- 1Laboratório de Ecologia, Manejo e Conservação de Fauna Silvestre, Departamento de Ciências Florestais, Escola Superior de Agricultura “Luiz de Queiroz”, Universidade de São Paulo, Piracicaba, Brazil
- 2Núcleo de Ecologia de Rodovias e Ferrovias, Departamento de Ecologia, Universidade Federal do Rio Grande do Sul, Porto Alegre, Brazil
- 3Programa USPSusten – Superintendência de Gestão Ambiental, Universidade de São Paulo, São Paulo, Brazil
- 4Programa de Pós-Graduação em Ecologia, Universidade Federal do Rio Grande do Sul, Porto Alegre, Brazil
- 5PRECISA Consultoria Ambiental LTDA, Porto Alegre, Brazil
Roads cause biodiversity loss and the effects of wildlife-vehicle collisions may ripple from individuals and populations to ecosystem functioning. Amphibians are threatened worldwide and, despite being particularly prone to roadkill impacts, they are often neglected in assessments. Here, we develop a sampling and analytical framework for spatially prioritizing mitigation actions for anuran amphibian roadkills based on fatality estimation and landscape conversion. The framework is composed of the six following steps: (1) pre-selection of segments to survey using the wetland coverage in the surroundings and the presence of roadkills of aquatic reptiles as a proxy for wet areas; (2) spatiotemporally replicated counts with a dependent double-observer protocol, that is, each segment is sampled multiple times by two pairs of people on foot; (3) extraction of covariates hypothesized to affect spatial and temporal variation in roadkill rates and persistence; (4) hierarchical open-population N-mixture modelling to estimate population dynamics parameters, which accounts for imperfect detection and spatiotemporal heterogeneity in removal, detection, and roadkill rates, and explicitly estimates carcass entries per time interval. (5) Assessment of land cover transition to infer landscape stability; and (6) prioritization of segments based on higher fatality rates and lower landscape conversion rates. We estimated a mean of 136 (95%CrI = 130–142) anurans roadkill per km per day in the 50 sample sites selected. The initial number of carcasses had a positive relationship with the percentage occupied by wetlands and a negative association with the percentage occupied by urban areas. The number of entrant carcass per interval was higher in the presence of rainfall and had a positive association with the wetlands cover. Carcass persistence probability was higher at night and lower in sites with high traffic volume. Ten segments (~1% of road extension) were prioritized using the median as threshold for fatality estimates and landscape conversion. It is urgent to appropriately evaluate the number of amphibians roadkilled aiming to plan and implement mitigation measures specifically designed for these small animals. Our approach accounts for feasibility (focused on sites with greater relevance), robustness (considering imperfect detection), and steadiness (less prone to loss of effectiveness due to landscape dynamics).
Introduction
Road infrastructures are an important source of biodiversity loss and are spreading across the globe (Meijer et al., 2018; Wenz et al., 2020). Besides the increase in environmental degradation and the decrease in ecological connectivity, direct removal of individuals by roadkill can be a major cause of local population decline (Fahrig and Rytwinski, 2009).Road effects on wild populations can ripple to other levels of ecological organization, affecting ecosystem functioning (van der Ree et al., 2015). To mitigate efficiently the negative impacts of roads on wildlife, sound knowledge on where deaths are concentrated is fundamental (Gunson and Teixeira, 2015 but see Teixeira et al., 2017), as well as accounting for landscape stability to ensure that proposed measures are long-term lasting (Zeller et al., 2020). Generating such high-quality information can be challenging given the usually large extent of road networks, scarce time and financial resources available for field work.
It is possible to assess roadkill patterns based on road features, landscape characteristics, and species occurrence (Patrick et al., 2012; Girardet et al., 2015; Visintin et al., 2016). Nevertheless, probably the best information to assess where roadkills concentrate along a road is still by estimating fatalities based on observation/counts of carcasses. However, fatality estimations using raw counts can be biased, as observers might not detect all available carcasses. Moreover, removal of carcass from the road by scavengers or traffic may also be an important factor affecting estimates, especially when smaller animals are the study target (Santos et al., 2011; Villegas-Patraca et al., 2012; Barrientos et al., 2018; Schwartz et al., 2018). Not properly addressing these sources of error (detection and removals) in fatality assessments might produce biased roadkill estimations. As both errors may vary in space and time, their spatiotemporal heterogeneity should also be accounted for. If such aspects are not assessed, mitigation actions may be proposed at less effective sites.
Usual approaches to estimate wildlife fatalities at man-made infrastructures, when considering the sources of error, commonly use ad-hoc formulas based on detection rates and carcass removal trials from experiments or from comparisons with an assumed perfect-detection method (e.g., surveys on foot; Simonis et al., 2018; Teixeira et al., 2013). However, there have been claims for the application of process-based approaches while accounting for imperfect detection in carcass observations (e.g., wind farms, Péron et al., 2013; roads, Guinard et al., 2012). Such process-based approaches, typically based on open population capture-recapture models, tend to represent more accurately the dynamics of carcasses entering and leaving the sampled road segment (Guinard et al., 2015; Péron, 2018).
Even when roadkill hotspots are robustly estimated, mitigation measures can result in resource wasting if populations are locally affected due to other anthropic pressures. The effectiveness of an installed mitigation structure could rapidly decline in regions where anthropogenic landscape changes are more pronounced, causing shifts in the distribution and movement patterns of a species along a road. Hence, habitat stability is an important aspect to be included in the spatial prioritization of mitigation structures, especially when planning long-term measures (Clevenger and Ford, 2010; Zeller et al., 2020).
While often neglected in road fatality assessments, amphibians are one of the most affected taxa by roadkills, representing more than 90% of the fatalities in some cases (Fahrig et al., 1995; Glista et al., 2008; Coelho et al., 2012; Silva et al., 2021). Amphibians are the most threatened vertebrate group with 41% of the species at risk of extinction (IUCN, 2021). Their life cycle, with most species presenting an aquatic larval phase, imposes to adults and juveniles challenges in arriving and leaving water bodies every reproductive season. This can increase road encounter probability by amphibians and the potential negative population-level effects, especially for anurans species with lower reproductive rates, smaller body sizes, and younger ages at sexual maturity (Rytwinski and Fahrig, 2012). Moreover, their small size results in low detection and fast removal (Teixeira et al., 2013; Pereira et al., 2018) demanding sampling on foot and with short time intervals between occasions, which would represent a challenge for the survey of extensive road networks.
Here, we develop a framework for prioritizing road segments for amphibian roadkill mitigation based on fatality estimation and landscape transition (Figure 1). Our framework is composed of the six following steps:
1. Pre-selection of road segments with higher potential occurrence of amphibians;
2. Spatiotemporally replicated carcass count surveys by dependent double observers on foot;
3. Extraction of covariates that may influence the spatial and temporal variation in roadkill rates and persistence;
4. Fatality estimation with hierarchical modelling, taking into account imperfect detection and spatiotemporal heterogeneity in persistence and roadkill rates;
5. Landscape conversion using a transition rate from native to non-native land covers;
6. Road segment prioritization using higher roadkill rates and lower landscape transition rates as criteria.
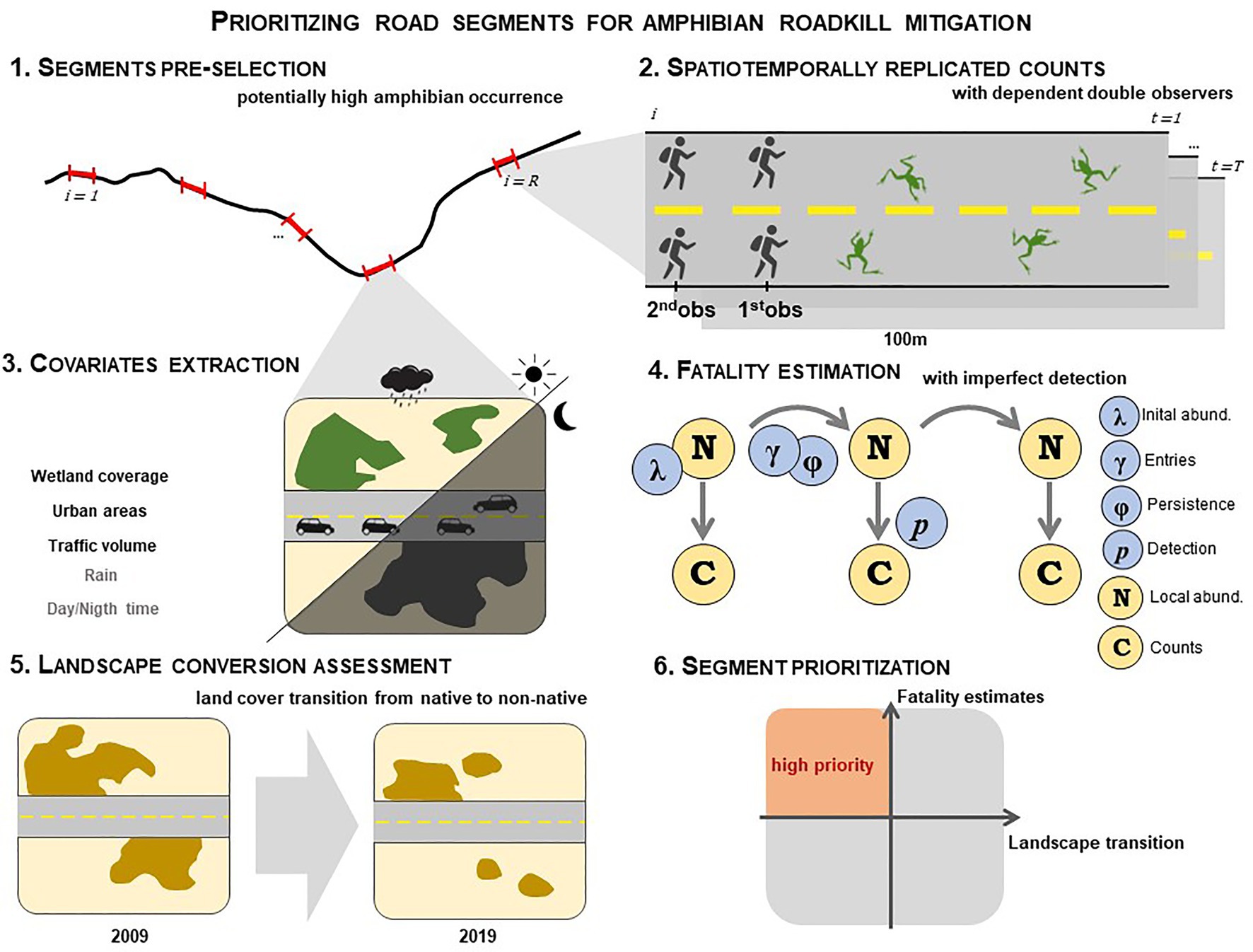
Figure 1. Framework to spatially prioritize road segments for amphibian roadkill mitigation based on fatality estimation and landscape stability. (1) R road sites of 100 m length, where amphibian occurrence is expected to be higher, are pre-selected for sampling; (2) Each site i is surveyed T times searching for carcasses using a dependent double-observer protocol; (3) Covariates hypothesized to influence spatial and temporal variation in roadkill rates and persistence are compiled (black and gray letters indicate spatial and temporal covariates respectively); (4) Carcass counts C and covariates are fitted under a hierarchical open N-mixture model to explicitly estimate roadkill rates. Dynamics in the population of carcasses N is modelled as a result of two processes, entries and persistence. A roadkill rate is derived from the entry parameter γ; (5) Landscape stability is assessed by measuring the transition rates from native to non-native covers; and (6) Priority segments for mitigation are defined based on high fatality rates and low landscape transition rates.
We applied this framework to define priority segments for mitigation of amphibian fatalities on two roads surrounded by a mosaic of grasslands, pastures, wetlands, rice fields, and urban areas in southernmost Brazil. In the fatality estimation step, we used dynamic N-mixture models to evaluate the influence of land use and cover on the distribution of carcasses, the impact of raining on roadkill, traffic volume and day/night time on carcass persistence. We expected that wetlands and urban areas in the surroundings of a segment would have a positive and negative influence, respectively, on the spatial distribution of carcasses; that fatality rates would be higher in rainy occasions; that at segments with higher traffic volume, carcass would persist less; and that carcass persistence would be higher at night.
Materials and methods
Study area
Located in southernmost Brazil, the roads ERS-040 and ERS-784 have 84 and 15 km of length, respectively (Figure 2). The ERS-040 is surrounded by a heterogeneous landscape, with a mosaic of wetlands, urban areas, rice field and cattle ranching, while the ERS-784 is bordered by extensive exotic Pinus sp. plantations, scattered human occupation and wetlands. As the roads are designed to access the coast, there is a strong increase in the daily traffic volume during the spring and more markedly in the summer months, that coincide with high amphibian activity.
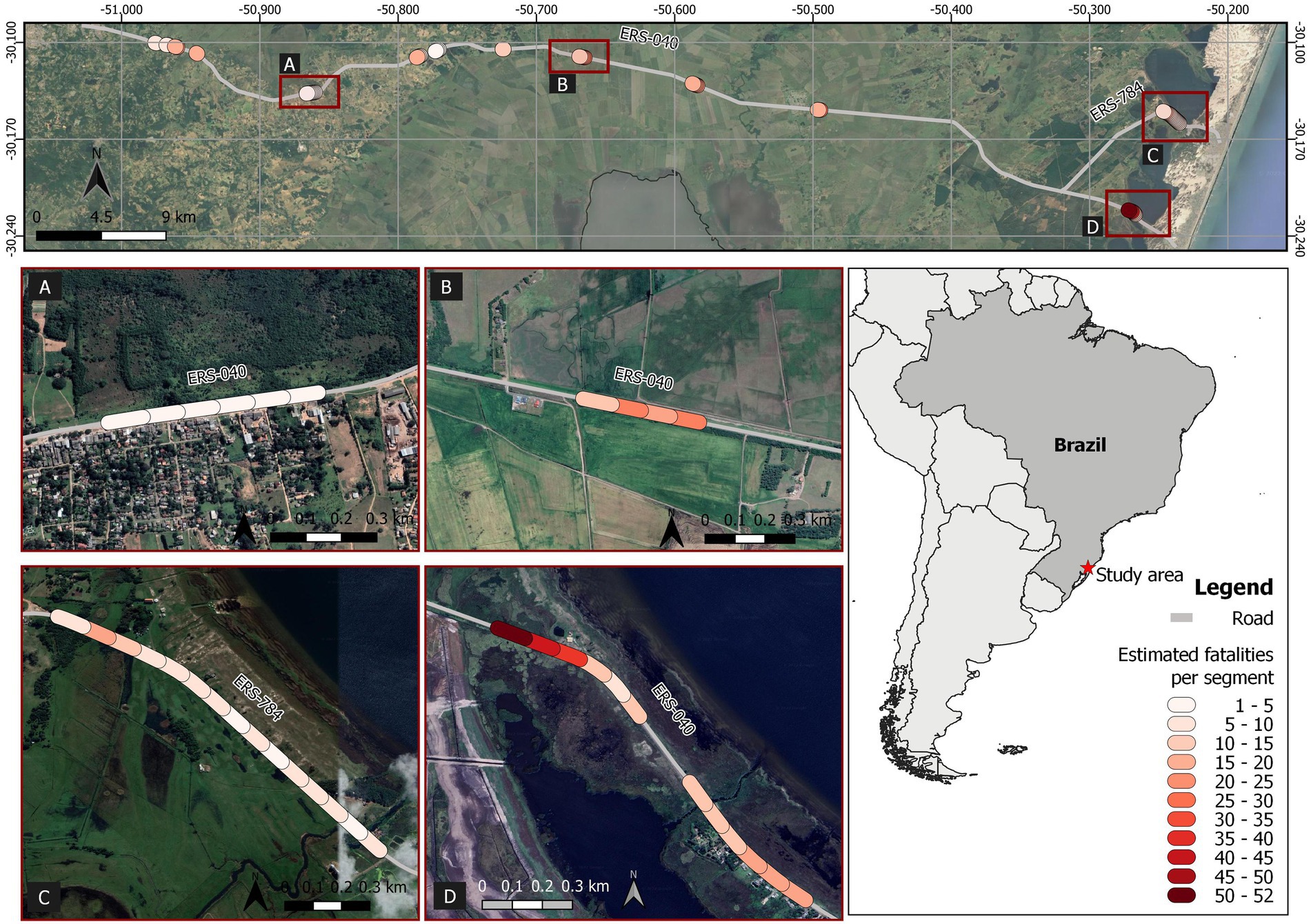
Figure 2. Road segments (100 m length) sampled for amphibian fatality estimation in southernmost Brazil (ERS-040 and ERS-784 roads) aiming at identifying priority locals for mitigation. In the upper image, colored circles represent estimated fatalities for the sampled segments, from which some of them (framed by squares) are shown in detail from (A–D). Map data © OpenStreetMap contributors, 2022.
Segments pre-selection for sampling
We identified road segments where we expected a higher concentration of amphibians (Figure 1.1). In the context of this study, the vast majority of amphibian species in the region are dependent on lentic environments (such as temporary and permanent pools, swamps, the edge of lagoons) and/or need these areas to complete their reproductive cycle. Hereafter, we will refer to these areas as wetlands. We selected 50 road segments with 100 m length using two sources of information: (i) percentage occupied by wetlands coverage in the surroundings obtained from remote sensing and field checking; and (ii) number of observed fatalities of aquatic reptiles (unpublished data obtained from a systematic survey of reptiles by car from the road concessionaire). Each segment was adopted as our sampling unit (site) and their extent was selected considering displacement capacities/willingness of amphibians along a fence (Duellman and Trueb, 1994; Brehme et al., 2021).
We calculated the percentage occupied by wetlands in a 1 km buffer centered on each segment, using two different classification sources followed by the inspection of high-resolution images using Google Earth Pro: the index of flooded surface in wetlands (Water In Wetlands-WIW- Lefebvre et al., 2019) and the Vegetation Coverage Map of Rio Grande do Sul - base year 2015, with 1:250,000 scale (Hoffmann et al., 2015). The WIW index was obtained through Google Earth Engine platform using Sentinel-2 satellite images available for spring and summer period (from September 2019 to January 2020), and the median of the pixel values between the dates obtained for the near-infrared (B8A) and short-wave infrared (B12) bands. To facilitate the identification of the wetlands, we modified the cut-off threshold of the B8A band to the value of 2000 nm and excluded the areas of rivers and deep lagoons. The WIW result is a raster in which each pixel with water on the surface received value 1 and the others value zero. We overlapped the WIW map with the classes water bodies, wetland, and wet grasslands from the Vegetation Coverage Map of Rio Grande do Sul. With this final map, we selected road segments with more than 30% of wetland within the 1 km buffer centered on each segment. We also selected segments based on the presence of at least two fatalities of aquatic reptiles (EGR, 2020). We use the occurrence of aquatic reptiles as an indicator for wet areas that could not be detected by remote sensing. We specifically considered aquatic snakes that feed on amphibians (water snake, Helicops infrataeniatus; and green snake, Erythrolamprus poecilogyrus) and turtles.
Carcass surveys
We conducted spatiotemporally replicated counts with a dependent double-observer protocol, that is, each of the 50 selected 100 m segments was sampled multiple times by two pairs of people on foot (i.e., front-pair is the first observer and the back-pair is the second; Figure 1.2). Each member of the front pair and back pair sampled one road lane and its respective shoulder. Surveys occurred twice a day – one survey at dawn and one at dusk – during three consecutive days in January of 2021, resulting in six sampling occasions (i.e., visits) per site. The first observer of each lane walked ahead and recorded all possible carcasses and the second walked behind and only recorded the carcasses not detected by the first (i.e., dependent double observers; Figure 1.2). Detected carcasses were not removed until the last sampling occasion was finished. For each detected carcass, we took a picture, recorded date, occasion, observer (1st or 2nd) and segment ID. Each anuran carcass record was identified, whenever possible, on the field, or based on pictures or carcasses collected after the last sampling occasion. We did not estimate fatality numbers per species since our aim was to exemplify a general application of the framework for amphibian roadkill estimation.
Covariates extraction
We included five covariates to estimate fatalities accounting for imperfection detection and its spatiotemporal heterogeneity (Figure 1.3): (i) wetland coverage, (ii) urban areas coverage, (iii) traffic volume, (iv) the presence/absence of rainfall, and (v) day/night time. We obtained the percentage occupied by wetland coverage and urban areas in 200 m buffers centered on each road segment. Wetland coverage was obtained from the same classification used for the site pre-selection. Urban areas were manually classified based on a 2019 high resolution image using Google Earth Pro, considering polygons encompassing each edification and human settlement within the 200 m buffer. We categorized the traffic volume into three levels (low, medium, and high) based on the proximity to the populous human settlements and the traffic distribution along a road corridor from populous cities to the coast. Higher traffic was considered for all segments located in the most populous city (Viamão) which is the connection to the main city in the region (Porto Alegre); medium traffic segments were in an agricultural area that connects the studied road to a federal road; and lower traffic segments were in the end of the road that have access to two coastal cities (Cidreira and Balneário Pinhal). The presence/absence of rain in the previous interval for each occasion was based on recordings of rainfall between 6 am and 5 pm for dusk sampling occasions and between 6 pm and 5 am for dawn occasions. Rainfall data were obtained from the closest weather station for each site: Tramandaí (code A834 from the Brazilian National Institute of Meteorology) and Viamão (code 432300202A from the National Center for Monitoring and Natural Disaster Alerts; CEMADEN, 2022; INMET, 2022). Each interval between occasions was defined as nighttime if sampling visit occurred during dawn and as daytime if occurred at dusk.
Dynamic N-mixture model for fatality estimation
We applied a dynamic N-mixture model to the double-observer carcass counts in each visit for each site (Figure 1.4), derived from the Dail-Madsen formulation with a robust design used for living populations (Dail and Madsen, 2011; Zhao and Royle, 2019). This model assumes that the local carcass population size varies throughout the visits as a result of two dynamic parameters: (i) carcass entries per interval; and (ii) carcass persistence probability between two visits so that , in which is the number of remaining carcasses from the previous visit and is the number of entering carcasses in the previous interval. The population size of the first visit is estimated using a Poisson distribution with mean (and variance) λ. The number of remaining carcasses is assumed to be a result of a binomial distribution in which each carcass from the population has a probability φ to persist until the next visit. The number of entering carcasses is assumed to follow a Poisson distribution with mean and variance γ. In the observation process, as surveys were conducted with a dependent double-observer protocol, the counts of each pair of observers are assumed to follow a multinomial distribution. Then, each carcass available on the population has a probability p of being detected by the first pair of observers and a probability of being detected by the second pair. Spatial and temporal variation (i.e., heterogeneity) in the four basic parameters (λ, φ, γ, and p) can be modelled as linear functions of covariates using the corresponding link functions (logit for probabilities and log for Poisson).
This approach permits to explicitly derive roadkill rate estimates (i.e., number of entering carcasses per interval), while taking into account imperfect detection and spatiotemporal heterogeneity in all parameters. Furthermore, it has the advantage of not requiring marking individual carcasses, neither trial experiments to separately estimate persistence and detection.
We considered effects of wetland coverage and urban areas on the initial carcass abundance (number of carcasses in the first occasion); wetland coverage and rain on entrant carcasses; and traffic volume and day/night time on carcass persistence. Carcass detection probability by each pair of observers was considered as constant. We estimated, as a derived parameter, an average roadkill rate per segment ( ) by calculating the mean number of entrant carcasses between the first and the last occasion and an overall roadkill rate for all segments We fitted the carcass count data to the dynamic N-mixture model under a Bayesian approach using software JAGS (Plummer, 2003) accessed from the package jagsUI (Kellner, 2015) in R (R Core Team, 2022). We ran three parallel Monte Carlo Markov Chains with 10,000 steps in the adaptive phase, followed by 100,000 steps from which the first 20,000 were discarded. This resulted in 240,000 samples of the posterior distribution from which we calculated the mean and 95% credible intervals for each parameter. We assigned vague prior distributions for all estimated parameters. Model convergence was assessed by visually inspecting the chains’ traceplots and using the R-hat statistics (R-hat ≤ 1.1). R and JAGS code are provided in Supplementary material 1.
Landscape conversion
To determine landscape stability for each segment, we used a landscape transition metric based on land cover transitions from native to non-native (Figure 1.5). The segments with lower transition rates represent sites that had a lower conversion of their surrounding landscape, and we assumed they are more prone to be stable over the long term, and thus are more suitable to receive mitigation actions that are fixed in space, such as fences associated to underpasses. We defined a 200 m buffer centered on each segment to extract the land-cover map to calculate the landscape transition rate. We extracted the maps for the years of 2009 and 2019 from the Mapbiomas V5.0 (Souza et al., 2020) and reclassified, grouping them into two classes: native and non-native land covers, using the software QGIS V3.12 (QGIS.org, 2022). With the Dinamica EGO software (Soares-Filho et al., 2009), we obtained the transition rate for each segment buffer by calculating the proportion of native land cover in 2009 that became non-native in 2019. We have considered here that this stability in landscape conversion would indicate areas in which mitigation measures would last longer because the region where the roads are embedded has a consolidated historical land use. However, we note that in different contexts, other criteria may be used.
Segment prioritization
We used a four-quadrant prioritization matrix to select segments for mitigation, considering: (i) the highest estimated fatality rates and (ii) the lowest transition rates from native to non-native land cover on the surrounding landscape (Figure 1.6). Quadrants were delimited by the median of the estimated fatality rates and the land-cover transition rate. Hence, road segments located in the quadrant formed by values above the median of estimated fatality rates and below the median of land-cover transition rate are the high-priority segments for mitigation.
Results
We found amphibian carcasses in 49 of the 50 sampled segments in at least one of the six occasions. Maximum count per visit in these segments varied from one to 127 carcasses detected by the two pairs of observers, while the mean count for all segments was 12 carcasses. Hylidae and Leptodactylidae families represented 75% of carcasses identified, whereas Dendropsophus spp. and Leptodactylus luctator, Leptodactylus gracilis, and Pseudis minuta were the most recorded species (Supplementary material 2).
The estimated average roadkill rate at the 50 segments during the 3 days was 136 (95%CrI = 130–142) amphibian fatalities per km per day. Mean roadkill rate for the 100 m segments ranged from 1.3 (95%CrI = 0.4–3.2) to 52.7 (95%CrI = 48.4–57.6) fatalities/day (Figure 2). We found a positive relationship of the initial carcass abundance with wetlands coverage (Figure 3A) and a negative relationship with urban areas coverage (Figure 3B). The number of entrant carcasses per interval was positively influenced by the wetland coverage and was twice higher when rain occurred (Figure 3C). Carcass persistence was higher during the night and very low for segments with high traffic volume (Figure 3D). The probability of each pair of observers to detect an amphibian carcass was 0.69 (95%CrI = 0.66–0.72; Table 1).
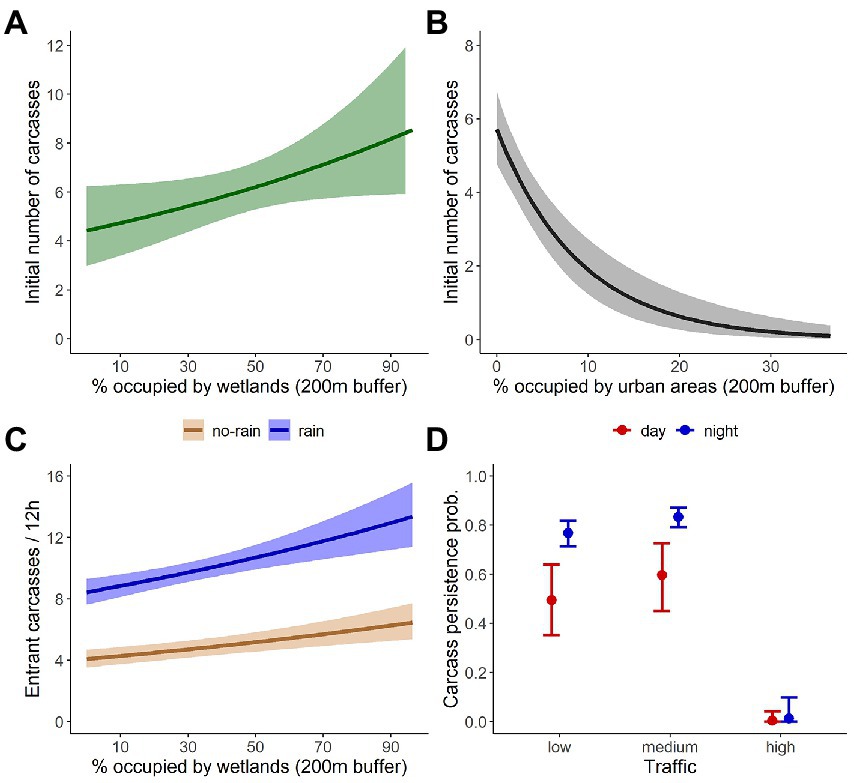
Figure 3. Predicted relations from the dynamic N-mixture model applied for dependent double-observer counts of amphibian carcasses. Relationship of the initial number of carcasses with (A) wetlands coverage, and (B) coverage of urban areas; (C) the number of entrant carcasses with wetlands coverage for rainy and not-rainy intervals; and (D) the carcass persistence probability with traffic volume and time of the day. Shaded areas and error bars represent the 95% credibility intervals.
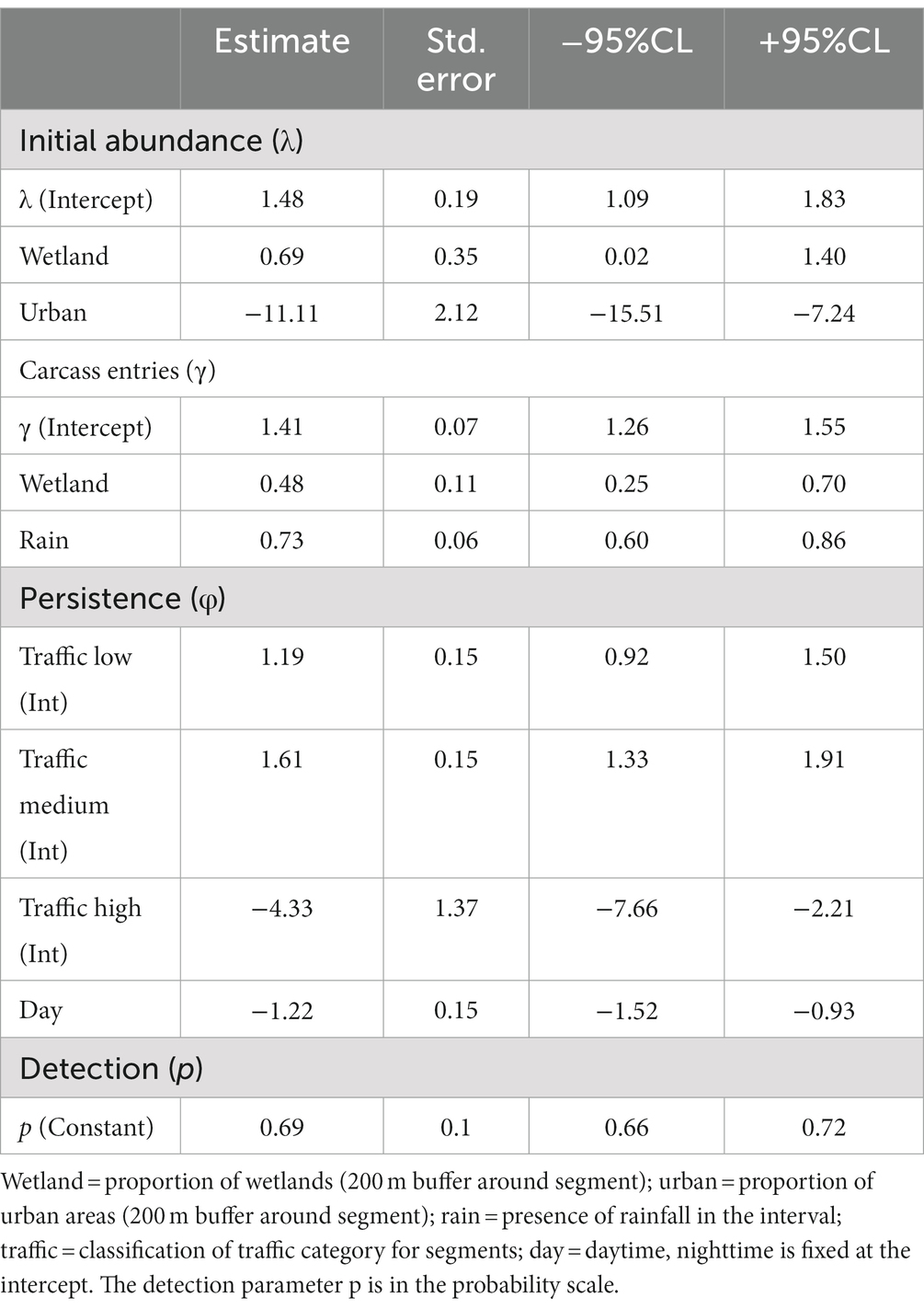
Table 1. Coefficient estimates obtained from the dynamic N-mixture model applied for dependent double-observer counts of amphibian carcasses.
Landscape transition rate varied from 0 to 98% of conversion. The median of the estimated fatality rate was 9.99 individuals per day and the median of the landscape transition rate was 5% in a 10-year interval. Ten segments were prioritized to receive the mitigation actions with these thresholds for fatality estimates and landscape stability, meaning a 5-fold reduction of segment numbers (Figure 4).
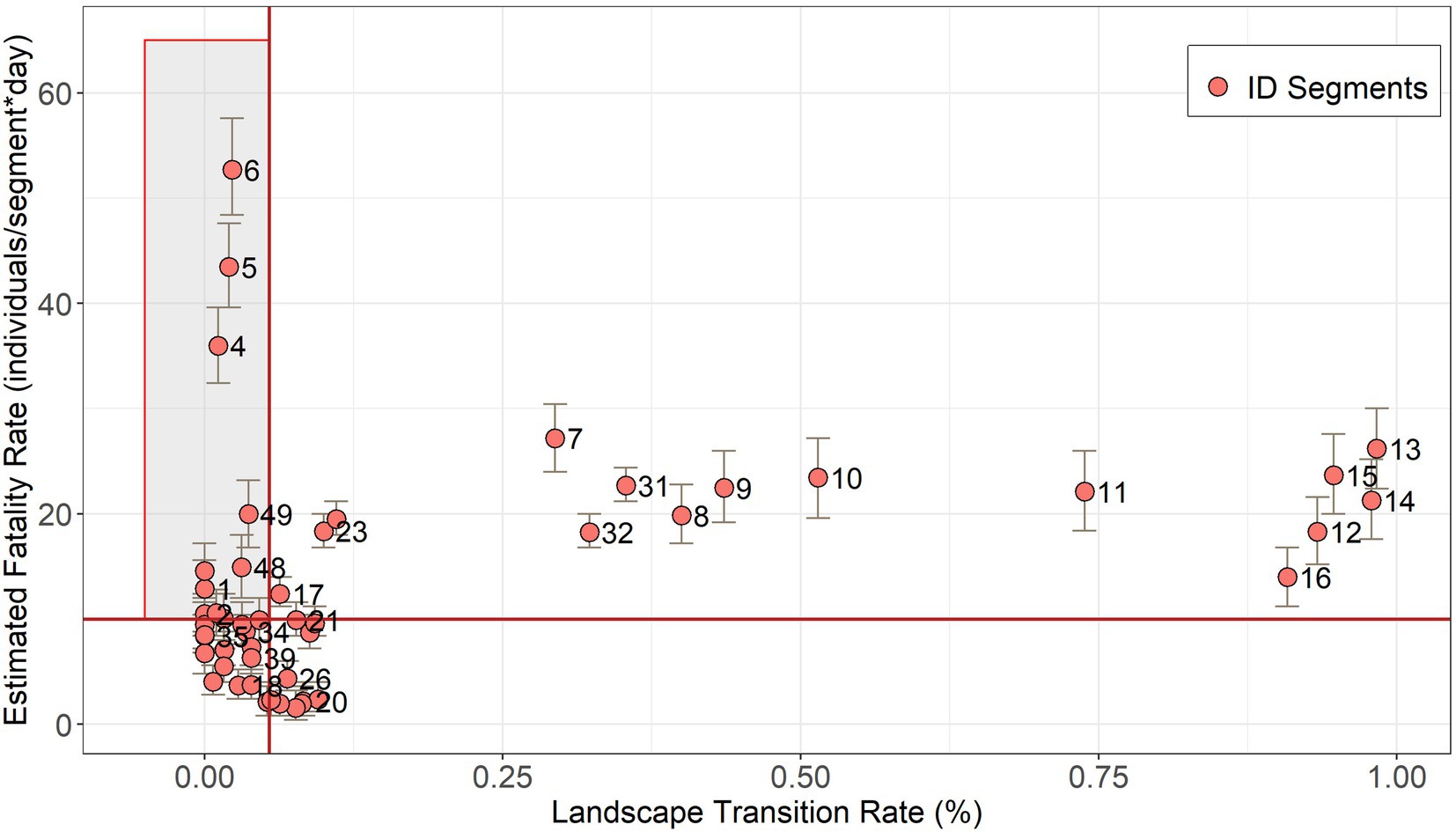
Figure 4. Segment prioritization to receive mitigation actions for amphibian roadkills, based on fatality estimation and landscape stability. The red lines are the threshold defined by the median values of the land-cover transition rate (5% of landscape transition) and the fatality estimate (9.99 individuals per day). Priority segments are within the shaded box. Numbers correspond to segment IDs and bars to the credibility intervals of fatality estimates (Supplementary material 3).
Discussion
Although amphibians are often a major group affected by roadkill (Fahrig et al., 1995; Glista et al., 2008; Coelho et al., 2012), planning efficient mitigation actions for this group imposes challenges given the usual difficulty to survey their carcasses. In order to obtain reliable estimates of amphibian roadkill rates and propose enduring mitigation measures, we present a prioritization framework based on a pre-selection of segments to be surveyed, an explicit modeling of fatalities with imperfect detection, and an evaluation of landscape stability. With this approach, we were able to identify 10 high-priority 100 m-segments to receive mitigation measures, i.e., 1 km in a context of about 100 km of road.
By choosing segments more likely to concentrate amphibian roadkills, we have reduced to 5% the length of road to be sampled. The usual small size of amphibians makes them hard to detect from traditional carcass survey methods (i.e., by car). By searching amphibian carcasses on foot, we obtained a carcass detection probability of ~70% by each pair of observers, which we considered quite good for this group. Moreover, the spatiotemporally replicated design proposed here requires carrying out counts in multiple visits at the segments, making more difficult to cover a large road extent. Therefore, given the logistic constraints to survey amphibian carcasses on foot at extensive roads, pre-selecting segments based on habitat features is a way to make sampling more feasible. Importantly, the pre-selection criteria must be chosen according to the habitat associations of each target group.
Spatiotemporally replicated counts of carcasses can be a cost-effective method to robustly estimate roadkill patterns. Fitting these counts with dynamic N-mixture models enables to explicitly derive roadkill rates for known time intervals (time between visits), while formally accommodating the potential sources of error (and their heterogeneity) in the same modelling structure. Previous studies have also used carcass counts or occurrences split into segments to model spatial variation on roadkills and identify hotspots based on a Poisson distribution (e.g., Santos et al., 2017; Lin et al., 2019). However, such approaches fail in accounting for imperfect detection (and even less the possibility of modeling the heterogeneity in persistence and detection) and do not provide reliable explicit rates of roadkills. Our directly derived roadkill rates also have the advantage of being comparable among studies, species or regions. Sources of errors (persistence and detection) in roadkill assessments are commonly addressed using trial experiments in which a known number of carcasses is disposed on the road (Barrientos et al., 2018; Gonçalves et al., 2018). However, there might be spatial and temporal variations in persistence and detection that are unfeasible to capture and represent with experiments. For example, as we found here, carcass persistence presented considerable variations according to the traffic volume at the segment and the period of the day. Not accounting in trial experiments for such heterogeneities may produce biased estimation of roadkill patterns. Some studies have made attempts to use hierarchical models in the context of roadkill data to identify priority segments while accounting for imperfect detection (e.g., Santos et al., 2018; Hallisey et al., 2022). Nevertheless, the approach adopted in these studies uses detection/non-detection data and makes inferences on “carcass occupancy” for a wide-time window, and not roadkill numbers.
Revealing effects of spatial and/or temporal covariates in the roadkill rates can be useful, for example, to predict fatalities hotspots in roads planned to be constructed or to plan carcass surveys in moments that patterns would be more highlighted in data. As expected, we found here that wetland coverage (high-quality habitat for most amphibian species in the studied region) in the road surroundings influenced the mean number of amphibians roadkilled. Segments with 90% of wetland coverage can present on average 47% more fatalities than segments with 10%. Such identified relationships could be applied to predict segments with potential higher roadkill rates in other roads with similar landscape characteristics. Moreover, the presence of rain during the interval between sampling visits resulted in about twice higher amphibian fatalities than intervals without rain. When planning carcass surveys, this kind of temporal variation should be taken into account to prioritize periods that might maximize the detection of spatial patterns in roadkill rates.
One advantage of the modeling approach we used is that it allows ecologists to estimate the dynamic parameters without marking individuals (Dail and Madsen, 2011; Dénes et al., 2015), as it was proposed by Péron et al. (2013). An important assumption of capture-recapture models is that marked and unmarked individuals have the same persistence and detection probabilities. By fitting carcass count data with N-mixture models, we avoided the need of marking the small amphibian carcasses, procedure that is logistically difficult and could, for example, influence later detections or affect the persistence of carcasses that were adhered to the substrate. However, because of the lack of information on individual capture histories, dynamic N-mixture models could sometimes present problems in parsing out entry and persistence processes (Kéry and Royle, 2021). Despite this issue may result in biased absolute roadkill estimation, this approach is still useful in relative terms (i.e., which sites present higher roadkill rates) to identify priority sites for mitigation. One alternative to ensure unbiased absolute roadkill estimations is to mark just a few individuals to directly inform entry and persistence processes.
Our framework recognized the priority road segments for amphibian mitigation not only identifying at which places animals tend to die more, but also including landscape conversion as a long-lasting criterion. We highlight the importance of considering the endurance of mitigation actions jointly with higher roadkill rates to ensure effective measures in long-term. Other criteria than lower landscape conversion rates could be used depending on the context of the surrounding landscape, such as where roads which are built in pristine areas (e.g., Amazonian Arc of Deforestation). Moreover, depending on the mitigation objectives, prioritization could consider other criteria, such as the number of threatened species per site, or per capita mortality, if cascading population effects are the main concern (Teixeira et al., 2017). When data on the population in the surroundings are available and when the maintenance of a population is a conservation target, this kind of information can provide important assets to use in the prioritization step. There have been cost-effective manners of obtaining population data for one or more amphibian species in the surrounding of roads, in order to integrate information in mitigation planning, such as automatic acoustic recordings (Marques et al., 2013) or citizen science programs that monitor amphibian migrations [e.g., Toads on Roads (Petrovan et al., 2020); Big Night programs (Sterrett et al., 2019)].
We proposed our sampling and analytical framework to estimate amphibian roadkill rates accounting for imperfect detection and its heterogeneity. The modeling approach can be expanded in order to accommodate other sources of variation, for example, differentiating species and including them as random effects in multi-species models (Yamaura et al., 2012; Dorazio et al., 2015). This might be particularly important when there is interest in threatened species. Also, although we have focused on amphibians, this framework could be useful to identify locals for mitigation measures for any roadkill target, especially when marking individuals is a difficult task.
Our study set out to better support mitigation prioritization decisions and such information can be used to inform where to implement road management actions. However, in terms of conservation, an imperative further step is to indicate and implement appropriate mitigation measures for road management to reduce roadkill of the target group studied here. Fencing is the most suitable spatial mitigation structure to maintain amphibians off the road and mitigate roadkills (Cunnington et al., 2014). Also, it is essential to promote safe crossings for amphibians daily movements with the implementation of wildlife passages (Woltz et al., 2008; Beebee, 2013; Jarvis et al., 2019). We highlight that the implementation of those mitigation measures is not the final step either since their effectiveness should be evaluated with robust designs aiming to collect the relevant evidence (Helldin and Petrovan, 2019; Ottburg and van der Grift Edgar, 2019; Schmidt et al., 2020).
In conclusion, our study developed a sampling and analytical framework to improve road management toward spatial prioritization of mitigation measures for amphibian roadkill. We proposed six steps to better support amphibian conservation decision making and such information can be used to inform where to implement road management actions. Our findings are also useful to plan future carcass surveys in locations and moments that patterns would be more suitable.
Data availability statement
The original contributions presented in the study are publicly available. This data can be found here: https://figshare.com/s/0cebdfcedb591dea6fed.
Author contributions
All authors conceived the idea of the manuscript, designed the survey, interpreted the results, wrote sections in all drafts, contributed critically to the writing, and gave final approval for publication. IB developed the analytical approach. JB and CZ collected roadkill data. JB, CZ, and LG compiled and synthesized data. JB and IB performed data analyses. JB, CZ, and IB made figures and tables. LG and IB led the writing process, working on the final version of the manuscript. All authors contributed to the article and approved the submitted version.
Funding
Financial support was provided by Fundação Empresa Escola de Engenharia da Universidade Federal do Rio Grande do Sul for LG, IB, CZ, JB, and AK (Projects FEENG/307 and FEENG/437). LG is funded by Programa USP Sustentabilidade da Superintendência de Gestão Ambiental da Universidade de São Paulo.
Acknowledgments
We thank STE – Serviços Técnicos de Engenharia S.A., EGR – Empresa Gaúcha de Rodovias, and Fepam – Fundação Estadual de Proteção Ambiental Henrique Luis Roessler for the opportunity to apply our framework in the mitigation planning of roads in the renewing process of the operating environmental license. We thank Guilherme Iablonoviski and Igor Pfeifer Coelho for the support in the land cover maps; Dener Heiermann and Maria Eduarda Bernardino Cunha for amphibian identification; and all survey teams for their effort: Ingridi Camboim Franceschi, Samuel Ferreira Gohlke, Priscila Cortêz Barth, Maria Eduarda Bernardino Cunha, Nikolas Raphael Oliveira Giannakos, Giulia Dorneles Barbieri de Campos, Isabel Salgueiro Lermen, Igor Oliveira Gonçalves, Maitê Zanella Busanello, Dener Heiermann, Érico Moreira de Miranda, and Deivid Pereira.
Conflict of interest
The authors declare that the research was conducted in the absence of any commercial or financial relationships that could be construed as a potential conflict of interest.
Publisher’s note
All claims expressed in this article are solely those of the authors and do not necessarily represent those of their affiliated organizations, or those of the publisher, the editors and the reviewers. Any product that may be evaluated in this article, or claim that may be made by its manufacturer, is not guaranteed or endorsed by the publisher.
Supplementary material
The Supplementary material for this article can be found online at: https://www.frontiersin.org/articles/10.3389/fevo.2023.1123292/full#supplementary-material
References
Barrientos, R., Martins, R. C., Ascensão, F., D’Amico, M., Moreira, F., and Borda-de-Água, L. (2018). A review of searcher efficiency and carcass persistence in infrastructure-driven mortality assessment studies. Biol. Conserv. 222, 146–153. doi: 10.1016/j.biocon.2018.04.014
Beebee, T. J. C. (2013). Effects of road mortality and mitigation measures on amphibian populations. Conserv. Biol. 27, 657–668. doi: 10.1111/cobi.12063
Brehme, C. S., Tracey, J. A., Ewing, B. A. I., Hobbs, M. T., Launer, A. E., Matsuda, T. A., et al. (2021). Responses of migratory amphibians to barrier fencing inform the spacing of road underpasses: a case study with California tiger salamanders (Ambystoma californiense) in Stanford, CA, USA. Glob. Ecol. Conserv. 31:e01857. doi: 10.1016/j.gecco.2021.e01857
CEMADEN (2022). Centro Nacional de Monitoramento e Alertas de Desastres Naturais. Available at: http://www2.cemaden.gov.br/ [Accessed March 10, 2022]
Clevenger, A. P., and Ford, A. T. (2010). “Wildlife crossing structures, fencing, and other highway design considerations” in Safe passages: Highways, wildlife, and habitat connectivity. eds. J. P. Beckmann, A. P. Clevenger, and M. Huijser (Washington DC: Island Press), 17–50.
Coelho, I. P., Teixeira, F. Z., Colombo, P., Coelho, A. V. P., and Kindel, A. (2012). Anuran road-kills neighboring a peri-urban reserve in the Atlantic Forest, Brazil. J. Environ. Manag. 112, 17–26. doi: 10.1016/j.jenvman.2012.07.004
Cunnington, G. M., Garrah, E., Ewen, E., and Fahrig, L. (2014). Culverts alone do not reduce road mortality in anurans. Ecoscience 21, 69–78. doi: 10.2980/21-1-3673
Dail, D., and Madsen, L. (2011). Models for estimating abundance from repeated counts of an open metapopulation. Biometrics 67, 577–587. doi: 10.1111/j.1541-0420.2010.01465.x
Dénes, F. V., Silveira, L. F., and Beissinger, S. R. (2015). Estimating abundance of unmarked animal populations: accounting for imperfect detection and other sources of zero inflation. Methods Ecol. Evol. 6, 543–556. doi: 10.1111/2041-210X.12333
Dorazio, R. M., Connor, E. F., and Askins, R. A. (2015). Estimating the effects of habitat and biological interactions in an avian community. PLoS One 10:e0135987. doi: 10.1371/journal.pone.0135987
Duellman, W. E., and Trueb, L. (1994). Biology of amphibians. Baltimore, Maryland, EUA: Reprint Edn. Johns Hopkins University Press.
EGR (2020). Plano de Mitigação ao Atropelamento de Fauna do Programa de Proteção e Monitoramento de Fauna. Available at: https://www.egr.rs.gov.br/upload/arquivos/202206/23154012-20210825162958anexo-ii-plano-de-mitigacao-fauna-egr.pdf [Accessed March 10, 2022].
Fahrig, L., Pedlar, J. H., Pope, S. E., Taylor, P. D., and Wegner, J. F. (1995). Effect of road traffic on amphibian density. Biol. Conserv. 73, 177–182. doi: 10.1016/0006-3207(94)00102-V
Fahrig, L., and Rytwinski, T. (2009). Effects of roads on animal abundance: an empirical review and synthesis. Ecol. Soc. 14:21. doi: 10.5751/ES-02815-140121
Girardet, X., Conruyt-Rogeon, G., and Foltête, J. C. (2015). Does regional landscape connectivity influence the location of roe deer roadkill hotspots? Eur. J. Wildl. Res. 61, 731–742. doi: 10.1007/s10344-015-0950-4
Glista, D. J., DeVault, T. L., and DeWoody, J. A. (2008). Vertebrate road mortality predominantly impacts amphibians. Herpetol. Conserv. Biol. 3, 77–87.
Gonçalves, L. O., Alvares, D. J., Teixeira, F. Z., Schuck, G., Coelho, I. P., Esperandio, I. B., et al. (2018). Reptile road-kills in southern Brazil: composition, hot moments and hotspots. Sci. Total Environ. 615, 1438–1445. doi: 10.1016/j.scitotenv.2017.09.053
Guinard, É., Julliard, R., and Barbraud, C. (2012). Motorways and bird traffic casualties: carcasses surveys and scavenging bias. Biol. Conserv. 147, 40–51. doi: 10.1016/j.biocon.2012.01.019
Guinard, É., Prodon, R., and Barbraud, C. (2015). “Case study: a robust method to obtain defendable data on wildlife mortality” in Handbook of road ecology. eds. R. van der Ree, D. J. Smith and C. Grilo (Chichester, UK: John Wiley & Sons, Ltd), 96–100.
Gunson, K., and Teixeira, F. Z. (2015). “Road-wildlife mitigation planning can be improved by identifying the patterns and processes associated with wildlife-vehicle collisions” in Handbook of road ecology. eds. R. van der Ree, D. J. Smith and C. Grilo (Chichester, UK: John Wiley & Sons, Ltd), 101–109.
Hallisey, N., Buchanan, S. W., Gerber, B. D., Corcoran, L. S., and Karraker, N. E. (2022). Estimating road mortality hotspots while accounting for imperfect detection: a case study with amphibians and reptiles. Land 11:739. doi: 10.3390/land11050739
Helldin, J. O., and Petrovan, S. O. (2019). Effectiveness of small road tunnels and fences in reducing amphibian roadkill and barrier effects at retrofitted roads in Sweden. PeerJ 7:e7518. doi: 10.7717/peerj.7518
Hoffmann, G. S., Weber, E. J., and Hasenack, H. (2015). Mapa de Cobertura vegetal do Rio Grande do Sul - ano base 2015, obtido por interpretação visual de imagens Landsat. Nível de detalhe compatível com escala 1:250.000. Porto Alegre UFRGS IB Cent. Ecol. 2018. 1a ed.
INMET (2022). INMET - Instituto Nacional de Meteorologia. Available at: https://bdmep.inmet.gov.br/ [Accessed March 10, 2022].
IUCN (2021). The IUCN red list of threatened species. Version 2021-1. Available at: https://www.iucnredlist.org [Accessed March 10, 2022].
Jarvis, L. E., Hartup, M., and Petrovan, S. O. (2019). Road mitigation using tunnels and fences promotes site connectivity and population expansion for a protected amphibian. Eur. J. Wildl. Res. 65, 1–11. doi: 10.1007/s10344-019-1263-9
Kéry, M., and Royle, A. (2021). Applied hierarchical modeling in ecology: analysis of distribution, abundance and species richness in R and BUGS: volume 2: dynamic and advanced models, 1st edition. London, UK: Academic Press.
Lefebvre, G., Davranche, A., Willm, L., Campagna, J., Redmond, L., Merle, C., et al. (2019). Introducing WIW for detecting the presence of water in wetlands with landsat and sentinel satellites. Remote Sens. 11, 10–14. doi: 10.3390/rs11192210
Lin, Y. P., Anthony, J., Lin, W. C., Lien, W. Y., Petway, J. R., and Lin, T. E. (2019). Spatiotemporal identification of roadkill probability and systematic conservation planning. Landsc. Ecol. 34, 717–735. doi: 10.1007/s10980-019-00807-w
Marques, T. A., Thomas, L., Martin, S. W., Mellinger, D. K., Ward, J. A., Moretti, D. J., et al. (2013). Estimating animal population density using passive acoustics. Biol. Rev. 88, 287–309. doi: 10.1111/brv.12001
Meijer, J. R., Huijbregts, M. A. J., Schotten, K. C. G. J., and Schipper, A. M. (2018). Global patterns of current and future road infrastructure. Environ. Res. Lett. 13:064006. doi: 10.1088/1748-9326/aabd42
Ottburg, F. G. W. A., and van der Grift Edgar, A. (2019). Effectiveness of road mitigation for common toads (Bufo bufo) in the Netherlands. Front. Ecol. Evol. 7:23. doi: 10.3389/fevo.2019.00023
Patrick, D. A., Gibbs, J. P., Popescu, V. D., and Nelson, D. A. (2012). Multi-scale habitat-resistance models for predicting road mortality “hotspots” for turtles and amphibians. Herpetol. Conserv. Biol. 7, 407–426.
Pereira, A., Do, N., Calabuig, C., and Wachlevski, M. (2018). Less impacted or simply neglected? Anuran mortality on roads in the Brazilian semiarid zone. J. Arid Environ. 150, 28–33. doi: 10.1016/j.jaridenv.2017.11.015
Péron, G. (2018). Process-based vs. ad-hoc methods to estimate mortality using carcass surveys data: a review and a note about evidence complacency. Ecol. Model. 384, 111–118. doi: 10.1016/j.ecolmodel.2018.06.021
Péron, G., Hines, J. E., Nichols, J. D., Kendall, W. L., Peters, K. A., and Mizrahi, D. S. (2013). Estimation of bird and bat mortality at wind-power farms with superpopulation models. J. Appl. Ecol. 50, 902–911. doi: 10.1111/1365-2664.12100
Petrovan, S. O., Vale, C. G., and Sillero, N. (2020). Using citizen science in road surveys for large-scale amphibian monitoring: are biased data representative for species distribution? Biodivers. Conserv. 29, 1767–1781. doi: 10.1007/s10531-020-01956-0
Plummer, M. (2003). “JAGS: a program for analysis of Bayesian graphical models using Gibbs sampling.” in 3rd international workshop on distributed statistical computing. pp. 1–10. Available at: https://www.r-project.org/conferences/DSC-2003/Proceedings/Plummer.pdf [Accessed March 10, 2022].
Rytwinski, T., and Fahrig, L. (2012). Do species life history traits explain population responses to roads? A meta-analysis. Biol. Conserv. 147, 87–98. doi: 10.1016/j.biocon.2011.11.023
Santos, R. A. L., Ascensão, F., Ribeiro, M. L., Bager, A., Santos-Reis, M., and Aguiar, L. M. (2017). Assessing the consistency of hotspot and hot-moment patterns of wildlife road mortality over time. Perspect. Ecol. Conserv. 15, 56–60. doi: 10.1016/j.pecon.2017.03.003
Santos, S. M., Carvalho, F., and Mira, A. (2011). How long do the dead survive on the road? Carcass persistence probability and implications for road-kill monitoring surveys. PLoS One 6:e25383. doi: 10.1371/journal.pone.0025383
Santos, R. A. L. L., Mota-Ferreira, M., Aguiar, L. M. S. S., and Ascensão, F. (2018). Predicting wildlife road-crossing probability from roadkill data using occupancy-detection models. Sci. Total Environ. 642, 629–637. doi: 10.1016/j.scitotenv.2018.06.107
Schmidt, B. R., Brenneisen, S., and Zumbach, S. (2020). Evidence-based amphibian conservation: a case study on toad tunnels. Herpetologica 76, 228–239. doi: 10.1655/0018-0831-76.2.228
Schwartz, A. L. W., Williams, H. F., Chadwick, E., Thomas, R. J., and Perkins, S. E. (2018). Roadkill scavenging behaviour in an urban environment. J. Urban Ecol. 4, 1–7. doi: 10.1093/jue/juy006
Silva, I., Crane, M., and Savini, T. (2021). The road less traveled: addressing reproducibility and conservation priorities of wildlife-vehicle collision studies in tropical and subtropical regions. Glob. Ecol. Conserv. 27:e01584. doi: 10.1016/j.gecco.2021.e01584
Simonis, J., Dalthorp, D., Huso, M., Mintz, J., Madsen, L., Rabie, P., et al. (2018). GenEst user guide—Software for a generalized estimator of mortality: U.S. Geological Survey Techniques and Methods, book 7, chap. 72. doi: 10.3133/tm7C19
Soares-Filho, B. S., Rodrigues, H. O., Costa, W., and Schlesinger, P. (2009). Modeling environmental dynamics with Dinamica EGO. Cent. Sensoriamento Remoto. Univ. Fed. Minas Gerais. Belo Horizonte, Minas Gerais 115.
Souza, C. M., Shimbo, Z. J., Rosa, M. R., Parente, L. L., Alencar, A. A., Rudorff, B. F. T., et al. (2020). Reconstructing three decades of land use and land cover changes in brazilian biomes with landsat archive and earth engine. Remote Sens. 12:2735. doi: 10.3390/rs12172735
Sterrett, S. C., Katz, R. A., Fields, W. R., and Campbell Grant, E. H. (2019). The contribution of road-based citizen science to the conservation of pond-breeding amphibians. J. Appl. Ecol. 56, 988–995. doi: 10.1111/1365-2664.13330
Teixeira, F. Z., Coelho, A. V. P., Esperandio, I. B., and Kindel, A. (2013). Vertebrate road mortality: effects os sampling methods and carcass removal. Biol. Conserv. 157, 317–323. doi: 10.1016/j.biocon.2012.09.006
Teixeira, F. Z., Kindel, A., Hartz, S. M., Mitchell, S., and Fahrig, L. (2017). When road-kill hotspots do not indicate the best sites for road-kill mitigation. J. Appl. Ecol. 54, 1544–1551. doi: 10.1111/1365-2664.12870
van der Ree, R., Smith, D. J., and Grilo, C. (2015). “The ecological effects of linear infrastructure and traffic” in Handbook of road ecology. eds. R. van der Ree, D. J. Smith and C. Grilo (Chichester, UK: John Wiley & Sons, Ltd), 1–9.
Villegas-Patraca, R., Macías-Sánchez, S., MacGregor-Fors, I., and Muñoz-Robles, C. (2012). Scavenger removal: bird and bat carcass persistence in a tropical wind farm. Acta Oecol. 43, 121–125. doi: 10.1016/j.actao.2012.06.004
Visintin, C., van der Ree, R., and McCarthy, M. A. (2016). A simple framework for a complex problem? Predicting wildlife–vehicle collisions. Ecol. Evol. 6, 6409–6421. doi: 10.1002/ece3.2306
Wenz, L., Weddige, U., Jakob, M., and Steckel, J. C. (2020). Road to glory or highway to hell? Global road access and climate change mitigation. Environ. Res. Lett. 15:075010. doi: 10.1088/1748-9326/ab858d
Woltz, H. W., Gibbs, J. P., and Ducey, P. K. (2008). Road crossing structures for amphibians and reptiles: informing design through behavioral analysis. Biol. Conserv. 141, 2745–2750. doi: 10.1016/j.biocon.2008.08.010
Yamaura, Y., Royle, J. A., Shimada, N., Asanuma, S., Sato, T., Taki, H., et al. (2012). Biodiversity of man-made open habitats in an underused country: a class of multispecies abundance models for count data. Biodivers. Conserv. 21, 1365–1380. doi: 10.1007/s10531-012-0244-z
Zeller, K. A., Wattles, D. W., Conlee, L., and Destefano, S. (2020). Response of female black bears to a high-density road network and identification of long-term road mitigation sites. Anim. Conserv. 24, 167–180. doi: 10.1111/acv.12621
Keywords: mitigation prioritization, anurans, imperfect detection, persistence probability, landscape transition, hierarchical models
Citation: Gonçalves LO, Brack IV, Zank C, Beduschi J and Kindel A (2023) Spatially prioritizing mitigation for amphibian roadkills based on fatality estimation and landscape conversion. Front. Ecol. Evol. 11:1123292. doi: 10.3389/fevo.2023.1123292
Edited by:
Silviu O. Petrovan, University of Cambridge, United KingdomReviewed by:
Fernando Ascensão, University of Lisbon, PortugalClara Grilo, University of Lisbon, Portugal
Copyright © 2023 Gonçalves, Brack, Zank, Beduschi and Kindel. This is an open-access article distributed under the terms of the Creative Commons Attribution License (CC BY). The use, distribution or reproduction in other forums is permitted, provided the original author(s) and the copyright owner(s) are credited and that the original publication in this journal is cited, in accordance with accepted academic practice. No use, distribution or reproduction is permitted which does not comply with these terms.
*Correspondence: Larissa Oliveira Gonçalves, bGFyaXNzYS5vbGlnb25AZ21haWwuY29t
†These authors have contributed equally to this work and share first authorship