- 1Jilin Provincial Key Laboratory of Animal Resource Conservation and Utilization, Northeast Normal University, Changchun, China
- 2Key Laboratory of Vegetation Ecology, Ministry of Education, Changchun, China
- 3School of Life Sciences, Central China Normal University, Wuhan, China
- 4College of Life Science, Northeast Agricultural University, Harbin, China
- 5College of Life Science, Jilin Agricultural University, Changchun, China
Understanding trophic niche differentiation is critical for exploring interspecific competition and stable coexistence among morphologically similar sympatric species. Bats are an ideal model for studying trophic niche differentiation among species because of their high taxonomic and ecological diversities, as well as their special life history traits. Although many factors can affect bat trophic niches, few studies have combined multiple factors to investigate the influences on bat trophic niches. In this study, we analyzed the summer diet and potential influencing factors of five sympatric rhinolophid bats in southwestern China: Rhinolophus macrotis, Rhinolophus osgoodi, Rhinolophus ferrumequinum, Rhinolophus affinis, and Rhinolophus pusillus. All five species mainly fed on Lepidopteran and Dipteran insects, with a large trophic niche breadth for each species and a low degree of dietary overlap. With the exception of R. affinis and R. pusillus, significant differences in diet composition were detected among species, which indicated relatively low interspecific competition. Canonical correspondence analysis showed that both echolocation calls and body size significantly affected interspecific diet differentiation, while wing morphology and bite force had relatively weak effects. This study suggests that the combined effects of multiple factors may drive trophic niche differentiation among five rhinolophid bat species in the study area.
Introduction
Niche theory predicts that the stable coexistence of morphologically and ecologically similar species in a community depends on resource partitioning to minimize competition (Hutchinson, 1959; Schoener, 1974; Chesson, 2000; Letten et al., 2017). In recent years, numerous studies have shown that trophic niche differentiation often plays a key role in shaping the structure of animal communities (Holt, 2009; Matthews et al., 2010; Codron et al., 2015; Novella-Fernandez et al., 2020), especially for sympatric closely related species. This knowledge can be vital to the understanding of diet composition, interspecific competition, and the mechanism of trophic niche differentiation (Salinas-Ramos et al., 2015; Arrizabalaga-Escudero et al., 2018).
Bats are a highly ecologically and species-diverse (> 1,400 species) mammalian group with real flying capability (Kunz and Fenton, 2003; Ingala et al., 2018; Wilson and Mittermeier, 2019). Bats play an important role in global ecosystems, such as in pollination, seed dispersal, and pest control (Kunz and Fenton, 2003; Kasso and Balakrishnan, 2013). Approximately 70% of bat species feed on insects (Kunz and Fenton, 2003) and often co-occur in large multi-species assemblages, with extensive variation in foraging strategies, echolocation calls, and wing morphology, making bats an ideal model for studying the differentiation of trophic niches in communities and the potential influencing factors.
Many investigations have demonstrated that differences in body size and wing morphology among insectivorous bats are closely associated with niche partitioning and can lead to prey differences (Norberg and Rayner, 1987; Saunders and Barclay, 1992; Ashrafi et al., 2011; Schoeman and Jacobs, 2011). Body size is known to be an important factor that directly influences the diet of bats in multi-species assemblages (Andreas et al., 2013; Gnocchi et al., 2019). For instance, three coexisting rhinolophid bats (Rhinolophus hipposideros, Rhinolophus euryale, and Rhinolophus ferrumequinum) differ in body size, resulting in significant differences in the size of captured insects (Andreas et al., 2013). Furthermore, wing morphology in bats significantly influences flight patterns and the choice of foraging areas. For example, due to minor differences in wing morphology, the sympatric sister species Rhinolophus hemelyi and R. euryale utilize different foraging areas and therefore capture different types of insects, resulting in trophic niche divergence (Salsamendi et al., 2012).
In addition to body size and wing morphology, different echolocation calls and bite forces may also lead to divergence in trophic niches among bat species. Echolocation calls are critical to navigation and foraging, and they exhibit great variability as adaptations to different environments, such as foraging habitats of varying complexity, different foraging strategies, and diverse food types (Bogdanowicz et al., 1999; Schoeman and Jacobs, 2003; Razgour et al., 2011; Arrizabalaga-Escudero et al., 2018; Pavey, 2020). Bat species with different peak frequencies exhibit significant differences in activity areas and prey types, contributing to the full utilization of local food resources and promoting coexistence (Fullard et al., 1991; Pavey, 2020). For example, six sympatric Jamaican bat species with different echolocation calls differed significantly in their foraging areas and diet composition, minimizing the degree of overlap in resource use between species (Emrich et al., 2014). The bite force of bats is a well-studied ecological trait that is closely related to feeding habits and can partly reflect the adaptation of bats to food hardness and prey handling (Nogueira et al., 2009; García-Herrera et al., 2021). Usually, bats that prefer to feed on hard-shelled prey, such as beetles, have robust skulls, while bats that feed mainly on soft-bodied insects, such as moths, have weaker skulls and bite forces (Aguirre et al., 2003; Krüger et al., 2014).
While the abovementioned factors may affect the trophic niche differentiation of insectivorous bats, few studies have examined the mechanisms of trophic niche differentiation in bats in terms of multiple factors (Biscardi et al., 2007). To some extent, this research gap has limited the comprehensive understanding of the mechanisms of bat dietary differentiation and how they shape resource use across a community. Furthermore, studies that employ traditional morphological methods in the examination of guano typically have a lower resolution, such as ordinal or family-level identification, which may overlook some prey that have been thoroughly digested, resulting in a biased view of bat feeding habits (Rolfe et al., 2014). In recent years, high-throughput sequencing techniques have been widely used in dietary studies because of their efficiency and productivity, which can improve the taxonomic assignment to the species level through analyzing DNA residues in the guano and thus reveal more subtle niche differentiation (Leal et al., 2018; Jusino et al., 2019). High-throughput sequencing has been used in many studies on trophic ecology and the differentiation of trophic niches in coexisting species (Gordon et al., 2019; Novella-Fernandez et al., 2020; Andriollo et al., 2021).
This study investigated the trophic niche differentiation of five sympatric rhinolophid bats, including Rhinolophus macrotis, Rhinolophus osgoodi, Rhinolophus ferrumequinum, Rhinolophus affinis, and Rhinolophus pusillus, and determined the relative importance of body size, wing morphology, echolocation calls, and bite force to the trophic niche differentiation of these species. First, high-throughput sequencing technology was used to analyze the diets of the five rhinolophid bats to reveal the diet composition and the niche overlap relationships among species. Second, body size, wing morphology, echolocation calls, and bite force were combined to determine their effects on trophic niches. This study contributes to the understanding of the trophic niche differentiation in sympatric bats and helps to improve the knowledge of the mechanisms of coexistence among bats.
Materials and methods
Study site and species
The sampling site, Xianren Cave (102°20′E, 24°30′N), is located on the Yunnan-Guizhou Plateau at an altitude of 2049 m in Yunnan Province, China. The site has a subtropical climate with an average annual temperature of 15.5°C and an average annual rainfall of 973.5 mm, with precipitation mainly concentrated in June–October. The vegetation surrounding the cave mainly included Juglans catanensis, Pinus yunnanensis furnace, Cryptomeria fortunei, and Phyllostachys sulphurea, as well as agricultural fields and villages, all of which provided a good foraging habitat for bats. Preliminary monitoring revealed that five rhinolophid bat species coexisted stably in the cave, including R. macrotis (about 50 individuals), R. osgoodi (about 40 individuals), R. ferrumequinum (about 20 individuals), R. affinis (about 20 individuals), and R. pusillus (about 20 individuals) (unpublished data).
Sample collection
To minimize the disturbance to lactating bats, we chose to conduct the study in August–September 2017 and 2018, from 21:00 to 4:00 the following morning. Bats were captured with mist nets at the entrance of the Xianren cave after they returned to the cave. Species and sex were identified based on external morphological characteristics, and whether bats were adults or juveniles was judged based on epiphyseal fusions (Stebbings, 1968). Once juveniles were identified, they were immediately released in situ. Captured bats were placed singly in sterilized kraft paper bags for 2 h while droppings were collected. The bags were checked frequently to ensure the freshness of the droppings. The droppings were placed into lyophilized tubes containing RNAlater (RNA-EZ Reagents RNA-Be-Locked A, Sangon Biotech, Shanghai, China) using sterilized forceps. At least five droppings per bat were collected, returned to the laboratory, and stored at −80°C for DNA extraction.
Collection of data on morphology, bite force, and echolocation calls
After the guano was collected, the forearm length of each bat was measured using a digital caliper (TESA-CAL IP67, Tesa Technology, Renens, Switzerland, 0.01 mm) and body mass was determined with an electronic balance (ProScale LC-50, Accurate Technology, Inc., Asheville, NC, United States, 0.01 g). The wing morphology of each bat was drawn with reference to Thabah’s method (Thabah et al., 2007). The bite force of the molars was recorded five times for each bat following the method of Freeman and Lemen (2008) using a bite force measurement system (Nanjing Bioinspired Intelligent Technology Co., Ltd., Nanjing, China) with an accuracy of 0.01 N. The system includes a sensor (NBIT-S1-100NHL-001) and data acquisition system (NBIT-Dus-2404A), and was used following the manufacturer’s protocol. The maximum bite force was taken as the bat’s bite force value. The echolocation calls were recorded using an ultrasound recorder (UltraSound Gate 116, Avisoft Bioacoustics, Berlin, Germany) when the bats were resting in a temporary laboratory (5 m × 4 m × 3 m), with the recording microphone aimed at the resting bat’s head at a distance of 30 cm. The sampling frequency was 375 kHz, and the resolution was 16 bits. The data were input into a laptop computer and analyzed using Avisoft SASLab Pro software. Each bat was recorded for at least 1 min (Jacobs et al., 2007; Li et al., 2007). After all measurements and acoustic recordings were finished, the bats were released in situ. A total of 98 bats were captured in the field, including 36 R. macrotis (18 females and 18 males), 29 R. osgoodi (21 females and eight males), 11 R. ferrumequinum (six females and five males), 12 R. affinis (six females and six males), and 10 R. pusillus (two females and eight males).
DNA extraction, PCR amplification, and sequencing
Genomic DNA was extracted from 200-mg guano per bat using the E.Z.N.A™ Mag-Bind Soil DNA Kit (OMEGA Bio-Tek, Norcross, GA, United States) according to the manufacturer’s instructions. After DNA extraction, DNA integrity was tested using 2% agarose gels. A 225-bp fragment of the cytochrome c oxidase subunit I (COI) was amplified using the primers LCO-1490 (5′-GGTCAACAAATCATAAAGATATTGG-3′) and ZBJ-ArtR2c (5′-WACTAATCAATTWCCAAATCCTCC-3′) (Zeale et al., 2011; Chang et al., 2019). Genomic DNA was accurately quantified using the Qubit 2.0 DNA Assay Kit (Life Technologies, Thermo Fisher Scientific, Waltham, MA, United States) to determine the amount of DNA added to the PCR reaction. The first step of PCR amplification reaction was conducted in a final volume of 30 μl containing 15 μl 2 × Taq Master Mix, 10 ng of genomic DNA, 1 μl primer F, and 1 μl primer R, with water added to reach a volume of 30 μl. The conditions for PCR were as follows: 94°C for 3 min; five cycles at 94°C for 30 s, 45°C for 20 s, and 65°C for 30 s; 20 cycles at 94°C for 20 s, 55°C for 20 s, and 72°C for 30 s; and a final extension at 72°C for 5 min. After the first step, the PCR products were checked using 2% agarose gel. The second step of the PCR amplification reaction was conducted in a final volume of 30 μl containing 15 μl 2 × Taq Master Mix, 10 ng of genomic DNA (from the first step), 1 μl primer F, 1 μl primer R, and water added to reach a volume of 30 μl. The PCR amplification reaction conditions were as follows: 95°C for 3 min; five cycles at 94°C for 20 s, 55°C for 20 s, and 72°C for 30 s; and a final extension at 72°C for 5 min. The PCR products were purified using Agencourt AMPure XP (Beckman Coulter, United States), and the DNA concentration of each sample quantified using the Qubit 2.0 DNA Assay Kit (Life Technologies, Thermo Fisher Scientific, Waltham, MA, United States) to normalize samples according to the manufacturer’s protocol. The final products were sequenced using the Illumina Miseq platform (2 × 300 bp) at Sangon Biotech (Shanghai, China).
Sequencing data analysis
Primers and adaptors were removed from the raw sequences using cutadapt v1.2.1 (Martin, 2011), and paired-end reads were merged using PEAR v0.9.6 (Zhang et al., 2014) based on barcode tags to distinguish samples. Finally, the data files were quality-filtered using Prinseq v0.20.4 (Schmieder and Edwards, 2011). The operational taxonomic units (OTUs) were clustered using Usearch after the singletons and chimeras were removed (Edgar, 2010). All optimized sequences were mapped to representative sequences, and OTU tables with a 97% similarity threshold were generated. The samples with fewer than 12,000 sequences were discarded, and the data were rarefied to 12,000 sequences per sample. Following this standard, six individuals with fewer than 12,000 sequences were removed (three R. macrotis, one R. osgoodi, one R. ferrumequinum, and one R. affinis). Finally, to minimize the effect of sequencing errors, the OTUs representing <0.1% of the normalized sequences for each sample were removed to prevent the generation of potentially erroneous results (Bokulich et al., 2013). Representative sequences of each OTU were compared with the reference sequences in the Barcode of Life Database (BOLD1) and the Genbank database2 to obtain taxonomic information. OTUs that did not match any taxonomic information were excluded. The identification criteria were based on slightly modified “strict” and “best” matching methods (Ross et al., 2008; Razgour et al., 2011).
Measurement of wing parameters and echolocation calls
After scanning the mapped wing shape, the wing span and wing area of each individual were measured using AutoCAD 2021 (Autodesk, United States), and the aspect ratio and wing loading were calculated for each individual (Norberg and Rayner, 1987; Salsamendi et al., 2012). For each individual bat, after removing the first and last syllables of each echolocation call sequence, 15 syllables with a signal-to-noise ratio higher than 40 dB were randomly selected. Due to the special cochlear structure and Doppler-shift compensation of rhinolophid bats during flight, bats are able to actively adjust their call frequency to ensure the dominant frequency keep stable (Russell and Kossl, 1999; Metzner et al., 2002; Davies et al., 2013). Therefore, three acoustic parameters, including peak frequency (PF), minimum frequency (Fmin), and maximum frequency (Fmax) were analyzed on the power spectrogram and oscillogram with Avisoft-SASLab Pro version 5.2.9 (Avisoft Bioacoustics, Berlin, Germany) based on a 1,024 FFT, 100% frame size, and 93.75% temporal overlap.
Statistical analysis
We quantified the diet composition using the percent of occurrence (POO) and relative read abundance (RRA) at the order level (Deagle et al., 2018). Chi-square tests (SPSS 22.0) and Kruskal–Wallis tests (R 4.1.1) with a post hoc Dunn test were used to test for differences in the prey orders consumed between bat species. The dietary niche breadth of each bat species was calculated using a standardized Levins’ index (BA) (Razgour et al., 2011), the diet composition overlap between bat species was measured using Pianka’s measure of niche overlap (Ojk) in the “EcoSimR” package in R (Gotelli et al., 2015), and the “qgraph” package was used to generate a network graph of dietary overlap between species. The ordination of samples was visualized depending on dietary composition at the OTU level with non-metric multidimensional scaling (NMDS), and an analysis of similarities (ANOSIM) test was used to determine whether the Bray–Curtis distance of dietary composition at the OTU level was greater between than within bat species (Oksanen et al., 2020). The ANOSIM test generates a global R statistic that provides a measure of the magnitude of difference among groups, with a range between −1 and + 1. When R > 0, the difference between groups was greater than the difference within each group. If the test results were significantly different overall, then a pairwise analysis between species was performed, and the p-values were corrected with Bonferroni correction. The differences between species were calculated for forearm length, body weight, aspect ratio, wing loading, bite force, and echolocation call parameters using one-way ANOVA or non-parametric Kruskal–Wallis tests depending on the test of normal distributions or the homogeneity of variances according to Shapiro and Bartlett tests in the package stats. The p-values for multiple comparisons were corrected using Bonferroni correction. All the data are presented as the means ± SD. Spearman correlation analysis was conducted to test the relationship between bite force and weight. To determine the difference in the alpha diversity of the diet among five rhinolophid bats at the OTU level, Kruskal–Wallis tests were used to compare the values of the Shannon–Wiener diversity and Gini–Simpson diversity with non-normal distributions in the “vegan” package. The effects of body size, wing morphology, bite force, and echolocation call parameters on the diet composition of bats were investigated using Canonical correspondence analysis (CCA) with the “vegan” package. The response variable (dietary species abundance data) and explanatory variables (body size, wing morphology, bite force, and echolocation calls) were Hellinger transformed and logarithmically transformed, respectively. The variance inflation factors (VIF) were calculated to identify the collinearity between the explanatory variables until the values of all factors were less than 10. To distinguish the contribution of a single variable, the relative importance of each influencing factor independently accounting for the variations in diet composition was quantified by applying a hierarchical partitioning analysis using the “rdacca.hp” package (Lai et al., 2022). All statistical analyzes and visualizations were conducted in R 4.1.1 (R Core Team, 2021).
Results
Sequencing data and dietary pattern
A total of 7.64 million raw sequences were obtained, with an average of 83,061 sequences per sample. Following quality control, 6.19 million sequences were obtained, with an average of 67,303 sequences per sample. After deleting OTUs that did not match the taxonomic information, 1,031 OTUs were obtained through comparison with the BOLD and Genbank databases, all of which were from the Arthropoda phylum. Among all OTUs, 83.41% were identified at the order level, 45.59% at the family level, 19.79% at the genus level, and 14.06% at the species level (Supplementary Table S1). Insects comprised the majority (98.81%) of phylum detected. Lepidoptera (58.65%) and Diptera (21.72%) comprised the majority of all 15 orders detected. Noctuidae (20.21%), Geometridae (14.04%), Erebidae (10.00%) and Tortricidae (4.68%) comprised the majority of families detected. Some differences were detected in the diet composition among bat species. The diets of R. macrotis and R. ferrumequinum were mainly composed of Lepidoptera (R. macrotis: 96.31%, R. ferrumequinum: 89.88%). The diet of R. osgoodi consisted mainly of Lepidoptera (73.49%) and Diptera (17.28%). The diet of R. affinis mainly consisted of Diptera (59.39%) and Lepidoptera (26.64%). The diet of R. pusillus mainly consisted of Diptera (75.13%) and Coleoptera (15.40%).
Dietary overlap and resource partitioning
At the OTU level, no significant differences were found in the Shannon–Wiener diversity or Gini–Simpson diversity between five rhinolophid bats (p = 0.94 and p = 0.91, respectively; Supplementary Figure S1). Overall, low levels of dietary overlap were found among bat species, with the greatest overlap value between R. affinis and R. pusillus (0.33), and the lowest value between R. macrotis and R. affinis (0.01; Table 1; Figure 1). The Levins’ standardized measure of niche breadth showed that dietary niche breadth ranged from 0.42 to 0.76, with the largest value for R. ferrumequinum and the smallest for R. macrotis (Table 1). The RRA analysis of the diet composition of each bat species at the order level showed significant differences in the consumption of Coleoptera, Diptera, Lepidoptera, Hemiptera, and Neuroptera (Kruskal–Wallis test, p < 0.05; Table 2; Figure 2). In addition, analysis of POO at the order level showed significant differences in the consumption of Coleoptera, Diptera, Hemiptera, and Neuroptera (Chi-squared test, p < 0.05; Table 2; Figure 2).

Table 1. Breadth of trophic niches and dietary overlap between five rhinolophid bats. BA: standardized Levins’ measure of niche breadth. For the dietary overlap index, values range from 0 (no dietary overlap) to 1 (full dietary overlap).
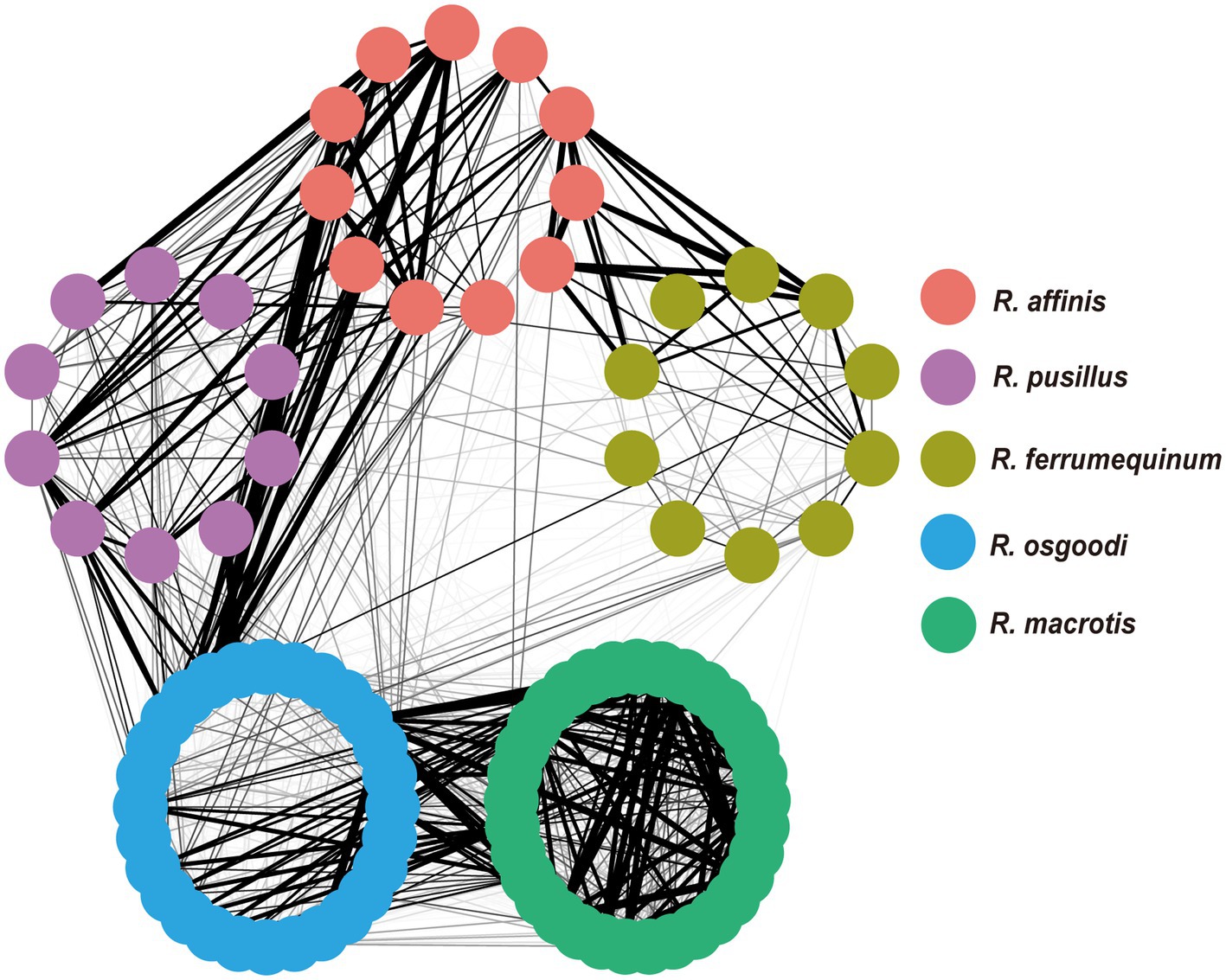
Figure 1. Network of five rhinolophid bat species. Each filled circle represents an individual, and the thicker the connection between circles, the higher dietary overlap between the individuals.
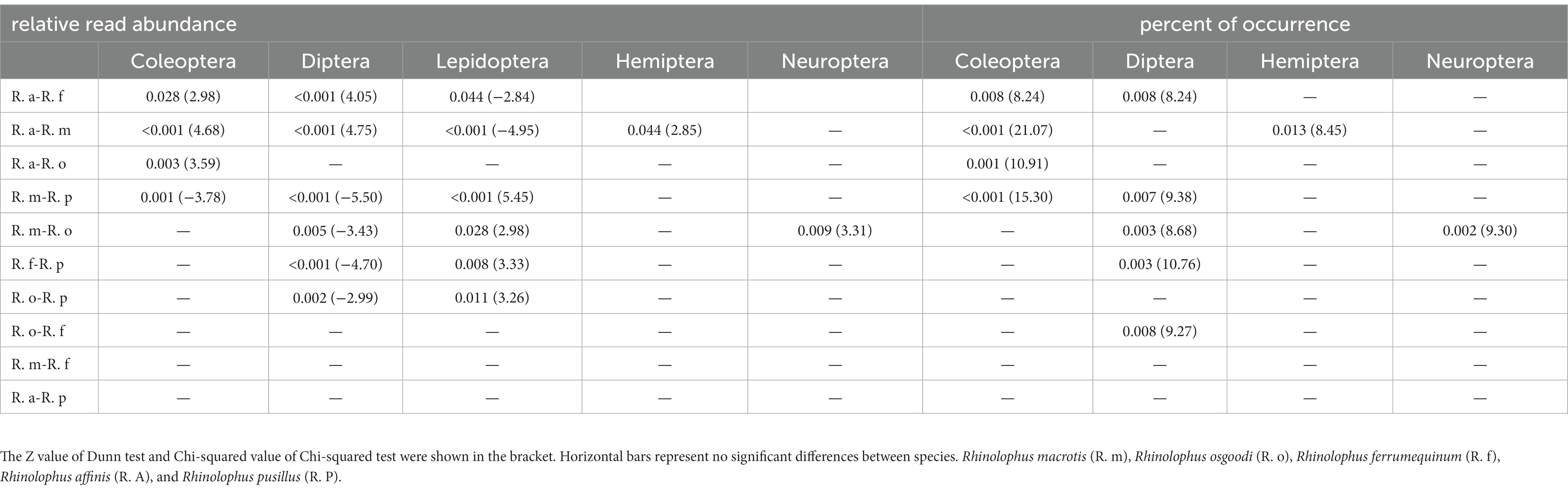
Table 2. Significant differences in the relative read abundance (Kruskal–Wallis test with a post hoc Dunn test) and percent of occurrence (Chi-squared test) in the diet composition of five rhinolophid bats at the order level.
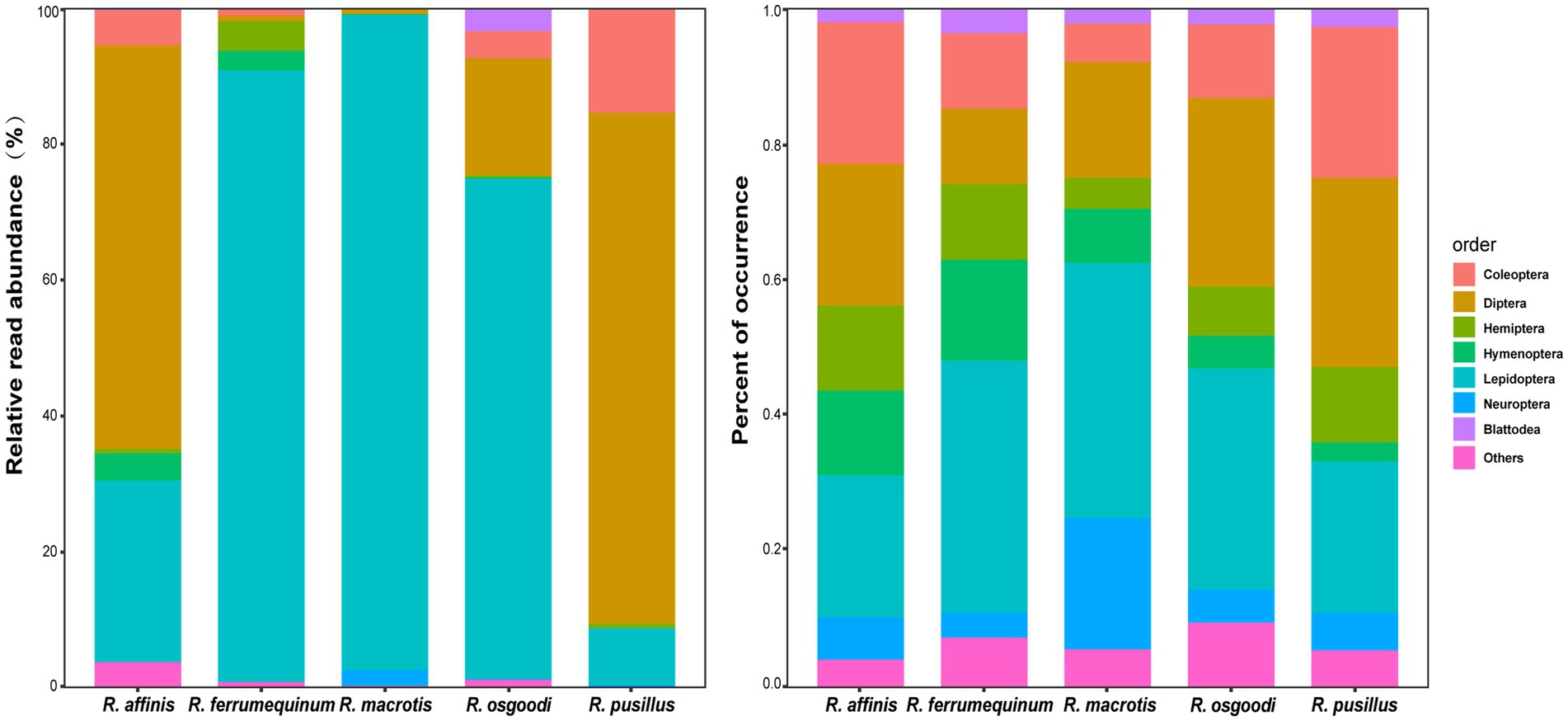
Figure 2. Relative read abundance (RRA) and percent of occurrence (POO) of diet composition at the order level in five rhinolophid bats.
Interspecific differences in body size, wing morphology, bite force, and echolocation calls
Body size differed greatly among bat species (Table 3; Supplementary Table S2), with the largest body weight and forearm length found in R. ferrumequinum (body weight: 18.58 ± 0.97 g; forearm length: 61.17 ± 0.40 mm) and the smallest found in R. pusillus (body weight: 4.90 ± 0.34 g; forearm length: 39.18 ± 1.21 mm). The maximum bite force was also found in R. ferrumequinum (5.65 ± 0.51 N), and the minimum was found in R. pusillus (1.02 ± 0.14 N). A significant positive correlation was detected between bite force and the body weight of bats (Spearman r = 0.87, p < 0.001). After removing the effect of body weight, there was still a significant difference between the four paired groups (R. ferrumequinum–R. macrotis, R. ferrumequinum–R. osgoodi, R. ferrumequinum–R. pusillus, and R. affinus–R. osgoodi) (Kruskal–Wallis test, p < 0.05; Table 3; Supplementary Table S2). R. macrotis had the lowest peak frequency (57.88 ± 0.86 kHz) and R. pusillus had the highest peak frequency (101.92 ± 1.49 kHz; Table 3; Figure 3). Significant differences were detected between bat species in body weight, forearm length, wing loading, bite force, peak frequency, minimum frequency, and maximum frequency (Kruskal–Wallis test, p < 0.001), as well as in the aspect ratio among species (one-way ANOVA, F = 5.16, p < 0.001; Table 3; Supplementary Table S2).
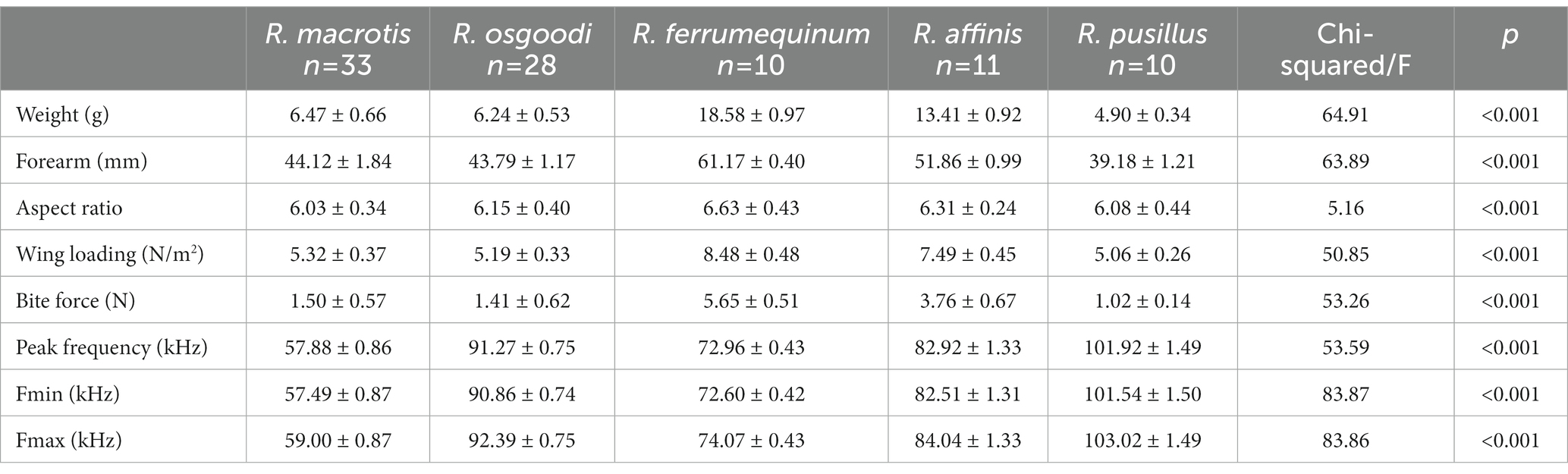
Table 3. Results of Kruskal–Wallis (all factors except aspect ratio) and analysis of variance (ANOVA) (aspect ratio) tests examining the variation (mean ± SD) of influencing factors among five bat species. The p-values were adjusted with the Bonferroni correction method for multiple comparisons.
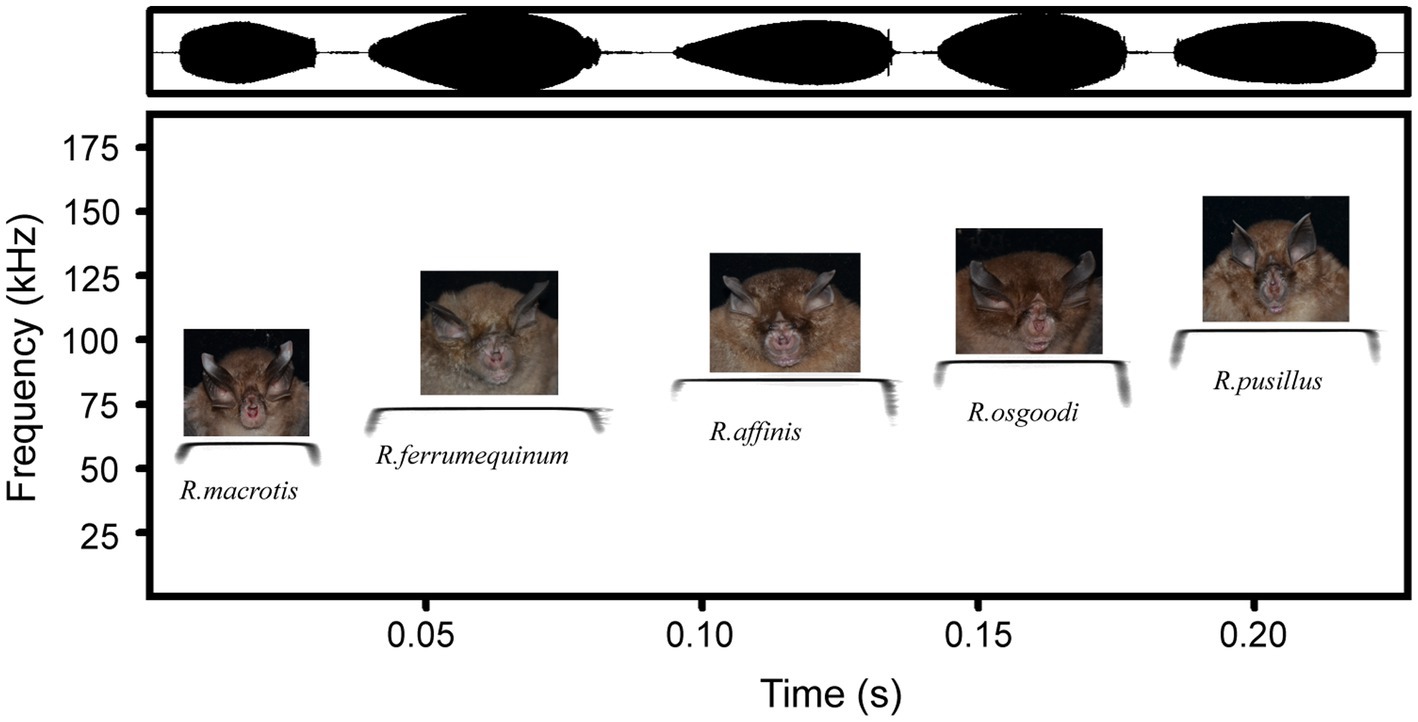
Figure 3. Sound envelopes (top) and acoustic spectrograms (bottom) corresponding to the peak frequencies of echolocation calls of five rhinolophid bats.
Factors influencing trophic niche differentiation
The NMDS ordination of diet composition between groups of five rhinolophid bats resulted in a two-dimensional solution with final stress of 0.103. The samples obtained from the five rhinolophid bats were spread out in the diagram, and the ANOSIM analysis showed significant differences in dietary composition in all pairwise comparisons (Figure 4A; r = 0.17, p = 0.001), except between R. affinis and R. pusillus (r = 0.09, p = 0.07). Because all factors had VIF values of less than 10 (Table 4), all factors were added to the model. The results of the CCA showed a significant relationship between all factors and diet data (adjusted r2 = 1.63%, p = 0.001; Table 4; Figure 4B), and the results of the permutations tests for each influencing factor individually showed that body weight, forearm length, peak frequency were all significantly correlated with dietary composition (p < 0.05; Table 4; Figure 4B). Hierarchical partitioning analysis showed that echolocation calls (peak frequency) were the most important factors, followed by body size factors (forearm length and body weight), while parameters such as wing loading and bite force had no significant effect on the diet composition structure for each bat species (Table 4; Figure 4B).
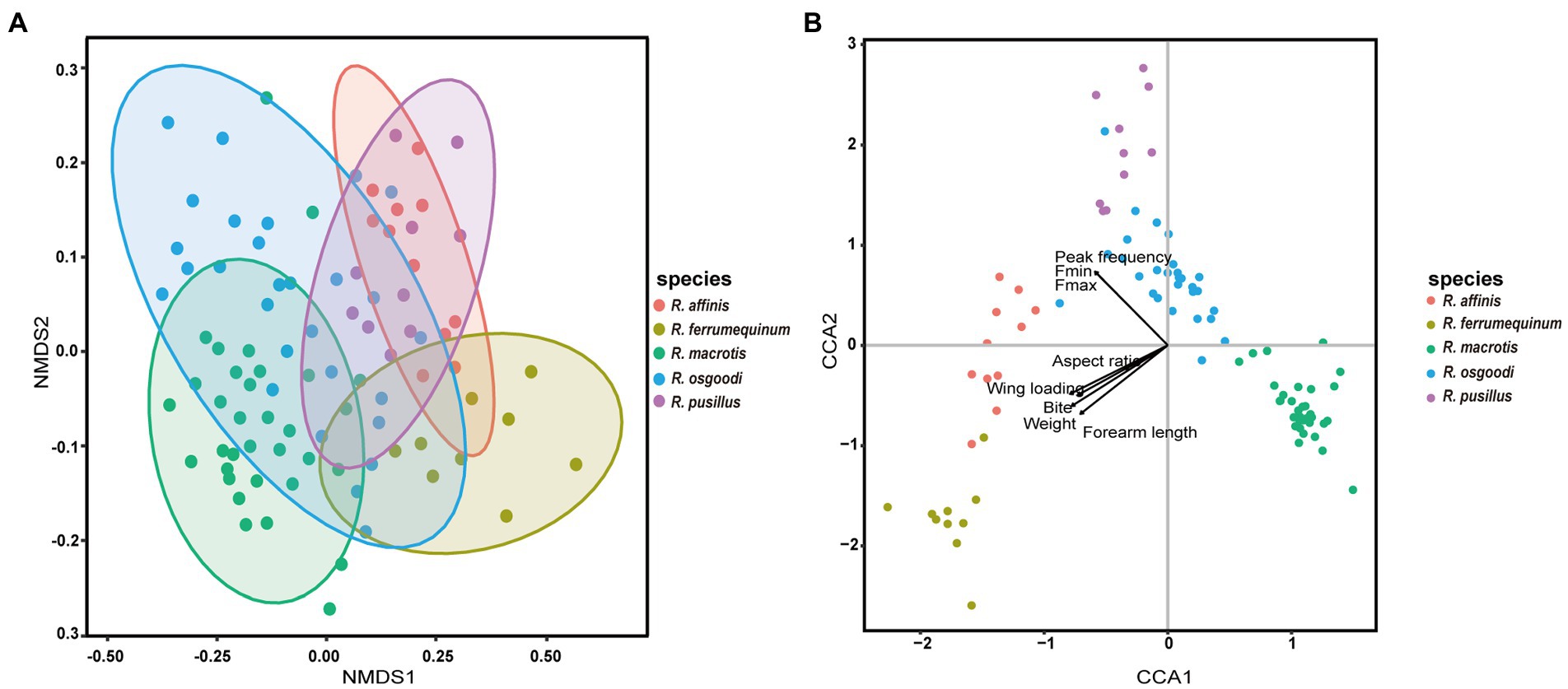
Figure 4. (A) Non-metric multidimensional scaling (NMDS) of the diet composition of five bat species based on the operational taxonomic unit (OTU) level. Each point represents an individual. (B) Canonical correspondence analysis (CCA) plot showing the correlation between diet composition and the body size, wing morphology, bite force, and echolocation calls of five bat species. Each point in the graph represents an individual, and the arrows indicate different variables, where the longer the ray, the greater the influence of that variable, and the angles between arrows represent the degree of correlation between them (acute angle: positive correlation; obtuse angle: negative correlation; right angle: no correlation).
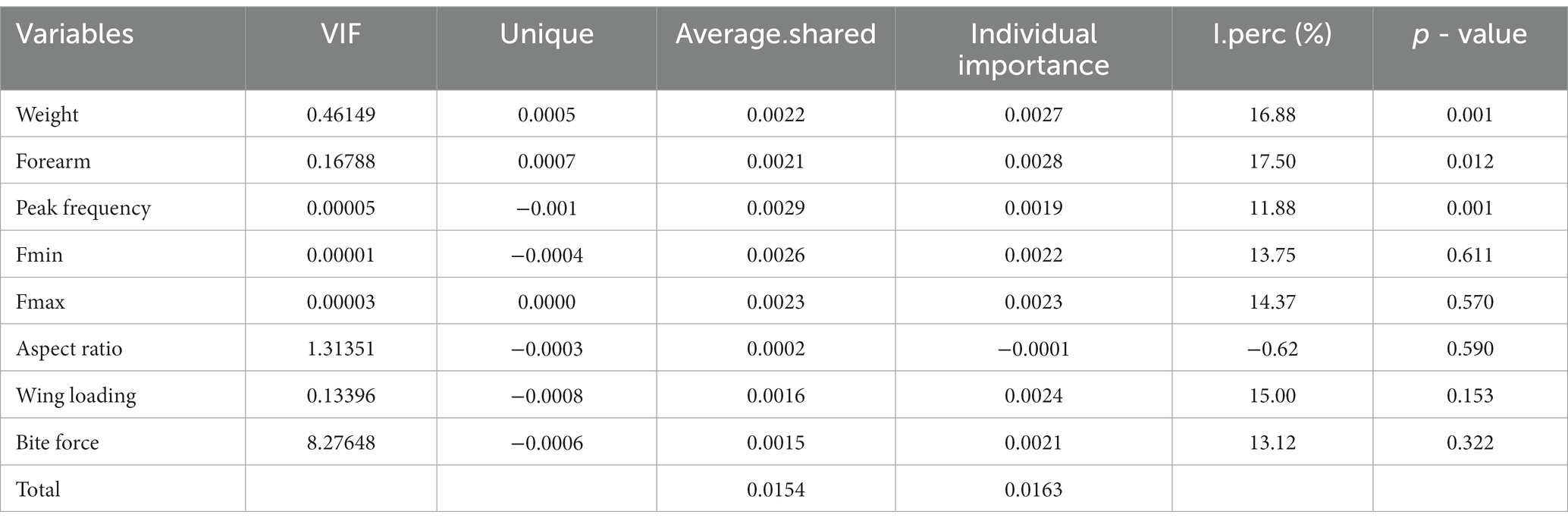
Table 4. Hierarchical partitioning of the relative importance of each influencing factor relative to the total explanatory variables.
Discussion
Diet composition and trophic niche overlap
All five rhinolophid bats investigated in this study relied on Lepidoptera as their main diet type. This finding was similar to the conclusions of previous studies conducted in summer [R. ferrumequinum (Jin et al., 2005; Andreas et al., 2013); R. affinis (Ye et al., 2009); R. pusillus (Wei et al., 2006); R. osgoodi and R. macrotis (Shi et al., 2009)]. However, there are some differences between previous findings and the current study. For example, studies on R. affinis and R. pusillus showed that Coleoptera were an important diet component (R. affinis: 39.51%, R. pusillus: 41.08%; Wei et al., 2006; Ye et al., 2009), while the present study found that Coleoptera make up only a relatively small part of the diet (R. affinis: 5.14%, R. pusillus: 15.40%), which may be due to the difference in available insect resources at different study sites or different research methods used. These insects provide a substantial, high-quality food source for bats that can ensure that their nutritional needs are met (Clare et al., 2011; Emrich et al., 2014; Rolfe et al., 2014; Chang et al., 2019). The present study used high-throughput sequencing to identify the species of insect residues remaining in bat guano, yielding a total of 145 identified insect species. This method has a high resolution compared with traditional guano analysis methods. For instance, this study detected small, soft-bodied Chironomidae in bat guano, which are easily overlooked in traditional guano analysis methods (Krüger et al., 2014).
Trophic niche differentiation is an effective way for sympatric bats to reduce competition for food resources and thus promote coexistence (Alley, 1982; Letten et al., 2017), and it has been demonstrated in numerous studies, such as Pteronotus macleayii and Mormoops blainvillii in Jamaica, and M. blainvillei, Pteronotus quadridens, and Pteronotus parnellii portoricensis on the island of Puerto Rico (Rolfe and Kurta, 2012; Emrich et al., 2014). In this study, all five rhinolophid bats had wide trophic niche breadth (0.42–0.76) and low overlap in trophic niche among species (0.01–0.33). This may have been due to the fact that sampling was conducted during the rainy season, when insects were abundant in our studied region (Zhang et al., 2017). However, significant differences on food composition among species (Figure 4A) could also suggest that morphological and acoustic differences shape their trophic relationships. In addition, according to optimal foraging theory, bats can prey upon insects based on their phenotypic characteristics; for example, bats with different peak frequencies will prey upon insects with different body sizes (Pyke et al., 1977; Perry and Pianka, 1997; Mello et al., 2004; Rollinson et al., 2013). In fact, significant differences were found in the insects captured by bats at the order level, such as Lepidoptera, which were the most abundant in the diet compositions of R. macrotis, R. osgoodi, and R. ferrumequinum. This may be because Lepidoptera are frequently a primary food resource of insectivorous bats due to their high abundance and wide global distribution (Hebert et al., 2003). This finding may also be due to the large size of lepidopterans, which have soft bodies and contain more energy, making them preferred prey for many predators (Krüger et al., 2014; Wray et al., 2021).
Factors influencing trophic niche differentiation
It has been shown that the trophic niche differentiation and stable coexistence of sympatric bats are influenced by many factors. In this study, differences were detected in the echolocation calls, body size, wing morphology, and bite force among five rhinolophid bat species. These differences may affect the flight patterns, foraging areas, and prey sizes of bats, and ultimately affect bat diet differentiation (Schoener, 1971; Norberg and Rayner, 1987; Mancina et al., 2012; Emrich et al., 2014).
Bats used echolocation calls to locate insects during foraging, and the parameters of echolocation calls, such as peak frequency, can significantly affect bat foraging habitats and prey types (Fullard et al., 1991; Bogdanowicz et al., 1997; Andreas et al., 2012; Gordon et al., 2019). In the present study, the results revealed that the peak frequency is a significant variance of all explanatory variables. This suggests that the echolocation calls of different bat species have an important influence on their dietary habits, similar to the findings of a previous study (Andreas et al., 2012). In general, bats with higher peak frequencies (short wavelengths) have smaller body size and fly at slower speeds, although they are more maneuverable than larger bats. These smaller bats are well-suited for foraging in dense spaces, and high-frequency echolocation calls help the bats move quickly and locate insects in cluttered environments (Jacobs et al., 2007). The prey detection hypothesis suggests that differences in echolocation frequencies may cause differentiation in prey sizes and that high-frequency calls are more sensitive to detecting smaller prey items (Barclay and Brigham, 1991; Jones, 1997). For example, R. euryale and R. mehelyi often exhibit sympatric distribution and are acoustically similar (R. euryale: 104.4 kHz; R. mehelyi: 106.8 kHz), but R. euryale tends to consume larger moths, while R. mehelyi prefers smaller moths (Salsamendi et al., 2006; Arrizabalaga-Escudero et al., 2018). A study on two cryptic species of pipistrelle bats found that 10 kHz difference in echolocation call frequency was not sufficient to influence the target strengths of the main prey types (Jones and Barlow, 2001). However, the large range of peak frequencies (57.88–101.92 kHz) detected among the bats in this study may have contributed to the differences in diet composition. For instance, in a previous study (Shi et al., 2009) conducted in the same cave as our study, R. macrotis had lower peak frequencies (~58 kHz) and a higher consumption of larger insects than coexisting R. lepidus (later identified as R. osgoodi, ~91 kHz) based on the measurement of prey size (Shi et al., 2009).
The morphological characteristics of bats are an important factor influencing the trophic niche differentiation of sympatric species. To some extent, morphological differences can reflect functional differences, which in turn affect the trophic niches of bat species (Pyke et al., 1977; Norberg and Rayner, 1987; Barclay and Brigham, 1991; Gnocchi et al., 2019). In the present study, body weight and forearm length were greater in R. ferrumequinum and R. affinis compared with the other species, with no significant difference between these two species and a significant difference from the remaining three species. The result of hierarchical partitioning analysis implies that forearm length and body weight play important roles in determining the trophic niche of sympatric bats. For instance, R. ferrumequinum and R. pusillus with different body sizes have significant differences in predation on Dipteran and Lepidopteran insects. Salsamendi et al. (2012) found that the niche partitioning of two sympatric sibling rhinolophid bats, R. euryale and R. mehelyi, was due to their differences in wing morphology, although their echolocation calls are almost identical. Andreas et al. (2013) suggested that the body size of three occurring horseshoe bats significantly affected the size of the prey. This suggests that, in addition to differences in echolocation calls, the body size of coexisting bats plays an important role in the differentiation of trophic niches, which is consistent with the predictions of morphological ecology (Findley and Black, 1983; Jacobs and Barclay, 2009).
Wing loading is an important parameter used to measure the flight ability of bats, and species with different wing morphology adapt to the local environment and food resources, allowing the more efficient use of food and habitat resources (Norberg and Rayner, 1987). In general, bats with high wing loading fly fast and forage in more open environments (Ye et al., 2009; Andreas et al., 2012; Mancina et al., 2012; Salsamendi et al., 2012). In this study, wing loading explained 15.0% of the diet composition, implying that wing loading had influence on inter-species trophic niche differentiation in bats. Not all pairwise comparisons of wing loading among bat species have shown significant differences, which may dilute the role of wing loading on interspecific trophic niche differentiation in bats. Furthermore, the bite force of bats is closely related to prey type, is influenced by factors including bat body weight and skull morphology, and is an important factor influencing the differentiation of trophic ecological of bats (Freeman and Lemen, 2010; García-Herrera et al., 2021). The bite force of bats in this study only explained a small part of diet composition, but was significantly correlated with body weight. This was similar to previous studies, in which larger bat species were generally found to have strong jaw and bite muscles that could produce greater bite force (Aguirre et al., 2003; Herrel et al., 2005; Freeman and Lemen, 2008). However, R. pusillus in this study consumed more Coleoptera than other species (RRA: 0.15; POO: 0.22) with small body size and low bite force, implying that bite force was not the main factor determining differences in the trophic ecology of bats. Because of its particularity (flight, nocturnal activity, migration, and social life), the trophic niche of a given bat species is dynamically affected by many factors, such as the spatial and temporal variation of food resources and environmental condition, as well as body condition, so the main factors affecting diet composition may change. It is difficult for a single factor to determine the trophic niche differentiation of bat species, and studying single factors alone may negatively impact our comprehensive understanding of the mechanisms of species coexistence in communities (Vesterinen et al., 2018; Gordon et al., 2019; Novella-Fernandez et al., 2020; Wray et al., 2021).
The CCA showed a low total explanation in this study. This pattern is more typical in ecological data (<10% of the variance explained) (ter Braak and Verdonschot, 1995) due to the nature of the presence–absence data type and the data including a large number of zeros, as was the case in this study. The dropping sample obtained from a single individual would not contain the full range of prey at the order level, but only one or a few arthropod orders. Although second-generation sequencing methods were used to obtain OTUs for species identification, this process generated more zeros in the data than traditional guano analysis methods.
In summary, this study investigated the trophic ecology and the effects of body size, wing morphology, bite force, and echolocation calls on the diet composition of five sympatric rhinolophid bats. The results revealed that all bats occupied a wide breadth of trophic niches in summer and that there was a low degree of trophic niche overlap between species. Significant differences in diet composition between species (except for between R. affinis and R. pusillus) were probably caused by the combined effect of the factors included in the study. CCA showed that body size and echolocation calls are the main factors that influenced the diet composition of bat communities, causing differentiation in trophic ecological niches among species, suggesting that the combined effects of multiple factors influenced the stability of bat communities.
Data availability statement
The original contributions presented in the study are publicly available. The data can be found in NCBI under BioProject PRJNA949878. Further inquiries can be directed to the corresponding author.
Ethics statement
The animal study was reviewed and approved by the Laboratory Animal Welfare and Ethics Committee of Jilin Agricultural University.
Author contributions
WD conceived and designed the study, participated in the field work and in data analysis and contributed to an original draft of the writing. AL contributed to the field work and data analysis. YC contributed to the data analysis and participated in the manuscript’s design. TL, LZ, JL, HL, and ZL contributed to the field work and data analysis. LJ, KS, and JF developed the study design, participated in the writing of manuscript and provided support for the study. All authors read and approved the final manuscript.
Funding
This work was supported by the National Natural Science Foundation of China (Grant Nos. 32171525, 32071492, and 31961123001) and Jilin Provincial Natural Science Foundation (Grant No. 20220101291JC).
Acknowledgments
We thank Xiaobin Huang and Yue Zhu for their assistance in field sampling, and Bo Luo and Xiaolin Li for their help in data analysis. We thank LetPub (www.letpub.com) for its linguistic assistance during the preparation of this manuscript.
Conflict of interest
The authors declare that the research was conducted in the absence of any commercial or financial relationships that could be construed as a potential conflict of interest.
Publisher’s note
All claims expressed in this article are solely those of the authors and do not necessarily represent those of their affiliated organizations, or those of the publisher, the editors and the reviewers. Any product that may be evaluated in this article, or claim that may be made by its manufacturer, is not guaranteed or endorsed by the publisher.
Supplementary material
The Supplementary material for this article can be found online at: https://www.frontiersin.org/articles/10.3389/fevo.2023.1108514/full#supplementary-material
Abbreviations
R. m, Rhinolophus macrotis; R. o, Rhinolophus osgoodi; R. f, Rhinolophus ferrumequinum; R. a, Rhinolophus affinis; R. p, Rhinolophus pusillus; OTUs, operational taxonomic units; PF, peak frequency; Fmin, minimum frequency; Fmax, maximum frequency; POO, percent of occurrence; RRA, relative read abundance.
Footnotes
References
Aguirre, L. F., Herrel, A., Van Damme, R., and Matthysen, E. (2003). The implications of food hardness for diet in bats. Funct. Ecol. 17, 201–212. doi: 10.1046/j.1365-2435.2003.00721.x
Alley, T. R. (1982). Competition theory, evolution, and the concept of an ecological niche. Acta Biotheor. 31, 165–179. doi: 10.1007/BF01857239
Andreas, M., Reiter, A., and Benda, P. (2012). Dietary composition, resource partitioning and trophic niche overlap in three forest foliage-gleaning bats in Central Europe. Acta Chiropt. 14, 335–345. doi: 10.3161/150811012x661657
Andreas, M., Reiter, A., Cepáková, E., and Uhrin, M. (2013). Body size as an important factor determining trophic niche partitioning in three syntopic rhinolophid bat species. Biologia 68, 170–175. doi: 10.2478/s11756-012-0139-1
Andriollo, T., Michaux, J. R., and Ruedi, M. (2021). Food for everyone: differential feeding habits of cryptic bat species inferred from DNA metabarcoding. Mol. Ecol. 30, 4584–4600. doi: 10.1111/mec.16073
Arrizabalaga-Escudero, A., Clare, E. L., Salsamendi, E., Alberdi, A., Garin, I., Aihartza, J., et al. (2018). Assessing niche partitioning of co-occurring sibling bat species by DNA metabarcoding. Mol. Ecol. 27, 1273–1283. doi: 10.1111/mec.14508
Ashrafi, S., Beck, A., Rutishauser, M., Arlettaz, R., and Bontadina, F. (2011). Trophic niche partitioning of cryptic species of long-eared bats in Switzerland: implications for conservation. Eur. J. Wildl. Res. 57, 843–849. doi: 10.1007/s10344-011-0496-z
Barclay, R. M. R., and Brigham, R. M. (1991). Prey detection, dietary niche breadth, and body size in bats: why are aerial insectivorous bats so small? Ame Nat. 137, 693–703. doi: 10.1086/285188
Biscardi, S., Russo, D., Casciani, V., Cesarini, D., Mei, M., and Boitani, L. (2007). Foraging requirements of the endangered long-fingered bat: the influence of micro-habitat structure, water quality and prey type. J. Zool. 273, 372–381. doi: 10.1111/j.1469-7998.2007.00337.x
Bogdanowicz, W., Csada, R. D., and Fenton, M. B. (1997). Structure of noseleaf, echolocation, and foraging behavior in the Phyllostomidae (Chiroptera). J. Mammal. 78, 942–953. doi: 10.2307/1382954
Bogdanowicz, W., Fenton, M. B., and Daleszczyk, K. (1999). The relationships between echolocation calls, morphology and diet in insectivorous bats. J. Zool. 247, 381–393. doi: 10.1111/j.1469-7998.1999.tb01001.x
Bokulich, N. A., Subramanian, S., Faith, J. J., Gevers, D., Gordon, J. I., Knight, R., et al. (2013). Quality-filtering vastly improves diversity estimates from Illumina amplicon sequencing. Nat. Methods 10, 57–59. doi: 10.1038/nmeth.2276
Chang, Y., Song, S., Li, A., Zhang, Y., Li, Z., Xiao, Y., et al. (2019). The roles of morphological traits, resource variation and resource partitioning associated with the dietary niche expansion in the fish-eating bat Myotis pilosus. Mol. Ecol. 28, 2944–2954. doi: 10.1111/mec.15127
Chesson, P. (2000). Mechanisms of maintenance of species diversity. Annu. Rev. Ecol. Syst. 31, 343–366. doi: 10.1146/annurev.ecolsys.31.1.343
Clare, E. L., Barber, B. R., Sweeney, B. W., Hebert, P. D. N., and Fenton, M. B. (2011). Eating local: influences of habitat on the diet of little brown bats (Myotis lucifugus). Mol. Ecol. 20, 1772–1780. doi: 10.1111/j.1365-294X.2011.05040.x
Codron, J., Duffy, K. J., Avenant, N. L., Sponheimer, M., Leichliter, J., Paine, O., et al. (2015). Stable isotope evidence for trophic niche partitioning in a south African savanna rodent community. Curr. Zool. 61, 397–411. doi: 10.1093/czoolo/61.3.397
Davies, K. T., Maryanto, I., and Rossiter, S. J. (2013). Evolutionary origins of ultrasonic hearing and laryngeal echolocation in bats inferred from morphological analyses of the inner ear. Front. Zool. 10, 2–15. doi: 10.1186/1742-9994-10-2
Deagle, B. E., Thomas, A. C., McInnes, J. C., Clarke, L. J., Vesterinen, E. J., Clare, E. L., et al. (2018). Counting with DNA in metabarcoding studies: how should we convert sequence reads to dietary data? Mol. Ecol. 28, 391–406. doi: 10.1111/mec.14734
Edgar, R. C. (2010). Search and clustering orders of magnitude faster than BLAST. Bioinformatics 26, 2460–2461. doi: 10.1093/bioinformatics/btq461
Emrich, M. A., Clare, E. L., Symondson, W. O. C., Koenig, S. E., and Fenton, M. B. (2014). Resource partitioning by insectivorous bats in Jamaica. Mol. Ecol. 23, 3648–3656. doi: 10.1111/mec.12504
Findley, J. S., and Black, H. (1983). Morphological and dietary structuring of a Zambian insectivorous bat community. Ecology 64, 625–630. doi: 10.2307/1937180
Freeman, P. W., and Lemen, C. A. (2008). Measuring bite force in small mammals with a piezo-resistive sensor. J. Mammal. 89, 513–517. doi: 10.1644/07-MAMM-A-101R.1
Freeman, P. W., and Lemen, C. A. (2010). Simple predictors of bite force in bats: the good, the better and the better still. J. Zool. 282, 284–290. doi: 10.1111/j.1469-7998.2010.00741.x
Fullard, J. H., Koehler, C., Surlykke, A., and Mckenzie, N. L. (1991). Echolocation ecology and flight morphology of insectivorous bats (Chiroptera) in South-Western Australia. Aust. J. Zool. 39, 427–438. doi: 10.1071/ZO9910427
García-Herrera, L. V., Ramírez-Fráncel, L. A., Guevara, G., Reinoso-Flórez, G., Sánchez-Hernández, A., Lim, B. K., et al. (2021). Foraging strategies, craniodental traits, and interaction in the bite force of Neotropical frugivorous bats (Phyllostomidae: Stenodermatinae). Ecol. Evol. 11, 13756–13772. doi: 10.1002/ece3.8014
Gnocchi, A. P., Huber, S., and Srbek-Araujo, A. C. (2019). Diet in a bat assemblage in Atlantic Forest in southeastern Brazil. Trop. Ecol. 60, 389–404. doi: 10.1007/s42965-019-00042-z
Gordon, R., Ivens, S., Ammerman, L. K., Fenton, M. B., Littlefair, J. E., Ratcliffe, J. M., et al. (2019). Molecular diet analysis finds an insectivorous desert bat community dominated by resource sharing despite diverse echolocation and foraging strategies. Ecol. Evol. 9, 3117–3129. doi: 10.1002/ece3.4896
Gotelli, N., Hart, E. M., and Ellison, A. (2015). EcoSimR: Null Model Analysis for Ecological Data. Available at: https://github.com/GotelliLab/EcoSimR/ (Accessed April 2015).
Hebert, P. D. N., Cywinska, A., Ball, S. L., and deWaard, J. R. (2003). Biological identifications through DNA barcodes. Proc. R. Soc. B-Biol. Sci. 270, 313–321. doi: 10.1098/rspb.2002.2218
Herrel, A., Podos, J., Huber, S. K., and Hendry, A. P. (2005). Bite performance and morphology in a population of Darwin's finches: implications for the evolution of beak shape. Funct. Ecol. 19, 43–48. doi: 10.1111/j.0269-8463.2005.00923.x
Holt, R. D. (2009). Bringing the Hutchinsonian niche into the 21st century: ecological and evolutionary perspectives. Proc. Natl. Acad. Sci. U. S. A. 106, 19659–19665. doi: 10.1073/pnas.0905137106
Hutchinson, G. E. (1959). Homage to Santa Rosalia or why are there so many kinds of animals? Ame Nat. 93, 145–159. doi: 10.1086/282070
Ingala, M. R., Simmons, N. B., and Perkins, S. L. (2018). Bats are an untapped system for understanding microbiome evolution in mammals. mSphere 3:e00397. doi: 10.1128/mSphere.00397-18
Jacobs, D. S., and Barclay, R. M. R. (2009). Niche differentiation in two sympatric sibling bat species, Scotophilus dinganii and Scotophilus mhlanganii. J. Mammal. 90, 879–887. doi: 10.1644/08-mamm-a-235.1
Jacobs, D. S., Barclay, R. M. R., and Walker, M. H. (2007). The allometry of echolocation call frequencies of insectivorous bats: why do some species deviate from the pattern? Oecologia 152, 583–594. doi: 10.1007/s00442-007-0679-1
Jin, L., Feng, J., Sun, K., Liu, Y., Wu, L., Li, Z., et al. (2005). Foraging strategies in the greater horseshoe bat (Rhinolophus ferrumequinum) on Lepidoptera in summer. Chin. Sci. Bull. 50, 1477–1482. doi: 10.1360/982004-831
Jones, G. (1997). “Acoustic signals and speciation: the roles of natural and sexual selection in the evolution of cryptic species” in Advances in the Study of Behavior. eds. P. J. B. Slater, J. S. Rosenblatt, C. T. Snowdon, and M. Milinski (California: Academic Press), 317–354.
Jones, G., and Barlow, K. E. (2001). “Cryptic species of echolocating bats” in Echolocation in Bats and Dolphins. eds. J. A. Thomas, C. F. Moss, and M. Vater (Chicago: University of Chicago Press), 345–349.
Jusino, M. A., Banik, M. T., Palmer, J. M., Wray, A. K., Xiao, L., Pelton, E., et al. (2019). An improved method for utilizing high-throughput amplicon sequencing to determine the diets of insectivorous animals. Mol. Ecol. Resour. 19, 176–190. doi: 10.1111/1755-0998.12951
Kasso, M., and Balakrishnan, M. (2013). Ecological and economic importance of bats (order Chiroptera). Int. Sch. Res. Notices 2013, 1–9. doi: 10.1155/2013/187415
Krüger, F., Clare, E. L., Greif, S., Siemers, B. M., Symondson, W. O. C., and Sommer, R. S. (2014). An integrative approach to detect subtle trophic niche differentiation in the sympatric trawling bat species Myotis dasycneme and Myotis daubentonii. Mol. Ecol. 23, 3657–3671. doi: 10.1111/mec.12512
Lai, J., Zou, Y., Zhang, J., and Peres-Neto, P. R. (2022). Generalizing hierarchical and variation partitioning in multiple regression and canonical analyses using the rdacca.Hp R package. Methods Ecol. Evol. 13, 782–788. doi: 10.1111/2041-210x.13800
Leal, E. S. B., Chaves, L. D. S., Prado Neto, J. G. D., Passos Filho, P. B. D., Ramalho, D. D. F., Guerra Filho, D. D. Q., et al. (2018). What constitutes the menu of Trachops cirrhosus (Chiroptera)? A review of the species' diet. Neotrop. Biol. Conserv. 13, 337–346. doi: 10.4013/nbc.2018.134.08
Letten, A. D., Ke, P.-J., and Fukami, T. (2017). Linking modern coexistence theory and contemporary niche theory. Ecol. Monogr. 87, 161–177. doi: 10.1002/ecm.1242
Li, G., Liang, B., Wang, Y., Zhao, H., Helgen, K. M., Lin, L., et al. (2007). Echolocation calls, diet, and phylogenetic relationships of Stoliczka’s trident bat, Aselliscus stoliczkanus (Hipposideridae). J. Mammal. 88, 736–744. doi: 10.1644/06-MAMM-A-273R.1
Mancina, C. A., García-Rivera, L., and Miller, B. W. (2012). Wing morphology, echolocation, and resource partitioning in syntopic Cuban mormoopid bats. J. Mammal. 93, 1308–1317. doi: 10.1644/11-mamm-a-331.1
Martin, M. (2011). Cutadapt removes adapter sequences from high-throughput sequencing reads. EMBnet J. 17, 10–12. doi: 10.14806/ej.17.1.200
Matthews, A. K., Neiswenter, S. A., and Ammerman, L. K. (2010). Trophic ecology of the free-tailed bats Nyctinomops femorosaccus and Tadarida brasiliensis (Chiroptera: Molossidae) in big bend National Park, Texas. Southwest. Nat. 55, 340–346. doi: 10.1894/JKF-08.1
Mello, M. A. R., Schittini, G. M., Selig, P., and Bergallo, H. G. (2004). Seasonal variation in the diet of the bat Carollia perspicillata (Chiroptera: Phyllostomidae) in an Atlantic Forest area in southeastern Brazil. Mammalia 68, 49–55. doi: 10.1515/mamm.2004.006
Metzner, W., Zhang, S., and Smotherman, M. (2002). Doppler-shift compensation behavior in horseshoe bats revisited auditory feedback controls both a decrease and an increase in call frequency. J. Exp. Biol. 205, 1607–1616. doi: 10.1242/jeb.205.11.1607
Nogueira, M. R., Peracchi, A. L., and Monteiro, L. R. (2009). Morphological correlates of bite force and diet in the skull and mandible of phyllostomid bats. Funct. Ecol. 23, 715–723. doi: 10.1111/j.1365-2435.2009.01549.x
Norberg, U. M., and Rayner, J. M. V. (1987). Ecological morphology and flight in bats (Mammalia; Chiroptera): wing adaptations, flight performance, foraging strategy and echolocation. Philos. Trans. R. Soc. B Biol. Sci. 316, 335–427. doi: 10.1098/rstb.1987.0030
Novella-Fernandez, R., Ibañez, C., Juste, J., Clare, E. L., Doncaster, C. P., and Razgour, O. (2020). Trophic resource partitioning drives fine-scale coexistence in cryptic bat species. Ecol. Evol. 10, 14122–14136. doi: 10.1002/ece3.7004
Oksanen, J., Blanchet, F. G., Friendly, M., Kindt, R., Legendre, P., McGlinn, D., et al. (2020). Vegan: Community Ecology Package. Availarble at: https://github.com/vegandevs/vegan/ (Accessed November 2020).
Pavey, C. R. (2020). Comparative echolocation and foraging ecology of horseshoe bats (Rhinolophidae) and Old World leaf-nosed bats (Hipposideridae). Aust. J. Zool. 68, 382–392. doi: 10.1071/ZO20047
Perry, G., and Pianka, E. R. (1997). Animal foraging: past, present and future. Trends Ecol. Evol. 12, 360–364. doi: 10.1016/S0169-5347(97)01097-5
Pyke, G. H., Pulliam, H. R., and Charnov, E. L. (1977). Optimal foraging: a selective review of theory and tests. Q. Rev. Biol. 52, 137–154. doi: 10.1086/409852
R Core Team. (2021). R: A Language and Environment for Statistical Computing. R Foundation for Statistical Computing. Available at: https://www.r-project.org (Accessed August 2021).
Razgour, O., Clare, E. L., Zeale, M. R. K., Hanmer, J., Schnell, I. B., Rasmussen, M., et al. (2011). High-throughput sequencing offers insight into mechanisms of resource partitioning in cryptic bat species. Ecol. Evol. 1, 556–570. doi: 10.1002/ece3.49
Rolfe, A. K., and Kurta, A. (2012). Diet of mormoopid bats on the Caribbean Island of Puerto Rico. Acta Chiropt. 14, 369–377. doi: 10.3161/150811012x661684
Rolfe, A. K., Kurta, A., and Clemans, D. L. (2014). Species-level analysis of diets of two mormoopid bats from Puerto Rico. J. Mammal. 95, 587–596. doi: 10.1644/13-mamm-a-190
Rollinson, D. P., Coleman, J. C., and Downs, C. T. (2013). Seasonal differences in foraging dynamics, habitat use and home range size of Wahlberg's epauletted fruit bat in an urban environment. Afr. Zool. 48, 340–350. doi: 10.3377/004.048.0218
Ross, H. A., Murugan, S., and Li, W. L. S. (2008). Testing the reliability of genetic methods of species identification via simulation. Syst. Biol. 57, 216–230. doi: 10.1080/10635150802032990
Russell, I. J., and Kossl, M. (1999). Micromechanical responses to tones in the auditory fovea of the greater mustached bat's cochlea. J. Neurophysiol. 82, 676–686. doi: 10.1152/jn.1999.82.2.676
Salinas-Ramos, V. B., Herrera Montalvo, L. G., Leon-Regagnon, V., Arrizabalaga-Escudero, A., and Clare, E. L. (2015). Dietary overlap and seasonality in three species of mormoopid bats from a tropical dry forest. Mol. Ecol. 24, 5296–5307. doi: 10.1111/mec.13386
Salsamendi, E., Aihartza, J., Goiti, U., Almenar, D., and Garin, I. (2006). Echolocation calls and morphology in the Mehelyi's (Rhinolophus mehelyi) and mediterranean (R. euryale) horseshoe bats: implications for resource partitioning. Hystrix 16, 149–158. doi: 10.4404/hystrix-16.2-4353
Salsamendi, E., Garin, I., Arostegui, I., Goiti, U., and Aihartza, J. (2012). What mechanism of niche segregation allows the coexistence of sympatric sibling rhinolophid bats? Front. Zool. 9, 1–12. doi: 10.1186/1742-9994-9-30
Saunders, M. B., and Barclay, R. M. R. (1992). Ecomorphology of insectivorous bats: a test of predictions using two morphologically similar species. Ecology 73, 1335–1345. doi: 10.2307/1940680
Schmieder, R., and Edwards, R. (2011). Quality control and preprocessing of metagenomic datasets. Bioinformatics 27, 863–864. doi: 10.1093/bioinformatics/btr026
Schoeman, M. C., and Jacobs, D. S. (2003). Support for the allotonic frequency hypothesis in an insectivorous bat community. Oecologia 134, 154–162. doi: 10.1007/s00442-002-1107-1
Schoeman, M. C., and Jacobs, D. S. (2011). The relative influence of competition and prey defences on the trophic structure of animalivorous bat ensembles. Oecologia 166, 493–506. doi: 10.1007/s00442-010-1854-3
Schoener, T. W. (1971). Theory of feeding strategies. Annu. Rev. Ecol. Syst. 2, 369–404. doi: 10.1146/annurev.es.02.110171.002101
Schoener, T. W. (1974). Resource partitioning in ecological communities. Science 185, 27–39. doi: 10.1126/science.185.4145.27
Shi, L., Feng, J., Liu, Y., Ye, G., and Zhu, X. (2009). Is food resource partitioning responsible for deviation of echolocation call frequencies from allometry in Rhinolophus macrotis? Acta Theriol. 54, 371–382. doi: 10.4098/j.at.0001-7051.099.2008
Stebbings, R. E. (1968). Measurements, composition and behaviour of a large colony of the bat Pipistrellus pipistrellus. J. Zool. 156, 15–33. doi: 10.1111/j.1469-7998.1968.tb08576.x
ter Braak, C. J. F., and Verdonschot, P. F. M. (1995). Canonical correspondence analysis and related multivariate methods in aquatic ecology. Aquat. Sci. 57, 255–289. doi: 10.1007/BF00877430
Thabah, A., Li, G., Wang, Y., Liang, B., Hu, K., Zhang, S., et al. (2007). Diet, echolocation calls, and phylogenetic affinities of the great evening bat (Ia io; Vespertilionidae): another carnivorous bat. J. Mammal. 88, 728–735. doi: 10.1644/06-MAMM-A-167R1.1
Vesterinen, E. J., Puisto, A. I. E., Blomberg, A. S., and Lilley, T. M. (2018). Table for five, please: dietary partitioning in boreal bats. Ecol. Evol. 8, 10914–10937. doi: 10.1002/ece3.4559
Wei, L., Zhou, S., Zhang, L., Liang, B., Hong, T., and Zhang, S. (2006). Characteristics of echolocation calls and summer diet of three sympatric insectivorous bats species. Zool. Res. 27, 235–241. doi: 10.3321/j.issn:0254-5853.2006.03.002
Wilson, D. E., and Mittermeier, R. A. (2019). Handbook of the Mammals of the World, Bats Barcelona: Lynx Edicions.
Wray, A. K., Peery, M. Z., Jusino, M. A., Kochanski, J. M., Banik, M. T., Palmer, J. M., et al. (2021). Predator preferences shape the diets of arthropodivorous bats more than quantitative local prey abundance. Mol. Ecol. 30, 855–873. doi: 10.1111/mec.15769
Ye, G., Shi, L., Sun, K., Zhu, X., and Feng, J. (2009). Coexistence mechanism of two sympatric horseshoe bats (Rhinolophus sinicus and Rhinolophus affinis) (Rhinolophidae) with similar morphology and echolocation calls. Shengtai Xuebao 29, 5330–5338.
Zeale, M. R. K., Butlin, R. K., Barker, G. L. A., Lees, D. C., and Jones, G. (2011). Taxon-specific PCR for DNA barcoding arthropod prey in bat faeces. Mol. Ecol. Resour. 11, 236–244. doi: 10.1111/j.1755-0998.2010.02920.x
Zhang, H., Chen, F., Wang, Y., Xu, X., Yuan, Q., Yang, Y., et al. (2017). The temporal dynamics and structure of insect Community in the Vegetable Garden in Jinning County, Kunming. Chin. J. Biol. Control 33, 56–62. doi: 10.16409/j.cnki.2095-039x.2017.01.009
Keywords: rhinolophid bats, diet, trophic niche, sympatric, high-throughput sequencing
Citation: Dai W, Li A, Chang Y, Liu T, Zhang L, Li J, Leng H, Li Z, Jin L, Sun K and Feng J (2023) Diet composition, niche overlap and partitioning of five sympatric rhinolophid bats in Southwestern China during summer. Front. Ecol. Evol. 11:1108514. doi: 10.3389/fevo.2023.1108514
Edited by:
Ilaria Agostini, National Scientific and Technical Research Council (CONICET), ArgentinaReviewed by:
Luis Gerardo Herrera M., Universidad Nacional Autónoma de México, MexicoMariano Sebastian Sánchez, CONICET Institute of Subtropical Biology (IBS), Argentina
Copyright © 2023 Dai, Li, Chang, Liu, Zhang, Li, Leng, Li, Jin, Sun and Feng. This is an open-access article distributed under the terms of the Creative Commons Attribution License (CC BY). The use, distribution or reproduction in other forums is permitted, provided the original author(s) and the copyright owner(s) are credited and that the original publication in this journal is cited, in accordance with accepted academic practice. No use, distribution or reproduction is permitted which does not comply with these terms.
*Correspondence: Keping Sun, sunkp129@nenu.edu.cn; Longru Jin, jinlr915@nenu.edu.cn