- 1School of Geosciences and Info-Physics, Central South University, Changsha, China
- 2Key Laboratory of Spatio-Temporal Information and Intelligent Services, Chinese Ministry of Natural Resources, Changsha, China
- 3Key Laboratory of Urban Land Resources Monitoring and Simulation, Ministry of Natural Resources, Shenzhen, China
The surface radiation is a crucial variable for understanding global climate and eco-environment change, which exhibits significant variations over time and space. In this study, we used in situ ground observations to estimate variations of the surface radiation budget over grassland and urban areas in a subtropical humid region. Our results revealed a positive radiation budget that varied over different land covers. Specifically, grassland exhibited a higher shortwave radiation, while urban area was characterized by the higher longwave radiation. Notably, the surface radiation budget (Rn) was much greater in grassland (77.60 W2/m) than that in urban area (61.93 W2/m), which was mainly attributed to the difference in longwave radiation. Additionally, the atmospheric pressure showed a strong correlation with the radiations, while precipitation and relative humidity presented relatively weak correlations. Furthermore, the correlations with climate were stronger in grassland than that in urban areas, suggesting complex interactions with anthropogenic factors during the process of urbanization. Results of this study would help reveal the characteristics and corresponding mechanisms of surface radiation budgets, which would support climatic adaptation and ecology management.
1. Introduction
The surface radiation budget acts as the predominant driver of Earth’s ecosystem evolution (Wild et al., 2005; Pinker et al., 2006; Lean and David, 2009; Lee et al., 2017), which strongly affects global and regional climate change (Mahmood and Hubbard, 2005; Brown and Caldeira, 2017; Lee et al., 2017), hydrological processes (Sirdas and Duran, 2008; DeAngelis et al., 2015), and terrestrial productivity (Monteith, 1972; Zhao et al., 2019). It is therefore crucial to capture the variation in the surface radiation budget to further support the sustainable management and planning (Palmer, 2012; Brown and Caldeira, 2017).
Globally, solar radiation decreases from the equator to the poles, generating an overall pattern with a positive budget at low latitudes and a negative budget at high latitudes (Trenberth et al., 2009; Feng et al., 2020, 2022). At finer scales (i.e., regional or local scales), however, the surface radiation budget varies in different regions because of the impacts of the atmosphere (Ramanathan et al., 2007; Boucher et al., 2013; Matus and L'Ecuyer, 2017; Loeb et al., 2021) and surface influences (i.e., land cover change; Dickinson and Hanson, 1984; Betts, 2001; Tubiello et al., 2015) and their complex interactions (Trenberth et al., 2009; Feng et al., 2020, 2022). For example, the higher cloud coverage in humid regions enhances the absorption of solar radiation and the release of longwave radiation to the surface, leading to complicated variations of the radiation budget (Ramanathan and Collins, 1991). Moreover, the radiation budget in urban area fluctuates significantly due to the impact of buildings and pollutants (Jin et al., 2005). Therefore, the complex interactions leave significant uncertainties on the variation in the surface radiation budget at the regional scale., which needs a full investigation.
Characterized by preferable hydrothermal conditions and dense vegetation coverage, radiation variations in subtropical humid regions exert significant effects on global climate and environmental change, which has drawn increasing attentions (Allan et al., 2010; Wohl et al., 2012; Yu et al., 2014; Li et al., 2015; Fan et al., 2018; Peters et al., 2019; Mustafa et al., 2022). In particular, different land covers strongly affect radiation variations by altering surface thermal properties (i.e., radiation reflectivity and emissivity) and land-atmospheric interactions (Dickinson and Hanson, 1984; Betts, 2001; Tubiello et al., 2015; Feng et al., 2020), representing one focus for the investigation of radiation variations.
Several approaches could be adopted to monitor surface radiation. Some researchers depicted radiation variation over large regions by using climate models (Kothe et al., 2011; Lee et al., 2017) or satellite remote sensing (Kiehl and Trenberth, 1997; Trenberth et al., 2009). Specifically, climate models utilize mathematical equations to simulate the physical processes that govern the Earth’s climate system, thereby generating estimates of the radiation budget variation on a large spatial scale. However, the various assumptions, structures, and input parameters in different climate models result in significant uncertainties in the modeling results (Kothe et al., 2011). Satellite remote sensing measures the reflection and emission of radiation, providing a large-scale view of the earth’s radiation budget. However, it is strongly affected by atmospheric conditions, which weakens the quality of the observations and the consequent radiation budget estimation (Trenberth et al., 2009). Considering these uncertainties, ground observations are required to precisely capture the radiation budget (Wild et al., 2015; Driemel et al., 2018).
This study explores the variation in the surface radiation budget across different land covers in a subtropical humid region. In our previous studies, we estimated the surface radiation balance at global (Feng et al., 2022) and regional (Ye et al., 2021) scales, but ground validation results are still lacking. Therefore, this study details the variations from ground observations, providing a complete insight for understanding the variations in surface radiation. Specifically, radiation budget variations and their correlations with climate variables were examined over grassland and urban areas by using ground observations at daily and monthly scales. The structure of this study is as follows: Section 2 details the methods and corresponding datasets used, Section 3 presents the results, Section 4 provides the discussion, and Section 5 is the conclusion. The results of this study offer more precise guidance for investigating the surface radiation budget, which could also support the validation of modeling or satellite remote sensing results.
2. Methodologies and materials
2.1. Study area
Two in situ sites in the Dongting Lake Basin of China are designed to execute the investigation (Figure 1). This basin is an important ecological zone of the middle and lower reaches of the Yangtze River, which is characterized by a subtropical monsoon climate (Yeh et al., 2014). Land cover is dominated by vegetation (i.e., forest, grassland, and farmland) in the Dongting Lake Basin, while it has suffered from rapid urbanization in recent decades and exerted significant influences on the radiation budget and local climate (Zhang and Zhu, 2009; Ye et al., 2021). Two ground observations were installed in the grassland and urban sites of the study area, respectively. The annual average temperature and precipitation are 17.9°C and 1214.9 mm in the grassland site, and 18.3°C and 1389.1 mm in the urban site. Furthermore, the two stations have similar geographic conditions, with elevations of 20 m (grassland site) and 30 m (urban site).
2.2. Data collection and pre-processing
According to the concept of the surface radiation budget (Kiehl and Trenberth, 1997; Trenberth et al., 2009), solar radiation reaching the surface is subsequently converted into surface reflection and absorption. The absorbed radiation warms the surface and atmosphere and then triggers the emission of longwave radiation. Therefore, four components of the radiation budget [downward shortwave radiation (Rsd), upward shortwave radiation (Rsu), downward longwave radiation (Rld), and upward longwave radiation (Rlu)] are measured in this study to estimate the net surface radiation (Rn), shortwave (Rsn), and longwave (Rln) budgets. Specifically, Rsd refers to the solar radiation that reaches the earth’s surface, while Rsu is the radiation reflected or scattered by earth’s surface and transmitted toward the atmosphere. Rld is the atmosphere’s thermal emission to the surface, and the Rlu is the reflection of thermal longwave radiation from the surface. The relationships of the four components in the surface radiation budget can be expressed as:
CNR4 and Sensors of Onset Hobo U30 are used to monitor radiation and the corresponding auxiliary variables, respectively. Specifically, the Onset HOBO U30 portable automatic weather station is an all-weather monitoring system that ensures long-term stable field observations. With the aid of 15 channels, the system allows for real-time monitoring of various environmental parameters by combining different intelligent sensors. Meanwhile, shortwave (Rsd and Rsu) and longwave (Rld and Rlu) radiation are measured by CNR4, with a sensitivity of 5 ~ 20 μV/W/m2 for shortwave radiation and 5 ~ 15 μV/W/m2 for longwave radiation. Auxiliary atmospheric variables include precipitation, atmospheric pressure, and relative humidity. The relative humidity is measured by sensors of S-THB-M002 with accuracies of ±3%. Precipitation is measured by sensors of S-RGB-M002 with a calibration accuracy of ±1%, and atmospheric pressure is measured by sensors of S-BPB-CM50 with an accuracy of ±3.0 mbar.
The radiation described above is recorded at a temporal frequency of 1 min, with meteorological data recorded at temporal frequencies of 5 min and 1 min for the grassland and urban sites, respectively. All datasets used are available for 1 Aug 2021 to 31 July 2022. We resampled the datasets at daily and monthly scales to capture the overall characteristics of the radiation variations. Variations in radiation (Rsd, Rsu, Rld, and Rlu) and the corresponding budgets are evaluated over grassland and urban areas. Furthermore, the relationships between radiation budgets and auxiliary climatic variables are analyzed to understand the related potential causality.
3. Results
3.1. Temporal variations in radiation over different land covers
Our results demonstrate the complex variations in surface radiation over different land covers (Figure 2). Generally, the difference in downward shortwave radiation (Rsd) is relatively weak between the grassland (153.30 W/m2, the annual average value) and urban areas (140.11 W/m2; Figure 2A). The lower Rsd of the urban area may result from a high concentration of aerosols, which scatter more solar radiation (Penner et al., 1992; Yang et al., 2016; Dong et al., 2019; Feng and Zou, 2019). In contrast, upward shortwave radiation (Rsu) varies across the grassland (30.61 W/m2) and urban areas (22.34 W/m2) (Figure 2B) due to the presence of heterogeneous surface thermal properties. Specifically, the lower Rsu of the urban area is mainly attributed to large areas of cement or asphalt surfaces, which help absorb more radiation (Crutzen, 2004; Zhou et al., 2014; He et al., 2022). Radiation reaching the surface subsequently warms the surface and near-surface atmosphere, triggering the emissions of longwave radiation. Due to a low heat capacity, a stronger warming effect is usually found in urban areas (Arnfield, 2003; Chen et al., 2006). This is the main physical driver of urban heat islands (Gago et al., 2013; Feng et al., 2014a,b). As a result, both Rld and Rlu are higher in the urban area (381.95 W/m2 and 437.80 W/m2) than for the grassland (373.45 W/m2 and 418.55 W/m2; Figures 2C,D).
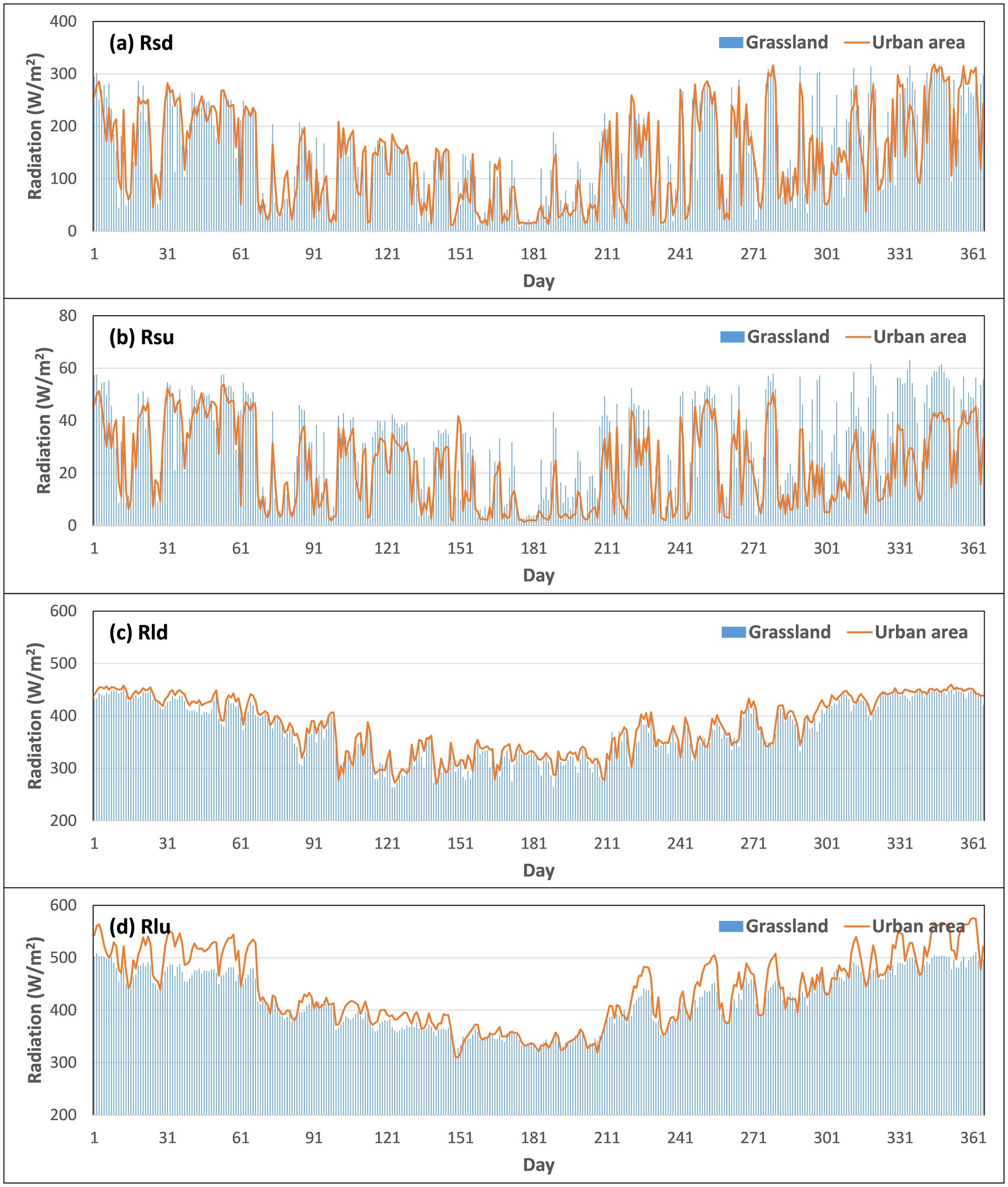
Figure 2. Temporal variations of surface radiation of (A) Rsd, (B) Rsu, (C) Rld, (D) Rlu in grassland and urban areas from Aug 1st in 2021 to July 31st in 2022.
Another noteworthy issue concerns temporal fluctuations of radiation. Generally, shortwave radiation (Figures 2A,B) presents strong temporal fluctuations relative to longwave radiation (Figures 2C,D). Specifically, the coefficients of variation (CV) are 0.59 and 0.57 for Rsd and Rsu over grassland and 0.14 and 0.13 for Rld and Rlu. These values of CV are 0.64 (Rsd) and 0.70 (Rsu) over the urban area, while values of 0.14 and 0.16 are found for the longwave radiation measures, respectively. The main reason for this phenomenon is that shortwave radiation is mainly controlled by the direct effects of the scattering and reflection of atmospheric variables (particularly clouds and aerosols), which are characterized by significant daily fluctuations. On the other hand, longwave radiation relies on emissions from the atmosphere and surface, which are affected by the temperature with the temporal hysteretic effect (Manoli et al., 2020).
Based on Equation (1), we then estimated the shortwave (Rsn) and longwave (Rln) radiation budgets and total net surface radiation (Rn). Generally, Rsn shows a positive budget (Figure 3A), while Rln shows a negative budget (Figure 3B). Specifically, Rsn is higher for grassland (122.70 W/m2) than in urban areas (117.77 W/m2), suggesting that more radiation is stored in vegetated surfaces. In addition, the Rln for grassland (−45.10 W/m2) is lower than that for the urban area (−55.84 W/m2), which demonstrates less radiation loss. Consequently, the total net radiation of Rn is much greater for grassland (77.60 W/m2) than in the urban area (61.93 W/m2; Figure 3C). It is crucial to clarify variations in the surface radiation budget to provide useful insights for understanding the physical mechanisms of terrestrial ecosystem evolution and climate change. Physically, partial radiation is absorbed by vegetation, enhancing the ecosystem productivity (Monteith, 1972). Other adsorption radiation stored on the surface warms the surface and subsequently the air, which plays a crucial role in global climate change (Xie et al., 2015; Brown and Caldeira, 2017; Lee et al., 2017). However, synergetic estimations of components and the corresponding driving factors still pose great challenges and require full investigations.
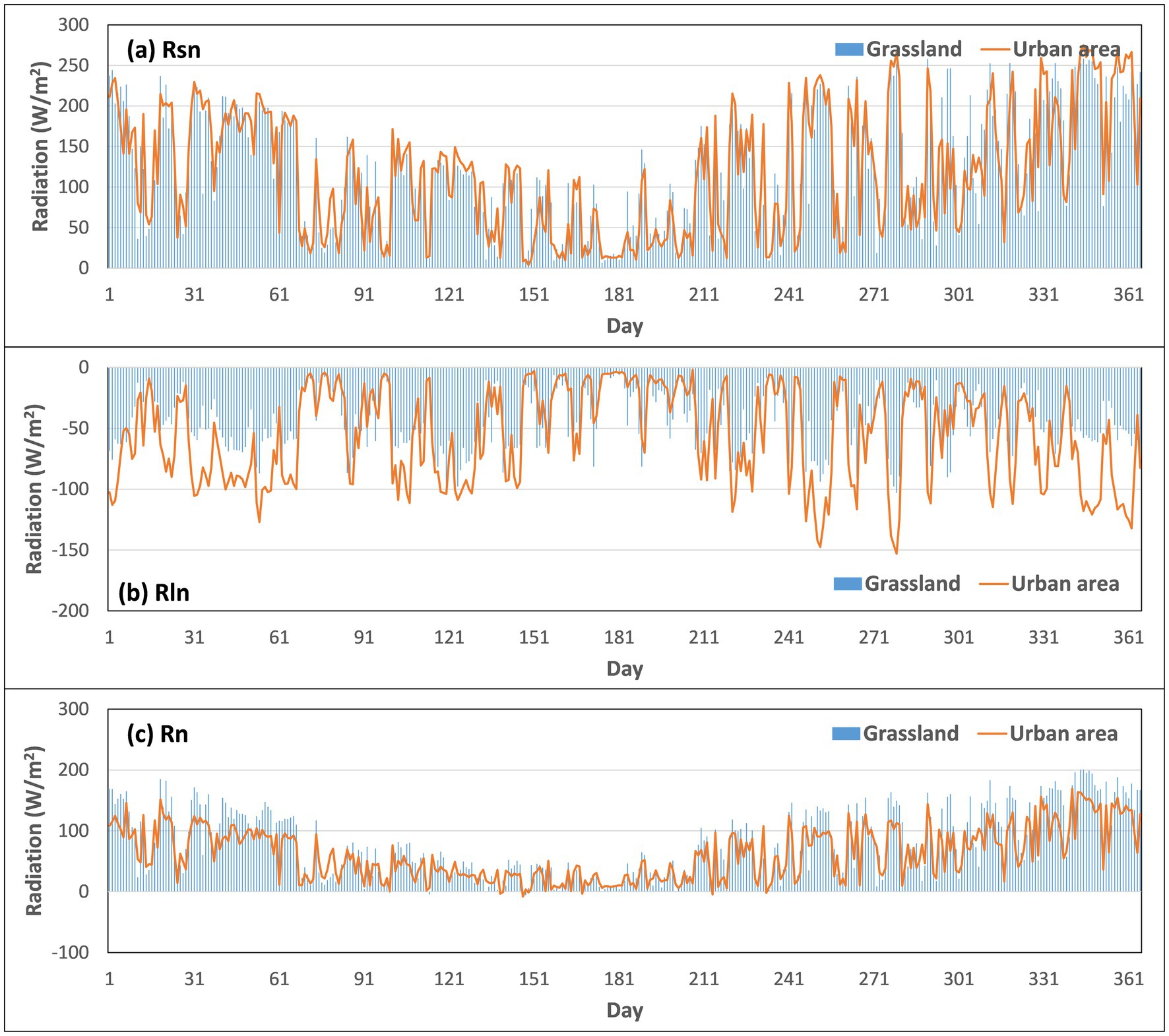
Figure 3. Temporal variations of surface radiation of (A) Rsn, (B) Rln, and (C) Rn in grassland and urban areas from Aug 1st in 2021 to July 31st in 2022.
We also explored monthly variations in surface radiation (Figure 4). Similar to the daily results, monthly shortwave radiation (Rsd and Rsu) is higher for grassland than the urban area, while longwave radiation (Rld and Rlu) shows the opposite pattern. Furthermore, the land cover-induced Rn difference mainly originates from variations in longwave radiation, with heterogeneous surface reflectivity and thermal emissivity being the main factors involved (Dickinson and Hanson, 1984; Foley et al., 2005; Zeng et al., 2017; Li et al., 2018; Feng et al., 2020). The monthly results help reveal the potential impacts of seasonal climatic change. Most of the radiation components show single peaks, with the maximum values occurring in July. Meanwhile, the land cover-induced Rn difference is also high in the summer, with the greatest variation (35.08 W2/m) in July. The main factor involved is the direct solar radiation of the subtropical humid area in the summer, which results in the highest levels of radiation reaching the surface.
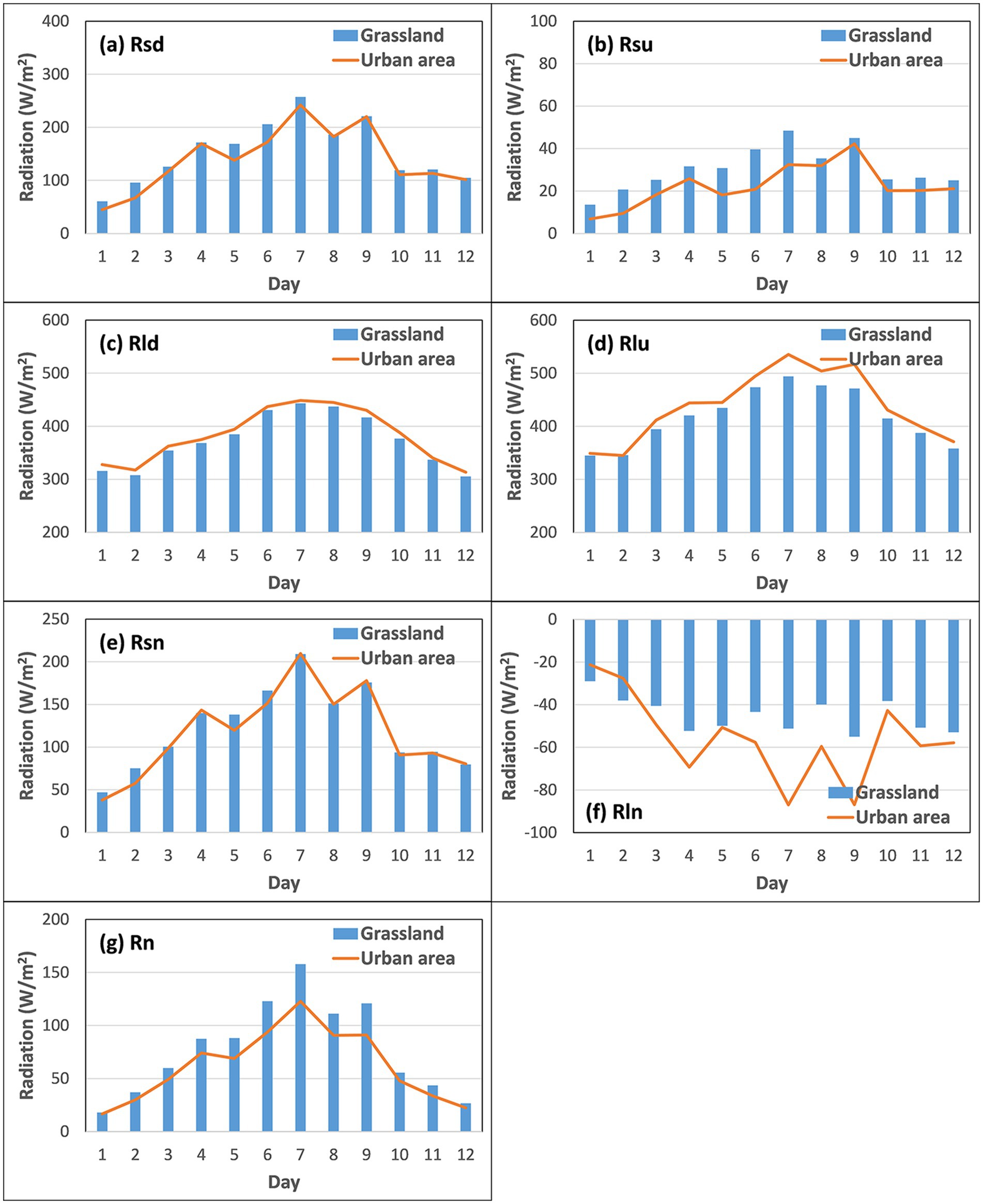
Figure 4. Monthly variations in surface radiation among grassland and urban areas for (A) Rsd, (B) Rsu, (C) Rld, (D) Rlu, (E) Rsn, (F) Rln, and (G) Rn.
3.2. Correlations with the climatic factors over different land covers
As mentioned in the “Introduction” section, the surface radiation budget strongly correlates with climate change, which might be affected by land covers with complex interactions. We therefore evaluated the correlations between the surface radiation budget and the climatic variables of precipitation, relative humidity, and atmospheric pressure over the grassland and urban areas (Figure 5). Generally, the radiation budget shows significant correlations with atmospheric pressure (0.54 of grassland, 0.41 of urban area) but relatively weak correlations with precipitation and relative humidity. Specifically, the radiation budget shows a negative correlation with atmospheric pressure. According to the Ideal-Gas Equation, warming air tends to amplify the volume of unit air, which would result in low atmospheric pressure. Meanwhile, the correlations between Rn and precipitation and relative humidity are relatively weak, suggesting complex interactions with other factors.
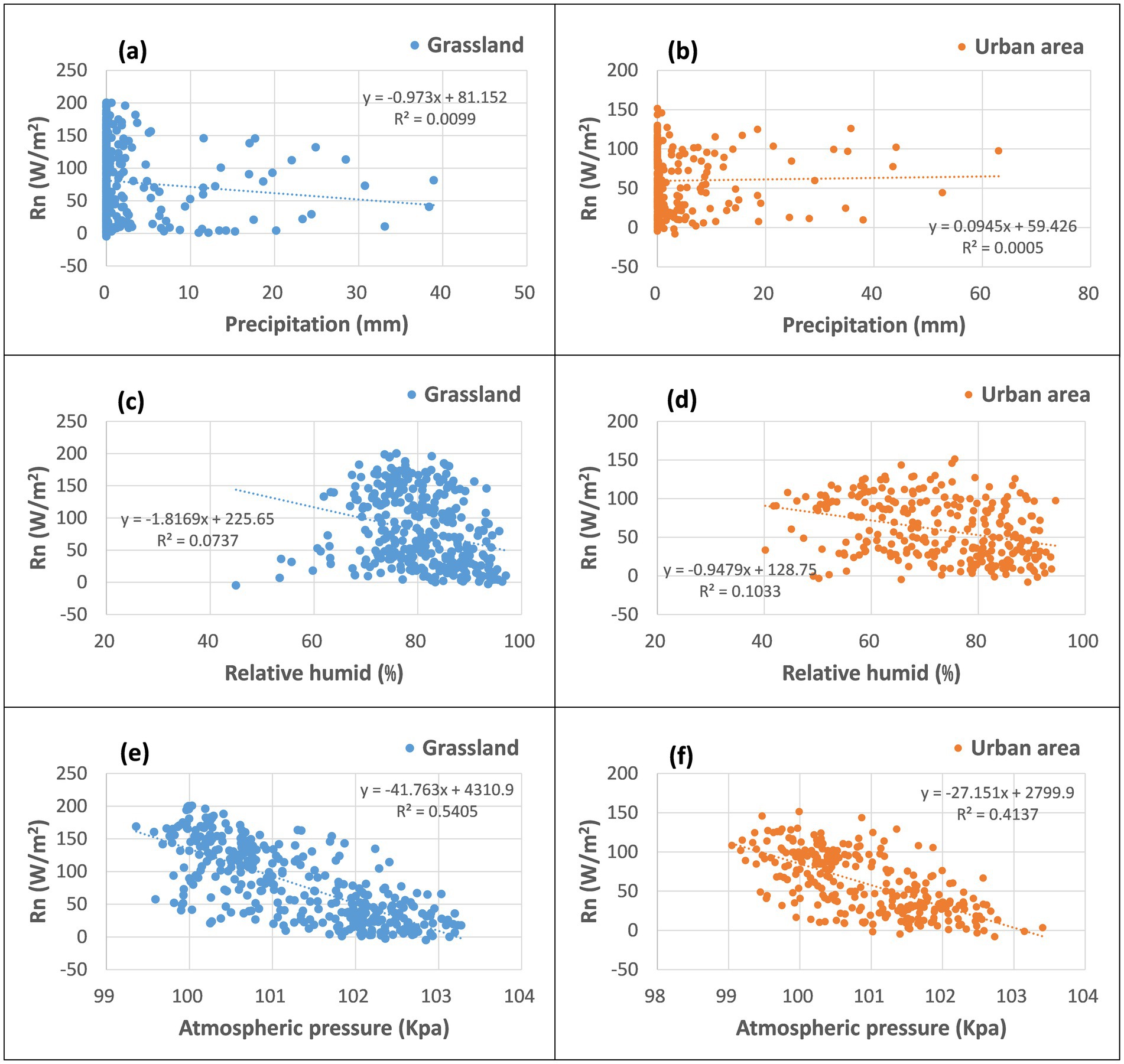
Figure 5. Correlations between Rn and the atmospheric variables of precipitation (A,B), relative humidity (C,D), and atmospheric pressure (E,F) in grassland and urban areas.
In addition, the correlations are more significant for grassland than for the urban area. This might mainly be attributed to the complex anthropogenic activities occurring in urban areas, weakening the correlations between the radiation budget and natural climatic factors. In urban areas, the components of radiation are strongly affected by anthropogenic activities of urbanization. For example, the high concentrations of artificial aerosols in urban areas exert significant radiative forcing, strongly altering surface budgets and weakening their correlations with atmospheric variables (Kim and Ramanathan, 2008; Myhre et al., 2013; Lee et al., 2016).
4. Discussion
Although only two in situ sites were used in this study, the precise observations provide meaningful information for detailed analysis of radiation variations. This would minimize uncertainties in modeling-or satellite-based investigations (particularly in studies of global climate change). Global climate change is highly sensitive to radiation variations, and even minor radiation fluctuations generate intensive climatic responses (Kothe and Ahrens, 2010; Kothe et al., 2011). Generally, the annual variation in global radiation is less than 1 W/m2 (Kiehl and Trenberth, 1997; Trenberth et al., 2009; Loeb et al., 2021). To capture this variation, the error of radiation should be less than the threshold. However, the uncertainties of most modeling and satellite products are much greater than such variation. For example, Rsn obtained from CERES_EBAF-Surface_Ed4.0 achieves a mean bias and root mean square error (RMSE) of 3.40 W·m−2 and 25.57 W·m−2, respectively, at the global scale with an uncertainty level of 20.00 W·m−2 (Jia et al., 2016; CERES, 2021). Therefore, the application of in situ observations is expected to validate and improve the performance of modeling-and satellite-based investigations.
The surface radiation budget acts as the predominant driver of global climate change. A positive radiation budget existed in the study area, suggesting intensive heatwave risk in the subtropical humid region (Yu et al., 2021). Because of the high biological diversity and terrestrial productivity of the subtropical humid region, the warming climate might trigger more complex ecological or environmental responses, potentially exerting serious impacts on human survival and development, which have drawn increasing attentions (Huang et al., 2007; Sniderman et al., 2019; Aspinwall et al., 2022). Temporally, the radiation components mainly peak in the summer particularly downward radiation. An increasing frequency and intensity of summer heatwaves have been reported in recent decades (Sun et al., 2014; Christidis et al., 2015; Luo et al., 2022). A full investigation of the correlation between radiation variations and heatwaves is required to reveal the physical mechanisms of extreme events and support relevant climatic adaptations.
Anthropogenic land cover change caused significant variations in the surface radiation budget, which should be given more attention in sustainable ecosystem management and planning. It is a major focus to clarify and identify driving factors of the surface radiation budget. Most researchers have focused on the climatic impacts of radiation absorption, emissions and scattering by greenhouse gasses (GHGs), atmospheric aerosols, and clouds (Ramanathan et al., 2007; Kim and Ramanathan, 2008; Boucher et al., 2013; Matus and L'Ecuyer, 2017; Feng and Zou, 2019). In addition to atmospheric influences, characteristics of the surface, particularly vegetation change, could also affect reflection and emission by altering the surface thermal properties of albedo and emissivity (Foley et al., 2005; Zeng et al., 2017). There is consensus that albedo is much lower in vegetated regions than in sparse regions due to strong radiative absorption in the visible and near-infrared bands by chlorophylls (Knipling, 1970; Campbell and Wynne, 2011). As a result, less radiation is reflected back to space. Meanwhile, the level of emissivity is relatively high because of the water content of vegetation, suggesting that more longwave radiation is expected to be emitted (Griend and Owe, 1993; Valor and Caselles, 1996). The warming effect of low albedo and the cooling effect of high emissivity leads to a complex impact of vegetation on the surface radiation budget (Kleidon et al., 2000). Meanwhile, climatic and surface factors affect radiation with complex nonlinear interactions, leaving a great gap in clarifying the associated individual contributions (Feichter et al., 2004), which requires a full investigation in future researches.
Unavoidable uncertainty remains in our work. The greatest uncertainty is related to the limited sites used for the investigation, which made it difficult to depict spatial radiation variations. As mentioned above, this study explored the physical mechanisms of radiation variations supported by precise ground observations. It is important to systematically analyze overall patterns and physical mechanisms across different spatiotemporal scales. Therefore, multiple sources should be integrated in future research, while continuous efforts in monitoring and analysis approaches are needed.
5. Conclusion
This study used ground observations to examine the variation in the surface radiation budget and the correlations with climatic variables over grassland and urban areas in a subtropical humid region. Several conclusions can be drawn from our results:
The radiation budget varied across different land covers. Specifically, there was relatively weak variation in Rsd between grassland (153.30 W/m2) and urban areas (140.11 W/m2), while Rsu presented relatively strong variations (30.61 W/m2 for grassland and 22.34 W/m2 in the urban area). With respect to longwave radiation, both Rld and Rlu were higher in the urban area (381.95 W/m2 and 437.80 W/m2) than for grassland (373.45 W/m2 and 418.55 W/m2). This is mainly attributed to homogeneous climate conditions and heterogeneous surface properties, which controlled solar radiation reaching the surface and the emission of longwave radiation. Monthly, most of the radiation components showed single peaks, with the maximum values occurring in July. Meanwhile, the land cover-induced Rn difference was also considerable in the summer, with the most variation (35.08 W2/m) occurring in July. Finally, the Rn was much greater for grassland (77.60 W2/m) than in the urban area (61.93 W2/m), which was mainly caused by the difference in longwave radiation.
Furthermore, the correlation between the radiation budget and atmospheric variables varied across different land covers, providing useful means to understand the natural and anthropogenic factors affecting the budget. Generally, the radiation budget showed obvious correlations with atmospheric pressure, while relatively weak correlations with precipitation and relative humidity. Furthermore, the correlations are more significant for grassland than for urban area. This might be mainly attributed to the complex anthropogenic activities occurring in urban areas, weakening the correlations between the radiation budget and natural climatic factors.
Our results could be used to support modeling-or satellite-based investigations, which tend to improve the performance and reliability of research on the radiation budget. Several issues should be addressed in future research. First, the physical mechanism between the radiation budget and climate change should be explored for sustainable land management and climatic adaptation. Second, the integration of in situ and satellite observations should be executed to improve the reliability of results for large regions. The full investigations above highlight the importance of research on the radiation budget and its impacts on global climatic and environmental change. Finally, although we analyzed the temporal variation of the radiation budget through two in-situ observations, the spatial variation would be explored by integrating the ground observation and satellite remote sensing in our future researches.
Data availability statement
The raw data supporting the conclusions of this article will be made available by the authors, without undue reservation.
Author contributions
HF contributed to conception and design of the study and wrote the manuscript. SW and JX collected the data. BZ and WW did some revision work. All authors contributed to manuscript revision, read, and approved the submitted version.
Funding
This work was supported in part by the National Natural Science Foundation of China [Grant No. 42071378], the Natural Science Foundation of Hunan Province [Grant No. 2020JJ3045], and the Open Fund of Key Laboratory of Urban Land Resources Monitoring and Simulation, Ministry of Natural Resources [Grant No. KF-2022-07-021].
Conflict of interest
The authors declare that they have no known competing financial interests or personal relationships that could have appeared to influence the work reported in this paper.
Publisher’s note
All claims expressed in this article are solely those of the authors and do not necessarily represent those of their affiliated organizations, or those of the publisher, the editors and the reviewers. Any product that may be evaluated in this article, or claim that may be made by its manufacturer, is not guaranteed or endorsed by the publisher.
Supplementary material
The Supplementary material for this article can be found online at: https://www.frontiersin.org/articles/10.3389/fevo.2023.1153733/full#supplementary-material
References
Allan, R. P., Soden, B. J., John, V. O., Ingram, W., and Good, P. (2010). Current changes in tropical precipitation. Environ. Res. Lett. 5:25205. doi: 10.1088/1748-9326/5/2/025205
Arnfield, A. J. (2003). Two decades of urban climate research: a review of turbulence, exchanges of energy and water, and the urban heat island. Int. J. Climatol. 23, 1–26. doi: 10.1002/joc.859
Aspinwall, M. J., Chieppa, J., Gray, E., Golden-Ebanks, M., and Davidson, L. (2022). Warming impacts on photosynthetic processes in dominant plant species in a subtropical forest. Physiol. Plant. 174:e13654. doi: 10.1111/ppl.13654
Betts, R. A. (2001). Biogeophysical impacts of land use on present-day climate: near-surface temperature change and radiative forcing. Atmos. Sci. Lett. 2, 39–51. doi: 10.1006/asle.2001.0023
Boucher, O., Randall, D., Artaxo, P., Bretherton, C., Feingold, G., Forster, P., et al. (2013). Clouds and aerosols. In: Climate change 2013: The physical science basis. Contribution of working group I to the fifth assessment report of the intergovernmental panel on climate change. Cambridge: Cambridge University Press.
Brown, P. T., and Caldeira, K. (2017). Greater future global warming inferred from Earth’s recent energy budget. Nature 552, 45–50. doi: 10.1038/nature24672
CERES. (2021). CERES_EBAF_Ed4.1 data quality summary. Available at: https://ceres.larc.nasa.gov/documents/DQ_summaries/CERES_EBAF_Ed4.1_DQS.pdf.
Chen, X.-L., Zhao, H.-M., Li, P.-X., and Yin, Z.-Y. (2006). Remote sensing image-based analysis of the relationship between urban heat island and land use/cover changes. Remote Sens. Environ. 104, 133–146. doi: 10.1016/j.rse.2005.11.016
Christidis, N., Jones, G. S., and Stott, P. A. (2015). Dramatically increasing chance of extremely hot summers since the 2003 European heatwave. Nat. Clim. Chang. 5, 46–50. doi: 10.1038/nclimate2468
Crutzen, P. J. (2004). New directions: the growing urban heat and pollution island effect–impact on chemistry and climate. Atmos. Environ. 38, 3539–3540. doi: 10.1016/j.atmosenv.2004.03.032
DeAngelis, A. M., Qu, X., Zelinka, M. D., and Hall, A. (2015). An observational radiative constraint on hydrologic cycle intensification. Nature 528, 249–253. doi: 10.1038/nature15770
Dickinson, R. E., and Hanson, B. (1984). “Vegetation-albedo feedbacks” in Climate processes and climate sensitivity. eds. J. E. Hansen, T. Takahashi (Washington, DC: American Geophysical Union), 180–186.
Dong, B., Wilcox, L. J., Highwood, E. J., and Sutton, R. T. (2019). Impacts of recent decadal changes in Asian aerosols on the east Asian summer monsoon: roles of aerosol–radiation and aerosol–cloud interactions. Clim. Dyn. 53, 3235–3256. doi: 10.1007/s00382-019-04698-0
Driemel, A., Augustine, J., Behrens, K., Colle, S., Cox, C., Cuevas-Agulló, E., et al. (2018). Baseline surface radiation network (BSRN): structure and data description (1992–2017). Earth Syst. Sci. Data 10, 1491–1501. doi: 10.5194/essd-10-1491-2018
Fan, J., Wang, X., Wu, L., Zhang, F., Bai, H., Lu, X., et al. (2018). New combined models for estimating daily global solar radiation based on sunshine duration in humid regions: a case study in South China. Energy Convers. Manag. 156, 618–625. doi: 10.1016/j.enconman.2017.11.085
Feichter, J., Roeckner, E., Lohmann, U., and Liepert, B. (2004). Nonlinear aspects of the climate response to greenhouse gas and aerosol forcing. J. Clim. 17, 2384–2398. doi: 10.1175/1520-0442(2004)017<2384:NAOTCR>2.0.CO;2
Feng, H., Liu, H., and Wu, L. (2014a). Monitoring the relationship between the land surface temperature change and urban growth in Beijing, China. IEEE J. Sel. Top. Appl. Earth Obs. Remote Sens. 7, 4010–4019. doi: 10.1109/JSTARS.2013.2264718
Feng, H., Xiong, J., Ye, S., Zou, B., and Wang, W. (2022). Vegetation change enhanced the positive global surface radiation budget. Adv. Space Res. 70, 324–335. doi: 10.1016/j.asr.2022.04.038
Feng, H., Ye, S., and Zou, B. (2020). Contribution of vegetation change to the surface radiation budget: a satellite perspective. Glob. Planet. Chang. 192:103225. doi: 10.1016/j.gloplacha.2020.103225
Feng, H., Zhao, X., Chen, F., and Wu, L. (2014b). Using land use change trajectories to quantify the effects of urbanization on urban Heat Island. Adv. Space Res. 53, 463–473. doi: 10.1016/j.asr.2013.11.028
Feng, H., and Zou, B. (2019). Satellite-based estimation of the aerosol forcing contribution to the global land surface temperature in the recent decade. Remote Sens. Environ. 232:111299. doi: 10.1016/j.rse.2019.111299
Foley, J. A., DeFries, R., Asner, G. P., Barford, C., Bonan, G., Carpenter, S. R., et al. (2005). Global consequences of land use. Science 309, 570–574. doi: 10.1126/science.1111772
Gago, E. J., Roldan, J., Pacheco-Torres, R., and Ordóñez, J. (2013). The city and urban heat islands: a review of strategies to mitigate adverse effects. Renew. Sust. Energ. Rev. 25, 749–758. doi: 10.1016/j.rser.2013.05.057
Griend, A. A. V. D., and Owe, M. (1993). On the relationship between thermal emissivity and the normalized difference vegetation index for natural surfaces. Int. J. Remote Sens. 14, 1119–1131. doi: 10.1080/01431169308904400
He, B., Wang, J., Zhu, J., and Qi, J. (2022). Beating the urban heat: situation, background, impacts and the way forward in China. Renew. Sust. Energ. Rev. 161:112350. doi: 10.1016/j.rser.2022.112350
Huang, M., Ji, J., Li, K., Liu, Y., Yang, F., and Tao, B. (2007). The ecosystem carbon accumulation after conversion of grasslands to pine plantations in subtropical red soil of South China. Tellus 59, 439–448. doi: 10.1111/j.1600-0889.2007.00280.x
Jia, A., Jiang, B., Liang, S., Zhang, X., and Ma, H. (2016). Validation and spatiotemporal analysis of CERES surface net radiation product. Remote Sens. 8:90. doi: 10.3390/rs8020090
Jin, M., Dickinson, R. E., and Zhang, D. (2005). The footprint of urban areas on global climate as characterized by MODIS. J. Clim. 18, 1551–1565. doi: 10.1175/JCLI3334.1
Kiehl, J. T., and Trenberth, K. E. (1997). Earth's annual global mean energy budget. Bull. Am. Meteorol. Soc. 78, 197–208. doi: 10.1175/1520-0477(1997)078<0197:EAGMEB>2.0.CO;2
Kim, D., and Ramanathan, V. (2008). Solar radiation budget and radiative forcing due to aerosols and clouds. J. Geophys. Res. Atmos. 113:D02203. doi: 10.1029/2007JD008434
Kleidon, A., Fraedrich, K., and Heimann, M. (2000). A green planet versus a desert world: estimating the maximum effect of vegetation on the land surface climate. Clim. Chang. 44, 471–493. doi: 10.1023/A:1005559518889
Knipling, E. B. (1970). Physical and physiological basis for the reflectance of visible and near-infrared radiation from vegetation. Remote Sens. Environ. 1, 155–159. doi: 10.1016/S0034-4257(70)80021-9
Kothe, S., and Ahrens, B. (2010). On the radiation budget in regional climate simulations for West Africa. J. Geophys. Res. Atmos. 115:D014331. doi: 10.1029/2010JD014331
Kothe, S., Dobler, A., Beck, A., and Ahrens, B. (2011). The radiation budget in a regional climate model. Clim. Dyn. 36, 1023–1036. doi: 10.1007/s00382-009-0733-2
Lean, J., and David, R. (2009). Climate forcing by changing solar radiation. J. Clim. 11, 3069–3094. doi: 10.1175/1520-0442(1998)011<3069:CFBCSR>2.0.CO;2
Lee, S., Gong, T., Feldstein, S. B., Screen, J. A., and Simmonds, L. (2017). Revisiting the cause of the 1989–2009 Arctic surface warming using the surface energy budget: downward infrared radiation dominates the surface fluxes. Geophys. Res. Lett. 44:75375. doi: 10.1002/2017GL075375
Lee, L. A., Reddington, C. L., and Carslaw, K. S. (2016). On the relationship between aerosol model uncertainty and radiative forcing uncertainty. Proc. Natl. Acad. Sci. U. S. A. 113, 5820–5827. doi: 10.1073/pnas.1507050113
Li, H., Cao, F., Bu, X., and Zhao, L. (2015). Models for calculating daily global solar radiation from air temperature in humid regions—a case study. Environ. Prog. Sustain. Energy 34, 595–599. doi: 10.1002/ep.12018
Li, Q., Ma, M., Wu, X., and Yang, H. (2018). Snow cover and vegetation-induced decrease in global albedo from 2002 to 2016. J. Geophys. Res. Atmos. 123, 124–138. doi: 10.1002/2017JD027010
Loeb, N. G., Johnson, G. C., Thorsen, T. J., Lyman, J. M., Rose, F. G., and Kato, S. (2021). Satellite and ocean data reveal marked increase in Earth’s heating rate. Geophys. Res. Lett. 48:e2021GL093047. doi: 10.1029/2021GL093047
Luo, M., Lau, N.-C., Liu, Z., Wu, S., and Wang, X. (2022). An observational investigation of spatiotemporally contiguous heatwaves in China from a 3D perspective. Geophys. Res. Lett. 49:e2022GL097714. doi: 10.1029/2022GL097714
Mahmood, R., and Hubbard, K. G. (2005). Assessing bias in evapotranspiration and soil moisture estimates due to the use of modeled solar radiation and dew point temperature data. Agric. For. Meteorol. 130, 71–84. doi: 10.1016/j.agrformet.2005.02.004
Manoli, G., Fatichi, S., Bou-Zeid, E., and Katul, G. G. (2020). Seasonal hysteresis of surface urban heat islands. PNAS 117, 7082–7089. doi: 10.1073/pnas.1917554117
Matus, A. V., and L'Ecuyer, T. S. (2017). The role of cloud phase in Earth's radiation budget. J. Geophys. Res. Atmos. 122, 2559–2578. doi: 10.1002/2016JD025951
Monteith, J. L. (1972). Solar radiation and productivity in tropical ecosystems. J. Appl. Ecol. 9, 747–766. doi: 10.2307/2401901
Mustafa, J., Husain, S., Khan, U. A., and Akhtar, M. M. (2022). Prediction of diffuse solar radiation using machine learning models based on sunshine period and sky-clearness index for the humid-subtropical climate of India. Environ. Prog. Sustain. Energy 42:e13973. doi: 10.1002/ep.13973
Myhre, G., Shindell, D., Bréon, F., Collins, W., Fuglestvedt, J., Huang, J., et al. (2013). Anthropogenic and natural radiative forcing. Climate Change 423, 658–740.
Palmer, M. D. (2012). Climate and Earth's energy flows. Surv. Geophys. 33, 351–357. doi: 10.1007/s10712-011-9165-8
Penner, J. E., Dickinson, R. E., and O'Neill, C. A. (1992). Effects of aerosol from biomass burning on the global radiation budget. Science 256, 1432–1434. doi: 10.1126/science.256.5062.1432
Peters, M. K., Hemp, A., Appelhans, T., Becker, J. N., Behler, C., Classen, A., et al. (2019). Climate–land-use interactions shape tropical mountain biodiversity and ecosystem functions. Nature 568, 88–92. doi: 10.1038/s41586-019-1048-z
Pinker, R. T., Zhang, B., and Dutton, E. G. (2006). Do satellites detect trends in surface solar radiation? Science 308, 850–854. doi: 10.1126/science.1103159
Ramanathan, V., and Collins, W. (1991). Thermodynamic regulation of ocean warming by cirrus clouds deduced from observations of the 1987 El Nino. Nature 351, 27–32. doi: 10.1038/351027a0
Ramanathan, V., Ramana, M. V., Roberts, G., Kim, D., Corrigan, C., Chung, C., et al. (2007). Warming trends in Asia amplified by brown cloud solar absorption. Nature 448, 575–578. doi: 10.1038/nature06019
Sirdas, S., and Duran, A. (2008). Relationship between drought and solar irradiation variables. Hydrol. Process. 22, 1460–1472. doi: 10.1002/hyp.6698
Sniderman, J. M. K., Brown, J. R., Woodhead, J. D., King, A. D., Gillett, N. P., Tokarska, K. B., et al. (2019). Southern hemisphere subtropical drying as a transient response to warming. Nat. Clim. Chang. 9, 232–236. doi: 10.1038/s41558-019-0397-9
Sun, Y., Zhang, X., Zwiers, F. W., Song, L., Wan, H., Hu, T., et al. (2014). Rapid increase in the risk of extreme summer heat in eastern China. Nat. Clim. Chang. 4, 1082–1085. doi: 10.1038/nclimate2410
Trenberth, K. E., Fasullo, J. T., and Kiehl, J. (2009). Earth's global energy budget. Bull. Am. Meteorol. Soc. 90, 311–324. doi: 10.1175/2008BAMS2634.1
Tubiello, F. N., Salvatore, M., Ferrara, A. F., House, J., Federici, S., Rossi, S., et al. (2015). The contribution of agriculture, forestry and other land use activities to global warming, 1990–2012. Glob. Chang. Biol. 21, 2655–2660. doi: 10.1111/gcb.12865
Valor, E., and Caselles, V. (1996). Mapping land surface emissivity from NDVI: application to European, African, and south American areas. Remote Sens. Environ. 57, 167–184. doi: 10.1016/0034-4257(96)00039-9
Wild, M., Folini, D., Hakuba, M. Z., Schär, C., Seneviratne, S. I., Kato, S., et al. (2015). The energy balance over land and oceans: an assessment based on direct observations and CMIP5 climate models. Clim. Dyn. 44, 3393–3429. doi: 10.1007/s00382-014-2430-z
Wild, M., Gilgen, H., Roesch, A., Ohmura, A., Long, C. N., Dutton, E. G., et al. (2005). From dimming to brightening: decadal changes in solar radiation at Earth's surface. Science 308, 847–850. doi: 10.1126/science.1103215
Wohl, E., Barros, A., Brunsell, N., Chappell, N. A., Coe, M., Giambelluca, T., et al. (2012). The hydrology of the humid tropics. Nat. Clim. Chang. 2, 655–662. doi: 10.1038/nclimate1556
Xie, S.-P., Kosaka, Y., and Okumura, Y. M. (2015). Distinct energy budgets for anthropogenic and natural changes during global warming hiatus. Nat. Geosci. 9, 29–33. doi: 10.1038/ngeo2581
Yang, X., Zhao, C., Guo, J., and Wang, Y. (2016). Intensification of aerosol pollution associated with its feedback with surface solar radiation and winds in Beijing. J. Geophys. Res. Atmos. 121, 4093–4099. doi: 10.1002/2015JD024645
Ye, S., Feng, H., Zou, B., Ding, Y., Zhu, S., Li, F., et al. (2021). Satellite-based estimation of the influence of land use and cover change on the surface shortwave radiation budget in a Humid Basin. Remote Sens. 13:1447. doi: 10.3390/rs13081447
Yeh, S. W., Kug, J. S., and An, S. I. (2014). Recent progress on two types of El Nio: observations, dynamics, and future changes. Asia-Pac. J. Atmos. Sci. 50, 69–81. doi: 10.1007/s13143-014-0028-3
Yu, G., Chen, Z., Piao, S., Peng, C., Ciais, P., Wang, Q., et al. (2014). High carbon dioxide uptake by subtropical forest ecosystems in the east Asian monsoon region. PNAS 111, 4910–4915. doi: 10.1073/pnas.1317065111
Yu, Y., Shao, Q., Lin, Z., and Kang, I.-S. (2021). Characteristics analysis and synoptic features of event-based regional heatwaves over China. J. Geophys. Res. Atmos. 126:e2020JD033865. doi: 10.1029/2020JD033865
Zeng, Z., Piao, S., Li, L. Z. X., Zhou, L., Ciais, P., Wang, T., et al. (2017). Climate mitigation from vegetation biophysical feedbacks during the past three decades. Nat. Clim. Chang. 7, 432–436. doi: 10.1038/nclimate3299
Zhang, H., and Zhu, X. (2009). Study on the dynamic monitoring and succession analysis of wetland types in Dongting Lake area. 2009 2nd IEEE International Conference on Computer Science and Information Technology.
Zhao, J., Ma, J., and Zhu, Y. (2019). Evaluating impacts of climate change on net ecosystem productivity (NEP) of global different forest types based on an individual tree-based model FORCCHN and remote sensing. Glob. Planet. Chang. 182:103010. doi: 10.1016/j.gloplacha.2019.103010
Keywords: surface radiation budget, land cover, climate change, ground observation, subtropical humid region
Citation: Feng H, Wang S, Xiong J, Zou B and Wang W (2023) Variation in the surface radiation budget over different land covers in a subtropical humid region: evidence from ground observations. Front. Ecol. Evol. 11:1153733. doi: 10.3389/fevo.2023.1153733
Edited by:
Shuisen Chen, Guangzhou Institute of Geography, ChinaReviewed by:
Kun Jia, Beijing Normal University, ChinaJi Zhou, University of Electronic Science and Technology of China, China
Copyright © 2023 Feng, Wang, Xiong, Zou and Wang. This is an open-access article distributed under the terms of the Creative Commons Attribution License (CC BY). The use, distribution or reproduction in other forums is permitted, provided the original author(s) and the copyright owner(s) are credited and that the original publication in this journal is cited, in accordance with accepted academic practice. No use, distribution or reproduction is permitted which does not comply with these terms.
*Correspondence: Wei Wang, d2FuZ3dlaWNuQGNzdS5lZHUuY24=