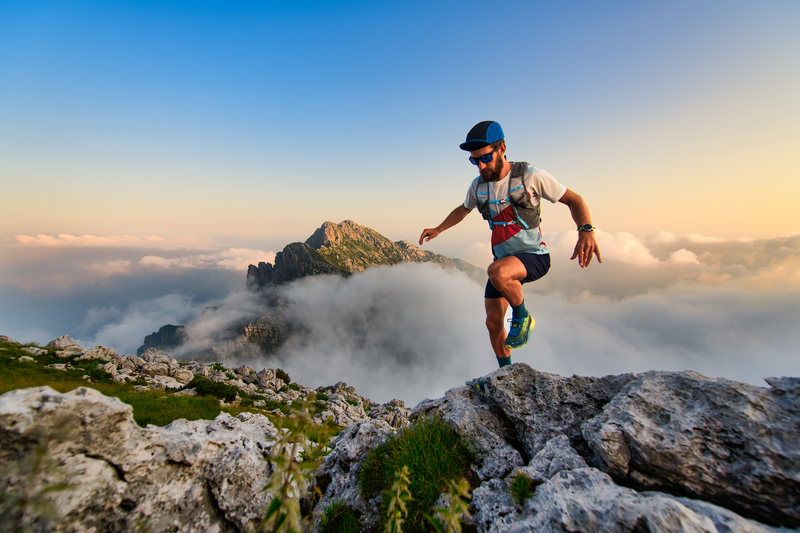
95% of researchers rate our articles as excellent or good
Learn more about the work of our research integrity team to safeguard the quality of each article we publish.
Find out more
ORIGINAL RESEARCH article
Front. Ecol. Evol. , 01 February 2023
Sec. Interdisciplinary Climate Studies
Volume 11 - 2023 | https://doi.org/10.3389/fevo.2023.1119048
This article is part of the Research Topic Climate Change and/or Pollution on the Carbon Cycle in Terrestrial Ecosystem View all 6 articles
Introduction: Theoretically and practically, studying the zero-carbon production supervision of marine ranching is crucial to the attainment of my country’s fishery carbon peak and carbon neutral goals.
Methods: This study introduces a third-party detection agency to construct a tripartite evolutionary game model based on the possibility of problems such as a lack of government supervision and imperfect detection mechanisms, through an analysis of the evolution stability and evolution path of each participant’s strategy choice, the mechanism of each element’s influence on the tripartite strategy choice is revealed, as well as the evolution law of the tripartite game.
Results: ① The change in strategic choice of each participant is significantly influenced by the change in strategic choice of the other participants, and the comprehensive consideration of the maximization of the comprehensive value of each participant is conducive to the development of all three parties. ② Government efforts to increase low-carbon subsidies and penalties will aid in promoting the normative behavior of zero-carbon production by marine ranching businesses and the rejection of rent-seeking by third parties. ③The accountability of higher-level governments for the dereliction of duty of regulatory agencies is particularly important to enhance the robustness of zero-carbon production of marine ranching enterprises.
Discussion: In this study, the ideal state of carbon balance of Marine ranching enterprises is taken as the condition to establish a tripartite evolutionary game, and the numerical simulation analysis results based on the model’s evolutionary stable equilibrium put forward countermeasures and suggestions for the government to improve the supervision mechanism of zero-carbon production of Marine ranching. In order to provide reference for the development of the marine ranching industry and promote the realization of zero emissions in the production activities of the marine ranching industry.
As the global warming problem caused by the increase in the concentration of greenhouse gasses such as CO2 has become more apparent in recent years (Zhang et al., 2022a,b,c,d), carbon peaking and carbon neutrality have gradually become the focus of attention of the world’s major countries (Han and Jiang, 2022; Zhang et al., 2022a,b,c,d). China is a significant carbon emitter and responds actively to climate change (Tan et al., 2022; Wang et al., 2022; Zhang et al., 2022a,b,c,d). The Chinese government has proposed carbon peaking by 2030 and carbon neutrality by 2060 as objectives. Controlling carbon sources and reducing carbon emissions is a consensus among Chinese citizens (China, 2022; Gao and Gao, 2022; Zhang et al., 2022a,b,c,d). Fishing is a major contributor to carbon emissions (Kristófersson et al., 2021). Marine ranching is a strategic measure for China’s fishery to combat climate change, reduce emissions, and increase sinks (Jinsheng et al., 2018; Jiao et al., 2022). 60–70% of the total energy consumption of fisheries is accounted for by fishing industry (Ha, 2008; Aragão et al., 2022). Therefore, encouraging the low-carbonization of the fishing industry is an effective way to reduce carbon emissions (Dağtekin et al., 2022). Therefore, ocean carbon sinks and low-carbon technologies (such as the installation of low-carbon engines or other emission reduction equipment on fishing vessels) are crucial to achieving zero-carbon production in marine ranching (Krause-Jensen and Duarte, 2016; Grabowski et al., 2019; Kim et al., 2021). However, the government may choose to relax regulations in response to performance factors such as the number of marine ranches. As an impartial and independent supervisor, the third-party testing agency offers carbon emission testing services to the government and marine ranch businesses, thereby enhancing the government’s confidence in monitoring the zero-carbon production of marine ranches (Lilong et al., 2022). With the promotion and widespread adoption of inspection and testing, it is anticipated that third-party testing agencies will introduce the field of marine pasture carbon emission supervision as a supplement to existing oversight. It has become an important test condition for marine ranching companies to apply for low-carbon subsidies (Hussain et al., 2020), avoid fines for high-carbon emissions (Liu et al., 2021), and undertake the social responsibility of China’s fishery to address climate change with government supervision departments (Gao K. et al., 2022; Wang and Wang, 2022).
In reality, however, there may be rent-seeking third-party testing agencies for ocean ranching businesses (Sha, 2019; Haoyang et al., 2022). In the production and service process of marine ranching enterprises, selecting a zero-carbon production strategy (carbon emissions = carbon sinks) will increase production costs and decrease profit margins, whereas selecting a non-zero-carbon production strategy (carbon emissions > carbon sinks) will reduce production costs and increase profit margins. Under limited government oversight, marine ranching companies may choose non-zero carbon production strategies and rent from third-party testing agencies in order to obtain low-carbon subsidies and avoid high-carbon emission fines (Nie et al., 2022). Interest-driven third-party testing agencies may choose intentional rent-seeking strategies, causing marine ranching enterprises to choose non-zero-carbon production strategies during the production process, thereby impeding their transition to a low-carbon footprint. Therefore, the possibility of excessive greenhouse gas emissions caused by non-zero-carbon production strategies of marine ranching enterprises still exists, and it is crucial to regulate marine ranching enterprises’ zero-carbon production strategies.
At present, many scholars around the world have used evolutionary game models to carry out more research on the direction of government departments to regulate carbon emissions. Xue et al. constructed a three-party evolutionary game model, analyzed the low-carbon regulation of manufacturing enterprises and put forward suggestions (Xue et al., 2022). Qu et al. analyzed the multi-party collaborative governance of energy conservation and emission reduction from the perspective of low-carbon supply chain through the three-party evolutionary game model (Qu et al., 2021). Gao et al. derived the evolutionary stability strategy of the government and smart transportation companies based on the two-party evolutionary game model, and used simulation to analyze the impact of various policy tools on the green innovation strategy (Gao L. et al., 2022). Yu et al. built an evolutionary game model involving the government, new energy companies and farmers, and analyzed the evolution path and stabilization strategy of the three parties in the process of low-carbon transformation of rural clean energy (Yu et al., 2022). Sun et al. constructed an evolutionary game model based on prospect theory, analyzed corporate carbon emission regulation issues, and put forward corresponding countermeasures and suggestions (Sun et al., 2022). Sun and Gao constructed and simulated an evolutionary game model, analyzed the impact of government regulation on corporate carbon emission behavior and industry market structure through the evolutionary game model, provided management suggestions, and promoted carbon emission reduction activities (Sun and Gao, 2022). Zhao established an evolutionary game model to analyze the evolutionary stability, discussed the systemic dynamic evolution process and put forward suggestions for the conflict of interest between the Chinese government and coal-fired power plants in CCS adoption (Zhao and Liu, 2019). It can be seen that most studies on the evolutionary game model of the regulatory mechanism start from the perspectives of corporate carbon emission management, carbon capture and storage (CCS), and clean energy promotion, and pay less attention to the direction of carbon balance. Simultaneously, research on the strategy and role of third-party participation in supervision is relatively abundant, and numerous countermeasures and suggestions for efficient government supervision have been proposed. Khan and Malik analyzed the role of the Healthcare Commission’s accreditation and argued that third-party accreditation facilitates the efficient oversight of healthcare by government agencies (Khan and Malik, 2020). Third-party certification in the food supply chain can reduce asymmetric information in the anonymous market and establish a relationship of trust between consumers and producers, according to Gonzalez-Azcarate et al. (2022). Konefal and Hatanaka noted that the technical and objective nature of third-party certification is conducive to enhancing the governance mechanism (Konefal and Hatanaka, 2011). Li et al. suggested that the introduction of a third party to participate in coal mine safety supervision could alleviate the problems associated with the government seeking power and public participation in supervision (Li et al., 2021). He et al. developed an evolutionary game model of green product quality supervision involving government regulators, third-party e-commerce platforms, online sellers, and consumers, and analyzed the evolutionary stability strategies of the four parties to improve the consumer feedback mechanism (He et al., 2021). Zorn et al. proposed a heuristic game theory model for analyzing various control quality levels, demonstrating the importance of monitoring organic food certification (Zorn et al., 2012). Wang pointed out that the third party has bolstered the initiative and independence of food safety supervision and facilitated its effective operation (Wang, 2014). To sum up, it can be seen that the research on the third-party monitoring mechanism is mostly from the perspective of quality management of food, medical and consumer products. At present, there is no research on third-party inspection of marine ranches.
In addition, the academic community is becoming increasingly interested in policy research on marine ranching, and these studies have significant reference value for improving the regulatory mechanism for low-carbon transformation of marine ranching. Wan, XL et al. constructed a three-party evolutionary game model, and put forward suggestions for the supervision mechanism of the marine ranch blue carbon trading market (Wan et al., 2021a,b). Qin, Man and Sun, Mingxue collected field survey data from 25 marine ranchings and analyzed the impact of marine ranching policies on the ecological efficiency of marine ranching (Qin and Sun, 2021). Qin et al. conducted a multi-dimensional empirical analysis on marine ranching policy documents, pointing out the direction for marine ranching policies (Qin et al., 2020). Qin et al. analyzed the administrative and social process of marine ranching policy implementation, and explored the key factors affecting the performance of marine ranching projects, in order to solve the key problems of high performance and low performance (Qin et al., 2021). Yu and Wang analyzed the goals and objectives of marine ranching management policies, and put forward strategies and suggestions for improving the quality and speed of marine ranching development (Yu and Wang, 2021). Wan et al. analyzed the blue carbon trading mechanism of marine ranching based on the tripartite game model, and put forward suggestions on how to design government subsidy policies to encourage blue carbon trading of marine ranching (Wan et al., 2021a,b). Du et al. created a three-party evolutionary game model (marine ranch enterprises, local governments, and central government), analyzed the ecological safety supervision mechanism of marine ranch, and proposed relevant measures to improve supervision efficiency (Du et al., 2022). It can be seen that most of the policy research on marine ranching starts from the perspectives of blue carbon trading, ecological benefits, and project performance management. At present, there is no research on carbon emission policy management of marine ranching.
In conclusion, the research on evolutionary game, zero-carbon production, third-party participation in regulation, and marine ranch policy has yielded abundant results, which serve as important references for the research on the regulation of marine ranch zero-carbon transformation. However, no direct research results on the regulation of zero-carbon production in marine ranches have been discovered. Therefore, on the basis of introducing a third-party testing agency as a supplementary supervision, this study constructed a model for marine ranching companies, third-party testing agencies, and government regulatory agencies based on the strict characteristics of zero-carbon emissions of marine ranching companies and the rent-seeking phenomenon of third-party testing. Based on the three-party evolutionary game model, Matlab2018a is used to conduct visual simulation analysis on the strategic equilibrium, evolution path and factor influence of each subject, in order to provide theoretical reference for zero-carbon production of marine ranches and improvement of government supervision mechanism.
The model developed in this paper consists of three participants: the marine ranching enterprise, the third-party testing agency, and the government supervision division. Figure 1 illustrates the evolutionary game model relationship among the players.
On the basis of an analysis of the characteristics of the participants, strategy sets, revenue functions, and other factors, as well as the indicators of the three participants in the regulatory game process presented in Table 1, this paper advances the following hypotheses regarding the game process of enterprise production eco-efficiency regulation.
Hypothesis 1: The strategy space for enterprises to choose is α = (α1, α2), the proportion of choosing the zero-carbon production strategy (α1) is x, and the proportion of not choosing the zero-carbon production strategy (α2) is 1-x, and x∈[0,1]. The strategy space chosen by the third-party testing agency is β = (β1, β2), the proportion of rejecting the rent-seeking strategy (β1) is y, the proportion of the intentional rent-seeking strategy (β2) is 1-y, and y∈[0,1]. The strategy space for government departments to choose is γ = (γ1, γ2), the proportion of choosing a strict regulatory strategy (γ1) is z, and the proportion of choosing a loose regulatory strategy (γ2) is 1-z, and z∈[0,1].
Hypothesis 2: The profit of the enterprise from selling goods and providing services is P1, the cost of choosing the zero-carbon production strategy is C1, and the cost of not choosing the zero-carbon production strategy is C2, and C1 > C2. When the enterprise chooses the zero-carbon production strategy for production, the zero-carbon production certification is qualified; when the enterprise does not choose the zero-carbon production strategy for production, it will seek rent from a third-party testing agency to obtain a zero-carbon permit through testing, and the rent-seeking cost is C3, and C3 < (C1-C2). At the same time, when the enterprise does not choose the zero-carbon production strategy, it will generate speculative costs, mainly including operating and management expenses such as falsified production records and false publicity. The speculative cost of the marine ranch enterprise is C4. When a company chooses a zero-carbon production strategy, it will generate additional income P3, such as blue carbon sinks.
Hypothesis 3: The government entrusts a third-party agency to test whether the enterprise is eligible for the subsidy S. After passing a third-party inspection agency’s inspection of zero-carbon production, businesses are eligible for low-carbon certification. If they fail the inspection, they will not be eligible for subsidies. P2 is the inspection revenue of the third-party inspection firm. The inspection will fail if the third-party inspection agency refuses to seek rent when the enterprise does not select the zero-carbon production strategy for production. If the third-party testing organization intends to seek rent, it will engage in rent-seeking behavior with businesses in order to assist businesses in obtaining subsidies if they do not choose zero-carbon production strategies. The speculative cost of the third-party testing agency’s intention to seek rent is C5, primarily including falsified testing records, issuing false reports, enhancing information security, etc., and C3 > C5.
When the government strictly regulates, if an enterprise does not choose a zero-carbon production strategy, the enterprise will be fined F1 and the third-party inspection agency that intends to seek rent will be fined F2 and the subsidy S will be canceled. When the government is laxly regulated, there is no way to obtain the strategic choice information of businesses and third parties, and the government regulatory department will issue subsidies S based on the inspection results of third-party institutions without imposing any penalties. Set C6 as the price of strict government regulation.
Hypothesis 5: Companies that do not choose zero-carbon production strategies promote ecological security and provide the government with a P4 political performance rating. When an enterprise does not choose a zero-carbon production strategy and reaches a rent-seeking agreement with a third-party testing agency, excessive CO2 emissions will increase environmental governance costs, and the cost of government departments to control excessive CO2 emissions will be C7. The lack of supervision results from the government supervision department’s adoption of a lax supervision strategy. When fisheries production generates excessive CO2 emissions, the credibility of the government department in charge of monitoring will suffer. Let the cost of duty dereliction be C8, and C8 > C6.
According to the aforementioned assumptions, the tripartite game income matrix of the marine ranching enterprise (MRES), the third-party inspection agency, and the government regulatory agency is constructed as shown in Table 2.
Based on the research conducted by Ha (2000), the replication dynamic equation analysis method for evolutionary games is adopted. Replication dynamics is a dynamic differential equation that describes the frequency with which a specific strategy is adopted in a population. Its general form is as follows:
Among them, is the proportion or probability of employing a pure strategy in the population, is the fitness (expected value) when employing a pure strategy, and is the average fitness (average expected value). In this paper represents the anticipated benefits of j strategies of the i-th stakeholder in this paper, where i = m represents the marine ranching enterprise. j = 1,2, respectively, represent the first and second strategies. For instance, represents the marine ranching operation’s progress toward adopting the “zero carbon production” strategy.
The expected benefits (U(x), U(1-x)) of marine ranching enterprises selecting zero-carbon or non-zero-carbon production strategies are as follows:
Likewise, the average income of marine ranching operations is represented by the symbol (U m) respectively. And obtain the average yield by:
Combining , with the preceding income matrix, the replication dynamics equation of marine ranching enterprise strategy selection is as follows:
According to formula (1), the expected returns (U(y), U(1-y)) of a third-party testing agency selecting either a non-rent-seeking or a rent-seeking strategy are as follows:
Similarly, the average income of the third-party testing organization is represented by respectively. And arrive at the average yield by:
Combining with the preceding income matrix, the replication dynamics equation for the third-party testing agency’s strategy selection is as follows:
According to formula (1), the expected returns (U(z), U(1-z)) of government regulators selecting a strict regulation strategy versus a lax regulation strategy are as follows:
In addition, the average returns of government regulatory departments are denoted by () respectively. And arrive at the average yield by:
Using in conjunction with the preceding income matrix, the replication dynamics equation for the government regulatory agency’s strategy selection is as follows:
The stability of the equilibrium point in this paper is determined by analyzing the Jacobian matrix (Daniel, 1990; Zhang et al., 2021). Based on analysis of Lyapunov stability (Bomze and Weibull, 1995; Somanathan, 1997). First, we assume that the replication dynamic equations of marine ranching enterprises, third-party inspection agencies, and government regulatory agencies are all zero. That is
According to Friedman (1991), evolutionarily stable strategies (ESS) occur only among pure strategies, thus ruling out mixed strategies in the first place. From this, 8 pure strategy points can be obtained, that is, (0,0,0), (0,0,1), (0,1,0), (0,1,1), (1,0,0), (1,0,1), (1,1,0), (1,1,1). In addition, the stability of the equilibrium point can be deduced from the Jacobian matrix result (Zhao and Liu, 2019). On the basis of Lyapunov’s stability criterion, the Jacobian matrix J of the model replicating the dynamic equation and its determinants Det[J] and Tr[J] are obtainable. For the general tripartite evolutionary strategy, the equilibrium point is ESS when the eigenvalues of the Jacobian matrix are all negative; when all eigenvalues of the Jacobian matrix are positive, the equilibrium point is unstable; when one or two eigenvalues are positive, the equilibrium point is saddle point (Shan and Yang, 2019). The main eigenvalues of various equilibrium points of the Jacobian matrix J and the equilibrium points’ stability conditions are presented in Table 3 and the proposition, respectively.
The Jacobian for the mixed strategy has opposite eigenvalues, this makes the hybrid strategy a saddle point. According to the aforementioned parameter assumptions and ESS evaluation criteria, it is not difficult to conclude that only E4(1,1,0) and E7(0,1,1) may satisfy all of the requirements for becoming equilibrium points.
1) Scenario 1: When C1<C2 + C4 + P3 + S, the replication dynamic system has only one stable point, E4(1,1,0).
To satisfy the conditions of the equilibrium point (1,1,0), we set the parameters to the following values:C1 = 60, C2 = 20, C3 = 20, C4 = 10, C5 = 10, C6 = 20, C8 = 40, F1 = 30, F2 = 30, S = 10, P3 = 30.
2) Scenario 2: When C2 + C4 + F1 + P3 + S C1, the replication dynamic system has only a single stable point, E7(0,1,1).
To satisfy the conditions of the equilibrium point (0,1,1), we set the parameters to the following values:C1 = 120, C2 = 20, C3 = 80, C4 = 10, C5 = 60, C6 = 20, C8 = 40, F1 = 30, F2 = 30, S = 10, P3 = 30.
It can be seen from Figure 2, the pure strategy balance point E4(1,1,0) indicates that marine ranching companies opt for zero-carbon production, third-party inspection agencies refuse to seek rent, and government departments supervise laxly, which is the optimal state for green supervision of marine ranching companies. This paper simulates the stable equilibrium path of system convergence under different parameter values for policy tools and analyzes the impact of policy tools on the tripartite game.
Figure 2. Two-dimensional path evolution and three-dimensional path evolution diagrams of equilibrium points E4(1, 1, 0) and E7(0, 1, 1).
Matlab 2018a is used for simulation analysis to verify the efficacy of the evolutionary stability analysis by assigning numerical values to the model and comparing it to the actual situation. This study establishes two sets of parameters in order to meet the requirements of Scenario 1 (E4) and Scenario 2 (E7), namely:C1 = 60, C2 = 20, C3 = 20, C4 = 10, C5 = 10, C6 = 20, C8 = 40, F1 = 30, F2 = 30, S = 10, P3 = 30/C1 = 120, C2 = 20, C3 = 80, C4 = 10, C5 = 60, C6 = 20, C8 = 40, F1 = 30, F2 = 30, S = 10, P3 = 30. Analyze the impact of C3, S, F1, F2, C6, and C8 on the evolution of the game and its outcomes.
First, to analyze the impact of C3 changes on the evolutionary game process and results, when other basic parameters remain unchanged, C3 = 5, 20, 60/C3 = 20, 80, 85 are brought in, Figures 3, 4 depicts the simulation results of replicating the dynamic equations evolving 50 times over time. To analyze the influence of S changes on the evolutionary game process and results, when other basic parameters remain unchanged, S = 10, 30, 50/S = 5, 10, 20 are brought in, Figures 5, 6 depict the simulation results of replicating dynamic equations evolving 50 times over time.
Figures 3, 4 illustrate that Scenario 1 and Scenario 2 are consistent. During the evolution of the system, the increase in rent-seeking costs can hasten the evolution of marine ranching enterprises’ stable selection of zero-carbon production strategies. With an increase in C3, the likelihood of marine ranching enterprises opting for a zero-carbon production strategy rises, as does the likelihood of third-party testing agencies seeking rent hikes. Therefore, the government can take measures such as increasing the entry threshold for third-party inspection agencies, reviewing the relevant qualifications of candidate inspection agencies, and establishing the integrity and legal compliance of inspection agencies, increasing the rent-seeking cost of marine ranching operations increases the likelihood that a zero-carbon production strategy will be selected.
The evolution processes of Scenario 1 and Scenario 2 are consistent, as evidenced by Figures 5, 6. During the process of system evolution, an increase in government subsidies can expedite the rate of evolution of marine ranching enterprises, allowing them to choose the zero-carbon production strategy more consistently. With the increase of S, the marine ranching industry the likelihood of businesses opting for carbon-neutral production methods increases. Therefore, it is reasonable for the government to increase the subsidy for zero-carbon production of marine ranching enterprises and increase the likelihood that marine ranching enterprises will choose zero-carbon production strategies.
Next, leaving all other fundamental parameters unchanged, assign F1 = 10, 30, 50/F1 = 20, 30, 40; the simulation results are depicted in Figures 7, 8; assign F2 = 10, 30, 50/F2 = 30, 50, 70; the simulation results are depicted in Figures 9, 10.
Figure 7. Map of the impact of the government on the amount of fines imposed on marine ranching companies (Scenario 1).
Figure 8. Map of the impact of the government on the amount of fines imposed on marine ranching companies (Scenario 2).
Figure 9. The impact of the government on the amount of fines imposed by third-party testing agencies (Scenario 1).
Figure 10. The impact of the government on the amount of fines imposed by third-party testing agencies (Scenario 2).
The evolution processes of Scenario 1 and Scenario 2 are inconsistent, as depicted in Figures 7, 8. In the evolution process of Scenario 1, as F1 increases, the probability that marine ranching enterprises will choose a zero-carbon production strategy rises, while the probability that government departments will choose strict supervision declines; In Scenario 2’s evolution process, as F1 rises, the likelihood of marine ranching businesses opting for a zero-carbon production strategy and the likelihood of government agencies opting for stringent oversight rises.
The evolution processes of Scenario 1 and Scenario 2 in Figures 9, 10 are inconsistent. In Scenario 1’s evolution process, as F2 increases, the likelihood of third-party inspection agencies rejecting the rent-seeking strategy and the likelihood of government departments opting for strict supervision rises; as F2 increases in the evolution process of Scenario 2, the probability that the third-party inspection agency rejects the rent-seeking strategy rises, while the probability that the government department chooses strict supervision falls. In order for marine ranching companies and third-party testing agencies to share the social responsibility of carbon neutrality, the government should formulate a punishment mechanism that is based on scientific evidence.
In addition, when other fundamental parameters remain unchanged, the simulation results are depicted in Figures 11, 12 when C6 = 10, 20, 30/C6 = 10, 20, 30 is assigned; when C8 = 20, 40, 60/C8 = 30, 40, 50 is assigned, the simulation results are depicted in Figures 13, 14.
Figures 11, 12 demonstrate the consistency of C6’s evolution in Scenario 1 and Scenario 2. As C6 increases during the evolution process, the likelihood that government departments will choose strict regulation decreases. Figures 13, 14 show that the evolution process of C8 in Scenario 1 and Scenario 2 is consistent. During the evolution process, as C8 increases, the probability of government departments choosing strict regulation increases. Therefore, the excessively high cost of strict regulation is not conducive to the performance of the supervisory department itself, and the severe administrative punishment imposed by the superior government can prompt the supervisory department to choose the robustness of the strict supervision strategy.
This study constructs a tripartite evolutionary game model among marine ranching enterprises, third-party testing institutions and government regulatory authorities for the regulation of low-carbon transformation of marine ranching enterprises. Lyapunov stability discriminant method was used to calculate the stability of evolutionary game and the conditions of evolutionary stability. MATLAB 2018a is used to simulate and analyze the impact of enterprise rent seeking costs, low-carbon subsidies and other factors on the evolution and stability strategy of marine ranching enterprises, third-party inspection agencies and government regulators. In addition, relevant countermeasures and suggestions were put forward to regulate the low-carbon transformation of marine ranching according to the influence relationship and stable conditions of various factors. The main conclusions are as follows.
Main conclusions: The changes in the strategic choices of each participant are greatly affected by the changes in the strategic choices of other participants. It is necessary to comprehensively consider the maximization of the comprehensive value of each participant to effectively promote the common and healthy development of the three parties; the government appropriately increases low-carbon subsidies and penalties Both help to promote the normative behavior of zero-carbon production of marine ranching enterprises and the third party’s rejection of rent-seeking; the accountability of the higher-level government for the dereliction of duty of the supervisory department is particularly important for enhancing the robustness of zero-carbon production of marine ranching enterprises; The sales and service income and increasing the rent-seeking cost of enterprises are also effective ways to avoid excessive carbon emissions of marine ranching enterprises.
Under the background of carbon neutrality, this paper analyzes the changes of behavior strategies of all participants in the production process of marine ranching enterprises. We discuss the application of evolutionary game theory in the regulation of zero carbon production of marine ranching enterprises, which enriches the research on the choice of subject behavior strategies in the field of zero carbon production of marine ranching. However, this paper also has some shortcomings. For example, in the process of building the model, only the asymmetric information and limited rationality of the supervision of marine ranching enterprises in the production and detection link are considered, and the impact of other external factors on the strategic behavior of each participant is not considered. In the future research, we will consider the impact of these factors as much as possible to optimize the subject behavior strategy in the field of zero carbon production of marine ranching. Therefore, by introducing consumer feedback and media and other influencing factors, constructing a dynamic and repeated game model with consumer participation, and studying the mechanism of the influence of various elements in the sales and service links of marine ranching enterprises on carbon emissions, in order to improve the regulation of carbon emissions in marine ranching Putting forward innovative suggestions will be our next research direction.
The raw data supporting the conclusions of this article will be made available by the authors, without undue reservation.
JL: data collection, processing, and thesis writing. MZ: build the model. ZY: article writing guide and project sponsor. KN, YL, SH, and JY: revision of the article. MX: revision of the article. All authors contributed to the article and approved the submitted version.
This research was funded by National Key R&D Program of China (2019YFD0901302) and Dalian Science and Technology Fund (2021JJ11CG001).
The authors declare that the research was conducted in the absence of any commercial or financial relationships that could be construed as a potential conflict of interest.
All claims expressed in this article are solely those of the authors and do not necessarily represent those of their affiliated organizations, or those of the publisher, the editors and the reviewers. Any product that may be evaluated in this article, or claim that may be made by its manufacturer, is not guaranteed or endorsed by the publisher.
Aragão, G. M., Saralegui-Díez, P., Villasante, S., López-López, L., Aguilera, E., and Moranta, J. (2022). The carbon footprint of the hake supply chain in Spain: accounting for fisheries, international transportation and domestic distribution. J. Clean. Prod. 360:131979. doi: 10.1016/j.jclepro.2022.131979
Bomze, I., and Weibull, J. (1995). Does neutral stability imply lyapunov stability. Games Econ. Behav. 11, 173–192. doi: 10.1006/game.1995.1048
China, (2022). Address to the General Debate of the Seventy-fifth United Nations General assembly, Beijing: Seventy-fifth United Nations General Assembly.
Dağtekin, M., Gücü, A. C., and Genç, Y. (2022). Concerns about illegal, unreported and unregulated fishing, carbon footprint, and the impact of fuel subsidy-an economic analysis of the Black Sea anchovy fishery. Mar. Policy 140:105067. doi: 10.1016/j.marpol.2022.105067
Du, Y., Sun, H., and Wan, X. (2022). Tripartite supervision mechanism and evolutionary strategies for marine ranching ecological security: policy tools perspective. Reg. Stud. Mar. Sci. 50:102125. doi: 10.1016/j.rsma.2021.102125
Friedman, D. (1991). Evolutionary games in economics. Econometrica 59, 637–666. doi: 10.2307/2938222
Gao, Y., and Gao, R. (2022). Research and analysis of environmental legal compensation mechanisms related to waste incineration in the context of “double carbon”. Front. Ecol. Evol. 10:979482. doi: 10.3389/fevo.2022.979482
Gao, K., Guo, X., Liu, T., and Han, R. (2022). Government low-carbon policies optimization for smart transportation enterprises. Discret. Dyn. Nat. Soc. 2022, 1–14. doi: 10.1155/2022/1099147
Gao, L., Yan, A., and Yin, Q. (2022). An evolutionary game study of environmental regulation strategies for marine ecological governance in China. Front. Mar. Sci. 9:1048034.doi: 10.3389/fmars.2022.1048034
Gonzalez-Azcarate, M., Cruz-Macein, J., and Bardaji, I. (2022). Certifications in short food supply chains in the region of Madrid. Part of the alternative? Ecol. Econ. 195:107387. doi: 10.1016/j.ecolecon.2022.107387
Grabowski, E., Letelier, R., Laws, E., and Karl, D. M. (2019). Coupling carbon and energy fluxes in the North Pacific subtropical gyre. Nat. Commun. 10:1895. doi: 10.1038/s41467-019-09772-z
Ha, X. (2000). Population, technology, and growth: from Malthusian stagnation to the demographic transition and beyond. Am. Econ. Rev. 90, 806–828. doi: 10.1257/aer.90.4.806
Han, J., and Jiang, T. (2022). Does the development of the digital economy improve carbon emission efficiency? Front. Ecol. Evol. 10:1031722. doi: 10.3389/fevo.2022.1031722
Haoyang, W., Lei, G., and Ying, J. (2022). The predicament of clean energy technology promotion in China in the carbon neutrality context: lessons from China’s environmental regulation policies from the perspective of the evolutionary game theory. Energy Rep. 8, 4706–4723. doi: 10.1016/j.egyr.2022.03.142
He, H., Zhang, S., and Zhu, L. (2021). Green product quality supervision strategy in online shopping with consumer evaluation and complaint. Front. Environ. Sci. 9:702151. doi: 10.3389/fenvs.2021.702151
Hussain, J., Pan, Y., Ali, G., and Xiaofang, Y. (2020). Pricing behavior of monopoly market with the implementation of green technology decision under emission reduction subsidy policy. Sci. Total Environ. 709:136110. doi: 10.1016/j.scitotenv.2019.136110
Jiao, L., Mengdi, L., Pihai, G., and Changtao, G. (2022). Research Progress on fishery carbon sinking associated with marine ranching. Progress Fish. Sci. 43, 142–150. doi: 10.19663/j.issn2095-9869.20220118002
Jinsheng, S., Zepeng, W., and Xuanqi, W. (2018). A study on incentive subsidy of blue carbon in marine ranch. J. Ocean Univ. China 25, 16–21. doi: 10.16497/j.cnki.1672-335x.2018.02.003
Khan, A., and Malik, M. (2020). Regulation, quality reporting and third-party certification of healthcare providers. J. Pak. Med. Assoc. 70, 1820–1827. doi: 10.47391/jpma.910
Kim, K., Kim, D.-H., and Kim, Y. (2021). Fisheries: a missing link in greenhouse gas emission policies in South Korea. Sustainability 13:5858. doi: 10.3390/su13115858
Konefal, J., and Hatanaka, M. (2011). Enacting third-party certification: a case study of science and politics in organic shrimp certification. J. Rural. Stud. 27, 125–133. doi: 10.1016/j.jrurstud.2010.12.001
Krause-Jensen, D., and Duarte, C. M. (2016). Duarte substantial role of macroalgae in marine carbon sequestration. Nat. Geosci. 9, 737–742. doi: 10.1038/ngeo2790
Kristófersson, D., Gunnlaugsson, S., and Valtysson, H. (2021). Factors affecting greenhouse gas emissions in fisheries: evidence from Iceland’s demersal fisheries. ICES J. Mar. Sci. 78, 2385–2394. doi: 10.1093/ICESJMS/FSAB109
Li, X., Dong, C., Guo, F., Yang, C., and Nie, B. (2021). Research on “internet plus” third-party participation model and method of coal mine safety supervision. Energy Sourc. Recov. Utiliz. Environ. Effect. 43, 1–16. doi: 10.1080/15567036.2021.1985018
Lilong, Z., Junmei, R., and Siyi, Z. (2022). Three-party evolutionary game and simulation analysis of drug quality supervision under the reward and punishment mechanism. Chin. J. Manag. Sci. 29, 55–67. doi: 10.16381/j.cnki.issn1003-207x.2019.0481
Liu, S., Xing, J., Westervelt, D. M., Liu, S., Ding, D., Fiore, A. M., et al. (2021). Role of emission controls in reducing the 2050 climate change penalty for PM in China.5. Sci. Total Environ. 765:144338. doi: 10.1016/j.scitotenv.2020.144338
Nie, Q., Zhang, L.-H., Tong, Z., and Hubacek, K. (2022). Strategies for applying carbon trading to the new energy vehicle market in China: an improved evolutionary game analysis for the bus industry. Energy 259:124904. doi: 10.1016/j.energy.2022.124904
Qin, M., and Sun, M. (2021). Effects of marine ranching policies on the ecological efficiency of marine ranching-based on 25 marine ranching in Shandong Province. Mar. Policy 134:104788. doi: 10.1016/j.marpol.2021.104788
Qin, M., Tao, Q., and Du, Y. (2021). Policy implementation and project performance: a qualitative comparative analysis based on 29 National Marine ranchings in China. Mar. Policy 129:104527. doi: 10.1016/j.marpol.2021.104527
Qin, M., Yue, C., and Du, Y. (2020). Evolution of China’s marine ranching policy based on the perspective of policy tools. Mar. Policy 117:103941. doi: 10.1016/j.marpol.2020.103941
Qu, G., Wang, Y., Xu, L., Qu, W., Zhang, Q., and Xu, Z. (2021). Low-carbon supply chain emission reduction strategy considering the supervision of downstream enterprises based on evolutionary game theory. Sustainability 13:2827. doi: 10.3390/su13052827
Sha, K. (2019). Incentive strategies for construction project manager: a common agency perspective. Constr. Manag. Econ. 37, 461–471. doi: 10.1080/01446193.2018.1554255
Shan, H., and Yang, J. (2019). Sustainability of photovoltaic poverty alleviation in China: an evolutionary game between stakeholders. Energy 181, 264–280. doi: 10.1016/j.energy.2019.05.152
Somanathan, E. (1997). Evolutionary stability of pure-strategy equilibria in finite games. Games Econ. Behav. 21, 253–265. doi: 10.1006/game.1997.0533
Sun, H., and Gao, G. (2022). Research on the carbon emission regulation and optimal state of market structure: based on the perspective of evolutionary game of different stages. RAIRO Operat. Res. 56, 2351–2366. doi: 10.1051/ro/2022088
Sun, H., Gao, G., and Li, Z. (2022). Evolutionary game analysis of enterprise carbon emission regulation based on prospect theory. Soft. Comput. 26, 13357–13368. doi: 10.1007/s00500-022-07527-5
Tan, S., Zhang, M., Wang, A., Zhang, X., and Chen, T. (2022). How do varying socio-economic driving forces affect China’s carbon emissions? New evidence from a multiscale geographically weighted regression model. Environ. Sci. Pollut. Res. 28, 41242–41254. doi: 10.1007/s11356-021-13444-1
Wan, X., Li, Q., Qiu, L., and Du, Y. (2021a). How do carbon trading platform participation and government subsidy motivate blue carbon trading of marine ranching? A study based on evolutionary equilibrium strategy method. Mar. Policy 130:104567. doi: 10.1016/j.marpol.2021.104567
Wan, X., Xiao, S., Li, Q., and Du, Y. (2021b). Evolutionary policy of trading of blue carbon produced by marine ranching with media participation and government supervision. Mar. Policy 124:104302. doi: 10.1016/j.marpol.2020.104302
Wang, Y.. (2014). Food safety supervision mechanism in Japan and its enlightment. Proceedings of 2014 International Conference on Public Administration (10th) China: University of Electronic Science & Technology China Press.
Wang, Q., and Wang, S. (2022). Carbon emission and economic output of China’s marine fishery - a decoupling efforts analysis. Mar. Policy 135:104831. doi: 10.1016/j.marpol.2021.104831
Wang, C., Wang, Z., Zheng, L., and Lu, S. (2022). Strategies for spatial analysis of carbon emissions from human-social systems: a framework based on energy consumption and land use. Front. Ecol. Evol. 10:990037. doi: 10.3389/fevo.2022.990037
Xue, J., He, Y., Gao, P., Tang, Y., and Xu, H. (2022). Multi-agent evolutionary game model: corporate low-carbon manufacturing, Chinese government supervision, and public media investigation. Sustainability 14:5587. doi: 10.3390/su14095587
Xu, H. (2008). Report on fishery industry energy conservation and emissions reduction research in China. Fish. Modern. 35, 1–7.
Yu, J., and Wang, Y. (2021). Exploring the goals and objectives of policies for marine ranching management: performance and prospects for China. Mar. Policy 122:104255. doi: 10.1016/j.marpol.2020.104255
Yu, Y., Yin, S., and Zhang, A. (2022). Clean energy-based rural low carbon transformation considering the supply and demand of new energy under government participation: a three-participators game model. Energy Rep. 8, 12011–12025. doi: 10.1016/j.egyr.2022.09.037
Zhang, M., Zhang, C., Kafy, A. A., and Tan, S. (2022d). Simulating the relationship between land use/cover change and urban thermal environment using machine learning algorithms in Wuhan city, China. Land. 11:14. doi: 10.3390/land11010014
Zhang, M., Zhang, Q., Zhou, D., and Wang, L. (2021). Punishment or reward? Strategies of stakeholders in the quality of photovoltaic plants based on evolutionary game analysis in China. Energy 220:119754. doi: 10.1016/J.ENERGY.2021.119754
Zhang, M., Kafy, A. A., Ren, B., Zhang, Y., Tan, S., and Li, J. (2022a). Application of the optimal parameter geographic detector model in the identification of influencing factors of ecological quality in Guangzhou, China. Land 11:1303. doi: 10.3390/land11081303
Zhang, M., Kafy, A. A., Xiao, P., Han, S., Zou, S., Saha, M., et al. (2022b). Impact of urban expansion on land surface temperature and carbon emissions using machine learning algorithms in Wuhan, China. Urban Clim. 47:101347. doi: 10.1016/j.uclim.2022.101347
Zhang, M., Tan, S., Pan, Z., Hao, D., Zhang, X., and Chen, Z. (2022c). The spatial spillover effect and nonlinear relationship analysis between land resource misallocation and environmental pollution: evidence from China. J. Environ. Manag. 321:115873. doi: 10.1016/j.jenvman.2022.115873
Zhao, T., and Liu, Z. (2019). A novel analysis of carbon capture and storage (CCS) technology adoption: an evolutionary game model between stakeholders. Energy 189:116352. doi: 10.1016/j.energy.2019.116352
Zhao, X., Xue, Y., and Ding, L. (2020). Implementation of low carbon industrial symbiosis systems under financial constraint and environmental regulations: an evolutionary game approach. J. Clean. Prod. 277:124289. doi: 10.1016/j.jclepro.2020.124289
Keywords: marine ranching, zero carbon, marine carbon sink, third-party testing facility, government supervision, tripartite evolutionary game
Citation: Liang J, Zhang M, Yin Z, Niu K, Li Y, Zhi K, Huang S, Yang J and Xu M (2023) Tripartite evolutionary game analysis and simulation research on zero-carbon production supervision of marine ranching against a carbon-neutral background. Front. Ecol. Evol. 11:1119048. doi: 10.3389/fevo.2023.1119048
Received: 08 December 2022; Accepted: 11 January 2023;
Published: 01 February 2023.
Edited by:
Junjie Lin, Chongqing Three Gorges University, ChinaCopyright © 2023 Liang, Zhang, Yin, Niu, Li, Zhi, Huang, Yang and Xu. This is an open-access article distributed under the terms of the Creative Commons Attribution License (CC BY). The use, distribution or reproduction in other forums is permitted, provided the original author(s) and the copyright owner(s) are credited and that the original publication in this journal is cited, in accordance with accepted academic practice. No use, distribution or reproduction is permitted which does not comply with these terms.
*Correspondence: Zengqiang Yin, ✉ enF5aW5AZGxvdS5lZHUuY24=
†These authors have contributed equally to this work and share first authorship
Disclaimer: All claims expressed in this article are solely those of the authors and do not necessarily represent those of their affiliated organizations, or those of the publisher, the editors and the reviewers. Any product that may be evaluated in this article or claim that may be made by its manufacturer is not guaranteed or endorsed by the publisher.
Research integrity at Frontiers
Learn more about the work of our research integrity team to safeguard the quality of each article we publish.