- 1Laboratory of Genomics, Maurice Lamontagne Institute, Fisheries and Oceans Canada, Mont-Joli, QC, Canada
- 2Marine Ecological and Evolutionary Physiology Laboratory, Département de Biologie, Chimie et Géographie, Université du Québec à Rimouski, Rimouski, QC, Canada
- 3Maurice Lamontagne Institute, Fisheries and Oceans Canada, Mont-Joli, QC, Canada
Introduction: Genetic variation underlies the populations’ potential to adapt to and persist in a changing environment, while phenotypic plasticity can play a key role in buffering the negative impacts of such change at the individual level.
Methods: We investigated the role of genetic variation in the thermal response of the northern shrimp Pandalus borealis, an ectotherm species distributed in the Arctic and North Atlantic Oceans. More specifically, we estimated the proportion transcriptomic responses explained by genetic variance of female shrimp from three origins after 30 days of exposure to three temperature treatments.
Results: We characterized the P. borealis transcriptome (170,377 transcripts, of which 27.48% were functionally annotated) and then detected a total of 1,607 and 907 differentially expressed transcripts between temperatures and origins, respectively. Shrimp from different origins displayed high but similar level of transcriptomic plasticity in response to elevated temperatures. Differences in transcript expression among origins were not correlated to population genetic differentiation or diversity but to environmental conditions at origin during sampling.
Discussion: The lack of genetic variation explaining thermal plasticity suggests limited adaptability in this species’ response to future environmental changes. These results together with higher mortality observed at the highest temperature indicate that the thermal niche of P. borealis will likely be restricted to higher latitudes in the future. This prediction concurs with current decreases in abundance observed at the southern edge of this species geographical distribution, as it is for other cold-adapted crustaceans.
1. Introduction
Increasing temperature is one major manifestation of global changes. To cope with increasing temperatures, some species may shift their distribution tracking their thermal niche, while others may persist in their local habitat (Perry et al., 2005; Lima et al., 2007; Bonebrake et al., 2018). Predicting a species’ ability to cope with global changes requires attaining an in-depth critical understanding of the mechanisms underpinning acclimatization and adaptation allowing species to persist and thrive in changing environments (Román-Palacios and Wiens, 2020). Acclimatization relies on phenotypic plasticity, the capacity of a given genotype to change its phenotypes according to the environment (Levins, 1963; Bradshaw, 1965). Phenotypic plasticity can act as a buffer for the negative effect of environmental changes on the performance and fitness of organisms (Ghalambor et al., 2007). Phenotypic plasticity is adaptive in environments that change predictably, i.e., when the cues for the development of a phenotype are reliable (Gavrilets and Scheiner, 1993; Reed et al., 2010; Scheiner and Holt, 2012). It represents a key mechanism for individuals and populations to persist in changing environments, and ultimately influencing both population dynamics and extinction risk (Reed et al., 2010; Ashander et al., 2016; Rescan et al., 2020).
Global changes are characterized by increased mean temperature, amplified environmental variation, and greater frequency and intensity of extreme thermal events (Alley, 2000; Rahmstorf and Coumou, 2011; Hobday et al., 2016; IPCC, 2022). These phenomena result in increasing environmental unpredictability and new selection horizons for living organisms. Under unpredictable changes, the reliability of environmental cues required to trigger the development of a given phenotype will diminish. In those conditions, phenotypic plasticity may be maladaptive, favorizing the evolution toward alternative strategies (Gavrilets and Scheiner, 1993; Reed et al., 2010; Scheiner and Holt, 2012; Tufto, 2015; Dey et al., 2016; Leung et al., 2020). For instance, individuals from multiple populations may evolve to a single phenotype that represents the optimal compromise among environmental conditions (e.g., Scheiner and Yampolsky, 1998). The lack of variation in plasticity levels may have for consequence to reduce the potential for evolutionary responses to climate changes (Oostra et al., 2018). Characterizing a species plasticity to temperature and the genetic variation underpinning these responses may help to predict a species’ vulnerability to global changes (Landy et al., 2020).
Genomic tools can help to improve prediction of organisms’ response to global changes by disentangling the molecular mechanisms involved in acclimatization and adaptation (De Wit et al., 2016; Kelly, 2019; Waldvogel et al., 2020). Gene expression is considered as a molecular phenotype to study phenotypic plasticity (Aubin-Horth and Renn, 2009; Pavey et al., 2010). Changes in gene expression underly phenotypic plasticity, as most changes in mRNA expression are associated with changes in protein production and abundance. It is shaped by both environmental and genetic components and evolves in response to selective pressures (Gerken et al., 2015; Leder et al., 2015). Alternatively, genome sequencing allows the inference of the evolutionary potential of a population, by characterizing and contrasting genetic compositions. Higher levels of genetic variation across a species distribution usually enable greater levels of phenotypic variation (Hansen, 2006), and a higher capacity of a species to undergo further adaptation via natural selection (Fisher, 1930; Hughes et al., 2008). Combined, these genomics tools help shedding light on the role of phenotypic plasticity and its genetic variation in the mechanisms of species’ responses to global changes.
Marine ectotherms are models of choice to understand the role of phenotypic plasticity and genetic variation in a species vulnerability to global changes (Huey et al., 2012; Paaijmans et al., 2013; Seebacher et al., 2014; Pinsky et al., 2019). In fact, ectotherms are particularly sensitive to temperature fluctuations due to their limited ability to use metabolic heat and maintain their body temperature (Angilletta, 2009). The adjustment of their body temperature to environmental conditions is therefore usually coupled with physiological and behavioral plasticity (Huey and Stevenson, 1979; Stevenson, 1985; Amarasekare and Savage, 2012; Abram et al., 2017). However, the level of plasticity varies largely among ectotherms (e.g., Somero, 2005, 2010; Logan et al., 2015).
The northern shrimp Pandalus borealis (Krøyer, 1838) is a circumboreal species distributed in the colder regions of North Atlantic Ocean. Adults and juveniles experience temperatures ranging from−1.7 to 11.1°C but are found to be most abundant between 0 and 5°C in the northwest Atlantic (Allen, 1959; Haynes and Wigley, 1969; Shumway et al., 1985; Garcia, 2007). Previous studies have shown that P. borealis is sensitive to increasing temperature, resulting in reduced survival, lowered recruitment, increased growth and metabolism rates (Chabot and Ouellet, 2005; Ouellet and Chabot, 2005; Daoud et al., 2007, 2010; Richards, 2012; Arnberg et al., 2013; Dupont-Prinet et al., 2013; Chemel et al., 2020). As a consequence, an important reduction in abundance has been observed over the last decade in the species southern distribution (Apollonio et al., 1986; Koeller, 2000; DFO, 2021, 2022; Richards and Hunter, 2021). This together with the high economic value of the species highlight the urgency of understanding the vulnerability of the northern shrimp to increasing temperatures of populations at higher latitudes.
In this study, we investigated the effect of elevated temperature to shed light on the molecular mechanisms underlying thermal plasticity in P. borealis and predict its vulnerability to ocean warming. We hypothesize a high level of transcriptomic plasticity in the northern shrimp in response to temperature, as an adaptation to the predictable variation of temperature that the species encounters on an annual or a life-cycle basis (Koeller et al., 2009). We thus predicted differences in plasticity among populations from contrasted environments and underlay by genetic variation. To test these hypotheses, we collected shrimp from three origins in a study area in the northwest Atlantic, with one origin close to a steep climatic gradient (Stanley et al., 2018). We exposed shrimp to three temperatures under laboratory conditions, mimicking current conditions, and future ocean warming scenarios. We built a reference transcriptome and identified differentially expressed transcripts (DETs) associated with temperature treatment, shrimp origin, or their interaction. The DETs were grouped in modules of expression profiles and their functions were explored to understand the molecular mechanisms underlying response to temperature. We also assessed the standing genetic variation and how it could explain temperature transcriptomic response to clarify the role of acclimatization and adaptation in thermal plasticity of P. borealis.
2. Materials and methods
2.1. Specimen collection, transport and experimental design
Female P. borealis were collected from three different origins along the northwest Atlantic representing different oceanographic conditions: St. Lawrence Estuary (SLE), Eastern Scotian Shelf (ESS) and Northeast Newfoundland Coast (NNC) (Figure 1; Supplementary Table S1). Collection, transport and maintenance were conducted as described in Guscelli et al. (submitted for review). Briefly, shrimp were collected using a rigid frame trawl (SLE), shrimp traps (ESS), or commercial shrimp trawl (NNC). Supplementary Table S1 provides dates and positions. Live shrimp were transported to the Maurice Lamontagne Institute (MLI) in 750 L tank filled with 2–3°C water. The only long-distance transportation that took place when air temperature was above 5°C (NNC to MLI) used a refrigerated truck. Air bubbling kept water well oxygenated during transport. Upon arrival at MLI, shrimp were transferred into shallow (40–50 cm depth) rectangular tanks (1700 L) filled with sea water at a temperature of 4.5°C, pH of 7.9 (total scale), salinity of 32 PSU (Practical Salinity Unit) and 100% O2 saturation relative to air. Shrimp were fed ad libitum with a mixture of capelin (Mallotus villosus, Müller, 1776) and shrimp (Pandalus spp.) three times a week prior the beginning of the experiments.
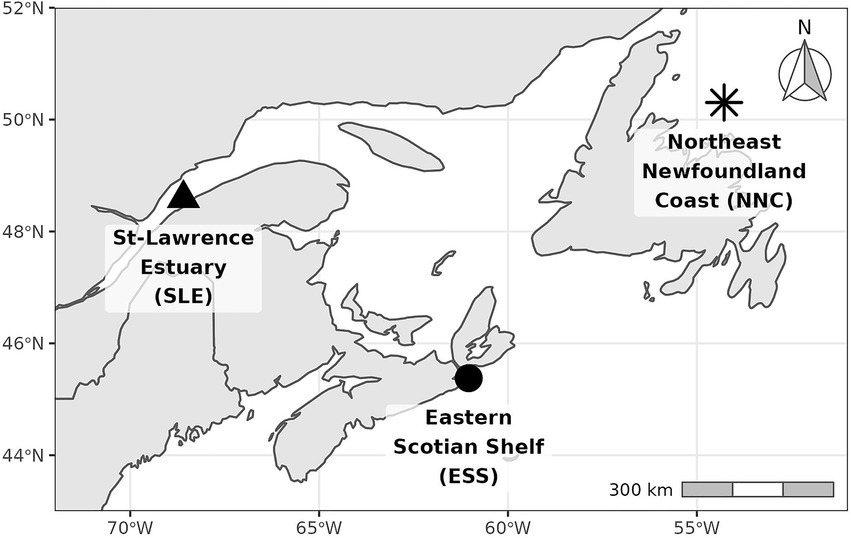
Figure 1. Pandalus borealis collection sites. Map of the southeast of Canada with the three geographic locations where shrimp used in this study originated from. Collection conditions are described in Supplementary Table S1.
After approximately eight weeks, female shrimp were transferred into experimental tanks kept at 2, 6 or 10°C (two replicate tanks per temperature treatment) for 30 days. As this experiment was part of a project also dealing with ocean acidification, all tanks were maintained at a slightly acidified pH, 7.75 (total scale), matching current conditions of the deep waters of the Gulf of St. Lawrence (Mucci et al., 2011, 2018). The coldest temperature, 2°C, represents the temperature where shrimp are most abundant along the Labrador and Newfoundland coast (Orr and Sullivan, 2013) and within the range where most SLE shrimp were found in 2008–2021 (1–5.5°C; DFO, 2022). The intermediate temperature represents a predicted 4°C increase by the end of this century according to the RCP 8.5 scenario (IPCC, 2014) for shrimp living close to 2°C presently, and it is also the current temperature (5–7°C) in the deep waters of the Gulf of St. Lawrence, where shrimp populations are found (Bourdages et al., 2022). The highest temperature, 10°C, represents predicted conditions at the end of the century for populations that are already exposed to 6°C (Lavoie et al., 2020). Dead and moulting individuals were counted daily. Dead individuals were frozen until waste collection according to MLI policy. Shrimp from different origins were exposed to the same experimental conditions but they could not be exposed simultaneously, due to space limitations within the experimental system and also to keep the period between capture and the beginning of the experimentation constant. Consequently, exposure to all the three experimental temperatures occurred in August 2018, April 2019, and December 2019 for shrimp from SLE, ESS, and NNC, respectively. After 30 days of exposure, six randomly chosen individuals (three from each tank) were collected from the experimental system and immediately flash-frozen in liquid nitrogen. Samples were then stored at−80°C until nucleic acid extraction was conducted. The duration of exposure was chosen to mimic northern latitude deep-water temperature fluctuation where intra-seasonal variability occurs at a period of 10–30 days (Fischer et al., 2004). At the end of the experiment, we did not find any differences in shrimp size (cephalothorax length = 23.91 mm, s.d. = 1.50) among temperature (F(2,47) = 2.097, p = 0.134) or origin (F(2,47) = 1.845, p = 0.169).
2.2. DNA/RNA extraction, sequencing and bioinformatics preprocessing
We assessed genetic variation and gene expression levels among individuals by generating ddRAD-sequencing and RNA-sequencing (seq), respectively. Genomic DNA and RNA extraction of the 54 shrimp (three temperature treatments × three origins × six shrimp, combining three from each replicate tank) was carried out on ca. 2 g of muscle tissue from shrimp abdomen, using QIAGEN DNeasy Blood & Tissue kit and Qiazol RNA extraction reagent (Qiagen), respectively, following the manufacturer’s protocol. Muscle was chosen to ensure enough material was available as input for sequencing, and because this tissue displayed metabolic changes in response to temperature changes in studies of other shrimp species, in addition to be responsible for general locomotion and escape-behavior responses in decapods (e.g., Rosa et al., 2014; Madeira et al., 2020). RNA-seq methodology was selected to investigate the changes in the entire transcriptome, with no a priori and less bias manner than selecting specific genes, in addition to displaying more power in revealing gene expression changes that could be difficult to discerned through more targeted approaches.
Preparation of ddRAD-seq libraries for the genomic DNA was carried out by the Institut de Biologie Intégrative et des Systèmes (IBIS at Université Laval, Quebec City, QC, Canada) with PstI and MspI restriction enzymes, and using the procedure described by Poland et al. (2012), with the following exceptions. First, a blue Pippin (SAGE sciences) was used to size libraries before PCR amplification (elution set between 50 and 65 min, on a 2% agarose gel); second, plate barcoding was used to enable sequencing on a shared Illumina NovaSeq 6,000 S4 PE150 lane, as described in Colston-Nepali et al. (2019). The 54 DNA samples were added to a larger pool of samples (total 1858 samples), and split into 20 libraries of 96 samples. Libraries were sequenced at Génome Québec (Montreal, QC, Canada).
For the RNA samples, library construction was performed by Génome Québec, using NEB mRNA stranded library preparation kit. In addition to the 54 libraries (one per individual), a supplemental library was constructed from a pool of 120 shrimp exposed in different environmental conditions and from various populations (Supplementary Table S2), to maximize the number of detected genes during de novo transcriptome assembly. Finally, high-throughput sequencing steps for all libraries (Illumina NovaSeq 6,000 S4 PE100) were performed by Génome Québec.
For the resulting DNA sequencing dataset, adapters were removed with Trimmomatic v0.39 (Bolger et al., 2014), and three base pairs were removed from the R2 (the MspI restriction site) to account for lower quality in the first nucleotides. Demultiplexing and quality filtering were performed with the process_radtags module of STACKS v2.4 (Catchen et al., 2013; Rochette et al., 2019), with a truncation at 135 pb and a check for the PstI restriction site. Cleaned reads were aligned to a P. borealis reference genome (newly assembled by C3G–McGill University, Montreal, QC, Canada) with mem from BWA-MEM with default parameters. Aligned paired-end reads were assembled with the gstacks modules and only samples with a mean depth of coverage of at least 5× were kept in the analysis. SNPs were first filtered with populations module of STACKS to only include SNPs present in all three origins, for at least 75% of individuals and with a minimum minor allele frequency of 5%. Furthermore, SNPs with more than 10% missingness and in Hardy–Weinberg disequilibrium in more than one origin were removed. Only the first SNP by loci was kept to avoid linkage disequilibrium. The ddRAD-seq final dataset consisted of 22,227 SNPs over 54 individuals.
Transcriptome assembly and annotation, and isoform count matrix estimation, were performed by C3G. De novo transcriptome assembly was performed following the pipeline described by Haas et al. (2013) and based on the Trinity assembly software suite. In brief, raw reads were trimmed using Trimmomatic from the 3′ end to have a minimum Phred score of 30 and a minimum length of 50 bp. Normalization was performed using the Trinity normalization utility v2.0.4 (Haas et al., 2013). An abundance estimate was computed using RNA-Seq by Expectation Maximization (RSEM) v1.2.12 (Li and Dewey, 2011) via Trinity align_and_estimate_abundance.pl utility. Expected count values from RSEM were used for downstream analysis. Transcripts were annotated with the Trinotate suite v2.0.2 (Haas et al., 2013), based on a homology search to known sequence data (BLAST+/SwissProt/Uniref90), protein domain identification (HMMER/PFAM), protein signal peptide and transmembrane domain prediction (signalP/tmHMM), comparison to currently curated annotation databases (EMBL UniProt eggNOG/GO Pathways databases). Each transcript was aligned against a custom protein database of the Pacific white shrimp Penaeus vannamei (Boone, 1931, GenBank: QCYY00000000.1). An annotation was assigned to the longest putative coding transcript based on the best BLAST hit with an associated gene name and an Expect (E) value cut-off <1e−5 whenever possible. All of the above described analyzes were performed using the GenPipes pipeline framework [PMID: 31185495].
2.3. Statistical analyzes
All statistical analyzes were carried out using R software, version 4.1.0 (R Core Team, 2020).
2.3.1. Mortality and molting rates
Mortality data used in this study originate from Chemel et al. (2020) and Guscelli et al. (submitted for review). We performed an univariate two-ways ANOVA test on arcsin square-root transformed percentage values (Sokal and Rohlf, 1995) to investigate how temperature affected shrimp mortality and molting rates per day, with Origin and Temperature as fixed factor tested in isolation and combined (Origin × Temperature). In addition, a Tukey’s Honestly Significant Difference (HSD) test was used to conduct post-hoc analyzes, when significant effects were detected by the ANOVA.
2.3.2. Differential transcript expression analysis
Variation partitioning of gene expression and differential expression analyzes were performed using the R package vegan version 2.6–2 (Oksanen et al., 2022) and the Bioconductor’s package DESeq2 version 1.36 (Love et al., 2014). Expected read count values from RSEM were used to identified differentially expressed transcripts (DETs). The count matrix was first pre-filtered to remove every transcripts not or sparsely expressed according to the criterion based on Chen et al. (2016). Briefly, a transcript was considered expressed if it had a count of at least 10–15 in at least some libraries, but basing the filtering on count-per-million (CPM) values so as to avoid favoring genes that are expressed in larger libraries over those expressed in smaller libraries. We used a cutoff = 10/L where L is the minimum library size in millions. From the 118,609 identified transcripts, the pre-filtering process retained a total of 39,065 transcripts. To quantify the proportion of the total gene expression variation that was explained by the main factors or their interactions, we applied partial redundancy analyzes (RDA; Borcard et al., 1992), using the normalized count matrix (i.e., applying a variance stabilizing transformation (VST) to the count data, as implemented in DESeq2) as the response variable and the Origin and Temperature as categorical explanatory factors. Note that the six randomly chosen individuals were combining individuals from two tank replicates. Prior to this variation partitioning, we have tested for the tank effect on gene expression by performing a partial RDA adding tank identity as an explanatory variable.
We also identified any transcripts that showed changes in expression across the same three terms (i.e., Origin, Temperature and Origin × Temperature interaction), by building a general linear model, as implemented in DESeq2. Significance of each explanatory factor was assessed by applying a Likelihood Ratio Test (LRT) approach, comparing the full model to reduced ones (Love et al., 2014). Transcripts with FDR < 0.001 [value of p after Benjamini-Hochberg (BH) adjustment] were considered as differentially expressed. For all identified DETs, we assessed how expression levels varied across the three temperatures and origins by identifying modules of co-expressed transcripts. Transcripts whose expression profiles were highly correlated were grouped within a given module, using the WGCNA version 1.71 (Langfelder and Horvath, 2008). Enriched gene ontology (GO) terms were then identified, using Fisher’s exact test as implemented in the topGO version 2.48 (Alexa and Rahnenfuhrer, 2022), to assess the functional role of DETs among origins or according to temperature treatment. For each GO term with FDR < 0.05, we calculated the proportion of identified co-expressed module.
2.3.3. Origin effect on gene expression
The origin effect on the measured gene expression could arise from different sources: 1–genetic differences among sampling sites, or 2–environmental conditions in shrimp natural habitat (as individuals were sampled as adults, each of them might express specific genes programmed during their development). We attempted to clarify the role of these two potential sources of shrimp gene expression by comparing the effect of population genetic variation, and environmental conditions at origins on transcript expression. Genetic variation was assessed using 22,227 SNPs called from ddRAD-seq dataset, and comparing the same 54 individuals as transcriptomic analysis. We assessed intra-population genetic diversity by calculating the expected heterozygosity (HE) for each origin, using poppr version 2.9.3 (Kamvar et al., 2014), and genetic differentiation among collection sites with pairwise FST, using dartR version 2.0.4 (Gruber et al., 2018). Environmental conditions at origins were characterized by monthly mean salinity and temperature for both surface and bottom sea water, obtained from Bedford Institute of Oceanography North Atlantic Model (BNAM) that averaged values over 1990 to 2015 period (Wang et al., 2018) and selected by removing highly correlated variables (|r| > 0.90; Supplementary Figure S2). To disentangle the different sources of gene expression that could explain the origin effect, we performed a variation partitioning (Peres-Neto et al., 2006; Legendre, 2008) of the transcriptomic response. This enabled us to assess the proportion of transcript expression explained by the terms Temperature, Environmental conditions at origin (hereafter Environment) and Genetic Differentiation (hereafter Genetics).
Factors explaining shrimp transcript expression were also determined by a model selection analysis using the function ordiR2step from vegan R package. We performed a model selection to obtain a parsimonious model maximizing the adjusted R2 and p-value of a constrained ordination (Blanchet et al., 2008). We used the gene expression normalized count matrix as the response variable, and PCoA coordinates from pairwised FST matrix (for genetic differentiation) and Environment as the explanatory variables, in addition to the interaction terms Temperature × Genetics and Temperature × Environment, to test for standing genetic variation for plasticity and effect of past environmental memory on gene expression, respectively.
3. Results
3.1. Mortality and molting rates variation
Variation in mortality and molting rates were observed for female P. borealis collected from three origins and exposed to 2, 6, or 10°C temperatures. Mean mortality rates increased proportionally with temperature (F2, 9 = 6.917; p = 0.015), independently of origin (F4, 9 = 1.835; p = 0.206). Mean percentages of death per day differed between 2°C (0.084 ± 0.009) and 10°C (0.180 ± 0.018; p < 0.05) but not with 6°C (0.110 ± 0.023; Figure 2A).
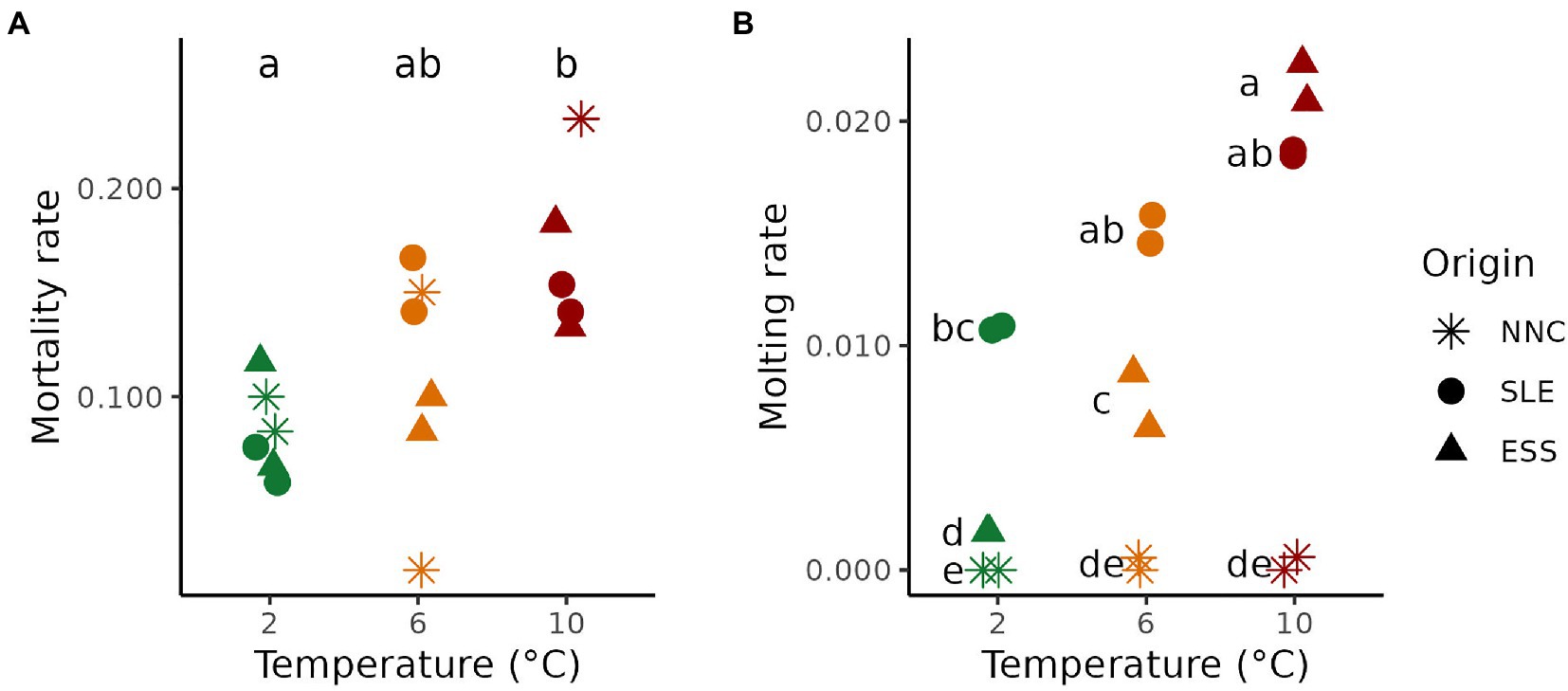
Figure 2. Pandalus borealis mortality and molting rates as function of Temperature and Origin. (A) Mortality rate at each treatment temperature (green, orange and red for 2, 6 and 10°C, respectively) for shrimp from different origins (shape). (B) Molting rate at each treatment temperature for shrimp from different origins. The results of Tukey HSD post hoc test are displayed as letters, with different letters representing a significant difference between means (p < 0.05). ESS = Eastern Scotian Shelf, SLE = St. Lawrence Estuary, NNC = Northeast Newfoundland Coast. Both replicate tanks for each treatment are shown.
Molting rates varied between 0.00 ± 0.00 (at 2°C in NNC) and 0.022 ± 0.001 (at 10°C in ESS). Mean molting rates differed with increasing temperatures for shrimp from the three origins, as indicated by the presence of an interaction between the terms Origin and Temperature (F4, 9 = 16.412; p < 0.001; Figure 2B). Shrimp from NNC and SLE displayed no significant changes along the temperature gradient tested, but shrimp from NNC, and not those from SLE, displayed a quasi-absence of molt during the whole experiment (Figure 2B). For ESS, molting rate increased with temperature (Figure 2B).
3.2. Reference transcriptome
The reference transcriptome of P. borealis was built using RNA from pooled individuals in various physiological conditions and samples from the current study experiment (Supplementary Table S2), maximizing thereby the number of detected genes. We obtained a total of 2.81 × 109 raw paired reads, from which 97.48% remained after the trimming step. The de novo assembly resulted in 170,377 transcriptome isoforms that could be grouped into 118,609 components loosely representing genes. Transcript contig length ranged from 224 to 28,419 bp, with a N50 value of 1820 bp.
Functional annotations were performed on homology search to known sequence data based on the protein database of another shrimp species, P. vannamei. About 27.48% of the transcripts were successfully annotated to a specific function. Filtration of assembled transcriptome based on the functional annotation resulted in high quality contigs, composed of 46,828 transcript groups into 24,122 genes. Transcripts lengths from this filter assembled transcriptome ranged from 224 bp to 28,419 bp, with a N50 value of 3,049 bp.
3.3. Differential gene expression analysis revealed extensive transcriptomic plasticity
For shrimp collected from the temperature experiment, a total of 39,065 transcripts were expressed at a count greater than 10 reads in at least one sample. Variation partitioning analysis revealed marginal effects of Temperature (adj. R2 = 0.87%; p = 0.015), Origin (adj. R2 = 1.75%; p = 0.001) and their interaction (adj. R2 = 1.34%; p = 0.006) on gene expression levels. Differential expression analysis detected a higher number of DETs across the temperatures tested (n = 1,607; 4.11%), than across origins (n = 907; 2.32%), and a very low number of significantly DETs were detected for the interaction term (n = 13; 0.03%). Ordination plot of distance-based RDA (Figure 3A) showed that the gene expression level gradually changed with increasing temperature (db-RDA 1: 22.50%; p = 0.001). In addition, the gene expression level of shrimp from NNC differed markedly from those reported for shrimp from SLE and ESS (db-RDA 2: 20.11%; p = 0.001).
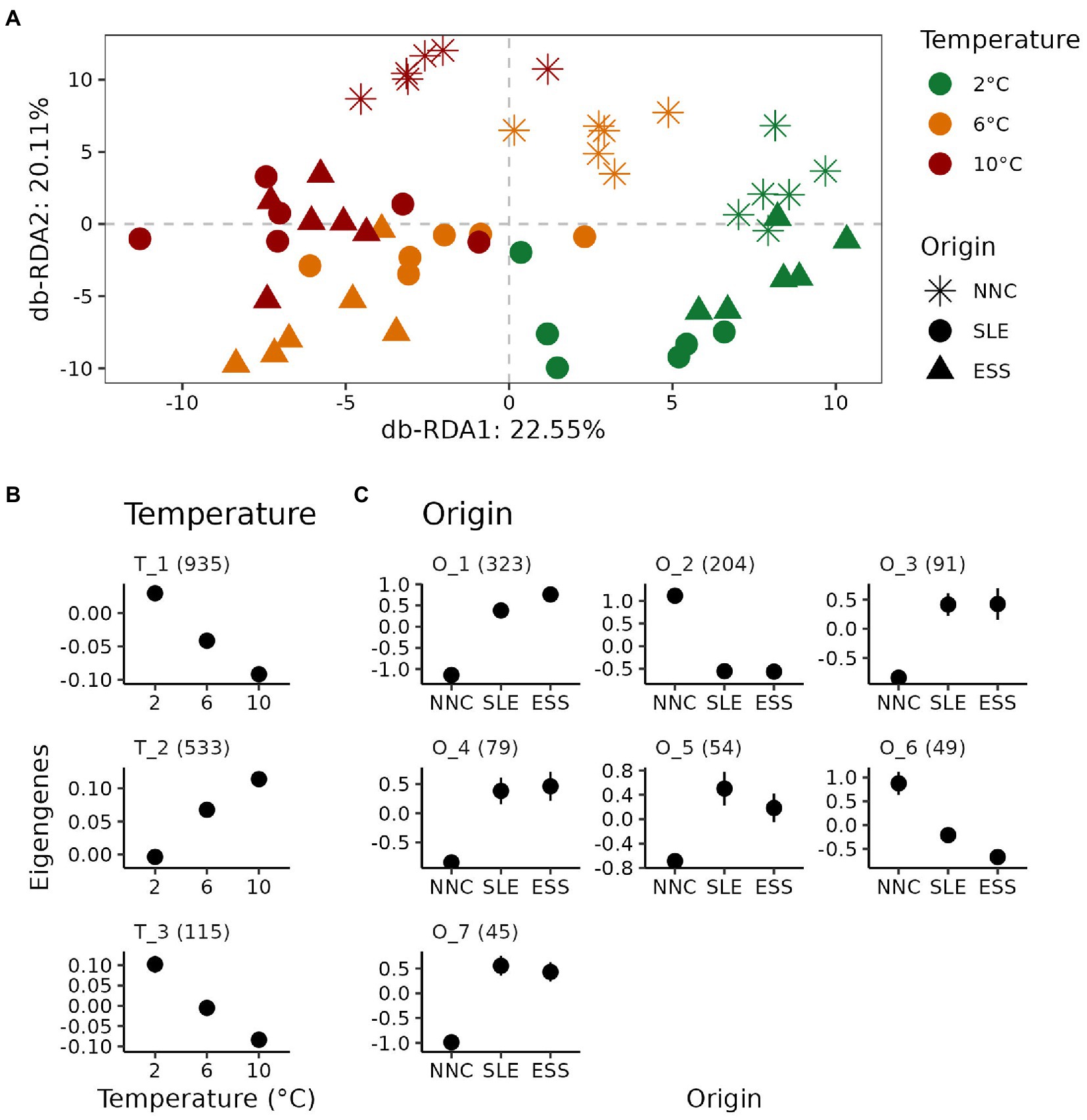
Figure 3. Gene expression response to temperature exposure of P. borealis shrimp from three different origins. (A) Variation of gene expression levels. Distance-based redundancy analysis (db-RDA) plot performed on gene expression levels according to origins (shapes) and temperature treatments (colors). (B,C) Relative expression level for each co-expression modules for differentially expressed transcripts (DETs) among temperatures (T) and origins (O), respectively. Means with their standard errors are represented as dots and error bars, respectively. Numbers in parentheses indicate the number of transcripts belonging to each co-expression module.
The patterns of expression profiles of DETs identified through co-expression module analysis differed between Temperature and Origin effects. A total of 1,583 (98.51%) and 845 (93.16%) DETs were grouped across temperatures and origins, respectively, into modules of co-expressed transcripts (Figures 3B,C). We identified three and seven modules for DETs across temperatures and origins, respectively (Figures 3B,C). For temperature, gene expression levels increased (module T_2) or decreased (modules T_1 and T_3) linearly with increasing temperature for all three modules identified (Supplementary Figure S1A). For origin, six out of the seven modules identified showed SLE and ESS displaying similar expression levels (modules O_2 and O_7), but differing from those of NNC (Figure 3C).
GO enrichment analyzes revealed that DETs associated with temperature treatments or origins involved distinct functions. For instance, Temperature affected the expression of genes belonging to the three ontology categories (i.e., biological processes, cellular components and molecular functions), whereas Origin affected the expression of genes associated only to cellular components and molecular functions (Supplementary Figure S1A).
For biological processes affected by temperature, a large proportion of transcripts belonging to the largest module, T_1, involved catabolic activities (88.47%, s.d. 2.76%; Supplementary Figure S1B), where catabolic activities mostly decreased with increasing temperatures. In contrast, the gene expression of module T_2 increased with increasing temperatures. This module has a larger proportion of genes involved in metabolic activities (30.27%, s.d. 2.81%; Supplementary Figure S1B) compared to those involved in catabolic activities (11.53%, s.d. 2.76%; Supplementary Figure S1B).
In terms of cellular components, transcripts associated to protein, catalytic and channel complexes were mostly downregulated with increasing temperature whereas those involved in cell structures, such as actin and myosin, differed significantly among shrimp from different origins. Most cellular components associated with DETs across shrimp origins were part of module O_1 with the expression of these specific transcripts being higher in shrimp from ESS and progressively lower in shrimp from SLE and from NNC (Supplementary Figure S1B), except extracellular functions. These latter functions were represented by up to five modules characterized by similar expression levels for shrimp from ESS and SLE, which were different from NNC. Some DETs with extracellular functions were associated with cytoskeleton and non-membrane-bounded organelle. In terms of molecular functions, Temperature modified the expression of genes associated with nucleic and amino acid activities, whereas Origin involved DETs associated with structural activities. For temperature, transcripts associated with threonine related activities decreased with increasing temperature.
3.4. Identifying factors affecting Pandalus borealis transcriptomic plasticity
3.4.1. Genetics differences among origins
Shrimp displayed genetic differences among origins. Individuals from SLE had lower genetic diversity compared to shrimp from NNC and ESS (Tukey HSD, p < 0.001; Figure 4A) but differences in gene diversities were small (HE ranging from 0.215 to 0.223). No genetic differentiation was detected between NNC and SLE (FST = 0, p = 0.940), whereas ESS was differentiated from the two other origins (FST > 0.003, p < 0.001; Figure 4B).
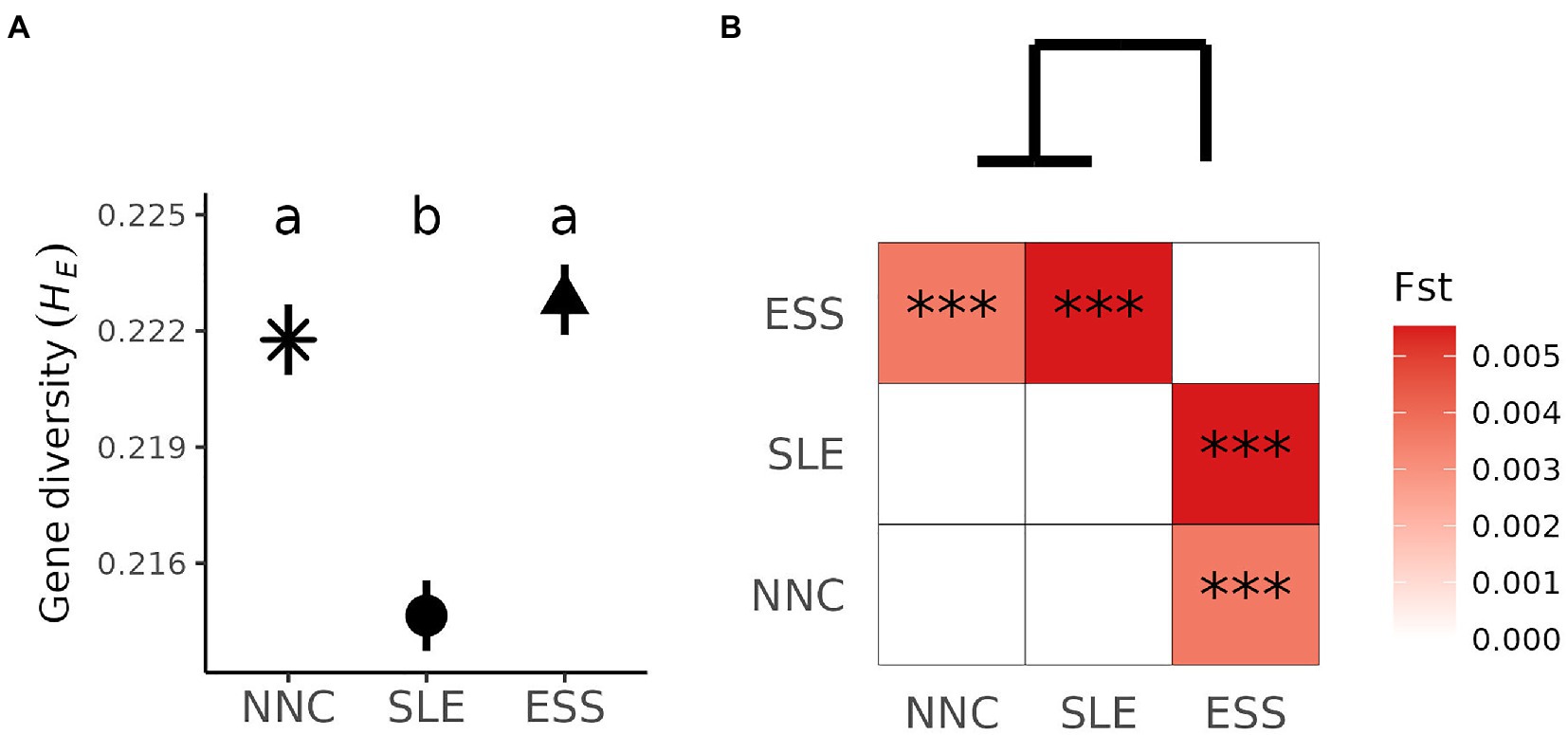
Figure 4. Pandalus borealis genetic variation. (A) Within origin genetic variation. Mean and standard error of gene diversity (HE) for each origin. The result of Tukey HSD (honest significant difference) post hoc tests are displayed as letters, with different letters representing significant differences among origins’ mean values (p < 0.001). (B) Among origins genetic variation. Pairwise genetic differentiation (FST) among shrimp’s origin varied from white (zero) to red (high), as shown on the right-hand side of the heat-map and values significantly different from zero are denoted by *** for p < 0.001. The dendrogram on the top resulted from hierarchical cluster analysis based on the pairwise FST. ESS = Eastern Scotian Shelf, SLE = St. Lawrence Estuary, NNC = Northeast Newfoundland Coast.
3.4.2. Environmental conditions at origins
Environmental conditions at origins (hereafter Environment) were contrasted. We used only the January and July bottom temperatures since these two variables characterized female shrimp habitat and were not strongly correlated (r = 0.32), unlike other variables (Supplementary Figure S2 and see method section for more details about variables selection). The NCC origin had the most different environmental conditions (Euclidean distance with SLE and ESS > 4.04) compared to SLE and ESS, which were more similar (Euclidean distance between SLE and ESS = 2.93). The origin NNC was characterized by cold temperatures compared to ESS and SLE (Figure 5).
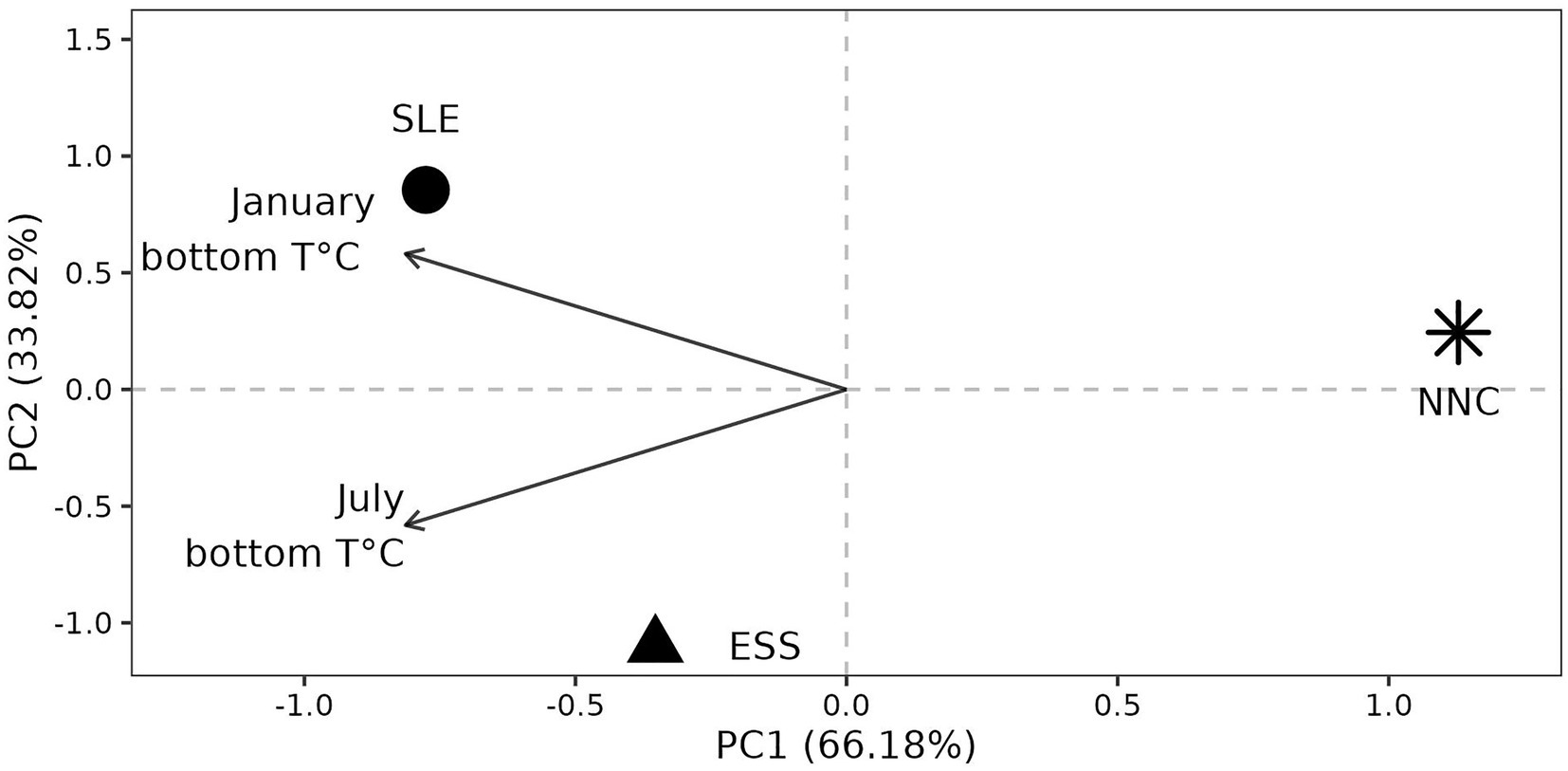
Figure 5. Environmental conditions at origin. Principal Component Analysis (PCA) using two monthly environmental variables that are not highly correlated (see methods for more details). ESS = Eastern Scotian Shelf, SLE = St. Lawrence Estuary, NNC = Northeast Newfoundland Coast, PC = Principal Component.
3.4.3. Temperature treatment and environmental conditions at origin affected Pandalus borealis plasticity but not genetic differentiation
We did not detect any tank effect on gene expression (partial RDA analysis; adjusted R2 = 0.047%; p = 0.429), and consequently tank identity was not taken into account in the subsequent analyzes. A variation partitioning analysis identified marginal effects of Temperature (adj. R2 = 2.98%; p = 0.001) and Environment (adj. R2 = 2.22%; p = 0.001) explaining transcript expression levels (Figure 6). However, genetic differentiation among origins did not explain transcript expression levels (Figure 6). In addition, model selection retained Temperature, Environment and the Temperature × Environment as terms explaining transcript expression (Table 1). The terms Genetics and the interaction between Temperature and Genetics were not selected.
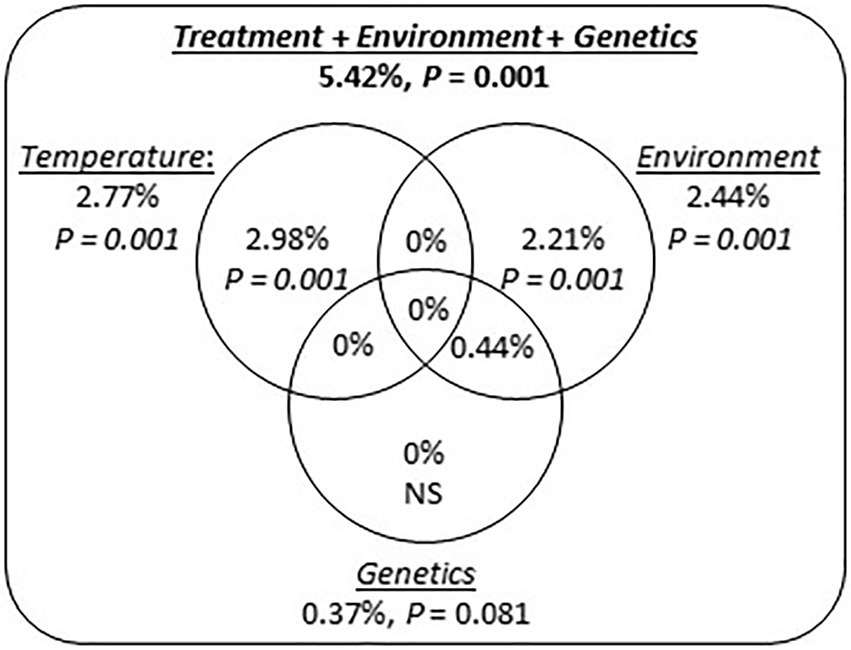
Figure 6. Factors influencing transcript expression in P. borealis. Venn diagram representing the proportion (adj. R2) of gene expression level explained by exposure temperature (Temperature), environmental conditions at origin (Environment) and population genetic differentiation (Genetics, i.e., pairwise FST between origins). Number outside circles refer to total percentage of variation explained by each underlined factor. Numbers inside circles indicate the marginal contribution to transcript expression by each factor. Percentages within intersections indicate shared contribution across different variables. Significance of total and marginal contribution to gene expression was tested by permutations test, using 999 randomizations.
4. Discussion
Understanding the molecular mechanisms underlying ectotherms’ responses to elevated temperature is paramount to improve our ability to predict their vulnerability to the ongoing global change. Genomic tools can help to improve prediction of organisms’ response to changing environments. In this study, we provides a first reference transcriptome of an important marine ectotherm species, Pandalus borealis. Using this new resource, we showed that changes in transcript levels are mainly explained by temperature exposure and environmental conditions at origin. The lack of genetics or genetics-by-temperature interaction effects on transcript expression levels suggested limited evolutionary potential in the species’ transcriptomic plasticity in response to temperature changes.
4.1. Temperature drove transcriptomic plasticity
Our results showed that P. borealis displays a high level of plasticity in gene expression with changing temperatures. The observed transcriptomic response supports the hypothesis that this species possesses an elevated degree of plasticity to cope with the temperature variation it could encounter throughout its life-time. Temperature-mediated gene expression changes have been reported in several ectotherm species (e.g., Gleason and Burton, 2015; Veilleux et al., 2015; Oostra et al., 2018; Sirovy et al., 2021; Valenza-Troubat et al., 2022). Our results are also in agreement with previous studies showing physiological responses of P. borealis to temperature, including changes in cellular energetic metabolism, and metabolic and growth rates (Chabot and Ouellet, 2005; Ouellet and Chabot, 2005; Arnberg et al., 2013; Dupont-Prinet et al., 2013; Chemel et al., 2020).
The linear transcriptomic response we reported across the three temperatures suggests that the northern shrimp can cope with increasing temperatures up to 10°C, at least within 30 days of exposure. This result confirms the suggestion that the temperature levels used for the plasticity assay were within the northern shrimp range of thermal tolerance (Chabot et al., 2013; Guscelli et al. submitted for review). However, the greater mortality observed at 10°C compared to 2°C (10%) supports the idea that the highest temperature level used in this study is close to the upper limit of thermal tolerance of P. borealis (Guscelli et al. submitted for review). Such incongruence in the responses to temperature between the mortality rates and the linearity of gene expression may be explained by the fact that only survivors could be analyzed for gene expression. Besides, other tissues than the abdominal muscle analyzed in this study, such as the gills and heart, may show thermal transcriptomic responses more closely associated to survival given their vital physiological functions (Buckley et al., 2006; Liu et al., 2013; Valenza-Troubat et al., 2022). The analysis of such tissues could extend our understanding of mechanisms involved in P. borealis responses to global changes.
We also reported changes in biological functions of differentially expressed transcripts (DETs) with increasing temperatures in P. borealis. Lipid catabolic activities have been shown to decrease with increasing temperatures in polar fishes (Pörtner, 2002). Similarly, we also observed catabolic activities decreasing with increasing temperature. For P. borealis, we identified protein catabolism at low temperatures which may be specific to this species (Supplementary Figure S1). We also observed increasing expression of genes associated to metabolic activities with increasing temperature, which supports previous observations of increased metabolic rate of P. borealis at higher temperatures (Ouellet and Chabot, 2005; Arnberg et al., 2013; Dupont-Prinet et al., 2013; Guscelli et al. submitted for review).
Our study also identified a molecular mechanism possibly involved in cold tolerance in P. borealis. Transcripts associated with threonine related activities decreased with increasing temperature. In Atlantic salmon Salmo salar (Linnaeus, 1758), threonine-type proteins were shown to be involved in low temperature conditioning (Silva et al., 2020). These proteins were also observed in response to cold treatment in the Pacific white shrimp P. vannamei (Huang et al., 2017), where threonine-type proteins are downregulated in the warmest temperatures, as for P. borealis.
4.2. Variation in gene expression was associated with environmental factors, but not genetic variation
The origin of northern shrimp was associated with contrasting transcriptomic patterns, with shrimp from NNC showing more distinctive gene expression patterns when compared to the SLE and ESS origins. Such difference could be due to acclimatization (environment) or local adaptation (genetics) effects. We used a model selection analysis with temperature, environmental conditions at origin, and genetic differentiation to disentangle acclimatization and adaptation effects on transcript expression levels. Our results showed that experimental temperature and environmental conditions at origin are the two factors explaining differences in transcript levels.
Local adaptation can translate into differential gene expression patterns in distinct populations. In this study, we confirmed the existence of different populations in P. borealis in northwest Atlantic by detecting significant genetic differentiation among origins. Our results also showed that shrimp from SLE display the smallest genetic diversity compared to other origins. Despite such genetic differences, we failed to detect any genetic effect on gene expression, either qualitatively with genetic diversity or quantitatively with genetic differentiation (variation partitioning and model selection analyzes). Our results suggest that local adaptation do not underly differential gene expression for shrimp from these three origins. Yet, single or a few loci might be associated with local adaptation and contribute to the geographic variation in gene expression (Whitehead and Crawford, 2006). This hypothesis is now under investigation in a distinct study.
The similarity in transcriptomic patterns and environmental conditions at origins observed in this study may suggest acclimation of shrimp to temperature at origins. However, the interpretation of these results is not straight forward. The variable developmental status of female shrimp was also highlighted with difference in molting rates across origins in this. The SLE shrimp were tested in early fall, when molting occurs in the field, whereas the ESS shrimp were tested in spring, close to hatching and before molting. In contrast, NNC shrimps were tested in winter and adult females are not molting during this season (DFO, 2012, 2021; Bourdages et al., 2022). Consequently, the effect of environmental conditions at origins also included difference in developmental status of female shrimp. Note that differences in transcript levels among shrimp from distinct origins also mirror those we report for molting rates. In addition, most gene functions involved in DETs across origins were associated with cell structures. Transcripts involved in actin and myosin production, cytoskeletal motor activity, and cuticle structural components were also upregulated in SLE and ESS. Such gene functions were previously associated to the molting process in shrimp (de Oliveira Cesar et al., 2006; Corteel et al., 2012; Zhang et al., 2019).
4.3. Limited potential of adaptation to global changes of northern shrimp
Ecologically divergent populations can evolve different transcriptomic plasticity, as observed in the redband trout Oncorhynchus mykiss gairdneri (Richardson, 1836) from desert and montane climates (Narum and Campbell, 2015), and in the threespine stickleback Gasterosteus aculeatus (Linnaeus, 1758) from marine and freshwater environments (Morris et al., 2014). However, we did not observed different thermal transcriptomic platicity in P. borealis among genetically different populations. This result indicates that this species did not evolve locally adapted transcriptomic plasticity in response to the temperature range tested. Furthermore, this lack of genetic variation underlying transcriptomic responses to changing temperature suggests a limited evolutionary potential of P. borealis thermal plasticity, at least at the transcriptional level. It is unclear to what extent this limited evolutionary potential of thermal plasticity at the transcriptomic level reflects a limited evolutionary potential for the plasticity of integrative traits such as growth and reproduction. However, plastic response of gene expression could be adaptive (Koch and Guillaume, 2020), and the evolution of plasticity for the transcriptional phenotypes could propagate across hierarchical levels of the organisms (from gene expression to integrative phenotypes), as observed in another organism (Leung et al., 2022). Our results would also suggest a limited evolutionary potential of integrative traits plasticity.
In conclusion, understanding the molecular mechanisms underpinning a species’ thermal plasticity and its vulnerability to global change drivers can ultimately help developing appropriate conservation and management plans, this being paramount also for species of commercial importance that supports the socio-economic development of coastal communities, including first nation ones. Our results show that individuals of P. borealis surviving 30 days exposure to elevated temperature are able to buffer the immediate impact of ongoing ocean warming by altering their gene expression. However, the lack of standing variation underlying phenotypic plasticity in response to temperature suggests a limited potential for the evolution of its plasticity. Our results emphasise its vulnerability under future global changes scenarios, as observed in other ectotherm organisms (Gunderson and Stillman, 2015; Sirovy et al., 2021). Consequently, the higher mortality reported at the highest temperature in this study also supports that the thermal niche of P. borealis will be progressively restricted to higher latitudes with the ongoing ocean warming (Hobday et al., 2016; Kleisner et al., 2017; Morley et al., 2018; McHenry et al., 2019). This prediction concurs with current decreases in abundance already observed at lower latitudes for this species, prediction also based on modeling (Barria et al. submitted for review) and other cold-adapted crustaceans (Neumann et al., 2013; Lenoir et al., 2020; Melo-Merino et al., 2020; Simões et al., 2021).
Data availability statement
The datasets presented in this study can be found in online repositories. The names of the repository/repositories and accession number(s) can be found below: https://www.ncbi.nlm.nih.gov/, PRJNA909194 and https://github.com/GenomicsMLI-DFO/PANOMICS_Transcripto_manuscript.
Ethics statement
The Canadian Council for Animal Care does not require that projects using invertebrates other than cephalopods be approved by an Animal Care Committee.
Author contributions
GP obtained the funding for the genomic analyzes. DC and PC obtained the funding for the experimental work. CL and GP conceived the tested hypothesis. EG, DC, and PC conceived, designed, and ran experiment. AB performed the bioinformatic analyzes of ddRADseq data. CL analyzed the transcriptomic data, prepared figures and tables, and wrote the original draft. All authors contributed to the article and approved the submitted version.
Funding
The experimental work was funded by: (i) an OURANOS grant (554023) to DC and PC; (ii) a DFO Strategic Program for Ecosystem-Based Research and Advice grant and an Aquatic Climate Change Adaptation Services Program grant to DC; (iii) a FIR UQAR grant, a Canada Foundation for Innovation (CFI) grant and a Natural Sciences and Engineering Research Council of Canada (NSERC) Discovery grants (RGPIN-2015-06500 and RGPIN-2020-05627) and a MITACS-Ouranos Accelerate grant, all granted to PC. EG is supported by a Fonds de Recherche du Québec - Nature et Technologies (FRQNT) scholarship (PBEEE, 289597) and a Réal-Decoste Ouranos scholarship (286109).
The genomic work was funded by: Genomics R&D Initiative (GRDI), Government of Canada.
Acknowledgments
We thank T. Hansen, J. Gagnon, and D. Picard for technical support during the experiments and the molecular work at Maurice Lamontagne Institute (DFO, Canada). We are grateful to G. Leveque (C3G) for the RNA-seq bioinformatic preprocessing and G. Cortial and L.-A. Dumoulin for RNA/DNA extractions (DFO, Canada). GP, EG, DC, and PC are working under the FRQ-NT funded research networks of excellence Québec-Océans or Ressources Aquatiques Québec.
Conflict of interest
As PC was a Topic Editor of this special issue, he did not participate in any form to editorial decision nor the editorial processing of this article.
The remaining authors declare that the research was conducted in the absence of any commercial or financial relationships that could be construed as a potential conflict of interest.
Publisher’s note
All claims expressed in this article are solely those of the authors and do not necessarily represent those of their affiliated organizations, or those of the publisher, the editors and the reviewers. Any product that may be evaluated in this article, or claim that may be made by its manufacturer, is not guaranteed or endorsed by the publisher.
Supplementary material
The Supplementary material for this article can be found online at: https://www.frontiersin.org/articles/10.3389/fevo.2023.1125134/full#supplementary-material
References
Abram, P. K., Boivin, G., Moiroux, J., and Brodeur, J. (2017). Behavioural effects of temperature on ectothermic animals: unifying thermal physiology and behavioural plasticity. Biol. Rev. 92, 1859–1876. doi: 10.1111/BRV.12312
Alexa, A., and Rahnenfuhrer, J. (2022). topGO: Enrichment Analysis for Gene Ontology. R Packag Version 2.0.
Allen, J. A. (1959). On the biology of Pandalus borealis Krøyer, with reference to a population off the Northumberland coast. J. Mar. Biol. Assoc. U. K. 38, 189–220. doi: 10.1017/S002531540001568X
Alley, R. B. (2000). Ice-core evidence of abrupt climate changes. Proc. Natl. Acad. Sci. U. S. A. 97, 1331–1334. doi: 10.1073/PNAS.97.4.1331/ASSET/F6F66AAD-E997-44E8-9C57-6F4F4C372A73/ASSETS/GRAPHIC/PQ0103809001.JPEG
Amarasekare, P., and Savage, V. (2012). A framework for elucidating the temperature dependence of fitness. Am. Nat. 179, 178–191. doi: 10.1086/663677/SUPPL_FILE/52973APE.PDF
Angilletta, M. J. (2009). Thermal Adaptation: A Theoretical and Empirical Synthesis. Oxford: Oxford University Press.
Apollonio, S., Stevenson, D. K., and Dunton, J. E. E. (1986). Effects of Temperature on the Biology of the Northern Shrimp, Pandalus borealis, in the Gulf of Maine. NOAA Technical Report NMFS; 42. Available at: https://repository.library.noaa.gov/view/noaa/23116 (Accessed August 4, 2022).
Arnberg, M., Calosi, P., Spicer, J. I., Tandberg, A. H. S., Nilsen, M., Westerlund, S., et al. (2013). Elevated temperature elicits greater effects than decreased pH on the development, feeding and metabolism of northern shrimp (Pandalus borealis) larvae. Mar. Biol. 160, 2037–2048. doi: 10.1007/S00227-012-2072-9/FIGURES/5
Ashander, J., Chevin, L. M., and Baskett, M. L. (2016). Predicting evolutionary rescue via evolving plasticity in stochastic environments. Proc. R. Soc. B Biol. Sci. 283:20161690. doi: 10.1098/RSPB.2016.1690
Aubin-Horth, N., and Renn, S. C. P. (2009). Genomic reaction norms: using integrative biology to understand molecular mechanisms of phenotypic plasticity. Mol. Ecol. 18, 3763–3780. doi: 10.1111/J.1365-294X.2009.04313.X
Blanchet, F. G., Legendre, P., and Borcard, D. (2008). Forward selection of explanatory variables. Ecology 89, 2623–2632. doi: 10.1890/07-0986.1
Bolger, A. M., Lohse, M., and Usadel, B. (2014). Trimmomatic: a flexible trimmer for Illumina sequence data. Bioinform. 30, 2114–2120.
Bonebrake, T. C., Brown, C. J., Bell, J. D., Blanchard, J. L., Chauvenet, A., Champion, C., et al. (2018). Managing consequences of climate-driven species redistribution requires integration of ecology, conservation and social science. Biol. Rev. 93, 284–305. doi: 10.1111/BRV.12344
Borcard, D., Legendre, P., and Drapeau, P. (1992). Partialling out the spatial component of ecological variation. Ecology 73, 1045–1055. doi: 10.2307/1940179
Bourdages, H., Roux, M.-J., Marquis, M.-C., Galbraith, P., and Isabel, L. (2022). Assessment of Northern Shrimp Stocks in the Estuary and Gulf of St. Lawrence in 2021: Commercial Fishery and Research Survey Data. DFO Canadian Science Advisory 2022/027.197.
Bradshaw, A. D. (1965). Evolutionary significance of phenotypic plasticity in plants. Adv. Genet. 13, 115–155. doi: 10.1016/S0065-2660(08)60048-6
Buckley, B. A., Gracey, A. Y., and Somero, G. N. (2006). The cellular response to heat stress in the goby Gillichthys mirabilis: a cDNA microarray and protein-level analysis. J. Exp. Biol. 209, 2660–2677. doi: 10.1242/JEB.02292
Catchen, J., Hohenlohe, P. A., Bassham, S., Amores, A., and Cresko, W. A. (2013). Stacks: an analysis tool set for population genomics. Mol. Ecol. 22, 3124–3140. doi: 10.1111/mec.12354
Chabot, D., Guénette, S., and Stortini, C. (2013). “A review of the physiological susceptibility of commercial species of fish and crustaceans of the Northwest Atlantic to changes in water temperature, dissolved oxygen, pH and salinity” in Climate Change Impacts, Vulnerabilities and Opportunities Analysis of the Marine Atlantic Basin. eds. N. L. Shackell, B. J. W. Greenan, P. Pepin, D. Chabot, and A. Warburton (Canadian Manuscript Report of Fisheries and Aquatic Sciences), 83–168.
Chabot, D., and Ouellet, P. (2005). Rearing Pandalus borealis larvae in the laboratory: II. Routine oxygen consumption, maximum oxygen consumption and metabolic scope at three temperatures. Mar. Biol. 147, 881–894. doi: 10.1007/s00227-005-1626-5
Chemel, M., Noisette, F., Chabot, D., Guscelli, E., Leclerc, L., and Calosi, P. (2020). Good news–bad news: combined ocean change drivers decrease survival but have no negative impact on nutritional value and organoleptic quality of the northern shrimp. Front. Mar. Sci. 7:611. doi: 10.3389/fmars.2020.00611
Chen, Y., Lun, A. T. L., Smyth, G. K., Burden, C. J., Ryan, D. P., Khang, T. F., et al. (2016). From reads to genes to pathways: differential expression analysis of RNA-Seq experiments using Rsubread and the edgeR quasi-likelihood pipeline. F1000 Res. 5:1438. doi: 10.12688/f1000research.8987.2
Colston-Nepali, L., Tigano, A., Boyle, B., and Friesen, V. (2019). Hybridization does not currently pose conservation concerns to murres in the Atlantic. Conserv. Genet. 20, 1465–1470. doi: 10.1007/s10592-019-01223-y
Corteel, M., Dantas-Lima, J. J., Wille, M., Alday-Sanz, V., Pensaert, M. B., Sorgeloos, P., et al. (2012). Moult cycle of laboratory-raised Penaeus (Litopenaeus) vannamei and P. monodon. Aquac. Int. 20, 13–18. doi: 10.1007/s10499-011-9437-9
Daoud, D., Chabot, D., Audet, C., and Lambert, Y. (2007). Temperature induced variation in oxygen consumption of juvenile and adult stages of the northern shrimp, Pandalus borealis. J. Exp. Mar. Bio. Ecol. 347, 30–40. doi: 10.1016/J.JEMBE.2007.02.013
Daoud, D., Lambert, Y., Audet, C., and Chabot, D. (2010). Size and temperature-dependent variations in intermolt duration and size increment at molt of northern shrimp, Pandalus borealis. Mar. Biol. 157, 2655–2666. doi: 10.1007/s00227-010-1526-1
de Oliveira Cesar, J. R., Zhao, B., Malecha, S., Ako, H., and Yang, J. (2006). Morphological and biochemical changes in the muscle of the marine shrimp Litopenaeus vannamei during the molt cycle. Aquaculture 261, 688–694. doi: 10.1016/J.AQUACULTURE.2006.08.003
De Wit, P., Dupont, S., and Thor, P. (2016). Selection on oxidative phosphorylation and ribosomal structure as a multigenerational response to ocean acidification in the common copepod Pseudocalanus acuspes. Evol. Appl. 9, 1112–1123. doi: 10.1111/EVA.12335
Dey, S., Proulx, S. R., and Teotónio, H. (2016). Adaptation to temporally fluctuating environments by the evolution of maternal effects. PLoS Biol. 14:e1002388. doi: 10.1371/JOURNAL.PBIO.1002388
DFO. (2012). Assessment of Northern Shrimp on the Eastern Scotian Shelf (SFAs 13-15). DFO Canadian Science Advisory Secretariat Maritimes Region Science Advisory Report, No. 2012/114.
DFO. (2021). An Assessment of Northern Shrimp (Pandalus borealis) in Shrimp Fishing Areas 4–6 and of Striped Shrimp (Pandalus montagui) in Shrimp Fishing Area 4 in 2020. DFO Canadian Science Advisory Secretariat Maritimes Region Science Advisory Report, No. 2021/049.
DFO. (2022). Assessment of Northern Shrimp Stocks in the Estuary and Gulf of St. Lawrence in 2021. DFO Canadian Science Advisory Secretariat Maritimes Region Science Advisory Report, No. 2022/006.
Dupont-Prinet, A., Pillet, M., Chabot, D., Hansen, T., Tremblay, R., and Audet, C. (2013). Northern shrimp (Pandalus borealis) oxygen consumption and metabolic enzyme activities are severely constrained by hypoxia in the estuary and gulf of St. Lawrence J. Exp. Mar. Bio. Ecol. 448, 298–307. doi: 10.1016/J.JEMBE.2013.07.019
Fischer, J., Schott, F. A., and Dengler, M. (2004). Boundary circulation at the exit of the Labrador Sea. J. Phys. Oceanogr. 34, 1548–1570. doi: 10.1175/1520-0485(2004)034<1548:BCATEO>2.0.CO;2
Garcia, E. G. (2007). The northern shrimp (Pandalus borealis) offshore fishery in the Northeast Atlantic. Adv. Mar. Biol. 52, 147–266. doi: 10.1016/S0065-2881(06)52002-4
Gavrilets, S., and Scheiner, S. M. (1993). The genetics of phenotypic plasticity. V. Evolution of reaction norm shape. J. Evol. Biol. 6, 31–48. doi: 10.1046/J.1420-9101.1993.6010031.X
Gerken, A. R., Eller, O. C., Hahn, D. A., and Morgan, T. J. (2015). Constraints, independence, and evolution of thermal plasticity: probing genetic architecture of long–and short-term thermal acclimation. Proc. Natl. Acad. Sci. 112, 4399–4404. doi: 10.1073/PNAS.1503456112
Ghalambor, C. K., McKay, J. K., Carroll, S. P., and Reznick, D. N. (2007). Adaptive versus non-adaptive phenotypic plasticity and the potential for contemporary adaptation in new environments. Funct. Ecol. 21, 394–407. doi: 10.1111/J.1365-2435.2007.01283.X
Gleason, L. U., and Burton, R. S. (2015). RNA-seq reveals regional differences in transcriptome response to heat stress in the marine snail Chlorostoma funebralis. Mol. Ecol. 24, 610–627. doi: 10.1111/MEC.13047
Gruber, B., Unmack, P. J., Berry, O. F., and Georges, A. (2018). Dartr: an r package to facilitate analysis of SNP data generated from reduced representation genome sequencing. Mol. Ecol. Resour. 18, 691–699. doi: 10.1111/1755-0998.12745
Gunderson, A. R., and Stillman, J. H. (2015). Plasticity in thermal tolerance has limited potential to buffer ectotherms from global warming. Proc. R. Soc. B Biol. Sci. 282:20150401. doi: 10.1098/RSPB.2015.0401
Haas, B. J., Papanicolaou, A., Yassour, M., Grabherr, M., Blood, P. D., Bowden, J., et al. (2013). De novo transcript sequence reconstruction from RNA-seq using the trinity platform for reference generation and analysis. Nat. Protoc. 8, 1494–1512. doi: 10.1038/nprot.2013.084
Hansen, T. F. (2006). The evolution of genetic architecture. Annu. Rev. Ecol. Evol. Syst. 37, 123–157. doi: 10.1146/annurev.ecolsys.37.091305.110224
Haynes, E. B., and Wigley, R. L. (1969). Biology of the northern shrimp, Pandalus borealis, in the Gulf of Maine. Trans. Am. Fish. Soc. 98, 60–76. doi: 10.1577/1548-8659
Hobday, A. J., Alexander, L. V., Perkins, S. E., Smale, D. A., Straub, S. C., Oliver, E. C. J., et al. (2016). A hierarchical approach to defining marine heatwaves. Prog. Oceanogr. 141, 227–238. doi: 10.1016/J.POCEAN.2015.12.014
Huang, W., Ren, C., Li, H., Huo, D., Wang, Y., Jiang, X., et al. (2017). Transcriptomic analyses on muscle tissues of Litopenaeus vannamei provide the first profile insight into the response to low temperature stress. PLoS One 12:e0178604. doi: 10.1371/JOURNAL.PONE.0178604
Huey, R. B., Kearney, M. R., Krockenberger, A., Holtum, J. A. M., Jess, M., and Williams, S. E. (2012). Predicting organismal vulnerability to climate warming: roles of behaviour, physiology and adaptation. Philos. Trans. R. Soc. B Biol. Sci. 367, 1665–1679. doi: 10.1098/RSTB.2012.0005
Huey, R. B., and Stevenson, R. D. (1979). Integrating thermal physiology and ecology of ectotherms: a discussion of approaches. Integr. Comp. Biol. 19, 357–366. doi: 10.1093/ICB/19.1.357
Hughes, A. R., Inouye, B. D., Johnson, M. T. J., Underwood, N., and Vellend, M. (2008). Ecological consequences of genetic diversity. Ecol. Lett. 11, 609–623. doi: 10.1111/J.1461-0248.2008.01179.X
IPCC. (2022). Climate change 2022: Impacts Adaptation and Vulnerability. Geneva, Szwitzerland: IPCC.
Kamvar, Z. N., Tabima, J. F., and Gr̈unwald, N. J. (2014). Poppr: an R package for genetic analysis of populations with clonal, partially clonal, and/or sexual reproduction. PeerJ 2014, 1–14. doi: 10.7717/PEERJ.281/TABLE-6
Kelly, M. (2019). Adaptation to climate change through genetic accommodation and assimilation of plastic phenotypes. Philos. Trans. R. Soc. B 374:20180176. doi: 10.1098/RSTB.2018.0176
Kleisner, K. M., Fogarty, M. J., McGee, S., Hare, J. A., Moret, S., Perretti, C. T., et al. (2017). Marine species distribution shifts on the U.S. northeast continental shelf under continued ocean warming. Prog. Oceanogr. 153, 24–36. doi: 10.1016/J.POCEAN.2017.04.001
Koch, E. L., and Guillaume, F. (2020). Additive and mostly adaptive plastic responses of gene expression to multiple stress in Tribolium castaneum. PLoS Genet. 16:e1008768. doi: 10.1371/journal.pgen.1008768
Koeller, P. A. (2000). Relative importance of abiotic and biotic factors to the Management of the Northern Shrimp (Pandalus Borealis) fishery on the Scotian shelf. J. Northwest Atl. Fish. Sci. 27, 21–33. doi: 10.2960/J.v27.a3
Koeller, P., Fuentes-Yaco, C., Platt, T., Sathyendranath, S., Richards, A., Ouellet, P., et al. (2009). Basin-scale coherence in phenology of shrimps and phytoplankton in the North Atlantic Ocean. Science 80, 791–793. doi: 10.1126/SCIENCE.1170987/SUPPL_FILE/KOELLER.SOM.PDF
Landy, J. A., Oschmann, A., Munch, S. B., and Walsh, M. R. (2020). Ancestral genetic variation in phenotypic plasticity underlies rapid evolutionary changes in resurrected populations of waterfleas. Proc. Natl. Acad. Sci. U. S. A. 117, 32535–32544. doi: 10.1073/pnas.2006581117
Langfelder, P., and Horvath, S. (2008). WGCNA: an R package for weighted correlation network analysis. BMC Bioinformatics 9, 1–13. doi: 10.1186/1471-2105-9-559/FIGURES/4
Lavoie, D., Lambert, N., Rousseau, S., Dumas, J., Chassé, J., Long, Z., et al. (2020). Projections of Future Physical and Biogeochemical Conditions in the Gulf of St. Lawrence, on the Scotian Shelf and in the Gulf of Maine. Canadian Technical Report of Hydrography and Ocean Sciences.
Leder, E. H., McCairns, R. J. S., Leinonen, T., Cano, J. M., Viitaniemi, H. M., Nikinmaa, M., et al. (2015). The evolution and adaptive potential of transcriptional variation in sticklebacks—signatures of selection and widespread heritability. Mol. Biol. Evol. 32, 674–689. doi: 10.1093/MOLBEV/MSU328
Legendre, P. (2008). Studying beta diversity: ecological variation partitioning by multiple regression and canonical analysis. J. Plant Ecol. 1, 3–8. doi: 10.1093/jpe/rtm001
Lenoir, J., Bertrand, R., Comte, L., Bourgeaud, L., Hattab, T., Murienne, J., et al. (2020, 2020). Species better track climate warming in the oceans than on land. Nat. Ecol. Evol. 48, 1044–1059. doi: 10.1038/s41559-020-1198-2
Leung, C., Grulois, D., Quadrana, L., and Chevin, L. M. (2022). The molecular basis of phenotypic plasticity evolves in response to environmental predictability. bioRxiv 2022:514467. doi: 10.1101/2022.10.31.514467
Leung, C., Rescan, M., Grulois, D., and Chevin, L. M. (2020). Reduced phenotypic plasticity evolves in less predictable environments. Ecol. Lett. 23, 1664–1672. doi: 10.1111/ELE.13598
Levins, R. (1963). Theory of fitness in a heterogeneous environment. II. Developmental flexibility and niche selection. Am. Nat. 97, 75–90. doi: 10.1086/282258
Li, B., and Dewey, C. N. (2011). RSEM: accurate transcript quantification from RNA-Seq data with or without a reference genome. BMC Bioinformatics 12, 1–16. doi: 10.1186/1471-2105-12-323
Lima, F. P., Ribeiro, P. A., Queiroz, N., Hawkins, S. J., and Santos, A. M. (2007). Do distributional shifts of northern and southern species of algae match the warming pattern? Glob. Chang. Biol. 13, 2592–2604. doi: 10.1111/J.1365-2486.2007.01451.X
Liu, S., Wang, X., Sun, F., Zhang, J., Feng, J., Liu, H., et al. (2013). RNA-Seq reveals expression signatures of genes involved in oxygen transport, protein synthesis, folding, and degradation in response to heat stress in catfish. Physiol. Genomics 45, 462–476. doi: 10.1152/PHYSIOLGENOMICS.00026.2013/ASSET/IMAGES/LARGE/ZH70121338400004.JPEG
Logan, C. A., Buckley, B. A., Podrabsky, J. E., Stillman, J. H., and Tomanek, L. (2015). Transcriptomic responses to environmental temperature in eurythermal and stenothermal fishes. J. Exp. Biol. 218, 1915–1924. doi: 10.1242/JEB.114397
Love, M. I., Huber, W., and Anders, S. (2014). Moderated estimation of fold change and dispersion for RNA-seq data with DESeq2. Genome Biol. 15, 1–21. doi: 10.1186/S13059-014-0550-8/FIGURES/9
Madeira, D., Araújo, J. E., Madeira, C., Mendonça, V., Vitorino, R., Vinagre, C., et al. (2020). Seasonal proteome variation in intertidal shrimps under a natural setting: connecting molecular networks with environmental fluctuations. Sci. Total Environ. 703:134957. doi: 10.1016/j.scitotenv.2019.134957
McHenry, J., Welch, H., Lester, S. E., and Saba, V. (2019). Projecting marine species range shifts from only temperature can mask climate vulnerability. Glob. Chang. Biol. 25, 4208–4221. doi: 10.1111/GCB.14828
Melo-Merino, S. M., Reyes-Bonilla, H., and Lira-Noriega, A. (2020). Ecological niche models and species distribution models in marine environments: a literature review and spatial analysis of evidence. Ecol. Model. 415:108837. doi: 10.1016/J.ECOLMODEL.2019.108837
Morley, J. W., Selden, R. L., Latour, R. J., Frölicher, T. L., Seagraves, R. J., and Pinsky, M. L. (2018). Projecting shifts in thermal habitat for 686 species on the north American continental shelf. PLoS One 13:e0196127. doi: 10.1371/JOURNAL.PONE.0196127
Morris, M. R., Richard, R., Leder, E. H., Barrett, R. D., Aubin‐Horth, N., and Rogers, S. M. (2014). Gene expression plasticity evolves in response to colonization of freshwater lakes in threespine stickleback. Mol. Ecol. 23, 3226–3240.
Mucci, A., Levasseur, M., Gratton, Y., Martias, C., Scarratt, M., Gilbert, D., et al. (2018). Tidally induced variations of pH at the head of the Laurentian Channel. Can. J. Fish. Aquat. Sci. 75, 1128–1141. doi: 10.1139/cjfas-2017-0007
Mucci, A., Starr, M., Gilbert, D., and Sundby, B. (2011). Acidification of lower St. Lawrence estuary bottom waters. Atmosphere-Ocean 49, 206–218. doi: 10.1080/07055900.2011.599265
Narum, S. R., and Campbell, N. R. (2015). Transcriptomic response to heat stress among ecologically divergent populations of redband trout. BMC. Genom. 16, 1–12.
Neumann, H., De Boois, I., Kröncke, I., and Reiss, H. (2013). Climate change facilitated range expansion of the non-native angular crab Goneplax rhomboides into the North Sea. Mar. Ecol. Prog. Ser. 484, 143–153. doi: 10.3354/MEPS10299
Oksanen, J., Simpson, G. L., Blanchet, F. G., Kindt, R., Legendre, P., Minchin, P. R., et al. (2022). Egan: Community Ecology Package Version 2.6-2 from CRAN. Available at: https://rdrr.io/cran/vegan/ (Accessed July 29, 2022).
Oostra, V., Saastamoinen, M., Zwaan, B. J., and Wheat, C. W. (2018). Strong phenotypic plasticity limits potential for evolutionary responses to climate change. Nat. Commun. 91, 1–11. doi: 10.1038/s41467-018-03384-9
Orr, D., and Sullivan, D. (2013). The February 2013 Assessment of Northern Shrimp (Pandalus borealis) off Labrador and Northeastern Newfoundland. DFO Canadian Science Advisory Secretariat Research Document, 2013/055. p. 144.
Ouellet, P., and Chabot, D. (2005). Rearing Pandalus borealis (Krøyer) larvae in the laboratory: I. development and growth at three temperatures. Mar. Biol. 147, 869–880. doi: 10.1007/S00227-005-1625-6/FIGURES/10
Paaijmans, K. P., Heinig, R. L., Seliga, R. A., Blanford, J. I., Blanford, S., Murdock, C. C., et al. (2013). Temperature variation makes ectotherms more sensitive to climate change. Glob. Chang. Biol. 19, 2373–2380. doi: 10.1111/GCB.12240
Pavey, S. A., Collin, H., Nosil, P., and Rogers, S. M. (2010). The role of gene expression in ecological speciation. Ann. N. Y. Acad. Sci. 1206, 110–129. doi: 10.1111/J.1749-6632.2010.05765.X
Peres-Neto, P. R., Legendre, P., Dray, S., and Borcard, D. (2006). Variation partitioning of species data matrices: estimation and comparison of fractions. Ecology 87, 2614–2625. doi: 10.1890/0012-9658(2006)87[2614:VPOSDM]2.0.CO;2
Perry, A. L., Low, P. J., Ellis, J. R., and Reynolds, J. D. (2005). Ecology: climate change and distribution shifts in marine fishes. Science 80, 1912–1915. doi: 10.1126/SCIENCE.1111322/SUPPL_FILE/PERRY.SOM.REVISED.PDF
Pinsky, M. L., Eikeset, A. M., McCauley, D. J., Payne, J. L., and Sunday, J. M. (2019). Greater vulnerability to warming of marine versus terrestrial ectotherms. Nature 569, 108–111. doi: 10.1038/s41586-019-1132-4
Poland, J. A., Brown, P. J., Sorrells, M. E., and Jannink, J. L. (2012). Development of high-density genetic maps for barley and Wheat using a novel two-enzyme genotyping-by-sequencing approach. PLoS One 7:e32253. doi: 10.1371/JOURNAL.PONE.0032253
Pörtner, H. O. (2002). Physiological basis of temperature-dependent biogeography: trade-offs in muscle design and performance in polar ectotherms. J. Exp. Biol. 205, 2217–2230. doi: 10.1242/JEB.205.15.2217
R Core Team. (2020). R: A Language and Environment for Statistical Computing. Vienna, Austria: R Foundation for Statistical Computing.
Rahmstorf, S., and Coumou, D. (2011). Increase of extreme events in a warming world. Proc. Natl. Acad. Sci. U. S. A. 108, 17905–17909. doi: 10.1073/PNAS.1101766108/ASSET/45E632EF-D52B-4B90-8492-5D7B773D3028/ASSETS/GRAPHIC/PNAS.1101766108EQ10.JPEG
Reed, T. E., Waples, R. S., Schindler, D. E., Hard, J. J., and Kinnison, M. T. (2010). Phenotypic plasticity and population viability: the importance of environmental predictability. Proc. R. Soc. B Biol. Sci. 277, 3391–3400. doi: 10.1098/rspb.2010.0771
Rescan, M., Grulois, D., Ortega-Aboud, E., and Chevin, L. M. (2020). Phenotypic memory drives population growth and extinction risk in a noisy environment. Nat. Ecol. Evol. 42, 193–201. doi: 10.1038/s41559-019-1089-6
Richards, R. A. (2012). Phenological shifts in hatch timing of northern shrimp Pandalus borealis. Mar. Ecol. Prog. Ser. 456, 149–158. doi: 10.3354/MEPS09717
Richards, R. A., and Hunter, M. (2021). Northern shrimp Pandalus borealis population collapse linked to climate-driven shifts in predator distribution. PLoS One 16:e0253914. doi: 10.1371/JOURNAL.PONE.0253914
Rochette, N. C., Rivera-Colón, A. G., and Catchen, J. M. (2019). Stacks 2: analytical methods for paired-end sequencing improve RADseq-based population genomics. Mol. Ecol. 28, 4737–4754. doi: 10.1111/mec.15253
Román-Palacios, C., and Wiens, J. J. (2020). Recent responses to climate change reveal the drivers of species extinction and survival. Proc. Natl. Acad. Sci. U. S. A. 117, 4211–4217. doi: 10.1073/pnas.1913007117
Rosa, R., Lopes, A. R., Pimentel, M., Faleiro, F., Baptista, M., Trübenbach, K., et al. (2014). Ocean cleaning stations under a changing climate: biological responses of tropical and temperate fish-cleaner shrimp to global warming. Glob. Chang. Biol. 20, 3068–3079. doi: 10.1111/gcb.12621
Scheiner, S. M., and Holt, R. D. (2012). The genetics of phenotypic plasticity. X. Variation versus uncertainty. Ecol. Evol. 2, 751–767. doi: 10.1002/ece3.217
Scheiner, S. M., and Yampolsky, L. Y. (1998). The evolution of Daphnia pulex in a temporally varying environment. Genet. Res. 72, 25–37. doi: 10.1017/S0016672398003322
Seebacher, F., White, C. R., and Franklin, C. E. (2014). Physiological plasticity increases resilience of ectothermic animals to climate change. Nat. Clim. Chang 51, 61–66. doi: 10.1038/nclimate2457
Shumway, S. E., Perkins, H. C., Schick, D. F., and Stickney, A. P. (1985). Synopsis of Biological Data on the Pink Shrimp, Pandalus borealis Krøyer, 1838. FAO Fisheries Synopsis No. 144, p. 57.
Silva, A. M. M., Ige, T., Goonasekara, C. L., and Heeley, D. H. (2020). Threonine-77 is a determinant of the Low-temperature conditioning of the Most abundant isoform of tropomyosin in Atlantic Salmon. Biochemistry 59, 2859–2869. doi: 10.1021/ACS.BIOCHEM.0C00416/ASSET/IMAGES/LARGE/BI0C00416_0006.JPEG
Simões, M. V. P., Saeedi, H., Cobos, M. E., and Brandt, A. (2021). Environmental matching reveals non-uniform range-shift patterns in benthic marine Crustacea. Clim. Chang. 168, 1–20. doi: 10.1007/S10584-021-03240-8/FIGURES/3
Sirovy, K. A., Johnson, K. M., Casas, S. M., La Peyre, J. F., and Kelly, M. W. (2021). Lack of genotype-by-environment interaction suggests limited potential for evolutionary changes in plasticity in the eastern oyster, Crassostrea virginica. Mol. Ecol. 30, 5721–5734. doi: 10.1111/MEC.16156
Sokal, R. R., and Rohlf, F. J. (1995). “Biometry: the principles and practice of statistics” in Biological Research. ed. W. H. Freeman. 3rd ed (New York: WH Freeman and Company)
Somero, G. N. (2005). Linking biogeography to physiology: evolutionary and acclimatory adjustments of thermal limits. Front. Zool. 2, 1–9. doi: 10.1186/1742-9994-2-1/FIGURES/3
Somero, G. N. (2010). The physiology of climate change: how potentials for acclimatization and genetic adaptation will determine ‘winners’ and ‘losers.’. J. Exp. Biol. 213, 912–920. doi: 10.1242/JEB.037473
Stanley, R. R. E., DiBacco, C., Lowen, B., Beiko, R. G., Jeffery, N. W., Van Wyngaarden, M., et al. (2018). A climate-associated multispecies cryptic cline in the Northwest Atlantic. Sci. Adv. 4:eaaq0929. doi: 10.1126/sciadv.aaq0929
Stevenson, R. D. (1985). The relative importance of behavioral and physiological adjustments controlling body temperature in terrestrial ectotherms. Am. Nat. 126, 362–386. doi: 10.1086/284423
Tufto, J. (2015). Genetic evolution, plasticity, and bet-hedging as adaptive responses to temporally autocorrelated fluctuating selection: a quantitative genetic model. Evolution 69, 2034–2049. doi: 10.1111/evo.12716
Valenza-Troubat, N., Davy, M., Storey, R., Wylie, M. J., Hilario, E., Ritchie, P., et al. (2022). Differential expression analyses reveal extensive transcriptional plasticity induced by temperature in New Zealand silver trevally (Pseudocaranx georgianus). Evol. Appl. 15, 237–248. doi: 10.1111/EVA.13332
Veilleux, H. D., Ryu, T., Donelson, J. M., Van Herwerden, L., Seridi, L., Ghosheh, Y., et al. (2015). Molecular processes of transgenerational acclimation to a warming ocean. Nat. Clim. Chang. 512, 1074–1078. doi: 10.1038/nclimate2724
Waldvogel, A. M., Feldmeyer, B., Rolshausen, G., Exposito-Alonso, M., Rellstab, C., Kofler, R., et al. (2020). Evolutionary genomics can improve prediction of species’ responses to climate change. Evol. Lett. 4, 4–18. doi: 10.1002/EVL3.154
Wang, Z., Lu, Y., Greenan, B., Brickman, D., and DeTracey, B. (2018). BNAM: An Eddy Resolving North Atlantic Ocean Model to Support Ocean Monitoring. Canadian Technical Report of Hydrography and Ocean Sciences 327, p. 18.
Whitehead, A., and Crawford, D. L. (2006). Neutral and adaptive variation in gene expression. Proc. Natl. Acad. Sci. U. S. A. 103, 5425–5430. doi: 10.1073/PNAS.0507648103/SUPPL_FILE/07648TABLE2.XLS
Keywords: phenotypic plasticity, climate change, ocean warming, crustacean, decapod, fisheries, RNA-seq
Citation: Leung C, Guscelli E, Chabot D, Bourret A, Calosi P and Parent GJ (2023) The lack of genetic variation underlying thermal transcriptomic plasticity suggests limited adaptability of the Northern shrimp, Pandalus borealis. Front. Ecol. Evol. 11:1125134. doi: 10.3389/fevo.2023.1125134
Edited by:
Vera Maria Fonseca Almeida-Val, National Institute of Amazonian Research (INPA), BrazilReviewed by:
Richelle Li Tanner, Chapman University, United StatesLuciana Fé, Fundação de Vigilância em Saúde–Dra. Rosemary Costa Pinto (FVS-RCP), Brazil
Copyright © 2023 Leung, Guscelli, Chabot, Bourret, Calosi and Parent. This is an open-access article distributed under the terms of the Creative Commons Attribution License (CC BY). The use, distribution or reproduction in other forums is permitted, provided the original author(s) and the copyright owner(s) are credited and that the original publication in this journal is cited, in accordance with accepted academic practice. No use, distribution or reproduction is permitted which does not comply with these terms.
*Correspondence: Christelle Leung, christelle.leung@dfo-mpo.gc.ca; Geneviève J. Parent, geneviève.parent@dfo-mpo.gc.ca