- 1U.S. Geological Survey, Wetland and Aquatic Research Center, Gainesville, FL, United States
- 2National Park Service, Everglades National Park, Homestead, FL, United States
- 3U.S. Geological Survey, Wetland and Aquatic Research Center, Davie, FL, United States
Understanding of the Everglades’ ecological vulnerabilities and restoration needs has advanced over the past decade but has not been applied in an integrated manner. To address this need, we developed the Everglades Vulnerability Analysis (EVA), a decision support tool that uses modular Bayesian networks to predict the ecological outcomes of a subset of the ecosystem’s health indicators. This tool takes advantage of the extensive modeling work already done in the Everglades and synthesizes information across indicators of ecosystem health to forecast long-term, landscape-scale changes. In addition, the tool can predict indicator vulnerability through comparison to user-defined ideal system states that can vary in the level of certainty of outcomes. An integrated understanding of the Everglades system is essential for evaluation of trade-offs at local, regional, and system-wide scales. Through EVA, Everglades restoration decision makers can provide effective guidance during restoration planning and implementation processes to mitigate unintended consequences that could result in further damage to the Everglades system.
1. Introduction
The Greater Everglades is a large (~ 47,000 km2; Gleason and Stone, 1994), unique sub-tropical wetland ecosystem in the southeastern United States. Before it was severely damaged by the construction of a network of canal and levee systems that drained water from the landscape, the Everglades was characterized by the slow movement of freshwater from the northern reaches of the system in central Florida to its eventual emptying into the Gulf of Mexico and Florida Bay (Douglas, 1947; Lodge, 2016). The slow movement of the water and slight changes in topography throughout the landscape facilitated the growth of wetland vegetation dependent on different hydrologic regimes and supported an abundance of iconic wildlife such as wading birds and alligators (Davis and Ogden, 1994). However, during the 1900s, much of the Everglades was drained to provide freshwater resources for agricultural development (National Academies of Sciences, Engineering, and Medicine (NASEM), 2018). This caused severe degradation of the ecosystem in the form of biodiversity loss, population declines of both animal and plant communities, declines in water quality, and saltwater intrusion into groundwater (Davis and Ogden, 1994, National Academies of Sciences, Engineering, and Medicine (NASEM), 2018). In 2000, the United States Congress passed a Water Resources Development Act which authorized the Comprehensive Everglades Restoration Plan (CERP) to build the infrastructure necessary to restore freshwater flow throughout the Everglades, especially to the southern portions of the system, to facilitate the recovery of the unique habitats historically present. CERP is one of the largest and most expensive restoration efforts in the world and its implementation requires extensive cooperation among stakeholders to ensure restoration efforts are successful (LoSchiavo et al., 2013).
Ecological models facilitate evaluation and assessment of potential impacts of restoration on the Greater Everglades system (REstoration COordination and VERification (RECOVER), 2020). Currently, decision makers must consider the output of models for each species or habitat individually when deciding on water management scenarios that need to meet a diversity of stakeholder needs. These outputs are often generated at different scales and are not easily comparable to one another. Additionally, the uncertainty around these model outputs is either unavailable or not considered when comparing across management scenarios (National Academies of Sciences, Engineering, and Medicine (NASEM), 2021; Romañach and Pearlstine, 2021). RECOVER (Restoration Coordination & Verification), a team of scientists and representatives of key Everglades stakeholders, identified the need to address uncertainty and integration of ecological models in a recent Five-Year Plan (REstoration COordination and VERification (RECOVER), 2016). This Plan recognized that the understanding of the Everglades’ ecological vulnerabilities and restoration needs has greatly advanced over the past decade but that this understanding has yet to be applied in a system-wide, integrated manner. Here, we define vulnerability as the degree to which a system or part of the system is damaged or harmed resulting from environmental stressors or disturbances. Through RECOVER’s Five-Year Plan, the need emerged for an analytical tool that could connect various models into a common framework and spatial scale. In addition, the tool would need to provide a system-wide response to stressors or restoration actions to facilitate CERP implementation in the face of an uncertain future due to climate change or funding constraints. Generating outputs that are on a common temporal and spatial scale would increase the ability of Everglades stakeholders to assess trade-offs between prioritizing different habitats or species more effectively when choosing between restoration plans for implementation (Romañach et al., 2022).
Bayesian networks (BNs) are used extensively in the development of decision support tools and analyses to investigate environmental risk, project impacts from restoration projects or development, and forecast the potential impacts of disturbances such as recreation, fire, and climate change (McCann et al., 2006; Kaikkonen et al., 2020). BNs produce model outputs as the probability of outcomes generated by influence diagrams and conditional probability tables (CPTs; Scutari and Denis, 2014). BNs are an ideal modeling framework to meet RECOVER’s needs because of their flexibility, connectivity, and ability to be easily updated. BNs are flexible in that it is easy to incorporate data from different sources such as monitoring, experimental, or expert knowledge into a single network (Landuyt et al., 2013). Individual BNs can be connected to one another through common or latent nodes, allowing a modular building of larger networks that can facilitate collaboration among groups of researchers (Marcot and Penman, 2019). BNs are built using Bayes’ Theorem, where prior knowledge of the system is utilized to generate output, and thus regular updating using new data is built into the modeling process (Koski and Noble, 2009).
We constructed a flexible, modular, and easily updated decision support tool known as the Everglades Vulnerability Analysis (EVA) to address the system-wide evaluation and assessment needs of RECOVER, who will use this information to communicate the expected ecological impact of restoration plans to managers and decision makers. This tool takes advantage of the extensive modeling work already done in the Everglades and synthesizes information across indicators of ecosystem health to forecast long-term, landscape-scale changes. In addition, the tool calculates a vulnerability score for these indicators by comparing model outputs to ideal system states defined by the user. Uncertainties in both the model outputs and the ideal states are incorporated into the calculation of the vulnerability score; uncertainty within the ideal states can in turn be adjusted to explore how limiting uncertainty tolerance impacts user conclusions and decision making. Here, we describe the EVA tool and illustrate its utility as a decision support tool through example outputs.
2. Materials and methods
2.1. Study area
The EVA tool focuses on the managed areas of the southern Everglades system which includes parts of Big Cypress Seminole Indian Reservation, the Miccosukee Federal Indian Reservation, Water Conservation Areas (WCA-1, 2A, 2B, 3A, and 3B), Big Cypress National Preserve (BCNP), and Everglades National Park (ENP; Figure 1). Water is managed on these lands for a variety of uses including flood control, municipal water supply, and wildlife use (LoSchiavo et al., 2013). Several hydrologic models are commonly used in the southern Everglades to predict and evaluate water stage and depths across the landscape. These models include the Everglades Depth Estimation Network (EDEN; Palaseanu and Pearlstine, 2008; Haider et al., 2020), the Biscayne and Southern Everglades Coastal Transport (BISECT; Swain et al., 2019) model, and the Regional Simulation Model (RSM; South Florida Water Management District (SFWMD), 2005). EDEN is an interpolation of a network of water gages across the system that measure water stage and water depth (by subtracting elevation) at a 400 meter resolution. The footprint of this model extends from the northern Water Conservation Areas (WCAs) down into ENP but does not reach to the coast. BISECT is a physical simulation model of hydrologic conditions for the southern coast of Florida stretching up to the southern edge of WCA-3A and WCA-3B and parts of Big Cypress National Preserve. Because BISECT provides hydrology along the coast and can generate estimates of salinity, it is the hydrologic model used when exploring impacts on the coastal Everglades. The RSM is a finite volume surface water/groundwater model that extends from above Lake Okeechobee down to the southern coast of Florida. The RSM is used by Everglades managers and decision makers to explore the long-term trends in hydrology resulting from planned construction of new water management structures or water management strategies. To maintain consistency among the three models when using EVA, the BISECT and RSM models were each converted to a 400 meter grid that aligns with EDEN. The original BISECT model operates on a 500 meter grid and was resampled using bilinear interpolation, while the original RSM operates on a variable mesh grid and was converted to an orthogonal 400 meter grid aligning with EDEN. Converting each of these hydrologic models to a common spatial grid can streamline the modeling process, but we acknowledge that in some areas, finer-scale information may be lost. Because much of the data used to build the current network modules came from within ENP, we focus on EVA output from within the ENP boundary for this manuscript.
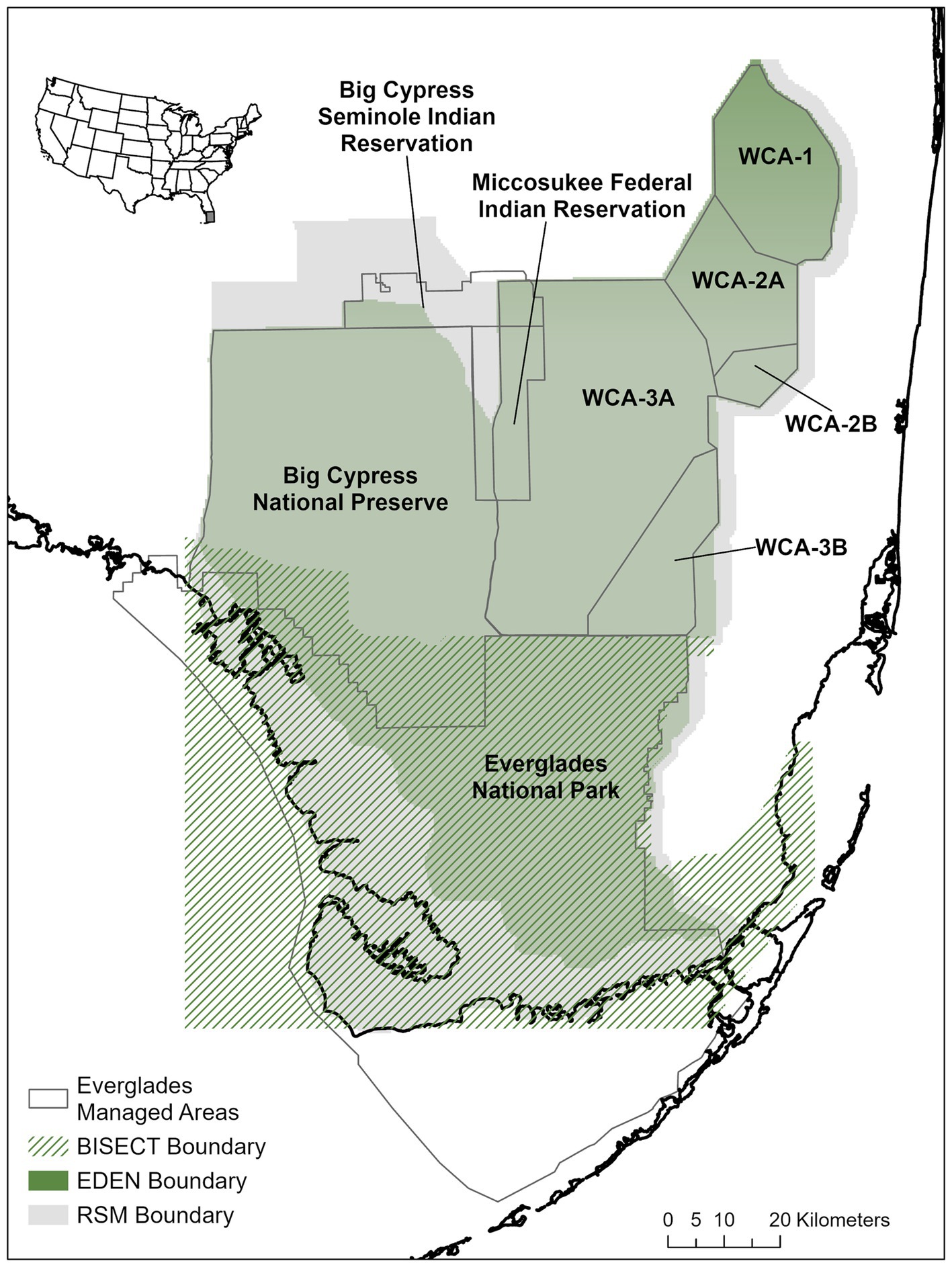
Figure 1. Spatial extent of the managed areas of the Everglades and the hydrologic models that the Everglades Vulnerability Analysis (EVA) can use as inputs. WCA = Water Conservation Area; BISECT = Biscayne and Southern Everglades Coastal Transport; EDEN = Everglades Depth Estimation Network; and RSM = Regional Simulation Model.
2.2. Everglades vulnerability analysis modules and data
Everglades Vulnerability Analysis is a series of connected Bayesian networks, where each ‘module’ represents an individual indicator of ecosystem health. By building the tool using a modular framework, we can easily add new indicators to the tool in the future. Each module predicts spatially and temporally explicit outcomes on a 400 meter resolution on an annual basis. Because we built EVA to work with any of the three main hydrologic models used in the Everglades, EVA can predict annual outcomes based on historical or predicted daily hydrologic conditions. Currently, EVA models four indicators of Everglades ecosystem health: (1) vegetation type; (2) American alligator nesting potential; (3) wading bird colony size; and (4) sawgrass peat accumulation. Each module was selected because of its significance as an indicator of overall Everglades ecosystem functionality, as models that predict both vegetation and key wildlife responses are regularly used by RECOVER to assess potential restoration impacts (for an example, see REstoration COordination and VERification (RECOVER), 2020). All modules except for the sawgrass peat accumulation module were built using long-term monitoring data. Because we did not have long-term monitoring data of sawgrass peat accumulation across the landscape, we instead used observations of peat accumulation reported in the literature from experimental data to build this module. The vegetation module predicts the probability of six vegetation types across the landscape. It was built using several different sources of vegetation observations (n = 4,256) across the freshwater and coastal Everglades from 2000 to 2017 collected by the National Park Service (NPS; Sah et al., 2018; Ruiz et al., 2021). The American alligator nesting potential module predicts the probability of alligator nesting on the landscape. The American alligator nesting potential module was built using alligator nest locations (n = 1,439) from Systematic Reconnaissance Flight (SRF) surveys in Everglades National Park from 1992 to 2018. Because absence of nests in the SRF survey is much more frequent than presence we randomly sampled from the set of nest absences (collected during the SRF surveys) to create a presence-absence dataset totaling 2,878 observations. The wading bird colony size module predicts the probability of different size classes of wading bird colonies across the landscape. The wading bird module was built using wading bird colony sizes from 2000 to 2017 reported in the South Florida Water Management District’s annual wading bird nesting reports1 (n = 376). The sawgrass peat accumulation module predicts the probability of sawgrass peat accretion or subsidence classes across the landscape. The sawgrass peat accumulation module was built using sawgrass peat accretion or subsidence observations derived from within the literature (Craft and Richardson, 1998; Macek and Rejmankova, 2007; Wilson, 2018; Charles et al., 2019).
2.3. Building the Bayesian network modules
Bayesian networks are built first by defining an influence diagram which represents how the researchers believe the system operates. The influence diagram can be defined using nodes and arcs in a directed acyclic graph (DAG) by the researcher, algorithms that use data to learn the network structure, or a combination of both (Scutari and Denis, 2014). The relationships between variables that are connected in the DAG are defined by conditional probability distributions. These distributions are most efficient when variables are discretized into bins, and the conditional probabilities are defined in conditional probability tables (Koski and Noble, 2009). To build each EVA module, we first identified the variables that likely influenced the outcome (vegetation type, alligator nesting potential, wading bird colony size, or sawgrass peat accumulation). The Everglades community has developed several conceptual diagrams which explain how the greater ecosystem processes propagate through the system, and we used these conceptual diagrams (e.g., the Trophic Hypothesis, Trexler and Goss, 2009) along with the breadth of Everglades modeling literature (e.g., Shinde et al., 2014; Sah et al., 2018; Flower et al., 2019; Gibble et al., 2020; D’Acunto et al., 2021) to identify input variables for each module within EVA. Using the published literature and data exploration, we discretized each variable into no more than six classes to avoid creating unnecessarily complicated CPTs (Marcot et al., 2006; Chen and Pollino, 2012). Each module’s CPT was fit in the R package bnlearn (Scutari, 2010). After defining the module’s CPTs, we explored each module’s structure by assessing the prior probability distributions of each node. These distributions describe the range of, and most common conditions present within, the network module (Koski and Noble, 2009). The conceptual framework used to build each model (Figure 2) details the general process we went through to develop each module, assess it, and then predict it onto the whole landscape. Specific details for each module follow in the next sections and the R code used to build the modules and predict across the landscape are available at D’Acunto et al. (2023).
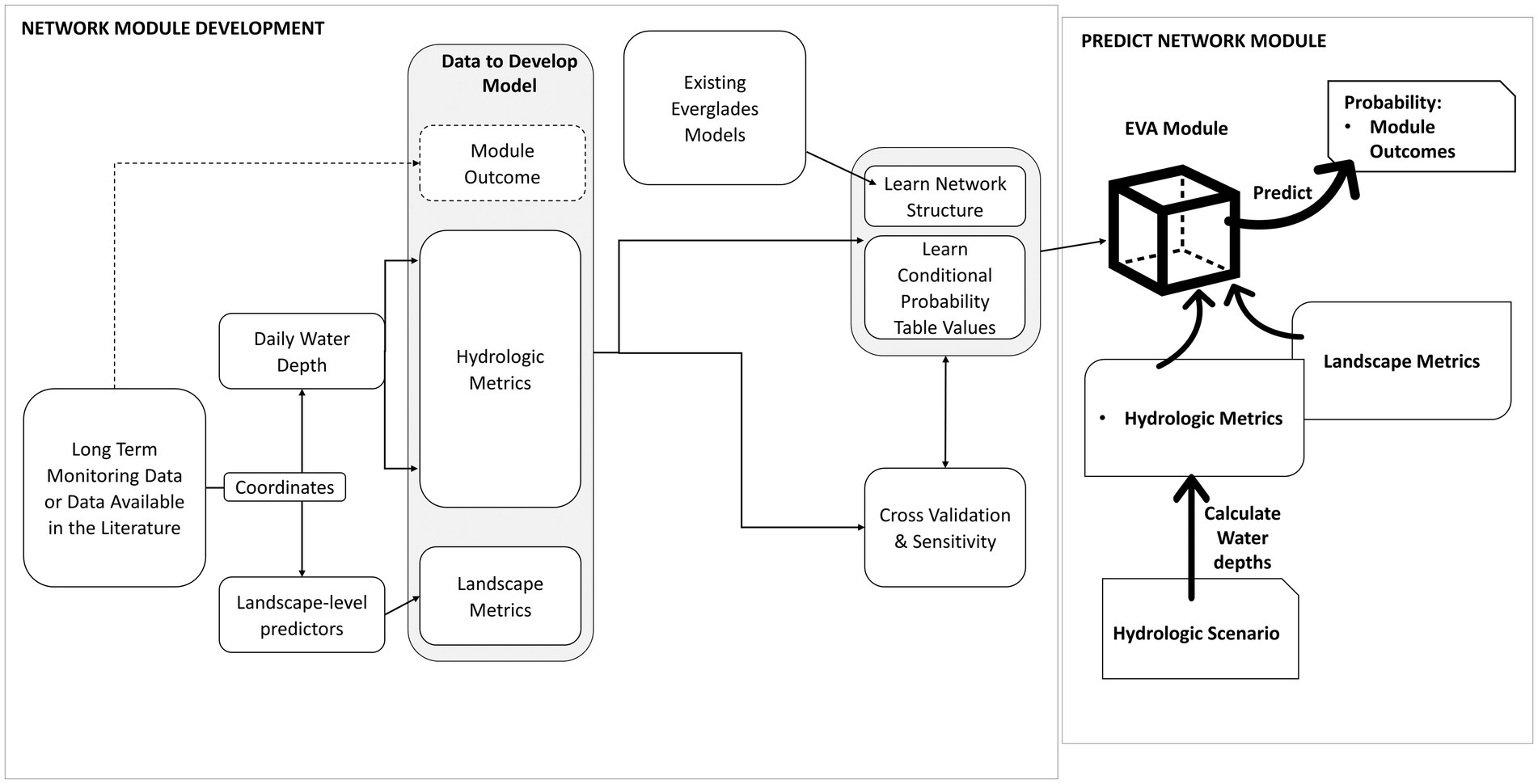
Figure 2. Conceptual framework detailing how each Bayesian network module was built and then predicted across the landscape. The left-hand box describes the process used to develop the module, including assessing module accuracy and sensitivity. The right-hand box describes the process used to predict the module outcomes across the landscape.
2.4. Vegetation type module
We chose eight variables to influence vegetation type on the Everglades landscape (Figure 3). Hydrologic metrics were generated as inputs to the vegetation model from EDEN interpolations (Haider et al., 2020) or the BISECT hydrodynamic model (Swain et al., 2019), dependent of where the observations fell within the spatial domain. We calculated hydrometrics for climatic years, May 1 of the current year – April 30 of the next calendar year. Four-year average hydrometrics were generated from the annual hydrometrics because Ross et al. (2004) reported improved correlation of vegetation response to a 4-year hydroperiod rather than just the current year’s hydroperiod. Mangrove species along the coastal Everglades and sawgrass are vegetation types in the system that additionally respond to salinity and disturbances. We used salinity modeled by the hydrologic model BISECT to characterize the salinity stress within each 400 meter grid cell. Distance to coastline was used to provide a proxy measure to both nutrients (e.g., phosphorous loads; Childers et al., 2006; Dessu et al., 2018) and disturbance from sea level rise which can impact vegetation succession and establishment across the landscape (Romañach et al., 2018). We extracted the environmental variables described in Supplementary Table S1 at each observation for the year it was sampled. Each variable was discretized into 4 to 6 classes (Supplementary Table S1). We aggregated vegetation communities into six freshwater marsh and coastal communities: Freshwater prairie, Mangrove, Mangrove scrub, Open water, Sawgrass, and Upland. Vegetation categories were aggregated according to the crosswalk table located in the Supplementary material. Once the data were discretized according to our defined categories, we determined both the network structure and values within the CPT using the R package bnlearn (Scutari, 2010). We used a hill-climbing (HC) score-based structure learning algorithm (Russell and Norvig, 2009) to fully construct the network using the available data. All eight nodes were forced to connect to vegetation type, while the algorithm produced additional connections in the network between correlated nodes. The CPT values were determined using Bayesian parameter learning (method ‘bayes’ within the bnlearn package).
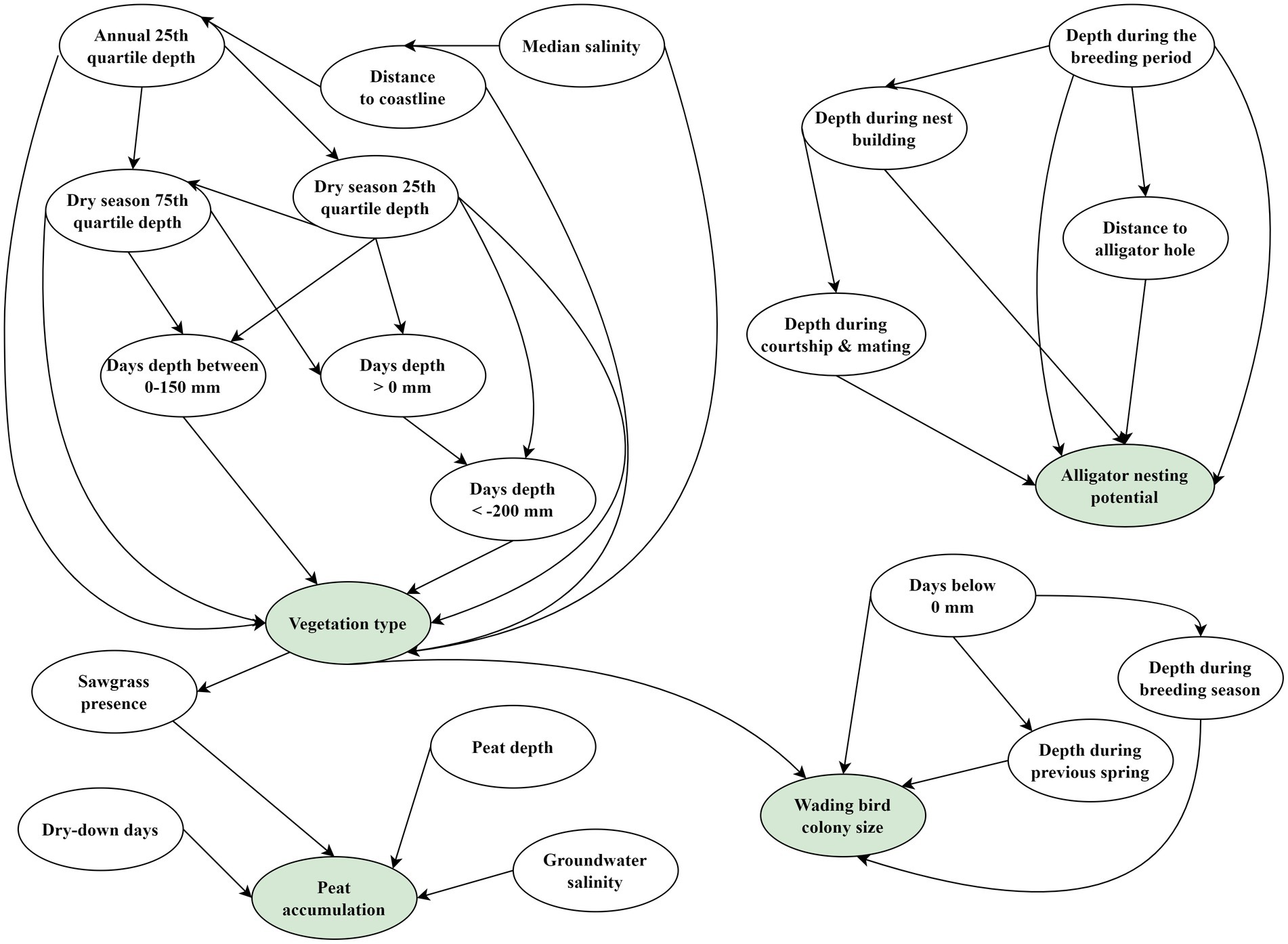
Figure 3. Structure of the Bayesian network modules currently used in the Everglades Vulnerability Analysis (EVA). Green shaded nodes are output nodes, while white non-shaded nodes are variable input nodes. For descriptions of each node and the discretization levels used, see Supplementary Table S1 in Supplementary material.
2.5. American alligator nesting potential module
The alligator module was developed based on a previous modeling effort known as the Alligator Production Suitability Index model (APSI; Shinde et al., 2014) which uses expert knowledge to determine how hydrology within the Everglades impacts the species at different times during their seasonal cycles. To produce young, alligators require slightly different conditions on the landscape depending on the time of the year (or timing during the breeding cycle). Conditions during the mating period and conditions during nest building can influence where and if alligators build nests. Therefore, the module contained three hydrologic variables that describe each of these conditions on the landscape (Figure 3). Depth during the breeding period influences female alligator body condition; excessively deep waters (> 122 cm) can reduce food availability and cause stress (Dalrymple, 1996a; Dalrymple, 1996b; Barr, 1997), while excessively shallow waters (< 15 cm) can severely limit an alligator’s ability to move through the marsh (Rice et al., 2004). Depth during the courtship and mating period was included because alligators typically need relatively deep, open water for mating (Newsom et al., 1987; Rice et al., 2004). During the nest building season, alligators require shallower depths typically within proximity to deeper ponds known as alligator holes (Newsom et al., 1987; Fleming, 1990). Thus, we also included the distance to known alligator hole as a variable to predict alligator nest presence. Hydrologic variables (depth during the breeding period, depth during nest building, and depth during courtship and mating) were extracted for each observation from EDEN. We calculated distance to alligator holes from a point dataset generated from several sources of survey data spanning WCA-1 (Mazzotti et al., 2004), WCA-2 (Mazzotti et al., 1999), WCA-3 (Campbell and Mazzotti, 2004), and ENP (Rice and Mazzotti, 2006, 2007). Each variable was discretized into 3–4 classes, and we determined both the network structure and values within the CPT using the R package bnlearn (Scutari, 2010; Supplementary Table S1). We used a HC score-based structure learning algorithm (Russell and Norvig, 2009) to fully construct the network using the available data. All variables were forced to connect to nest presence, while the algorithm produced additional connections in the network between correlated nodes. We constructed the CPT values using Bayesian parameter learning (method ‘bayes’ within the bnlearn package).
2.6. Wading bird colony size module
Wading bird colony size is influenced by three hydrologic variables and one landscape variable (Figure 3). Water depth during the breeding season reflects the current year’s general foraging conditions. Wading birds require water depth ranges based on species-specific leg lengths and foraging strategies of the species (Gawlik, 2002; Beerens et al., 2015). Water depth during the previous year’s spring (March 1–May 31) reflect past hydrologic conditions that can impact the populations of fish and crayfish available in the breeding season to support nestlings (Botson et al., 2016; van der Heiden and Dorn, 2017). The number of days that water depth is less than 0 cm over the last three years is indicative of the level of long-term drought across the landscape, which can impact prey availability and habitat type (Todd et al., 2010). Finally, vegetation type present on the landscape serves as an indicator of the availability of nesting substrate. Wading birds typically use woody vegetation such as mangroves, hardwood hammock, or shrub scrub types for nesting. Each variable was discretized into 2–6 classes, and we determined both the network structure and values within the CPT using the R package bnlearn (Scutari, 2010; Supplementary Table S1). We used a HC score-based structure learning algorithm (Russell and Norvig, 2009) to fully construct the network using the available data. All variables were forced to connect to wading bird colony size, while the algorithm produced additional connections in the network between correlated nodes. We constructed the CPT values using Bayesian parameter learning (method ‘bayes’ within the bnlearn package).
2.7. Sawgrass peat accumulation module
The sawgrass peat accumulation module is influenced by two hydrologic variables (dry-down days and salinity), whether sawgrass peat is present at the site, and the current depth of the peat layer (Figure 3; Supplementary Table S1). Salinity intrusion and the amount of drought the site experiences are known drivers of peat subsidence in the system (Wilson et al., 2018; Chambers et al., 2019; Charles et al., 2019). Because we focused on sawgrass peat accumulation, we added a node that indicated whether sawgrass peat was currently present on the landscape. We used the predicted probability of the Sawgrass vegetation type from the vegetation module to generate three states. A site with a predicted Sawgrass probability of > 50% was classified as “Sawgrass.” Sites with < 50% probability of Sawgrass but were predicted to be Sawgrass in the first year of the simulation were classified as “Has Sawgrass Peat.” Otherwise, sites were classified as “Not Sawgrass.” Finally, we binned the peat depth variable so that the classes would reflect where on the landscape peat was deep enough to subside. The threshold for the two classes is established at 5 cm to reflect the limited precision of the input, a spatially interpolated soil depth data layer. We created the starting soil depth for the model using universal kriging to estimate soil depth at a 400 meter resolution for management areas across the EDEN and BISECT footprints with data from the Environmental Protection Agency’s Everglades Regional Environmental Monitoring and Assessment Program (REMAP; U.S. Environmental Protection Agency (USEPA), 2020) and 264 additional soil depth field estimates provided by L. Lamb-Wotton (personal communication). We used soil depth from 687 coordinate point locations across all sampling years which ranged from 1964 to 2018 and occurred in all three WCAs and ENP. We calculated mean soil depth when multiple depths were documented at the same location. We examined soil depth within each management area for normality and used a cube root transformation on the data to normalize the distribution. We also observed spatial trends in soil depth, and therefore we examined easting and northing as covariates when kriging. We used the ‘autoKrige’ function from the R package automap (Hiemstra et al., 2008) to fit variograms with easting and northing and to perform kriging. We selected the best fitting model, back-transformed, and bias corrected interpolated depths (Gregoire et al., 2008) and used the kriged depths to create the starting soil depth layer. The values for the 6 expected sawgrass peat accretion bins were estimated from 6 field sites with observations found in the literature (Craft and Richardson, 1998). We determined the mean accretion or subsidence rate and associated standard deviation expected for each category within the CPT from the literature (Table 1). When conditions favored peat accretion (low salinities, peat depth > 5 cm, and little drought), we used a value of 0.15 ± 0.09 cm/year, which was a yearly accretion observed in the literature with these conditions (Craft and Richardson, 1998). During conditions of drought and high salinities, we used subsidence values of −2 or − 4 cm/year with a standard deviation of ± 0.75. We manually populated the peat accretion CPT node by generating a normal probability distribution from the values in Table 1 and calculating the probability that each value fell within our defined bins. All other nodes in the CPT were set as equally likely (probabilities split evenly across each bin) as we did not have adequate information to design more precise CPTs. The CPTs were defined manually within bnlearn to match the framework of the other nodes in EVA.
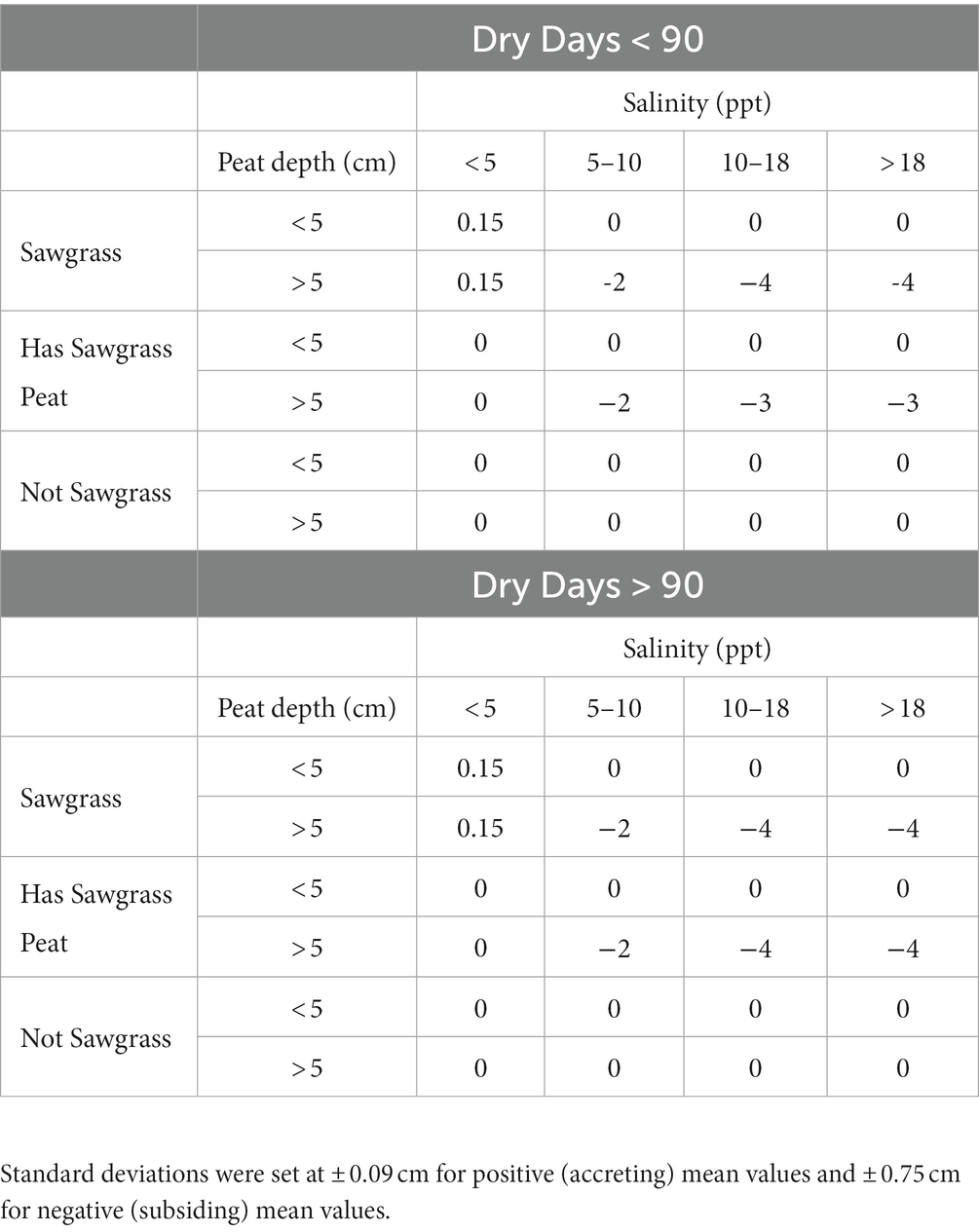
Table 1. Mean sawgrass peat accumulation values (cm) used in computation of joint conditional probabilities.
2.8. Module accuracy and sensitivity
Module accuracy was not explicitly assessed for the sawgrass peat accumulation module, as we did not have access to a long-term dataset for assessment. For the modules built using long-term monitoring data (vegetation type, American alligator nesting potential, and wading bird colony size), we assessed the network’s accuracy by calculating the posterior classification error rate using a 10-fold cross-validation process (Marcot, 2012; Marcot and Hanea, 2021), where we randomly assigned each observation to one of 10 folds. The network was then re-fit 10 times, each time holding one of the folds out of the data set. The fold held out of the fitting process was used as a testing dataset, where we predicted the probability of each outcome bin using the fitted network. Network predictions were generated with the R package bnlearn using Bayesian likelihood weighting and 500 iterations. Bayesian likelihood weighting performs Monte Carlo simulations of the network to produce a stochastic sample of probability predictions of the node chosen for prediction. The class with the highest probability is therefore the chosen predicted class. We calculated the posterior classification error rate through the ‘bn_cv’ function in the R package bnlearn; rates closer to zero indicate a more accurate model. We also calculated the multi-class Brier score (Brier, 1950) to assess the network’s overall ability to predict the correct class. The Brier score measures how close a predicted probability is to the observed class but averages across all possible outcomes. Therefore, it considers the certainty of an outcome along with whether the class was predicted accurately. Multi-class Brier scores range from 0 to 2, with scores closest to zero indicating a model with less error and uncertainty. We assessed the ability of the module to accurately predict each outcome by constructing Receiver Operating Characteristic (ROC) curves and area under the curve (AUC) values (Fielding and Bell, 1997). To explore network sensitivity, we performed a one-way sensitivity analysis on each module by comparing the range of predicted probabilities of the outcome bins across the range of possible input node values. This was done iteratively, where only one input node’s values were changed at a time and the network was adjusted via proportional covariation so that we could assess each node’s impact on the outcome independently (Leonelli and Riccomagno, 2022). Nodes that produced the largest range in probabilities of an outcome bin were considered the most important nodes for predicting that outcome.
2.9. Module output and uncertainty
We visualized the output of all modules by using the BISECT hydrodynamic model to predict each module for a dry, average, and wet water depth year between 2000 and 2017 (2014 was the dry year, 2007 was the average year, and 2003 was the wet year). We predicted the probability of all outcomes using the bnlearn R package on the landscape and mapped the outcome with the highest predicted probability. We assessed spatial uncertainty for all modules by calculating the Posterior Probability Certainty Index (PPCI; Marcot, 2012). This index is a measure of the evenness of the posterior probabilities of each binned outcome; a more even distribution of probabilities would indicate a high level of uncertainty because there would be no clear category that is most probable.
2.10. Mapping vulnerability
We translated the American alligator nesting potential, wading bird colony size, and sawgrass peat accumulation module predictions for the dry, average, and wet years into surfaces of vulnerability. The vegetation type module was not translated into a surface of vulnerability, but its output feeds into both the wading bird colony size and sawgrass peat accumulation modules. We chose not to develop vulnerability surfaces for the vegetation module because there is not a clear landscape-wide favored vegetation type for restoration. We built vulnerability surfaces by first defining a set of target site outcomes and comparing those target sites to the predicted network outcomes from BISECT. Each network module’s output is a set of bins that are each assigned a probability based on inputs to the network. For example, the wading bird colony size module has three outcomes: ‘0–10 birds’, ‘10–100 birds’, and ‘> 100 birds’. We may define a target site as one where the most likely outcome is the ‘> 100 birds’ bin. If we want to define absolute certainty in the target outcomes, we could set our target site to have a 0 probability in all bins other than ‘> 100 birds’ and set a probability of 1 to the ‘> 100 birds’ bin. However, we know there is considerable uncertainty in this system and that other bins such as the ‘10–100 birds’ bin could be considered acceptable in some areas of the landscape by managers or decision makers. To capture this variation, we generated 1,000 theoretical target sites by simulating outcome bin probabilities from a Dirichlet distribution, where we defined each shape parameter α according to the desired probability of that outcome for a target state (Table 2). In practice, these probabilities will be set by the user of the tool and the probabilities used here are for illustration purposes only. The distance between the target sites and the actual outcomes of the model were compared by first conducting a Principal Components Analysis (PCA; Jolliffe and Cadima, 2016) on a combined dataset of both the ideal sites and network outcomes. A PCA is an ordination process which takes a multivariate set of data and collapses the variables into new, orthogonal variables known as principal components. We then used the first two principal components as coordinates within the variable space and calculated an average distance between the network outcomes and the target sites. We standardized this distance to scale from 0 to 1 so that values closer to 1 indicate higher vulnerability. In this sense, higher vulnerability areas (closer to 1) are areas that are furthest from the user-defined target and at most risk of not meeting that target.
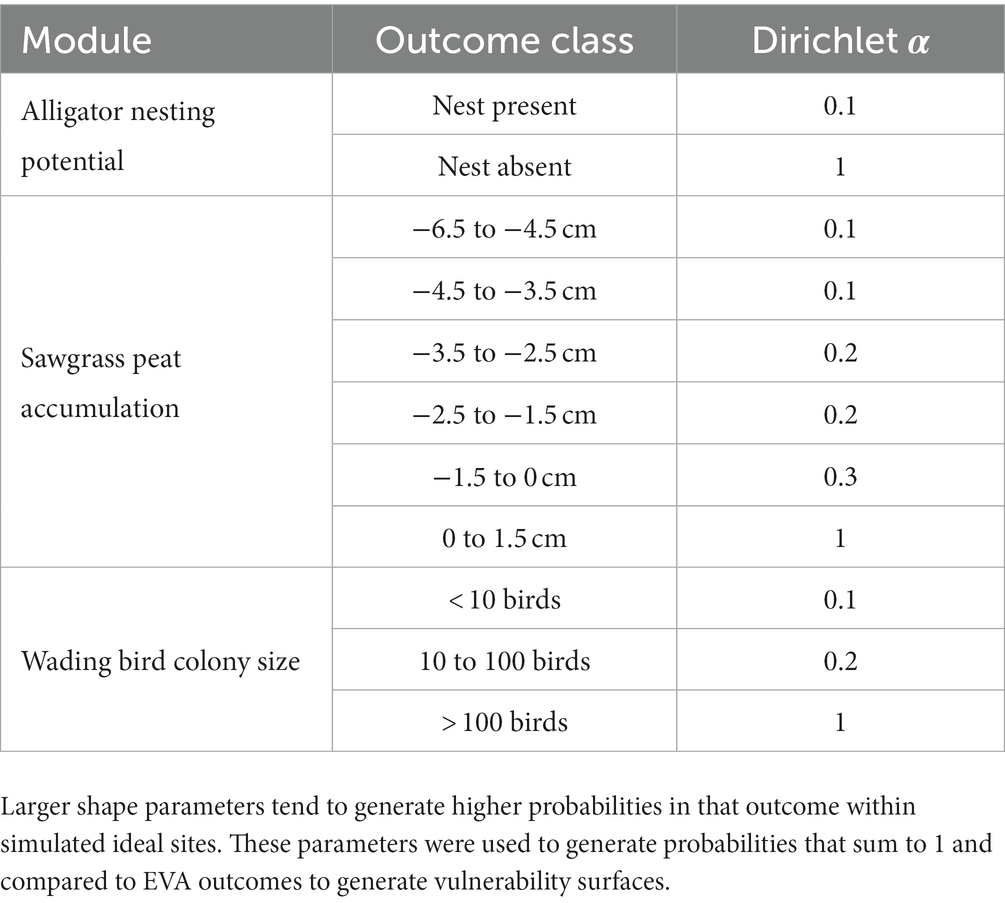
Table 2. Dirichlet shape parameters (α) assigned to each outcome for the alligator nesting potential, sawgrass peat accumulation, and wading bird colony size modules in EVA.
3. Results
We examined the structure, accuracy, certainty, and sensitivity in addition to how the calculated vulnerability changes over time for each EVA module.
3.1. Module prior probabilities
The prior probability distribution for the vegetation type module exhibits a diverse range of conditions across both the input and output nodes (Supplementary Figure S1). The most likely vegetation type outcome given all conditions in the data set is mangrove scrub (p = 28.6%), followed by open water and sawgrass types (p = 20.9% and p = 20.2%, respectively). For the American alligator nesting potential module, the outcomes of whether a nest is present or not are almost tied at p = 50.1% for nests being absent in a cell versus p = 49.9% for nests being present in a cell (Supplementary Figure S2). The prior probabilities for the rest of the input variables in the alligator nesting potential module tend to skew heavily towards one or two bins. For the wading bird colony size module, greater than 10 but less than 100 birds was the most likely outcome (p = 40.3%). The prior probabilities for the rest of the wading bird colony size module input variables were spread across the bins, but the vegetation type node had low prior probabilities of both mangrove and freshwater prairie types (Supplementary Figure S3). Because we built the peat accumulation module from information gleaned from a small number of observations and not long-term monitoring data, we assigned uninformative prior probabilities to the network module for the input nodes (Supplementary Figure S4). The most probable accumulation value is therefore subsidence between 4.5 and 3.5 cm (p = 70.1%).
3.2. Module accuracy and sensitivity
The modules we could assess error rates for (vegetation type, alligator nesting potential, and wading bird colony size) exhibited low to moderate mean posterior classification error rates (0.291–0.441) and low mean Brier scores (0.368–0.584; Table 3). Individual outcome mean AUC scores for the vegetation type module revealed that the module was fairly successful at discriminating between classes (all AUC scores > 0.70). The module was able to discriminate open water most successfully with a mean AUC score of 0.922 and was least successful at discriminating upland habitat with a mean AUC score of 0.719. The alligator nesting potential module was successful at discriminating between nest presence and absence with a mean AUC of 0.759. The wading bird colony size module showed a low ability to discriminate low and moderate numbers of birds (mean AUC score < 0.70) but was more successful at discriminating areas with large numbers of wading birds (Table 3). The one-way sensitivity analyses revealed which input nodes were the strongest influence on the outcome node probabilities (Table 4). The vegetation type module was most sensitive to the number of days the depth was greater than 0 mm and the annual 25th quartile depth. The alligator nesting potential module was most sensitive to depths during the breeding season and during courtship and mating. The wading bird colony size module was most sensitive to the depth during the breeding season and vegetation type. The sawgrass peat accumulation module was most sensitive to the sawgrass presence and current peat depth.
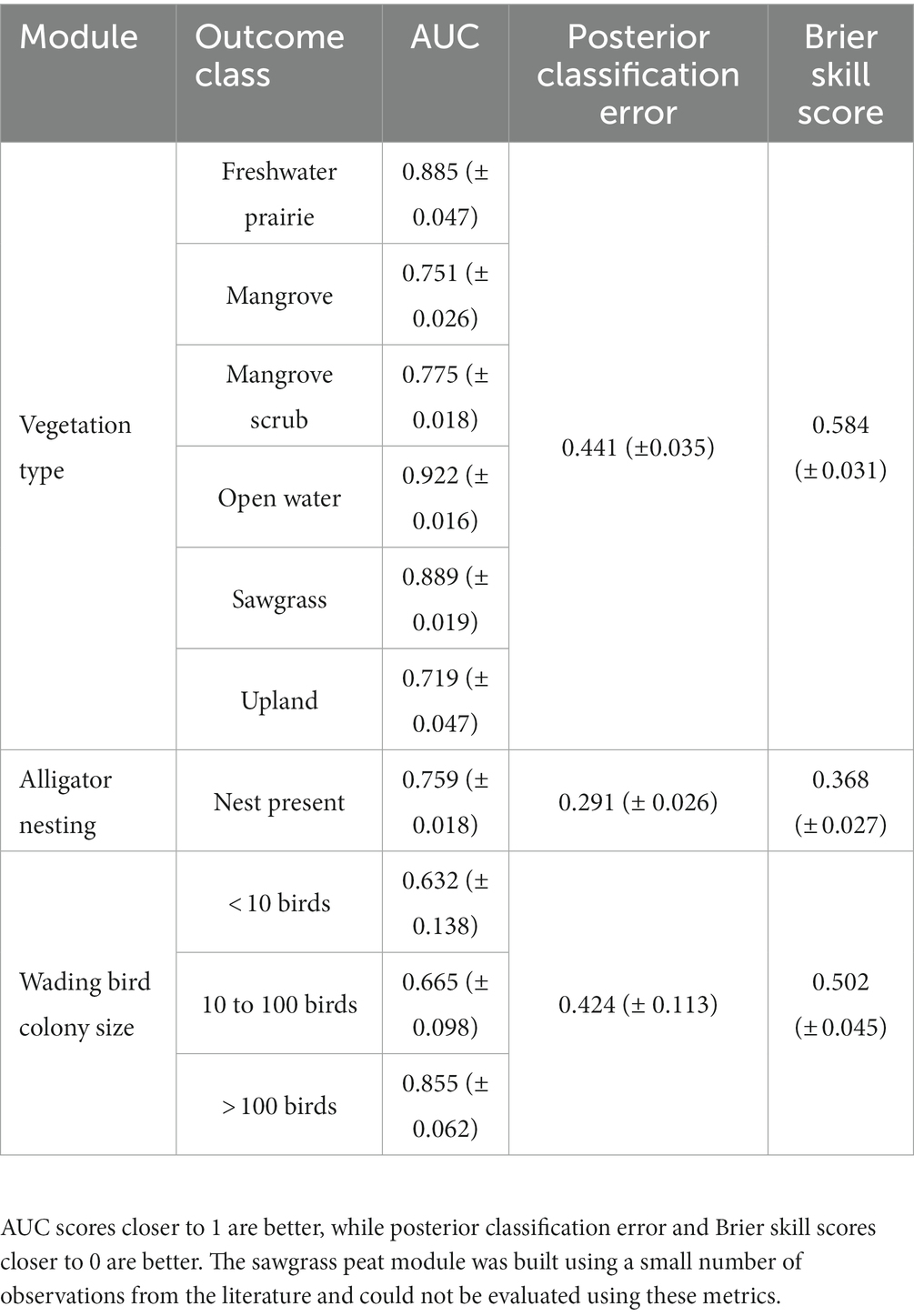
Table 3. Accuracy measures (AUC, posterior classification error, and Brier skill score) for three of the four Everglades vulnerability analysis (EVA) modules.
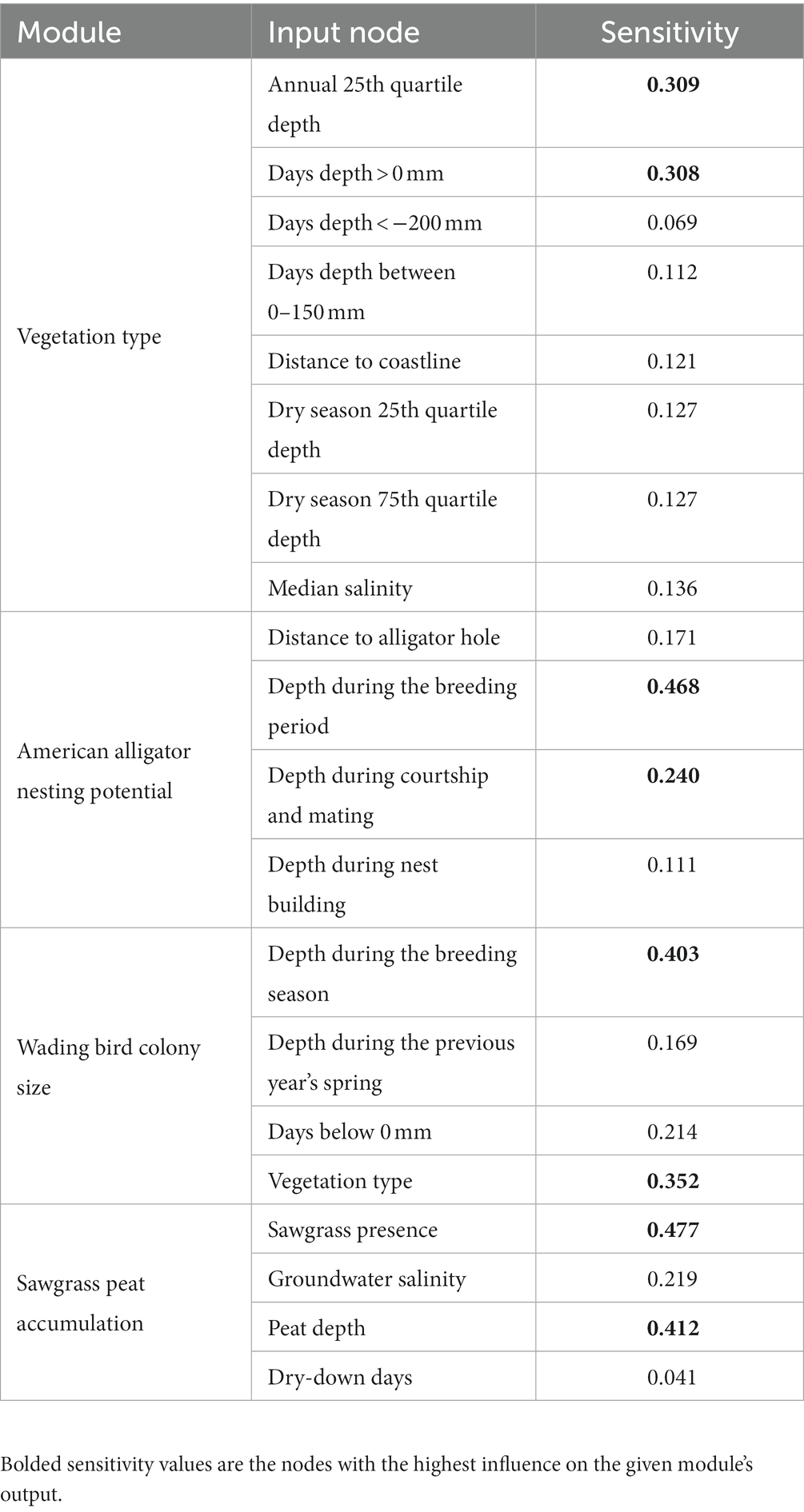
Table 4. One-way sensitivity analysis results for each Everglades vulnerability analysis (EVA) module’s input nodes.
3.3. Module output and uncertainty
The vegetation module responded to hydrologic changes on the landscape, notably much of the landscape predicted as mangrove in the dry year is replaced by mangrove scrub in the average and wet year. The vegetation module PPCI maps showed highest certainty in areas consistently predicted as sawgrass, whereas the northern center of the landscape was consistently uncertain (Figure 4). The alligator nesting potential module showed lower nesting potential in the dry year compared to the average and wet years and was most certain along the coastal areas (Figure 5). The wading bird colony size module showed greatest numbers of nesting wading birds along the coastal areas, with an expanded footprint during the wet year. The PPCI maps showed generally lower certainty across the landscape (Figure 6). The sawgrass peat accumulation module showed peat subsidence along the coast, with a greater footprint of subsidence during the dry year than the average or wet year. The PPCI map revealed generally high certainty, with the highest certainty occurring along coastal areas (Figure 7).
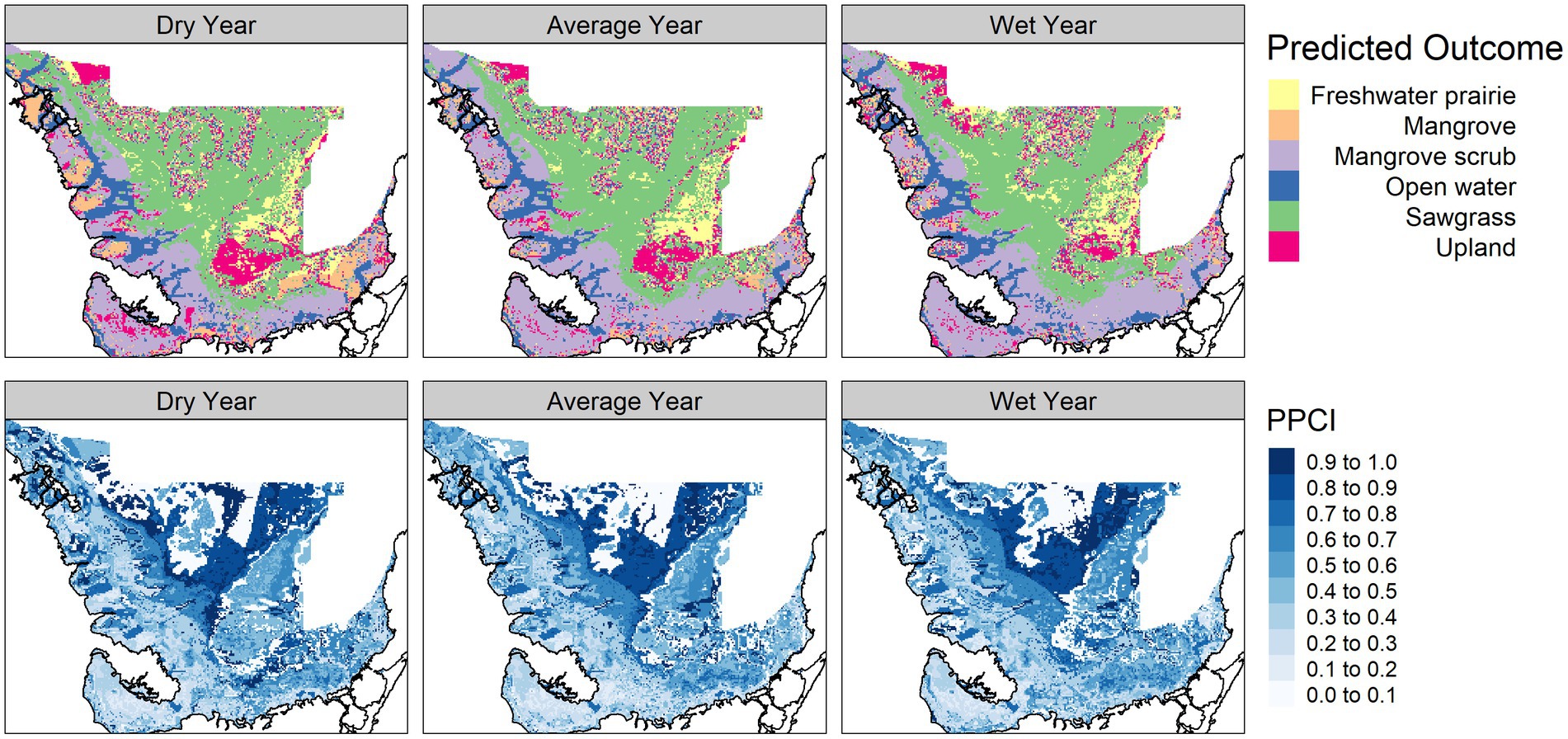
Figure 4. Predicted vegetation type module outcome (top panel) and posterior probability certainty index (PPCI, bottom panel) for a dry (2014), average (2007), and wet year (2003) chosen from 2000–2017.
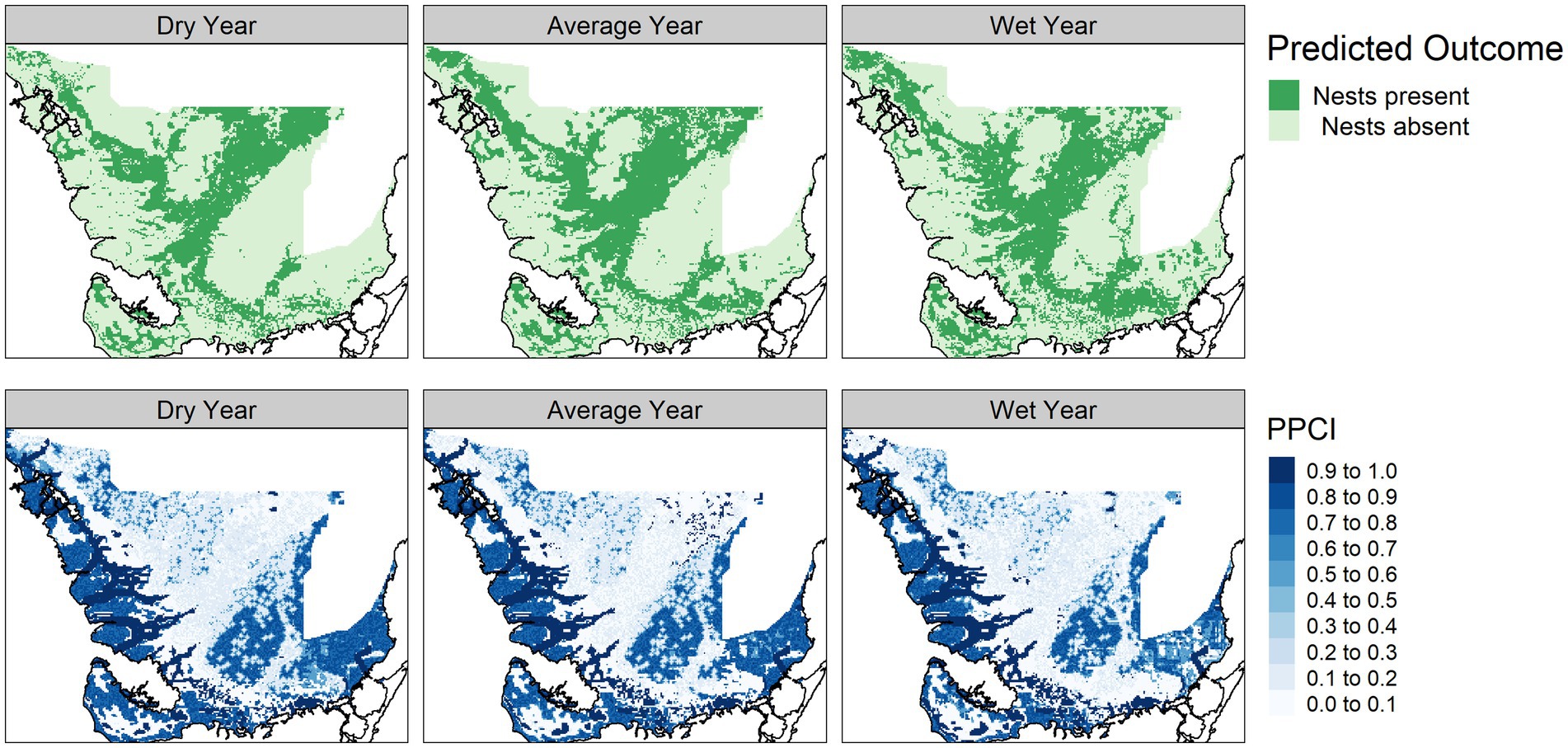
Figure 5. Predicted alligator nesting potential module outcome (top panel) and posterior probability certainty index (PPCI, bottom panel) for a dry (2014), average (2007), and wet (2003) year chosen from 2000 to 2017.
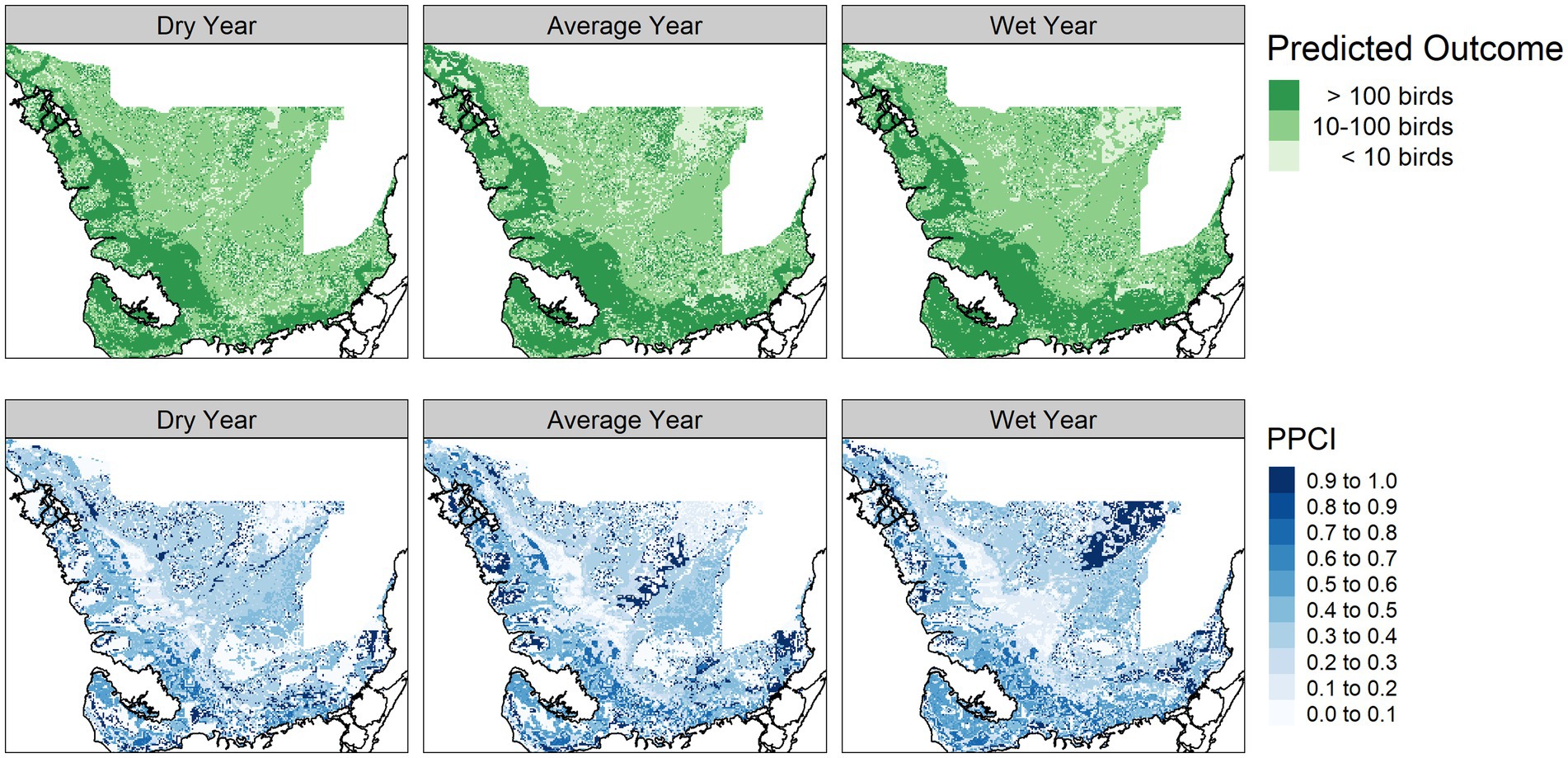
Figure 6. Predicted wading bird colony size module outcome (top panel) and posterior probability certainty index (PPCI, bottom panel) for a dry (2014), average (2007), and wet (2003) year chosen from 2000–2017.
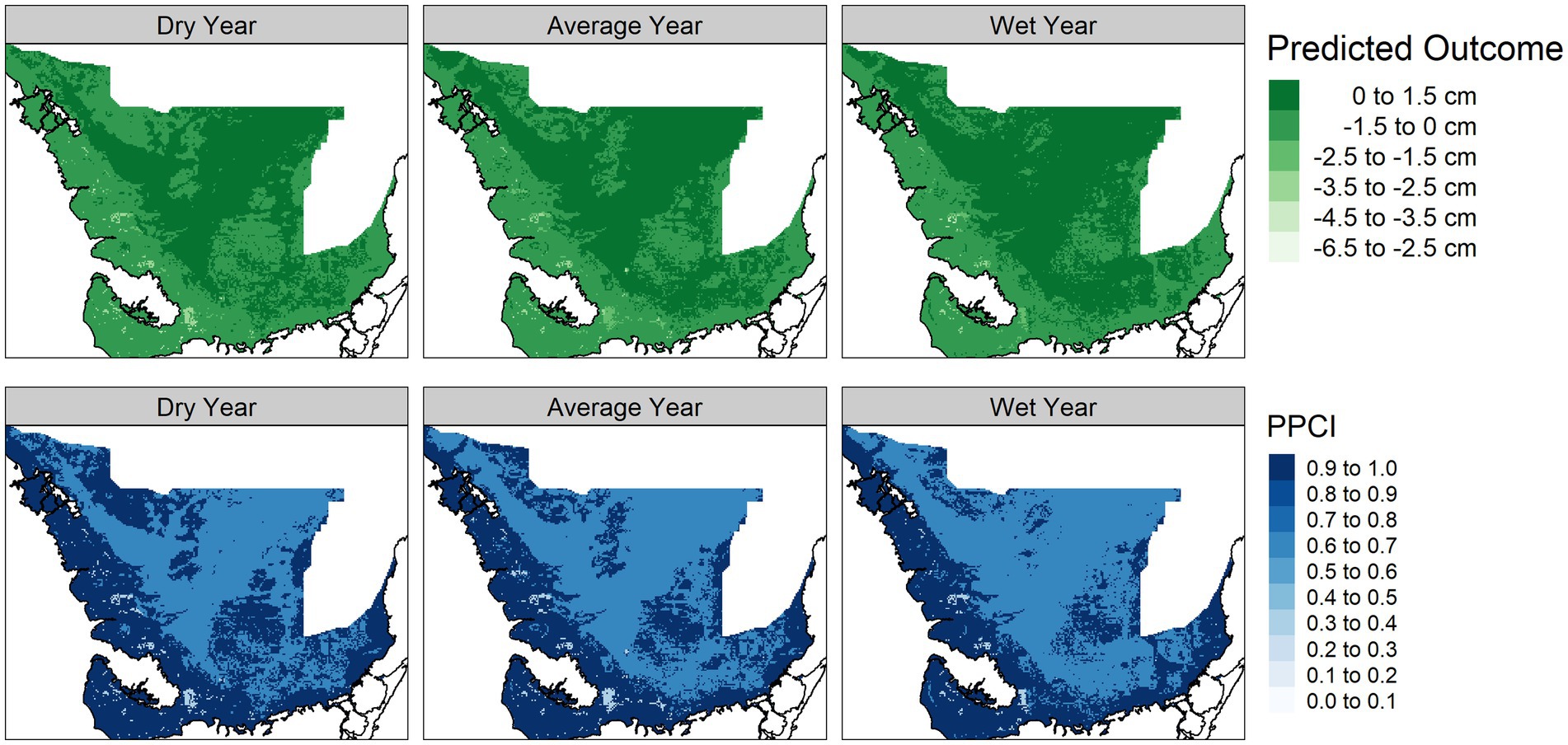
Figure 7. Predicted sawgrass peat accumulation module outcome (top panel) and posterior probability certainty index (PPCI, bottom panel) for a dry (2014), average (2007), and wet (2003) year chosen from 2000–2017.
3.4. Visualizing vulnerability
Areas that were most vulnerable on the landscape varied across the network modules (Figure 8). The alligator module showed most vulnerability along the northwest coast and the southeast interior of the landscape, while the wading bird module showed the most vulnerability in the northern portions of the landscape. The sawgrass peat accretion module revealed the most vulnerability in small sections of the southern and northwestern coast. When viewed across time, we saw that in general, alligator nesting vulnerability remained relatively stable over time, but the sawgrass peat accretion and wading bird modules both increased in vulnerability over time (Figure 9).
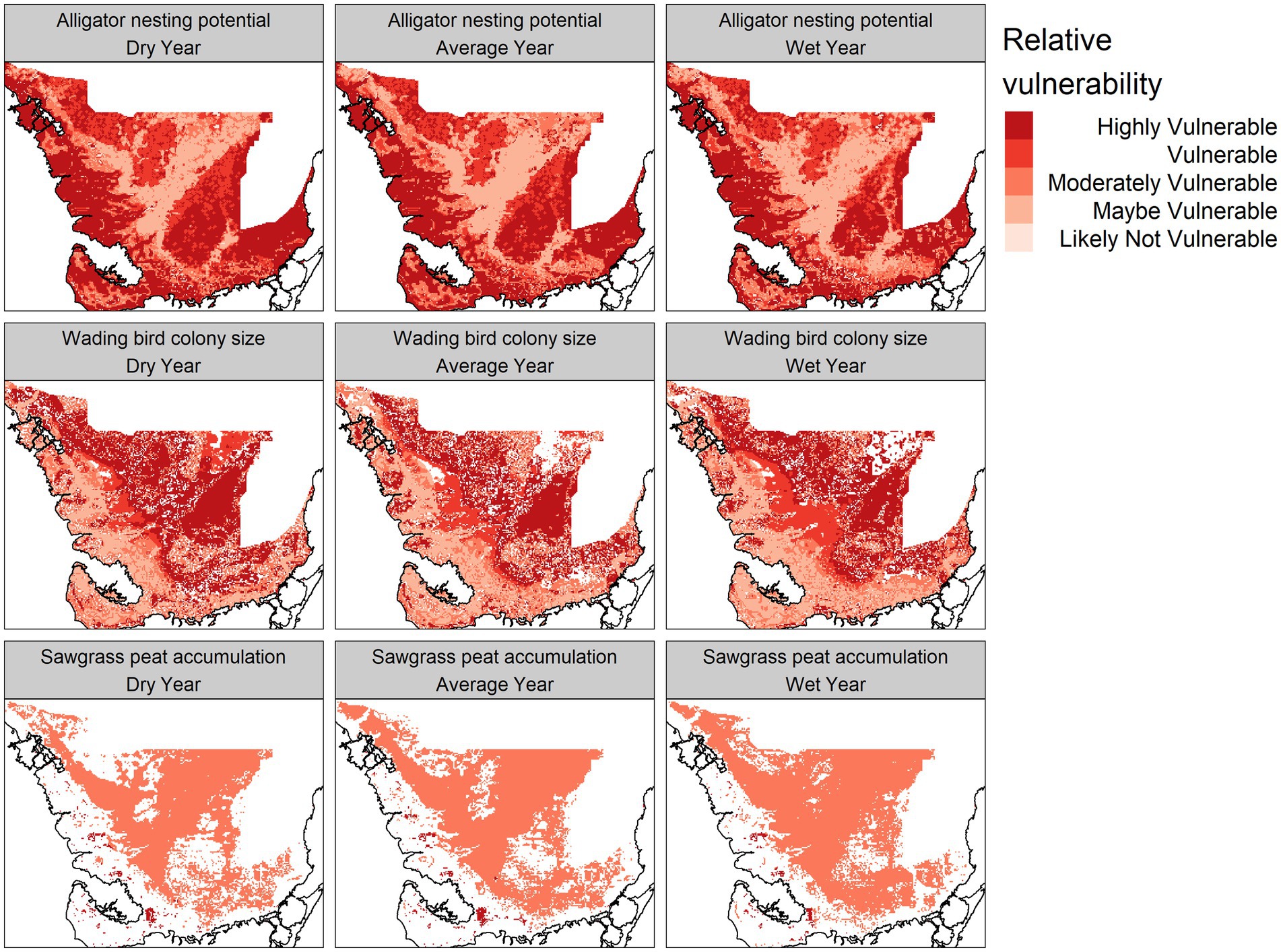
Figure 8. Predicted vulnerability (distance from a user-defined target state, standardized from 0 to 1) of the alligator nesting potential, wading bird colony size, and sawgrass peat accumulation modules for a dry (2014), average (2007), and wet (2003) year chosen from 2000 to 2017.
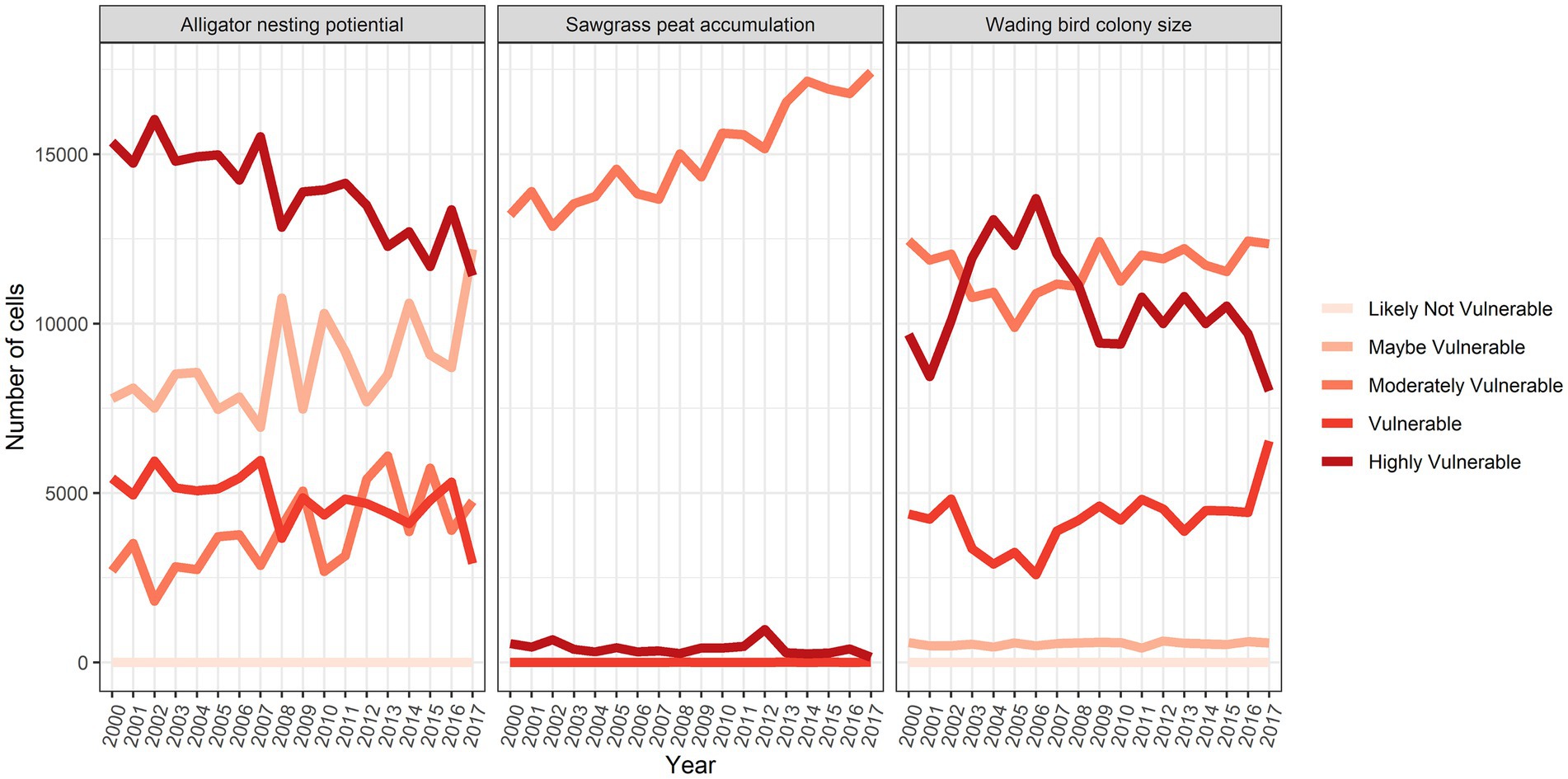
Figure 9. Number of grid cells that fell into each vulnerability category in each year across the landscape.
4. Discussion
The Everglades is a spatiotemporally dynamic ecosystem that is managed for wildlife and human needs that may sometimes conflict (Romañach et al., 2018). In these conflicting scenarios, it is important for decision-makers to be able to view indicators of the ecosystem’s health and vulnerability in a system-wide manner (REstoration COordination and VERification (RECOVER), 2016). EVA accomplishes this task by providing a framework for the prediction of ecological outcomes and translating these outcomes to surfaces of vulnerability for consideration by decision-makers.
Everglades vulnerability analysis is built using Bayesian network modules which have several advantages over other modeling efforts currently used for decision-making. Additional modules (created as separate or connected Bayesian networks) can be added to EVA that represent additional indicators of ecosystem health. Here we present four indicators of ecosystem health (vegetation type, alligator nesting potential, wading bird colony size, and sawgrass peat accumulation), but additional indicators may be needed to consider EVA a truly system-wide tool. Bayesian networks can be easily updated and refined as new information (such as monitoring data) becomes available, and this process is specifically built into the theoretical underpinnings of the model (Koski and Noble, 2009). Updating EVA’s network module prior probabilities using new or newly available data can improve outcome accuracy and certainty (Marcot and Hanea, 2021). EVA’s framework makes it possible to integrate different forms of knowledge within the Everglades system. For example, we have incorporated experimental data alongside monitoring data in this current iteration of EVA. The modeling framework necessitates the inclusion of uncertainty in module outcomes even when built from non-empirical data, allowing assessment of uncertainty to be standard across all modules. Finally, EVA generates surfaces of vulnerability as compared to ideal states of the ecosystem which can be defined as historical conditions or acceptable conditions, dependent on the user’s priorities.
Everglades vulnerability analysis’s modeling framework characteristics (simple updating, integrating different forms of knowledge, modeling of uncertainty) make it an attractive tool for application in other regions and ecosystems. Although most of our modules are built using years of empirical knowledge, our inclusion of sawgrass peat accumulation is an example of the ability to weigh less well-understood criteria in the vulnerability evaluation by explicit incorporation of uncertainties, along with a framework for updating the module as our knowledge improves. This approach is especially useful for projects that involve large-scale, long-term restoration efforts where there are multiple conflicting needs. Using flexible, modular Bayesian networks, we demonstrate a pathway to connect decades of monitoring and modeling work into a new decision support tool that can be updated regularly with new science.
The modules exhibited varying degrees of overall accuracy. The vegetation and wading bird colony size modules exhibited moderate rates of error. Vegetation patterns in the Everglades are likely driven by both environmental factors and competition between plant species with overlapping niches (O’Reilly-Nugent et al., 2018). This complexity makes predicting the location of vegetation types with precision difficult, especially at a 400 meter resolution. The overall pattern of vegetation types across the landscape predicted by the network does reflect the general patterns observed through mapping exercises using aerial imagery (Ruiz et al., 2021), and this similarity is reflected in the mean AUC scores for each individual outcome class in the module. Currently, EVA does not incorporate time-lags in vegetation type switching and vegetation types switching within 1 year could be unrealistic. It is important to note that the vegetation module predicts vegetation type based on the previous 4 years of hydrologic conditions, which helps keep vegetation patterns realistic even if the module allows vegetation types to switch within 1 year. The wading bird colony size module showed difficulty discriminating between low and moderate numbers of birds but was better able to identify areas with large numbers of birds. Even in years with low wading bird colony sizes, there are few birds present in colonies across the landscape which may make it difficult for the network to differentiate between poor and moderate conditions for wading birds (Frederick et al., 2009; Essian et al., 2022). However, the network was more successful at identifying areas with > 100 birds in a colony with accuracy and therefore can identify conditions that are ideal for wading birds. The alligator nesting potential module exhibited a low rate of error. The higher accuracy for this module is likely because the module output is split into only two classes. The sawgrass peat accretion module was built using experimental results and we were unable to assess the module for accuracy, but we were able to assess the module’s uncertainty.
Modules generated with monitoring data were much more uncertain, especially the alligator nesting potential and wading bird colony size modules. The vegetation module exhibited moderate uncertainty, while the sawgrass peat accumulation module exhibited the lowest uncertainty. These results show the trade-offs between using different sources of data for building Bayesian networks. The alligator nesting potential and wading bird colony size modules were built using datasets collected via aerial surveys prone to issues of detection probability (Conroy et al., 2008; Ugarte et al., 2013; D’Acunto et al., 2021). In addition, the counts of wading birds from these monitoring data may be inconsistently biased across years due to differing observer counting biases (Crozier and Gawlik, 2003). In contrast, the vegetation module showed higher levels of certainty in its predictions. The vegetation data used to build the network came from on-the-ground surveys of vegetation and therefore are less prone to detection issues than the alligator and wading bird modules. Uncertainty in the vegetation module may also be related to the mismatch in spatial scale of the output and the monitoring data. More than one vegetation type is likely present in each 400 meter grid cell, but the network is forced to choose just one type. Generating annual predictions at a smaller spatial scale currently would present computational issues with generating output, so some amount of uncertainty is likely to remain in the network even when additional observations are added. While the sawgrass peat accumulation module was most certain, we were unable to assess its accuracy on the landscape, and therefore it is important to exercise caution about the module’s output despite its high certainty. Uncertainty was also likely introduced into each module through the hydrologic models used to provide water depth data inputs. The Everglades can exhibit vastly different water depths dependent on relatively small changes in elevation, but a 400 meter resolution may obfuscate these areas during an interpolation process. Because the goal of building this modeling framework is to synthesize system-wide trends across the landscape, we settled on a spatial resolution that allowed us to do so without needing prohibitively long simulation times. Ultimately, we chose to accept some amounts of uncertainty within EVA’s output to be able to visualize multiple ecosystem health indicators in a common framework on a common spatio-temporal scale. Regardless of the source of the uncertainty, the dynamic nature of the Everglades ecosystem introduces uncertainty that can be missed when using only deterministic output or ignoring statistical uncertainty. Without addressing uncertainty explicitly during the modeling process, restoration planners are missing important information that could impact the decision they ultimate make.
The sensitivity analysis and the visualizations of the module outcomes for a representative dry, average, and wet year showed that each module responds to changes within the landscape. The input nodes producing the largest range of outcomes in the vegetation type, alligator nesting potential, and wading bird colony size modules all were associated with water depth on ecologically relevant temporal scales. This is not surprising for the Everglades system as most of the restoration goals for the system hinge on the correct timing and amount of water across the landscape (Davis and Ogden, 1994). In contrast, the sawgrass peat accumulation node was most sensitive to the presence of sawgrass or the existing peat depth on the landscape. The values of these two nodes determine whether sawgrass peat can accumulate (sawgrass presence) and how much is available to subside if subsidence is the outcome (peat depth). Currently, sawgrass presence is determined by the most probable outcome of the vegetation module. We know that there is sometimes considerable uncertainty associated with this module’s outcomes. Given that the sawgrass peat accumulation module is most sensitive to the sawgrass presence input, it is important to use caution when interpreting the results of the sawgrass peat accumulation module for decision-making exercises such as evaluating whether a restoration plan will meet user-defined targets.
We used an ordination process to calculate the distance from model outcomes to user-defined target states to generate vulnerability surfaces. This approach is unique in that it acknowledges that a decision maker could accept a range of targets in addition to the uncertainty present within the network outcomes alone. Adjusting the uncertainties within the target states can help decision makers or managers explore how changing their uncertainty tolerance can impact the resulting vulnerability surface and thus ultimately impact their decisions. Changes in vulnerability because of restoration can be compared to a baseline scenario to determine whether the restoration plan results overall in a less vulnerable landscape for that module, which is an approach already commonly implemented in Everglades decision making (REstoration COordination and VERification (RECOVER), 2020). EVA would improve on this effort by providing output on a common spatial and temporal scale for every indicator. Modules can be built to reflect what are currently known as performance measures, which are targets used to assess the success of CERP projects (LoSchiavo et al., 2013). EVA can then assess how well each module meets its target or performance measure within the uncertainty bounds set by the user. Allowing the user to define acceptable levels of uncertainty in target states for different modules not only provides a way for decision makers to assess risk to an indicator (Parrish et al., 2003), but also illustrates areas of the system that may need to be prioritized for future monitoring or research. The Bayesian framework also facilitates an iterative process where modules can be updated when new information is made available, thus reducing uncertainty and generating more confident predictions with each iteration (Brudvig and Catano, 2021). EVA’s framework allows for a continuous feedback loop between modeling and monitoring research that can accommodate the diversity of science occurring on the landscape.
We emphasize that EVA is not a replacement for all Everglades modeling. EVA uses relationships identified through decades of research and modeling efforts to generate a common framework to explore annual, system-wide trends on a landscape scale. EVA is not meant to necessarily identify new mechanisms or relationships between module outcomes and environmental conditions but is meant to synthesize this knowledge into a framework and set of outputs that assess whether indicators of Everglades ecosystem health are meeting user-defined targets. For example, new understandings of the drivers of wading bird colony size could emerge from further scientific research that could then be incorporated into an updated module in EVA. While EVA provides important visualizations and synthesis of the system-wide trends, it is still dependent on robust monitoring and scientific investigation across the landscape and indeed is improved by continued work in these areas.
Everglades vulnerability analysis currently models four indicators of ecosystem health, but likely needs additional modules to be considered a fully system-wide decision support tool. We built EVA using a modular framework to facilitate the addition of modules representing the suite of Everglades ecosystem indicators (Doren et al., 2009). Module updates and additions such as increasing the number of vegetation types predicted by the vegetation module, including other peat soil sources beyond sawgrass, and predicting small prey fish density across the landscape are potential immediate avenues for improvement. Another consideration for future improvement is the incorporation of feedback loops in the Bayesian network. One such example would be that the sawgrass peat accumulation or subsidence over time will change the hydrologic metrics driving much of the other modules within the spatial extent through changes in the soil depth. Without these improvements, EVA is still a useful tool that provides potential landscape-scale outcomes for key indicators of Everglades ecosystem health and provides the certainty associated with those outcomes in a common framework. This tool is a necessary step toward considering uncertainty and system-wide perspectives in Everglades restoration.
Data availability statement
The data presented in the study are deposited in the ScienceBase repository, https://doi.org/10.5066/P9JPVPGV
Ethics statement
Ethical review and approval was not required for the animal study because all data used was collected by other entities, no handling or data collection of animals was completed by the authors.
Author contributions
LD’A, LP, and SR conceived of the idea and managed the project. LD’A, LP, SH, CH, and DS developed the models. SH and CH streamlined the model code. LD’A performed the accuracy and sensitivity analyses and wrote the manuscript with input from all authors. All authors contributed to the article and approved the submitted version.
Funding
This work was funded by the United States Army Corps of Engineers (agreement W32CS512325164).
Acknowledgments
We thank the members of RECOVER who remained engaged in the model development process of this work and provided feedback throughout its development. We also thank L. Lamb-Wotton for sharing 558 additional soil depth observations. Any use of trade, product, or firm names is for descriptive purposes only and does not imply endorsement by the United States Government.
Conflict of interest
The authors declare that the research was conducted in the absence of any commercial or financial relationships that could be construed as a potential conflict of interest.
Publisher’s note
All claims expressed in this article are solely those of the authors and do not necessarily represent those of their affiliated organizations, or those of the publisher, the editors and the reviewers. Any product that may be evaluated in this article, or claim that may be made by its manufacturer, is not guaranteed or endorsed by the publisher.
Supplementary material
The Supplementary material for this article can be found online at: https://www.frontiersin.org/articles/10.3389/fevo.2023.1111551/full#supplementary-material
Footnotes
References
Barr, B. (1997). Food habits of the American alligator, Alligator Mississippians, in the Southern Everglades. Dissertation. University of Miami, Miami, FL. p. 243.
Beerens, J. M., Noonburg, E. G., and Gawlik, D. E. (2015). Linking dynamic habitat selection with wading bird foraging distributions across resource gradients. PLoS One 10:e0128182. doi: 10.1371/journal.pone.0128182
Botson, B. A., Gawlik, D. E., and Trexler, J. C. (2016). Mechanisms that generate resource pulses in a fluctuating wetland. PLoS One 11:e0158864. doi: 10.1371/journal.pone.0158864
Brier, G. W. (1950). Verification of forecasts expressed in terms of probability. Mon. Weather Rev. 78, 1–3. doi: 10.1175/1520-0493(1950)078<0001:VOFEIT>2.0.CO;2
Brudvig, L. A., and Catano, C. P. (2021). Prediction and uncertainty in restoration science. Restor. Ecol. :e13380. doi: 10.1111/rec.13380
Campbell, M. R., and Mazzotti, F. J. (2004). Characterization of natural and artificial alligator holes. Southeast. Nat. 3, 583–594. doi: 10.1656/1528-7092(2004)003[0583:CONAAA]2.0.CO;2
Chambers, L. G., Steinmuller, H. E., and Breithaupt, J. L. (2019). Toward a mechanistic understanding of “peat collapse” and its potential contribution to coastal wetland loss. Ecology 100:e02720. doi: 10.1002/ecy.2720
Charles, S. P., Kominoski, J. S., Troxler, T. G., Gaiser, E. E., Servais, S., Wilson, B. J., et al. (2019). Experimental saltwater intrusion drives rapid soil elevation and carbon loss in freshwater and brackish everglades marshes. Estuar. Coasts 42, 1868–1881. doi: 10.1007/s12237-019-00620-3
Chen, S. H., and Pollino, C. A. (2012). Good practice in Bayesian network modelling. Environ. Model Softw. 37, 134–145. doi: 10.1016/j.envsoft.2012.03.012
Childers, D. L., Boyer, J. N., Davis, S. E., Madden, C. J., Ridnick, D. T., and Sklar, F. H. (2006). Relating precipitation and water management to nutrient concentrations in the oligotrophic “upside-down” estuaries of the Florida Everglades. Limnol. Oceanogr. 51, 602–616. doi: 10.4319/lo.2006.51.1_part_2.0602
Conroy, M. J., Peterson, J. T., Bass, O. L., Fonnesbeck, C. J., Howell, J. E., Moore, C. T., et al. (2008). Sources of variation in detection of wading birds from aerial surveys in the Florida Everglades. Auk 125, 731–743. doi: 10.1525/auk.2008.07134
Craft, C. B., and Richardson, C. J. (1998). Recent and long-term organic soil accretion and nutrient accumulation in the Everglades. Soil Sci. Soc. Am. J. 62, 834–843. doi: 10.2136/sssaj1998.03615995006200030042x
Crozier, G. E., and Gawlik, D. E. (2003). Wading bird nesting effort as an index to wetland ecosystem integrity. Waterbirds 26, 303–324. doi: 10.1675/1524-4695(2003)026[0303:WBNEAA]2.0.CO;2
D’Acunto, L. E., Pearlstine, L., Haider, S. M., Hackett, C. E., Shinde, D., and Romañach, S. S. (2023). Everglades vulnerability analysis (EVA) modeling scripts and output. U.S. Geological Survey data release. doi: 10.5066/P9JPVPGV
D’Acunto, L. E., Pearlstine, L., and Romañach, S. S. (2021). Joint species distribution models of Everglades wading birds to inform restoration planning. PLoS One 16:e0245973. doi: 10.1371/journal.pone.0245973
Dalrymple, G. H. (1996a, 1996). Growth of American alligators in the Shark Valley region of Everglades National Park. Copeia 1996, 212–216. doi: 10.2307/1446962
Dalrymple, G. H. (1996b). The effect of prolonged high water levels in 1995 on the American alligator in the Shark Valley area of Everglades National Park. Pages 125–136 in proceedings of conference on ecological assessment of the 1994–1995 high water conditions in the Southern Everglades. National Park Service, Everglades National Park, South Florida Natural Research Center, Homestead, Florida.
Davis, S. M., and Ogden, J. C. (1994). Everglades: The Ecosystem and Its Restoration. Delray Beach, FL: St. Lucie Press.
Dessu, S. B., Price, R. M., Troxler, T. G., and Kominoski, J. S. (2018). Effects of sea-level rise and freshwater management on long-term water levels and water quality in the Florida Coastal Everglades. J. Environ. Manag. 211, 164–176. doi: 10.1016/j.jenvman.2018.01.025
Doren, R. F., Trexler, J. C., Gottlieb, A. D., and Harwell, M. C. (2009). Ecological indicators for system-wide assessment of the greater everglades ecosystem restoration program. Ecol. Indic. 9, S2–S16. doi: 10.1016/j.ecolind.2008.08.009
Essian, D. A., Paudel, R., and Gawlik, D. E. (2022). Predicting effects of water management on breeding abundance of three wading bird species. J. Wildl. Manag. 86:e22155. doi: 10.1002/jwmg.22155
Fielding, A. H., and Bell, J. F. (1997). A review of methods for the assessment of prediction errors in conservation presence/absence models. Environ. Conserv. 24, 38–49. doi: 10.1017/S0376892997000088
Fleming, D. M. (1990). American alligator distribution and abundance in relation to landscape pattern and temporal characteristics of the Everglades. Report. National Park Service, Everglades National Park, South Florida Research Center, Homestead, Florida.
Flower, H., Rains, M., Fitz, H. C., Orem, W., Newman, S., Osborne, T. Z., et al. (2019). Shifting ground: landscape-scale modeling of biogeochemical processes under climate change in the Florida Everglades. Environ. Manag. 64, 416–435. doi: 10.1007/s00267-019-01200-8
Frederick, P., Gawlik, D. E., Ogden, J. C., Cook, M. I., and Lusk, M. (2009). The white ibis and wood stork as indicators for restoration of the everglades ecosystem. Ecol. Indic. 9, S83–S95. doi: 10.1016/j.ecolind.2008.10.012
Gawlik, D. E. (2002). The effects of prey availability on the numerical response of wading birds. Ecol. Monogr. 72, 329–346. doi: 10.1890/0012-9615(2002)072[0329:TEOPAO]2.0.CO;2
Gibble, R., Miller, L., and Harwell, M. C. (2020). “Using stakeholder engagement, translational science and decision support tools for ecosystem-based management in the Florida Everglades,” in Ecosystem-based Management, Ecosystem Services and Aquatic Biodiversity (Berlin: Springer), 517–541.
Gleason, P. J., and Stone, P. (1994). “Age, origin, and landscape evolution of the Everglades peatland,” in Everglades—The Ecosystem and Its Restoration. eds. S. M. Davis and J. C. Ogden (Delray Beach: St. Lucie Press), 149–197.
Gregoire, T. G., Lin, Q. F., Boudreau, J., and Nelson, R. (2008). Regression estimation following the square-root transformation of the response. For. Sci. 54, 597–606. doi: 10.1093/forestscience/54.6.597
Haider, S., Swain, E., Beerens, J., Petkewich, M., McCloskey, B., and Henkel, H. (2020). The Everglades depth estimation network (EDEN) surface-water interpolation model, version 3. U.S. Geological Survey scientific investigations report 2020-5083. p. 31.
Hiemstra, P. H., Pebesma, E. J., Twenhofel, C. J. W., and Heuvelink, G. B. M. (2008). Real-time automatic interpolation of ambient gamma dose rates from the Dutch radioactivity monitoring network. Computers and Geosciences. Available at: https://cran.r-project.org/web/packages/automap/index.html
Jolliffe, I. T., and Cadima, J. (2016). Principal component analysis: a review and recent developments. Philos. Trans. R. Soc. A Math. Phys. Eng. Sci. 374:20150202. doi: 10.1098/rsta.2015.0202
Kaikkonen, L., Parviainen, T., Rahikainen, M., Uusitalo, L., and Lehikoinen, A. (2020). Bayesian networks in environmental risk assessment: a review. Integr. Environ. Assess. Manag. 17, 62–78. doi: 10.1002/ieam.4332
Landuyt, D., Broekx, R, S., Dhondt, G. E., Aertsens, J., and Goethals, P. L. M. (2013). A review of Bayesian belief networks in ecosystem service modelling. Environ. Model Softw. 46, 1–11. doi: 10.1016/j.envsoft.2013.03.011
Leonelli, M., and Riccomagno, E. (2022). A geometric characterization of sensitivity analysis in monomial models. Int. J. Approx. Reason. 151, 64–84. doi: 10.1016/j.ijar.2022.09.006
Lodge, T. E. (2016). The Everglades Handbook: Understanding the Ecosystem. Boca Raton, FL: CRC Press.
LoSchiavo, A. J., Best, R. G., Burns, R. E., Gray, S., Harwell, M. C., Hines, E. B., et al. (2013). Lessons learned from the first decade of adaptive management in comprehensive Everglades restoration. Ecol. Soc. 18:70. doi: 10.5751/ES-06065-180470
Macek, P., and Rejmankova, E. (2007). Response of emergent macrophytes to experimental nutrient and salinity additions. Funct. Ecol. 21, 478–488. doi: 10.1111/j.1365-2435.2007.01266.x
Marcot, B. G. (2012). Metrics for evaluating performance and uncertainty of Bayesian network models. Ecol. Model. 230, 50–62. doi: 10.1016/j.ecolmodel.2012.01.013
Marcot, B. G., and Hanea, A. M. (2021). What is an optimal value of k in k-fold cross-validation in discrete Bayesian network analysis? Comput. Stat. 36, 2009–2031. doi: 10.1007/s00180-020-00999-9
Marcot, B. G., and Penman, T. D. (2019). Advances in Bayesian network modelling: integration of modelling technologies. Environ. Model Softw. 111, 386–393. doi: 10.1016/j.envsoft.2018.09.016
Marcot, B. G., Steventon, J. D., Sutherland, G. D., and McCann, R. K. (2006). Guidelines for developing and updating Bayesian belief networks applied to ecological modeling and conservation. Can. J. For. Res. 36, 3063–3074. doi: 10.1139/x06-135
Mazzotti, F. J., Brandt, L. A., Campbell, M. R., and Palmer, M. (1999). An ecological characterization of Everglades alligator holes. Final Report. Submitted to Everglades Agricultural Area Environmental Protection District and National Fish and Wildlife Foundation.
Mazzotti, F. J., Brandt, L. A., Rice, K. G., Borkhataria, R. R., Martin, G., and Minkowski, K. (2004). Ecological characterization of aquatic refugia in the Arthur R. Marshall Loxahatchee National Wildlife Refuge. Report. Submitted to National Park Service, Everglades National Park.
McCann, R. K., Marcot, B. G., and Ellis, R. (2006). Bayesian belief networks: applications in ecology and natural resource management. Can. J. For. Res. 36, 3053–3062. doi: 10.1139/x06-238
National Academies of Sciences, Engineering, and Medicine (NASEM) (2018). Progress Toward Restoring the Everglades: The Seventh Biennial Review. Washington, DC: The National Academies Press.
National Academies of Sciences, Engineering, and Medicine (NASEM) (2021). Progress Toward Restoring the Everglades: The Eighth Biennial Review. Washington, DC: The National Academies Press.
Newsom, J. D., Joanen, T., and Howard, R. (1987). Habitat suitability index models: American alligator. U.S. Fish and Wildlife Service biological report 82/10.136. U.S. Fish and Wildlife Service, Florida. p. 14.
O’Reilly-Nugent, A., Wandrag, E. M., Catford, J. A., Gruber, B., Driscoll, D., and Duncan, R. P. (2018). Measuring competitive impact: joint-species modelling of invaded plant communities. J. Ecol. 108, 449–459. doi: 10.1111/1365-2745.13280
Palaseanu, M., and Pearlstine, L. (2008). Estimation of water surface elevations for the Everglades, Florida. Comput. Geosci. 34, 815–826. doi: 10.1016/j.cageo.2007.08.004
Parrish, J. D., Braun, D. P., and Unnasch, R. S. (2003). Are we conserving what we say we are? Measuring ecological integrity within protected areas. Bio. Sci. 53, 851–860. doi: 10.1641/0006-3568(2003)053[0851:AWCWWS]2.0.CO;2
REstoration COordination and VERification (RECOVER). (2016). Five-year plan: Fiscal years 2017–2021. Restoration Coordination and Verification, United States Army Corps of Engineers, Jacksonville, Florida, and South Florida water Management District, West Palm Beach, Florida. Accessed 30 September 2016. Available at: https://evergladesrestoration.gov/content/scg/minutes/2017meetings/012317/RECOVER_5-yr.pdf
REstoration COordination and VERification (RECOVER). (2020). The RECOVER Team’s recommendations for revisions to the interim goals and interim targets for the Comprehensive Everglades restoration plan: 2020. Restoration Coordination and Verification. US Army Corps of Engineers, Jacksonville District, Jacksonville, FL and South Florida Water Management District, West Palm Beach FL. Available at: https://usace.contentdm.oclc.org/utils/getfile/collection/p16021coll7/id/14710. [Accessed Aug 8 2022].
Rice, K. G., and Mazzotti, F. J. (2006). American alligator distribution, size, and hole occupancy and American crocodile juvenile growth and survival. Annual Report. Submitted to U.S. Army Corps of Engineers.
Rice, K. G., and Mazzotti, F. J. (2007). American alligator distribution, size, and hole occupancy and American crocodile juvenile growth and survival. Annual Report. Submitted to U.S. Army Corps of Engineers, Jacksonville, Florida.
Rice, K. G., Mazzotti, F. J., Brandt, L. A., and Tarboton, K. C. (2004). “Alligator habitat suitability index” in Habitat Suitability Indices for Evaluating Water Management Alternatives. eds. K. C. Tarboton, M. M. Irizarry-Ortiz, D. P. Loucks, S. M. Davis, and J. T. Obeysekera (West Palm Beach, FL: South Florida Water Management District), 93–110.
Romañach, S. S., DeAngelis, D. L., Koh, H. L., Li, Y., Teh, S. Y., Barizan, R. S. R., et al. (2018). Conservation and restoration of mangroves: global status, perspectives, and prognosis. Ocean Coast. Manag. 154, 72–82. doi: 10.1016/j.ocecoaman.2018.01.009
Romañach, S. S., Haider, S. M., Hackett, C., McKelvy, M., and Pearlstine, L. G. (2022). Managing multiple species with conflicting needs in the Greater Everglades. Ecol. Indic. 136:108669. doi: 10.1016/j.ecolind.2022.108669
Romañach, S. S., and Pearlstine, L. G. (2021). “Forecasting ecological responses for wetland restoration planning in Florida’s Everglades,” in The Encyclopedia of Conservation. eds. D. A. DellaSala and M. I. Goldstein (Elsevier), 371–380.
Ross, M. S., Sah, J. P., Ruiz, P. L., Jones, D. T., Cooley, H., Travieso, R., et al. (2004). Effect of hydrologic restoration on the habitat of the cape sable seaside sparrow. Annual report. Submitted to National Park Service, Everglades National Park. Southeast Environmental Research Center, Florida International University, Miami, Florida. p. 39.
Ruiz, P. L., Schall, T. N., Shamblin, R. B., and Whelan, K. R. (2021). The vegetation of Everglades National Park: Final report. Natural Resource Report. NPS/SFCN/NRR—2021/2256. National Park Service. Fort Collins, Colorado.
Russell, S. J., and Norvig, P. (2009). Artificial Intelligence: A Modern Approach, 3rd. London: Pearson Education.
Sah, J. P., Ruiz, P. L., and Ross, M. S. (2018). Spatio-temporal pattern of plant communities along a hydrologic gradient in Everglades tree islands. For. Ecol. Manag. 421, 16–31. doi: 10.1016/j.foreco.2018.03.022
Scutari, M. (2010). Learning Bayesian networks with the bnlearn R package. J. Stat. Softw. 35, 1–22. doi: 10.18637/jss.v035.i03
Shinde, D., Pearlstine, L., Brandt, L. A., Mazzotti, F. J., Parry, M. W., Jeffrey, B., et al. (2014). Alligator production suitability index model (GATOR-PSIM v. 2.0): Ecological and design documentation. South Florida natural resources center technical series 2014: 1.
South Florida Water Management District (SFWMD). (2005). Regional Simulation Model (RSM). Theory Manual, South Florida Water Management District. 308. Available at: https://www.sfwmd.gov/sites/default/files/documents/rsmtheoryman.pdf. [Accessed 09 August 2022].
Swain, E. D., Lohmann, M. A., and Goodwin, C. R. (2019). The hydrologic system of the South Florida peninsula: Development and application of the Biscayne and Southern Everglades coastal transport (BISECT) model. U.S. Geological Survey scientific investigations report 2019-5045. pp. 114.
Todd, M. J., Muneepeerakul, R., Pumo, D., Azaele, S., Miralles-Wilhelm, F., Rinaldo, A., et al. (2010). Hydrological drivers of wetland vegetation community distribution within Everglades National Park, Florida. Adv. Water Res. 33, 1279–1289. doi: 10.1016/j.advwatres.2010.04.003
Trexler, J. C., and Goss, C. W. (2009). Aquatic fauna as indicators for Everglades restoration: applying dynamic targets in assessments. Ecol. Indic. 9, S108–S119. doi: 10.1016/j.ecolind.2008.11.001
U.S. Environmental Protection Agency (USEPA). (2020). Environmental monitoring in the Everglades. Available at: https://www.epa.gov/everglades/environmental-monitoring-everglades. [Accessed 14 2020].
Ugarte, C. A., Bass, O. L., Nuttle, W., Mazzotti, F. J., Rice, K. G., Fujisaki, I., et al. (2013). The influence of regional hydrology on nesting behavior and nest fate of the American alligator. J. Wildl. Manag. 77, 192–199. doi: 10.1002/jwmg.463
Van der Heiden, C. A., and Dorn, N. (2017). Benefits of adjacent habitat patches to the distribution of a crayfish population in a hydro-dynamic wetland landscape. Aquat. Ecol. 51, 219–233. doi: 10.1007/s10452-016-9612-1
Wilson, B. J. (2018). Drivers and Mechanisms of Peat Collapse in Coastal Wetlands. Dissertations. Florida International University, Miami, Florida. p. 215.
Keywords: Bayesian networks, ecological indicators, ecohydrology, decision support, uncertainty
Citation: D’Acunto LE, Pearlstine L, Haider SM, Hackett CE, Shinde D and Romañach SS (2023) The Everglades vulnerability analysis: Linking ecological models to support ecosystem restoration. Front. Ecol. Evol. 11:1111551. doi: 10.3389/fevo.2023.1111551
Edited by:
Ruscena P. Wiederholt, Everglades Foundation, United StatesReviewed by:
Tian Xie, Beijing Normal University, ChinaMichael Alister Reid, University of New England, Australia
Denise Reed, University of New Orleans, United States
Copyright © 2023 D’Acunto, Pearlstine, Haider, Hackett, Shinde and Romañach. This is an open-access article distributed under the terms of the Creative Commons Attribution License (CC BY). The use, distribution or reproduction in other forums is permitted, provided the original author(s) and the copyright owner(s) are credited and that the original publication in this journal is cited, in accordance with accepted academic practice. No use, distribution or reproduction is permitted which does not comply with these terms.
*Correspondence: Laura E. D’Acunto, bGRhY3VudG9AdXNncy5nb3Y=