- 1State Key Laboratory of Freshwater Ecology and Biotechnology, Institute of Hydrobiology, Chinese Academy of Sciences, Wuhan, China
- 2College of Advanced Agricultural Sciences, University of Chinese Academy of Sciences, Beijing, China
- 3Wuhan Sinoeco Ecological Science & Technology Co., Ltd., Wuhan, China
Biodiversity and ecosystem functioning (BEF) relationships are important research issues in the context of biodiversity loss. However, most studies only explored BEF relationships using species richness as a proxy for biodiversity without considering the effects of species evenness and importance of functional diversity on ecosystem functioning. In the present study, we explored multiple relationships among in-stream environmental variables, species diversity (species richness and species evenness), functional diversity (functional richness, functional evenness and Rao’s quadratic entropy index) and biomass of benthic diatom communities from a Chinese mountainous river network. We tested two hypotheses: (1) species richness and evenness affect community biomass independently, and (2) species diversity directly affects community biomass and also indirectly affects community biomass by influencing functional diversity. We found that benthic diatom diversity and biomass were influenced by wetted width, conductivity, and pH. Species richness and evenness had positive and negative effects on community biomass, respectively; while, the effect of richness (with a pathway coefficient of 0.39) was stronger than that of evenness (−0.28). However, we have not found an interaction pathway between species richness and species evenness in structural equation models, indicating that the two effects on community biomass were independent of each other. Both functional richness and Rao’s quadratic entropy index had positive effects on community biomass, although effects of other variables blurred the relationship between functional richness and community biomass in structural equation modeling. Besides direct effects, species diversity also indirectly affected community biomass through influencing Rao’s quadratic entropy index, with direct effects (0.27) were more than twice stronger than indirect effects (0.12). Our findings imply that the selection effect played a dominant role in the relationship between diatom diversity and biomass, indicated by the negative species evenness and biomass relationship; meanwhile, the complementarity effect was also important. The inclusion of more diversity indices to explore the biodiversity-biomass relationship would help to provide a deeper and more comprehensive understanding of this relationship and its driving mechanisms. We suggest carrying out more studies on BEF relationships in streams and rivers to support protection practices for sustaining biodiversity and their ecosystem functions in natural lotic ecosystems.
1. Introduction
The biodiversity and ecosystem functioning (BEF) relationship has attracted more and more attention in the context of unprecedented loss of biodiversity in recent decades (Chapin et al., 2000; Cardinale et al., 2012; Tilman et al., 2014; Malhi et al., 2020). As a result, numerous studies ranging from controlled experiments to field observations have been carried out to explore how ecosystem functioning responds to changes in biodiversity. Most studies discovered positive biodiversity effects on community functioning such as community biomass production, stability, and nutrient uptake as commonly expected (Baert et al., 2016; Duffy et al., 2017; Barry et al., 2020). Meanwhile, negative, non-significant, or environmental context-dependent BEF relationships were also reported (Dangles et al., 2011; van der Plas, 2019). Inconsistent BEF relationships stimulated further exploration of mechanisms underlying BEF relationships and two general mechanisms for positive BEF relationships were proposed: the complementarity effect and the selection effect (Garnier et al., 1997; Tilman et al., 1997; Loreau and Hector, 2001). The complementarity effect refers to that when species diversity increases in a community, complementary niches between species will promote resource utilization, thus enhancing ecosystem functioning. By contrast, the selection effect suggests that when species diversity increases, the possibility of species with highly productive traits in the community increases, and these species may become dominant and thus contribute more to ecosystem functioning. Vanelslander et al. (2009) found that mixtures contributed more to production than most productive component species in naturally intertidal mudflats diatom communities, indicating that the positive diversity effects were most driven by complementarity effects. However, a study on fish communities found that high fish biomass was associated with a few dominant species who can exploit a wider range of energy, which indicated the importance of selection effects (Maureaud et al., 2019). The two effects usually coexist in a given assemblage and jointly drive a positive species richness-biomass relationship, with one being dominant and the other subordinate (Polley et al., 2003; Hillebrand et al., 2008; Smeti et al., 2019).
Although our knowledge on BEF relationships is steadily growing, some issues are still unresolved. One issue is whether more indices, besides richness, should be concerned to indicate species diversity in BEF relationship studies. Species richness was the most commonly used diversity index as it means the number of species in a given assemblage. Although species richness is an intuitive measure of biodiversity, it does not provide information on species abundance distribution that would also affect ecosystem functioning mechanically. Species evenness, i.e., a measure indicating how equitably distribution of species abundance within a given community, together with species richness, has been included in recent BEF relationship studies. Species richness and species evenness cover different diversity components, and many studies have shown they are not always positively correlated, so their effects on biomass are not always consistent (Hillebrand et al., 2008). Positive, negative, and non-significant relationships between evenness and ecosystem functioning were observed (Mulder et al., 2004; Passy and Larson, 2019; Sonkoly et al., 2019). Differences in relationships between the two species diversity indices and ecosystem functioning imply variable importance of the two underlying mechanisms (Ali et al., 2018; Maureaud et al., 2019). When the BEF relationship is mainly driven by the complementarity effect, it is the number of species rather than the degree of species dominance that determine community functioning, and there would be a non-significant or positive evenness-functioning relationship. In contrast, if the selection effect is the main mechanism, the dominant species will contribute most to community functioning, thus a negative evenness-functioning relationship will emerge while the species richness-functioning relationship is still positive. Various evenness-functioning relationships were supported by some controlled experiments with a small number of species (Polley et al., 2007; Isbell et al., 2009). However, it is unclear whether both species richness and evenness would be important to ecosystem functioning in natural ecosystems where species are generally diverse while species abundance distribution is severely uneven.
Another issue is whether functional diversity should also be considered in BEF relationship studies. Some researchers suggested that species diversity affected ecosystem functioning via functional diversity (Hooper et al., 2005). Functional diversity is a quantification of the distribution of species traits within a community, considered as a proxy for niche or species function. Therefore, functional diversity has a mechanistic link with ecosystem functioning (Cadotte et al., 2011; Chao et al., 2014). Like species diversity, functional diversity also has quantitative indices with different focuses, such as functional richness, functional evenness, functional divergence and other trait-based indices. Functional diversity usually increases with species richness in communities that with inconspicuous functional redundancy. For a given community, high functional diversity may lead to more efficient utilization of resources, thereby enhancing ecosystem functioning. Moreover, functional diversity may be associated with the portion of functional changes caused by community turnover that cannot be captured by species diversity (Cadotte et al., 2011). For instance, the number of traits may change when community structure changes while species richness remains constant. However, research on couplings between functional diversity and ecosystem functioning, as well as pathways that species diversity affects ecosystem functioning through functional diversity, remains scarce, especially for natural ecosystems.
Natural streams and rivers exhibit a disproportionately high biodiversity, possessing greater diversity in smaller area compared to terrestrial ecosystems; nevertheless, lotic ecosystems are highly threatened by human activities (Strayer and Dudgeon, 2010; Geist, 2011). Therefore, exploring BEF relationships in lotic ecosystems could provide meaningful guidance for conservation practices. However, compared with other ecosystems, studies on species diversity-functional diversity-ecosystem functioning relationships in lotic ecosystems are scarce. In the present study, we explored multiple relationships among species diversity, functional diversity, and community biomass of benthic diatom assemblages by considering multiple biodiversity indices in a subtropical mountainous river network in China. Benthic diatoms are main primary producers in mountainous rivers with diverse ecological functions and services (Litchman, 2007; Burliga and Kociolek, 2016; Rhodes et al., 2017; Zhang et al., 2020). Diatoms have a high species richness while only a handful of species have high abundance (low evenness). The primary aims of this study were twofold: (1) to analyze the magnitude and direction of the effects of diatom species richness and evenness on community biomass, with the goal of providing insight into the underlying mechanisms driving the diversity-biomass relationships; and (2) to elucidate the pathways through which species diversity impacts diatom community biomass, not only directly but also indirectly through influencing functional diversity.
2. Materials and methods
2.1. Study area
The Xiangxi river originates from the Shennongjia Forest Region in western Hubei province of China. The river is 94 km long with a watershed area of 3099 km2, and includes three tributaries Jiuchong, Gufu, and Gaolan rivers (Tang et al., 2006; Wang et al., 2012). It is characterized by a subtropical monsoon climate zone. The annual average temperature is 12–19°C and annual average precipitation is 1000–1300 mm (Wang et al., 2011). In this mountainous watershed (210–2000 m above sea level), the summers are hot and humid and the winters are cold and dry (Tang et al., 2017). The main land use is forest but scattered with farmland and towns and generally low levels of human disturbance within the watershed (Li et al., 2009; Jin et al., 2019).
2.2. Diatom collection and data preparation
We surveyed 76 sites across the Xiangxi River network in March/April 2013 during the base-flow period (Figure 1). At each site, 15 stones were randomly collected along a 100 m length of river reach. A plastic circle of 2.5 cm radius was used to quantity sampling area by covering the stone surface. The circled areas were cleaned using a scrubbing brush and washed several times with stream water. Algal slurry from the 15 stones was collected into one container and the slurry were volumed to 550 mL. Then, the algal slurry was transferred to the specimen bottle and fixed with 5% formaldehyde solution in the field. After brought back to the laboratory, several milliliter algal samples were used to make diatom slides following the acidification procedure (Racca et al., 2003; Hu and Wei, 2006). Then, diatoms were identified to the species or variety level at 1,000× magnification under a compound microscope (Olympus CX21: Olympus Optical Co., Japan). A minimum of 600 diatom valves were counted for each site. Diatom identification was performed according to (Lange-Bertalot and Krammer, 2000; Hu and Wei, 2006; Li and Qi, 2010, 2018). Relative abundance and density of each component species were calculated for each site based on counting data. Species richness and Pielou’s evenness index (i.e., species evenness) were also calculated using R package vegan (Oksanen et al., 2019).
Algal traits, including cell sizes, life forms and morphological guilds, were assigned to each of observed species (Supplementary Table 1). Cell sizes were classified into nano, micro, meso, macro or very large categories, and it is considered as response associated with available resources (Finkel et al., 2009; Berthon et al., 2011; Rimet and Bouchez, 2012). It is obvious that assemblages with more macroalgae can have higher biomass. Life forms were categorized as non-colonial group or colonial group (Lange et al., 2016). The colonial diatom species usually exist as form of diatom chains, which have different resources utilization strategies and interactions with predators compared to single cell diatoms (Kenitz et al., 2020). Morphological guilds contained high profile, low profile, motile and planktonic guilds (Passy, 2007; Stenger-Kovacs et al., 2013). These guilds response differently to turbulence and nutrient enrichment, lead to different growth conditions in varied environments (Passy, 2007; Ding et al., 2017). Functional diversity indices including functional richness (FRic), functional evenness (FEve) and Rao’s quadratic entropy index (RaoQ) were calculated for each site based on the relative abundance data and trait data (Villeger et al., 2008). Functional richness refers to the size of niche space occupied by species in a community, while functional evenness refers to the evenness of functional traits distribution in niche space (Petchey et al., 2004). Rao’s quadratic entropy index is one of the generalized forms of Simpson’s diversity index (Cibils et al., 2015; Mammola et al., 2021), which takes both functional richness and functional evenness into account and reflects the probability that two individuals randomly selected from the community that are functionally different. And functional diversity indices were calculated using package FD (Laliberté et al., 2014).
Diatom community biomass was estimated for each site as the sum of biovolume of all component species at the site, considering that it represents an accurate measure of algal biomass (Stevenson, 1996). The biovolume for each diatom species was calculated using its density multiplying the geometric equation supposed by Hillebrand et al. (1999), with length, width and height data measured under the microscope during the identification process.
2.3. In-stream environmental variables
At each site, in-stream environmental variables were also monitored during the fieldwork. Flow velocity (m/s) was measured with an LJD-10 water current meter. Wetted width (m) and water depth (cm) were measured with a measuring tape. Wetted width was averaged after three measurements at each site, and flow velocity and water depth were averaged after five measurements at each site to increase credibility. Hydration indices pH, dissolved oxygen (DO, mg/L), conductivity (Cond, μs/cm), and total dissolved solids (TDS, mg/L) were measured with a multi-parameter probe (HACHHQ40d, Yellow Springs Instruments, United States). An additional 100 mL of river water was collected into a clean polythene bottle and adjusted to pH < 2 by adding concentrated sulfuric acid in the field. After transported to the laboratory, water chemical variables including total nitrogen (TN, mg/L), total phosphorus (TP, mg/L), ammonia nitrogen (NH4-N, mg/L) and phosphate (PO4-P, mg/L) were determined by a continuous flow water quality analyzer (SAN++, Skalar) according to standard methods (MEE, 2003).
2.4. Data analysis
A preliminary analysis was conducted to determine correlations among environmental variables. Environmental variables that were highly correlated (Pearson r > 0.8) with other variables but weakly correlated with biological indices of diatom assemblages were excluded from further analysis to reduce collinearity. Then, all remained environmental variables, except pH, as well as diatom species and functional diversity indices and biomass data were log(x + 1) transformed to reduce influences of extreme values.
Firstly, bivariate regression was used to explore relationships among environmental variables, diatom species diversity indices, functional diversity indices, and community biomass. This simple analysis can provide preliminary information on BEF relationships for further confirmation (Grace et al., 2014). Considering the possible non-linear relationships, we fitted both linear and quadratic models between variables, and then chose the model with the larger R2 and smaller p value for subsequent analysis (Chettri and Acharya, 2020).
Then, multiple relationships among in-stream environments, diatom diversity, and community biomass were tested using structural equation modeling (SEM). SEM is an extension of the general linear model, in which a variable can be either a predictor or a response variable, and is a confirmatory tool for a priori established hypotheses (Grace and Grace, 2006; Asparouhov and Muthen, 2009). Significant bivariate relationships would be excluded from the SEM if the relationships may reduce the overall reliability of the model (Grace and Grace, 2006). We used piecewise SEM to conduct confirmation analysis. Compared with traditional SEM, piecewise SEM relaxes the restrictions on data distribution with only requirement of degrees of freedom, which has obvious advantages in practical application where sample sizes are often small (Lefcheck, 2016). We constructed two alternative models differing in excluding or including functional diversity indices in the model to test the importance of functional diversity in the relationship between diatom diversity and biomass (Supplementary Figure 1). Model 1 included in-stream important environmental variables and species diversity indices of diatom identified using bivariate regressions and diatom community biomass. We assumed that in-stream environments had direct effects on species diversity and community biomass, and community biomass is also influenced by species diversity. Compared to model 1, model 2 also included important indicators of diatom functional diversity determined by binary regression, with two additional pathways: (1) in-stream environments affect community biomass via functional diversity, and (2) functional diversity affect community biomass; simultaneously, species diversity affect community biomass via functional diversity. Composite variables were constructed in SEMs to represent nonlinear relationships identified by bivariate regressions (Grace and Bollen, 2008; Song et al., 2013). A composite variable was constructed by extracting coefficient estimates of the linear and quadratic term of the predictors and then multiplying the linear and quadratic term. The linear and quadratic terms of the given variable in the model were replaced by their composite variable for subsequent fitting. To fit the model, the predictors with the maximum non-significant p value (>0.05) were successively removed, and the model was refitted until all predictors had p values less than 0.05. Then, statistically significant (p < 0.05) and ecologically meaningful pathways were added sequentially according to the degree of significance, and model was refitted. The model with all significant pathways (p < 0.05), insignificant Chi-square test statistics (p > 0.05), and the lowest AIC (Akaike’s Information Criterion), was selected as the final model. Model 1 and 2 were fitted separately. Piecewise SEMs were implemented using R package piecewiseSEM (Lefcheck, 2016).
3. Results
Values of most in-stream environmental variables were low (Supplementary Table 2). Wetted width varied from 1.26 to 31.50 m among sampling sites; while velocity and depth had a relatively small range of 0.09–0.93 m/s and 6.6–41.6 cm, respectively. The average concentrations of NH4-N (0.070 mg/L), TP (0.031 mg/L), and DO (9.51 mg/L) met the class I standard of the Chinese environmental quality standards for surface water (SEPA, 2002), respectively. By contrast, the average concentrations of TN (1.000 mg/L) met the class III standard. The mean value of total dissolved solids (173.8 mg/L) and conductivity (207.3 μs/cm) were also low. The stream water was weakly alkaline with a mean pH of 8.36.
A total of 150 diatom species or varieties were identified. Cocconeis placentula Ehrenberg (with an average relative abundance of 33.76%), Achnanthidium minutissimuma Kützing (23.95%) and Achnanthidium lineare Smith (5.54%) were the three predominant species with an average relative abundance >5%. Another seven species were also abundant, with an average relative abundance >1%, including Achnanthidium biasolettianum Grunow (4.99%), Rossithidium pusillum Grunow (4.70%), Cocconeis pediculus Ehrenberg (3.94%), Achnanthidium subatomus Hustedt (2.38%), Achnanthidium caledonicum Lange-Bertalot (1.87%), Reimeria sinuata W. Gregory (1.46%) and Gomphonema tenuissimum Fricke (1.18%). Mean relative abundances of the other 140 species were less than 1%.
Diatom species richness among all sites varied between 4 and 38 with a mean richness of 16 taxa. Of all sites, only 12 sites had richness less than 10 taxa. Pielou’s evenness varied from 0.714 to 0.976, with a mean value of 0.881. The mean values for functional richness, evenness, and Rao’s quadratic entropy index were 8.40 (range: 0.162–13.785), 0.392 (0.122–0.676), and 5.741 (1.388–15.549), respectively. Diatom community biomass varied from 2.91 × 106 to 4.78 × 108 μm3/cm2, with mean biomass of 7.09 × 107 μm3/cm2. For each site, dominant species with mean relative abundance >1% contributed disproportionately to community biomass that averagely 97.2% of the entire community biomass.
3.1. Bivariate relationships among environmental variables, diatom diversity indices and community biomass
There were positive linear relationships between wetted width and species richness and biomass, between conductivity and species evenness, and between pH and diatom community biomass (Figures 2A,B,D,E). A negative linear relationship was found between conductivity and two diatom indices of species richness and FRic. Significant quadratic relationships were identified between conductivity, water depth and community biomass (Figures 2C,F).
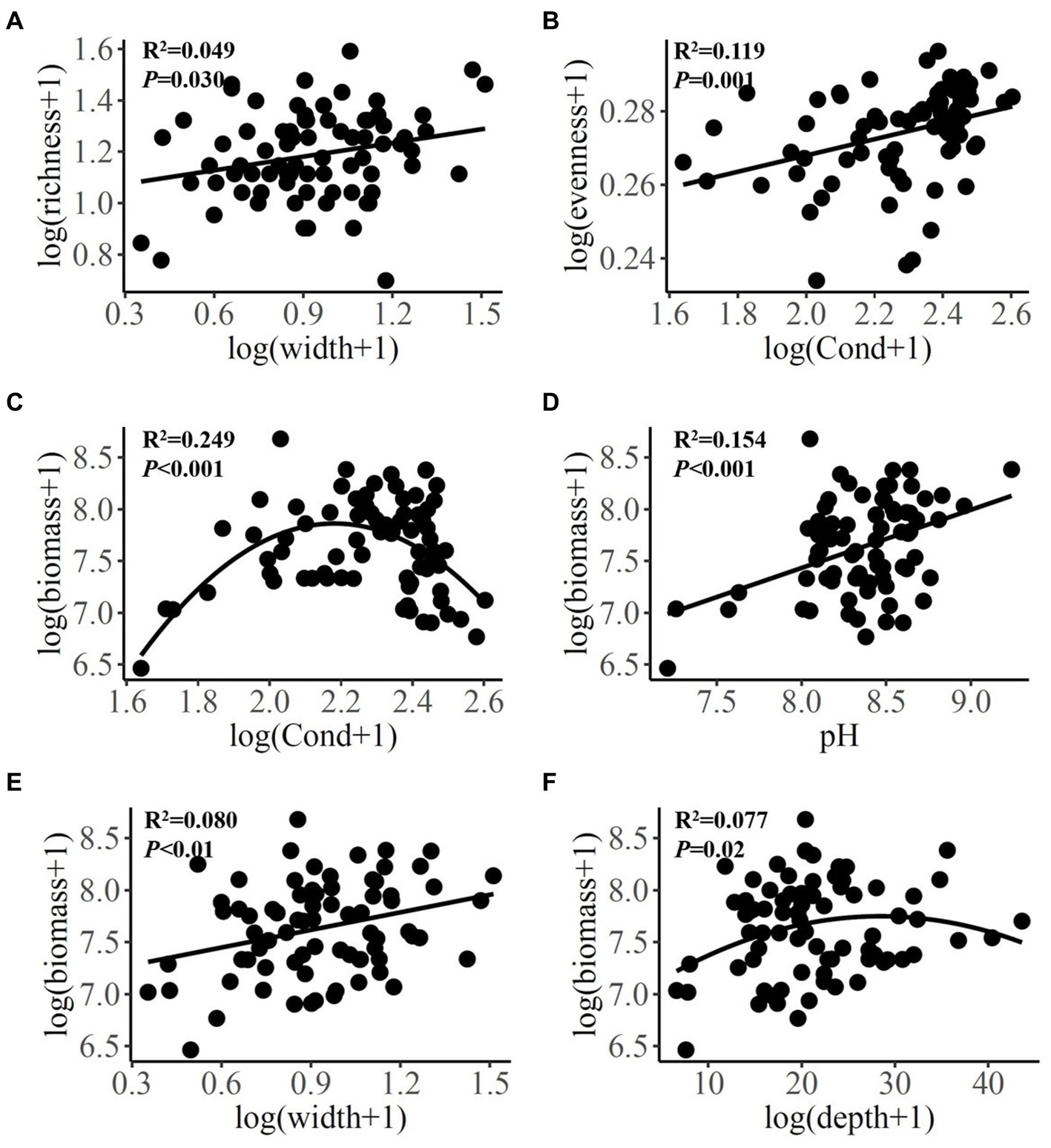
Figure 2. Bivariate relationships between environmental variables and diatom species diversity indices and community biomass. Cond, conductivity.
As for diatom, positive linear relationships were observed between species richness and FRic and RaoQ (Figures 3A,B), as well as between species evenness and FEve and RaoQ (Figures 3C,D). Diatom community biomass showed positive linear relationships with species richness, FRic, and RaoQ (Figures 4A,C,D). By comparison, species evenness displayed a negative linear association with community biomass (Figure 4B).
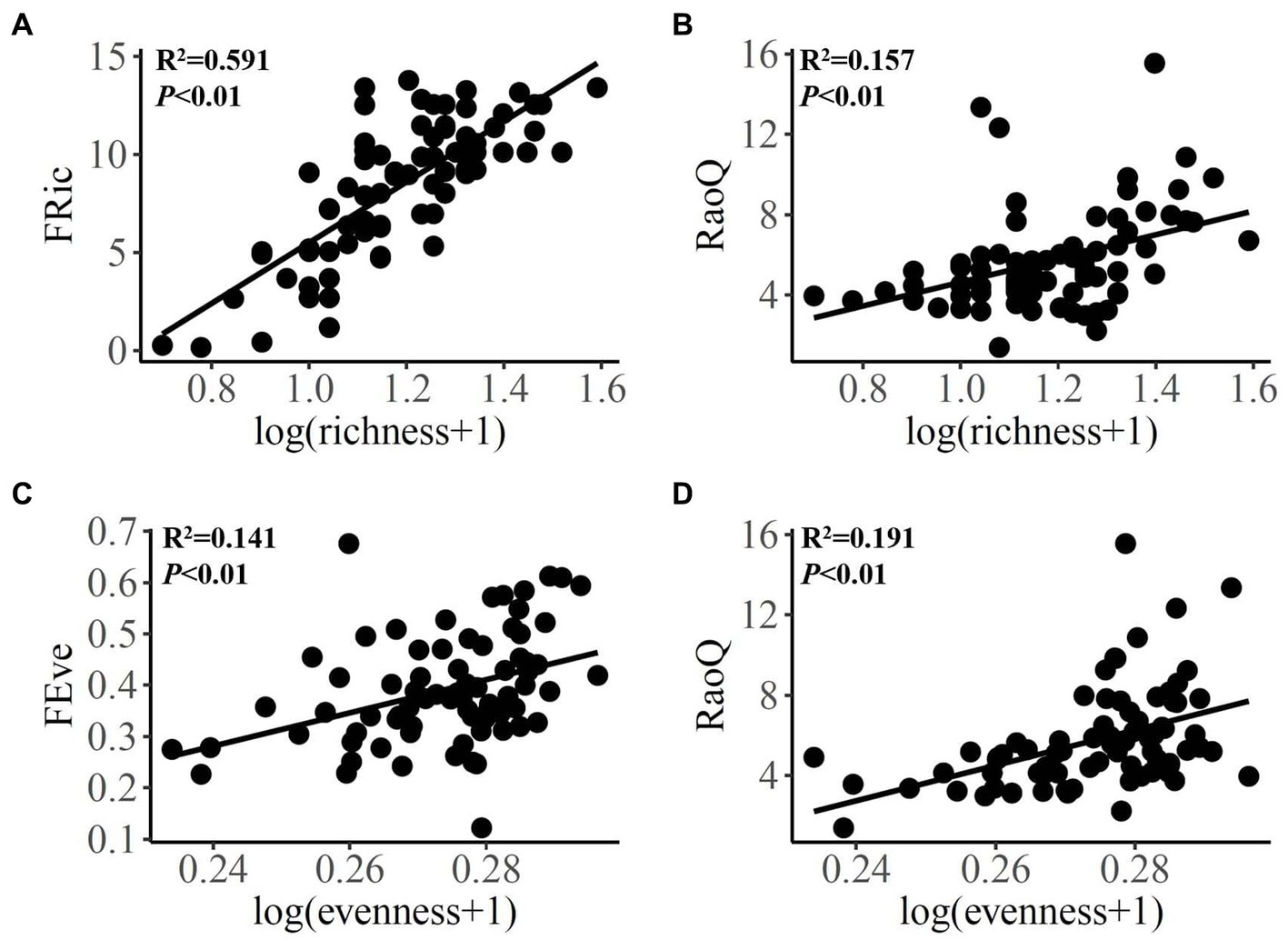
Figure 3. Bivariate relationships between diatom species and functional diversity indices. FRic, functional richness; FEve, functional evenness; RaoQ, Rao’s quadratic entropy index.
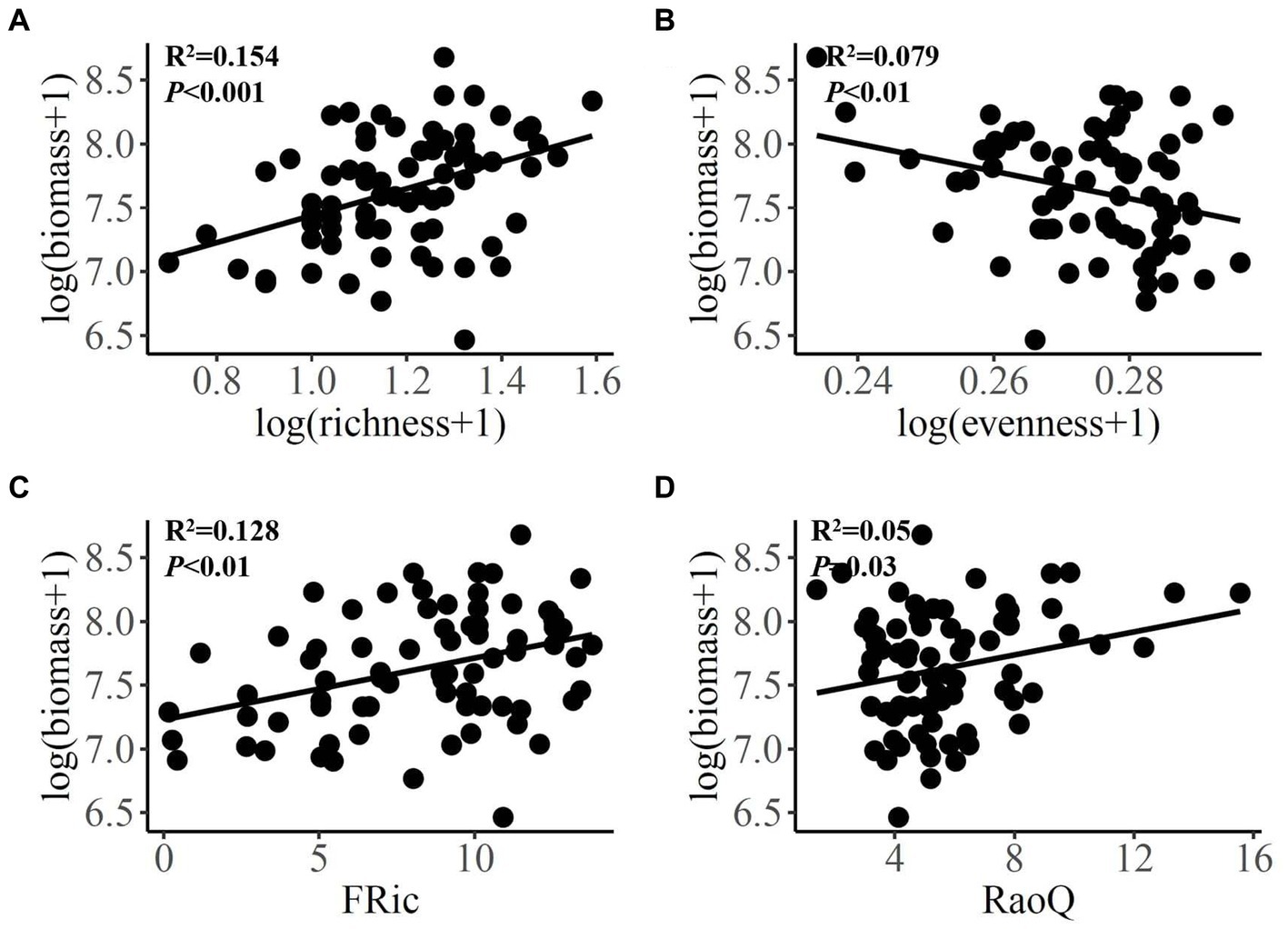
Figure 4. Bivariate relationships between diatom diversity indices and community biomass. FRic, functional richness; RaoQ, Rao’s quadratic entropy index.
3.2. Multiple relationships among environmental variables, diatom diversity, and community biomass
Model 1 confirmed the most important bivariate relationships identified by regression analysis. Wetted width (with a pathway coefficient of 0.28) and conductivity (−0.28) had similarly strong but opposite effects on diatom species richness. Conductivity had similarly positive effects on species evenness and community biomass. By contrast, pH only affected diatom community biomass. Moreover, species richness (0.39) and evenness (−0.28) had significant but opposite effects to community biomass. Besides direct effects, we found that wetted width had an indirect effect, with an indirect pathway coefficient of 0.11, on diatom community biomass via affecting species richness. Furthermore, conductivity not only affected diatom community biomass directly but also indirectly via diatom richness and evenness, while the direct effect (0.31) was stronger than of the indirect effect (0.21) (Figure 5). Overall, model 1 explained 14, 13, and 53% of variance in species richness, evenness, and community biomass, respectively.
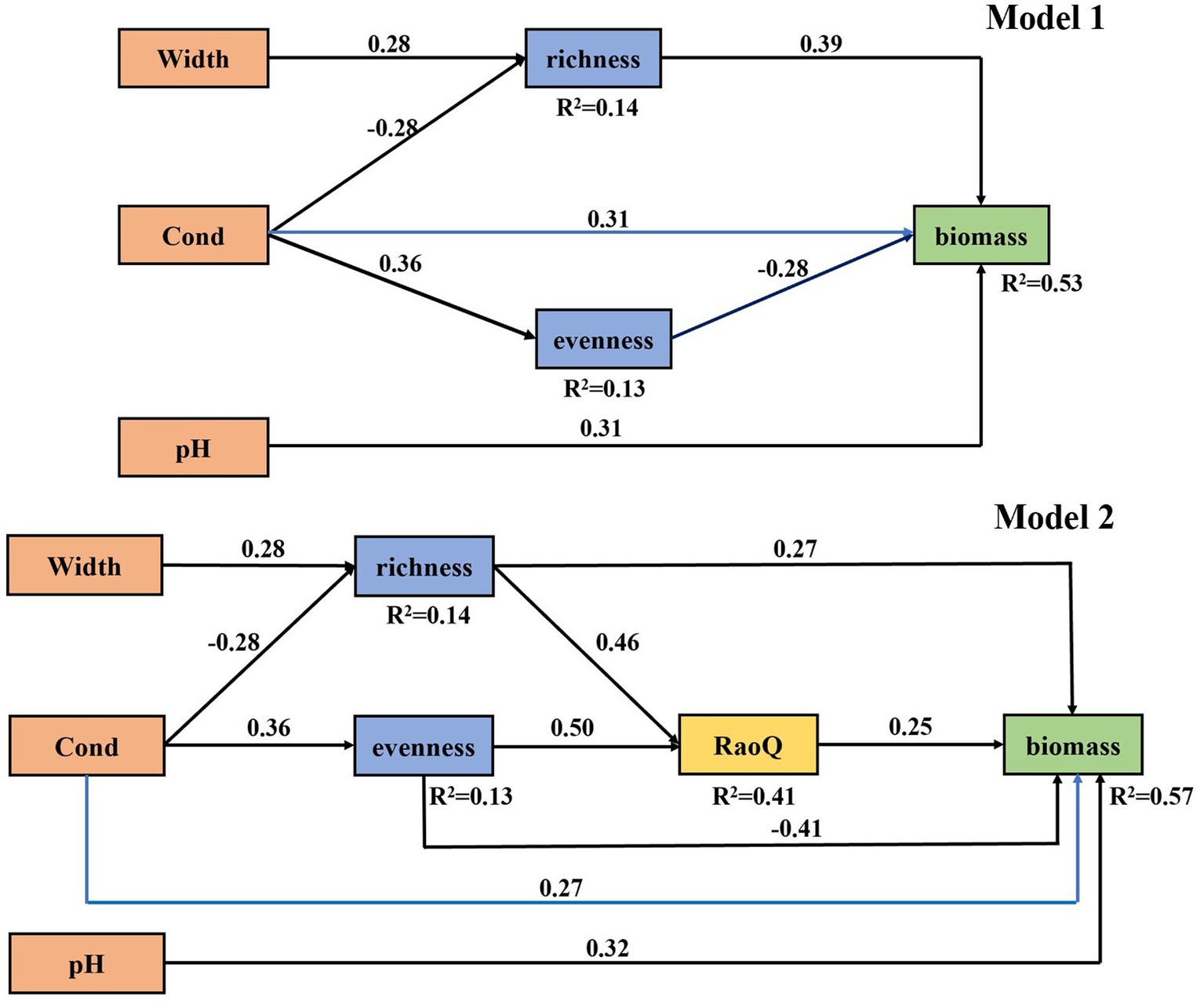
Figure 5. The best-fit structural equation model demonstrating multiple associations among environmental variables, diatom diversity, and community biomass without (Model 1) or with (Model 2) diatom functional diversity included in the model. Standardized coefficients for each pathway (i.e., pathway coefficients) are presented. Black lines represent significant linear relationships (p ≤ 0.05) and blue lines for quadratic relationships. The numbers above the line indicate correlation coefficients associated with each causal relationship. R2 denotes the proportion of variance explained. Model 1: Fisher’ C = 11.10, p = 0.80, AIC = 37.10. Model 2: Fisher’ C = 21.91, p = 0.59, AIC = 57.91. Cond, conductivity; RaoQ, Rao’s quadratic entropy index.
When diatom functional richness, functional evenness and Rao’s quadratic entropy were added to analysis, in addition to the pathways confirmed by Model 1, Model 2 identified indirect pathways that diatom species diversity affected community biomass via functional diversity. Species richness enhanced community biomass not only by a direct effect but also by an indirect effect via RaoQ, in which strength of the direct effect (0.27) was about twice as strong as that of the indirect effect (0.12). Species evenness also affected community biomass through a negative direct effect (−0.41) and a positive indirect (0.13) effect via RaoQ, where the direct effect was three times stronger than the indirect effect (Figure 5). Model 2 totally explained 14, 13, 41, and 57% of variance in species richness, evenness, RaoQ and community biomass, respectively.
4. Discussion
We found that environmental variables including wetted width, conductivity and pH had important effects on benthic diatom diversity and community biomass in the Xiangxi River. Wetted width had positively effects on diatom richness. For streams and rivers, wide wetted width usually means low forest canopy and more available sunlight with more living space, which may allow more algae species to coexist (Whitcraft and Levin, 2007; Bixby et al., 2009; Ishikawa et al., 2012). Conductivity had a negative effect on diatom species richness, while positive effects on species evenness. Conductivity is an effective indicator of human disturbance and increases in conductivity was reported to negatively affect algal richness (Morgan et al., 2012; Simonin et al., 2021). It is likely that only few species with high relative abundance in an algal community could survive under high conductivity conditions, resulting in increased evenness. A quadratic relationship between conductivity and diatom biomass implies that low conductivity may promote diatom biomass, while high conductivity is detrimental to algal assemblages. Such responses were also demonstrated in a control experiment (James et al., 2009). pH had a positive effect on diatom biomass in our study. pH affects in-stream chemical and biological processes, such as CO2 dissolution, nutrient availability, adsorption of metal ions by algae, etc. (Devlin and Witham, 1986; Kuo et al., 2016). An increase in pH means an increase in the dissolved CO2 content in the water body, that is, an increase in raw materials available for diatom photosynthesis, leading to an increase in algal biomass (Kuo et al., 2016).
Diatom community biomass was found to increase with species richness while decreased with species evenness in the present study. Our findings indicate that diatom species richness enhanced community biomass, which was also extensively observed in other studies (Gamfeldt et al., 2013; Duffy et al., 2016; van der Plas, 2019). One possible explanation is that more diatom species in a community can use resources more thoroughly, resulting in more community biomass production. This positive richness-biomass relationship could be partly driven by the complementarity effect. Meanwhile, our finding of the negative evenness-biomass relationship suggests that the selection effect was also an important mechanism for the diatom diversity and biomass relationship in our study. This finding is coincident with the fact that the three dominant species with relative abundance >5% accounted for 63.3% of the total relative abundance of the benthic diatom communities but contributed 72.4% of community biomass in the Xiangxi River. Similar results were also reported in other studies focusing on algal assemblages (Daam et al., 2019; Passy and Larson, 2019). The results of the different effects of species richness and evenness on biomass, and the absence of interaction pathway between the two species diversity indices in SEMs, indicated that species richness and evenness affected biomass independently and both two indices played important roles in BEF relationships of benthic diatom communities.
Diatom functional richness, evenness, and RaoQ were found to be positively associated with species richness or evenness, and species diversity affected community biomass through RaoQ. These results indicate that the benthic diatom species and functional diversity were significantly coupled, and functional diversity played an important role in the diatom diversity-biomass relationship. In addition, we found that the positive effects of dominant species on biomass were more likely to come from species with larger cells. For example, the Cocconeis placentula with very large category cell size contributed 69.16% of diatom biomass while occupied 33.75% of total relative abundance of benthic diatom assemblages, while the Achnanthidium minutissimuma with cell size of Nano contributed 2.93% of biomass but occupied 23.95% of relative abundance. That is, the community biomass can increase with the abundance of species with lager cell size. Positive correlations between diatom species and function diversity implies that the number of functional traits carried by diatom species increased with species richness and there was no apparent functional redundancy in benthic diatom communities in the Xiangxi River. Increased in functional diversity implies a wider variety of traits in the community (Petchey and Gaston, 2002), inducing a more thoroughly utilization of resources thus leading to a greater community biomass (Petchey et al., 2004). In addition, communities composed of species with more diverse characteristics may be better adapted to the environments than those with functionally specialized (Hong et al., 2022). In our study, Rao’s quadratic entropy, an index containing information on both relative abundance of functional groups and differences among groups (Botta-Dukat, 2005), was more integrative than functional richness and evenness in linking species diversity and community biomass. Communities with more traits carried by more species generally have higher Rao’s quadratic entropy, especially when species distributed evenly. High Rao’s quadratic entropy not only represents more traits, but also represents high niche complementarity of coexist species and low competition in communities, which leads to an efficient use of resources and better adaptation to diverse environments, and eventually results in high community biomass (Rao, 1982; Otto et al., 2012; Hao et al., 2020; Yue et al., 2022).
We have attempted to explain the complex relationships among the environmental variables, diversity indices and community biomass of lotic diatom assemblages in a subtropical mountainous watershed, and explored the mechanisms driving of these relationships. The study can fill the relative gap of BEF researches in natural mountainous rivers. Also, different roles of diversity indices in BEF studies are highlighted by revealing different pathways and strengths of the effects of environmental variables, species diversity and functional diversity on diatoms biomass. The results provide insights to help the conservation and management of river ecosystems. However, there are still several limitations to our study. The first limitation of our study is that our data were taken based on only one filed survey, making it difficult to detect the impact of the climate changes on the biodiversity and ecosystem functions of primary producers of rivers. Secondly, although the negative effect of conductivity on species richness in the results can indicate the threat of human disturbances to river biodiversity to some extent, it is still difficult to reflect the state of lotic benthic diatom communities under human disturbances because our study area is less disturbed by human activities. Climate changes and human disturbances are two major threats to almost all natural environments especially to freshwater ecosystems, and how natural communities respond to these threats and maintain ecosystem functioning remains one of the topics worth exploring in the future (Dudgeon et al., 2006; Strayer and Dudgeon, 2010).
5. Conclusion
In conclusion, our analysis has shed light on the species diversity-functional diversity-biomass relationships in natural lotic benthic diatom assemblages, as well as the driving mechanisms behind these relationships. We confirmed that pH and conductivity are the most crucial environmental variables influencing benthic diatom assemblages. Our finding indicate that species richness has a positive effect on community biomass, while species evenness has a negative effect on community biomass, suggesting the presence of selection effect. Furthermore, besides direct effects, species diversity also indirectly affected community biomass through functional diversity. Therefore, inclusion of more diversity indices to explore the biodiversity-biomass relationship can contribute to a deeper and more complete understanding of the relationship and its mechanisms. In the face of climate change and increasing human disturbance in nowadays, a better understanding of the mechanisms by which the environmental conditions affect biodiversity and community biomass will help define better river regulation measures. In the future, it would be of interest to identify environmental conditions under which the complementarity or the selection effect dominates the diatom diversity-biomass relationship. Further explorations on couplings between diatom functional traits and community biomass would also be valuable in revealing the mechanisms of diatom functional diversity affecting community biomass. Such studies may also aid protection practices for sustaining diatom diversity and their ecosystem functions in natural streams and rivers.
Data availability statement
The original contributions presented in the study are included in the article/Supplementary material, further inquiries can be directed to the corresponding author.
Author contributions
CL was responsible for the data analysis and wrote the first draft of the manuscript. TS contributed to the diatom specimen identification. XW provided the article polish at the final stage. LT completed the chemical analysis of water samples. QC and TT provided the initial idea of the study. TT was responsible for writing—review and modification. All authors agreed to be accountable for the content of the work.
Funding
This study was funded by the National Natural Science Foundation of China (32071589).
Acknowledgments
We thank Henglun Shen, Changqun Zhang, Meiqin Sun, and Bin Li for their help in the field and laboratory work.
Conflict of interest
TS is employed by Wuhan Sinoeco Ecological Science & Technology Co., Ltd.
The remaining authors declare that the research was conducted in the absence of any commercial or financial relationships that could be constructed as a conflicts of interest.
Publisher’s note
All claims expressed in this article are solely those of the authors and do not necessarily represent those of their affiliated organizations, or those of the publisher, the editors and the reviewers. Any product that may be evaluated in this article, or claim that may be made by its manufacturer, is not guaranteed or endorsed by the publisher.
Supplementary material
The Supplementary material for this article can be found online at: https://www.frontiersin.org/articles/10.3389/fevo.2023.1150001/full#supplementary-material
References
Ali, A., Lohbeck, M., and Yan, E.-R. (2018). Forest strata-dependent functional evenness explains whole-community aboveground biomass through opposing mechanisms. For. Ecol. Manag. 424, 439–447. doi: 10.1016/j.foreco.2018.05.015
Asparouhov, T., and Muthen, B. (2009). Exploratory structural equation modeling. Struct. Equ. Modeling 16, 397–438. doi: 10.1080/10705510903008204
Baert, J. M., De Laender, F., Sabbe, K., and Janssen, C. R. (2016). Biodiversity increases functional and compositional resistance, but decreases resilience in phytoplankton communities. Ecology 97, 3433–3440. doi: 10.1002/ecy.1601
Barry, K. E., van Ruijven, J., Mommer, L., Bai, Y., Beierkuhnlein, C., Buchmann, N., et al. (2020). Limited evidence for spatial resource partitioning across temperate grassland biodiversity experiments. Ecology 101:e02905. doi: 10.1002/ecy.2905
Berthon, V., Bouchez, A., and Rimet, F. (2011). Using diatom life-forms and ecological guilds to assess organic pollution and trophic level in rivers: a case study of rivers in South-Eastern France. Hydrobiologia 673, 259–271. doi: 10.1007/s10750-011-0786-1
Bixby, R. J., Benstead, J. P., Douglas, M. M., and Pringle, C. M. (2009). Relationships of stream algal community structure to catchment deforestation in eastern Madagascar. J. North. Am. Benthol. Soc. 28, 466–479. doi: 10.1899/07-171.1
Botta-Dukat, Z. (2005). Rao's quadratic entropy as a measure of functional diversity based on multiple traits. J. Veg. Sci. 16, 533–540. doi: 10.1111/j.1654-1103.2005.tb02393.x
Burliga, A. L., and Kociolek, J. P. (2016). “Diatoms (Bacillariophyta) in rivers” in River algae. ed. O. Necchi Jr. (Springer Cham: Cham), 93–128.
Cadotte, M. W., Carscadden, K., and Mirotchnick, N. (2011). Beyond species: functional diversity and the maintenance of ecological processes and services. J. Appl. Ecol. 48, 1079–1087. doi: 10.1111/j.1365-2664.2011.02048.x
Cardinale, B. J., Duffy, J. E., Gonzalez, A., Hooper, D. U., Perrings, C., Venail, P., et al. (2012). Biodiversity loss and its impact on humanity. Nature 486, 59–67. doi: 10.1038/nature11148
Chao, A., Chiu, C.-H., and Jost, L. (2014). Unifying species diversity, phylogenetic diversity, functional diversity, and related similarity and differentiation measures through hill numbers. Annu. Rev. Ecol. Evol. Syst. 45, 297–324. doi: 10.1146/annurev-ecolsys-120213-091540
Chapin, F. S., Zavaleta, E. S., Eviner, V. T., Naylor, R. L., Vitousek, P. M., Reynolds, H. L., et al. (2000). Consequences of changing biodiversity. Nature 405, 234–242. doi: 10.1038/35012241
Chettri, B., and Acharya, B. K. (2020). Distribution of amphibians along an elevation gradient in the Eastern Himalaya, India. Basic Appl. Ecol. 47, 57–70. doi: 10.1016/j.baae.2020.07.001
Cibils, L., Principe, R., Marquez, J., Gari, N., and Albarino, R. (2015). Functional diversity of algal communities from headwater grassland streams: how does it change following afforestation? Aquat. Ecol. 49, 453–466. doi: 10.1007/s10452-015-9538-z
Daam, M. A., Teixeira, H., Lillebo, A. I., and Nogueira, A. J. A. (2019). Establishing causal links between aquatic biodiversity and ecosystem functioning: status and research needs. Sci. Total Environ. 656, 1145–1156. doi: 10.1016/j.scitotenv.2018.11.413
Dangles, O., Crespo-Perez, V., Andino, P., Espinosa, R., Calvez, R., and Jacobsen, D. (2011). Predicting richness effects on ecosystem function in natural communities: insights from high-elevation streams. Ecology 92, 733–743. doi: 10.1890/10-0329.1
Ding, N., Yang, W., Zhou, Y., Gonzalez-Bergonzoni, I., Zhang, J., Chen, K., et al. (2017). Different responses of functional traits and diversity of stream macroinvertebrates to environmental and spatial factors in the Xishuangbanna watershed of the upper Mekong River BASIN, China. Sci. Total Environ. 574, 288–299. doi: 10.1016/j.scitotenv.2016.09.053
Dudgeon, D., Arthington, A. H., Gessner, M. O., Kawabata, Z.-I., Knowler, D. J., Leveque, C., et al. (2006). Freshwater biodiversity: importance, threats, status and conservation challenges. Biol. Rev. 81, 163–182. doi: 10.1017/s1464793105006950
Duffy, J. E., Godwin, C. M., and Cardinale, B. J. (2017). Biodiversity effects in the wild are common and as strong as key drivers of productivity. Nature 549, 261–264. doi: 10.1038/nature23886
Duffy, J. E., Lefcheck, J. S., Stuart-Smith, R. D., Navarrete, S. A., and Edgar, G. J. (2016). Biodiversity enhances reef fish biomass and resistance to climate change. PNAS 113, 6230–6235. doi: 10.1073/pnas.1524465113
Finkel, Z. V., Vaillancourt, C. J., Irwin, A. J., Reavie, E. D., and Smol, J. P. (2009). Environmental control of diatom community size structure varies across aquatic ecosystems. Proc. Royal Soc. B 276, 1627–1634. doi: 10.1098/rspb.2008.1610
Gamfeldt, L., Snall, T., Bagchi, R., Jonsson, M., Gustafsson, L., Kjellander, P., et al. (2013). Higher levels of multiple ecosystem services are found in forests with more tree species. Nat. Commun. 4:1340. doi: 10.1038/ncomms2328
Garnier, E., Navas, M. L., Austin, M. P., Lilley, J. M., and Gifford, R. M. (1997). A problem for biodiversity-productivity studies: how to compare the productivity of multispecific plant mixtures to that of monocultures? Acta Oecol 18, 657–670. doi: 10.1016/s1146-609x(97)80049-5
Geist, J. (2011). Integrative freshwater ecology and biodiversity conservation. Ecol. Indic. 11, 1507–1516. doi: 10.1016/j.ecolind.2011.04.002
Grace, J. B., Adler, P. B., Harpole, W. S., Borer, E. T., and Seabloom, E. W. (2014). Causal networks clarify productivity-richness interrelations, bivariate plots do not. Funct. Ecol. 28, 787–798. doi: 10.1111/1365-2435.12269
Grace, J. B., and Bollen, K. A. (2008). Representing general theoretical concepts in structural equation models: the role of composite variables. Environ. Ecol. Stat. 15, 191–213. doi: 10.1007/s10651-007-0047-7
Grace, J.B., and Grace, J.B. (2006). Structural equation modeling and natural systems. Cambridge: Cambridge University Press.
Hao, M., Messier, C., Geng, Y., Zhang, C., Zhao, X., and von Gadow, K. (2020). Functional traits influence biomass and productivity through multiple mechanisms in a temperate secondary forest. Eur. J. For. Res. 139, 959–968. doi: 10.1007/s10342-020-01298-0
Hillebrand, H., Bennett, D. M., and Cadotte, M. W. (2008). Consequences of dominance: a review of evenness effects on local and regional ecosystem processes. Ecology 89, 1510–1520. doi: 10.1890/07-1053.1
Hillebrand, H., Durselen, C. D., Kirschtel, D., Pollingher, U., and Zohary, T. (1999). Biovolume calculation for pelagic and benthic microalgae. J. Phycol. 35, 403–424. doi: 10.1046/j.1529-8817.1999.3520403.x
Hong, P., Schmid, B., De Laender, F., Eisenhauer, N., Zhang, X., Chen, H., et al. (2022). Biodiversity promotes ecosystem functioning despite environmental change. Ecol. Lett. 25, 555–569. doi: 10.1111/ele.13936
Hooper, D. U., Chapin, F. S., Ewel, J. J., Hector, A., Inchausti, P., Lavorel, S., et al. (2005). Effects of biodiversity on ecosystem functioning: a consensus of current knowledge. Ecol. Monogr. 75, 3–35. doi: 10.1890/04-0922
Hu, H.J., and Wei, Y.X. (2006). The freshwater algae of China-systematics, taxonomy and ecology. Beijing: Science Press.
Isbell, F. I., Polley, H. W., and Wilsey, B. J. (2009). Biodiversity, productivity and the temporal stability of productivity: patterns and processes. Ecol. Lett. 12, 443–451. doi: 10.1111/j.1461-0248.2009.01299.x
Ishikawa, N. F., Doi, H., and Finlay, J. C. (2012). Global meta-analysis for controlling factors on carbon stable isotope ratios of lotic periphyton. Oecologia 170, 541–549. doi: 10.1007/s00442-012-2308-x
James, K. R., Hart, B. T., Bailey, P. C. E., and Blinn, D. W. (2009). Impact of secondary salinisation on freshwater ecosystems: effect of experimentally increased salinity on an intermittent floodplain wetland. Mar. Freshw. Res. 60, 246–258. doi: 10.1071/mf08099
Oksanen, J., Blanchet, F. G., Friendly, M., Kindt, R., Legendre, P., McGlinn, D., et al. (2019). vegan: Community Ecology Package. R Package Version 2.5–6.
Jin, J., Wells, S. A., Liu, D., Yang, G., Zhu, S., Ma, J., et al. (2019). Effects of water level fluctuation on thermal stratification in a typical tributary bay of three gorges reservoir, China. PeerJ 7:e6925. doi: 10.7717/peerj.6925
Kenitz, K. M., Orenstein, E. C., Roberts, P. L. D., Franks, P. J. S., Jaffe, J. S., Carter, M. L., et al. (2020). Environmental drivers of population variability in colony-forming marine diatoms. Limnol. Oceanogr. 65, 2515–2528. doi: 10.1002/lno.11468
Kuo, Y.-M., Yu, H.-L., Kuan, W.-H., Kuo, M.-H., and Lin, H.-J. (2016). Factors controlling changes in Epilithic algal biomass in the mountain streams of subtropical Taiwan. PLoS One 11:e0166604. doi: 10.1371/journal.pone.0166604
Laliberté, E., Legendre, P., Shipley, B., and Laliberté, M. (2014). FD: measuring functional diversity from multiple traits, and other tools for functional ecology. R Package version 1.0-12.1.
Lange, K., Townsend, C. R., and Matthaei, C. D. (2016). A trait-based framework for stream algal communities. Ecol. Evol. 6, 23–36. doi: 10.1002/ece3.1822
Lange-Bertalot, H., and Krammer, K. (2000). Diatoms of Europe. Liechtenstein: ARG Gantner Verlag KG.
Lefcheck, J. S. (2016). PIECEWISESEM: piecewise structural equation modelling in R for ecology, evolution, and systematics. Methods Ecol. Evol. 7, 573–579. doi: 10.1111/2041-210x.12512
Li, F., Cai, Q., Fu, X., and Liu, J. (2009). Construction of habitat suitability models (HSMs) for benthic macroinvertebrate and their applications to instream environmental flows: a case study in Xiangxi River of Three Gorges Reservior region, China. Prog. Nat. Sci. 19, 359–367. doi: 10.1016/j.pnsc.2008.07.011
Li, J., and Qi, Y. (2010). Flora Algarum Sinicarum Aquae Dulcis (Timus XIV): Bacillariophyta, Naviculaceae (I). Beijing: Science Press.
Li, J., and Qi, Y. (2018). Flora Algarum Sinicarum Aquae Dulcis (Timus XXIII): Bacillariophyta, Naviculaceae (III). Beijing: Science Press.
Litchman, E. (2007). “Resource competition and the ecological success of phytoplankton” in Evolution of primary producers in the sea. eds. P. G. Falkowski and A. H. Knoll (Amsterdam: Elsevier), 351–375.
Loreau, M., and Hector, A. (2001). Partitioning selection and complementarity in biodiversity experiments. Nature 412, 72–76. doi: 10.1038/35083573
Malhi, Y., Franklin, J., Seddon, N., Solan, M., Turner, M. G., Field, C. B., et al. (2020). Climate change and ecosystems: threats, opportunities and solutions. Philos. Trans. R. Soc. Lond. Ser. B Biol. Sci. 375:20190104. doi: 10.1098/rstb.2019.0104
Mammola, S., Carmona, C. P., Guillerme, T., and Cardoso, P. (2021). Concepts and applications in functional diversity. Funct. Ecol. 35, 1869–1885. doi: 10.1111/1365-2435.13882
Maureaud, A., Hodapp, D., van Denderen, P. D., Hillebrand, H., Gislason, H., Dencker, T. S., et al. (2019). Biodiversity-ecosystem functioning relationships in fish communities: biomass is related to evenness and the environment, not to species richness. Proc. Royal Soc. B 286:20191189. doi: 10.1098/rspb.2019.1189
MEE (2003). Technical specifications requirements for monitoring of surface water and waste water. Beijing: China Environmental Science Press.
Morgan, R. P. II, Kline, K. M., Kline, M. J., Cushman, S. F., Sell, M. T., Weitzell, R. E. Jr., et al. (2012). Stream conductivity: relationships to land use, chloride, and fishes in Maryland streams. N. Am. J. Fish Manag. 32, 941–952. doi: 10.1080/02755947.2012.703159
Mulder, C. P. H., Bazeley-White, E., Dimitrakopoulos, P. G., Hector, A., Scherer-Lorenzen, M., and Schmid, B. (2004). Species evenness and productivity in experimental plant communities. Oikos 107, 50–63. doi: 10.1111/j.0030-1299.2004.13110.x
Otto, S., Vasileiadis, V. P., Masin, R., and Zanin, G. (2012). Evaluating weed diversity with indices of varying complexity in North-Eastern Italy. Weed Res. 52, 373–382. doi: 10.1111/j.1365-3180.2012.00921.x
Passy, S. I. (2007). Diatom ecological guilds display distinct and predictable behavior along nutrient and disturbance gradients in running waters. Aquat. Bot. 86, 171–178. doi: 10.1016/j.aquabot.2006.09.018
Passy, S. I., and Larson, C. A. (2019). Niche dimensionality and herbivory control stream algal biomass via shifts in guild composition, richness, and evenness. Ecology 100:e02831. doi: 10.1002/ecy.2831
Petchey, O. L., and Gaston, K. J. (2002). Functional diversity (FD), species richness and community composition. Ecol. Lett. 5, 402–411. doi: 10.1046/j.1461-0248.2002.00339.x
Petchey, O. L., Hector, A., and Gaston, K. J. (2004). How do different measures of functional diversity perform? Ecology 85, 847–857. doi: 10.1890/03-0226
Polley, H. W., Wilsey, B. J., and Derner, J. D. (2003). Do species evenness and plant density influence the magnitude of selection and complementarity effects in annual plant species mixtures? Ecol. Lett. 6, 248–256. doi: 10.1046/j.1461-0248.2003.00422.x
Polley, H. W., Wilsey, B. J., and Tischler, C. R. (2007). Species abundances influence the net biodiversity effect in mixtures of two plant species. Basic Appl. Ecol. 8, 209–218. doi: 10.1016/j.baae.2006.02.006
Racca, J. M. J., Wild, M., Birks, H. J. B., and Prairie, Y. T. (2003). Separating wheat from chaff: diatom taxon selection using an artificial neural network pruning algorithm. J. Paleolimnol. 29, 123–133. doi: 10.1023/a:1022833421687
Rao, C. R. (1982). Diversity and dissimilarity coefficients – a unified approach. Theor. Popul. Biol. 21, 24–43. doi: 10.1016/0040-5809(82)90004-1
Rhodes, C., Bingham, A., Heard, A. M., Hewitt, J., Lynch, J., Waite, R., et al. (2017). Diatoms to human uses: linking nitrogen deposition, aquatic eutrophication, and ecosystem services. Ecosphere 8:e01858. doi: 10.1002/ecs2.1858
Rimet, F., and Bouchez, A. (2012). Life-forms, cell-sizes and ecological guilds of diatoms in European rivers. Knowl. Manag. Aquat. Ecosyst. 406, 1–14. doi: 10.1051/kmae/2012018
Simonin, M., Rocca, J. D., Gerson, J. R., Moore, E., Brooks, A. C., Czaplicki, L., et al. (2021). Consistent declines in aquatic biodiversity across diverse domains of life in rivers impacted by surface coal mining. Ecol. Appl. 31:e02389. doi: 10.1002/eap.2389
Smeti, E., von Schiller, D., Karaouzas, I., Laschou, S., Vardakas, L., Sabater, S., et al. (2019). Multiple stressor effects on biodiversity and ecosystem functioning in a Mediterranean temporary river. Sci. Total Environ. 647, 1179–1187. doi: 10.1016/j.scitotenv.2018.08.105
Song, M.-K., Lin, F.-C., Ward, S. E., and Fine, J. P. (2013). Composite variables when and how. Nurs. Res. 62, 45–49. doi: 10.1097/NNR.0b013e3182741948
Sonkoly, J., Kelemen, A., Valko, O., Deak, B., Kiss, R., Toth, K., et al. (2019). Both mass ratio effects and community diversity drive biomass production in a grassland experiment. Sci. Rep. 9:1848. doi: 10.1038/s41598-018-37190-6
Stenger-Kovacs, C., Lengyel, E., Crossetti, L. O., Uveges, V., and Padisak, J. (2013). Diatom ecological guilds as indicators of temporally changing stressors and disturbances in the small Torna-stream, Hungary. Ecol. Indic. 24, 138–147. doi: 10.1016/j.ecolind.2012.06.003
Stevenson, R.J. (1996). An introduction to algal ecology in freshwater benthic habitats. Salt Lake City: Academic Press.
Strayer, D. L., and Dudgeon, D. (2010). Freshwater biodiversity conservation: recent progress and future challenges. J. North. Am. Benthol. Soc. 29, 344–358. doi: 10.1899/08-171.1
Tang, T., Cai, Q. H., and Liu, J. K. (2006). Using epilithic diatom communities to assess ecological condition of Xiangxi River system. Environ. Monit. Assess. 112, 347–361. doi: 10.1007/s10661-006-7666-6
Tang, T., Tang, T., Tan, L., Gu, Y., Jiang, W., and Cai, Q. (2017). Identifying community thresholds for lotic benthic diatoms in response to human disturbance. Sci. Rep. 7:4134. doi: 10.1038/s41598-017-04445-7
Tilman, D., Isbell, F., and Cowles, J. M. (2014). “Biodiversity and ecosystem functioning” in Annual review of ecology, evolution, and systematics. ed. D. J. Futuyma , vol. 45 (Palo Alto, CA: Annual Reviews), 471–493.
Tilman, D., Lehman, C. L., and Thomson, K. T. (1997). Plant diversity and ecosystem productivity: theoretical considerations. PNAS 94, 1857–1861. doi: 10.1073/pnas.94.5.1857
van der Plas, F. (2019). Biodiversity and ecosystem functioning in naturally assembled communities. Biol. Rev. 94, 1220–1245. doi: 10.1111/brv.12499
Vanelslander, B., De Wever, A., Van Oostende, N., Kaewnuratchadasorn, P., Vanormelingen, P., Hendrickx, F., et al. (2009). Complementarity effects drive positive diversity effects on biomass production in experimental benthic diatom biofilms. J. Ecol. 97, 1075–1082. doi: 10.1111/j.1365-2745.2009.01535.x
Villeger, S., Mason, N. W. H., and Mouillot, D. (2008). New multidimensional functional diversity indices for a multifaceted framework in functional ecology. Ecology 89, 2290–2301. doi: 10.1890/07-1206.1
Wang, L., Cai, Q., Xu, Y., Kong, L., Tan, L., and Zhang, M. (2011). Weekly dynamics of phytoplankton functional groups under high water level fluctuations in a subtropical reservoir-bay. Aquat. Ecol. 45, 197–212. doi: 10.1007/s10452-010-9346-4
Wang, X., Cai, Q., Ye, L., and Qu, X. (2012). Evaluation of spatial and temporal variation in stream water quality by multivariate statistical techniques: a case study of the Xiangxi River basin, China. Quat. Int. 282, 137–144. doi: 10.1016/j.quaint.2012.05.015
Whitcraft, C. R., and Levin, L. A. (2007). Regulation of benthic algal and animal communities by salt marsh plants: impact of shading. Ecology 88, 904–917. doi: 10.1890/05-2074
Yue, Q., Hao, M., Geng, Y., Wang, X., von Gadow, K., Zhang, C., et al. (2022). Evaluating alternative hypotheses behind biodiversity and multifunctionality relationships in the forests of Northeastern China. For. Ecosyst. 9:100027. doi: 10.1016/j.fecs.2022.100027
Keywords: biodiversity-ecosystem functioning, the complementarity effect, the selection effect, structural equation modeling, benthic diatom
Citation: Liu C, Sun T, Wu X, Tan L, Cai Q and Tang T (2023) Disentangling multiple relationships of species diversity, functional diversity, diatom community biomass and environmental variables in a mountainous watershed. Front. Ecol. Evol. 11:1150001. doi: 10.3389/fevo.2023.1150001
Edited by:
Yixin Zhang, Soochow University, ChinaCopyright © 2023 Liu, Sun, Wu, Tan, Cai and Tang. This is an open-access article distributed under the terms of the Creative Commons Attribution License (CC BY). The use, distribution or reproduction in other forums is permitted, provided the original author(s) and the copyright owner(s) are credited and that the original publication in this journal is cited, in accordance with accepted academic practice. No use, distribution or reproduction is permitted which does not comply with these terms.
*Correspondence: Tao Tang, dGFuZ3Rhb0BpaGIuYWMuY24=