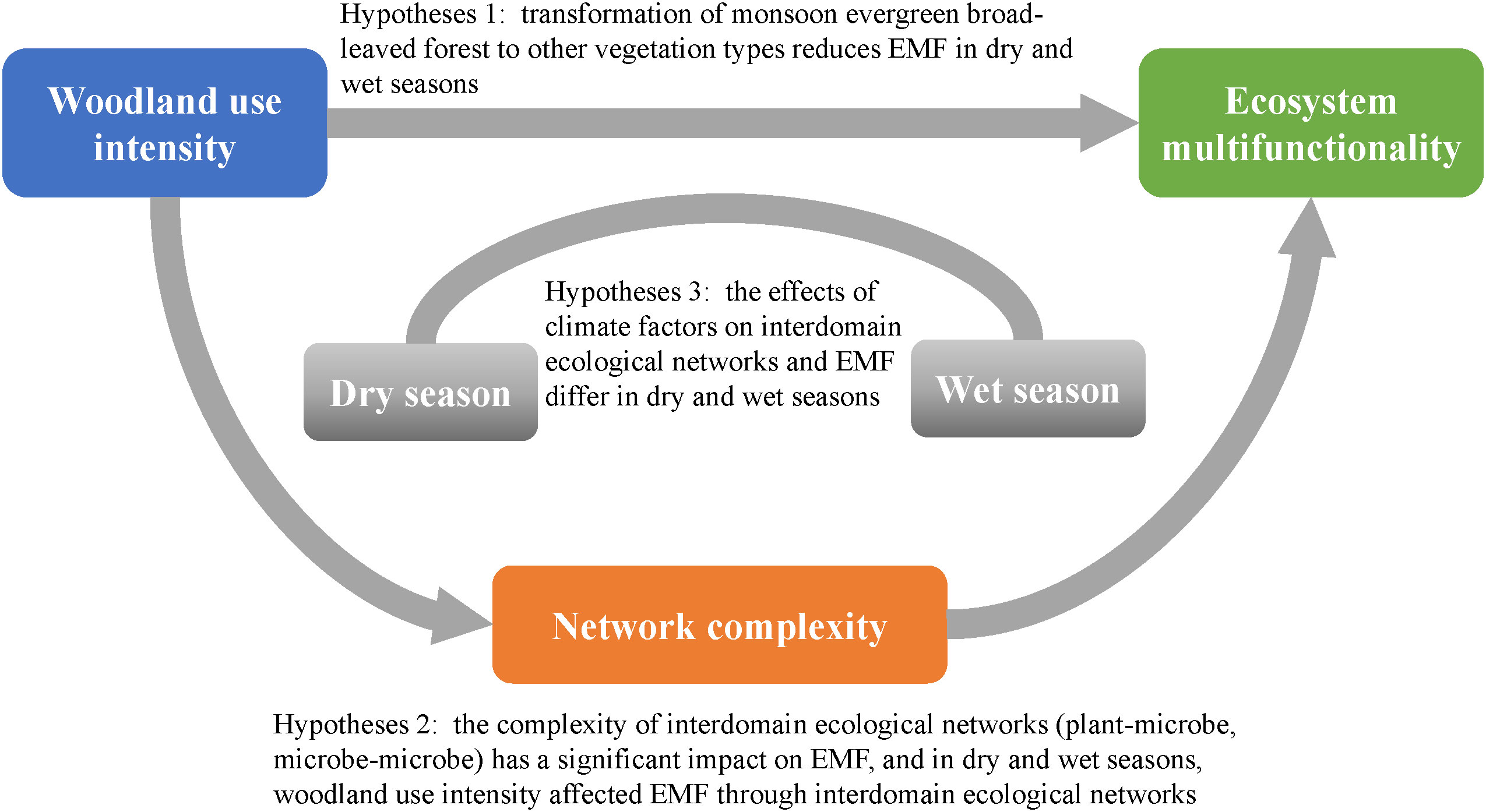
Figure 1 The conceptual framework for testing the hypotheses. First, quantify the intensity of woodland use, and then clarify the differences of network complexity and EMF among different woodland use intensity. Find out whether the intensity of woodland use affects EMF through network complexity. The effects of woodland use intensity on EMF in different seasons are revealed.
2 Materials and methods
2.1 Study sites and sample collection
The study area is located in Yixiang Township (22°32′N–22°52′N, 100°56′E–101°26′E), Pu’er City, Yunnan Province, Southwest China, with an altitude range of 800–1,964 m (Li et al., 2022). This study area has a warm and humid subtropical monsoon climate and distinct dry and wet seasons. Accordingly, samples were collected on March 3 (dry season) and August 23 (wet season) in 2021, respectively. The soil here is classified as Alfisols according to the United States Department of Agriculture soil classification system (Soil Survey Staff, 2014). Woodland use intensity was represented by the input and output of the ecosystem (Wen et al., 2020; Li et al., 2022), and the specific division of woodland use intensity refers to Li et al. (2022).
2.2 Soil sampling and ecosystem function measurements
Three types of woodland uses were elected: Pinus kesiya forest, shrub and grassland, and coffee plantation, all of which are at different levels of WUI arrived at through the human conversion of monsoon evergreen broad-leaved forests. The monsoon evergreen broad-leaved forests consisted of undisturbed native vegetation (). A secondary Pinus kesiya forest, naturally regenerated following selective logging of the primary forest in the 1940s, was considered a light disturbance () woodland use type; shrub and grassland communities formed by grazing of secondary forests in the 1980s were deemed an intermediate disturbance () type; coffee plantation established from secondary forests around the year 2000 and subsequently under continuous management represented a severe disturbance () type. The coffee company has unified management of all the plots of the coffee plantation (Li et al., 2022). In our previous study (Li et al., 2022), principal component analysis (PCA) was performed for all forest plots based on four parameters: Intensity (the percentage of biomass loss caused by the disturbance events), forest age, aboveground biomass, and species richness, and WUI index was expressed as reciprocal PCA axis 1 score (Wen et al., 2020) (Supplementary Table 1).
The four types of woodland use have the same soil type and climate conditions. These four forest land use types were more than 1.0 km from each other. Each region had 10 representative plots of 30 m × 30 m (total 40 plots), each plot spaced at least 20 m apart.
The data for the dry and wet seasons were collected in March 2021 and August 2021, respectively. The species identity, height, and diameter at breast height (DBH) of woody plants in each plot (DBH ≥ 1 cm) were recorded. We also recorded the species names and plant coverage of non-woody species. Five 0–10 cm topsoil samples were randomly collected along the diagonal of each plot. After removing the litter, roots, and other visible matter, the samples were screened by mixing evenly through a 2 mm sieve in each plot. Each composite soil sample was carried to the laboratory at −4°C, where a portion of it was immediately frozen at –20°C for its later microbiological analysis. The surplus soil was divided into two equal parts: one part was placed in a freezer (−4°C) for enzyme activity analysis, and the other part was dried for soil physical and chemical properties analysis (Ma et al., 2021).
Under a wide range of ecosystem services, this study quantified twelve recognized ecosystem functions of soil organisms. These consisted of nutrient supplying (soil organic C, N, P and K), material decomposition (extracellular enzyme activity associated with sugar degradation (β-glucosidase), nitrogen source (urease), and P mineralization (acid phosphatase)), and soil water cycle (maximum water holding capacity) (Supplementary Figure 1). Soil pH (soil : water ratio = 1:2.5) was measured using a pH meter (FE20K, Mettler–Toledo, Switzerland). Soil total nitrogen (TN) and soil organic carbon (SOC) were determined using a CHN elemental analyzer (2400 II CHN Elemental Analyzer, Boston, MA, USA). Determination of total phosphorus (TP) and available phosphorus (AP) was conducted using molybdenum antimony blue colorimetry and the olsen method. Hydrolysable nitrogen (HN) was determined by alkaline hydrolysis diffusion method (Bao, 2000). Total potassium (TK) and available potassium (AK) was determined by atomic absorption photometry (Bao, 2000). Urease (UR) activity was measured with 10% urea and citrate buffer (pH: 6.7, 37°C in 24 h), and release was measured with indophenol (Kandeler and Gerber, 1988). In order to determine acid phosphatase (APH) activity, soil subsamples were mixed with di-sodium phenyl phosphate solution in an acetate buffer (pH: 5.0, 37°C in 1 h). Next, the acid phosphatase activity was determined in terms of μmol pNPP kg–1 soil h–1 (Tabatabai, 1994). To determine β-glucosidase (BG) activity, soil subsamples were extracted with a sodium acetate buffer (50 mmol L–1). The soil cores measuring soil moisture content (SMC) and maximum water holding capacity (MWHC) were all determined by the core cutter method, following the procedures described in Duan et al. (2018).
Multifunctionality is the multidimensional metric of ecosystem functioning. This indicator quantifies the ability of an ecosystem to provide multiple ecosystem processes and services simultaneously (Manning et al., 2018). The quantitative multifunctional index of each plot was obtained following Manning et al. (2018)’s method. This method calculates ecosystem multifunctionality by assigning different weights to different ecosystem functions by the following three steps: (1) the clustering of closely related functions was determined using the cluster analysis of 12 ecosystem functions data, (three clusters were found to be the appropriate numbers); (2) quantification according to the threshold method (Byrnes et al., 2014), and the functional measurements were standardized according to the regional standardized maximum values of each ecosystem type prior to the analysis; (3) each cluster was given equal weight, and the functions within each cluster were also assigned an equal weight (e.g., if the cluster contains four functions, each weight was 0.25) in a threshold-based measurement to avoid the overweighting of some aspects of overall ecosystem functions (Manning et al., 2018).
2.3 Molecular analysis of soil microorganisms
Soil DNA was extracted using the NucleoSpin 96 Soil kit (Macherey-Nagel GmbH and Co. KG, Düren, Germany). Amplification of internal transcribed spacer (ITS) of fungi using the primers ITS1F (5′–CTTGGTCATTTAGAGGAAGTAA–3′) and ITS2 (5′–GCTGCGTTCTTCATCGATGC–3′) (Gomez-Montano et al., 2013). The primers included 338F (5’–ACTCCTACGGGAGGCAGCA–3’) and 806R (5’–GGACTACHVGGGTWTCTAAT–3’) for amplifying the V3–V4 region of bacterial 16S rRNA gene (Xu et al., 2017).
PCRs were performed with 50 μL reaction mixture, including 0.2 μL of Q5 High-Fidelity DNA polymerase, 10 μL of High GC Enhancer, 1 μL of dNTP, 10 μM of each primer, 50 ng of genomic DNA, and 10 μL of buffer. The thermal cycle conditions were as follows: 95°C for 5 minutes, followed by 25 cycles of 95°C for 30 seconds, 50°C for 30 seconds, and 72°C for 40 seconds, and finally extended at 72°C for 7 minutes. The amplicon sequencing was proceeded externally, on the Illumina MixSeq2500 platform of Beijing Biomarker Technologies Corporation. In the first step, the sequenced Raw Reads were filtered by Trimmomatic v0.33 software. In the second step, cutadapt 1.9.1 software was used to identify and remove primer sequences to obtain Clean Reads without primer sequences. Third, Clean Reads were spliced in Usearch v10 software, and the length of the data was filtered. In the fourth step, chimeric sequences were identified and removed in UCHIME v4.2 software to obtain final Effective Reads. Reads were clustered using USEARCH software at the 97.0% similarity level, and the taxon (OTU) of all sequenced sequences was filtered using the 0.005% threshold. The bacterial 16S database was SILVA and the fungal ITS database was UNITE (Bokulich et al., 2013; Edgar, 2013). QIIME software was used to generate species abundance tables at different taxonomic levels.
2.4 Interdomain ecological network construction and the complexity of network
Interdomain ecology network analysis was finished on the Galaxy-IDENAP platform2 (Feng et al., 2019). SparCC is a correlation-based method for inferring potential associations between plants and microorganisms (Friedman and Alm, 2012). We excluded data with an absolute correlation coefficient of less than 0.3. The SparCC results were filtered and the data were saved according to the p< 0.05 value. Based on the observed IDENs, network topology, module detection, and random network were analyzed based on the observed IDENs. and the salience of the observed IDEN and random network topological characteristics was compared (Deng et al., 2012). The network was visualized using the Gephi (0.9.2) (Bastian et al., 2009). The single sample t-test was used to determine the significance between observed and random networks. All analyses were executed using “bipartite” and “ggplot2” packages in R (4.1.3).
The microbial co-occurrence networks were created for four levels of WUI by using fungal and bacterial OTUs or “species” matrices estimated at the plot level. To reduce the low abundance and rare OTUs from the dataset, they were filtered out before the network is constructed. These OTUs presented in fewer than 10 sampling plots of each level of WUI, accounting for <0.01% of the total reads, were removed. Based on the random matrix theory (RMT) method, all pairwise correlation scores of the co-occurrence network were measured by using Spearman’s correlation and Jaccard’s dissimilarity measure (Deng et al., 2012; Yuan et al., 2021). The OTUs with within-module connectivity >2.5 and inter-module connectivity >0.62 were selected as network hubs (keystone species) (Zhou et al., 2011). The network properties were obtained by the “igraph” package (Csardi and Nepusz, 2006). The network topological features of each plot were carried out in the subgraph function through the “igraph” package as described by Ma et al. (2016). Visualization of co-occurrence networks was performed by Gephi (Bastian et al., 2009).
The procured site-level network meta-matric was used for subset networks matric (Ma et al., 2016; Wagg et al., 2019) by preserving the OTUs in the graph and all the edges between them in the site-level network for each sampled graph. The following subnetworks were used to figure the complexity of plot-level bacterial and fungal networks, represented by the number of nodes and links and linkage density (links per OTU) (Banerjee et al., 2019; Wagg et al., 2019). Since these topological parameters (indicating network complexity) were closely related, linkage density (number of links/nodes) (Wagg et al., 2019) was used to represent the network complexity index.
2.5 Data analysis
We tested spatial autocorrelation in averaged forest multifunctionality using Moran’s I test (Yuan et al., 2020), but did not find any strong spatial autocorrelation for any of the distance classes. Through two-way analysis of variance, we examined the effects of season and WUI on soil ecosystem functions, EMF, and the complexity of co-occurrence networks. Before the analysis, the homoscedasticity of variances and the normality of data were tested, with a log or log (x + 1) transformation of the data used as needed. We also used the two-way ANOVA to examine the effect of WUI between seasons (Qiu et al., 2021). The relationship between EMF and diversity was established by linear regression analysis as well as the parameters derived for the microbial ecological networks. Pearson correlation analysis was performed between ecosystem functions and network complexity. The Pearson correlation and linear regression analyses were implemented using SPSS 23 software and R 4.1.3 (http://www.r-project.org/) (R Development Core Team, 2019).
Structural equation modeling (SEM) was implemented to systematically understand the direct and indirect relationships between network complexity (fungi–bacteria and plant–microbe network complexity) and average multifunctionality. We also performed a random forest analysis (RF) to determine the main driving factors., We used their respective percentage increase in mean squared error (MSE) to estimate the relative importance of variables: a higher MSE% value implies a more important variable. This analysis was performed in R “rfPermute” package (Archer, 2013).
3 Results
3.1 Interdomain ecology network topological characterization and network differences of plant–microbe and fungi–bacteria associations
We constructed a total of 16 IDENs from fungi and bacteria in different seasons using plant richness datasets and high-throughput sequencing (Figure 2, Supplementary Tables 2, 3). There were differences in the relationship between plants and microorganisms in the four forest types. The number of network modules decreased with the increase of woodland use intensity in dry and wet seasons (Supplementary Table 2). In plant–microbe networks in dry and wet seasons, the number of plants, the number of OTUs, total links, web asymmetry, and links per species in shrub-grassland and coffee plantations were lower than those in natural forest and Pinus kesiya forest. However, their connectance, clustering coefficient, and nestedness were higher than the natural forest and Pinus kesiya forest.
The network topological properties of the four woodland use types varied substantially, indicating that the network organization is obviously different (Supplementary Tables 2, 3). In the dry season, the total number of nodes and links of the fungi–bacteria network was lower than natural forest; In the wet season, the number of nodes and links in shrub-grassland and coffee plantations was higher than in the natural forest (Supplementary Table 3). The specialization asymmetry and links per species in shrub-grassland and coffee plantations were higher than in the natural forest and Pinus kesiya forest.
The interdomain ecological network analysis revealed that the plant keystone species that associated with microbes in during the dry season were Fagaceae (Castanopsis calathiformis), Theaceae (Eurya groffii), Fabaceae (Craspedolobium unijugum). The plant species that interacted with microbes in during the dry season was Theaceae (Schima wallichii), Fagaceae (Lithocarpus truncates, Lithocarpus fenestratus), Lauraceae (Machilus pingii), Oleaceae (Fraxinus floribunda), Ericaceae (Craibiodendron stellatum, Vaccinium exaristatum), Pinaceae (Pinus kesiya), Araliaceae (Schefflera chinensis), Elaeocarpaceae (Elaeocarpus sylvestris), Staphyleaceae (Turpinia montana), and Anacardiaceae (Toxicodendron succedaneu). In the wet season, the key species of plants were only Fagaceae (C. calathiformis) and Myrsinaceae (Rapanea neriifolia), while the key species of plants were only Theaceae (Anneslea fragrans) Lauraceae (M. pingii) (Figures 2A, B, Supplementary Table 4). However, there were only module hubs but no network hubs in the plant–microbial ecological network of shrub-grass and plantations, which showed that arbor played a dominant role in the plant and the microbial ecological network. With the increase in woodland use intensity, the decrease in tree species had a greater impact on the key species in the ecological network.
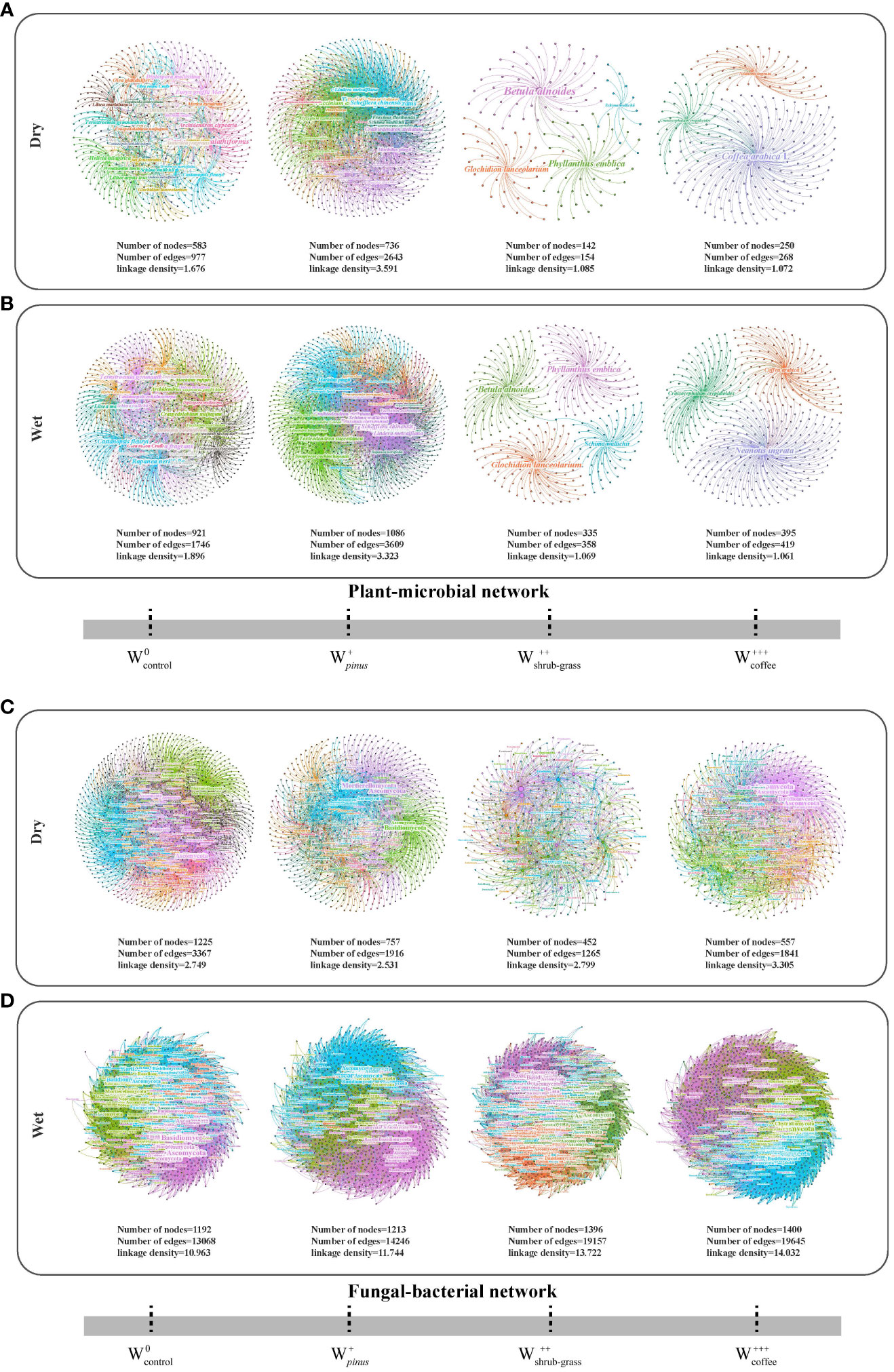
Figure 2 The plant–microbial (A, dry; B, wet) and fungal–bacterial (C, dry; D, wet) network architecture. For each plot network, plants and microbes within the same module are represented in the same color, and different colors represent different modules. Node size are related to node degree. , no disturbance (undisturbed monsoon evergreen broad-leaved forest); , light disturbance (Pinus kesiya forest); , intermediate disturbance (shrubs and grasses under grazing); , severe disturbance (coffee plantations).
In the fungi–bacteria network, fungi were found in the network hubs, including Ascomycota, Basidiomycota, Unclassified, Mortierellomycota, Rozellomycota, and Chytridiomycota, except for Pinus kesiya forest in the dry season. However, the key species of shrub and grass in the dry season was the bacterial Chloroflexi in addition to the fungal Ascomycota (Figures 2C, D, Supplementary Table 5). Most of the main network hubs in the shrub and grassland–microbial network in the dry season were fungi and bacteria, while the network hubs of the other woodland use types were fungi.
VPA analysis revealed that key species played an important role in the ecosystem (Figure 3). The key species were different in four woodland use intensities. In the dry season, the variance explained by the key species of the fungus–bacterial interdomain network was 3.43%–7.04%, which was higher than that before the change of the land use (0.3%), but in the wet season, the devoting rates of the key species was 0.66% ~ 10.98%, lower than that before the conversion (33.45%).
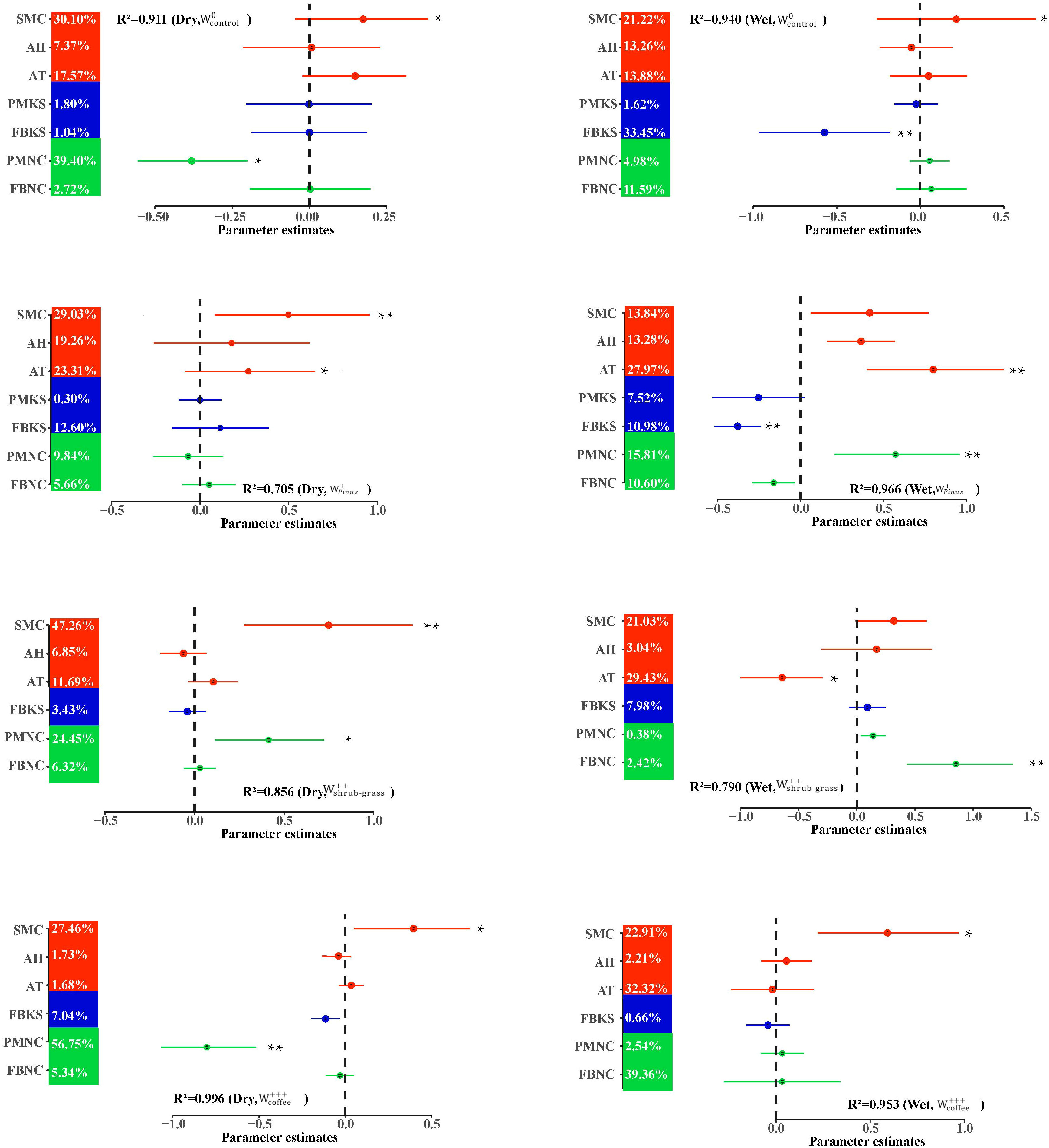
Figure 3 Effect of abiotic and biotic factors on ecosystem multifunctionality during different woodland use intensity in dry and wet season. The percentage of explained variance represents the average parameter estimate (standardized regression coefficient) of the model predictors. *P<0.05; **P<0.01. SMC, Soil moisture content; AT, Air temperature; AH, Air humidity; PMKS, Plant–microbe network keystone species; FBKS, fungi–bacteria network keystone species; PMNC, complexity of plant–microbe network; FBNC, complexity of fungi–bacteria network. , no disturbance (undisturbed monsoon evergreen broad-leaved forest); , light disturbance (Pinus kesiya forest); , intermediate disturbance (shrubs and grasses under grazing); , severe disturbance (coffee plantations).
3.2 Complexity of plant–microbe and fungi–bacteria and EMF relationships in the interdomain ecology network
Significant differences in network topological characteristics among different levels of WUI and between seasons were observed (Supplementary Tables 2, 3). The complexity inferred from network properties (numbers of nodes and edges) and the linkage density among taxa, all showed a consistent decrease with increasing WUI in the dry and wet seasons for the plant–microbial network. Conversely, the network complexity of the fungal–microbial network increased with increasing WUI in the dry and wet seasons (Figures 4A, B).
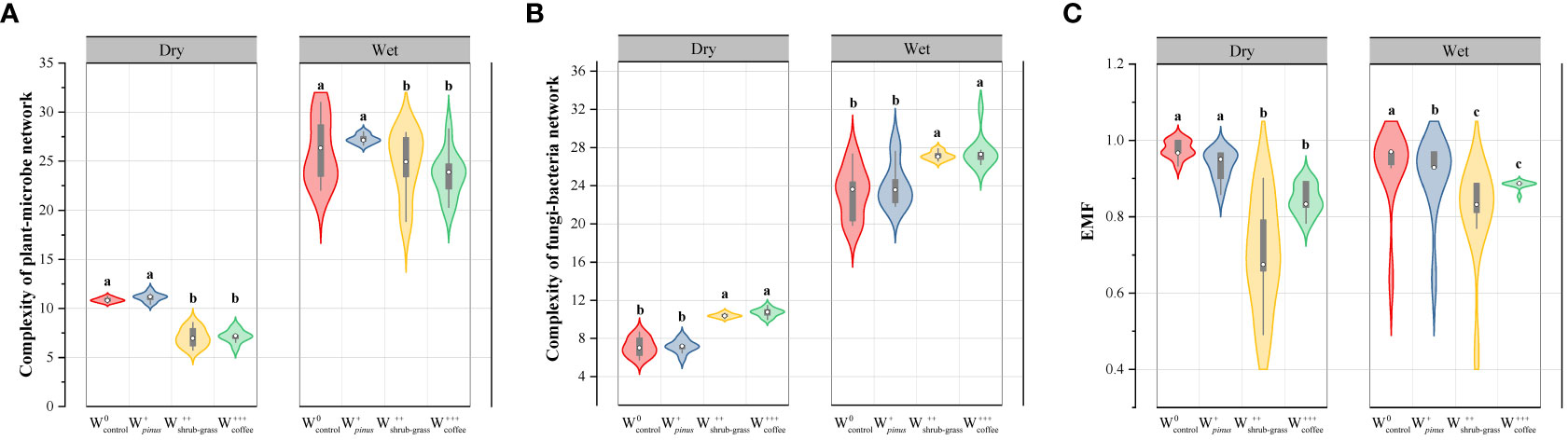
Figure 4 Violin plots of (A) complexity of plant–microbe network, (B) complexity of fungi–microbe network, and (C) EMF under different woodland use intensity in dry and wet season. Different letters on the violin plots figure significant differences (P< 0.05) among treatments. , no disturbance (undisturbed monsoon evergreen broad-leaved forest); , light disturbance (Pinus kesiya forest); , intermediate disturbance (shrubs and grasses under grazing); , severe disturbance (coffee plantations).
The change of woodland use led to significant alterations to soil functions and reduced soil multifunctionality in both seasons (Figure 4C, Supplementary Table 6). During the dry and wet seasons, the change of woodland use from forest to plantation significantly reduced Nutrient cycling, decomposition, water regulation, biomass, plant species richness, and EMF. Meanwhile, woodland use intensity had a greater impact than seasonal variation (Supplementary Table 6).
Using soil co-occurrence network, strong associations were observed among ecosystem multifunctionality of interdomain ecology network, and their relationship with multifunctionality (Figures 5A, B). In the dry and wet seasons, the complexity of the plant–microbial network had positive correlations with EMF (Figure 5A). In contrast, the complexity of the fungal–bacterial network was negatively correlated with EMF in the dry and wet seasons (Figure 5B). These results showed that there were significant differences among the microbial network patterns in different seasons microorganisms in relation to EMF. The change of woodland use and seasonal change significantly affected soil ecosystem functions.
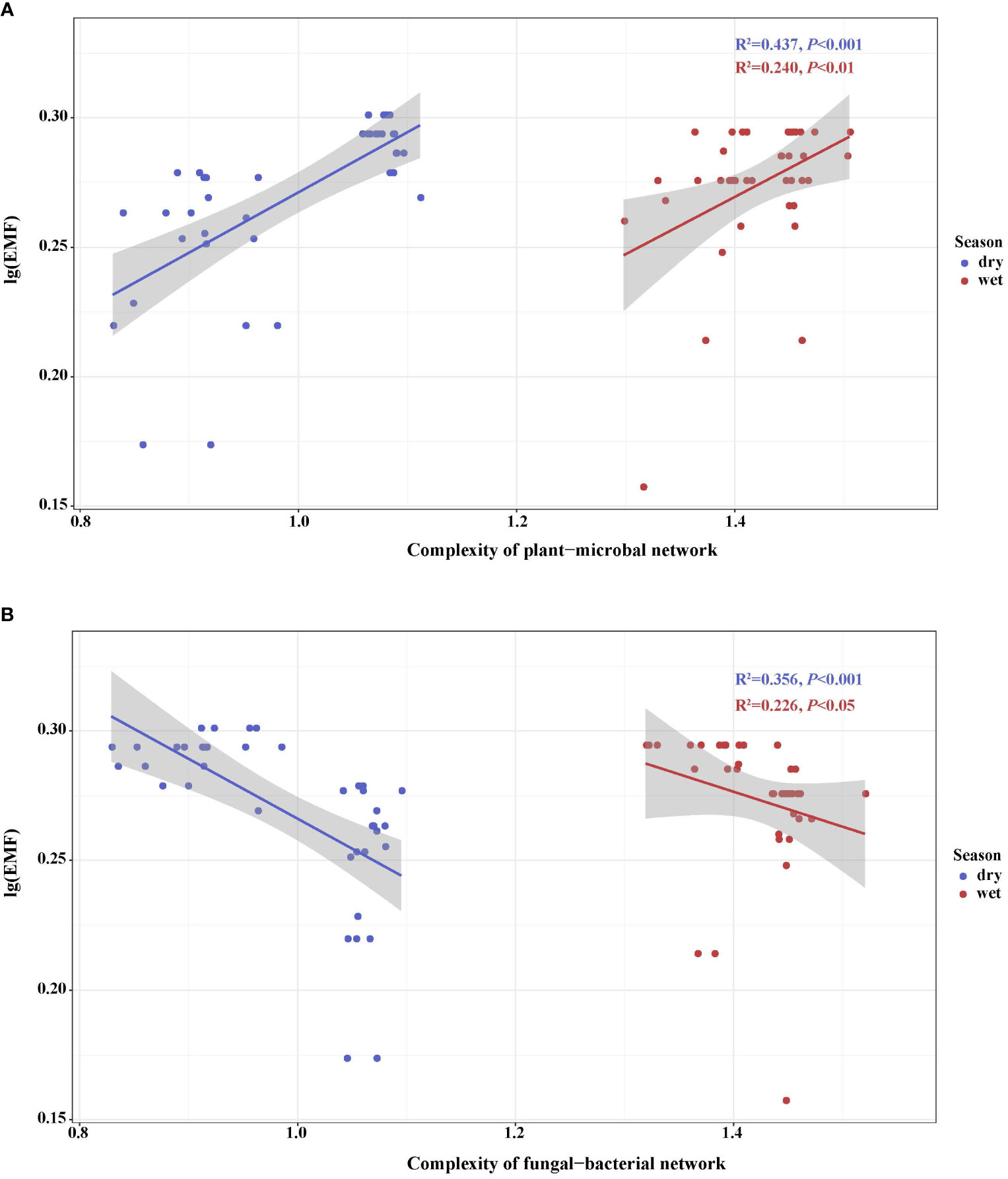
Figure 5 Regressions between ecosystem multifunctionality and complexity of plant–microbial network (A) and complexity of fungal–bacterial network (B) during different woodland use intensity in dry and wet season. Linear models and correlation coefficients are provided on each panel.
3.3 Effects of climate variables on interdomain ecology network in the dry and wet seasons
We used SEM to test whether EMF was maintained when accounting for the simultaneous and multiple drivers (such as microbial network). Firstly, we conducted EMF structural equation model analysis on woodland use intensity and microbial community network complexity (Figure 6). In the dry season, WUI had no direct negative effect on EMF, and affected EMF indirectly through the complexity of the plant–microbial networks. The complexity of the plant–microbial network had a positive effect on the dry season EMF. In the wet season, the complexity of the fungal–bacterial network had a negative effect on EMF, and the complexity of the plant–microbial network had a positive effect on EMF. The WUI had a direct effect on EMF but it indirectly affected EMF through the complexity of the plant–microbial and the fungal–bacterial network (Figure 6; Supplementary Table 7). Our random forest analysis indicated that the importance of WUI and the plant–microbial network complexity was significant in the dry season. The complexity of the fungi–bacteria network in the wet season also significantly affected EMF, while the plant–microbial network complexity was the highest in both the dry and wet seasons. The RF models further showed that WUI and soil network complexity were important and significant predictors. All of the above results indicated that the complexity of the plant–microbial network is the main driving factor of the dry and wet seasons.
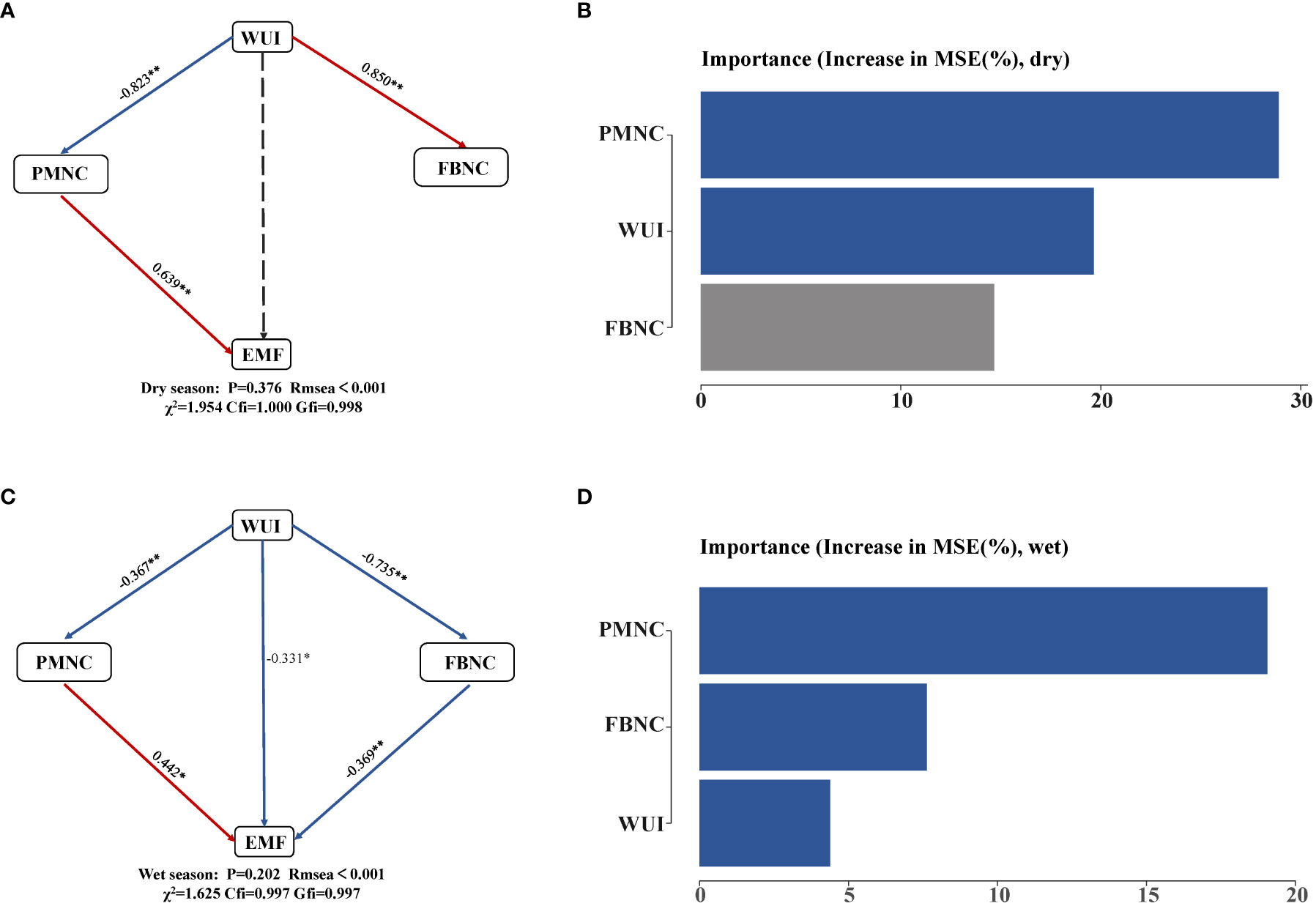
Figure 6 Links between network complexity and multifunctionality in dry and wet season. (A, C) SEM depicting the direct relationship between the complexity of soil microbiota and EMF. Red arrows represented positive paths, and blue arrows represented negative paths. Significance levels were as follows: *p< 0.05 and **p< 0.001. CFI, Comparative Fit Index. (B, D) The importance of random forest prediction for multifunctional influencing factors. PMNC, complexity of plant–microbe network; FBNC, complexity of fungi–bacteria network.
Different seasons had different climatic conditions affecting the interdomain ecology network (Figure 7). The rate of variation explained by air temperature, air humidity, and soil moisture content was calculated by the random forest model. It was found that air temperature (explained 47.2%) was the main climate variable affecting EMF in the dry season. The main climatic variable that affected EMF in the wet season was soil moisture content (explained 37.4%).
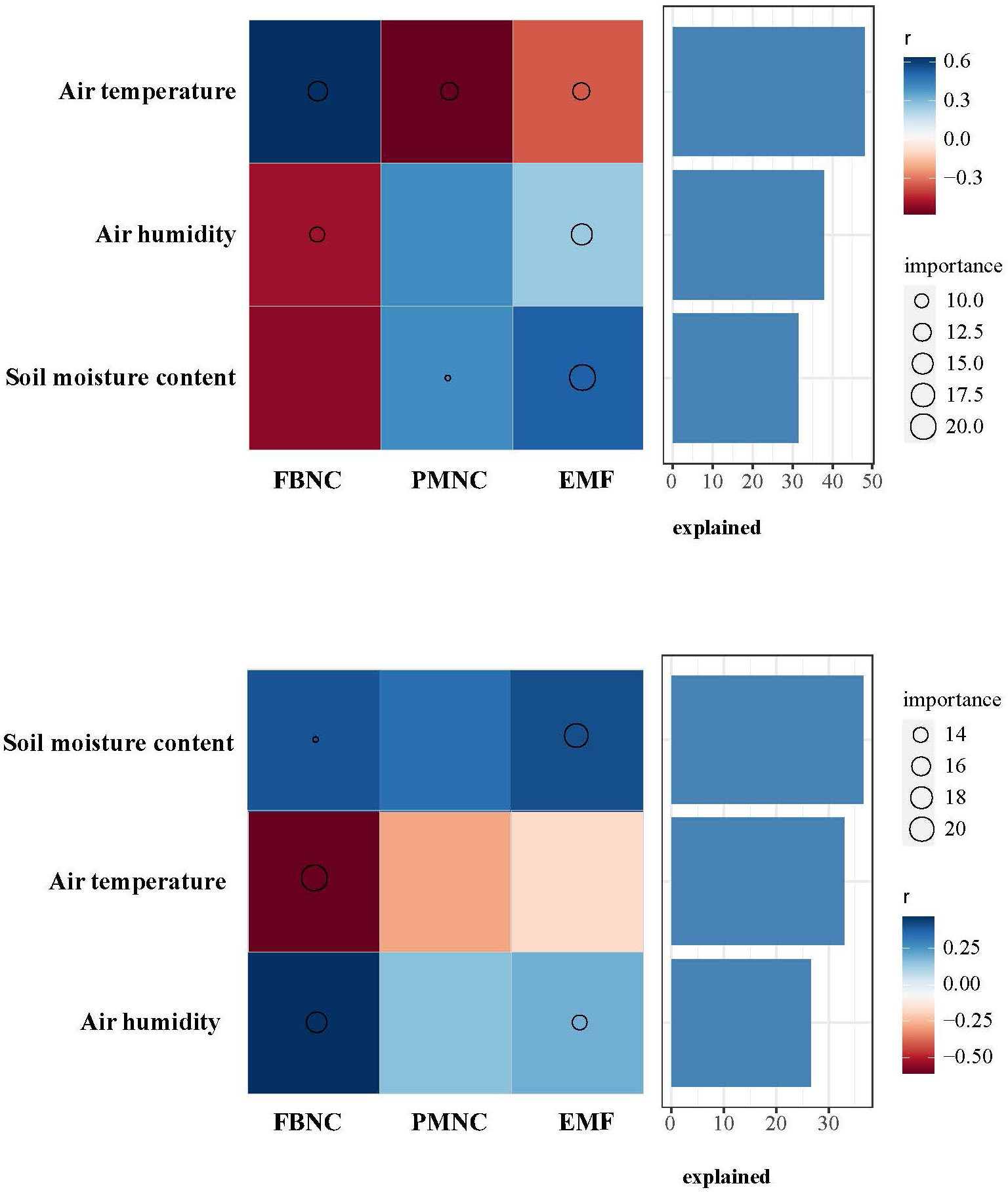
Figure 7 Random forest model was used to analyze the main climatic factors affecting soil microorganisms. PMNC, complexity of plant–microbe network; FBNC, complexity of fungi–bacteria network.
4 Discussion
Examining the responses of the interaction between aboveground and underground communities to different woodland use intensities and seasons can help us explore the mechanisms of forest ecosystem function changes under woodland use because this interaction is closely related to plant regeneration and nutrient transformation. It is well known that changes in forest land use have serious impacts on plant diversity, environmental quality, and soil fertility (Qin and Wang, 2018; Li et al., 2022), thereby affecting ecosystem functions and forest restoration. This study characterized the responses of soil fungi–bacteria and plant–microbe associations to ecosystem multifunctionality in woodland use changes and seasons, which will improve our understanding of the effects of different woodland use intensity on plant–microbe–soil continuity and help us find suitable forest management strategies that can maintain forest sustainability (Chen et al., 2021).
Our examination of two contrasting seasons across varying WUI revealed that the change of woodland caused a reduction in EMF; with the increase of woodland use intensity, ecosystem multifunctionality decreased in dry and wet seasons, supporting our first hypothesis. The function and composition of terrestrial ecosystem communities can be significantly altered by human activities, leading to significant modifications in multiple ecosystem functions (multifunctionality, EMF) (Fu et al., 2020). It is well known that land-use change results in the loss of nutrients, water availability, and soil functionality (Allan et al., 2014; Le Provost et al., 2020). However, our previous research also found that the change of woodland use reduces certain soil nutrients (C, N, P, and K), soil moisture, soil bulk density, and aboveground biomass. Such decreases in soil’s organic matter, nutrients, and moisture arising from woodland use may directly result in the loss of its functions, as reduced resource availability limits the metabolism and composition of microbial populations, thus undermining their contribution to and maintenance of ecosystem functionality (Delgado-Baquerizo et al., 2016; Chen et al., 2020).
Co-occurrence network analysis suggests direct and indirect competition or cooperation relationships among coexisting microbial taxa (Barberán et al., 2012; Xun et al., 2021). Our previous studies have found that woodland use can significantly effect EMF through interdomian ecological networks (fungus–fungi, bacteria–bacteria). Delgado-Baquerizo et al. (2020) have revealed that ecological networks and biodiversity play an important role in maintaining multiple functions required by global natural ecosystems. The complexity of networks is closely related to the stability of ecosystem functions (Zhu et al., 2021). We found that woodland use intensity can affect EMF through the interdomain ecological network, which is consistent with our second hypothesis. We also found that the complexity of the plant–microbe network decreased with the increase of woodland use intensity, while the complexity of the fungi–bacteria network increased with the increase of woodland use intensity, which may be related to the diversity because previous studies found that with the increase of woodland use intensity, plant diversity decrease while fungal and bacterial diversity increase (Li et al., 2022). Microbial diversity is high and connections are close, so this may be the reason for the high microbial network complexity. The ecological network results of this study suggest that aboveground plant species–coffee plantations with lower richness have higher connectivity, network asymmetry, and clustering coefficients, indicating that species are highly specific to microorganisms. In this sense, woodland use types enhance resistance to environmental stresses by enhancing the higher fungal and bacterial community stability. In this study, it was found that the complexity of the fungi–bacteria interdomain network increased with the increase of woodland use intensity, and was negatively correlated with EMF in both dry and wet seasons (Figures 2–4). IDEN analysis was used to explore the complex interrelationships between aboveground plants and underground soil microbes, and key species that may play a crucial role in sustaining community stability were identified. Here, we found many plants belonging to network hubs were highly associated with microorganisms (Supplementary Figures 2, 3), indicating that these species may be linked with specific ecosystem functions, and hub species should be given high priority for conservation (Olesen et al., 2007). It was found that there is no network hub in woodland utilization types without an arbor layer, so arbors are the main source of network hub.
It was found that different woodland use interdomain network characteristics differed in four woodland use types because of their different interactions between plants and microorganisms. We found that the effect of plant–microbe network complexity on EMF was highest in both wet and dry seasons based on our random forest analysis. It seems that the interdomain network of plant–microorganism is more important than the ecological network of microorganisms in the ecosystem. Changes in microbes and climate factors are normally covariants. Climate can directly and indirectly affect ecosystem functioning (Maestre et al., 2012; Bowker et al., 2013). For example, climate can directly influence it by expediting the activity and interaction among its detritivores, consumers, decomposers, and microorganisms (Wolters et al., 2000; Wardle et al., 2011), and it can also indirectly affect ecosystem functions by shifting community composition (Bardgett and van der Putten, 2014). Precipitation affects EMF mainly through plant species richness, soil pH, soil moisture content, and soil biodiversity (Jing et al., 2015). Soil nutrients in the wet season decreased as soil moisture increased in comparison with the dry season, which may be the reason for the loss of soil nutrients due to leaching from more rainfall. Accordingly, there were seasonal changes in soil EMF among the differing WUI. Temperature and soil moisture differ tremendously between dry and wet seasons, with both temperature and soil moisture presumed to be firmly linked to biochemical processes and microbial metabolic rates (Zhou et al., 2016). As such, temperature and soil moisture may impose direct restrictions on the formation of network communities by regulating metabolic activities or the evolution and assembly of microorganisms in the soil. Further, we found disparate climatic factors that mainly affected the EMF in the dry and wet seasons, namely temperature and soil moisture content, which is consistent with our third hypothesis. The differential between the climate factors that affect EMF in dry and wet seasons is probably related to water stress. Most soil microorganisms are sensitive to local changes in temperature (García-Palacios et al., 2015; van der Voort et al., 2016; Karimi et al., 2018). The direct effects of higher temperature and humidity can forcefully affect different ecosystem functions related to microbial productivity or metabolism (Yvon-Durocher et al., 2012). Climate change may alter the composition of plant and soil communities and thereby also their interactions (García-Palacios et al., 2015). Hence the differential relationship arising between network complexity and EMF in dry and wet seasons. This study supplies important information for the sustainable management of forests by adjusting fungal–bacterial taxa and plant–microbe interactions.
5 Conclusion
With the decrease in soil nutrient contents due to the change of woodland use, the interaction between plant–microbe and fungi–bacteria changed significantly. Our results suggest that such interactions have an important impact on regulating plant regeneration and soil nutrient availability during the logged forests restoration.
With the increase in woodland use intensity, the complexity of the plant–microbial network decreased, the complexity of the fungus–bacterial network increased, and ecosystem multifunctionality decreased in dry and wet seasons. During the dry season, WUI could indirectly affect EMF through plant–microbial network complexity. During the wet season, WUI could directly affect EMF and indirectly affect EMF through plant–microbial network complexity and fungi–bacteria network complexity. The main climate variable affecting microorganisms in the dry season is air temperature, while the soil moisture content is the main climate variable affecting microorganisms in the wet season. We identified key species among the sites through fungi–bacteria and plant–microbe networks, and some of the key species are rare species in the sample, which supplied an opportunity to know how the behavior of keystone species affects other species. Moreover, identifying the climatic factors affecting soil microorganisms in different seasons in this study provides valuable insights for comprehending the coexistence between belowground microorganisms and aboveground plants, as well as guiding the management of forest ecosystems. At the same time, more time scales should be used to study the stability of ecological networks, which can fully explore the importance of species interaction between networks in forest ecosystems.
Data availability statement
The original contributions presented in the study are publicly available. This data can be found here: NCBI Sequence Read Archive (SRA), projects PRJNA762704 (http://www.ncbi.nlm.nih.gov/bioproject/762704) and PRJNA950001 (http://www.ncbi.nlm.nih.gov/bioproject/950001).
Author contributions
JL, JS, and SL conceived the study and designed the methodology. JL, XH, SL, and RT collected the data. JL analyzed the data and wrote the manuscript. All authors contributed to the article and submitted and approved the submitted section.
Funding
Fundamental Research Funds of CAF (CAFYBB2021ZA002 and CAFYBB2020ZA002).
Conflict of interest
The authors declare that the research was conducted in the absence of any commercial or financial relationships that could be construed as a potential conflict of interest.
Publisher’s note
All claims expressed in this article are solely those of the authors and do not necessarily represent those of their affiliated organizations, or those of the publisher, the editors and the reviewers. Any product that may be evaluated in this article, or claim that may be made by its manufacturer, is not guaranteed or endorsed by the publisher.
Supplementary material
The Supplementary Material for this article can be found online at: https://www.frontiersin.org/articles/10.3389/fevo.2023.1154787/full#supplementary-material
References
Ahkami A. H., Allen White R., Handakumbura P. P., Jansson C. (2017). Rhizosphere engineering: enhancing sustainable plant ecosystem productivity. Rhizosphere 3, 233–243. doi: 10.1016/j.rhisph.2017.04.012
Allan E., Bossdorf O., Dormann C. F., Prati D., Gossner M. M., Tscharntke T., et al. (2014). Interannual variation in land-use intensity enhances grassland multidiversity. Proc. Natl. Acad. Sci. U.S.A. 111, 308–313. doi: 10.1073/pnas.1312213111
Archer E. (2013) rfPermute: Estimate permutation p-values for Random Forest importance metrics. R package version 1.5. 2. Available at: https://CRAN.R-project.org/package=rfPermute.
Banerjee S., Walder F., Büchi L., Meyer M., Held A. Y., Gattinger A., et al. (2019). Agricultural intensification reduces microbial network complexity and the abundance of keystone taxa in roots. ISME J. 13, 1722–1736. doi: 10.1038/s41396-019-0383-2
Barberán A., Bates S. T., Casamayor E. O., Fierer N. (2012). Using network analysis to explore co-occurrence patterns in soil microbial communities. ISME J. 6, 343–351. doi: 10.1038/ismej.2011.119
Bardgett R. D., van der Putten W. H. (2014). Belowground biodiversity and ecosystem functioning. Nature 515, 505–511. doi: 10.1038/nature13855
Bastian M., Heymann S., Jacomy M. (2009). “Gephi: an open source software for exploring and manipulating networks,” in Proceedings of the 3rd International Conference on Weblogs and Social Media, ICWSM, San Jose, CA, May 17-20, 2009.
Bokulich N. A., Subramanian S., Faith J. J., Gevers D., Gordon J. I., Knight R., et al. (2013). Quality-filtering vastly improves diversity estimates from Illumina amplicon sequencing. Nat. Methods 10, 57–59. doi: 10.1038/nmeth.2276
Bowker M. A., Maestre F. T., Mau R. L. (2013). Diversity and patch-size distributions of biological soil crusts regulate dryland ecosystem multifunctionality. Ecosystems 16, 923–933. doi: 10.1007/s10021-013-9644-5
Byrnes J. E. K., Gamfeldt L., Isbell F., Lefcheck J. S., Griffin J. N., Hector A., et al. (2014). Investigating the relationship between biodiversity and ecosystem multifunctionality: Challenges and solutions. Methods Ecol. Evol. 5, 111–124. doi: 10.1111/2041-210X.12143
Chaparro J. M., Sheflin A. M., Manter D. K., Vivanco J. M. (2012). Manipulating the soil microbiome to increase soil health and plant fertility. Biol. Fertil. Soils 48 (5), 489–499. doi: 10.1007/s00374-012-0691-4
Chen Q. L., Ding J., Zhu Y. G., He J. Z., Hu H. W. (2020). Soil bacterial taxonomic diversity is critical to maintaining the plant productivity. Environ. Int. 140, 105766. doi: 10.1016/j.envint.2020.105766
Chen J., Feng K., Emilia H. S. J., Kuzyakov Y., Li Y., Xu H. (2021). Interkingdom plant-microbial ecological networks under selective and clear cutting of tropical rainforest. For. Ecol. Manage. 491, 119182. doi: 10.1016/j.foreco.2021.119182
Csardi G., Nepusz T. (2006). The igraph software package for complex network research. InterJournal Complex Syst. Complex Sy 1695, 1–9.
Cui W., Liu J., Jia J., Wang P. (2021). Terrestrial ecological restoration in China: identifying advances and gaps. Environ. Sci. Eur. 33, 123. doi: 10.1186/s12302-021-00563-2
Delgado-Baquerizo M., Maestre F. T., Reich P. B., Jeffries T. C., Gaitan J. J., Encinar D., et al. (2016). Microbial diversity drives multifunctionality in terrestrial ecosystems. Nat. Commun. 7, 1–8. doi: 10.1038/ncomms10541
Delgado-Baquerizo M., Reich P. B., Trivedi C., Eldridge D. J., Abades S., Alfaro F. D., et al. (2020). Multiple elements of soil biodiversity drive ecosystem functions across biomes. Nat. Ecol. Evol. 4, 210–220. doi: 10.1038/s41559-019-1084-y
Deng Y., Jiang Y., Yang Y., He Z., Luo F., Zhou J. (2012). Molecular ecological network analyses. BMC Bioinf. 13, 113. doi: 10.1186/1471-2105-13-113
R Development Core Team (2019). R: A Language and Environment for Statistical Computing (Vienna, Austria: R Foundation for Statistical Computing).
Duan W. B., Qu M. X., Chen L. X., Zhang Y. S., Li S. B., Duan W. J. (2018). Effects of decay level of fallen trees and their formed microsite types on soil physicochemical properties in a spruce-fir forest. J. App. Ecol. 29 (12), 3977–3985. doi: 10.13287/j.1001–9332.201812.030
Edgar R. C. (2013). UPARSE: highly accurate OTU sequences from microbial amplicon reads. Nat. Methods 10, 996–998. doi: 10.1038/nmeth.2604
Feng K., Zhang Y., He Z., Ning D., Deng Y. (2019). Interdomain ecological networks between plants and microbes. Mol. Ecol. Resourc. 19, 1565–1577. doi: 10.1111/1755-0998.13081
Friedman J., Alm E. J. (2012). Inferring correlation networks from genomic survey data. PloS Comput. Biol. 8, e1002687. doi: 10.1371/journal.pcbi.1002687
Fu D., Wu X., Qiu Q., Duan C., Jones D. L. (2020). Seasonal variations in soil microbial communities under different land restoration types in a subtropical mountains region, Southwest China. Appl. Soil Ecol. 153, 103634. doi: 10.1016/j.apsoil.2020.103634
García-Palacios P., Vandegehuchte M. L., Shaw E. A., Dam M., Post K. H., Ramirez K. S., et al. (2015). Are there links between responses of soil microbes and ecosystem functioning to elevated CO2, N deposition and warming? A Global perspective Glob. Chang. Biol. 21, 1590–1600. doi: 10.1111/gcb.12788
Gomez-Montano L., Jumpponen A., Gonzales M. A., Cusicanqui J., Valdivia C., Motavalli P. P., et al. (2013). Do bacterial and fungal communities in soils of the bolivian altiplano change under shorter fallow periods? Soil Biol. Biochem. 65, 50–59. doi: 10.1016/j.soilbio.2013.04.005
Goss-Souza D., Mendes L. W., Borges C. D., Rodrigues J. L. M., Tsai S. M. (2019). Amazon forest-to-agriculture conversion alters rhizosphere microbiome composition while functions are kept. FEMS Microbiol. Ecol. 95(3), 1–33. doi: 10.1093/femsec/fiz009
Jing X., Sanders N. J., Shi Y., Chu H., Classen A. T., Zhao K., et al. (2015). The links between ecosystem multifunctionality and above-and belowground biodiversity are mediated by climate. Nat. Commun. 6 (1), 1–8. doi: 10.1038/ncomms9159
Kandeler E., Gerber H. (1988). Short-term assay of soil urease activity using colorimetric determination of ammonium. Biol. Fertil. Soils 6, 68–72. doi: 10.1007/BF00257924
Karimi B., Terrat S., Dequiedt S., Saby N. P. A., Horrigue W., Lelièvre M., et al. (2018). Biogeography of soil bacteria and archaea across France. Sci. Adv. 4, eaat1808. doi: 10.1126/sciadv.aat1808
Klaus V. H., Kleinebecker T., Busch V., Fischer M., Hölzel N., Nowak S., et al. (2018). Land use intensity, rather than plant species richness, affects the leaching risk of multiple nutrients from permanent grasslands. Glob. Chang. Biol. 24, 2828–2840. doi: 10.1111/gcb.14123
Layeghifard M., Hwang D., Guttman D. (2016). Disentangling interactions in the microbiome: a network perspective. Trends Microbiol. 25, 217–228. doi: 10.1016/j.tim.2016.11.008
Le Provost G., Badenhausser I., Le Bagousse-Pinguet Y., Clough Y., Henckel L., Violle C., et al. (2020). Land-use history impacts functional diversity across multiple trophic groups. Proc. Natl. Acad. Sci. U.S.A. 117, 1573–1579. doi: 10.1073/pnas.1910023117
Li J., Li S., Huang X., Tang R., Zhang R., Li C., et al. (2022). Plant diversity and soil properties regulate the microbial community of monsoon evergreen broad-leaved forest under different intensities of woodland use. Sci. Total Environ. 821, 153565. doi: 10.1016/j.scitotenv.2022.153565
Ma B., Wang H., Dsouza M., Lou J., He Y., Dai Z., et al. (2016). Geographic patterns of co-occurrence network topological features for soil microbiota at continental scale in eastern China. ISME J. 10, 1891–1901. doi: 10.1038/ismej.2015.261
Ma S., Zhu B., Chen G., Ni X., Zhou L., Su H., et al. (2021). Loss of soil microbial residue carbon by converting a tropical forest to tea plantation. Sci. Total Environ. 818, 151742. doi: 10.1016/j.scitotenv.2021.151742
Maestre F. T., Quero J. L., Gotelli N. J., Escudero A., Ochoa V., Delgado-Baquerizo M., et al. (2012). Plant species richness and ecosystem multifunctionality in global drylands. Science 335, 214–218. doi: 10.1126/science.1215442
Manning P., van der Plas F., Soliveres S., Allan E., Maestre F. T., Mace G., et al. (2018). Redefining ecosystem multifunctionality. Nat. Ecol. Evol. 2, 427–436. doi: 10.1038/s41559-017-0461-7
Olesen J., Bascompte J., Dupont Y., Jordano P. (2007). The modularity of pollination networks. Proc. Natl. Acad. Sci. U.S.A. 104, 19891–19896. doi: 10.1073/pnas.0706375104
Qin H. Y., Wang F. (2018). Response of soil nitrogen to cutting intensity based on meta-analysis. For. Res. 31, 90–97. doi: 10.13275/j.cnki.lykxyj.2018.04.013
Qiu L., Zhang Q., Zhu H., Reich P. B., Banerjee S., van der Heijden M. G. A., et al. (2021). Erosion reduces soil microbial diversity, network complexity and multifunctionality. ISME J. 15, 2474–2489. doi: 10.1038/s41396-021-00913-1
Rajkumar M., Prasad M. N. V., Swaminathan S., Freitas H. (2013). Climate change driven plant–metal–microbe interactions. Environ. Int. 53, 74–86. doi: 10.1016/j.envint.2012.12.009
Rezzonico F., Rupp O., Fahrentrapp J. (2017). Pathogen recognition in compatible plant-microbe interactions. Sci. Rep. 7 (1), 6383. doi: 10.1038/s41598-017-04792-5
Smith A. P., Marín-Spiotta E., Balser T. (2015). Successional and seasonal variations in soil and litter microbial community structure and function during tropical postagricultural forest regeneration: A multiyear study. Glob. Chang. Biol. 21, 3532–3547. doi: 10.1111/gcb.12947
Soil Survey Staff (2014). “Keys to Soil Taxonomy,” in 12th USDA-Natural Resources Conservation Service(Washington, DC: USDA-Natural Resources Conservation Service), 173–209.
Song Y. C. (2007). Degradation Mechanism and Ecological Restorations of Evergreen Broadleaved Forest Ecosystem in East China (Beijing: Science Press).
Sun S., Badgley B. D. (2019). Changes in microbial functional genes within the soil metagenome during forest ecosystem restoration. Soil Biol. Biochem. 135, 163–172. doi: 10.1016/j.soilbio.2019.05.004
Tabatabai A. (1994). Methods of soil analysis: microbiological and biochemical properties. Sci. Horticul. 63, 131–133. doi: 10.1016/0304-4238(95)90023-3
Toju H., Guimaraes P. R., Olesen J. M., Thompson J. N. (2014). Assembly of complex plant-fungus networks. Nat. Commun. 5, 5273. doi: 10.1038/ncomms6273
van der Heijden M. G. A., Hartmann M. (2016). Networking in the plant microbiome. PloS Biol. 14 (2), e1002378. doi: 10.1371/journal.pbio.1002378
van der Voort M., Kempenaar M., van Driel M., Raaijmakers J. M., Mendes R. (2016). Impact of soil heat on reassembly of bacterial communities in the rhizosphere microbiome and plant disease suppression. Ecol. Lett. 19, 375–382. doi: 10.1111/ele.12567
Wagg C., Schlaeppi K., Banerjee S., Kuramae E. E., van der Heijden M. G. (2019). Fungal bacterial diversity and microbiome complexity predict ecosystem functioning. Nat. Commun. 10 (1), 1–10. doi: 10.1038/s41467-019-12798-y
Walther G. R. (2010). Community and ecosystem responses to recent climate change. Philos. Trans. R Soc. B Biol. Sci. 365, 2019–2024. doi: 10.1098/rstb.2010.0021
Wardle D. A., Bardgett R. D., Klironomos J. N., Setälä H., Putten H. ,. W., Der V., et al. (2011). Ecological Linkages between Aboveground and Belowground Biota Published by: American Association for the Advancement of Science Stable URL: http://www.jstor.org/stable/3837023. Adv. Sci. 304, 1629–1633.
Wen Z., Zheng H., Zhao H., Xie S., Liu L., Ouyang Z. (2020). Land-use intensity indirectly affects soil multifunctionality via a cascade effect of plant diversity on soil bacterial diversity. Glob. Ecol. Conserv. 23, e01061. doi: 10.1016/j.gecco.2020.e01061
Wolters V., Silver W. L., Bignell D. E., Coleman D. C., Lavelle P., van der Putten W. H., et al. (2000). Effects of global changes on above- and belowground biodiversity in terrestrial ecosystems: Implications for ecosystem functioning. Bioscience 50, 1089–1098. doi: 10.1641/0006-3568(2000)050[1089:EOGCOA]2.0.CO;2
Xu X., Liu X., Li Y., Ran Y., Liu Y., Zhang Q., et al. (2017). High temperatures inhibited the growth of soil bacteria and archaea but not that of fungi and altered nitrous oxide production mechanisms from different nitrogen sources in an acidic soil. Soil Biol. Biochem. 107, 168–179. doi: 10.1016/j.soilbio.2017.01.003
Xun W., Liu Y., Li W., Ren Y., Xiong W., Xu Z., et al. (2021). Specialized metabolic functions of keystone taxa sustain soil microbiome stability. Microbiome 9 (1), 1–15. doi: 10.1186/s40168-020-00985-9
Yuan Z., Ali A., Loreau M., Ding F., Liu S., Sanaei A., et al. (2021). Divergent above- and below-ground biodiversity pathways mediate disturbance impacts on temperate forest multifunctionality. Glob. Chang. Biol. 27, 2883–2894. doi: 10.1111/gcb.15606
Yuan Z., Ali A., Ruiz-Benito P., Jucker T., Mori A. S., Wang S., et al. (2020). Above- and below-ground biodiversity jointly regulate temperate forest multifunctionality along a local-scale environmental gradient. J. Ecol. 00, 1–13. doi: 10.1111/1365-2745.13378
Yvon-Durocher G., Caffrey J. M., Cescatti A., Dossena M., Giorgio P., Gasol J. M., et al. (2012). Reconciling the temperature dependence of respiration across timescales and ecosystem types. Nature 487, 472–476. doi: 10.1038/nature11205
Zeller U., Starik N., Göttert T. (2017). Biodiversity, land use and ecosystem services—An organismic and comparative approach to different geographical regions. Glob. Ecol. Conserv. 10, 114–125. doi: 10.1016/j.gecco.2017.03.001
Zhou J., Deng Y., Luo F., He Z., Yang Y. (2011). Phylogenetic molecular ecological network of soil microbial communities in response to elevated CO2. Mbio 2, e00122–e00111. doi: 10.1128/mBio.00122-11
Zhou J., Deng Y., Shen L., Wen C., Yan Q., Ning D., et al. (2016). Temperature mediates continental-scale diversity of microbes in forest soils. Nat. Commun. 7, 1–10. doi: 10.1038/ncomms12083
Zhou J., Xue K., Xie J., Deng Y. E., Wu L., Cheng X., et al. (2012). Microbial mediation of carbon-cycle feedbacks to climate warming. Nat. Clim. Change 2 (2), 106–110. doi: 10.1038/nclimate1331
Keywords: woodland use intensity, climate factors, interdomain ecological networks (IDENs), dry season and wet season, complexity of network
Citation: Li J, Li S, Huang X, Lang X, Tang R, Zhang R and Su J (2023) Interdomain plant–microbe and fungi–bacteria ecological networks under different woodland use intensity during the dry and wet season. Front. Ecol. Evol. 11:1154787. doi: 10.3389/fevo.2023.1154787
Received: 31 January 2023; Accepted: 13 July 2023;
Published: 01 August 2023.
Edited by:
Yixin Zhang, Soochow University, ChinaReviewed by:
Xiaoyu Shan, Massachusetts Institute of Technology, United StatesQingchao Zeng, Beijing Forestry University, China
Copyright © 2023 Li, Li, Huang, Lang, Tang, Zhang and Su. This is an open-access article distributed under the terms of the Creative Commons Attribution License (CC BY). The use, distribution or reproduction in other forums is permitted, provided the original author(s) and the copyright owner(s) are credited and that the original publication in this journal is cited, in accordance with accepted academic practice. No use, distribution or reproduction is permitted which does not comply with these terms.
*Correspondence: Jianrong Su, amlhbnJvbmdzdUB2aXAuc2luYS5jb20=