- 1School of Economics, Hebei University, Baoding, China
- 2Research Center of Resources Utilization and Environmental Conservation, Hebei University, Baoding, China
- 3College of Economics and Management, Northwest A&F University, Yangling, China
- 4Center for Resource Economics and Environment Management, Northwest A&F University, Yangling, China
Introduction: The National Key Ecological Functional Areas (NKEFAs) are location-oriented ecological engineering of China, which rely on the main functional area planning. The co-benefits of ecological product supply and ecological environment improvement of NKEFAs has not been fully assessed in the literature.
Methods: NKEFAs is considered a quasi-natural experiment, and the time-varying difference-in-differences (DID) model is used to assess the impact of NKEFAs on carbon sequestration (CS) and environmental quality (EQ) based on the panel data of 330 cities in China from 2001 to 2019. Then, we explore whether the co-benefits of ecological product supply and eco-environment protection can be achieved.
Results and discussion: NKEFAs can enhance CS and EQ and thus achieve co-benefits for both. NKEFAs can achieve the co-benefits of CS and EQ through territory spatial allocation and labor force aggregation, but industrial structure upgrading only positively mediates the impact of NKEFAs on CS. The co-benefits of CS and EQ are heterogeneous across functional area types, geospatial locations, and quantiles, while only CS at windbreak-sand fixation area, northwestern region, and low quantile regions is enhanced. This study makes a theoretical and methodological contribution to the existing literature on the policy effect assessment of ecological engineering. It also provides a comprehensive framework for evaluating the ecological effects of relevant policies in other countries by integrating the co-benefits of ecological products and eco-environment, analyzing regional heterogeneity, and exploring the underlying mechanisms.
1. Introduction
The effectiveness of ecological policies and environmental regulation has received widespread global attention in recent decades, regarding that climate change and environmental degradation are already critical problems that threatening humankind (Dong et al., 2022). Facing the above threat, Chinese government has committed to reaching peak carbon emissions by 2030 and carbon neutrality by 2060 (Yu and Zhang, 2021). Therefore, achieving the goals of dual-carbon strategy and improving ecological environment are the basic need of high-quality green development. Territorial space use is mainly expressed as the interaction between ecosystems and human activities (Ball et al., 2010). Inappropriate ways and unreasonable structure of human activities induce terrestrial ecosystem degradation, which significantly explains the growing carbon emission intensity and environmental problems (Gao et al., 2013). The rough expansion of industrialization and urbanization results in structural contradiction in land space utilization, encroaching on the ecological space (Lin et al., 2020).
In order to reasonably use the territory, China issued the National Main Function Area Planning in December 2010, which specifies the main functions of different regions (Liu J. et al., 2017; Liu W. et al., 2017), gradually form a spatial development pattern of the national territory in harmony with population, economy, resources and environment. According to the development content, the main functional areas can be divided into urbanized areas, main agricultural producing areas, and key ecological functional areas. Among them, the National Key Ecological Function Areas (NKEFAs) are important to ensure national ecological security, primarily for protecting the ecological environment and enhancing the ecological product supply. NKEFAs bear important ecological functions such as water conservation, soil conservation, windbreak sand fixation and biodiversity maintenance. Currently, China established the first batch of NKEFAs covering 436 counties at the end of 2010 (Xu et al., 2018), to which 240 new counties were added in 2016 (Figure 1). Since 2011, NKEFAs have become the largest regional ecological policy in China (Zhu and Chen, 2020).
Over the years since the establishment of NKEFAs, it is worthwhile to explore its ecological benefits in the context of the dual carbon strategy. The existing studies focus on the effect of fiscal transfer payments, the quantitative of ecosystem service changes in NKEFAs, etc. (Miao and Zhao, 2019; Xu et al., 2019; Liu et al., 2020). Early studies indicated that the ecological benefits of NKEFAs is unobvious, which is related to the precision and target matching of its transfer payment policy (Li et al., 2013, 2014; Li and Li, 2014). found that the transfer payment of NKEFAs promoted environment quality in the Qinba Mountains of Shaanxi, but relatively weak. In contrast, Xu and Zhang (2017) found that the positive ecological effect of transfer payment was relatively significant for NKEFAs in Shaanxi Province, with the ecological environment in the base period playing an indispensable role. Additionally, by comparing the environmental changes before and after the NKEFAs through GIS technology, its eco-environment shows an improvement trend (Liu et al., 2018; Zhang M. et al., 2022, Zhang T. et al., 2022), varying with different function area types (Hou et al., 2018). In addition, The afforestation area of NKEFAs received the attention of scholars (Bryan et al., 2018; Pan, 2021), and there is also literature focusing on the carbon reduction effect or air pollution control of ecological policies (Dong et al., 2022; Gao et al., 2022; Liu et al., 2022).
Existing studies have deeply investigated the ecological effect of NKEFAs but have limitations. First, the relevant literature focuses on transfer payment, most of which is the experience summary and theoretical discussion. Second, the quantitative analysis is mostly case studies, and the interference of other ecological policies is nearly unavoidable in GIS-based analysis. More importantly, existing studies focus more on the eco-environmental effect, but neglect the major goal of NKEFAs to enhance the ecological product supply (Zhang M. et al., 2022; Zhang T. et al., 2022). Carbon sequestration (CS) is the ability of vegetation to absorb and store carbon dioxide, which is an important ecological product (Liu and Song, 2022). So, the achievement of NKEFAs’ ecological goals requires both enhancing CS and improving environmental quality (EQ), and to promote the joint improvement of both, which is also known as co-benefits. Therefore, it is necessary to assess the NKEFAs’ CS capacity and co-benefits of CS and EQ.
Specifically, we consider the NKEFAs as a “quasi-natural experiment” (Zhang M. et al., 2022; Zhang T. et al., 2022). Then the panel data of 330 cities in China from 2001 to 2019 and a time-varying difference-in-differences (DID) model are used to assess the impact of NKEFAs on CS and EQ, so as to identify the co-benefits of ecological product supply and ecological environment improvement. This study contributes to experience accumulation and institutional improvement to implement sustainable ecological policies in China. It also provides a reference for the synergistic balance between ecological product supply and eco-environmental protection in other countries.
This paper has the advantage of the policy stripping of DID model and large sample data to effectively assess the policy effect of NKEFAs. In addition, the marginal contributions are as follows. First, unlike previous studies, this paper integrates CS and EQ into a framework to investigate whether NKEFAs can achieve the co-benefits of ecological product supply and ecological environment. Second, existing assessments based on inter-provincial average treatment effect (ATE) can hardly accurately reflect individual differences in ecological features within provinces. So, a more detailed examination at a smaller-scale of prefecture level (Rosenthal and Strange, 2003) can eliminate the homogenization error in provincial macro data. Third, existing studies based on GIS technology to compare and analyze the changes of ecological effect before and after the policy implementation or between covered and non-covered areas are difficult to exclude the influence of other ecological policies. The identification using DID model can effectively strip the interference of other similar policies in the same period.
2. Materials and methods
2.1. Data sources
The panel data of 330 cities at the prefecture level and above in China from 2001 to 2019 are selected as the study samples. The areas covered by NKEFAs are manually collated according to relevant policy documents (Table 1). NPP is obtained from the MOD17A3HGF product based on the MODIS satellite launched by NASA, the spatial resolution is 500 m. EQ data came from the CHEQ dataset (2001–2019) released by China’s National Earth System Science Data Center,1 with a spatial resolution of 0.0089°. The land use data is derived from the global land cover product data2 of the European Space Agency’s (ESA) Climate Change Initiative (CCI), with a resolution of 300 m. The data of temperature, precipitation, and sunshine hours were obtained from the annual data set of China’s surface climate data from the China Meteorological Data Network.3 The socio-economic data is provided by the China City Statistical Yearbook, China Regional Economic Statistical Yearbook, and provincial statistical yearbooks of previous years.4 The missing data is supplemented by prefecture statistical yearbooks or by interpolation. The variance inflation factor (VIF) of each variable is significantly <10, there is no obvious multicollinearity problem among the explanatory variables.
2.2. Variable selection
2.2.1. Explained variables: Carbon sequestration and environmental quality
1. Carbon sequestration (CS). The scale of CS is measured by the net primary productivity (NPP) of vegetation, which can indicate the supply scale of ecological products. CS is mainly the process of absorbs CO2 from the atmosphere through photosynthesis of plants (UNFCCC, 1997). NPP is the residual of gross primary productivity (GPP) minus the value of autotrophs respiration, which can be deduced from the CO2 absorbed by plants and the dry matter produced (He et al., 2018). The chemical equation is 6CO2 + 6H2O → C6H12O6 + 6O2. Vegetation photosynthesis can fix 1.63 kg of CO2 for 1 kg of dry matter produced, and the carbon content of dry matter is about 45% in the total NPP. Therefore, the CO2 per unit area fixed by vegetation is WCO2 = NPP/0.45 × 1.63 (g/m2). The scale of CS is WCO2 multiplied by the vegetation cover area (Chen et al., 2020).
2. Environmental quality (EQ). EQ mainly reflects the overall condition of ecological environment, which is characterized by historical data from China’s High-resolution Eco-Environmental Quality (CHEQ) dataset (Xu et al., 2021). The dataset is developed based on indicators such as habitat, greenness, dryness, heat, humidity, and abundance index, with a data range of 0–1. It is highly consistent with the environmental index provided by the Ministry of Ecology and Environment of China and can reflect the quality effect of environmental benefits.
2.2.2. Core explanatory variable: NKEFAs
1. The scope of cities covered. Given that the list in the policy document is at the county level, according to the summary and collation of counties, there are 171 prefecture-level cities covered by the NKEFAs (111 first list and 60 additional list).
2. The time node of policy implementation. According to the different times of policy introduction and new list, 2011 and 2016 are the starting time of the two batches, respectively.
2.2.3. Control variables
In the econometric model, exogenous variables of socio-economic and natural climatic variables need to be controlled (Zhang M. et al., 2022; Zhang T. et al., 2022). In this paper, the following are included, population density (DEN), reflects the expansion of population size; economic growth (GDP per capita, PGDP), reflects the general situation of regional economic (Tang et al., 2021a); urbanization rate (URBAN), reflects the expansion of urbanization; industrial structure adjustment (STURC), reflects the change in the share of the three industries in total output (Guo et al., 2020); urban–rural income gap (GAP), reflects the change in income of urban and rural residents; opening to trade (OPEN), reflects the level of openness to foreign trade; transportation conditions (TRANS), reflects the gradual improvement of transportation infrastructure. The variables of natural conditions select precipitation (PRE), temperature (TEM), and sunshine hours (SUN) to test the shock of climate change on eco-environment.
2.3. Theoretical framework
As specific areas in the main function area plan to undertake ecological functions and maintain ecological security, the NKEFAs belong to the location-oriented ecological engineering in China (Lin and Qi, 2021). The positive incentives for NKEFAs are mainly formed by transfer payments to local governments. The ecological compensation transfer payment contract signed between central government and local governments form a principal-agent relationship, thus motivate local governments to invest more efforts in ecological governance (Zhang and Li, 2015), and also enhance the fiscal capacity of local governments to provide basic public services (Fu and Miao, 2015). The NKEFAs can mainly enhance the service function of ecological products and the eco-environment quality with government actions. To this end, we have established a framework system for NKEFAs to influence the co-benefits of CS and EQ (Figure 2).
Theoretically, fiscal support and social participation can help NEKFAs protect and restore the ecological environment, thereby enhancing CS and EQ. We argue that NKEFAs can influence the co-benefits of CS and EQ through territory space allocation, industrial structure upgrading, and labor force aggregation. First, the NKEFAs comprehensively delineate the ecological red line. This requires strict control of development intensity and scope in territorial development. Moreover, urban construction and industrial development should match the regional carrying capacity of resources and environments (Huang et al., 2021). Restricted development areas and ecological red lines assume different main functions, promote the optimization of the territorial utilization pattern, and help improve the efficiency of land spatial allocation (Jiang and Chen, 2021). The land spatial allocation reinforces the target requirement of prioritizing eco-environmental protection (DeFries et al., 2007), while the ecological space expansion contributes to co-benefits. Second, in NKEFA construction, a targeted negative list system is implemented for industrial access, which specifies a list of industries restricted and prohibited for suitable industry development. For industries that do not match the main function, strict industrial policy can promote the gradient transfer of polluting enterprises to areas with low environmental costs or to service industries with less pollution (Zhou et al., 2017; Luo and Qi, 2021). Then NKEFAs can promote industrial structure upgrading, conducive to pollutant emission reduction. In addition, the accompanying technical upgrade has also reduced the constraints of environment on economic development (Liu and Lin, 2020). Third, the reallocation of NKEFAs to the territory space and industrial layout has accelerated the adjustment of factors and the mobility of labor. Territorial allocation and industrial upgrading enable the labor force to move orderly from restricted development areas to key development areas or urbanized areas (Zhang M. et al., 2022; Zhang T. et al., 2022). As a result, urban population clustering also alleviates employment pressure (Fan, 2016). Labor force aggregation in space with permissible environmental capacity promotes the reallocation and structural adjustment of factors. This is conducive to preventing environmental pollution and effectively using resources.
2.4. Baseline regression
The DID (difference in difference) model is a widely used method for evaluating policy effects in recent years (Tang et al., 2021b). Its basic idea is to set the implementation of a policy as an exogenous “quasi-natural experiment” (Wang and Ge et al., 2022), so as to strip the net effect of this specific policy. The NKEFAs can lead to ecological differences not only between the policy-covered and non-covered areas but also before and after the policy implementation (Zhang M. et al., 2022; Zhang T. et al., 2022). Therefore, DID model can be applied to assess the policy effects of the NKEFAs.
Considering that the National Plan for Main Functional Areas was launched at the end of December 2010, the first batch of NKEFAs was announced simultaneously. And the list of additional counties was announced in 2016. This paper selects 2011 as the starting year for the NKEFAs. Considering the difference in time for the establishment of NKEFAs, time-varying DID model is employed, which is constructed as follows:
Where Yit denotes the explained variable, including CS and EQ, DIDit denotes the core explanatory variable, i.e., a dummy variable for this ecological policy. If region i is established as an NKEFA in year t, DIDit = 1; otherwise, DIDit = 0. β reflects the net effect of NKEFAs, if the NKEFAs help to enhance CS and EQ, β is significantly positive. controlit indicates the set of control variables, μi denotes the area-fixed effect, γt denotes the time-fixed effect, and εit is the random disturbance term.
The list in the document is at the county scale, while the scale of this research is at the prefecture level. In the DID model, for a prefecture-level city, if a NKEFA cover the county within its jurisdiction, this city belongs to the coverage of this NKEFA, then the list of counties can be summarized to the prefecture-level city. If the counties in a city are covered in both the first batch and the added batch, then the policy starting time of this city is 2011.
2.5. Parallel trend test and dynamic effect
DID model needs to satisfy the parallel trend hypothesis. Before the establishment of NKEFAs, the ecological effects of the treatment and control groups maintained basically the same trend of change. The parallel trend hypothesis test generally uses the event study approach (ESA), which also looks at the dynamic effect and persistence of policy impact. In this way, whether a significant difference exists in the changing trend can be accurately determined between the treatment and control groups in the NKEFAs (Wu et al., 2021). So, the ESA is applied to test the parallel trend hypothesis, and then analyze the dynamic policy effect (McGavock, 2021).
Considering that the Ministry of Finance promulgated the Transfer Payment (Pilot) Measures for NEKFAs in 2009, and some important ecological regions have started to pilot transfer payment. The year before the NKEFA implementation (2010) is used as the reference group for the parallel trend test. Referring to related studies (Chen, 2017; Tao et al., 2021), Eq. (1) is expanded as:
Where treati × yeart is the interaction term of the grouping variable treati and the year dummy variable yeart. The year before this policy (2010) is not introduced. βt denotes the policy effect in each year. If βt is not significant until 2010, the parallel trend hypothesis is satisfied.
3. Results
3.1. Baseline regression result
The baseline regression results show that (Table 2), whether or not controlling for city fixed effect and year fixed effect, or controlling for characteristic variables, the coefficients of DID are all positive significantly. NKEFAs exert a significant positive impact on CS, indicate that the NKEFAs enhanced the CS and EQ of the covered cities significantly, and achieve the ecological co-benefits. According to the coefficients of DID in columns (4) and (8), compared to that of cities in non-NKEFAs, the ATE of cities at the prefecture level is improved by about 2.1625 and 0.0031, respectively.
3.2. Parallel trend test and policy dynamic effect
The coefficients of the NKEFAs on CS and EQ are all insignificant, fluctuated around 0 for each year before 2010 (Figure 3). CS and EQ prior this policy was introduced did not differ significantly between treatment groups and control groups. Hence, the parallel trend hypothesis is satisfied, and the treatment and control groups are comparable ex ante, this policy is suitable for evaluation using DID model.
For dynamic effects, the coefficients of NKEFAs changed from negative to significantly positive, the positive contribution to ecological benefits gradually became prominent. After the establishment of NKEFAs, the impact on CS and EQ was significantly positive in the ATE, which showed a dynamic upward trend with time. For CS, the positive effect begins to stabilize 2 years after the establishment of the NKEFAs, and the obvious positive contribution after 2017 due to the second list of added regions, further contributing to the CS scale. For EQ, the positive effect basically maintained a stable upward trend. The dynamic effect results further showed that the NKEFAs promoted the co-benefits of CS and EQ in the covered areas.
3.3. Robustness test
3.3.1. Placebo test
The purpose of placebo test is to rule out the influence of omitted variables, randomness factors, etc., before and after this policy. Then a counterfactual framework is used to carry out the placebo test (Cisilino et al., 2019). Specifically, the non-parametric permutation test is employed with an unduplicated random sampling of all cities and times (Lu et al., 2021). Since there are 171 cities covered by the two batches, so 171 cities are first randomly selected from the overall samples as the treatment group and other cities as the control group. Then a certain year is randomly selected from 2001 to 2019 as the establishment time of the NKEFAs. Finally a quasi-randomized experiment at two levels of city-time is constructed. To improve the explanatory power of placebo test, this random process is repeated 500 times, and obtain the kernel density distribution map of the DID coefficients under multiple random shocks (Figure 4). The coefficient distribution of CS and EQ is slightly deviated from 0, most of the p-values are >0.1, and the DID coefficients of the baseline regression also are located in the low tail of the kernel distribution, which are obvious outliers. So, the placebo test based on the counterfactual perspective confirms that the model setting is less disturbed by issues such as omitted variables or random factors, and does not affect the reliability of the baseline results.
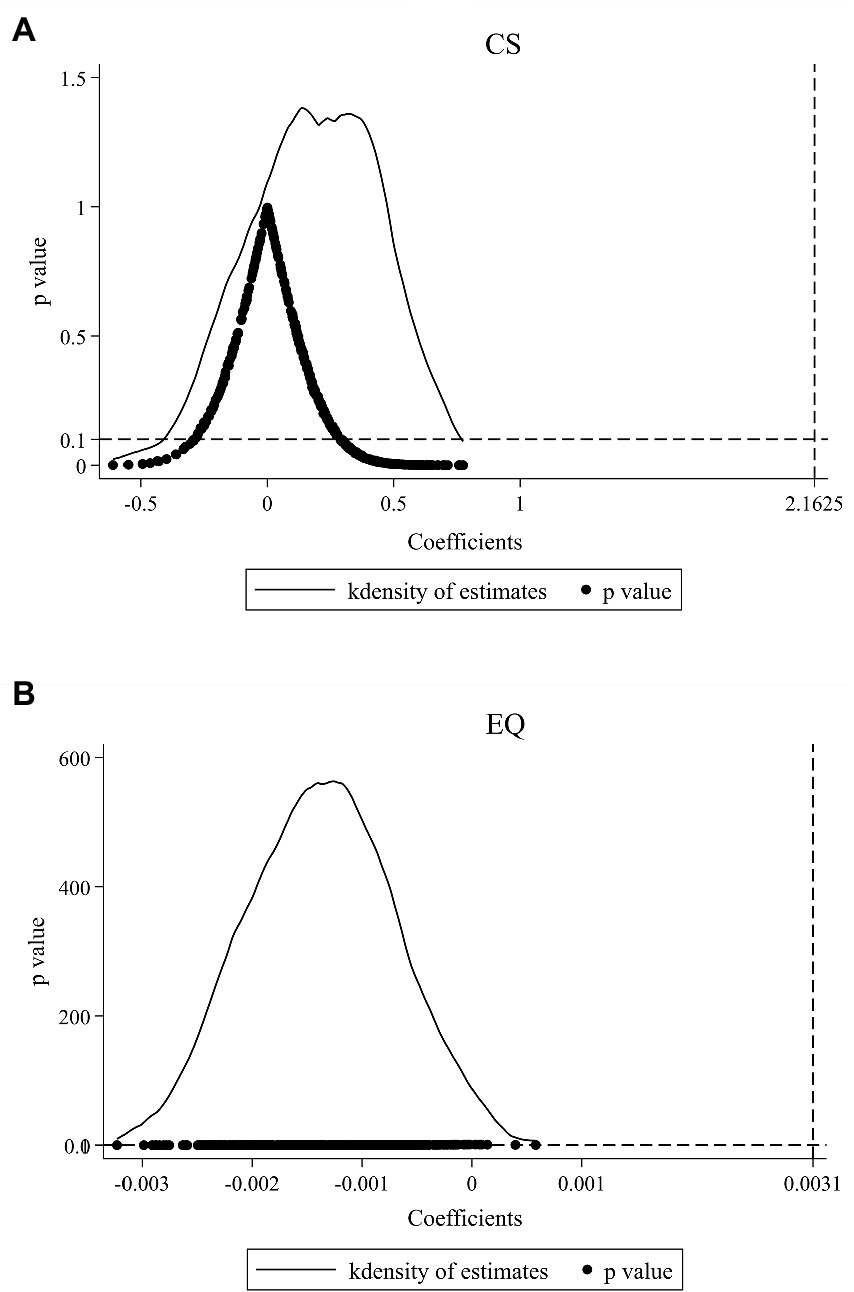
Figure 4. The placebo test of CS (A) and EQ (B). The X-axis is the coefficients of DID for the 500 random processes. The vertical lines on the right side are the baseline coefficients of DID, all significantly located in the low-tailed of the kernel density distribution.
3.3.2. PSM-DID
According to the goal of optimizing the spatial pattern of land use, the NKEFAs are important areas selected to undertake ecological functions and maintain ecological security, so their delineation may not be completely random (Lin and Qi, 2021), and prone to endogeneity caused by selection bias. If endogeneity exists, Propensity Score Matching (PSM) is an effective way to solve the problem under the non-randomized experiments (Shi et al., 2018). To reduce the selection bias of DID, the PSM combined with DID (PSM-DID) is used to assess the ecological co-benefits of NKEFAs. First, the control variables are used to predict the probability of each city being set as a NKEFA (Logit regression), and the treatment group is delineated. Second, the k-nearest neighbor matching method in the caliper (k takes 1, that is, matches according to 1:3) is used to match the control group for the treatment group, ensure that there is no significant systemic difference between the treatment group and the control group before the policy. Third, the matching sample is used for DID evaluation.
The regression results are shown in columns (1) and (2) of Table 3, the estimated coefficients of DID are 2.2004 and 0.0029 significantly, which are consistent with the baseline regression, respectively. So the positive promotion effects of NKEFAs on CS and EQ are robust.
3.3.3. Excluding other ecological policy interference
Some other ecological policies to improve the environment was underway at the same time when the NKEFAs was established. Other ecological policies such as the Grassland Ecological Protection Subsidy and Incentive Policy began in 2011, the New Round of Plans for Returning Farmland to Forests and Grasses in 2014, the Opinions on Accelerating the Construction of Ecological Civilization in 2015, may also affect the CS and EQ. To exclude the interference of other ecological policies, the interaction terms of the grouping variable (treat) with the time dummy variables for 2011, 2014, and 2015 are introduced in the baseline regression model (Tao et al., 2021). The results in column (3) and column (4) of Table 3 show that the impacts of NKEFAs on CS and EQ are still all significantly positive, the results are robust.
3.3.4. Substituting explained variables
Ecological improvement is positively correlated with vegetation change. Meanwhile, Normalized Difference Vegetation Index (NDVI) is closely related to vegetation cover, biomass and productivity, which can also indirectly reflect the supply capacity of ecological products. So, NDVI can be used as a proxy variable for CS. The EQ is mainly measured by the inverse indicator of atmospheric pollution level (Wang and Tang, 2019). In view of the availability of data, water environment-related indicators are not considered for the time being. The level of atmospheric pollution is characterized by PM2.5 concentration from RS interpretation, and PM2.5 is made positive by taking the inverse. It can be found that the estimated coefficients of DID on both NDVI and 1/PM2.5 are significantly positive. NKEFAs can significantly enhance NDVI and reduce haze pollution, indicating that the positive contribution of NKEFAs on CS and EQ remains robust.
3.3.5. Eliminating special samples
The NKEFAs is closely related to the ecological characteristics and environmental carrying capacity of the covered areas, while cities such as municipalities directly under the central government, provincial capitals, special economic zones may have obvious systematic differences in ecological endowments from other cities due to their own positioning. To further ensure reliability, the baseline models are re-estimated after eliminating these special samples (292 remaining). The results in columns (7) and (8) of Table 3 show that the coefficients of DID are still all significantly positive. After eliminating some samples, the positive effect of NKEFAs to CS and EQ remains robust.
3.4. Mechanism analysis
According to the framework system, the transmission mechanism of NKEFAs affecting CS and EQ is explored in terms of territorial spatial allocation, industrial structure upgrading, and labor force aggregation. For the model setting, the stepwise method is borrowed (Baron and Kenny, 1986; Chan et al., 2012; Tang et al., 2020): first test the impact of NKEFAs on mediating variables, second test the effects of NKEFAs and mediating variables on CS and EQ (Table 4).
Based on the theoretical framework, the mediating variables included territorial spatial allocation (TERRI), industrial structural upgrading (INDUS), and labor force transfer (LABOR). Ecological space mainly provides functions such as ecological products or ecological services, include forest land, grassland, water, et al. TERRI is characterized by the proportion of ecological space to the national territory. INDUS is characterized by the ratio of added-value of the tertiary industry to added-value of the secondary industry, so as to reflect the transformation trend of the leading industry to the service industry (Yu, 2015). Due to the high overlap in spatial distribution between counties covered by NKEFAs and national poverty-stricken counties (Wang and Gao, 2018), LABOR is characterized by the proportion of labor in agriculture, forestry, animal husbandry and fishery to the total population year-end, to reflect the rural labor mobility among urban–rural areas and industries.
3.4.1. Territory spatial allocation effect (TERRI)
The NKEFAs has a markedly positive impact on TERRI, and TERRI has an obvious positive influence on CS and EQ. Therefore, the territory space allocation positively mediates the co-benefits of NKEFAs on CS and EQ. NKEFAs can enhance CS and EQ by enhancing the share of ecological space to optimize the spatial allocation structure of the territory, thus achieving ecological co-benefits.
3.4.2. Industrial structure upgrading effect (INDUS)
NKEFAs has a remarkably positive influence on INDUS. However, INDUS only exerts a positive effect on CS but failed to obviously contribute to EQ in the covered areas. This indicates that INDUS only positively mediates the CS enhancement in NKEFAs. The industrial structure upgrading by increasing the tertiary industry proportion in the national economy only promotes the enhancement of CS in NKEFAs, instead of EQ, thus failing to realize ecological co-benefits.
3.4.3. Labor force aggregation effect (LABOR)
NKEFAs has a significant positive impact on the LABOR, which can increase the labor proportion in agriculture, forestry, animal husbandry, and fisheries. Meanwhile, NKEFAs makes a significant positive contribution to both CS and EQ. Therefore, labor force agglomeration positively mediates the effect of NKEFAs on CS and EQ. NKEFAs can enhance CS and EQ through inter-industrial mobility and labor agglomeration to achieve ecological co-benefits.
In summary, the NKEFAs can achieve ecological co-benefits of CS and EQ through territory spatial allocation and labor force aggregation, and industrial structure upgrading only plays a positive mediating role in the impact of NKEFAs on CS.
3.5. Heterogeneity analysis
The ecological co-benefits of NKEFAs vary according to ecological foundation, endowment conditions, geographical location and other factors, and it is necessary to understand them through heterogeneity analysis. Heterogeneity analysis is carried out from the following perspectives: different ecological function types, different geospatial locations, different quantiles of CS and EQ.
3.5.1. Differences in ecological function types
According to the differences in ecological product supply and functional positioning, NKEFAs can be classified into four types: water conservation, soil conservation, windbreak sand fixation, and biodiversity maintenance (Table 5). In summary, the NKEFAs of water conservation, soil conservation and biodiversity maintenance achieve co-benefits of CS and EQ. In contrast, the windbreak sand-fixation type only has the strongest enhancement effect on CS, followed by the soil conservation type. The soil conservation type showed the greatest improvement effect on EQ, followed by the water conservation type.
3.5.2. Differences in geospatial locations
According to the different geographical locations, the country is divided into six geographic regions, i.e., Northeast China (NEC), North China (NC), Northwest China (NWC), Southwest China (SWC), Middle and Lower Reaches of the Yangtze River regions (MLY), and Southeast Coastal regions (SEC). Among them, NEC, NC, and NWC belong to the North, while the SWC, MLY, and SEC are part of the South (Table 6).
Among the NKEFAs in the six geographic regions, the NKEFAs in SWC, MLY, and SEC of the South achieve co-benefits of CS and EQ, while NEC, NC, and NWC of the North only significantly enhance the CS. In comparison, NKEFAs represent the strongest enhancement effect on CS in NWC, followed by NEC; the NKEFAs show the strongest promotion effect on EQ in SWC and SEC, followed by MLY. Taken together, the enhancing effect of NKEFAs on CS is stronger in the North, while that on EQ is more obvious in the South.
3.5.3. Differences in quantiles of CS and EQ
There are differences in the degree of policy dependence and response among regions with different endowment conditions. The heterogeneity distribution of CS and EQ of NKEFAs is further examined by a panel quantile regression model (Table 7).
The NKEFAs show a rising and then declining positive effect on CS with the increasing quantile, and represent an overall growing trend. The highest positive effect appears near the 75% quantile, in areas with good ecological endowment base, the positive enhancement effect of NKEFAs on CS is higher. Regions with excellent ecological endowment have a larger scale of CS and can better absorb and store CO2. The NKEFAs promote the optimization of national land space, limit large-scale and high-intensity urbanization and industrialization, which help to release more potential of carbon sink.
The effect of NKEFAs on EQ shows a “positive → negative → positive” U-shaped trend with the increasing quantile, with an obvious negative effect near the 25% quantile and a gradual change to a positive effect after the 40% quantile. This identifies that the NKEFAs do not tremendously improve the EQ in areas with relatively poor ecological endowment and high ecological vulnerability, consistent with the results of the windbreak sand-fixation area and NWC. Additionally, NKEFAs can better improve the EQ in areas with excellent eco-environment.
4. Discussions
4.1. Ecological co-benefits of NEKFAs and its mechanism
As a command-and-control ecological policy (Blackman et al., 2018), the distribution of NKEFAs forms an important ecological barrier of China. DID analysis can effectively capture the co-benefits of ecological product supply and the eco-environment quality of NKEFAs, consistent with Pan and Tang (2020). The coefficient of CS turned significantly positive in 2009. We found in 2009, China has begun to pilot ecological transfer payments in some important ecological regions to enhance environmental investment, relevant government departments responded in advance following the policy guidance. That is, the NKEFAs can produce an expected effect on CS and EQ (Du et al., 2020). Moreover, the improvement of CS is superior to that of EQ, and thus the environment quality improvement still has a long way to go, this is similar to Liu et al. (2022). In general, the co-benefit enhancement of CS and EQ is a long-term process that evolves gradually. The ecological effect of transfer payment and policy rollout to NKEFAs is relatively weak in the short term, but gradually increase in the long term. Through providing continuous vertical ecological compensation to NKEFAs, the co-benefits can be effectively promoted in a sustainable manner (Zhu and Chen, 2021).
The NKEFAs can achieve co-benefits of CS and EQ by optimizing the territory spatial pattern and promoting the labor force transformation. The expansion of ecological space is the primary means of NKEFA establishment, which inevitably require urbanization and industrial development to match the carrying capacity of resources and the environment (Huang et al., 2021). It also provides carriers and opportunities for industrial aggregation and labor mobility. However, restrictive development and industrial upgrading make it challenging for ecological functional areas to carry a larger labor force. Although the overloaded population has transferred in an orderly manner, the active population migration policy can increase population aggregation and absorption capacity, and the development of ecological industries can also attract the return of outflowing labor. The NKEFAs encourage industries development to be compatible with the positioning of the main functional areas. Industrial upgrading enhances the ecological product supply capacity but minimally improves EQ in the short term.
In summary, the research findings make theoretical and methodological contributions to the scientific assessment of policy effect on ecological engineering. This article focuses on the ecological goals achievement of NKEFAs, and provides a complete framework for assessing the implementation effect of ecological engineering by integrating the co-benefits of ecological products supply and environment quality improvement. The assessment framework and policy insights can provide methodological reference and application value for ecological engineering in other countries. However, it should be noted that the selected indicators of policy assessment depend on the specific ecological engineering and socio-economic development reality in China. Once this framework is extended to other countries or regions, an appropriate assessment framework that fits the ecological conditions needs to be constructed according to the special operating dynamics of ecological engineering in this country or region.
4.2. Localization and precision in the management of NKEFAs
NKEFAs undertake important ecological functions, such as water and soil conservation, windbreak sand fixation, and biodiversity maintenance (Pan et al., 2021). The difference in the leading ecological function determines the direction of development and protection of each NKEFA (Zuo and Gao, 2021). The water conservation type can realize precipitation retention, runoff regulation, soil desertification control and vegetation restoration. The soil conservation type has the function of avoiding soil erosion through watershed management, restriction of resource development, returning farmland to forest and grass, etc. The biodiversity maintenance type has the function of maintaining and restoring the balance of wildlife species, preventing habitat changes due to ecological construction. All three types of NKEFAs can achieve co-benefits of CS and EQ, and similar to Liu et al. (2020), the soil conservation type has the greatest improvement effect. In contrast, the windbreak sand-fixation type only positively contributes to CS, probably because deserts, as an important part of terrestrial ecosystems, can sequester large amounts of carbon dioxide and play a key role as carbon sinks (Yang et al., 2020). However, the windbreak sand-fixation type is generally located in fragile ecosystems with high desertification and severe grassland degradation, with limited environmental benefits and still has a long way to go.
NWC presents a more consistent performance of the windbreak sand-fixation type. The functions of different types in the NWC are intertwined, which can improve the capacity of CS. Nevertheless, most areas of NWC show arid and semi-arid climate characteristics, with a poorer ecological endowment base and more prominent environmental problem. In contrast, areas with better ecological conditions are mainly located near the Qinling Mountains in Shaanxi. Differently, NC is a traditional agricultural production area, where the contradiction between population, economy, and environmental carrying capacity induces prominent haze problems. Although air quality has gradually improved in recent years, improving EQ is an evolutionary process that needs sustained effort in the long term.
The performance of different ecological function areas and geospatial locations conform to the quantile heterogeneity law of the ecological environment. Areas with richer ecological foundation and environmental conditions are the key areas restricted and prohibited from development. NKEFAs with higher environmental carrying capacity can provide more ecological goods and services, then improve ecological co-benefits. In contrast, it is difficult for NEKFAs with poorer ecological endowment base to improve EQ greatly in a short time, and the improvement of environmental benefits is a lasting and stable process. In short, there is a need for differentiation and precision in the institutional arrangement of NKEFAs (Gao et al., 2021).
5. Conclusion
This study considers the NKEFAs as quasi-natural experiment, a time-varying DID model is used to assess the impact of NKEFAs on CS and EQ. In conclusion, the NKEFAs enhance both CS and EQ and achieve the ecological co-benefits. Compared to those of non-NKEFAs, the CS and EQ of NKEFAs are elevated by 2.1625 and 0.0031 in ATE, respectively. The NKEFAs can enhance CS and EQ through the positive mediating role of territory spatial allocation and labor force aggregation. Nevertheless, the NKEFAs only enhance CS by industrial structure upgrading. The policy effects of NKEFAs are heterogeneous across ecological function types, geospatial locations, and quantiles. Except for the NKEFAs of windbreak sand-fixation type, northwestern region and low quantiles where only promote CS, all other function areas can achieve the ecological co-benefits.
The conclusions have abundant policy implications: (1) Improving the sustainability and stability of NKEFAs. While the establishment of NKEFAs has achieved ecological co-benefits currently, effective sustainability incentives depend on local government action in ecological governance and protection. In order to form a long-term positive incentive, avoid the ecosystem rebound in NKEFAs, a mechanism of continuous support and supervision is necessary nation widely. (2) Implementing a more dynamic and efficient industrial policy. The covered areas can actively develop eco-agriculture and service industries. More policies about the active population migration and household registration management should be introduced to attract transferred labor to new industries. By doing this, market-oriented and cross-regional mobility of labor and other factors will be promoted. (3) Building a diversified and precise ecological governance and compensation system. Due to the existence of heterogeneity, the institutional arrangement of NKEFAs should be more adapted to local conditions and more precise. The NKEFAs need to focus on areas with lower ecological endowment base to maintain synergy between land utilization and eco-environment. For example, the northwestern region in China mainly belongs to the ecological functional area of windbreak sand fixation, the transfer funds of the central government need to be tilted more toward these areas. In this way, ecological governance and service functions are expected to be strengthened.
Data availability statement
The original contributions presented in the study are included in the article/supplementary material, further inquiries can be directed to the corresponding author.
Author contributions
HC: methodology, data curation, and writing—original draft. MH: conceptualization, writing—review and editing, and software. ZX: writing—review and editing, data curation, and validation. XZ: data curation, software, and visualization. SY: conceptualization, writing—review and editing, investigation, and supervision. All authors contributed to the article and approved the submitted version.
Funding
This research was funded by the Social Science Foundation of Hebei Province (no. HB22YJ053); Scientific Research Initiation Project for High-level Talents of Hebei University (no. 521100222017); National Social Science Foundation of China (no. 17BTJ029); and National Natural Science Foundation of China (no. 71773091).
Acknowledgments
The valuable comments and suggestions of editors and reviewers are gratefully acknowledged.
Conflict of interest
The authors declare that the research was conducted in the absence of any commercial or financial relationships that could be construed as a potential conflict of interest.
Publisher’s note
All claims expressed in this article are solely those of the authors and do not necessarily represent those of their affiliated organizations, or those of the publisher, the editors and the reviewers. Any product that may be evaluated in this article, or claim that may be made by its manufacturer, is not guaranteed or endorsed by the publisher.
Footnotes
References
Aaron, D., Melanie, S., Liam, B., Michael, B., Jeffery, R., Michael, J., et al. (2021). Monthly global estimates of fine particulate matter and their uncertainty. Environ. Sci. Technol. 55, 15287–15300. doi: 10.1021/acs.est.1c05309
Ball, B., Kominoski, J., Adams, H., Jones, S., Kane, E., Loecke, T., et al. (2010). Direct and terrestrial vegetation-mediated effects of environmental change on aquatic ecosystem processes. Bioscience 60, 590–601. doi: 10.1525/bio.2010.60.8.5
Baron, R., and Kenny, D. (1986). The moderator-mediator variable distinction in social psychological research: conceptual, strategic, and statistical considerations. J. Pers. Soc. Psychol. 51, 1173–1182. doi: 10.1037//0022-3514.51.6.1173
Blackman, A., Li, Z., and Liu, A. (2018). Efficacy of command-and-control and market-based environmental regulation in developing countries. Annual Review of Resource Economics. 10, 381–404. doi: 10.1146/annurev-resource-100517-023144
Bryan, B. A., Gao, L., Ye, Y., Sun, X., Connor, J. D., Crossman, N. D., et al. (2018). China’s response to a national land-system sustainability emergency. Nature 559, 193–204. doi: 10.1038/s41586-018-0280-2
Chan, R., He, H., Chan, H., and Wang, W. (2012). Environmental orientation and corporate performance: the mediation mechanism of green supply chain management and moderating effect of competitive intensity. Ind. Mark. Manag. 41, 621–630. doi: 10.1016/j.indmarman.2012.04.009
Chen, S. (2017). The effect of a fiscal squeeze on tax enforcement: evidence from a natural experiment in China. J. Public Econ. 147, 62–76. doi: 10.1016/j.jpubeco.2017.01.001
Chen, J., Fan, W., Li, D., Liu, D., and Song, M. (2020). Driving factors of global carbon footprint pressure: based on vegetation carbon sequestration. Appl. Energy 267:114914. doi: 10.1016/j.apenergy.2020.114914
Cisilino, F., Bodini, A., and Zanoli, A. (2019). Rural development programs’ impact on environment: an ex-post evaluation of organic farming. Land Use Policy 85, 454–462. doi: 10.1016/j.landusepol.2019.04.016
DeFries, R., Hansen, A., Turner, B., Reid, R., and Liu, J. (2007). Land use change around protected areas: management to balance human needs and ecological function. Ecol. Appl. 17, 1031–1038. doi: 10.1890/05-1111
Dong, Z., Xia, C., Fang, K., and Zhang, W. (2022). Effect of the carbon emissions trading policy on the co-benefits of carbon emissions reduction and air pollution control. Energy Policy 165:112998. doi: 10.1016/j.enpol.2022.112998
Du, J., Liu, H., and Wu, H. (2020). Impact of environmental supervision system on enterprises’ investment in environmental protection. China Population, Resources and Environment. 30, 151–159. doi: 10.12062/cpre.20200423
Fan, J. (2016). Theoretical innovation in optimization of protection and development of China’s territorial space and coping strategy of 13th five-year plan. Bull. Chin. Acad. Sci. 31, 1–12. doi: 10.16418/j.issn.1000-3045.2016.01.001
Fu, R., and Miao, X. (2015). A new financial transfer payment system in ecological function areas in China: based on the spillover ecological value measured by the expansion energy analysis. Econ. Res. J. 50, 47–61.
Gao, Y., He, N., and Wang, Y. (2013). Characteristics of carbon sequestration by ecosystem and progress in its research. J. Nat. Resour. 28, 1264–1274. doi: 10.11849/zrzyxb.2013.07.018
Gao, X., Liu, N., and Hua, Y. (2022). Environmental protection tax law on the synergy of pollution reduction and carbon reduction in China: evidence from a panel data of 107 cities. Sustainable Prod. Consumption 33, 425–437. doi: 10.1016/j.spc.2022.07.006
Guo, S., Pei, Y., and Wu, Y. (2020). Research on industrial structure adjustment and upgrading effect of the development of productive service industry. J. Quant. Technol. Econ. 37, 45–62. doi: 10.13653/j.cnki.jqte.2020.10.003
Gao, J., Zuo, L., and Liu, W. (2021). Environmental determinants impacting the spatial heterogeneity of karst ecosystem services in Southwest China. Land Degradation & Development. 32, 1718–1731. doi: 10.1002/ldr.3815
He, Q., Chen, Z., Peng, X., Liu, Y., and Zhang, M. (2018). An assessment of forest biomass carbon storage and ecological compensation based on surface area: a case study of Hubei province, China. Ecological Indicators. 90, 392–400. doi: 10.1016/j.ecolind.2018.03.030
Hou, P., Zhai, J., Cao, W., Yang, M., Cai, M., and Li, J. (2018). Evaluation on ecosystem changes and protection of the national key ecological function zones in mountainous areas of Central Hainan Island. Acta Geograph. Sin. 73, 429–441. doi: 10.11821/dlxb201803004
Huang, X., Chen, Y., Zhao, Y., Shi, M., and Li, T. (2021). Optimization on land spatial development pattern in the Yellow River Basin: from the perspective of land development intensity. Geogr. Res. 40, 1554–1564. doi: 10.11821/dlyj020200546
Jiang, H., and Chen, L. (2021). Spatial allocation efficiency and control strategy of county land resources based on main functional areas of territorial space: a case study of Ganyu, Jiangsu province. J. Nat. Resour. 36, 2424–2436. doi: 10.31497/zrzyxb.20210918
Li, G., and Li, X. (2014). Allocation mechanism of national key ecological function areas transfer payment. China Popul. Resour. Environ. 24, 124–130. doi: 10.3969/j.issn.1002-2104.2014.05.019
Li, G., Li, X., and Wang, H. (2013). Ecological compensation effect of national key ecological function areas transfers payment policy. Modern Econ. Sci. 35, 58–64.
Li, G., Wang, H., and Liu, Q. (2014). The dual objectives and performance evaluation of transfer payment in national key ecological function areas. J. Northwest Univ. (Philos. Social Sci. Ed.) 44, 151–155. doi: 10.16152/j.cnki.xdxbsk.2014.01.032
Li, G., Yang, L., and Liu, S. (2016). Study on spatial spillover effects of ecological environment quality at the county level in national key ecological function areas. J. China Univ. Geosci. (Social Sci. Ed.). 16, 10–19. doi: 10.16493/j.cnki.42-1627/c.2016.01.002
Lin, G., Jiang, D., Fu, J., Cao, C., and Zhang, D. (2020). Spatial conflict of production–living–ecological space and sustainable-development scenario simulation in Yangtze River Delta agglomerations. Sustainability. 12:2175. doi: 10.3390/su12062175
Lin, S., and Qi, Y. (2021). The incentive effect and strategy choice of location-oriented ecological environmental policy. Public Finance Res. 06, 85–103. doi: 10.19477/j.cnki.11-1077/f.2021.06.005
Liu, L., Cao, W., Wu, D., and Huang, L. (2018). Temporal and spatial variations of ecosystem services in national key ecological function zones. Sci. Geogr. Sin. 38, 1508–1515. doi: 10.13249/j.cnki.sgs.2018.09.014
Liu, H., Gao, J., Liu, X., Zhang, H., and Xu, X. (2020). Monitoring and assessment of the ecosystem services value in the national key ecological function zones. Acta Ecol. Sin. 40, 1865–1876. doi: 10.5846/stxb201903010382
Liu, Z., and Lin, Y. (2020). Structural transformation, TFP and high-quality development. Manage. World 36, 15–29. doi: 10.19744/j.cnki.11-1235/f.2020.0100
Liu, W., Liu, J., Kuang, W., and Ning, J. (2017). Examining the influence of the implementation of major function-oriented zones on built-up area expansion in China. J. Geogr. Sci. 27, 643–660. doi: 10.1007/s11442-017-1398-0
Liu, J., Liu, Y., and Li, Y. (2017). Classification evaluation and spatial-temporal analysis of “production-living-ecological” spaces in China. Acta Geograph. Sin. 72, 1290–1304. doi: 10.11821/dlxb201707013
Liu, B., and Song, M. (2022). Basic framework and value realization of carbon sink ecological products. Nat. Resour. Econ. China 35, 4–11. doi: 10.19676/j.cnki.1672-6995.000744
Liu, H., Wang, C., Zhang, M., and Wang, S. (2022). Evaluating the effects of air pollution control policies in China using a difference-in-differences approach. Sci. Total Environ. 845:157333. doi: 10.1016/j.scitotenv.2022.157333
Lu, S., Dong, R., and Ye, C. (2021). Does “the belt and road initiative” promote high-quality exports—evidence from firms in China. China Ind. Econ. 03, 80–98. doi: 10.19581/j.cnki.ciejournal.2021.03.012
Luo, Z., and Qi, B. (2021). The effects of environmental regulation on industrial transfer and upgrading and banking synergetic development—evidence from water pollution control in Yangtze River basin. Econ. Res. J. 56, 174–189.
McGavock, T. (2021). Here waits the bride? The effect of Ethiopia’s child marriage law. J. Dev. Econ. 149:102580. doi: 10.1016/j.jdeveco.2020.102580
Miao, X., and Zhao, Y. (2019). Impact of transfer payment in eco-functional areas on eco-environmental improvement: capital compensation or institutional incentives? Public Finance Res. 05, 17–32. doi: 10.19477/j.cnki.11-1077/f.2019.05.003
Pan, D. (2021). The impact of command-and-control and market-based environmental regulations on afforestation area: quasi-natural experimental evidence from county data in China. Resour. Sci. 43, 2026–2041. doi: 10.18402/resci.2021.10.0
Pan, D., and Tang, J. (2020). The effects of heterogeneous environmental regulations on water pollution control: quasi-natural experimental evidence from China. Sci. Total Environ. 751:141550. doi: 10.1016/j.scitotenv.2020.141550
Pan, D., and Tang, J. (2021). The effects of heterogeneous environmental regulations on water pollution control: Quasi-natural experimental evidence from China. Science of The Total Environment. 751:141550. doi: 10.1016/j.scitotenv.2020.141550
Rosenthal, S., and Strange, W. (2003). Geography, industrial organization, and agglomeration. Rev. Econ. Stat. 85, 377–393. doi: 10.1162/003465303765299882
Shi, D., Ding, H., Wei, P., and Liu, J. (2018). Can smart city construction reduce environmental pollution? China Ind. Econ. 06, 117–135. doi: 10.19581/j.cnki.ciejournal.2018.06.008
Tang, K., Liu, Y., Zhou, D., and Qiu, Y. (2021a). Urban carbon emission intensity under emission trading system in a developing economy: evidence from 273 Chinese cities. Environ. Sci. Pollut. Res. 28, 5168–5179. doi: 10.1007/s11356-020-10785-1
Tang, K., Qiu, Y., and Zhou, D. (2020). Does command-and-control regulation promote green innovation performance? Evidence from China’s industrial enterprises. Sci. Total Environ. 712:136362. doi: 10.1016/j.scitotenv.2019.136362
Tang, K., Zhou, Y., Liang, X., and Zhou, D. (2021b). The effectiveness and heterogeneity of carbon emissions trading scheme in China. Environ. Sci. Pollut. Res. 28, 17306–17318. doi: 10.1007/s11356-020-12182-0
Tao, F., Zhao, J., and Zhou, H. (2021). Does environmental regulation improve the quantity and quality of green innovation----evidence from the target responsibility system of environmental protection. China Ind. Econ. 02, 136–154. doi: 10.19581/j.cnki.ciejournal.2021.02.016
UNFCCC. (1997). Kyoto protocol to the United Nations framework convention on climate change. Kyoto Climate Change Conference.
Wang, X., and Gao, Y. (2018). The road to rural revitalization in poor counties in national key ecological function areas. PRO 04, 94–100. doi: 10.16501/j.cnki.50-1019/d.2018.04.012
Wang, F., and Ge, X. (2022). Can low-carbon transition impact employment: Empirical evidence from Low-carbon city pilot policy. China Industrial Economics 05, 81–99. doi: 10.19581/j.cnki.ciejournal.2022.05.004
Wang, D., and Tang, M. (2019). How does land urbanization affect ecological environment quality? Analysis based on dynamic optimization and spatially adaptive semi-parametric model. Econ. Res. J. 54, 72–85.
Wu, Y., Qi, J., Xian, Q., and Chen, J. (2021). The carbon emission reduction effect of China’s carbon market-from the perspective of the coordination between market mechanism and administrative intervention. China Ind. Econ. 08, 114–132. doi: 10.19581/j.cnki.ciejournal.2021.08.007
Xu, J., Xie, G., Xiao, Y., Li, N., Jiang, Y., Chen, W., et al. (2019). Dynamic analysis of ecological environmental quality changes in national key ecological function areas in China. Acta Ecol. Sin. 39, 3039–3050. doi: 10.5846/stxb201806011227
Xu, J., Xie, G., Xiao, Y., Li, N., Yu, F., Pei, S., et al. (2018). Dynamic analysis of ecological environment quality combined with water conservation changes in National key Ecological Function Areas in China. Sustainability. 10:1202. doi: 10.3390/su10041202
Xu, D., Yang, F., Yu, L., Zhou, Y., Li, H., Ma, J., et al. (2021). Quantization of the coupling mechanism between eco-environmental quality and urbanization from multisource remote sensing data. J. Clean. Prod. 321:128948. doi: 10.1016/j.jclepro.2021.128948
Xu, H., and Zhang, W. (2017). Study on the ecological protection effect of the transfer payment of national key ecological function areas: an empirical study based on Shaanxi data. China Popul. Resour. Environ. 27, 141–148. doi: 10.12062/cpre.20170618
Yang, F., Huang, J., He, Q., Zheng, X., Zhou, C., Pan, H., et al. (2020). Impact of differences in soil temperature on the desert carbon sink. Geoderma 379:114636. doi: 10.1016/j.geoderma.2020.114636
Yu, B. (2015). Economic growth effects of industrial restructuring and productivity improvement-analysis of dynamic spatial panel model with Chinese city data. China Ind. Econ. 12, 83–98. doi: 10.19581/j.cnki.ciejournal.2015.12.007
Yu, Y., and Zhang, N. (2021). Low-carbon city pilot and carbon emission efficiency: Quasi-experimental evidence from China. Energy Economics. 96:105125. doi: 10.1016/j.eneco.2021.105125
Zuo, L., and Gao, J. (2021). Investigating the compounding effects of environmental factors on ecosystem services relationships for Ecological Conservation Red Line areas. Land Degradation & Development. 32, 4609–4623. doi: 10.1002/ldr.4059
Zhang, T., Hou, M., Chu, L., and Wang, L. (2022). Can the establishment of National key Ecological Function Areas enhance vegetation carbon sink? Quasi-natural experiment evidence from China. Int. J. Environ. Res. Public Health 19:12215. doi: 10.3390/ijerph191912215
Zhang, W., and Li, G. (2015). Dynamic incentive effect analysis of transfer payment in national key ecological function zone. China Popul. Resour. Environ. 25, 125–131. doi: 10.3969/j.issn.1002-2104.2015.10.017
Zhang, M., Zhang, L., He, H., Ren, X., Lv, Y., Niu, Z., et al. (2022). Improvement of ecosystem quality in National key Ecological Function Zones in China during 2000–2015. J. Environ. Manag. 324:116406. doi: 10.1016/j.jenvman.2022.116406
Zhou, X. Y., Lei, K., Meng, W., and Khu, S. T. (2017). Industrial structural upgrading and spatial optimization based on water environment carrying capacity. Journal of Cleaner Production. 165, 1462–1472. doi: 10.1016/j.jclepro.2017.07.246
Zhu, Y., and Chen, H. (2020). Did the transfer payment in key eco-functional areas improve the eco-environment? Based on PSM. South China J. Econ. 10, 125–140. doi: 10.19592/j.cnki.scje.371556
Keywords: National Key Ecological Function Areas, carbon sequestration, environmental quality, co-benefits, time-varying difference-in-differences, China
Citation: Chen H, Hou M, Xi Z, Zhang X and Yao S (2023) Co-benefits of the National Key Ecological Function Areas in China for carbon sequestration and environmental quality. Front. Ecol. Evol. 11:1093135. doi: 10.3389/fevo.2023.1093135
Edited by:
Cuicui Jiao, Sichuan University of Science and Engineering, ChinaReviewed by:
Mengmeng Gou, Chinese Academy of Forestry, ChinaLi Lipeng, Ningxia University, China
Haimeng Liu, Chinese Academy of Sciences (CAS), China
Copyright © 2023 Chen, Hou, Xi, Zhang and Yao. This is an open-access article distributed under the terms of the Creative Commons Attribution License (CC BY). The use, distribution or reproduction in other forums is permitted, provided the original author(s) and the copyright owner(s) are credited and that the original publication in this journal is cited, in accordance with accepted academic practice. No use, distribution or reproduction is permitted which does not comply with these terms.
*Correspondence: Mengyang Hou, ✉ aG91bWVuZ3lhbmdAaGJ1LmVkdS5jbg==