- Key Laboratory of Freshwater Biodiversity Conservation, Ministry of Agriculture and Rural Affairs of China, Yangtze River Fisheries Research Institute, Chinese Academy of Fishery Sciences, Wuhan, China
Lake phytoplankton communities are affected by environmental and spatial factors. We studied the relative importance of environmental and spatial factors on the phytoplankton community assembly in Lake Wuchang across three hydrological seasons, which were divided into dry (December to March), normal (April to June, October to November) and wet seasons (July to September) based on the water level and depth. Spatial and temporal patterns of environmental factors and phytoplankton community composition and diversity were studied using Kruskal–Wallis test, Wilcoxon test and NMDS. CCA, Mantel and partial Mantel tests, and PLS-PM were used to investigate the effects of environmental and spatial factors on phytoplankton community characteristics. Results showed that phytoplankton assemblages at the eight study sites were composed of totally 244 species belonging to 9 phyla, which changed from Bacillariophyta and Chlorophyta to Cyanophyta across the whole hydrological period. There was significantly higher abundance and biomass in the normal and wet seasons than that in the dry season. Phytoplankton alpha diversity exhibited uniform temporal distribution patterns with higher values in the dry season than in the normal and wet seasons. The Mantel and partial Mantel tests revealed that environmental (physicochemical conditions of lake water) and spatial factors (geographic distances among sites) jointly affected the phytoplankton community structure and beta diversity across the hydrological seasons, while spatial factors were more important in the wet season. Partial least squares path models showed that spatial factors exhibited a significant positive correlation with the phytoplankton diversity with the path coefficients of 0.53 and 0.71 in the normal and wet seasons, respectively. Phytoplankton composition had significant correlation with on phytoplankton diversity with the path coefficient of −0.75 and 0.61 in the normal and wet seasons, respectively. Our findings revealed that both environmental and spatial factors affected the phytoplankton community assembly in Lake Wuchang. Environmental factors played a more important role in the dry season, while spatial factors were more important in the wet season. With the exception of the abiotic factors (environmental and spatial), the impacts of biotic factors on phytoplankton community cannot be ignored. Therefore, it is also necessary to strengthen further research on the top-down control over phytoplankton communities in Lake Wuchang.
1 Introduction
It is a central goal to unravel the drivers of biological community structures and succession in response to environmental change in ecology, and there are still huge challenges to understand the process of natural species distribution and the formation of biodiversity patterns (Datry et al., 2016). Understanding the factors that affect the structure of the community is of great significance for understanding biodiversity and its changes, so as to achieve biodiversity conservation and formulate management measures (Bortolini et al., 2019). Species dispersal and biological community structures in aquatic ecosystems usually depend on hydrological connectivity (Cottenie et al., 2003; Beisner et al., 2006). Phytoplankton, as the primary producers and vital components of aquatic food webs (Zubkov and Tarran, 2008), have a wide distribution range and a short life cycle. They play an important role in the process of material circulation and energy flow in freshwater ecosystems (Nagy-László et al., 2020). Their community structure and dynamics have profound effects on biogeochemical cycles and the functioning of aquatic ecosystems; thus, they are often used as indicators for monitoring the health of aquatic ecosystems (Wang et al., 2011; Yang et al., 2016; Pomati et al., 2017; Cao et al., 2018; Li et al., 2021). Phytoplankton in freshwater lakes have been regarded as a good example of studying the mechanisms of community construction (Isabwe et al., 2018). Understanding the mechanisms that shape community assembly is essential in aquatic ecology, which can be used to establish how and to what extent ecological and evolutionary processes are responsible for driving local and regional biological assemblages (Zhou and Ning, 2017).
Biological assemblages are influenced by environmental, spatial, and seasonal factors. The effects of these factors on lake phytoplankton community structures are usually different in different regions (Vilmi et al., 2017; Wu et al., 2018; Wu et al., 2019; Chen et al., 2022). Environmental factors such as nutrients, water temperature, and light as well as climate and human activities have important impacts on phytoplankton communities (Zhang et al., 2007; Hayes et al., 2015). However, a growing number of studies have found that spatial factors can be more important than environmental factors for the phytoplankton community assembly in lakes and reservoirs (Wu et al., 2019; Chen et al., 2022). Environmental factors (e.g., salinity, temperature, nitrate) and spatial factors (e.g., urban land cover) together also determine plankton composition in highly urbanized estuarine ecosystems (Zhou et al., 2021). In addition, hydrological connectivity may vary temporally and/or spatially, which can be used to infer community assembly and predict the outcome of habitat loss and fragmentation (Isabwe et al., 2018). For example, there are seasonal and spatial variations in the plankton communities of the Pearl River Estuarine ecosystem in China, with seasonal variations being more remarkable (Shi et al., 2017). Although many studies have focused on the mechanisms that drive phytoplankton community assembly, their results may not be extrapolated to other regions (Heino et al., 2015). In addition, community assembly may be affected not only by a single factor but also by the interaction of environmental, spatial, and seasonal factors. Environmental or spatial factors can also play different roles in different seasons or hydrological conditions (Wu et al., 2018). However, previous studies have mainly focused on quantifying the relative importance of environmental and/or spatial factors in a single hydrological season. An understudied question is how the roles of environmental and/or spatial factors in the assembly of phytoplankton communities change across hydrological seasons. A study in Dongting Lake, a subtropical floodplain lake in the Yangtze River Basin, found that although environmental and spatial factors jointly determine the process of fish community assembly, spatial structuring (or mass effects) resulting from excessively high dispersal rates was more dominant during the flooding season, while environmental filtering had stronger effects than spatial structuring (or dispersal limitation) during the non-flooding season (Chen et al., 2022). Few studies have investigated how the relative importance of environmental and spatial factors for the phytoplankton community assembly changes across hydrological seasons, which is an important but overlooked issue.
In this study, we investigated the relative importance of environmental and spatial factors for the phytoplankton community assembly in Lake Wuchang during different hydrological seasons for the first time. Lake Wuchang is an important part of the Anqing Lake subregion in the Yangtze River Basin in Anhui Province, China, which is divided into four subregions by the World Wide Fund for Nature (WWF). In the past years, its ecological environment used to be one of the best among lakes at the middle–lower reaches of the Yangtze River. However, due to climate change, industrial and agricultural production, and other human activities, the lake ecosystem has undergone great changes in recent years, and there is a risk of cyanobacterial blooms in summer when the weather is warm and rainy (Meng et al., 2022). Therefore, Lake Wuchang may exemplify the ecological and environmental changes of the whole Anqing Lake subregion under climate and human stressors. We hypothesized that there are specific patterns in the composition and diversity of phytoplankton communities and that environmental and spatial factors played different roles in community assembly in different hydrological seasons. To test our hypothesis, we characterized the physical and chemical conditions in the lake; analyzed the spatiotemporal variation in phytoplankton abundance, biomass, and alpha and beta diversity; and evaluated the effects of environment and spatial factors on phytoplankton communities in different hydrological seasons. We aimed to answer the following questions: (i) What spatial patterns do phytoplankton communities exhibit in different hydrological seasons, and are these patterns similar or different across seasons? (ii) Which factors (environmental or spatial) are more dominant for the phytoplankton community assembly in different hydrological seasons? (iii) To what extent do environmental and spatial variables affect the community assembly? Novel insights can be gleaned from our findings about phytoplankton communities in the lake ecosystem.
2 Materials and methods
2.1 Study area
Lake Wuchang is a typical shallow lake (30°14′–30°20′N, 116°36′–116°53′E) located in Anhui Province, southeast China (Figure 1), which is divided into the upper and lower regions by the Anqing–Jiujiang Highway. The upper and lower regions are named Lake Wuchang (WC) and Lake Qingcao (QC), respectively. Lake Wuchang has an area of 87.5 km2 corresponding to a volume of 4.23 × 108 m3, which is the largest lake of the Wanhe River System in the Yangtze River Basin. It is 30.0 km from east to west and 9.5 km from south to north, with an average depth of 2.52 m and a maximum depth of 5.0 m. The lake is in the subtropical monsoon climate zone. The average annual mean temperature and annual total rainfall of this area are 16.6°C and 1,299.6 mm, respectively. The water level of Lake Wuchang has a typical seasonal variation with the lowest value in the dry season from December to March (11.4 m), the highest in the wet season from July to September (14.2 m), and the other months being the normal season (12.3 m). Lake Wuchang plays an important ecological role in fishery breeding, runoff regulation, climate improvement, and species protection. The national aquatic germplasm resource reserves of Monopterus albus and Trionyx sinensis are both located at Lake Wuchang. In addition, as an important fishery and breeding lake, the fishing output and value could reach 1,800 tons/year and more than 40 million yuan/year, respectively.
2.2 Sampling and analysis
Four sampling sites were set up in the upper lake region (WC1–WC4) as well as the lower lake region (QC1–QC3 and LHK). A total of 67 surface water samples (0–0.5 m) were collected in three different hydrological seasons from August 2019 to October 2021, respectively. A total of 24 samples were collected during the normal season (June 2020, October 2020, and October 2021), while 23 and 20 samples were collected during the wet (August 2019, August 2020, and July 2021) and dry seasons (December 2019, January 2021, and March 2021), respectively. Fewer samples were collected in the wet and dry seasons due to the bad weather in the upper lake region and lower water level in the lower lake region. A 1-L water sample was filtered and stored with acid-cleaned plastic containers for the analysis of total nitrogen (TN), ammonium nitrogen (NH4+-N), nitrate nitrogen (NO3−-N), nitrite nitrogen (NO2−-N), total phosphate (TP), and phosphate (PO43−-P). The analyses were conducted with a portable multi-parameter spectrophotometer (Hach DR1900, Hach, USA). Water temperature (WT), pH, dissolved oxygen (DO), and electrical conductivity (EC) were measured with a Hach HQ40d water quality analyzer on-site. Secchi depth (SD) and water depth (WD) were measured with a Saybolt disk and an SM-5 sounder, respectively. Another 1 L of water was collected and precipitated for 48 h. After siphoning off the supernatant and concentrating to 30 mL, 0.1 mL was taken and examined under the microscope for algal species identification and calculation of abundance and biomass according to Utermöhl (1931), which were determined using an inverted microscope with a 0.1 mL counting box containing 100 horizons at ×10 and ×40 magnifications (Cao et al, 2018). The phytoplankton qualitative samples were collected with a 64-μm pore size net, and the taxa (species level) were classified according to Hu and Wei (2006).
2.3 Statistical analysis
The statistical analyses in this study were mainly performed with R (version 4.1.1, R Core Team, 2021). Differences in the environmental factors, abundance, and biomass of phytoplankton among the dry, normal, and wet seasons were tested using the Kruskal–Wallis test and Wilcoxon test since the data did not pass the normal distribution test (p < 0.05, Shapiro–Wilk). The species richness and Shannon–Wiener and Pielou indices of phytoplankton communities were calculated with the “vegan” R package. Beta diversity based on the Bray–Curtis distance among sites was analyzed using the “bray” function in the “ape” R package. After that, non-parametric multivariate statistical tests were used to analyze the differences in alpha and beta diversity among seasons (Clarke and Ainsworth, 1993). Patterns of beta diversity indicated with the Bray–Curtis distance were visualized by non-metric multidimensional scaling (NMDS) (Kruskal, 1964). The stress value is the criterion for evaluating the outcome of NMDS and represents the inconsistency between the observed distance and the fitted distance. It can also be understood as the difference between the distance of the space formed by the sample after dimensionality reduction and the distance in the original multidimensional space. The smaller the stress value, the more reliable is the ordination result. It is generally accepted that when the stress value is <0.2, the ordination results have an appropriate level of explanatory significance (Legendre and Legendre, 2012). Then, analysis of dissimilarity among seasons was performed with permutation multivariate analysis of variance (PERMANOVA; 999 permutations).
Mantel tests with 9,999 permutations within the “vegan” R package (Jackson and Somers, 1989) were used to determine the correlations of phytoplankton composition dissimilarity with both the geographic distance and the Euclidean distance between environmental variables. The environmental variables were subdivided into physical factors (WT, pH, DO, EC, SD, and WD) and nutrient factors (TN, NH4+-N, NO3−-N, NO2−-N, TP, and PO43−-P). Following that, partial Mantel tests were conducted to examine the correlations among phytoplankton community dissimilarity with environmental distance and geographic distance, after controlling for the effects of seasons (Martiny et al., 2006). To determine the relative importance of factors structuring phytoplankton communities, the spatial distance between sites was derived by using the principal coordinates of neighbor matrices (PCNM) procedure based on their geographic coordinates (Griffith and Peres-Neto, 2006). After that, canonical correspondence analysis (CCA) was conducted by using the “vegan” R package in R to investigate how phytoplankton compositions (based on Hellinger) were affected by the spatial and environmental variables (based on the normalized Euclidean distance), By performing Monte Carlo permutation tests (999 permutations) with the function “ordistep,” we selected a parsimonious set of factors that explained a significant amount of variation in the phytoplankton in each sample (Borcard et al., 2011). Subsequently, to analyze the relationships between abiotic factors (spatial and environmental) and phytoplankton characteristics (composition and diversity), a partial least squares path model (PLS-PM) with the R package from Hulland (1999) was utilized. In the models, spatial factors were classified as exogenous variables, which were only used as a cause to explain endogenous variables such as environmental factors and phytoplankton composition. Coefficients of determination R2 (R2 > 0.3) and goodness of fit (GoF > 0.7) were used to evaluate the PLS-PM (Chin, 2010). R2 is the coefficient of determination of the endogenous variables, and its values indicate the amount of variance in the endogenous latent variable explained by its independent latent variables. The GoF index is a pseudo-goodness of fit measure that accounts for the model quality, which is calculated as the geometric mean of the average communality and the average R2 values (Sanchez, 2013; Liu et al., 2022).
3 Results
3.1 Variations in environmental variables
Variations in environmental factors are shown as mean ± standard deviation (Table A1). In general, across the hydrological seasons, there were no significant differences in physical and nutrient factors between the upper and lower lake regions except SD, EC, and DO content (p > 0.05). SD and EC (0.68 ± 0.33 cm, 148.6 ± 30.6 µS/cm) were significantly higher in the upper lake region (p < 0.05), while DO (7.58 ± 3.04 mg/L) was higher in the lower lake region (p < 0.05). In the dry season, the WD, DO, and NO3−-N content (1.5 ± 0.2 m, 10.93 ± 0.99 mg/L, and 0.5 ± 0.2 mg/L, respectively) of the upper lake were higher than those of the lower lake (p < 0.05), while other physical and nutrient factors were higher in the upper lake. PO43−-P and NO2−-N concentrations did not differ between the lake regions in the dry season (p > 0.05). The same as in the dry season, the EC (140.0 ± 26.1 µS/cm) and DO (7.58 ± 3.04 mg/L) of the lower lake were significantly higher and lower in the wet season, respectively (p < 0.05). Meanwhile, in the normal season, there were no significant differences in the environmental factors except EC, which was significantly higher in the lower lake region (p < 0.05).
For most environmental factors, the differences among seasons were significant (p < 0.05), except for pH, EC, and PO43−-P content (Figure 2). In addition, significantly higher WD (3.8 ± 1.0 m), WT (30.7 ± 0.8°C), and SD (1.1 ± 0.5 m) were found in the wet season than in the normal and dry seasons (p < 0.05). Significant differences in DO, TN, and NO3−-N content between the dry season (10.35 ± 1.25 mg/L, 1.52 ± 0.66 mg/L, and 0.45 ± 0.21 mg/L, respectively) and the normal and wet seasons (p < 0.05) were found, whereas there was no significant difference between the normal season and wet seasons (p > 0.05). The differences in TP and NO2−-N among seasons were not significant except for those between the dry season and normal season (p > 0.05). Ammonium (NH4+-N) in the wet season (0.02 ± 0.03 mg/L) was significantly lower than that in the normal season as well as the dry season (0.09 ± 0.10 and 0.07 ± 0.07 mg/L, respectively), and significant difference was not found between the normal and dry seasons (p > 0.05).
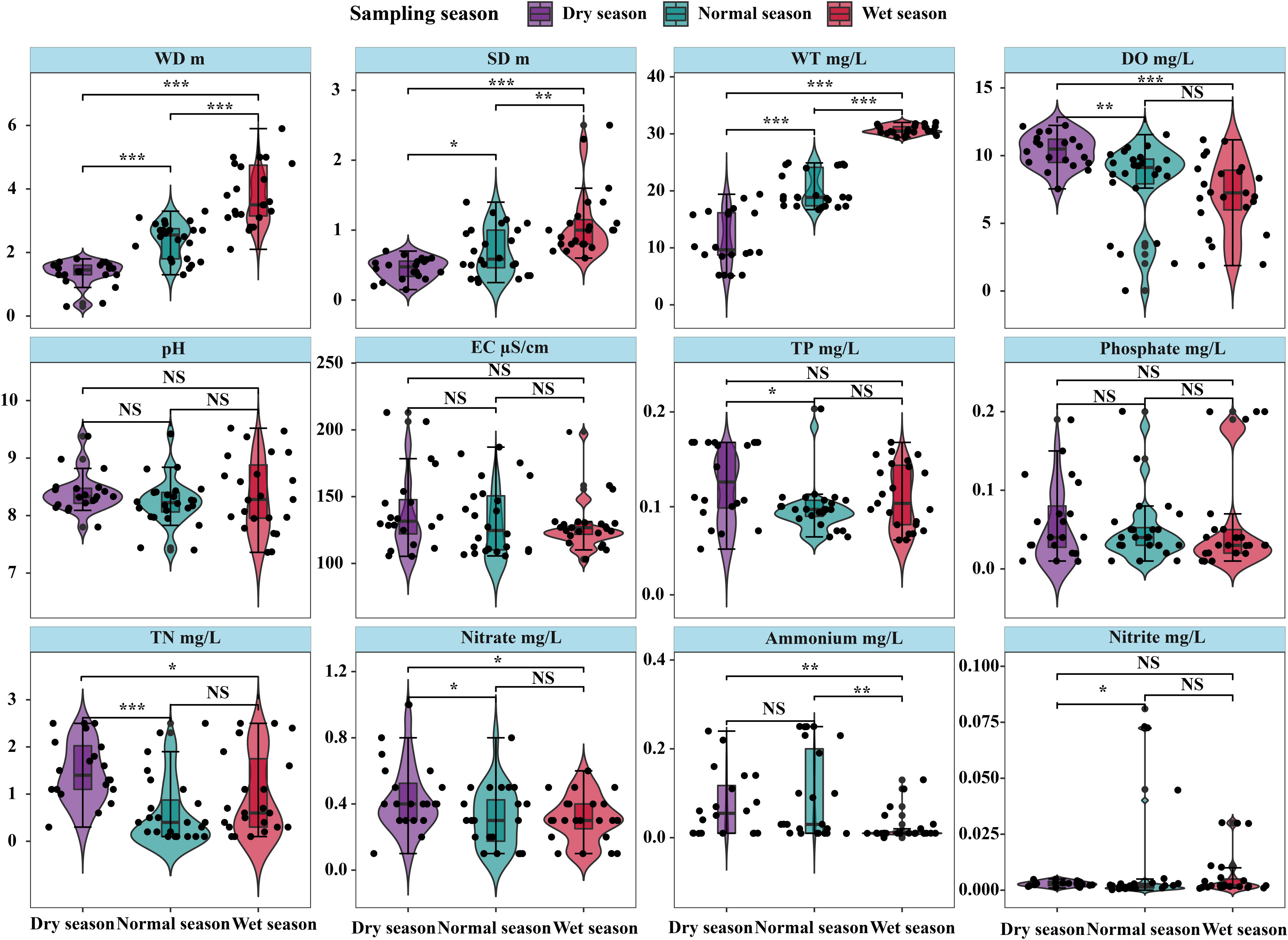
Figure 2 Seasonal variations in environmental factors in Lake Wuchang. The significance levels are indicated by * (p < 0.05), ** (p < 0.01) and *** (p < 0.001), NS indicates that the difference is not significant.
3.2 Variations in phytoplankton community composition and diversity
Across all the samples, nine phyla with 244 phytoplankton species were identified in Lake Wuchang across the hydrological seasons (Table 1), and most species belong to Chlorophyta, with 101 species, accounting for 41.39% (of total phytoplankton species), followed by Bacillariophyta (24.18%), Euglenophyta (11.07%), Cyanophyta (10.66%), and Cryptophyta (4.51%), with 59, 27, 26, and 11 species, respectively. Additionally, 9 species were from Pyrrophyta and Chrysophyta, and only 1 species each was identified from Haptophyta and Xanthophyta. In total, 160, 150, and 113 species were identified in the normal season, wet season, and dry season, respectively, and the number of species of Chlorophyta was the highest in the three seasons. The haptophyte Chrysochromulina parva was found only in the normal season.
Phytoplankton abundance and biomass showed substantial spatiotemporal variations in Lake Wuchang (Figure 3). Overall, at the phylum level, phytoplankton abundance levels in the normal season (77.00 × 106 cells/L) and wet season (37.35 × 106 cells/L) were higher than that in the dry season (6.26 × 106 cells/L) (p < 0.05), while it was not different between the normal and wet seasons (p > 0.05). Moreover, the normal season had a significantly higher biomass (29.44 mg/L) than the dry (1.55 mg/L) and wet seasons (2.88 mg/L) (p < 0.05). In the dry season, there were no significant differences in the average abundance of Bacillariophyta, Chlorophyta, and Cyanophyta, with cell densities of 1.32 × 106 cells/L, 1.13 × 106 cells/L, and 1.49 × 106 cells/L (p > 0.05), respectively. However, the biomass of Bacillariophyta (0.93 mg/L) was higher than those of the other phyla, followed by Chlorophyta (0.14 mg/L) and Cyanophyta (0.03 mg/L). The highest abundance, the same as biomass, was observed in the LHK sampling site in the lower lake (1.96 × 106 cells/L and 4.08 mg/L, respectively). In the normal season, the average abundance and biomass of Cyanophyta (70.1 × 106 cells/L, 8.20 mg/L) were significantly higher than those of other phyla, accounting for 91.87% and 72.52% of total phytoplankton abundance and biomass, respectively.
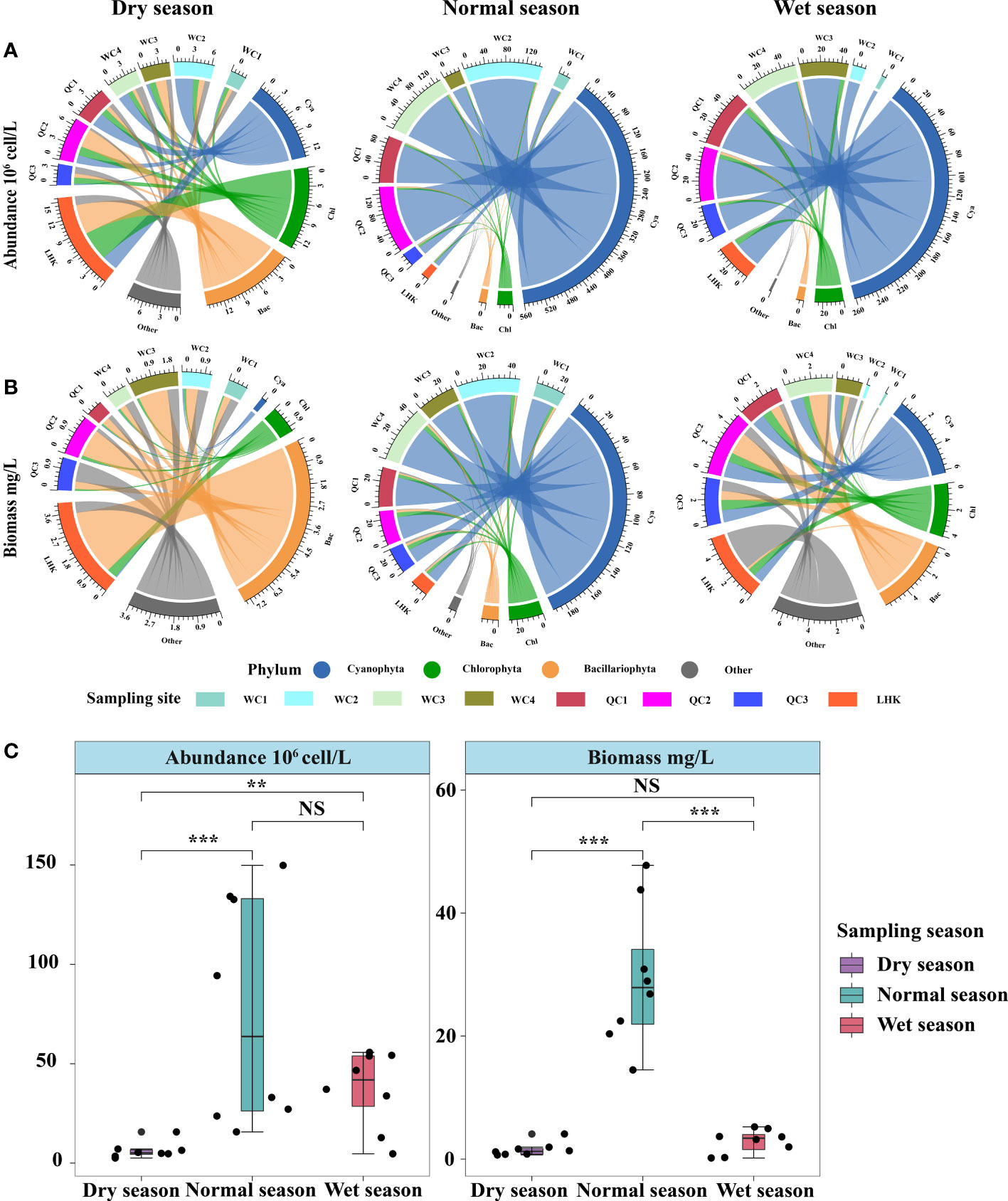
Figure 3 Spatiotemporal variations in phytoplankton community composition in Lake Wuchang. (A) and (B) represent the phytoplankton abundance and biomass of three different hydrological seasons, respectively. (C) showed the differences of phytoplankton abundance and biomass among hydrological seasons. The significance levels are indicated by ** (p < 0.01) and *** (p < 0.001), NS indicates that the difference is not significant.
Phytoplankton alpha diversity is shown in Figure 4, which exhibits spatiotemporal variations. In the dry season, the upper lake region had a higher species richness, especially in WC3; meanwhile, Shannon–Wiener and Pielou indices showed similar variation in both the upper and lower lake regions. In the normal season, the species richness in the lower lake region was higher than that in the upper region. Moreover, Shannon–Wiener and Pielou indices showed the same spatial patterns across different sampling sites. When it came to the wet season, all alpha diversity indices were similarly lower in the upper lake. The species richness at QC3 was the lowest, while WC2 had the lowest Shannon–Wiener and Pielou indices (1.3 and 0.26, respectively) across the hydrological seasons. In contrast to the spatial patterns, the seasonal changes in species richness were not significant (p > 0.05); the highest mean occurred in the normal season (70 ± 10), followed by the wet (60 ± 17) and dry seasons (59 ± 16). Shannon–Wiener and Pielou indices in the dry seasons (5.21 ± 0.47 and 0.89 ± 0.03, respectively) were both significantly higher than those in the wet and normal seasons (p < 0.05). However, there were no significant differences in the species richness and Shannon–Wiener and Pielou indices between the normal season and the wet season (p > 0.05).
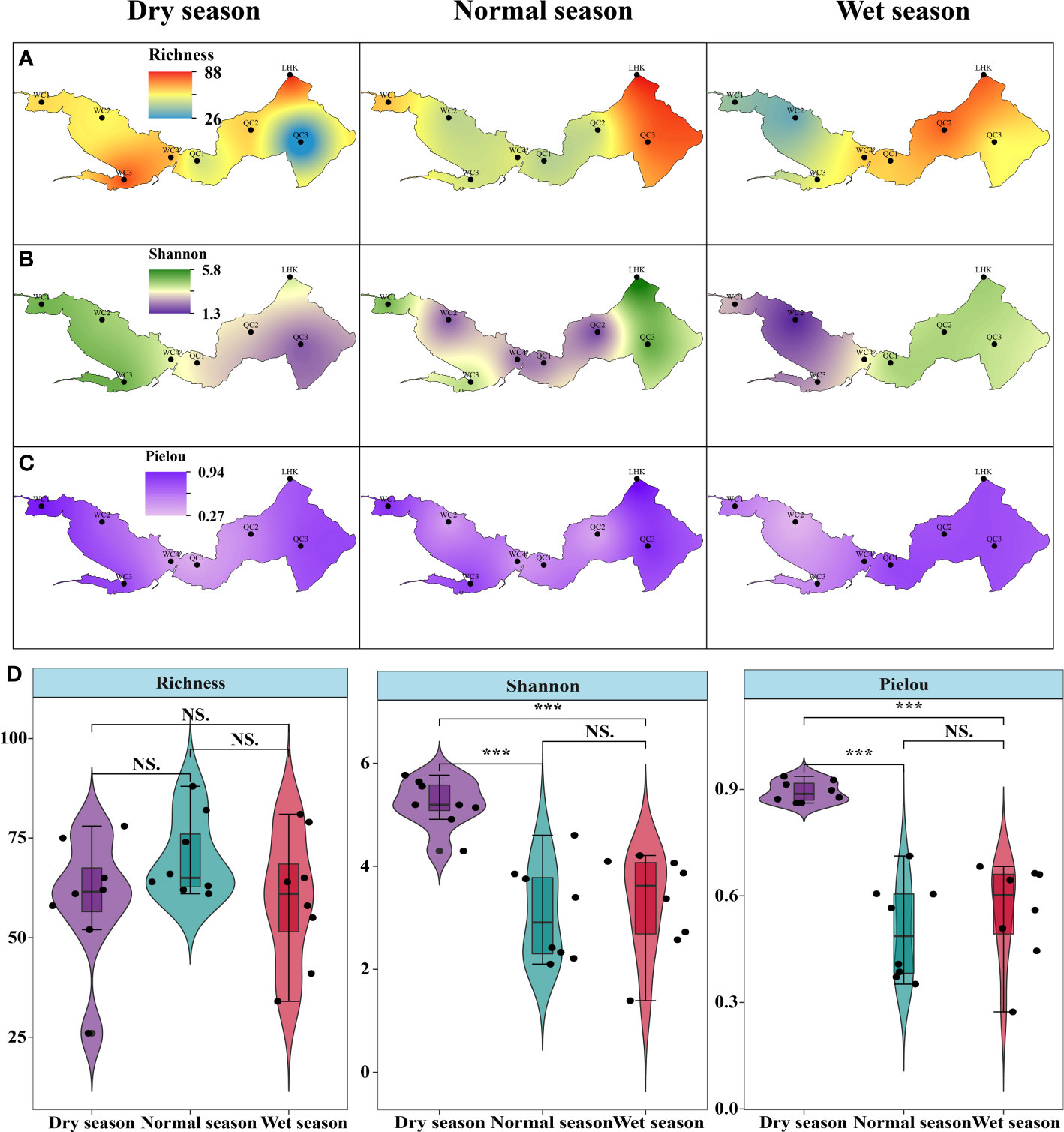
Figure 4 Spatiotemporal variations in phytoplankton alpha diversity in Lake Wuchang. (A–C) represent the spatial pattern of richness, Shannon-Winner and Pielou diversity indices in three different hydrological seasons, respectively. (D) showed the differences of three indices among hydrological seasons. The significance levels are indicated by *** (p < 0.001), NS indicates that the difference is not significant.
Phytoplankton beta diversity (shown as Bray–Curtis dissimilarity) was higher in the wet season (0.66 ± 0.24), than in the normal season (0.55 ± 0.24, p < 0.05). However, there were no significant differences between the dry and normal seasons (p > 0.05, Figure 5A). NMDS and PERMANOVA were performed to estimate the seasonal differences in phytoplankton community based on Bray–Curtis dissimilarity (Figure 5B). In general, phytoplankton community composition patterns across the seasons were delineated using the first two NMDS axes (stress = 0.092). PERMANOVA results further confirmed that there was a significant difference in Bray–Curtis dissimilarity among the hydrological seasons (R2 = 0.389, p = 0.001). The close clustering of samples, in the dry season, indicated a similar composition among sites, whereas larger spatial compositional differences were observed in the wet and normal seasons.
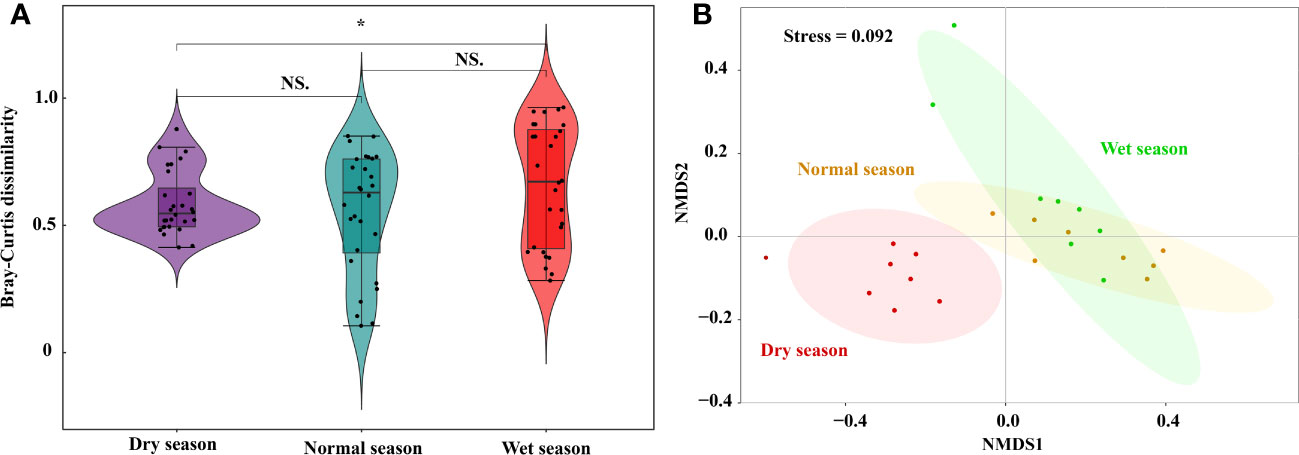
Figure 5 Seasonal variations and NMDS of phytoplankton beta diversity based on Bray-Curtis dissimilarity in Lake Wuchang. The significance levels are indicated by * (p < 0.05), NS indicates that the difference is not significant.
3.3 Correlation between phytoplankton communities and geographical and environmental variables
According to the results of CCA, all abiotic variables (including geographic, physical, and nutrient factors) could explain 75.74% of the total variance of the phytoplankton species composition, with CCA1 and CCA2 accounting for 45.51% and 12.30%, respectively (Figure 6). The composition of phytoplankton communities was mostly influenced by environmental factors such as SD (F = 3.046, p = 0.007), pH (F = 2.214, p = 0.045), WT (F = 4.670, p = 0.002), DO (F = 3.054, p = 0.008), TP (F = 3.596, p = 0.002), TN (F = 2.106, p = 0.039), and ammonium (F = 4.615, p = 0.002) and geographic factor PCNM1 (F = 2.408, p = 0.038) (Table A2).
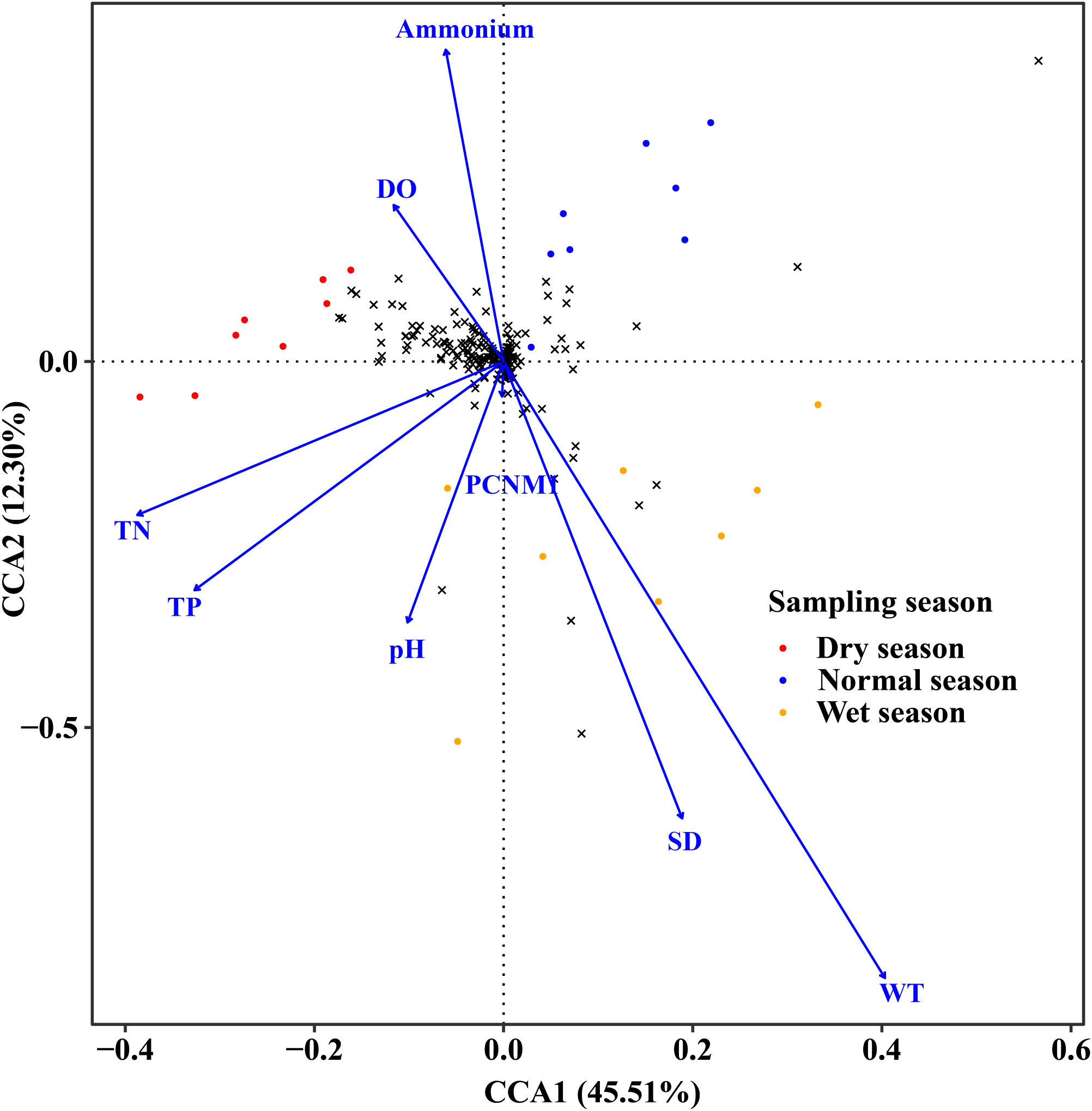
Figure 6 CCA plot of phytoplankton and environmental and spatial factors across different hydrological seasons.
In order to distinguish the effects of spatial, physical, and nutrient factors on phytoplankton beta diversity, Mantel and partial Mantel tests were employed based on Bray–Curtis dissimilarity (Table 2). The results revealed that phytoplankton beta diversity was strongly correlated with physical factors as well as the nutrient factors (rM = 0.519, p = 0.048 and rM = 0.366, p = 0.047, respectively) but not correlated with geographic distance (rM = 0.275, p > 0.05) in the dry season. In the normal season, phytoplankton community dissimilarity was closely correlated with physical and nutrient factors (rM = 0.487, p = 0.001 and rM = 0.339, p = 0.036, respectively) but not with geographic distance (rM = 0.091, p > 0.05). Similar trends were observed in the dry and normal seasons by partial Mantel tests as those by Mantel tests. However, in the wet season, there was a strong correlation of phytoplankton community only with geographic distance but not with physical or nutrient factors.
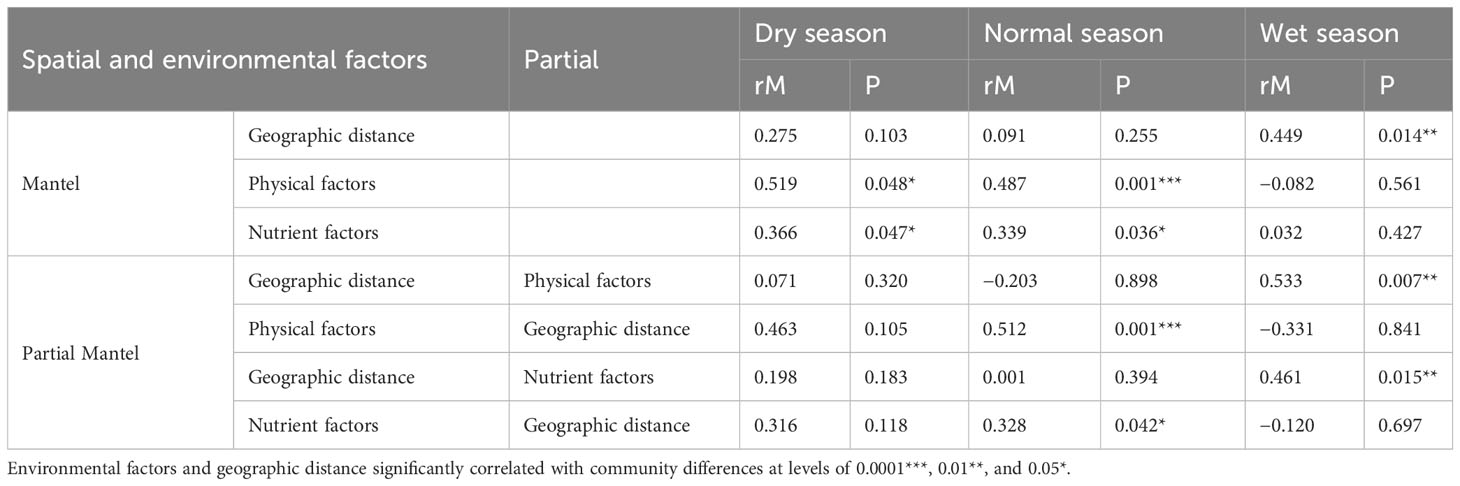
Table 2 Mantel and partial Mantel tests of the Bray–Curtis dissimilarity of phytoplankton communities with spatial factors (geographic distances) and environment factors (physical and nutrient factors).
A PLS-PM was constructed to further quantify the effects of environment variables (physical and nutrient factors) and spatial factors on phytoplankton community assembly in different hydrological seasons (Figure 7). Spatial factors exhibited a significant positive correlation with phytoplankton diversity in the normal and wet seasons with path coefficients of 0.53 and 0.71, respectively (p < 0.05), while they had a negative correlation with the phytoplankton composition in the dry season (p < 0.05). When it came to environmental factors (i.e., physical and nutrient factors), physical factors had a positive correlation with phytoplankton diversity in the dry and normal seasons but not in the wet season (p > 0.05). Nutrient factors had a positive correlation with the phytoplankton composition and diversity in the dry season with path coefficients of 0.72 and 1.56, respectively (p < 0.05), whereas the correlations were negative in the normal and wet seasons (p > 0.05). The associations of phytoplankton abundance and biomass with phytoplankton alpha diversity were positive in the wet season and negative in the normal season (p < 0.05), while the correlations in the dry season were not significant (p > 0.05). The PLS-PM explained 73%, 48%, and 70% of the variance in phytoplankton composition in the dry, normal, and wet seasons, respectively, while the variance in phytoplankton diversity was 87%, 98%, and 85%, respectively. DO had strong negative loading on physical factors in the three seasons, and so did pH (−0.97) in the normal and wet seasons. In the dry season, latitude, WT, and TP had the highest positive loading on spatial, physical, and nutrient factors (0.86, 0.97, and 0.92, respectively). WD and TN had the highest positive loading on physical and nutrient factors (0.83 and 0.96, respectively) in the normal season, while SD (0.93) and nitrate (0.96) had the highest positive loading on physical and nutrient factors in the wet season.
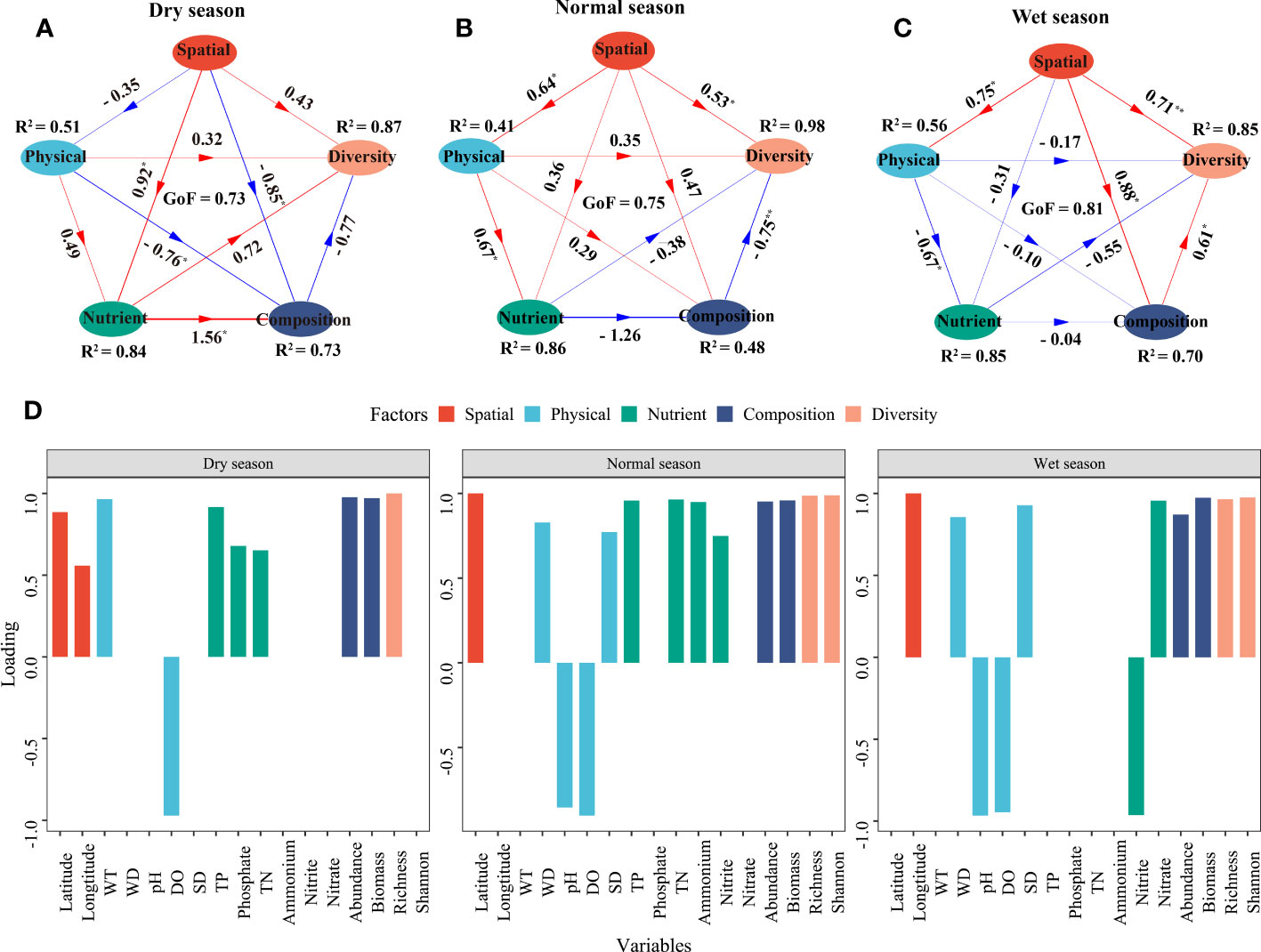
Figure 7 Partial least squares path model (PLS-PM) of the relationship between physical, nutrient, and spatial factors and phytoplankton community characteristics. (A–C) represent the dry, normal, and wet seasons, respectively. (D) shows the loading of different variables. Arrow widths represent the magnitude of the path coefficients, and red and blue colors indicate positive and negative effects, respectively. Path coefficients and coefficients of determination (R2) were calculated after 9,999 bootstraps, and significance levels are indicated by * (p < 0.05) and ** (p < 0.01). The goodness of fit (GoF) was used to assess the model.
4 Discussion
4.1 Phytoplankton community structure variation
Different types of lakes have different phytoplankton community structures (Deyab et al., 2019). In the present study, the phytoplankton community was mainly composed of Chlorophyta, Bacillariophyta, Euglenophyta, and Cyanophyta (Table 2), and these phyla were also common in lakes of the Yangtze River Basin (Peng et al., 2021). However, the differences in phytoplankton species among three different hydrological seasons were significant. The number of species of Cyanophyta and Chlorophyta showed an increasing trend from the dry season to the wet season, while the Bacillariophyta phylum showed the opposite trend. This may be related to the ecological habits of different algae; Bacillariophyta were mostly cold-water species, while green algae adapted to grow in warm water (Yang et al., 2018). Similar results were obtained for Lake Chaohu, a shallow lake of Anhui Province, where Cyanophyta, Chlorophyta, Bacillariophyta, and Euglenophyta represented 35.4%, 34.2%, 17.7%, and 8.9% of the total number of species, respectively (Jiang et al., 2014).
The phytoplankton abundance and biomass in Lake Wuchang also varied among the hydrological seasons. The dry and normal seasons had the lowest and highest abundance and biomass among the three seasons, respectively, which was consistent with the results for Lake Poyang (28°24′–29°46′N, 115°49′–116°46′E) (Yang et al., 2021). Furthermore, our results showed a major seasonal shift in phytoplankton biomass, from Bacillariophyta dominance in the dry season to Cyanophyta dominance in the normal and wet seasons (Figure 3). This shift in phytoplankton biomass could be attributed to the variation in the hydrological factors and water quality influenced by seasonal changes (Yang et al., 2022). For instance, water level fluctuations could affect the trophic state of lakes, with the water level rising, as well as the nutrient content increasing, which was conducive to the reproduction of algae, and the biomass increased accordingly (Calbet and Landry, 2004). Water quality alternation by increasing nitrogen, phosphorus, and turbidity may facilitate the dominance by a single or a few species. Increased water turbidity may lead to a decrease in light for phytoplankton growth (Shi et al., 2017), while increased water nutrient enrichment alters the community composition of phytoplankton (Zhang et al., 2021a).
The differences in the phytoplankton community structure in Lake Wuchang with different hydrological seasons were also reflected by the diversity indices. A higher diversity index may represent a healthier ecosystem (Manna et al., 2010). In our study, the species richness and Shannon–Wiener indices of the lower lake region were higher than those of the upper lake region in the wet season (Figure 4), which might be related to the differences in the submerged macrophyte coverage between the upper and lower lake regions in the wet season. Studies have shown that a higher submerged macrophyte coverage leads to a higher number of phytoplankton species (Liu et al., 2021; Zhang et al., 2021b). Compared to the upper lake region, there was a higher submerged macrophyte coverage in the lower lake region in Lake Wuchang (Ma, 2023). In the wet season, with the increase in rainfall, the nutrients flowing into the lake also increased, which was more conducive to the growth and reproduction of submerged macrophytes; the phytoplankton species increased at the same time. Nevertheless, compared with the dry season (0.45 ± 0.15 m), the higher SD in the normal and wet seasons (0.70 ± 0.34 m and 1.10 ± 0.48 m, respectively, Figure 2) may not favor the persistence of some Cyanophyta species such as Microcystis sp., Pseudanabaena sp., Limnothrix sp., and Planktolyngbya subtilis, which are more adapted to a lower SD (Seafarers et al., 2017; Liu et al., 2023), thereby reducing the Shannon–Wiener index of phytoplankton from the dry season to the wet season. A previous study suggested that phytoplankton were more inclined to absorb ammonium to synthesize amino acids for cells; thus, a higher ammonium concentration may lead to a higher phytoplankton diversity value (Yang et al., 2019). In our study, there were higher ammonium concentrations in the dry season than in the wet season (Figure 2), which might have caused the higher Shannon diversity. The Shannon–Wiener index was higher than 2.78, indicating moderately rich phytoplankton diversity in the dry season (Figure 4). The beta diversity index reflects the dissimilarity of community species composition in different regions.
4.2 The relationship between the phytoplankton community and environmental factors
As the primary producers in aquatic ecosystems, the community structure of phytoplankton is affected by many factors, especially environmental and spatial factors, whose relative influence depended on the dispersal capacity, spatial extent, and environmental gradients of biological communities (Heino et al., 2015). Previous studies indicated that environmental variables (such as water temperature, salinity, transparency, and nutrients such as NO3−-N and PO43−-P), spatial factors (altitude, etc.), and climatic change can lead to temporal variation in phytoplankton communities (Deng et al., 2019; Li et al., 2019; Li et al., 2021). Our CCA results showed that WT and SD were the important factors driving the variation in the phytoplankton community structure in the wet reason, while NH4+-N and DO were the main driving factors in the dry season. WT has both direct and indirect influences on the physiological and metabolic processes of phytoplankton, and the metabolic rate, productivity, and cell division of phytoplankton would be enhanced as the temperature increases (Sherman et al., 2016). The water temperature of Lake Wuchang was (11.5 ± 0.9)°C and (30.7 ± 0.8)°C in the dry and wet seasons, respectively (Figure 2), which may be the reason why the relative abundance and biomass of Cyanophyta remarkably rose from the dry season to the wet season, while Bacillariophyta declined in the present study. Nutrients had also an influence on the phytoplankton abundance, biomass, and diversity in different seasons (Kim et al., 2019). This was consistent with a study of 29 shallow lakes in Wuhan, China, which found that physicochemical parameters, such as TN, transparency, and DO, mainly affected the phytoplankton biomass, and water temperature and nutrients remained major predictors of phytoplankton abundance (Peng et al, 2021). Similar results were found for Lake Taihu, where Cyanophyta biomass was positively related to pH and temperature but negatively related to TN and TN/TP (Ke et al., 2019). Mantel and partial Mantel tests results showed that the relative importance of environmental factors and geographic distance to community dissimilarity differed among seasons (Table 2). The physical and nutrient factors appeared to have unique effects on phytoplankton community dissimilarity in the dry (rM = 0.519 and 0.366, respectively, p < 0.05) and normal seasons (rM = 0.487 and 0.339, respectively, p < 0.05), while the geographic factors had significant influence in the wet season (rM = 0.449, p < 0.05). Similar results were found for riverine diatoms (Wu et al., 2022), zooplankton (Gianuca et al., 2018), and macrophytes (Pozzobom et al., 2021).
4.3 Driving mechanisms of phytoplankton community assembly
In this study, a PLS-PM was used to quantify the effects and the importance of environmental and spatial factors on phytoplankton community assembly (Figure 7). Our research results enunciated that environmental factors were the main direct factors affecting phytoplankton composition in the dry season, while spatial factors had a significantly positive direct effect on phytoplankton diversity in the normal season. This is consistent with a study focusing on the phytoplankton community structure of another typical shallow lake (Lake Changhu) in the middle and lower reaches of the Yangtze River, whose phytoplankton assemblages were mainly determined by environmental factors, followed by spatial processes (Guo et al., 2019). In contrast, some studies have shown that spatial factors had more important impacts on phytoplankton community assembly than did environmental factors. These spatial factors include the geographical location and hydrological status of the lakes or reservoirs such as altitude, area, capacity, and water level (Vilmi et al., 2017; Bortolini et al., 2019). Recently, a field survey of phytoplankton assemblages in the Zhelin Reservoir of Lake Poyang Basin reported that spatial factors rather than environmental factors dominated the process of the phytoplankton community assembly (Chen et al., 2022). In this study, we found that spatial factors had a significant positive direct effect on phytoplankton composition and diversity, but no effects of physical or nutrient factors were found in the wet season. Both Wuchang Lake and Zhelin Reservoir were long (30 km and 80 km from east to west, respectively) and narrow in shape, which might have led to higher water connectivity and weakened the relative importance of environmental factors on the phytoplankton community structure. Thus, spatial factors played a more important role in structuring the phytoplankton community of Lake Wuchang in the wet season, when water exchange was more frequent than in the dry and normal seasons. In addition, in the dry and normal seasons, the water depth was significantly lower than that in the wet season; the connectivity between the upper and lower lakes was thus reduced due to the barrier of the Anjiu Highway Bridge, so the environmental factors dominated the assembly of the phytoplankton community.
5 Conclusions
Our results showed that the phytoplankton community structure exhibited spatiotemporal patterns in Lake Wuchang, and that environmental and spatial factors jointly affected the phytoplankton community structure. We identified eight variables that significantly affected the phytoplankton community structure in the three hydrological seasons, with four being physical (SD, pH, WT, and DO), three being nutrients (TN, TP, and ammonium), and one being spatial (PCNM1). Subsequently, spatial and environmental factors played different roles in phytoplankton community assembly in different hydrological seasons in Lake Wuchang. In the dry season, physical variables and nutrients had significant effects on the phytoplankton composition but not phytoplankton diversity, while spatial factors played a dominant role in affecting the phytoplankton composition and diversity in the wet season. Moreover, we further found that nutrients had higher relative importance in the dry and normal seasons, which means that controlling the input of nutrients from surface runoff, especially phosphate, may be the key to effectively reducing cyanobacterial blooms. Therefore, we suggest restoring macrophytes in the littoral zone and the lake inlets to reduce the impacts of the pollutants. In conclusion, our study provides insights into the regional distribution patterns and driving factors of phytoplankton communities in Lake Wuchang, which would help improve our understanding of plankton communities in shallow lake ecosystems and provide a scientific basis for ecological management of the shallow lakes in the middle and lower reaches of the Yangtze River. Yet, there are challenges in quantifying hydrological characteristics such as water flow velocity and water level at the local scale, which could have an important impact on phytoplankton community assembly by affecting the hydrological connectivity of lakes. Therefore, future studies need to also focus on ecological connectivity and species dispersal, especially at large spatial scales or timescales.
Data availability statement
The original contributions presented in the study are included in the article/Supplementary Material. Further inquiries can be directed to the corresponding authors.
Author contributions
ZM and XL conceived and designed the experiments; KC, LL, and FH performed the sample collection; ZM, XL, and KC analyzed the data; and ZM, XL, and DY wrote the manuscript. All authors contributed to the article and approved the submitted version.
Funding
This study was supported by the National Key R&D Program of China (2019YFD0900603), the China Agriculture Research System of MOF and MARA (CARS-46), and the Central Public-interest Scientific Institution Basal Research Fund, CAFS (2020XT1302, 2020TD57).
Conflict of interest
The authors declare that the research was conducted in the absence of any commercial or financial relationships that could be construed as a potential conflict of interest.
Publisher’s note
All claims expressed in this article are solely those of the authors and do not necessarily represent those of their affiliated organizations, or those of the publisher, the editors and the reviewers. Any product that may be evaluated in this article, or claim that may be made by its manufacturer, is not guaranteed or endorsed by the publisher.
Supplementary material
The Supplementary Material for this article can be found online at: https://www.frontiersin.org/articles/10.3389/fevo.2023.1154695/full#supplementary-material
References
Beisner B. E., Peres-Neto P. R., Lindström E. S., Barnett A., Longhi M. L. (2006). The role of environmental and spatial processes in structuring lake communities from bacteria to fish. Ecology 87 (12), 2985–2991. doi: 10.1890/0012-9658(2006)87[2985:TROEAS]2.0.CO;2
Bortolini J. C., da Silva P. R. L., Baumgartner G., Bueno N. C. (2019). Response to environmental, spatial, and temporal mechanisms of the phytoplankton metacommunity: comparing ecological approaches in subtropical reservoirs. Hydrobiologia 830 (1), 45–61. doi: 10.1007/s10750-018-3849-8
Calbet A., Landry M. R. (2004). Phytoplankton growth, microzooplankton grazing, and carbon cycling in marine systems. Limnol. Oceanogr. 49 (1), 51–57. doi: 10.4319/lo.2004.49.1.0051
Cao J., Hou Z., Li Z., Chu Z., Yang P., Zheng B. (2018). Succession of phytoplankton functional groups and their driving factors in a subtropical plateau lake. Sci. Total Environ. 631, 1127–1137. doi: 10.1016/j.scitotenv.2018.03.026
Chen K., Meng Z., Li X., Zhu T., Hu F., Liu L., et al. (2022). Phytoplankton community structure and driving mechanism of its construction process in autumn in Zhelin Reservoir, Lake Poyang Basin. J. Lake. Sci. 34 (02), 433–444. doi: 10.18307/2022.0206
Chin W. W. (2010). “How to Write Up and Report PLS Analyses,” in Handbook of Partial Least Squares: Concepts, Methods and Applications. Eds. Esposito Vinzi V., Chin W. W., Henseler J., Wang. H. (Berlin, Heidelberg: Springer Berlin Heidelberg), 655–690.
Clarke K. R., Ainsworth M. (1993). A method of linking multivariate community structure to environmental variables. Mar. Ecol. Prog. Ser. 92, 205–219. doi: 10.3354/meps092205
Cottenie K., Michels E., Nuytten N., De Meester L. (2003). Zooplankton metacommunity structure: Regional vs. local processes in highly interconnected ponds. Ecology 84 (4), 991–1000. doi: 10.1890/0012-9658(2003)084[0991:ZMSRVL]2.0.CO;2
Datry T., Bonada N., Heino J. (2016). Towards understanding the organisation of metacommunities in highly dynamic ecological systems. Oikos 125 (2), 149–159. doi: 10.1111/oik.02922
Deng J., Salmaso N., Jeppesen E., Qin B., Zhang Y. (2019). The relative importance of weather and nutrients determining phytoplankton assemblages differs between seasons in large Lake Taihu, China. Aquat. Sci. 81 (3), 1–14. doi: 10.1007/s00027-019-0645-0
Deyab M. A., Abu Ahmed S. E., Ward F. M. E. (2019). Comparative studies of phytoplankton compositions as a response of water quality at North El-Manzala Lake, Egypt. Int. J. Environ. Sci. Technol. 16 (12), 8557–8572. doi: 10.1007/s13762-019-02409-0
Gianuca A. T., Engelen J., Brans K. I., Hanashiro F. T. T., Vanhamel M., van den Berg E. M., et al. (2018). Taxonomic, functional and phylogenetic metacommunity ecology of cladoceran zooplankton along urbanization gradients. Ecography 41 (1), 183–194. doi: 10.1111/ecog.02926
Griffith D. A., Peres-Neto P. R. (2006). Spatial modeling in ecology: the flexibility of eigenfunction spatial analyses. Ecology 87 (10), 2603–2613. doi: 10.1890/0012-9658(2006)87[2603:SMIETF]2.0.CO;2
Guo K., Wu N., Wang C., Yang D., He Y., Luo J., et al. (2019). Trait dependent roles of environmental factors, spatial processes and grazing pressure on lake phytoplankton metacommunity. Ecol. Indic 103, 312–320. doi: 10.1016/j.ecolind.2019.04.028
Hayes N. M., Vanni M. J., Horgan M. J., Renwick W. H. (2015). Climate and land use interactively affect lake phytoplankton nutrient limitation status. Ecology 96 (2), 392–402. doi: 10.1890/13-1840.1
Heino J., Melo A. S., Siqueira T., Soininen J., Valanko S., Bini L. M. (2015). Metacommunity organisation, spatial extent and dispersal in aquatic systems: patterns, processes and prospects. Freshw. Biol. 60 (5), 845–869. doi: 10.1111/fwb.12533
Hu H. J., Wei Y. X. (2006). Freshwater algae in China—system, classification and ecology. (Beijing: Science Press).
Hulland J. (1999). Use of partial least squares (PLS) in strategic management research: a review of four recent studies. Strateg. Manage. J. 20 (2), 195–204. doi: 10.1002/(SICI)1097-0266(199902)20:2<195::AID-SMJ13>3.0.CO;2-7
Isabwe A., Yang J. R., Wang Y., Liu L., Chen H., Yang J. (2018). Community assembly processes underlying phytoplankton and bacterioplankton across a hydrologic change in a human-impacted river. Sci. Total Environ. 630, 658–667. doi: 10.1016/j.scitotenv.2018.02.210
Jackson D. A., Somers K. M. (1989). Are probability estimates from the permutation model of Mantel's test stable? Can. J. Zool. 67 (3), 766–769. doi: 10.1139/z89-108
Jiang Y. J., He W., Liu W. X., Qin N., Ouyang H. L., Wang Q. M., et al. (2014). The seasonal and spatial variations of phytoplankton community and their correlation with environmental factors in a large eutrophic Chinese lake (Lake Chaohu). Ecol. Indic 40, 58–67. doi: 10.1016/j.ecolind.2014.01.006
Ke Z., Xie P., Guo L. (2019). Ecological restoration and factors regulating phytoplankton community in a hypertrophic shallow lake, Lake Taihu, China. Sheng Tai Xue Bao 39 (1), 81–88. doi: 10.1016/j.chnaes.2018.05.004
Kim J. H., Lee D. H., Kang J.-H. (2019). Associating the spatial properties of a watershed with downstream Chl-a concentration using spatial analysis and generalized additive models. Water Res. 154, 387–401. doi: 10.1016/j.watres.2019.02.010
Kruskal J. B. (1964). Nonmetric multidimensional scaling: a numerical method. Psychometrika 29 (2), 115–129. doi: 10.1007/BF02289694
Li C., Feng W., Chen H., Li X., Song F., Guo W., et al. (2019). Temporal variation in zooplankton and phytoplankton community species composition and the affecting factors in Lake Taihu—a large freshwater lake in China. Environ. Pollut. 245, 1050–1057. doi: 10.1016/j.envpol.2018.11.007
Li Z., Gao Y., Wang S., Lu Y., Sun K., Jia J., et al. (2021). Phytoplankton community response to nutrients along lake salinity and altitude gradients on the Qinghai-Tibet Plateau. Ecol. Indic 128, 107848. doi: 10.1016/j.ecolind.2021.107848
Liu L., Li X., Meng Z., Chen K., Hu F., Zhu Y., et al. (2023). Succession characteristics of phytoplankton functional groups and water quality evaluation in Wuchang Lake. Chin. J. Ecol. 42 (1), 131–139. doi: 10.13292/j.1000-4890.202301.011
Liu Y., Li Z., Zhang X., Liu X., Xie J., Wang G., et al. (2021). Community charactreistics of phytoplankton : a case in a macrophyte-dominated sub-lake of Baiyangdian Lake during overgrowth period of submerged macrophyte. Fisheries Sci. 40 (6), 851–859. doi: 10.16378/j.cnki.1003-1111.20071
Liu S., Yu H., Yu Y., Huang J., Zhou Z., Zeng J., et al (2022). Ecological stability of microbial communities in Lake Donghu regulated by keystone taxa. Doctor Degree (Beijing: University of Science and Technology Beijing).
Ma S. (2023). The research of interaction mechanisms between plant-sediment microbial system and nutrient elements in the Wuchang Lake. Saline Syst. 6 (1), 8. doi: 10.1186/1746-1448-6-8
Manna S., Chaudhuri K., Bhattacharyya S., Bhattacharyya M. (2010). Dynamics of Sundarban estuarine ecosystem: eutrophication induced threat to mangroves. Saline Syst. 6 (1), 8. doi: 10.1186/1746-1448-6-8
Martiny J. B. H., Bohannan B. J. M., Brown J. H., Colwell R. K., Fuhrman J. A., Green J. L., et al. (2006). Microbial biogeography: putting microorganisms on the map. Nat. Rev. Microbiol. 4 (2), 102–112. doi: 10.1038/nrmicro1341
Meng Z., Chen K., Liu L., Hu F., Zhu Y., Li X., et al. (2022). Niche and interspecific association with respect to the dominant phytoplankton species in different hydrological periods of Lake Wuchang, China. Front. Environ. Sci. 10. doi: 10.3389/fenvs.2022.985672
Nagy-László Z., Padisák J., Borics G., Abonyi A., B-Béres V., Várbíró G. (2020). Analysis of niche characteristics of phytoplankton functional groups in fluvial ecosystems. J. Plankton Res. 42 (3), 355–367. doi: 10.1093/plankt/fbaa020
Peng X., Zhang L., Li Y., Lin Q., He C., Huang S., et al. (2021). The changing characteristics of phytoplankton community and biomass in subtropical shallow lakes: Coupling effects of land use patterns and lake morphology. Water Res. 200, 117235. doi: 10.1016/j.watres.2021.117235
Pomati F., Matthews B., Seehausen O., Ibelings B. W. (2017). Eutrophication and climate warming alter spatial (depth) co-occurrence patterns of lake phytoplankton assemblages. Hydrobiologia 787 (1), 375–385. doi: 10.1007/s10750-016-2981-6
Pozzobom U. M., Landeiro V. L., da Silva Brito M. T., Alahuhta J., Heino J. (2021). Multiple facets of macrophyte beta diversity are shaped by environmental factors, directional spatial processes, and connectivity across tropical floodplain lakes in the dry season. Hydrobiologia 848 (15), 3587–3602. doi: 10.1007/s10750-021-04613-x
R Core Team. (2021). R: A language and environment for statistical computing (Vienna, Austria: R Foundation for Statistical Computing). Available at: https://www.R-project.org/.
Sanchez G. (2013). PLS Path Modeling with R. Trowchez Editions (California: Berkeley). Available at: http://www.gastonsanchez.com/PLSPathModelingwithR.pdf.
Seafarers S. D., Lavender S., Beaugrand G., Outram N., Barlow N., Crotty D., et al. (2017). Seafarer citizen scientist ocean transparency data as a resource for phytoplankton and climate research. PloS One 12 (12), e0186092. doi: 10.1371/journal.pone.0186092
Sherman E., Moore J. K., Primeau F., Tanouye D. (2016). Temperature influence on phytoplankton community growth rates. Global Biogeochem. Cycles 30 (4), 550–559. doi: 10.1002/2015GB005272
Shi Z., Xu J., Huang X., Zhang X., Jiang Z., Ye F., et al. (2017). Relationship between nutrients and plankton biomass in the turbidity maximum zone of the Pearl River Estuary. J. Enviro. Sci. 57, 72–84. doi: 10.1016/j.jes.2016.11.013
Utermöhl H. (1931). Neue Wege in der quantitativen Erfassung des Plankton.(Mit besonderer Berücksichtigung des Ultraplanktons.). Internationale Vereinigung für theoretische und angewandte Limnologie: Verhandlungen 5 (2), 567–596. doi: 10.1080/03680770.1931.11898492
Vilmi A., Tolonen K. T., Karjalainen S. M., Heino J. (2017). Metacommunity structuring in a highly-connected aquatic system: effects of dispersal, abiotic environment and grazing pressure on microalgal guilds. Hydrobiologia 790 (1), 125–140. doi: 10.1007/s10750-016-3024-z
Wang Z., Wang Y., Hu M., Li Y., Liu Y., Shen Y., et al. (2011). Succession of the phytoplankton community in response to environmental factors in north Lake Erhai during 2009-2010. Fresenius Environ. Bull. 20 (9), 2221–2231.
Wu N., Qu Y., Guse B., Makarevičiūtė K., To S., Riis T., et al. (2018). Hydrological and environmental variables outperform spatial factors in structuring species, trait composition, and beta diversity of pelagic algae. Ecol. Evol. 8 (5), 2947–2961. doi: 10.1002/ece3.3903
Wu N., Wang Y., Wang Y., Sun X., Faber C., Fohrer N. (2022). Environment regimes play an important role in structuring trait-and taxonomy-based temporal beta diversity of riverine diatoms. J. Ecol. 110 (6), 1442–1454. doi: 10.1111/1365-2745.13859
Wu J., Xu N., ZHang W., Xu L., Hu J., Zhu X. (2019). Seasonal analysis of the niche and interspecific association of dominant species of phytoplankton in the Dinghai Moat, Zhoushan City. J. Lake. Sci. 31 (2), 429–439. doi: 10.18307/2019.0212
Yang Y., Chen H., Al M. A., Ndayishimiye J. C., Yang J. R., Isabwe A., et al. (2022). Urbanization reduces resource use efficiency of phytoplankton community by altering the environment and decreasing biodiversity. J. Enviro. Sci. 112, 140–151. doi: 10.1016/j.jes.2021.05.001
Yang J., Lv H., Yang J., Liu L., Yu X., Chen H. (2016). Decline in water level boosts cyanobacteria dominance in subtropical reservoirs. Sci. Total Environ. 557, 445–452. doi: 10.1016/j.scitotenv.2016.03.094
Yang X., Ma J., Zhang H., Zhou Q. (2021). Community structure and the water quality during different hydrological periods in Poyang Lake. Acta Hydrobiol. Sin. 45 (05), 1093–1103. doi: 10.7541/2021.2020.148
Yang L., Zhang W., Shang G., Zhang J., Wang L., Wei H. (2018). Succession characteristics of phytoplankton functional groups and their relationships with environmental factors in Dianshan Lake, Shanghai. Huan Jing Ke Xue 39 (7), 3158–3167. doi: 10.13227/j.hjkx.201710030
Yang S., Zu T., Wang H., Wang L., Chen T., Wang D., et al. (2019). Relationship between the structure of phytoplankton community and environmental factors in the Zhangye section of Heihe River. J. Lake. Sci. 31 (01), 159–170. doi: 10.18307/2019.0115
Zhang X., Xie P., Chen F., Li S., Qin J. (2007). Driving forces shaping phytoplankton assemblages in two subtropical plateau lakes with contrasting trophic status. Freshw. Biol. 52 (8), 1463–1475. doi: 10.1111/j.1365-2427.2007.01776.x
Zhang J., Xie J., Li Z., Zhang X., Wang G., Zhang K., et al. (2021b). Phytoplankton community structure in Baiyangdian Lake with different submerged macrophyte coveragers. J. Hydroecol. 42 (1), 75–83. doi: 10.15928/j.1674-3075.20190515012
Zhang H., Zong R., He H., Liu K., Yan M., Miao Y., et al. (2021a). Biogeographic distribution patterns of algal community in different urban lakes in China: Insights into the dynamics and co-existence. J. Enviro. Sci. 100, 216–227. doi: 10.1016/j.jes.2020.07.024
Zhou J., Ning D. (2021). Stochastic community assembly: does it matter in microbial ecology? Microbiol. Mol. Biol. Rev 81 (4), 10–1128. doi: 10.1128/MMBR
Zhou L., Wang P., Huang S., Li Z., Gong H., Huang W., et al. (2021). Environmental filtering dominates bacterioplankton community assembly in a highly urbanized estuarine ecosystem. Environ. Res. 196, 110934. doi: 10.1016/j.envres.2021.110934
Keywords: Lake Wuchang, phytoplankton, community assembly, hydrological season, Yangtze River
Citation: Meng Z, Chen K, Hu F, Liu L, Yang D and Li X (2023) Environmental and spatial factors play different roles in phytoplankton community assembly in different hydrological seasons in Lake Wuchang, China. Front. Ecol. Evol. 11:1154695. doi: 10.3389/fevo.2023.1154695
Received: 31 January 2023; Accepted: 05 September 2023;
Published: 22 September 2023.
Edited by:
Danny Chun Pong Lau, Swedish University of Agricultural Sciences, SwedenReviewed by:
Wen Xiong, Hubei Normal University, ChinaMing-Chih Chiu, Chinese Academy of Sciences (CAS), China
Copyright © 2023 Meng, Chen, Hu, Liu, Yang and Li. This is an open-access article distributed under the terms of the Creative Commons Attribution License (CC BY). The use, distribution or reproduction in other forums is permitted, provided the original author(s) and the copyright owner(s) are credited and that the original publication in this journal is cited, in accordance with accepted academic practice. No use, distribution or reproduction is permitted which does not comply with these terms.
*Correspondence: Xuemei Li, xmli@yfi.ac.cn; Deguo Yang, yangdg@yfi.ac.cn