- 1State Environmental Protection Key Laboratory of Wetland Ecology and Vegetation Restoration, School of Environment, Northeast Normal University, Changchun, China
- 2Key Laboratory of Wetland Ecology and Environment, Institute of Northeast Geography and Agroecology, Chinese Academy of Sciences, Changchun, China
- 3Institute of Grassland Science, Key Laboratory of Vegetation Ecology of the Ministry of Education, Jilin Songnen Grassland Ecosystem National Observation and Research Station, Northeast Normal University, Changchun, China
- 4Key Laboratory of Vegetation Ecology, Ministry of Education, Northeast Normal University, Changchun, China
- 5Jilin Provincial Key Laboratory of Animal Resource Conservation and Utilization, Northeast Normal University, Changchun, China
Introduction: Soil extracellular enzymes are central in terrestrial ecosystem responses to climate change, and their research can be crucial for assessing microbial nutrient demand. However, the effects of climate-induced precipitation patterns on soil microbial nutrient demand in different soil profiles of agroecosystems are rarely studied.
Methods: Here, we present how the precipitation gradient affects soil enzymes related to carbon (C), nitrogen (N) and phosphorus (P) cycling and identified microbial nutrient limitation determinants at five depth intervals (0–10, 10–20, 20–30, 30–40, and 40–50 cm) in seven agroecosystems.
Results and Discussion: We found that N- and P-acquiring enzymes have a tendency to decrease or increase, respectively, but C-acquiring enzymes did not change along the precipitation gradient throughout soil profiles. Soil pH and moisture were the most important factors affecting the enzyme activity in 0–50 cm. Our results also revealed a crucial soil boundary (at 20 cm) that differentiated responses of microbial nutrient limitation to precipitation changes. In the topsoil (0–20 cm), the stoichiometry of soil nutrients did not vary with precipitation. Microbial P limitation was exacerbated with increased precipitation, which was controlled by soil pH and moisture in the topsoil. In contrast, in the subsoil (20–50 cm), soil nutrient stoichiometry decreased with increasing precipitation, and microbial C and P limitation displayed a positive correlation with precipitation. Furthermore, microbial P limitation tended to be stronger in the subsoil than in the topsoil along the precipitation gradient. Microbial C and P limitation was regulated by the soil nutrients and their stoichiometry in the subsoil. Our study is an essential step in soil enzyme activity and stoichiometry response to precipitation in agroecosystems and provides novel insights into understanding microbial nutrient limitation mechanisms in soil profiles along the precipitation gradient.
1. Introduction
Changes in precipitation can lead to shifts in belowground ecological processes that are interlinked with primary production, damaging regional crop production in agroecosystems (Lesk et al., 2016; Franco et al., 2019; Sünnemann et al., 2021). Under rapid precipitation changes, carbon (C), nitrogen (N), and phosphorus (P) cycles may become uncoupled because of the different degrees of control exerted on the supply of these elements by biological and geochemical processes (Delgado-Baquerizo et al., 2013). To meet the food demand, farmers are continuously raising N and P inputs, altering C availability, and leading to a further imbalance in the stoichiometry of microbial substrates in soils (Peñuelas et al., 2013; Schleuss et al., 2021). A combination of precipitation and agricultural activities more drastically alters the patterns, magnitude, and extent of nutrient limitation in soil (Marklein and Houlton, 2012). Such limitation is directly relevant to the fate of soil microbial processes (Camenzind et al., 2018), but the extent and nature of microbial nutrient limitation has never been systematically assessed in agroecosystems along a precipitation gradient. Thus, the underlying ecological mechanisms governing microbial nutrient limitation in agroecosystems are required to support future predictions of precipitation changes.
Ecological stoichiometry and ionomics provide a research framework to facilitate improved mitigation and forecasting of the effects of changing precipitation patterns (Graham and Hofmockel, 2022). Ionomics may link ecological phenomena to the nutritional needs of organisms and responses to environmental stressors (Kaspari, 2021). Ecological stoichiometry takes into account the balance between the nutrient demand of organisms and the nutrient supply in their habitats, their interactions with abiotic and biotic factors and nutrient cycling of ecosystems (Filipiak and Filipiak, 2022). Nutrient availability, especially N and P, impacts microbial respiration and growth because microorganisms need to maintain a homeostasis composition of C and nutrients (Manzoni et al., 2012). Ecological stoichiometry could mediate the function of brown food webs and microbial nutrient metabolism by reallocating resources to extracellular enzymes (Zhang et al., 2019).
Extracellular enzyme activities (EEA) play a central role in regulating imbalances between resources and growth demands in microbe self-organizational processes (Sinsabaugh and Follstad Shah, 2011; Sinsabaugh et al., 2015). Extracellular enzymes are mediators of decomposition and mineralization of N and P (Schimel and Weintraub, 2003; Moorhead and Sinsabaugh, 2006), and are also indicators of microbial nutrient demand (Moorhead et al., 2016). According to resource allocation theory, when a resource is scarce, microorganisms preferentially invest metabolic resources into extracellular enzyme production to maximize the use of the limited element (Allison and Vitousek, 2005; Johnson et al., 2010; Mooshammer et al., 2014). Due to the context dependence of enzyme activity, EEA has gradually received greater attention and become a popular method for examining the direct effects of precipitation on the functional potential of the soil microbial community in field experiments (Weedon et al., 2011; Henry, 2013).
The theory of enzyme stoichiometry further reflects the balance between microbial nutrient demand and soil nutrient resource supplies, highlighting the limiting factors of biogeochemical cycling in terrestrial ecosystems (Sinsabaugh et al., 2009; Zechmeister-Boltenstern et al., 2015; Xu et al., 2017). Sinsabaugh et al. (2008) developed an approach to visualize the relative C, N, and P controls on soil microbial communities by plotting the ratios of activities for enzymes associated with C, N and P acquisition. Moorhead et al. (2016) proposed that vector lengths and angles can be used to improve the quantification and visualization of these controls. A global meta-analysis exhibited that enzymatic C:P and N:P ratios were inversely related to precipitation, while the ratio of C:N acquisition increased with precipitation (Sinsabaugh et al., 2008). In forest ecosystems, the C:N, C:P, and N:P acquisition ratios were negatively related to precipitation from a depth of 0–10 cm soil (Xu et al., 2017). However, Cui et al. (2019a) found a shift from P to N limitation of microorganisms with an increase in precipitation from 300 mm to 900 mm in grassland ecosystems from the 0–20 cm soil layer. Therefore, generalizing the effects of precipitation on soil EEA can be challenging and elusive, and it is necessary to strengthen our research on EEA patterns at regional scales. To the best of our knowledge, there remains a lack of studies on the impact of precipitation gradients on soil enzyme in agroecosystems, especially in deeper soil profiles.
The enzyme distribution in the soil matrix reflects the dynamic interactions among the soil structure, environmental conditions, and microbial nutrient limitation (Allison et al., 2007). Microbial nutrient limitation has been studied in soil profiles (Brockett et al., 2012; Peng and Wang, 2016; Jing et al., 2017; Zuo et al., 2018). Changes in abiotic environments due to long-term climate drivers, such as alterations in soil moisture (Morugán-Coronado et al., 2019), soil pH (Rousk et al., 2010; Kivlin and Treseder, 2014), soil C:N ratio (Zhou et al., 2020) and soil type (Wakelin et al., 2008), can affect microbial nutrient limitation in complex and unpredictable ways (Burns et al., 2013; Li et al., 2018; Xu S. et al., 2020). These changes often occur in strong gradients with depth (Stone et al., 2014; Jia et al., 2019; Dove et al., 2020). Especially in agroecosystems, cropland soils have separate soil layers due to different intensities of human disturbance: topsoil (0–20 cm) and subsoil (below 20 cm; Yan et al., 2017). It is therefore not surprising that most soil properties (e.g., moisture and nutrients) and microbial communities generally vary with soil depth (Kanal and Kõlli, 1996; Li et al., 2014). More importantly, emerging evidence shows that subsoil may exhibit a stronger response to climate change in microbial activities and functioning (Fontaine et al., 2007) in association with microbial nutrient limitation. However, it remains unclear whether the relationships between microbial nutrient limitation and precipitation in topsoil and subsoil differ at the regional scale.
The framework of ecological stoichiometry provides a convenient and ready-to-use tool to better understand the ecological processes linking organisms to the environment, including linking soil enzyme activity to soil physical and chemical properties (Graham and Hofmockel, 2022). Identifying the mechanisms that govern these divergent responses of microbial nutrient limitation to precipitation in soil profiles is critical to minimize uncertainty in future soil health and function. In this study, we focused on soil enzyme activity in five soil layers of seven maize fields along a steep precipitation gradient. We examined the effect of precipitation on microbial nutrient limitation and its drivers in agroecosystem soil profiles. We developed the following hypotheses: (1) microbial nutrient limitation may exhibit more significant responses to precipitation in the subsoil than in the topsoil; and (2) the driving factors of microbial nutrient limitation change with an increase in soil depth. This study determines the microbial nutrient limitation patterns of semi-arid to humid regions and identifies drivers of variations in these patterns across soil profiles in agroecosystems.
2. Materials and methods
2.1. Study site and experiment design
The study was conducted in seven agroecosystems in the Northeast China Transect (NECT), a climatic zone with significant precipitation gradients and a critical component of the International Geosphere-Biosphere Program terrestrial transects (Zhang et al., 1997; Ni and Zhang, 2000). At latitudes ranging from 42° N to 44° N and longitudes from 123° E to 128° E within the NECT, precipitation and aridity demonstrate steep gradients (Ni and Zhang, 2000). Meteorological data that included monthly observations of the precipitation and temperature from 7 meteorological stations in Jilin Province were collected from the National Meteorological Information Center1 for the period from 1971 to 2017. The NECT spans a wide large range of precipitation gradients from west to east, with mean annual precipitation (MAP) increasing from 300 mm to 900 mm, which MAP is the main driver of this transect. And the mean annual temperature (MAT) has slight difference among sites shown in Supplementary Table S1. We standardized our site selection by focusing on maize planting areas. These agricultural sites, which have existed for over 50 years, are located in flat areas and are managed conventionally under the same government and according to the same policies. Conventional tillage included fall plowing, spring cultivation, planting. The depth of agricultural tillage (commonly referred to as plow sole) is about 20 cm (Yan et al., 2017). Fertilizer for maize was applied with 200 kg N ha−1, 70 kg P ha−1 and 90 kg K ha−1 as the base fertilizer at planting. The weeds were controlled using broad-spectrum herbicides and supplemented as required with manual hoeing (Guo et al., 2020). The soil types are classified as salt-alkali, light chernozem, chernozem, black soil, and dark brown soil, based on the Chinese soil classification system (Xiong and Li, 1987). The salt-alkali and light chernozem profile is composed of a humus layer and a compact gray-white calcium carbonate deposition layer. The organic matter content of the surface layer is 1.5 to 4.5%. There are local alkalinization and salinization, and the soil is weakly alkaline. or alkaline. The cross-section structure of chernozem has obvious humus accumulation and calcification process, the organic matter content is high (surface content is 3.5 to 12%), and the soil is neutral. The topsoil of dark brown soil and black soil is rich in humus (5 to 10%, even up to 17%), and the profile is slightly acidic (Ni et al., 1999). The basic properties of the study sites are listed in Supplementary Table S1.
2.2. Soil sampling and characterization
The seven sites, Changling (CL), Sijianfang (SJF), Nongan (NOA), Changchun (CC), Dongliao (DL), Longwan (LW) and Baihe (BH), are positioned along a precipitation gradient. Three plots (approximately 50 × 50 m) situated 50–100 m from each other were randomly selected at each site. After removing the litter and rocks, the soil was sampled by horizon to a maximum depth of 50 cm. Samples were taken discretely at five depth intervals (0–10 cm, 10–20 cm, 20–30 cm, 30–40 cm and 40–50 cm). Three cores of soil, each with a diameter of 5.5 cm, were randomly collected and gently mixed to form one composite sample for each layer of each plot. In total, 105 soil samples (7 sites × 5 layers × 3 plots) were collected prior to maize harvest in September 2018 to minimize the effects of agricultural management. And all sampling was done within 2 days of sunny weather to ensure consistent weather and temperature.
Each sample was separated into two portions. The first fresh soil samples were immediately placed inside coolers containing ice packets for transport, returned to the laboratory, and stored at 4°C. Soil moisture (SM) was determined by oven-drying technique using 10 g of fresh soil samples dried at 105°C for 48 h to a constant weight. The amount of water in the sample can be determined and the moisture content calculated and expressed as a percentage of the dry soil weight (Schmugge et al., 1980). The second portion was air-dried for the analysis of edaphic properties. The soil pH was measured in a soil-water suspension (1:5 soil to water ratio) using a glass electrode with a pH meter (Thermo Fisher Scientific Inc., United States). Soil total carbon (TC) and total nitrogen (TN) concentrations were determined by combustion 25.000 mg of soil subsamples through a 0.149 mm mesh at 1100°C using an automatic elemental analyzer (Flash EA 1112 elemental, Italy) (Zhang et al., 2022). Total phosphorus (TP) concentration of soil was measured by first digesting 0.5 g soil sample with H2SO4-HClO4, making up 100 ml of the digested solution, and then the TP concentration was measured using a continuous flow analyzer (SKALAR SAN++, Netherlands; Wang et al., 2022).
2.3. Soil enzyme activity
The potential activities of five hydrolytic enzymes, including two C-acquiring enzymes, β-glucosidase (BG) and β-1,4-cellobiosidase (CB), two N-acquiring enzymes, β-N-acetylglucosaminidase (NAG) and Leucine aminopeptidase (LAP), one P-acquiring enzyme, phosphatase (AP), were measured at a temperature of 25°C based on the methods described by Saiya-Cork et al. (2002) and German et al. (2011). The types, functions and substrates of soil hydrolases are detailed in Table 1. The mass of 1.5 g of fresh soil was accurately recorded and 125 ml of 50 mM acetate buffer was added. We used glacial acetic acid to adjust acetate buffer pH to the pH of this study site. The samples were homogenized by blending on highest speed for 2 min to make a slurry with a magnetic stirrer. Blank wells received 250 μl acetate buffer. Standard wells received 50 μl of standard substrate and 200 μl acetate buffer. Substrate wells received 50 μl of 200 μM enzyme substrate solution in 200 μl of acetate buffer. Sample wells received 50 μl of acetate buffer and 200 μl of soil sample suspension. Quench Control wells received 50 μl of the standard substrate (10 μM 4-methylumvelliferyl or 7-amino-4-methylcoumarin) plus 200 μl of soil sample suspension. Assay wells received 50 μl of 200 μM enzyme substrate solution and 200 μl of soil sample suspension. The pre-experimental results exhibited that the activity was the highest in the dark after 4 h of incubation at 25°C. Fluorescence plates were read at 365 nm excitation and 450 nm emission using a microplate reader (BioTek Synergy HTX, Winooski, Vermont, United States). Eight analytical replicates were conducted per sample. Enzymatic activity was enumerated as nanomoles of substrate released per gram of dry soil and incubation time (nmol h−1 g−1).

Table 1. Names, Abbr. (abbreviations), functions, substrate and groups of soil enzymes that were measured in the current study.
2.4. Quantification of microbial nutrient limitation
The soil nutrient ratios (C:N, C:P and N:P ratios) were expressed by soil elements concentration ratio. The stoichiometry of soil enzymes, such as enzyme C:N ratio, enzyme C:P ratio and enzyme N:P ratio were expressed as ln BG: ln (NAG + LAP), ln BG: ln AP and ln (NAG + LAP): ln AP, respectively. Resource limitation was measured by vector analysis of enzymatic stoichiometry (length and angle) (Moorhead et al., 2013) according to the relative activities of enzymes that acquire C, N and P. The rationale for using the relative activities of C-, N-, and P-acquiring enzymes to interpret the relative limitation of C, N, and P for microorganisms is based on ecosystem stoichiometry and metabolic theory (Allison et al., 2010). Relatively longer vector lengths express a greater C limitation, while vector angles <45° and > 45° indicate the relative degrees of N and P limitation, respectively (Moorhead et al., 2016). The vector length (unitless) and angle (°) are calculated as follows:
2.5. Statistical analysis
The Shapiro–Wilk normality test was performed using the R version 3.5.3 ‘stats’ package (R Development Core Team, 2019). We examined the relationships between precipitation and different soil properties, enzyme activities, and C:N, C:P, and N:P ratios using linear regressions. C-acquiring enzymes were calculated as BG + CB, N-acquiring enzymes were calculated as NAG + LAP, and P-acquiring enzyme was represented by AP. Redundancy analysis (RDA) was performed to analyze the relationships between soil enzyme activities and selected soil properties for 105 samples by the packages “vegan” (Oksanen et al., 2019), “adespatial” (Dray et al., 2018). We used the variation inflation factor (VIF) (“vif.cca” function) to assess multicollinearity among soil properties (VIF < 10). The significance of RDA correlations was tested Monte Carlo permutation test.
Similarly, we explored the relationships between precipitation and vector length and angle for each soil profile. The analysis of covariance (ANCOVA) was used to detect the differences in the slope of regression lines between the angle and length of vector and precipitation among soil profiles by SPSS software version 26.0 (IBM Corporation, Armonk, New York, United Stated). The angle and length of vector were dependent variables, precipitation was covariates, and soil profiles were a grouping factor with five levels. A significant soil profiles × covariate interaction would indicate that the slope of the precipitation–vector relationship differs among soil profiles. When the interaction was significant, the homogeneity of slopes assumption of ANCOVA was rejected (Poorter et al., 2008). Differences at the p < 0.05 level were considered statistically significant. To determine the relative influences of environmental variables on the ecoenzymatic vectors in the five soil layers, we used the rdacca.hp. function to calculate the independent adjusted R-squared (R2) and the percentage of each explanatory variable in the RDA, running 999 permutations in the ‘rdacca.hp’ R package (Lai et al., 2022). This function performs variation partitioning and hierarchical partitioning to calculate the unique, shared, and independent contributions of each predictor to the explained variation (R2 and adjusted R2) in canonical analysis (RDA) by applying the hierarchy algorithm of Chevan and Sutherland (1991).
3. Results
3.1. Soil properties and nutrient stoichiometry
The physicochemical properties and soil nutrient ratios displayed various patterns in topsoil (0–20 cm) and subsoil (20–50 cm) along the precipitation gradient (Figure 1). The soil pH decreased significantly from 9.36 to 4.46 with increasing precipitation. In contrast, soil moisture and nutrients (TC, TN, and TP) were positively correlated with precipitation, but this strong correlation was not observed in the subsoil for TC. Interestingly, for these components of soil nutrient stoichiometry components (TC:TN, TC:TP, and TN:TP), significant positive relationships with precipitation were observed in the subsoil but not in the topsoil. The soil properties and the variation of soil properties with soil depth at seven sites were shown in Table 2 and Supplementary Figure S1, respectively.
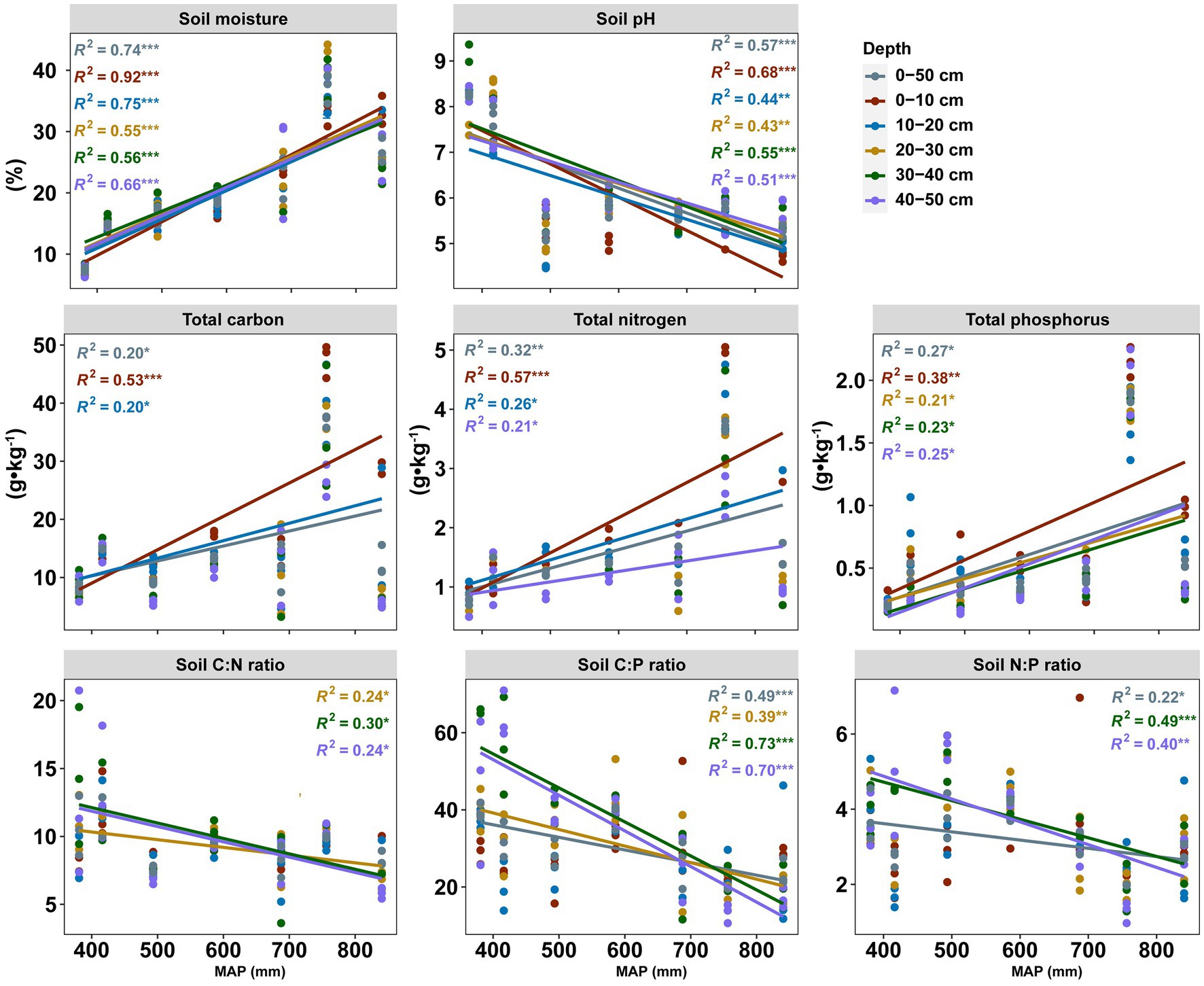
Figure 1. Linear regression between soil properties and MAP for each soil layer. Colored lines represent the regression of each soil layer (n = 105). Statistics (R2 and p values) for the regression are indicated using the corresponding colored text: *** p < 0.001, **p < 0.01, and *p < 0.05. Only significant differences are shown.
3.2. Soil enzyme activity and stoichiometry across precipitation gradient
The soil enzyme patterns were dissimilar to soil nutrient patterns along the precipitation gradient (Figure 2). C-acquiring enzyme activities were unaffected by precipitation. The N-acquiring enzyme activities decreased across the precipitation gradient in all soil profiles, while P-acquiring enzyme activities were positively correlated with precipitation at a depth of 0–30 cm but not at that of 30–50 cm. The C:P enzyme stoichiometry pattern was the opposite of that of the P-acquiring enzyme. With increasing precipitation, the enzymatic C:N and N:P ratios demonstrated increasing and decreasing trends, respectively; however, both stoichiometries responded to precipitation more drastically in the subsoil than in the topsoil. The variation of soil enzyme activities and stoichiometry with soil depth at seven sites was shown in Supplementary Figure S2. The major environmental variables shaping the soil enzyme pattern were determined through RDA (Figure 3). According to the RDA results, approximately 33.58% of the variance in total soil enzyme activity can be explained by environmental variables. The first two RDA axes explained 30.04% of the relationships between enzymes and the environment (24.79 and 5.25% for the first and second axes, respectively). According to the model selection results, soil pH and moisture were the most important factors affecting the enzyme activity.
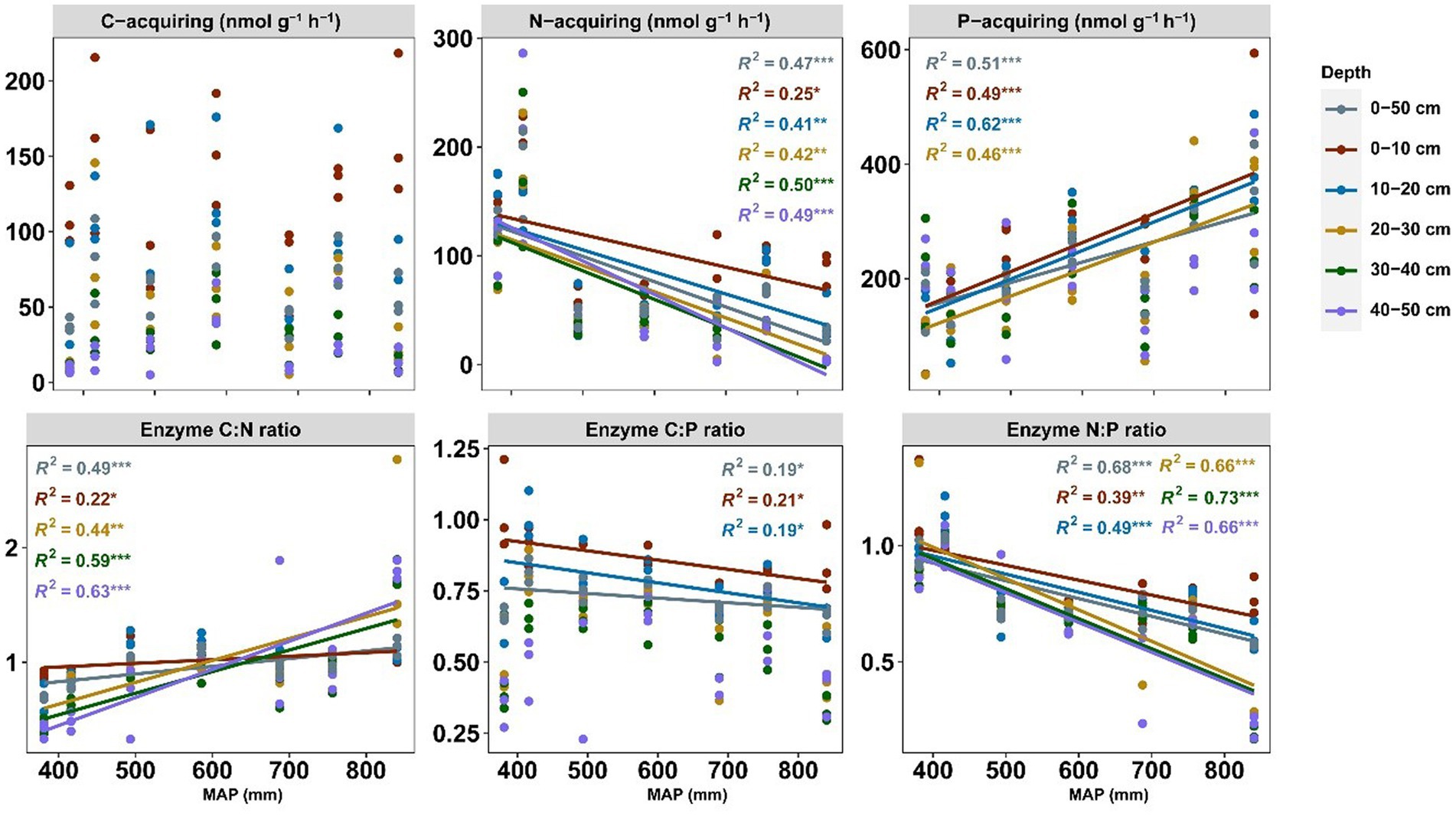
Figure 2. Patterns of enzyme activity and stoichiometry along the precipitation gradient for each soil layer. Colored lines represent the regression of each soil layer (n = 105). Statistics (R2 and p values) for the regression are indicated using the corresponding colored text: *** p < 0.001, **p < 0.01, and *p < 0.05. Only significant differences are shown. C, N, and P represent carbon, nitrogen, and phosphorus.
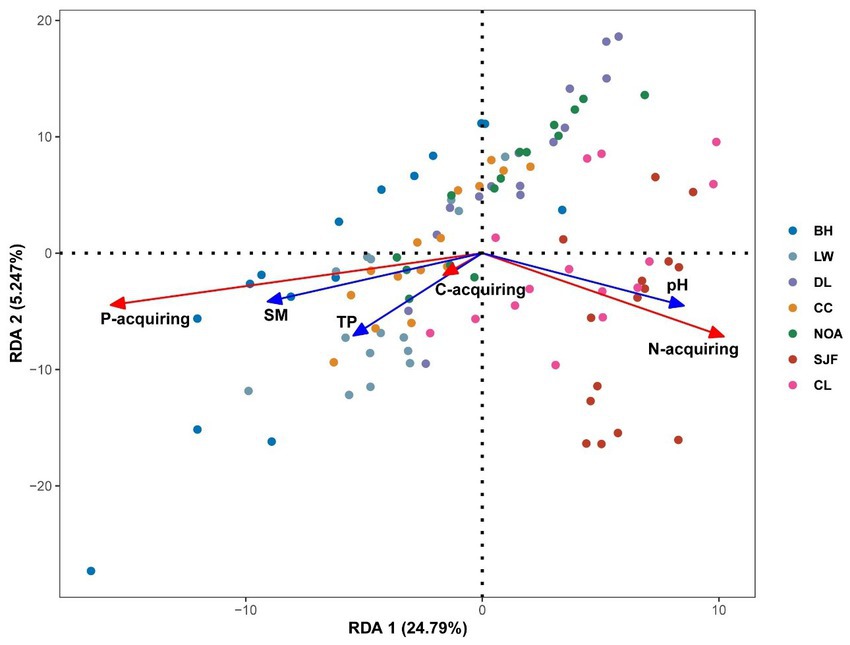
Figure 3. Redundancy analysis of 105 soil samples based on soil enzyme activity and dominant environmental factor ordination biplot. The overall model is significant (p < 0.001). Environmental factors: SM, soil moisture; pH, soil acidity and alkalinity; TP, total phosphorus. Site: BH, Baihe; LW, Longwan; DL, Dongliao; CC, Changchun; NOA, Nongan; SJF, Sijianfang; CL, Changling.
3.3. Soil microbe metabolic limitation and its driving factors
The nutrient limitation of soil microbes was calculated using enzyme stoichiometry and quantified by vector angle and length (Figure 4). Vector angles ranging from 36.1° to 80.5° were positively related to the precipitation, and they displayed a more intense correlation with precipitation in the subsoil than in the topsoil (Figure 4A; Supplementary Table S2). We also subdivided the driving factors for nutrient limitation according to the soil layer (Figure 5). We observed that pH had the largest independent effects on vector angle in topsoil, explaining 69.41 and 44.78% of the variance in the depth ranges of 0–10 cm and 10–20 cm, respectively (Figure 5A). From the topsoil to the subsoil, the soil physicochemical properties (soil pH and moisture) variance explanation ranged from 85.64 to 16.79% (Figure 5A). In contrast, the interpretations of soil nutrients and their stoichiometry ranged from 14.36 to 83.21% (Figure 5A). Vector length was positively correlated with precipitation only in the subsoil (Figure 4B). The interpretation of soil nutrient stoichiometry with respect to the vector length grew stronger with depth (Figure 5B). Furthermore, it was not found that the temperature would have an effect on our results by redundancy analysis and hierarchical partitioning analysis.
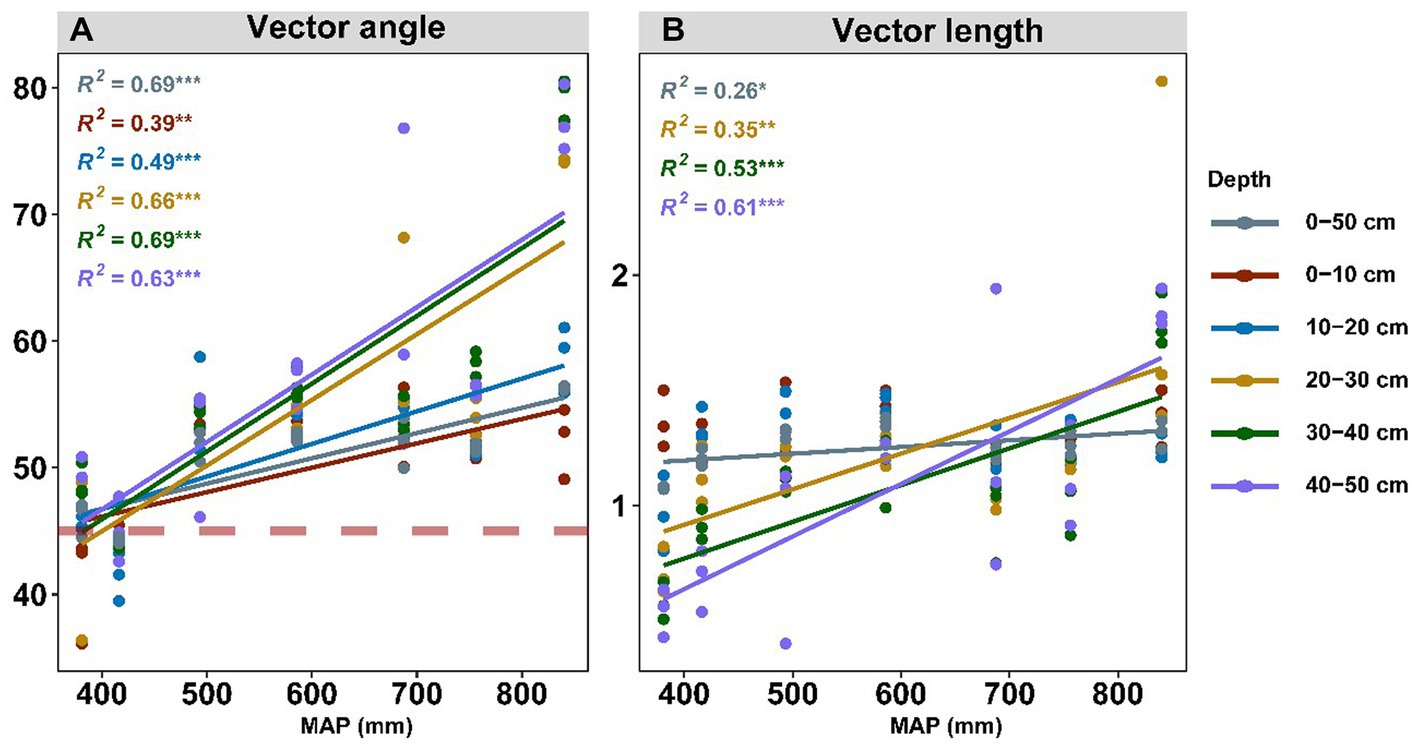
Figure 4. Effects of MAP (mm) on microbial N/P limitation (A) and microbial C limitation (B). Soil microbial N/P limitation is represented by vector angles: >45° represents P limitation, and < 45° represents N limitation. Soil microbial C limitation is represented by vector lengths, where microbial C limitation increases as the values increase. MAP represents mean annual precipitation.
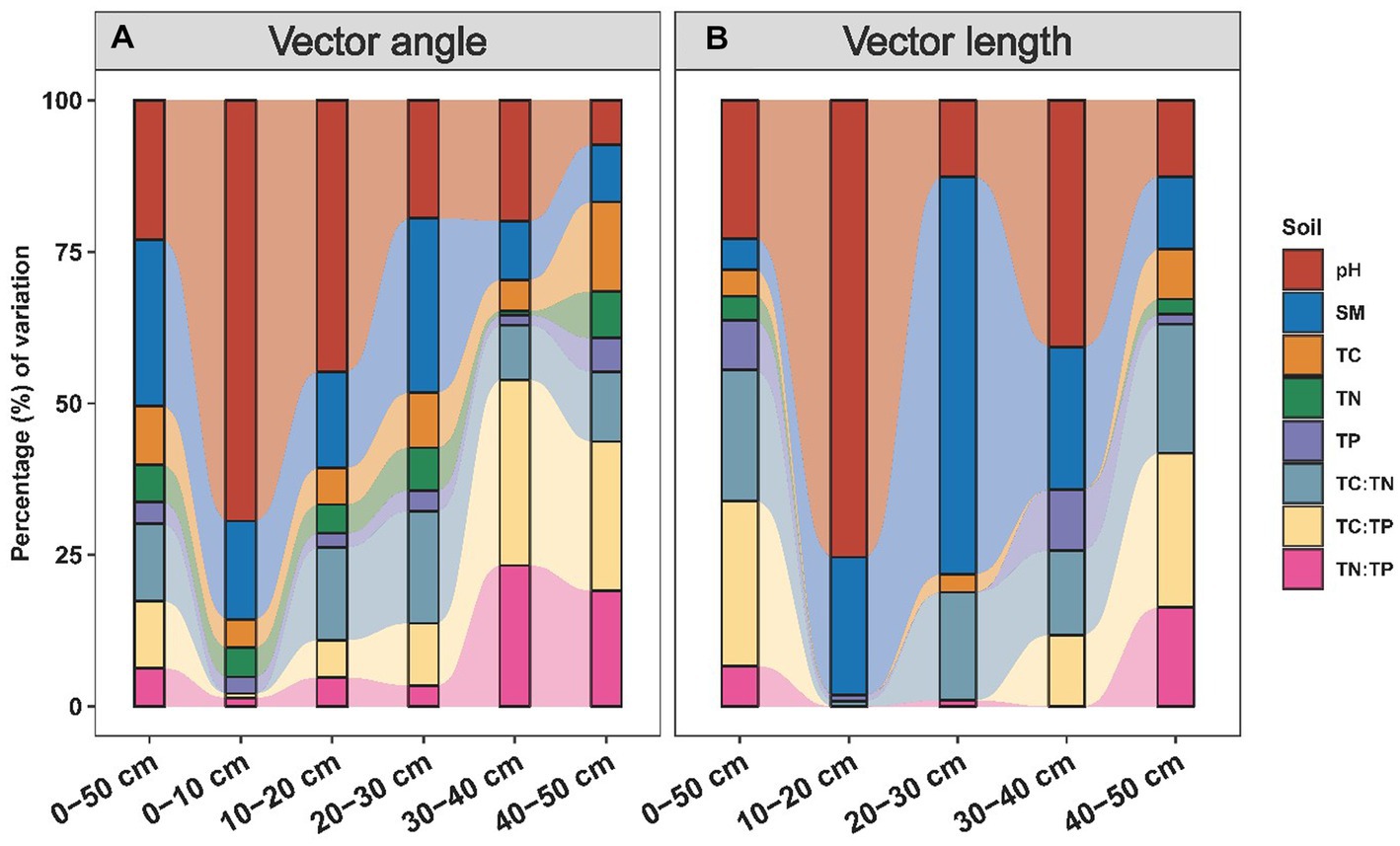
Figure 5. Influences of soil variables on vector angles (A) and lengths (B). Percentage of variation in vector angles and lengths explained by soil variables. SM, soil moisture; pH, soil acidity and alkalinity; TC, total carbon; TN, total nitrogen; TP, total phosphorus.
4. Discussion
4.1. Spatial variations of soil properties along the precipitation gradient
Soil moisture, total carbon, total nitrogen, total phosphorus increased as the MAP increased along the NECT, but the soil pH decreased as the MAP increased. These results are consistent with the results at the regional scale (Wang et al., 2002; Cui et al., 2019a). Due to the steep moisture gradient, the virgin vegetation changes gradually from mixed deciduous broad-leaved forest to meadow steppe along the transect (Prentice et al., 2011). The forest can improve the availability of soil nutrients by nitrogen fixation and phosphorus strategies (Nasto et al., 2014, 2017). In addition, soil properties are related to the interaction of precipitation and soil parent materials (Huston, 2012). The high pH of the soil in west of the transect is due to the fact that the soil is salt-alkali and the evaporation is three to four times higher than the precipitation (Wang and Ba, 2008; Yang et al., 2021). Therefore, less precipitation, high surface evaporation and parent salinity are the major reasons for high soil pH. Understanding soil properties at the regional scale is fundamental to predicting climate changes at the global scale.
4.2. Patterns of microbial nutrient limitation across precipitation regions throughout soil profiles
We did not observe a significant relationship between C-acquiring enzymes and precipitation (Figure 2), and surprisingly, the vector length increased with precipitation, indicating that microbial C limitation was gradually exacerbated along the precipitation gradient in 0–50 cm. Moreover, N-acquiring enzyme activity decreased and P-acquiring enzyme activity increased with increasing precipitation (Figure 2), which alters the stoichiometry of enzymes leading to C limitation. We attributed this pattern of microbial C limitation to changes in soil nutrients and their stoichiometry across precipitation regions (Figure 5B). Microbial resource limitation is relative (Chen et al., 2018); thus, microbial C limitation can depend on the combined effect of other nutrients.
We detected a decrease in the enzymatic C:P and N:P acquisition ratios with increasing precipitation (Figure 2). Microorganisms can shift from secreting more C-acquiring enzymes and N-acquiring enzymes to secreting more P-acquiring enzymes, which implies an increased demand for P. Moreover, the vector angle became greater than 45° with increasing precipitation (Figure 4A). Our results notably indicated that microbial P limitation was gradually exacerbated along the precipitation gradient. A perpendicular projection of P-acquiring enzyme onto the line overlaying the environmental variable arrow revealed that P-acquiring enzyme was influenced by soil pH and moisture (Figure 3). P is predominately derived from mineral aerosol deposition and weathering, and it is highly dependent on pH (Thingstad et al., 2005; Mahowald et al., 2008). High soil moisture and low soil pH increase P-acquiring enzyme activity, which facilitates P solubilization (Collavino et al., 2010; Xu Z. et al., 2020). In addition, soil pH and moisture were the main factor determining the pattern of soil enzymes along the precipitation gradient (Figure 3). Therefore, soil pH and moisture play an essential role in microbial P limitation throughout soil profiles.
Furthermore, soil nutrients and their stoichiometry and soil physicochemical properties are equally important for microbial phosphorus limitation in 0–50 cm (Figure 5A). More C and N might lead to microbial P limitation (Cui et al., 2020). This observation may be explained for two reasons. On the one hand, if the C and N accumulated in the soil reduces the relative concentration of soil P, the microorganisms may be limited by P due to the elemental stoichiometric balance of the microorganisms (Sinsabaugh et al., 2008, 2009; Chen et al., 2017). Thus, despite the increase in soil P, microbial P limitation remains because of the slow growth rate of P relative to that of C and N. On the other hand, phosphatases have relatively high C and N concentrations, and microorganisms require sufficient C and N to construct extracellular phosphatases (Treseder and Vitousek, 2001; Houlton et al., 2008). Phosphatase may be highly responsive to changes in soil elements, a critical strategy for organism adaption to human modifications of the global biogeochemical cycle that can delay the onset of P limitation (Güsewell and Freeman, 2005; Marklein and Houlton, 2012). These findings demonstrate that precipitation regulates soil physicochemical properties and nutrient status (Smith, 2011; Ma et al., 2015), which consequently greatly enhances microbial C and P limitation. Our current results take a new step and are valuable for understanding microbial nutrient limitation along the precipitation gradient in agroecosystems.
4.3. Contrasting mechanisms governing microbial nutrient limitation in topsoil and subsoil
Our findings are in support of our hypothesis that microbial nutrient limitations were stronger in the subsoil than in the topsoil along the precipitation gradient. Also, we show that the driving factors of microbial nutrient limitation are different in topsoil and subsoil. Soil properties and enzyme activities can change with soil profiles (Stone et al., 2014; Li et al., 2020; Luan et al., 2020), and we observed wide differences in soil and enzyme stoichiometry between the topsoil (0–20 cm) and subsoil (20–50 cm). Our study found that enzymatic C:P acquisition ratios were negatively correlated with precipitation only in the topsoil, which is attributable to the phosphatase distribution pattern. Enzymatic N:P acquisition ratios decreased, but enzymatic C:N acquisition ratio increased along the precipitation gradient, and changes substantially in the subsoil. The vector angles visualized that microbial P limitation increased and responded more strongly to precipitation in the subsoil than in the topsoil. In topsoil, soil pH and moisture are the major drivers of microbial P limitation (Figure 5A). Soil pH governs phosphatase spatial variations and is positively correlated with enzymatic C:P and N:P acquisition ratios (Xu et al., 2017). It is interesting to note that, compared to the topsoil, soil nutrient availability and their stoichiometry are important factors in determining microbial P limitation in subsoil (Figure 5A). Soil substrate availability greatly influences enzyme activity patterns (Stock et al., 2019), and low resources (C and N) and O2 concentrations can limit microbial metabolism (Fierer, 2017; Gu et al., 2017). Zhou et al. (2020) also found that the soil C:N ratio explains a large proportion of the variation in microbial nutrient acquisition. Emerging evidence suggests that subsoils, exhibit more varied organic matter sources, microbial communities, and substrate availability compared to those of the topsoil (Rumpel et al., 2012; Chen et al., 2019). Differences in soil properties across soil depths can lead to greater environmental filtering or competition for nutrients among microorganisms than in the topsoil (Bahram et al., 2015; Schlatter et al., 2020). Especially in agroecosystems, this difference between topsoil and subsoil may be attributed to the agricultural tillage depth, which is typically 0–20 cm. Cropland activities contribute to the homogenization of microbial communities (Rodrigues et al., 2013), and alter microbial resource limitations (Cui et al., 2021). On the contrary, the original soil conditions were preserved in the subsoil, resulting in microbes secreting soil enzymes that are less responsive to precipitation in the topsoil than that in the subsoil and attenuate the reaction of microbe nutrients limitation to precipitation in the topsoil.
Ecosystems cannot be limited by carbon or nutrients alone (Soong et al., 2020), and most microbial communities are co-limited by energy and key nutrients (Moorhead et al., 2016). Another marked trend is that the vector length increases in the subsoil with precipitation. This result indicates that microbial C limitation increased in the subsoil along the precipitation gradient. The detected relationships might result from that soil C:N and C:P ratios progressively decline across the precipitation region in the subsoil (Figure 1), which can lead to insufficient soil C (Chen et al., 2018). In contrast, there is no relationship between microbial C limitation and precipitation in topsoil. This might occur because the effect of precipitation is masked by cropland activity, causing soil nutrient stoichiometry in topsoil that exhibited no response to precipitation. Moreover, soil nutrients and their stoichiometry increase in their interpretation of C limitation with depth (Figure 4). Therefore, soil C:N:P stoichiometry tremendously affected microbial groups involved in major biogeochemical processes (Luo et al., 2020). Additionally, balanced nutrient stoichiometry is essential for maintaining microbial and elemental homeostasis (Cui et al., 2019b).
Further, P limitation changes rapidly in the subsoil, which contributes to C limitation. Nutrients are interdependent and they have a complex coupling, so changing in one nutrient cycle type can alter the availability of another nutrient (Marklein and Houlton, 2012). The role of P limitation in the storage capacity of the C ecosystem has previously been substantially underestimated (Peng and Thomas, 2010). P affects the C-storage capacity both directly and indirectly by affecting the plant growth capacity and limiting biological activities for N fixation (Peñuelas et al., 2012). A model predicted less efficient microbial growth in P-limited soils, indicating that P availability may affect C cycling (Waring et al., 2014). These nutrient interdependencies confirm that resource limitation coupling is complex, and excess precipitation leads to depletion of P and C and induces imbalances in agroecosystems. Our results highlight the contrasting mechanisms governing microbial nutrient limitation in topsoil and subsoil. Microbial P limitation was controlled by the soil pH and moisture in the topsoil, while microbial C and P limitation in the subsoil were regulated by the soil nutrients and their stoichiometry (Figure 6). The findings suggest that ecosystem functions and microbial nutrient limitation cannot be comprehensively assessed from topsoil observations alone.
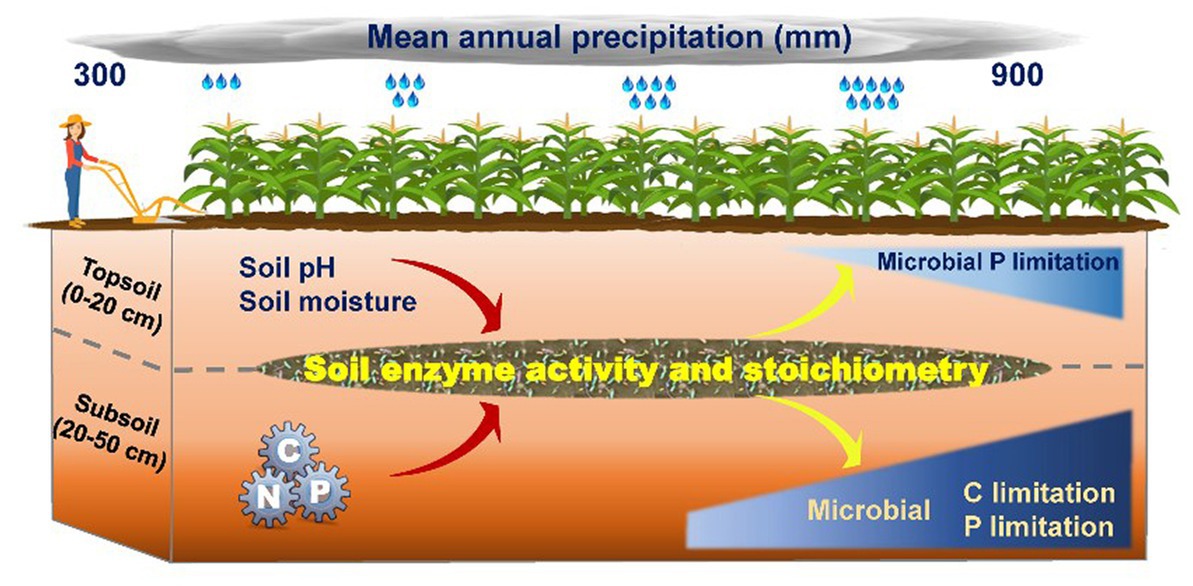
Figure 6. A conceptual framework of soil enzyme activity and stoichiometry along a precipitation gradient (300–900 mm) among soil profiles in agricultural ecosystems. Microbial C limitation, microbial carbon limitation; Microbial P limitation, microbial phosphorus limitation. pH, soil acidity and alkalinity; C, total carbon; N, total nitrogen; P, total phosphorus.
Ecological stoichiometry is commonly applied to explore relationships between brown food webs such as soils and microbes (Bell et al., 2014). When nutrient-limited, microorganisms reduce their uptake of C for nutrients and excrete excess C through enzymes and respiration, so that the ability to assimilate C into biomass tends to decrease (Manzoni et al., 2017). Nutrient-deprived foods are stoichiometrically unbalanced, due to differences in elemental concentrations in food and consumers reflecting stoichiometric mismatches that limit consumer growth and development, thereby affecting the metabolism and function of the brown food web (Filipiak, 2016). Further studies on soil profiles, we found that global change-induced stoichiometric mismatch in agricultural subsoil is more severe. Our results are an important step forward in better understanding soil microbial metabolism across the whole profile of agroecosystems under changing precipitation patterns. Future research could aim to study the metabolic capacity of brown food webs under precipitation changes through metagenomic next-generation sequencing to better understand how precipitation affects the function of whole-profile brown food webs in agroecosystems.
5. Conclusion
We observed an increase in microbial C and P limitation along the precipitation gradient in agroecosystems. Furthermore, our study showed stronger microbial P limitation in the subsoil compared to the topsoil. Given that the high sensitivity of microbial nutrient limitation was observed in the subsoil, our results suggest that the impact of precipitation on microorganisms may be underestimated if only the topsoil is assessed, especially in agroecosystems. Our study also provides insights to elucidate the differentiation in microbial nutrient limitation mechanisms among soil profiles, generating realistic predictions of how agroecosystems will respond to ongoing climate changes. Future research will incorporate deep tillage to maintain microbial nutrient balance in the subsoil to meet production goals and protect vital life-support systems in the context of climate change.
Data availability statement
The raw data supporting the conclusions of this article will be made available by the authors, without undue reservation.
Author contributions
JY: conceptualization, methodology, software, formal analysis, investigation, and writing—original draft. PG: methodology, formal analysis, data curation, and writing—review and editing. PZ: validation, investigation, and resources. YW: investigation, resources, visualization, and writing—review and editing. DeW: conceptualization, supervision, writing—review and editing. DoW: conceptualization, supervision, resources, writing—review and editing, project administration, and funding acquisition.
Funding
This project was supported by the National Science & Technology Fundamental Resources Investigation Program of China (grant no. 2018FY100300) and the National Natural Science Foundation of China (grant no. 41807046).
Acknowledgments
We would like to thank Sina Adl and Lichao Feng for their contributions to the fieldwork, Xuefeng Wu for their invaluable assistance in analyzing the data, and Wei Wang and Cao Hao for their help with proof-reading the article.
Conflict of interest
The authors declare that the research was conducted in the absence of any commercial or financial relationships that could be construed as a potential conflict of interest.
Publisher’s note
All claims expressed in this article are solely those of the authors and do not necessarily represent those of their affiliated organizations, or those of the publisher, the editors and the reviewers. Any product that may be evaluated in this article, or claim that may be made by its manufacturer, is not guaranteed or endorsed by the publisher.
Supplementary material
The Supplementary material for this article can be found online at: https://www.frontiersin.org/articles/10.3389/fevo.2023.1137172/full#supplementary-material
Footnotes
References
Allison, S. D., Gartner, T., Holland, K., Weintraub, M., and Sinsabaugh, R. L. (2007). “Soil enzymes: linking proteomics and ecological process” in Manual of Environmental Microbiology. 3rd edn (Washington, DC: ASM Press), 704–711.
Allison, S. D., and Vitousek, P. M. (2005). Responses of extracellular enzymes to simple and complex nutrient inputs. Soil Biol. Biochem. 37, 937–944. doi: 10.1016/j.soilbio.2004.09.014
Allison, S. D., Wallenstein, M. D., and Bradford, M. A. (2010). Soil-carbon response to warming dependent on microbial physiology. Nat. Geosci. 3, 336–340. doi: 10.1038/ngeo846
Bahram, M., Peay, K. G., and Tedersoo, L. (2015). Local-scale biogeography and spatiotemporal variability in communities of mycorrhizal fungi. New Phytol. 205, 1454–1463. doi: 10.1111/nph.13206
Bell, C., Carrillo, Y., Boot, C. M., Rocca, J. D., Pendall, E., and Wallenstein, M. D. (2014). Rhizosphere stoichiometry: are C: N: P ratios of plants, soils, and enzymes conserved at the plant species-level? New Phytol. 201, 505–517. doi: 10.1111/nph.12531
Brockett, B. F. T., Prescott, C. E., and Grayston, S. J. (2012). Soil moisture is the major factor influencing microbial community structure and enzyme activities across seven biogeoclimatic zones in western Canada. Soil Biol. Biochem. 44, 9–20. doi: 10.1016/j.soilbio.2011.09.003
Burns, R. G., DeForest, J. L., Marxsen, J., Sinsabaugh, R. L., Stromberger, M. E., Wallenstein, M. D., et al. (2013). Soil enzymes in a changing environment: current knowledge and future directions. Soil Biol. Biochem. 58, 216–234. doi: 10.1016/j.soilbio.2012.11.009
Camenzind, T., Hättenschwiler, S., Treseder, K. K., Lehmann, A., and Rillig, M. C. (2018). Nutrient limitation of soil microbial processes in tropical forests. Ecol. Monogr. 88, 4–21. doi: 10.1002/ecm.1279
Chen, H., Li, D., Xiao, K., and Wang, K. (2018). Soil microbial processes and resource limitation in karst and non-karst forests. Funct. Ecol. 32, 1400–1409. doi: 10.1111/1365-2435.13069
Chen, H., Luo, P., Wen, L., Yang, L., Wang, K., and Li, D. (2017). Determinants of soil extracellular enzyme activity in a karst region, Southwest China. Eur. J. Soil Biol. 80, 69–76. doi: 10.1016/j.ejsobi.2017.05.001
Chen, D., Saleem, M., Cheng, J., Mi, J., Chu, P., Tuvshintogtokh, I., et al. (2019). Effects of aridity on soil microbial communities and functions across soil depths on the Mongolian plateau. Funct. Ecol. 33, 1561–1571. doi: 10.1111/1365-2435.13359
Chevan, A., and Sutherland, M. (1991). Hierarchical partitioning. Am. Stat. 45, 90–96. doi: 10.1080/00031305.1991.10475776
Collavino, M. M., Sansberro, P. A., Mroginski, L. A., and Aguilar, O. M. (2010). Comparison of in vitro solubilization activity of diverse phosphate-solubilizing bacteria native to acid soil and their ability to promote Phaseolus vulgaris growth. Biol. Fert. Soils 46, 727–738. doi: 10.1007/s00374-010-0480-x
Cui, Y., Fang, L., Deng, L., Guo, X., Han, F., Ju, W., et al. (2019a). Patterns of soil microbial nutrient limitations and their roles in the variation of soil organic carbon across a precipitation gradient in an arid and semi-arid region. Sci. Total Environ. 658, 1440–1451. doi: 10.1016/j.scitotenv.2018.12.289
Cui, Y., Fang, L., Guo, X., Han, F., Ju, W., Ye, L., et al. (2019b). Natural grassland as the optimal pattern of vegetation restoration in arid and semi-arid regions: evidence from nutrient limitation of soil microbes. Sci. Total Environ. 648, 388–397. doi: 10.1016/j.scitotenv.2018.08.173
Cui, Y., Moorhead, D. L., Guo, X., Peng, S., Wang, Y., Zhang, X., et al. (2021). Stoichiometric models of microbial metabolic limitation in soil systems. Glob. Ecol. Biogeogr. 30, 2297–2311. doi: 10.1111/geb.13378
Cui, Y., Zhang, Y., Duan, C., Wang, X., Zhang, X., Ju, W., et al. (2020). Ecoenzymatic stoichiometry reveals microbial phosphorus limitation decreases the nitrogen cycling potential of soils in semi-arid agricultural ecosystems. Soil Till. Res. 197:104463. doi: 10.1016/j.still.2019.104463
Delgado-Baquerizo, M., Maestre, F. T., Gallardo, A., Bowker, M. A., Wallenstein, M. D., Quero, J. L., et al. (2013). Decoupling of soil nutrient cycles as a function of aridity in global drylands. Nature 502, 672–676. doi: 10.1038/nature12670
Dove, N. C., Arogyaswamy, K., Billings, S. A., Botthoff, J. K., Carey, C. J., Cisco, C., et al. (2020). Continental-scale patterns of extracellular enzyme activity in the subsoil: an overlooked reservoir of microbial activity. Environ. Res. Lett. 15:1040a1041. doi: 10.1088/1748-9326/abb0b3
Dray, S., Blanchet, G., Borcard, D., Guenard, G., Jombart, T., Larocque, G., et al. (2018). Package ‘Adespatial’. R Package 2018, pp. 3–8.
Fierer, N. (2017). Embracing the unknown: disentangling the complexities of the soil microbiome. Nat. Rev. Microbiol. 15, 579–590. doi: 10.1038/nrmicro.2017.87
Filipiak, M. (2016). Pollen stoichiometry may influence detrital terrestrial and aquatic food webs. Front. Ecol. Evol. 4:138. doi: 10.3389/fevo.2016.00138
Filipiak, M., and Filipiak, Z. M. (2022). Application of ionomics and ecological stoichiometry in conservation biology: nutrient demand and supply in a changing environment. Biol. Conserv. 272:109622. doi: 10.1016/j.biocon.2022.109622
Fontaine, S., Barot, S., Barré, P., Bdioui, N., Mary, B., and Rumpel, C. (2007). Stability of organic carbon in deep soil layers controlled by fresh carbon supply. Nature 450, 277–280. doi: 10.1038/nature06275
Franco, A. L. C., Gherardi, L. A., de Tomasel, C. M., Andriuzzi, W. S., Ankrom, K. E., Shaw, E. A., et al. (2019). Drought suppresses soil predators and promotes root herbivores in Mesic, but not in xeric grasslands. Proc. Natl. Acad. Sci. U. S. A. 116, 12883–12888. doi: 10.1073/pnas.1900572116
German, D. P., Weintraub, M. N., Grandy, A. S., Lauber, C. L., Rinkes, Z. L., and Allison, S. D. (2011). Optimization of hydrolytic and oxidative enzyme methods for ecosystem studies. Soil Biol. Biochem. 43, 1387–1397. doi: 10.1016/j.soilbio.2011.03.017
Graham, E. B., and Hofmockel, K. S. (2022). Ecological stoichiometry as a foundation for omics-enabled biogeochemical models of soil organic matter decomposition. Biogeochemistry 157, 31–50. doi: 10.1007/s10533-021-00851-2
Gu, Y., Wang, Y., Lu, S., Xiang, Q., Yu, X., Zhao, K., et al. (2017). Long-term fertilization structures bacterial and archaeal communities along soil depth gradient in a paddy soil. Front. Microbiol. 8:1516. doi: 10.3389/fmicb.2017.01516
Guo, Y., Fan, R., Zhang, X., Zhang, Y., Wu, D., McLaughlin, N., et al. (2020). Tillage-induced effects on SOC through changes in aggregate stability and soil pore structure. Sci. Total Environ. 703:134617. doi: 10.1016/j.scitotenv.2019.134617
Güsewell, S., and Freeman, C. (2005). Nutrient limitation and enzyme activities during litter decomposition of nine wetland species in relation to litter N:P ratios. Funct. Ecol. 19, 582–593. doi: 10.1111/j.1365-2435.2005.01002.x
Henry, H. A. L. (2013). Reprint of “soil extracellular enzyme dynamics in a changing climate”. Soil Biol. Biochem. 56, 53–59. doi: 10.1016/j.soilbio.2012.10.022
Houlton, B. Z., Wang, Y., Vitousek, P. M., and Field, C. B. (2008). A unifying framework for dinitrogen fixation in the terrestrial biosphere. Nature 454, 327–330. doi: 10.1038/nature07028
Huston, M. A. (2012). Precipitation, soils, NPP, and biodiversity: resurrection of Albrecht's curve. Ecol. Monogr. 82, 277–296. doi: 10.1890/11-1927.1
Jia, J., Cao, Z., Liu, C., Zhang, Z., Lin, L., Wang, Y., et al. (2019). Climate warming alters subsoil but not topsoil carbon dynamics in alpine grassland. Glob. Chang. Biol. 25, 4383–4393. doi: 10.1111/gcb.14823
Jing, X., Chen, X., Tang, M., Ding, Z., Jiang, L., Li, P., et al. (2017). Nitrogen deposition has minor effect on soil extracellular enzyme activities in six Chinese forests. Sci. Total Environ. 607-608, 806–815. doi: 10.1016/j.scitotenv.2017.07.060
Johnson, N. C., Wilson, G. W. T., Bowker, M. A., Wilson, J. A., and Miller, R. M. (2010). Resource limitation is a driver of local adaptation in mycorrhizal symbioses. Proc. Natl. Acad. Sci. U. S. A. 107, 2093–2098. doi: 10.1073/pnas.0906710107
Kanal, A., and Kõlli, R. (1996). Influence of cropping on the content, composition and dynamics of organic residue in the soil of the plough layer. Biol. Fert. Soils 23, 153–160. doi: 10.1007/BF00336056
Kaspari, M. (2021). The invisible hand of the periodic table: how micronutrients shape ecology. Annu. Rev. Ecol. Evol. Syst. 52, 199–219. doi: 10.1146/annurev-ecolsys-012021-090118
Kivlin, S. N., and Treseder, K. K. (2014). Soil extracellular enzyme activities correspond with abiotic factors more than fungal community composition. Biogeochemistry 117, 23–37. doi: 10.1007/s10533-013-9852-2
Lai, J., Zou, Y., Zhang, J., and Peres-Neto, P. R. (2022). Generalizing hierarchical and variation partitioning in multiple regression and canonical analyses using the rdacca. Hp R package. Methods Ecol. Evol. 13, 782–788. doi: 10.1111/2041-210X.13800
Lesk, C., Rowhani, P., and Ramankutty, N. (2016). Influence of extreme weather disasters on global crop production. Nature 529, 84–87. doi: 10.1038/nature16467
Li, G., Kim, S., Han, S. H., Chang, H., Du, D., and Son, Y. (2018). Precipitation affects soil microbial and extracellular enzymatic responses to warming. Soil Biol. Biochem. 120, 212–221. doi: 10.1016/j.soilbio.2018.02.014
Li, P., Li, W., Dumbrell, A. J., Liu, M., Li, G., Wu, M., et al. (2020). Spatial variation in soil fungal communities across paddy fields in subtropical China. mSystems 5, e00704–e00719. doi: 10.1128/mSystems.00704-19
Li, C., Yan, K., Tang, L., Jia, Z., and Li, Y. (2014). Change in deep soil microbial communities due to long-term fertilization. Soil Biol. Biochem. 75, 264–272. doi: 10.1016/j.soilbio.2014.04.023
Luan, L., Liang, C., Chen, L., Wang, H., Xu, Q., Jiang, Y., et al. (2020). Coupling bacterial community assembly to microbial metabolism across soil profiles. mSystems 5, e00298–e00220. doi: 10.1128/mSystems.00298-20
Luo, G., Xue, C., Jiang, Q., Xiao, Y., Zhang, F., Guo, S., et al. (2020). Soil carbon, nitrogen, and phosphorus cycling microbial populations and their resistance to global change depend on soil C:N:P stoichiometry. mSystems 5, e00162–e00120. doi: 10.1128/mSystems.00162-20
Ma, R., Cai, C., Li, Z., Wang, J., Xiao, T., Peng, G., et al. (2015). Evaluation of soil aggregate microstructure and stability under wetting and drying cycles in two Ultisols using synchrotron-based X-ray micro-computed tomography. Soil Till. Res. 149, 1–11. doi: 10.1016/j.still.2014.12.016
Mahowald, N., Jickells, T. D., Baker, A. R., Artaxo, P., Benitez-Nelson, C. R., Bergametti, G., et al. (2008). Global distribution of atmospheric phosphorus sources, concentrations and deposition rates, and anthropogenic impacts. Global Biogeochem. Cycles 22:GB4026. doi: 10.1029/2008GB003240
Manzoni, S., Čapek, P., Mooshammer, M., Lindahl, B. D., Richter, A., and Šantrůčková, H. (2017). Optimal metabolic regulation along resource stoichiometry gradients. Ecol. Lett. 20, 1182–1191. doi: 10.1111/ele.12815
Manzoni, S., Taylor, P., Richter, A., Porporato, A., and Ågren, G. I. (2012). Environmental and stoichiometric controls on microbial carbon-use efficiency in soils. New Phytol. 196, 79–91. doi: 10.1111/j.1469-8137.2012.04225.x
Marklein, A. R., and Houlton, B. Z. (2012). Nitrogen inputs accelerate phosphorus cycling rates across a wide variety of terrestrial ecosystems. New Phytol. 193, 696–704. doi: 10.1111/j.1469-8137.2011.03967.x
Moorhead, D., Rinkes, Z., Sinsabaugh, R., and Weintraub, M. (2013). Dynamic relationships between microbial biomass, respiration, inorganic nutrients and enzyme activities: informing enzyme-based decomposition models. Front. Microbiol. 4:223. doi: 10.3389/fmicb.2013.00223
Moorhead, D. L., and Sinsabaugh, R. L. (2006). A theoretical model of litter decay and microbial interaction. Ecol. Monogr. 76, 151–174. doi: 10.1890/0012-9615(2006)076[0151:Atmold]2.0.Co;2
Moorhead, D. L., Sinsabaugh, R. L., Hill, B. H., and Weintraub, M. N. (2016). Vector analysis of ecoenzyme activities reveal constraints on coupled C, N and P dynamics. Soil Biol. Biochem. 93, 1–7. doi: 10.1016/j.soilbio.2015.10.019
Mooshammer, M., Wanek, W., Zechmeister-Boltenstern, S., and Richter, A. (2014). Stoichiometric imbalances between terrestrial decomposer communities and their resources: mechanisms and implications of microbial adaptations to their resources. Front. Microbiol. 5:22. doi: 10.3389/fmicb.2014.00022
Morugán-Coronado, A., García-Orenes, F., McMillan, M., and Pereg, L. (2019). The effect of moisture on soil microbial properties and nitrogen cyclers in Mediterranean sweet orange orchards under organic and inorganic fertilization. Sci. Total Environ. 655, 158–167. doi: 10.1016/j.scitotenv.2018.11.174
Nasto, M. K., Alvarez-Clare, S., Lekberg, Y., Sullivan, B. W., Townsend, A. R., and Cleveland, C. C. (2014). Interactions among nitrogen fixation and soil phosphorus acquisition strategies in lowland tropical rain forests. Ecol. Lett. 17, 1282–1289. doi: 10.1111/ele.12335
Nasto, M. K., Osborne, B. B., Lekberg, Y., Asner, G. P., Balzotti, C. S., Porder, S., et al. (2017). Nutrient acquisition, soil phosphorus partitioning and competition among trees in a lowland tropical rain forest. New Phytol. 214, 1506–1517. doi: 10.1111/nph.14494
Ni, J., Li, Y., and Zhang, X. (1999). The scientific significance of the Northeast China transect (NECT) to global change study by its ecogeographical characteristics. Shengtai Xuebao Acta Ecol. Sin. 19, 622–629.
Ni, J., and Zhang, X. (2000). Climate variability, ecological gradient and the Northeast China transect (NECT). J. Arid Environ. 46, 313–325. doi: 10.1006/jare.2000.0667
Oksanen, J., Blanchet, F. G., Friendly, M., Kind, R., Legendre, P., McGlinn, D. M., et al. (2019). Vegan: Community Ecology Package Version 2.2-0. Available at: http://CRAN.Rproject.org/package=vegan.
Peng, Y., and Thomas, S. C. (2010). Influence of non-nitrogenous soil amendments on soil CO2 efflux and fine root production in an N-saturated northern hardwood forest. Ecosystems 13, 1145–1156. doi: 10.1007/s10021-010-9379-5
Peng, X., and Wang, W. (2016). Stoichiometry of soil extracellular enzyme activity along a climatic transect in temperate grasslands of northern China. Soil Biol. Biochem. 98, 74–84. doi: 10.1016/j.soilbio.2016.04.008
Peñuelas, J., Poulter, B., Sardans, J., Ciais, P., van der Velde, M., Bopp, L., et al. (2013). Human-induced nitrogen-phosphorus imbalances alter natural and managed ecosystems across the globe. Nat. Commun. 4:2934. doi: 10.1038/ncomms3934
Peñuelas, J., Sardans, J., Rivas-ubach, A., and Janssens, I. A. (2012). The human-induced imbalance between C, N and P in Earth's life system. Glob. Chang. Biol. 18, 3–6. doi: 10.1111/j.1365-2486.2011.02568.x
Poorter, L., Wright, S. J., Paz, H., Ackerly, D. D., Condit, R., Ibarra-Manríquez, G., et al. (2008). Are functional traits good predictors of demographic rates? Evidence from five neotropical forests. Ecology 89, 1908–1920. doi: 10.1890/07-0207.1
Prentice, I. C., Meng, T., Wang, H., Harrison, S. P., Ni, J., and Wang, G. (2011). Evidence of a universal scaling relationship for leaf CO2 drawdown along an aridity gradient. New Phytol. 190, 169–180. doi: 10.1111/j.1469-8137.2010.03579.x
R Development Core Team . (2019). R: A Language and Environment for Statistical Computing. R Foundation for Statistical Computing, Vienna, Austria.
Rodrigues, J. L. M., Pellizari, V. H., Mueller, R., Baek, K., Jesus, E. D. C., Paula, F. S., et al. (2013). Conversion of the Amazon rainforest to agriculture results in biotic homogenization of soil bacterial communities. Proc. Natl. Acad. Sci. U. S. A. 110, 988–993. doi: 10.1073/pnas.1220608110
Rousk, J., Bååth, E., Brookes, P. C., Lauber, C. L., Lozupone, C., Caporaso, J. G., et al. (2010). Soil bacterial and fungal communities across a pH gradient in an arable soil. ISME J. 4, 1340–1351. doi: 10.1038/ismej.2010.58
Rumpel, C.Chabbi, A.Marschner, B. (2012). Carbon storage and sequestration in subsoil horizons: knowledge, gaps and potentials. R. Lal, K. Lorenz, R. F. Hüttl, B. U. Schneider, and J. von Braun. Recarbonization of the Biosphere. Berlin: Springer, 445–464.
Saiya-Cork, K. R., Sinsabaugh, R. L., and Zak, D. R. (2002). The effects of long term nitrogen deposition on extracellular enzyme activity in an Acer saccharum forest soil. Soil Biol. Biochem. 34, 1309–1315. doi: 10.1016/S0038-0717(02)00074-3
Schimel, J. P., and Weintraub, M. N. (2003). The implications of exoenzyme activity on microbial carbon and nitrogen limitation in soil: a theoretical model. Soil Biol. Biochem. 35, 549–563. doi: 10.1016/S0038-0717(03)00015-4
Schlatter, D. C., Kahl, K., Carlson, B., Huggins, D. R., and Paulitz, T. (2020). Soil acidification modifies soil depth-microbiome relationships in a no-till wheat cropping system. Soil Biol. Biochem. 149:107939. doi: 10.1016/j.soilbio.2020.107939
Schleuss, P. M., Widdig, M., Biederman, L. A., Borer, E. T., Crawley, M. J., Kirkman, K. P., et al. (2021). Microbial substrate stoichiometry governs nutrient effects on nitrogen cycling in grassland soils. Soil Biol. Biochem. 155:108168. doi: 10.1016/j.soilbio.2021.108168
Schmugge, T. J., Jackson, T. J., and McKim, H. L. (1980). Survey of methods for soil moisture determination. Water Resour. Res. 16, 961–979. doi: 10.1029/WR016i006p00961
Sinsabaugh, R. L., and Follstad Shah, J. J. (2011). Ecoenzymatic stoichiometry of recalcitrant organic matter decomposition: the growth rate hypothesis in reverse. Biogeochemistry 102, 31–43. doi: 10.1007/s10533-010-9482-x
Sinsabaugh, R. L., Hill, B. H., and Follstad Shah, J. J. (2009). Ecoenzymatic stoichiometry of microbial organic nutrient acquisition in soil and sediment. Nature 462, 795–798. doi: 10.1038/nature08632
Sinsabaugh, R. L., Lauber, C. L., Weintraub, M. N., Ahmed, B., Allison, S. D., Crenshaw, C., et al. (2008). Stoichiometry of soil enzyme activity at global scale. Ecol. Lett. 11, 1252–1264. doi: 10.1111/j.1461-0248.2008.01245.x
Sinsabaugh, R. L., Shah, J. J. F., Findlay, S. G., Kuehn, K. A., and Moorhead, D. L. (2015). Scaling microbial biomass, metabolism and resource supply. Biogeochemistry 122, 175–190. doi: 10.1007/s10533-014-0058-z
Smith, M. D. (2011). The ecological role of climate extremes: current understanding and future prospects. J. Ecol. 99, 651–655. doi: 10.1111/j.1365-2745.2011.01833.x
Soong, J. L., Fuchslueger, L., Marañon-Jimenez, S., Torn, M. S., Janssens, I. A., Penuelas, J., et al. (2020). Microbial carbon limitation: the need for integrating microorganisms into our understanding of ecosystem carbon cycling. Glob. Chang. Biol. 26, 1953–1961. doi: 10.1111/gcb.14962
Stock, S. C., Köster, M., Dippold, M. A., Nájera, F., Matus, F., Merino, C., et al. (2019). Environmental drivers and stoichiometric constraints on enzyme activities in soils from rhizosphere to continental scale. Geoderma 337, 973–982. doi: 10.1016/j.geoderma.2018.10.030
Stone, M. M., DeForest, J. L., and Plante, A. F. (2014). Changes in extracellular enzyme activity and microbial community structure with soil depth at the Luquillo critical zone observatory. Soil Biol. Biochem. 75, 237–247. doi: 10.1016/j.soilbio.2014.04.017
Sünnemann, M., Siebert, J., Reitz, T., Schädler, M., Yin, R., and Eisenhauer, N. (2021). Combined effects of land-use type and climate change on soil microbial activity and invertebrate decomposer activity. Agric. Ecosyst. Environ. 318:107490. doi: 10.1016/j.agee.2021.107490
Thingstad, T. F., Krom, M. D., Mantoura, R. F. C., Flaten, G. A. F., Groom, S., Herut, B., et al. (2005). Nature of phosphorus limitation in the Ultraoligotrophic eastern Mediterranean. Science 309, 1068–1071. doi: 10.1126/science.1112632
Treseder, K. K., and Vitousek, P. M. (2001). Effects of soil nutrient availability on investment in acquisition of N and P in Hawaiian rain forests. Ecology 82, 946–954. doi: 10.1890/0012-9658(2001)082[0946:EOSNAO]2.0.CO;2
Wakelin, S. A., Macdonald, L. M., Rogers, S. L., Gregg, A. L., Bolger, T. P., and Baldock, J. A. (2008). Habitat selective factors influencing the structural composition and functional capacity of microbial communities in agricultural soils. Soil Biol. Biochem. 40, 803–813. doi: 10.1016/j.soilbio.2007.10.015
Wang, D., and Ba, L. (2008). Ecology of meadow steppe in Northeast China. Rangel. J. 30, 247–254. doi: 10.1071/RJ08005
Wang, W., Li, Y., Guan, P., Chang, L., Zhu, X., Zhang, P., et al. (2022). How do climate warming affect soil aggregate stability and aggregate-associated phosphorus storage under natural restoration? Geoderma 420:115891. doi: 10.1016/j.geoderma.2022.115891
Wang, S., Zhou, G., Lu, Y., and Zou, J. (2002). Distribution of soil carbon, nitrogen and phosphorus along Northeast China transect (NECT) and their relationships with climatic factors. Chin. J. Plant Ecol. 26, 513–517.
Waring, B. G., Weintraub, S. R., and Sinsabaugh, R. L. (2014). Ecoenzymatic stoichiometry of microbial nutrient acquisition in tropical soils. Biogeochemistry 117, 101–113. doi: 10.1007/s10533-013-9849-x
Weedon, J. T., Aerts, R., Kowalchuk, G. A., and van Bodegom, P. M. (2011). Enzymology under global change: organic nitrogen turnover in alpine and sub-Arctic soils. Biochem. Soc. Trans. 39, 309–314. doi: 10.1042/bst0390309
Xu, S., Geng, W., Sayer, E. J., Zhou, G., Zhou, P., and Liu, C. (2020). Soil microbial biomass and community responses to experimental precipitation change: a meta-analysis. Soil Ecol. Lett. 2, 93–103. doi: 10.1007/s42832-020-0033-7
Xu, Z., Yu, G., Zhang, X., He, N., Wang, Q., Wang, S., et al. (2017). Soil enzyme activity and stoichiometry in forest ecosystems along the north-south transect in Eastern China (NSTEC). Soil Biol. Biochem. 104, 152–163. doi: 10.1016/j.soilbio.2016.10.020
Xu, Z., Zhang, T., Wang, S., and Wang, Z. (2020). Soil pH and C/N ratio determines spatial variations in soil microbial communities and enzymatic activities of the agricultural ecosystems in Northeast China: Jilin Province case. Appl. Soil Ecol. 155:103629. doi: 10.1016/j.apsoil.2020.103629
Yan, D., Li, J., Pei, J., Cui, J., Nie, M., and Fang, C. (2017). The temperature sensitivity of soil organic carbon decomposition is greater in subsoil than in topsoil during laboratory incubation. Sci. Rep. 7:5181. doi: 10.1038/s41598-017-05293-1
Yang, J., Wu, X., Chen, Y., Yang, Z., Liu, J., Wu, D., et al. (2021). Combined attributes of soil nematode communities as indicators of grassland degradation. Ecol. Indic. 131:108215. doi: 10.1016/j.ecolind.2021.108215
Zechmeister-Boltenstern, S., Keiblinger, K. M., Mooshammer, M., Peñuelas, J., Richter, A., Sardans, J., et al. (2015). The application of ecological stoichiometry to plant-microbial-soil organic matter transformations. Ecol. Monogr. 85, 133–155. doi: 10.1890/14-0777.1
Zhang, J., Ai, Z., Liang, C., Wang, G., Liu, G., and Xue, S. (2019). How microbes cope with short-term N addition in a Pinus tabuliformis forest-ecological stoichiometry. Geoderma 337, 630–640. doi: 10.1016/j.geoderma.2018.10.017
Zhang, X., Gao, Q., Yang, D., Zhou, G., Ni, J., and Wang, Q. (1997). A gradient analysis and prediction on the Northeast China transect (NECT) for global change study. Acta Bot. Sin. 39, 785–799.
Zhang, P., Guan, P., Hao, C., Yang, J., Xie, Z., and Wu, D. (2022). Changes in assembly processes of soil microbial communities in forest-to-cropland conversion in Changbai Mountains, Northeastern China. Sci. Total Environ. 818:151738. doi: 10.1016/j.scitotenv.2021.151738
Zhou, L., Liu, S., Shen, H., Zhao, M., Xu, L., Xing, A., et al. (2020). Soil extracellular enzyme activity and stoichiometry in China's forests. Funct. Ecol. 34, 1461–1471. doi: 10.1111/1365-2435.13555
Keywords: cropland, ecological stoichiometry, extracellular enzyme, nutrient limitation, Northeast China transect
Citation: Yang J, Guan P, Zhang P, Wu Y, Wang D and Wu D (2023) Stronger microbial nutrient limitations in subsoil along the precipitation gradient of agroecosystem: insights from soil enzyme activity and stoichiometry. Front. Ecol. Evol. 11:1137172. doi: 10.3389/fevo.2023.1137172
Edited by:
Ming-Chih Chiu, Institute of Hydrobiology (CAS), ChinaReviewed by:
Michał Filipiak, Jagiellonian University, PolandLie Xiao, Xi'an University of Technology, China
Copyright © 2023 Yang, Guan, Zhang, Wu, Wang and Wu. This is an open-access article distributed under the terms of the Creative Commons Attribution License (CC BY). The use, distribution or reproduction in other forums is permitted, provided the original author(s) and the copyright owner(s) are credited and that the original publication in this journal is cited, in accordance with accepted academic practice. No use, distribution or reproduction is permitted which does not comply with these terms.
*Correspondence: Donghui Wu, wudonghui@neigae.ac.cn