- Department of Biological Sciences, Columbia University in the City of New York, New York, NY, United States
The olfactory world is one of complex mixtures and blends containing up to hundreds of molecules. Many of those molecules can act as agonists, antagonists or enhancers at different receptors. This complicates the mechanism by which higher centers construct perceptions of complex mixtures. We propose that along with structural chemistry, psychophysics, the techniques of medicinal chemistry and machine learning can begin to shed light on this difficult neural problem.
"It's not the notes you play, it's the notes you don't play." – Miles Davis
How the brain interprets peripheral chemosensory stimulation remains an enduring puzzle. In mammals, olfaction begins with a transformation of chemical information of odors into electrical signals in the peripheral olfactory sensory neurons. These chemosensory messages are further processed in the olfactory bulb, the primary olfactory cortex (piriform cortex), and other higher brain regions, where olfactory perceptions are presumably ultimately formed. In the hierarchical processing of olfactory information, the binding of odorant molecules to odorant receptors and the subsequent alterations of electrical activity in the peripheral olfactory sensory neurons are the essential initial steps for olfactory perception.
Most of the history of olfactory studies has been dominated by chemistry and psychology. Odorous molecules were extracted, purified or synthesized by chemists and then tested for perceptual qualities in psychophysical laboratories. Some attempts at understanding molecular structure – function relations were attempted using various primary odor systems and the classification of odors with a wide range of descriptors. None of these schemes were more than useful guides for perfumers and other fragrance developers. There were always exceptions to any rule and many inexplicable cases of molecules appearing chemically closely related if not identical and yet possessing clearly different perceptions (pear and banana), or the reverse where identical perceptions (sandalwood, musk) could be created by chemically unrelated molecules.
With the discovery of the large family of odor receptors in 1991 it appeared that a ‘molecular basis of odor discrimination’ might be within reach (Buck and Axel, 1991). Through the tremendous efforts of many researchers, odorant ligands for quite a number of odorant receptors have been identified. Even though persistent technical difficulties have made it a daunting task to identify all odorant ligands for every single odorant receptor, currently available data support the hypothesis that a combinatorial, additive code would be a straight forward model for encoding sensory inputs (Malnic et al., 1999). This model is based on visual trichromacy and the blending or summation of the activation of several receptors – only 3 for vision, but now hundreds or more for odors. The notion of a ‘reciprocal combinatorial code’ became popular, in which molecules could activate multiple receptors and any given receptor could be activated by numerous molecules, and the pattern of receptor activation would uniquely identify any given odor. Biology would finally come to play a role between chemistry and psychology in determining odor perception at the molecular level.
Attempts at chemical structure-based odor prediction have proven to be more challenging than expected. The lack of established odor primaries, the high dimensionality of the stimulus and the failure to uniquely fit chemical parameters to odor qualities have resulted in overly broad definitions of odor quality – for example ‘pleasantness’ as a prime descriptor (Khan et al., 2007). Nonetheless the pursuit of structure-perception models continues, now with the aid of machine learning and AI algorithms that are fed with large databases of odors and their descriptors and then asked to predict the likely odor quality of novel molecules. Some of these models indeed look promising in their ability to replicate human subject responses (Keller et al., 2017; Lee et al., 2022). However, as with most machine learning technology the precise method by which the machine develops its predictive strategy, and even what that strategy is, remain largely black boxes. The proof is in the pudding in this case – if they work they must be doing something right. This may be a useful tool, but since the algorithm remains opaque we are no closer to an understanding of olfactory perception, even though we have now penetrated to a deeply reductionist level of analysis.
Nonetheless the continued use of these computational approaches with applied biological data could provide a sufficient synthesis to bring light to what remains a recalcitrant problem in olfaction – how the brain gets from molecules to perception. While difficulties with heterologous expression of odor receptors continue to be an obstacle to identifying ligands for many of the odor receptors, the molecular receptive range for numerous receptors in mouse and human have been identified, generally showing activation by multiple related compounds (e.g., short chain aldehydes, esters. Etc.). The primary difficulty with this research program, and that of the machine learning approach, is that it only takes into account the activity of single molecules at single receptors. In the real world the olfactory system is confronted with blends and mixtures of molecules that may be quite complex – containing up to several hundred individual molecular components. Many of those component molecules appear to have varied effects at different receptors in the context of other odors – modulating responses by inhibition or enhancement. This greatly complicates the pattern recognition model. We and others have observed these effects in various experimental preparations and it is now becoming clear that they are far more widespread than we might have imagined, and therefore are likely to play a significant role in olfactory discrimination and perception. Here we review a number of the biological contributions to this issue.
The perceptual difference between odor mixtures and their individual components has been frequently observed in psychophysical experiments (Cain, 1974; Gillan, 1983; Laing et al., 1984; Kay et al., 2005; Cashion et al., 2006). More specifically, the intensity of an odor mixture is often found to be less than the summed intensity of its constituents. Though not as extensively studied as in psychophysics, modulated responses to odor mixtures in the periphery have also been occasionally reported (Sell, 2014). For example, citral and a few cycloalkyl ring-containing aldehydes were found to be antagonists of octanal at the OR-I7 receptor (Araneda et al., 2004; Peterlin et al., 2008), methyl isoeugenol an antagonist of eugenol at the mOR-EG receptor (Oka et al., 2004), and decanedioic acid an antagonist of nonanedioic acid at the mOR42-3 receptor (Abaffy et al., 2007). While these cases of antagonism between odors can be attributed to the high structural similarity within each odor pair, other studies showed interactions between chemically unrelated odors, such as undecanal as an antagonist of 3-(4-tert-butylphenyl) propanal at the OR1D2 receptor (Spehr et al., 2003), and isoamyl acetate as an antagonist of whiskey lactone at the OR1G1 receptor (Chaput et al., 2012). When a receptor is broadly tuned, its antagonists also tend to be chemically diverse, such as 1-hexanol, hexanal cyclohexanone and isoamyl acetate as antagonists at OR1G1 (Sanz et al., 2005; Chaput et al., 2012), 2-methylcyclohexanone, 2-phenylethyl acetate and valeric acid at OR17-4 (Izewska, 2006). Interestingly, an earlier study showed that odor-evoked responses in olfactory neurons could also be inhibited by adrenergic and muscarinic antagonists, suggesting a wide spectrum of possible antagonists (Firestein and Shepherd, 1992). However, most early experiments on the odor interactions were performed with a small number of individual olfactory neurons or in heterologous odorant receptor expression systems due to technical limitations.
The invention of Swept Confocally-Aligned Planar Excitation (SCAPE) microscopy has inspired a novel solution to this problem (Bouchard et al., 2015; Voleti et al., 2019). SCAPE is a single-objective lightsheet-based volumetric imaging technique, capable of acquiring a large field of view (over 1,000 × 1,000 × 300 μm3) at high temporal resolution (over 10 volumes/s). Using SCAPE microscopy of intact olfactory epithelium of OMP-Cre+/− − GCaMP6f−/− mice, we performed high throughput analysis of single-cell responses to odor blends, simultaneously imaging ~10,000 peripheral olfactory sensory neurons (OSN) (Xu et al., 2020).
To assess how odors in a mixture interact at the primary odorant receptor level, we performed a large-scale analysis of over 10,000 odor-responsive OSNs. Those cells dominantly activated by one of three individual odors permit straightforward comparison between responses to that individual odor and responses to the mixture containing that odor. The dominant odor responses of most OSNs were largely unaffected by the other two components in the mixture. However, numerous exceptions were observed, with some cells showing inhibited or suppressed responses to the mixture; conversely, responses of some cells were enhanced.
To obtain a deeper understanding of the mechanism underlying these modulation effects, we performed a series of dose–response experiments with pairs of molecules for which we had established modulatory interactions. The suppression effect was consistent with a mechanism of competitive antagonism. In a few cases, one odor could activate the neuron and suppress the response to another agonist, a pattern that fits the standard model of partial agonism.
Supra-additive enhanced responses are more difficult to explain mechanistically than antagonism. An enhanced response to an agonist is generally ascribed to an allosteric mechanism (Kenakin, 2016). Although small molecule allosteric modulation of Class A GPCRs has been only rarely observed (Gregory et al., 2007; Burford et al., 2015; Garcia-Carceles et al., 2017; Ahn et al., 2018; Stanczyk et al., 2019; Wold et al., 2019), the wide diversity of the odorant receptor family appears to have revealed a greater occurrence of this mechanism in GPCRs of this type. Although we are currently unable to determine the allosteric site, from a chemical perspective the odor molecules are relatively hydrophobic so they could easily access the lipid membrane and bind to sites within the transmembrane regions of the receptor or alter the lipid membrane environment.
While we have used a simple blend of a just a few odors and further concentrated on one or two of them as modulators, there is nothing inherently special about any of these molecules, which means response modulation could also occur between any pair of odors that happen to activate/antagonize/enhance the same receptor. To test the idea of widespread modulation between odors, we tested a single lead compound, acetophenone, against six compounds of varying chemical and perceptual quality. Numerous acetophenone activated cells showed both suppression and enhancement by one or more of the other compounds. Thus, we suspect that a wide variety of molecules, including both those with and even without an apparent smell, could potentially act as modulators at different receptors.
Consistent with our observations with SCAPE microscopy, several recent studies also demonstrated widespread modulation among odorants at the receptor level using various other techniques. These approaches include in vivo studies of dorsal OSNs (and glomeruli) in GCaMP3 mice with two-photon microscopy (Inagaki et al., 2019; Zak et al., 2020), identification of odorant receptors displaying odorant modulatory effects first in freely behaving mice (de March et al., 2020; McClintock et al., 2020) or in dissociated OSNs (Pfister et al., 2020) and then verification of the modulation effects in some of these odorant receptors with heterologous expression assays, as well as theoretical modeling predictions (Marasco et al., 2016; Reddy et al., 2018; Singh et al., 2019).
All these lines of evidence support competitive antagonism as the most dominant form of modulation. In addition to partial agonism and synergetic and/or allosteric modulation we and others have reported, inverse antagonism in which high baseline activity in certain OSNs could be reduced by odorants (de March et al., 2020; Inagaki et al., 2020; Pfister et al., 2020; Zak et al., 2020).
The promise of a combinatorial, additive olfactory code analogous to that for color vision is now a clear over simplification. A simple correspondence between the chemical properties of odor molecules and their perception through the activation of a subset of receptors fails to take into account the highly complex interactions that occur between molecules and receptors in complex multi compound blends. The biological effects of antagonism and enhancement serve to significantly expand the dynamic range of the olfactory system in the recognition of complex blends, but at the cost of increasing complexity at the peripheral sensory cell level. This opens new questions as to how the higher brain centers manage a complex and non-linear input from the peripheral receptors.
We note in particular that this sort of complex interaction does not typically occur at primary receptors which are thought to be faithful reporters of the physical stimulus. Photons of a red wavelength, for example, do not antagonize green opsins. While there are interactions between red and green color channels these occur at higher levels of processing. Precisely how the brain manages to interpret these highly modulated signals from olfactory primary receptors remains exceptional and frankly obscure. Further complications may arise from concentration and temporal dynamics of both the stimulus and the responding neurons.
We suggest taking a pluralistic approach to searching for the solution, with a willingness to consider various options, including that there is no olfactory code, and to pursue multiple lines of investigation in parallel. The notion that there may be no code may seem hard to comprehend, but there is no strong evidence that one exists and even less evidence that one is necessary. Many complex biological processes proceed without a code or any sort of ‘map’ based on a code. An embryo develops from fertilization to a highly organized organism with no code or map but essentially a set of instructions carried out in a more or less linear fashion. Even the so-called ‘genetic code’ is not really a code but a complex set of chemical interactions with a great deal of randomness inherent in the process. Languages are not codes except that they are made up of smaller pieces, often meaningless phonemes that can be combined in an immense number of ways. We all simply learn some 40,000 or more words. Although dictionaries (code books) exist we do not utilize them to comprehend language. It may very well be that from a biological perspective it’s simply easier to develop a large number of responses to whatever odor complexes are present without referring to a code to interpret the odor perception. That is, the olfactory system is not a chemical analyzer that builds a perception out of molecular parts. Perceptions are created in a holistic manner in the same way that we perceive words. There may still exist in parallel, highly specific dedicated paths for odors crucial to survival – for example burning, predator or rotting odors, and the like.
Even if there is no overall code to be revealed there are still good scientific reasons for performing analytic experiments using the olfactory system. For one, it is the largest family of GPCRs by more than an order of magnitude, and with a considerable heterogeneity of structures as indicated from the divergence in gene sequences. For another, it is a tissue in which many, probably most, of these receptors are expressed at the same time but in a rigidly cell specific manner. Thus it is possible to observe the activity of many receptors to a given stimulus - either a single compound or a mixture of odors. In this regard machine learning and AI approaches are likely to be useful, even if they remain limited to single odor molecules. One possible solution to this obstacle is the application of medicinal chemistry principles rather than strict physical analytical chemistry.
Medicinal chemistry approaches were first developed in the pharmaceutical industry to aid in drug design and discovery. This approach involves using chemical modifications of compounds that are tested for biological activity – not chemical activity. Similarity between odorants is defined by their ability to activate and/or to modulate the same receptors, in addition to structural features. It is therefore of considerable interest to apply medicinal chemistry concepts to explore the key features of odorant antagonists, addressing the important question of whether, in the same odor molecule, those features leading to its agonist function are also critical contributors in its antagonist activity. The olfactory system affords us the opportunity to investigate the additional effects of an agonist at one receptor having antagonistic activity at another, or the possibility of enhancement by one compound on another, possibly by allosteric interactions. This can help break the bottleneck of predicting the perceptual quality of odor mixtures from molecular structures by overcoming two main obstacles. First, is the difficulty of obtaining training datasets as it requires human subjects to smell and evaluate hundreds of monomolecular odors. As the number of components in an odor mixture grows, the task will become exponentially more difficult. Second, even if such a training dataset could be obtained, it is difficult to generalize the result to different molecules or different combinations of known molecules. In computer vision a classical face recognition algorithm starts by dividing an input image into basic elements – edges. All the subsequent feature recognition (mouth, eyes, and ears) is based on the combination of edges. Similarly, medicinal chemistry breaks a molecule into basic elements called pharmacophores, or fragments, that bear biological significance (Figure 1). At this submolecular level, an odor mixture consisting of several molecules can be treated as a combination of such pharmacophores. Although feature loss is inevitable during the conversion from an odor mixture to the corresponding matrix of pharmacophores, the latter serves as a good approximation that could facilitate feature-based prediction.
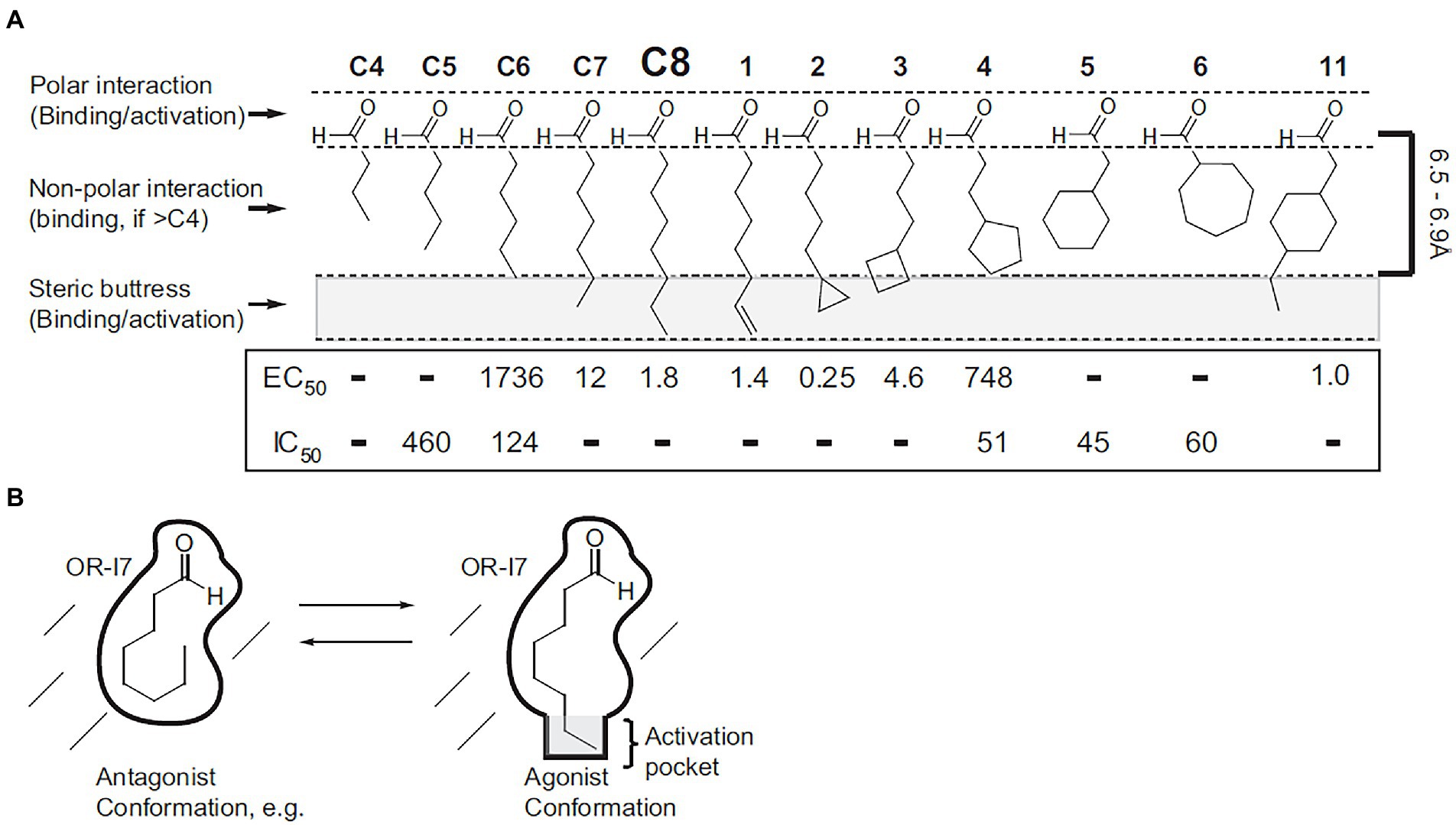
Figure 1. Using medicinal chemistry to determine the functional fragment required for odor activity. A series of conformation mimics were designed based on the structure of octanal, a known agonist of the odor receptor OR-I7. The biological activities of all chemicals were tested through calcium imaging of dissociated rat olfactory sensory neurons. (A) Structures, maximum lengths, and inhibition/activation constants. Regions of the structures responsible for binding and activation are indicated (left), as is the 6.5–6.9 Å length requirement for activation (right). Except for C4, which had neither type of activity, dashes in the IC50 row indicate that the compound was not tested for antagonism because it is strongly activating. Dashes in the EC50 row indicate that the compound had no activity within its solubility range. (B) Schematic depiction of octanal’s conformation on OR-I7’s activation. [Reprinted from Peterlin et al. (2008)].
The olfactory system then presents an added layer of medicinal chemistry application where we can observe the effects of a compound on multiple receptors simultaneously, not only on a single receptor as is common in pharmaceutical screening. This has revealed that there are compounds that act as both agonists and antagonists at different receptors and there is the possibility that even compounds that have no perceptible odor (at least to humans and mice) can nonetheless act as antagonists or enhancers at olfactory receptors in the presence of other agonists. One model that we have presented shows how this could be of considerable advantage by significantly expanding the range of discrimination between complex blends that are encountered by mammalian noses under natural conditions (Figure 2).
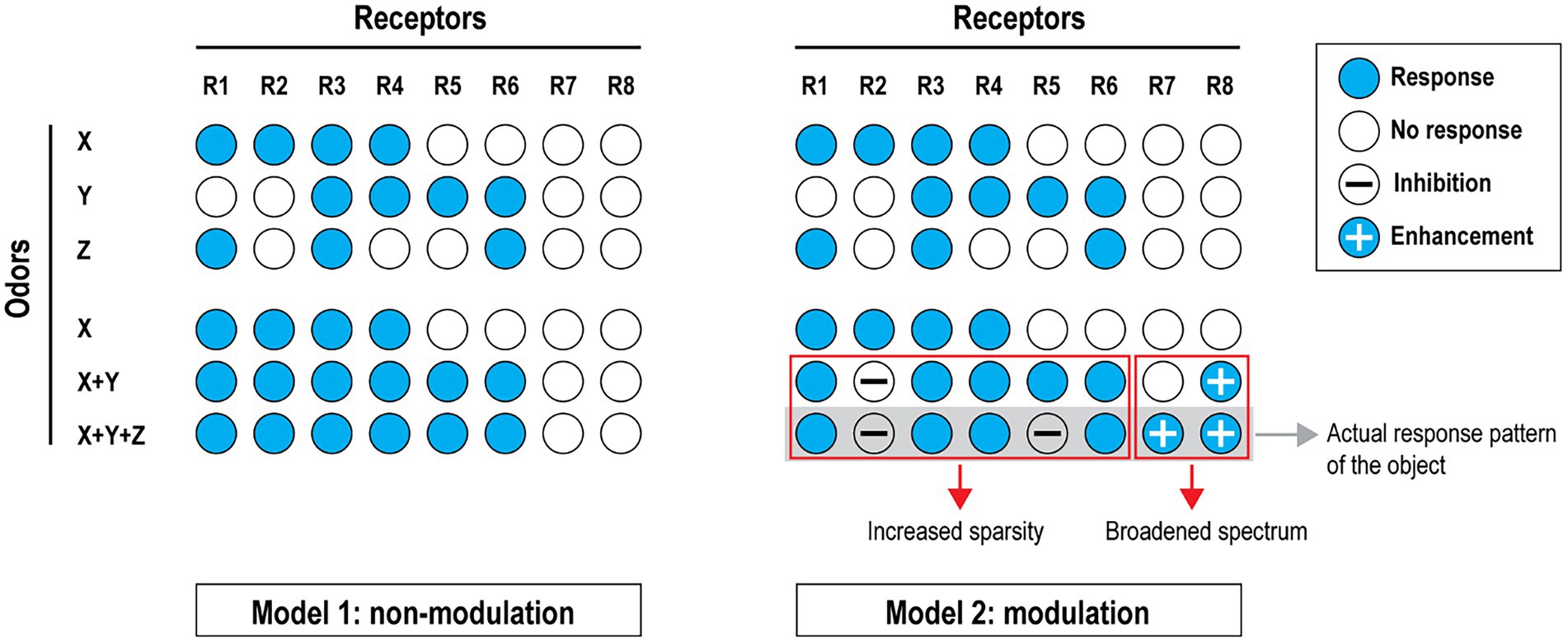
Figure 2. Diversified coding capacity through modulation. Two conceptual models are shown to contrast their robustness in odor mixture coding. Non-modulation model (left): Suppose odors X, Y and Z (all monomolecular compounds) can each activate a subset of odor receptors. In this model, mixing odor Y with odor X would recruit two more receptors but adding Z will not produce a different perception, although its response profile only partially overlaps with X and Y. Modulation model (right): In this model, all receptors are subject to modulation in addition to their activation profiles. Under one possible circumstance (similar to what we have observed), mixing odors X and Y results in the inhibition of receptor 2 and the enhancement of receptor 8. Adding odor Z into the mixture inhibits receptor 5 and enhances receptor 7. As a result, the sparsity is increased due to inhibition and the spectrum of odor coding is expanded through enhancement. Together these serve to increase the robustness of pattern detection as a mechanism of perception. This model also implies that ‘silent’ receptors (R7 and R8 in this case) might be as important as the activated ones in pattern recognition of an olfactory object. [Reprinted from Xu et al. (2020)].
Summary
In retrospect we should have expected that there would be significant modulation at the olfactory receptor level. Odorant receptors are after all canonical GPCRs and all other GPCRs are known to have strong antagonists as well as multiple agonists. In drug discovery, a well-established strategy is to screen the derivatives of a given ligand of the target receptor. The outcome can be highly diversified: when the original ligand is a full agonist, its derivatives can be inactive or any of the following: full agonists, partial agonists, inverse agonists, antagonists, etc. In the peripheral olfactory system, the same phenomenon exists to an even greater extent: on one hand, a given receptor that can be activated by one or more odors almost definitely has antagonists; on the other hand, many odorant receptor genes are located in the same gene cluster, meaning that they may share the same protein scaffold with only a few amino acid differences. This subtle difference, however, is sufficient to make a known agonist of one receptor an antagonist of the other. This certainly complicates any idea of a simple additive code and requires us to think more imaginatively about how the brain deciphers the notes that are not played.
Author contributions
All authors listed have made a substantial, direct, and intellectual contribution to the work and approved it for publication.
Conflict of interest
The authors declare that the research was conducted in the absence of any commercial or financial relationships that could be construed as a potential conflict of interest.
Publisher’s note
All claims expressed in this article are solely those of the authors and do not necessarily represent those of their affiliated organizations, or those of the publisher, the editors and the reviewers. Any product that may be evaluated in this article, or claim that may be made by its manufacturer, is not guaranteed or endorsed by the publisher.
References
Abaffy, T., Malhotra, A., and Luetje, C. W. (2007). The molecular basis for ligand specificity in a mouse olfactory receptor - a network of functionally important residues. J. Biol. Chem. 282, 1216–1224. doi: 10.1074/jbc.M609355200
Ahn, S., Pani, B., Kahsai, A. W., Olsen, E. K., Husemoen, G., Vestergaard, M., et al. (2018). Small-molecule positive allosteric modulators of the beta2-adrenoceptor isolated from DNA-encoded libraries. Mol. Pharmacol. 94, 850–861. doi: 10.1124/mol.118.111948
Araneda, R. C., Peterlin, Z., Zhang, X., Chesler, A., and Firestein, S. (2004). A pharmacological profile of the aldehyde receptor repertoire in rat olfactory epithelium. J. Physiol. 555, 743–756. doi: 10.1113/jphysiol.2003.058040
Bouchard, M. B., Voleti, V., Mendes, C. S., Lacefield, C., Grueber, W. B., Mann, R. S., et al. (2015). Swept confocally-aligned planar excitation (SCAPE) microscopy for high-speed volumetric imaging of behaving organisms. Nat. Photonics 9, 113–119. doi: 10.1038/nphoton.2014.323
Buck, L., and Axel, R. (1991). A novel multigene family may encode odorant receptors: a molecular basis for odor recognition. Cells 65, 175–187. doi: 10.1016/0092-8674(91)90418-X
Burford, N. T., Traynor, J. R., and Alt, A. (2015). Positive allosteric modulators of the mu-opioid receptor: a novel approach for future pain medications. Br. J. Pharmacol. 172, 277–286. doi: 10.1111/bph.12599
Cashion, L., Livermore, A., and Hummel, T. (2006). Odour suppression in binary mixtures. Biol. Psychol. 73, 288–297. doi: 10.1016/j.biopsycho.2006.05.002
Chaput, M. A., el Mountassir, F., Atanasova, B., Thomas-Danguin, T., le Bon, A. M., Perrut, A., et al. (2012). Interactions of odorants with olfactory receptors and receptor neurons match the perceptual dynamics observed for woody and fruity odorant mixtures. Eur. J. Neurosci. 35, 584–597. doi: 10.1111/j.1460-9568.2011.07976.x
de March, C. A., Titlow, W. B., Sengoku, T., Breheny, P., Matsunami, H., and McClintock, T. S. (2020). Modulation of the combinatorial code of odorant receptor response patterns in odorant mixtures. Mol. Cell. Neurosci. 104:103469. doi: 10.1016/j.mcn.2020.103469
Firestein, S., and Shepherd, G. M. (1992). Neurotransmitter antagonists block some odor responses in olfactory receptor neurons. Neuroreport 3, 661–664. doi: 10.1097/00001756-199208000-00001
Garcia-Carceles, J., Decara, J. M., Vázquez-Villa, H., Rodríguez, R., Codesido, E., Cruces, J., et al. (2017). A positive allosteric modulator of the serotonin 5-HT2C receptor for obesity. J. Med. Chem. 60, 9575–9584. doi: 10.1021/acs.jmedchem.7b00994
Gillan, D. J. (1983). Taste-taste, odor-odor, and taste-odor mixtures - greater suppression within than between modalities. Percept. Psychophys. 33, 183–185. doi: 10.3758/BF03202837
Gregory, K. J., Sexton, P. M., and Christopoulos, A. (2007). Allosteric modulation of muscarinic acetylcholine receptors. Curr. Neuropharmacol. 5, 157–167. doi: 10.2174/157015907781695946
Inagaki, S., Iwata, R., and Imai, T. (2019). Widespread inhibitory responses in the mouse olfactory sensory neurons in vivo. bioRxiv :803908. doi: 10.1101/803908
Inagaki, S., Iwata, R., Iwamoto, M., and Imai, T. (2020). Widespread inhibition, antagonism, and synergy in mouse olfactory sensory neurons in vivo. Cell Rep. 31:107814. doi: 10.1016/j.celrep.2020.107814
Izewska, P., Investigating the Molecular Basis of Ligand Binding to and Activation of Olfactory Receptors in Living Cells. (2006). Lausanne: EPFL.
Kay, L. M., Crk, T., and Thorngate, J. (2005). A redefinition of odor mixture quality. Behav. Neurosci. 119, 726–733. doi: 10.1037/0735-7044.119.3.726
Keller, A., Gerkin, R. C., Guan, Y., Dhurandhar, A., Turu, G., Szalai, B., et al. (2017). Predicting human olfactory perception from chemical features of odor molecules. Science 355, 820–826. doi: 10.1126/science.aal2014
Kenakin, T. (2016). “Identifying and quantifying allosteric drug function” in Allosterism in Drug Discovery (London: Royal Society of Chemistry), 24–39.
Khan, R. M., Luk, C. H., Flinker, A., Aggarwal, A., Lapid, H., Haddad, R., et al. (2007). Predicting odor pleasantness from odorant structure: pleasantness as a reflection of the physical world. J. Neurosci. 27, 10015–10023. doi: 10.1523/JNEUROSCI.1158-07.2007
Laing, D. G., Panhuber, H., Willcox, M. E., and Pittman, E. A. (1984). Quality and intensity of binary odor mixtures. Physiol. Behav. 33, 309–319. doi: 10.1016/0031-9384(84)90118-5
Lee, B. K., Mayhew, E. J., Sanchez-Lengeling, B., Wei, J. N., Qian, W. W., Little, K., et al. (2022). A principal odor map unifies diverse tasks in human olfactory perception. bioRxiv. doi: 10.1101/2022.09.01.504602
Malnic, B., Hirono, J., Sato, T., and Buck, L. B. (1999). Combinatorial receptor codes for odors. Cells 96, 713–723. doi: 10.1016/S0092-8674(00)80581-4
Marasco, A., De Paris, A., and Migliore, M. (2016). Predicting the response of olfactory sensory neurons to odor mixtures from single odor response. Sci. Rep. 6:24091. doi: 10.1038/srep24091
McClintock, T. S., Wang, Q., Sengoku, T., Titlow, W. B., and Breheny, P. (2020). Mixture and concentration effects on odorant receptor response patterns in vivo. Chem. Senses 45, 429–438. doi: 10.1093/chemse/bjaa032
Oka, Y., Omura, M., Kataoka, H., and Touhara, K. (2004). Olfactory receptor antagonism between odorants. EMBO J. 23, 120–126. doi: 10.1038/sj.emboj.7600032
Peterlin, Z., Li, Y., Sun, G., Shah, R., Firestein, S., and Ryan, K. (2008). The importance of odorant conformation to the binding and activation of a representative olfactory receptor. Chem. Biol. 15, 1317–1327. doi: 10.1016/j.chembiol.2008.10.014
Pfister, P., Smith, B. C., Evans, B. J., Brann, J. H., Trimmer, C., Sheikh, M., et al. (2020). Odorant receptor inhibition is fundamental to odor encoding. Curr. Biol. 30, 2574–2587.e6. doi: 10.1016/j.cub.2020.04.086
Reddy, G., Zak, J. D., Vergassola, M., and Murthy, V. N. (2018). Antagonism in olfactory receptor neurons and its implications for the perception of odor mixtures. elife 7:7. doi: 10.7554/eLife.34958
Sanz, G., Schlegel, C., Pernollet, J. C., and Briand, L. (2005). Comparison of odorant specificity of two human olfactory receptors from different phylogenetic classes and evidence for antagonism. Chem. Senses 30, 69–80. doi: 10.1093/chemse/bji002
Sell, C. S. (2014). “Interactions between odorants at the receptor” in Chemistry and the Sense of Smell (Hoboken, NJ: John Wiley & Sons), 114–118.
Singh, V., Murphy, N. R., Balasubramanian, V., and Mainland, J. D. (2019). Competitive binding predicts nonlinear responses of olfactory receptors to complex mixtures. Proc. Natl. Acad. Sci. U. S. A. 116, 9598–9603. doi: 10.1073/pnas.1813230116
Spehr, M., Gisselmann, G., Poplawski, A., Riffell, J. A., Wetzel, C. H., Zimmer, R. K., et al. (2003). Identification of a testicular odorant receptor mediating human sperm chemotaxis. Science 299, 2054–2058. doi: 10.1126/science.1080376
Stanczyk, M. A., Livingston, K. E., Chang, L., Weinberg, Z. Y., Puthenveedu, M. A., and Traynor, J. R. (2019). The delta-opioid receptor positive allosteric modulator BMS 986187 is a G-protein-biased allosteric agonist. Br. J. Pharmacol. 176, 1649–1663. doi: 10.1111/bph.14602
Voleti, V., Patel, K. B., Li, W., Perez Campos, C., Bharadwaj, S., Yu, H., et al. (2019). Real-time volumetric microscopy of in vivo dynamics and large-scale samples with SCAPE 2.0. Nat. Methods 16, 1054–1062. doi: 10.1038/s41592-019-0579-4
Wold, E. A., Chen, J., Cunningham, K. A., and Zhou, J. (2019). Allosteric modulation of class A GPCRs: targets, agents, and emerging concepts. J. Med. Chem. 62, 88–127. doi: 10.1021/acs.jmedchem.8b00875
Xu, L., Li, W., Voleti, V., Zou, D.-J., Hillman, E. M. C., Firestein, S., et al. (2020). Widespread receptor-driven modulation in peripheral olfactory coding. Science 368:eaaz5390. doi: 10.1126/science.aaz5390
Keywords: odor mixtures, antagonism, enhancement, allosteric, odors
Citation: Xu L, Zou D-J and Firestein S (2023) Odor mixtures: A chord with silent notes. Front. Ecol. Evol. 11:1135486. doi: 10.3389/fevo.2023.1135486
Edited by:
Claire Martin, Centre National de la Recherche Scientifique (CNRS), FranceReviewed by:
Sachiko Koyama, Indiana University Bloomington, United StatesRafi Haddad, Bar-Ilan University, Israel
Copyright © 2023 Xu, Zou and Firestein. This is an open-access article distributed under the terms of the Creative Commons Attribution License (CC BY). The use, distribution or reproduction in other forums is permitted, provided the original author(s) and the copyright owner(s) are credited and that the original publication in this journal is cited, in accordance with accepted academic practice. No use, distribution or reproduction is permitted which does not comply with these terms.
*Correspondence: Stuart Firestein, sjf24@columbia.edu