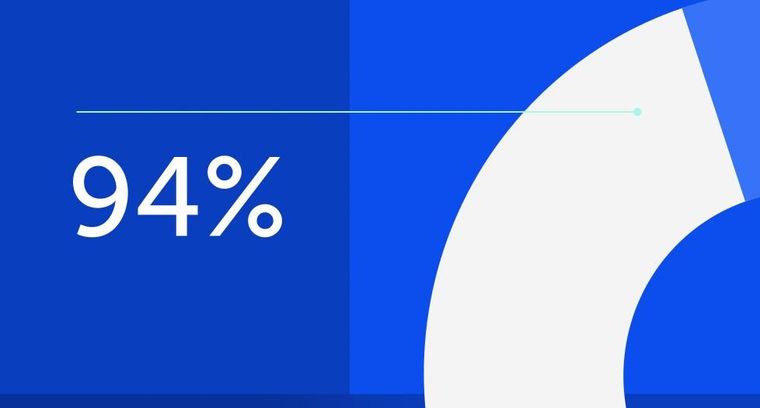
94% of researchers rate our articles as excellent or good
Learn more about the work of our research integrity team to safeguard the quality of each article we publish.
Find out more
ORIGINAL RESEARCH article
Front. Ecol. Evol., 25 July 2023
Sec. Conservation and Restoration Ecology
Volume 11 - 2023 | https://doi.org/10.3389/fevo.2023.1085272
This article is part of the Research TopicAdvances in the Conservation of Large Terrestrial MammalsView all 10 articles
The reduction and fragmentation of forests due to human activities are two primary factors that have led to forest biodiversity losses. The lack of forest continuation may prevent organisms from escaping areas that are no longer habitable due to altered environmental conditions and the reduction in size of habitat regions. Therefore, protecting and promoting forest connectivity has become one of the important objectives of forest management. The forests were regarded as independent elements, and the connectivity of the forest itself was directly evaluated in previous studies. However, this approach ignores the maintenance of forest connectivity, while requiring the participation of other landscape elements. In this study, we indirectly determine the forest priority by evaluating the landscape priority by integrating analysis with the habitat suitability (HS) model, MSPA and and and index. We studied the wild boar (Sus scrofa) habitats in and around Hupingshan and Houhe National Nature Reserves to illustrate the indirect evaluation method of forest priority. The results showed that forests with high priority, medium priority, low priority, and non-priority comprised 596, 64, 58 and 105 km2, respectively, accounting for the 41.2, 2.7, 3.0, and 11.9% of the total forest area. Our research revealed that evaluating the forest priority by analysing the landscape priority was an effective method for forest priority identification, and this strategy can be used to other regions or species for the goal of identifying the forest priority for biodiversity conservation.
The forest is the dominant component of the terrestrial ecosystem and plays a crucial role in the conservation and management of biodiversity. Reduced and fragmented forest habitats (caused by urban sprawl, forest logging, intensified agriculture, etc.) are widely regarded as the greatest hazards to biodiversity (Lõhmus et al., 2017). The lack of forest continuity may impede the ability of organisms to leave areas that are no longer habitable due to altered environmental conditions and the reduction in size of habitat regions (Campbell Grant et al., 2007; Baranyi et al., 2011). Connectivity enables dispersal and gene flow, both of which are crucial for preventing population reduction and extinction (Foltête et al., 2020). In this context, preserving and promoting forest connectivity has become one of the most important objectives of forest management, as suitable forest landscape connectivity facilitates the movement of individual animals between forest regions and ensures genetic exchange between populations (Martin et al., 2017; Velázquez et al., 2017).
Landscape connectivity is defined as “the extent to which the landscape facilitates or impedes species movement across habitat patches and for a particular organism” and is influenced by both the landscape and the species in question (Baranyi et al., 2011). The network method combined with landscape connectivity index is an effective way to identify the key elements (patches, corridors, and stepping stones) of landscape (Gil-Tena et al., 2013; Saura et al., 2018). The network technique proposes that landscapes can be represented by a graph with nodes representing habitat patches and connections reflecting the dispersal probabilities of a species to travel between patches. Numerous indicators have been devised to reflect the landscape connectivity with a focus on either the elements or the entire landscape, but probability of connectivity (PC) is the most widely used. The importance of each patch or link determined by how much the PC metric decreased when it was removed (Baranyi et al., 2011; Cabarga-Varona et al., 2016; Hernando et al., 2017). This approach implies that organisms that formerly moved through a specific element are able to find alternative dispersal pathways and that there is no competition among dispersers for the use of the fewer remnant pathways in the disturbed landscape. To overcome the limitations of remove experiments, the metric integrated the benefits of remove experiments and centrality, which gives greater weight to the paths that are anticipated to transport larger flows of organisms and that connect bigger and thus likely more ecologically significant patches (Bodin and Saura, 2010).
Land cover and vegetation type, patch area, and crown closure are three popular markers for designating nodes in a forest landscape network, and the connectivity between forest patches has been explicitly investigated in prior studies (Saura et al., 2011; Velázquez et al., 2019; Foltête et al., 2020). However, this network approach regards forests as independent elements and ignores the maintenance of forest connectivity, while requiring the participation of other landscape elements. This method only makes sense if the only location where the species lives is in a forest. However, species often affect the suitability of a habitat is, and it is a lot more complicated than just describing it in terms of plants or land cover (Avon and Bergès, 2016). For instance, not all forests identified on a land cover map may be suitable for certain animals (Avon and Bergès, 2016; Duflot et al., 2018). The network findings are only useful if the node and link properties are biologically important for the species under consideration (Urban et al., 2009).
In this study, we indirectly determine the priority of the forest through determining the priority levels of the landscape. To accomplish this, we initially evaluated the suitable area based on the habitat demand of the specific species by the habitat suitability (HS) model. The prediction accuracy of HS outputs was evaluated by the infrared monitoring data of the species. Furthermore, we developed the habitat networks and applied the landscape connectivity index ( and ) to assess the priority patches and corridors, respectively, and the priority of forest is consistent with the priority of the landscape area where it belongs to.
We studied the wild boar (Sus scrofa) habitats in Hupingshan–Houhe National Nature Reserves (Hupingshan–Houhe NNR) and three adjacent counties around them to illustrate the indirect evaluation method of forest priority. Hupingshan–Houhe NNR reconsidered as the most appropriate places to harbor a small population of the South China tiger (Panthera tigris amoyensis) individuals (Qin et al., 2015; Shurong et al., 2022). It has been claimed that population density, ungulate prey size and biomass are typically crucial to the survival and reproduction of wild tiger populations (Sunquist, 1981; Karanth and Sunquist, 1995; Miquelle et al., 1996; Burge, 1999; Karanth and Sunquist, 2000; Karanth et al., 2004; Hebblewhite et al., 2011; Hebblewhite et al., 2014; Qin et al., 2015; Kafley et al., 2016). Main tiger prey includes forest and grassland ungulates, ranging in size from tiny deer such as barking deer (Muntiacus muntjak) and wild boar to huge animals such as water buffalo (Bubalus bubalis) (Karanth et al., 2004; Smith et al., 2008; Karanth and Nichols, 2010; Xie and Smith, 2013; Froese et al., 2017). The infrared camera monitoring survey in Hupingshan NNR reveals that wild boars are one of the main prey that satisfy the demands of South China tigers (Shurong et al., 2020). As the major habitat of the tiger prey, the forest, which comprises more than 50 percent of the total area of the study area, plays a critical role in maintaining its population (Tang et al., 2023). Therefore, identifying the priority of forests at different locations in the study area for wild boar is of significance for maintaining the number of wild boar populations, which have a positive promoting effect to the survival and reproduction of South China tigers.
Hupingshan–Houhe NNR (110° 29′–110° 59′ E, 29° 50′–30°09′ N; Figure 1) consists of two adjacent regions, Hupingshan NNR is located on the northern border of the Hunan Province and Houhe NNR on the southern border of the Hubei Province. Located in the transition region between the Guizhou Plateau and hilly zones in southeast China, Hupingshan–Houhe NNR is topographically heterogeneous, including steep and deep ravines, narrow valleys, and high mountains. The terrain includes deep ravines, narrow valleys, and high altitudes, with the highest altitude at 2,299 m (Shu-Rong et al., 2019). The region has a subtropical mountain climate that is significantly impacted by the North Pacific warm current, resulting in hot and rainy summers and cold and snowy winters. The annual mean temperature is 9.2°C, and the annual mean precipitation is 1900 mm (Shurong et al., 2020). The study area consists of two NNRs and three adjacent counties: Shimen County in Hunan Province, Hefeng County, and the Wufeng Tujia Autonomous County in the Hubei Province. The overall area of these regions is approximately 12300 km2, and forests with canopy > 30% and shrubs with canopy > 40% account for approximately 54% of the total area. The area includes six typical soil types, among which yellow brown soil is the most widely distributed (Wei et al., 2020).
One hundred and twenty infrared cameras were set up in Hupingshan NNR to obtain the distribution data of wild boars (Figure 1). Infrared cameras are located in each 1km × 1km grid generated by ArcGIS, and a total of 120 infrared cameras are deployed (Yu et al., 2018). From October 2020 to October 2021, there were 579 independent and effective wild boar photos distributed in seventy-two infrared camera points were captured (Yu et al., 2018).
A diagrammatic summary of the analytical method is shown in Figure 2. Based on the work of Qin et al. (2015) and Tang et al. (2021), a habitat suitability (HS) model has been developed to identify the suitability of the study area. The HS model was computed using the geometric mean of six suitability indices (SIs) as described:
where LC, S, E, DTF, DTW, and DTD refer to landscape cover, slope, elevation, distance to the forest edge, distance to water bodies, and distance to disturbance, respectively. To account for uncertainty in parameterizations of habitat requirements (Bennett et al., 2009; Battisti et al., 2016), the SI habitat suitability was parameterized under three different scenarios (high: H, medium: M, and low: L) for the LC, S, E, DTF, and DTW indices. Only one scenario was established to parameterize the DTD. Habitat selection by wild boar seasonally varies, and particularly with elevation changes. Thus, summer (S) and winter (W) scenarios were constructed with different elevation parameterizations to accommodate such potential variability across seasons. Six HS scenarios were developed, including summer high SH, summer medium SM, summer low SL, winter high WH, winter medium WM, and winter low WL conditions. Then, the seventy-two infrared camera positions where wild boars appeared were utilized to evaluate the prediction accuracy of the six HS outputs. This scenario will be kept if the forecast accuracy is greater than 85%; otherwise, it will be removed. The patches with an HS > 6 and area >1 km2 were initially considered to be suitable habitats patches for wild boar, while others were considered unsuitable.
Then, morphological spatial pattern analysis (MSPA) (Soille and Vogt, 2009; https://forest.jrc.ec.europa.eu/en/activities/lpa/gtb/) was used to select the source patches from the suitable habitats. MSPA can divide the binary map, yielding seven classes including core, islet, edge, bridge, perforation, loop, and branch areas. In this study, the patch containing the core is considered as the source patch, which have more robust biological structures than sections lacking core regions (Tang et al., 2023). Using edge width (EW) parameters, the MSPA segmentation method can be fine-tuned. The EW was set to nineteen pixels, which corresponded to a Euclidean distance of 570 meters. The EW was equivalent to a 1 km2 circle with an optimal radius for wild boar habitat.
The effective distances were calculated using the cumulative costs along the path with the lowest cost, disregarding the distances between sections. To convert the suitability of habitats into resistance value, the HS results were classified into seven classes, with class 1: 0 ≤ HS ≤ 1; class 2: 1< HS ≤ 2; class 3: 2< HS ≤ 3; class 4: 3< HS ≤ 4; class 5: 4< HS ≤ 5; class 6: 5< HS ≤ 6; and class 7: 6< HS ≤ 10. The HS values were inverted such that appropriate sites for wild boar (i.e., areas with higher HSs) had lower mobility resistance, and vice a versa (Duflot et al., 2018). Using a negative exponential calculation (Préau et al., 2020; Tang et al., 2023), the resistance values for classes 1, 2, 3, 4, 5, 6, and 7 were calculated to be 373, 139, 52, 19, 7, 3, and 1, respectively. A decreasing exponential function was used to incorporate LCPs into the PC index (Saura and Pascual-Hortal, 2007). The cost distance-decay coefficient was also set at 0.05 for the maximum connectivity distance between patches (Urban et al., 2001). The habitat networks were developed in the Graphab v2.4 software (Foltête et al., 2012; http://thema.univ-fcomte.fr/productions/Graphab/).
To avoid the excessive connectivity between patches (Liu et al., 2017), the connectivity distance threshold was identified by exploring the correlation between the overall probability connectivity (PC) and the dispersal distance, in addition to using community analysis (Tang et al., 2021). Patches belonging to the same community are connected by edges, whereas different communities are not connected. In the community analysis, the area accounting for 85% of the total study area was used as the predetermined threshold for the largest community area (Tang et al., 2021).
In this study, we used the to evaluate the importance of nodes, which takes into account patch areas and maximum product probabilities between patches instead of only the number of shortest paths. This metric could assign more weight to the paths that carry larger flows of organisms and that connect bigger and therefore more ecologically important patches for wild boar populations (Bodin and Saura, 2010). As suggested by Saura and Rubio (2010), dPCk was derived from the overall PC index to evaluate the connectivity importance of edges. According to Saura and Torné (2009), the connectivity index was analyzed using the Conefor Sensinode v2.6 program (http://www.conefor.org/).
Patches and corridors were classified by connectivity index from the largest to the smallest, and then divided into three categories including 0–50%, 50.1–90%, and 90.1–100%, that corresponded to three connectivity importance levels (CIL; high H, medium M, and low L) that were assigned coded values of 3, 2, and 1 respectively (Tang et al., 2021). Buffer analysis for corridors was conducted using a Euclidean distance of 570 m that was equal to the edge width used in the MSPA. Since the corridors spatially intersect with patches in each scenario, the maximum CIL value between patches and corridors was taken as the value of overlapped pixels. Then, calculate the arithmetic mean of the landscape priority under the four assessment scenarios as the CIL of landscape, which were separated into four priority levels including high: 2 ≤ CIL ≤ 3, medium: 1 ≤ CIL ≤ 2, low: 0 ≤ CIL ≤ 1, and non: CIL=0. The forest priority was consistent with the priority of the landscape that it belongs to.
The evaluation of six different HS scenarios revealed priority, non-priority habitat ranges and prediction accuracy of the appearance positions of wild boars (Figure 3). Specifically, priority habitat (HS > 6) in summer ranged from 6,911 (SL) to 10,211 km2 (SH) in area, the non-priority habitat (HS ≤ 6) ranged from 2,095 (SH) to 5,395 km2 (SL) in area (Table 1). The priority habitat (HS > 6) in winter ranged from 6,044 (WL) to 10,117 km2 (WH) in area, the non-priority habitat (HS ≤ 6) ranged from 2,189 (SH) to 6,262 km2 (SL) in area (Table 1). The prediction accuracy of the appearance position ranged from 56.9 to 97.2%. Four habitat suitability assessment scenarios with prediction accuracy greater than 85% are shown in Figure 3.
Figure 3 Priority area and non-priority area in the four suitability assessment scenarios with prediction accuracy greater than eighty-five percent.
Table 1 Characteristics of suitable and non-priority areas and prediction accuracy the appearance position of wild boar under six different HSI scenarios.
In conjunction with a community analysis, a connectivity distance threshold of 530–550 cumulative resistance value (CRV) was established based on the correlation between the overall PC index and the dispersal distance. In this study, connectivity distances of 550 CRV were used to establish habitat networks.
Statistical characteristics for the six networks with connectivity distances threshold of a 550 CRV are shown in Table 2. In summer, the number of patches was 92 (M) and 94 (H), the average patch area was 99 km2 (M) and 108 km2 (H), the total patch area was 9,061 km2 (M) and 10,117 km2 (H), and the number of edges was 144 (M) and 152 (H). In winter, the number of patches was 95 (M) and 94 (H), the average patch area was 90 km2 (M) and 107 km2 (H), the total patch area was 8,524 km2 (M) and 10,013 km2 (H), and the number of edges was 149 (M) and 151 (H). Patches and corridors and their corresponding networks were shown in Figure 4.
The area of landscape and forest priority are shown in Figure 5, The area of landscape and forest priority are shown in Table 3. The landscape with high priority, medium priority, low priority, and non-priority comprised 2,027, 3,825, 3,828, and 2,627 km2, respectively. The forests with high priority, medium priority, low priority, and non-priority comprised 1,448, 2,396, 1,929, and 886 km2, respectively. The forests in Hupingshan–Houhe NNR with high priority, medium priority, low priority, and non-priority comprised 596, 64, 58 and 105 km2, respectively, accounting for the 41.2, 2.7, 3.0, and 11.9% of the total forest area.
The priority of forest is indirectly identified by determining the priority areas of the landscape, that is, the priority of the forest is consistent with the priority of the landscape where it is located in. As far as we know, this is a new perspective for assessing forest priorities. In previous study, forest patches with area larger than the predefined threshold were usually regarded as nodes, and then the connectivity between forest patches is directly evaluated. For example, Velázquez et al. (2017) defined areas labelled with the “forest” and “shrub and/or herbaceous vegetation associations” areas as nodes. Saura et al. (2011) selected broadleaved forest, coniferous forest and mixed forest for the connectivity analyses. Jie et al. (2012) selected forest and agricultural dryland patches with an area > 30 km2 as source patches to analyse the importance of agricultural landscape for forest restoration. Ruppert et al. (2016) defined the mature jack pine stands, rocky outcrops, and shallow soil sites as the most preferred habitat type for woodland caribou.
In this study, we built a HS model based on literature reviews, preliminary field work, and expert opinion to assess the suitability of the habitat. Furthermore, we utilized the field data of wild boar to evaluate the outputs of HS model, and only four scenarios (SH, SM, WH, WM) were retained for forest connectivity analysis. The review shows that landscape graphs can benefit from field data of different types at varying scales (Foltête et al., 2020). But biological data are usually gathered from a limited set of locations, as collecting species data on a large scale requires a significant amount of financial and time resources. Thus, we do not discard the results of HS model as they indirectly benefit from methodological improvements and ecological knowledge acquired in studies based on field data. Dutta et al. (2018) showed that HS assessment based on information derived from the literature and field data were a better proxy of empirical models than those designed exclusively from expert opinion.
In our investigation, we kept all patches that contained the core class as source patches. However, only the core class of MSPA analysis was regarded as a source patch in previous study, following the seminal paper of Saura et al. (2011). The patches containing cores and edges can better represent a more natural ecological interference system and provide more interior and edge environmental sources than islets, which then buffers species extinction events due to environmental changes. This novel application of MSPA results may theoretically improve the reliability of source patches analyses.
In this study, the habitat connectivity for wild boar was quantified by indices of and . The forests in Hupingshan–Houhe NNR with high priority comprised 596 km2, accounting for the 41.2% of the total forest area. The results showed that almost all forests in the Hupingshan–Houhe NNR were classified as high priority, which is consistent with the ecological important protection level of Hupingshan–Houhe NNR (Figure 5). But the more important discovery is that 59.8% of the high priority forests are located outside the reserve, which indicates that in order to maintain the connectivity of the wild boar population, the high-priority forests outside the reserve also need to be protected. Strengthening the connectivity between the high priority forests inside and outside the NNR is crucial for the continuation of the wild boar population.
The connectivity distance threshold of links in our study was evaluated by exploring the correlation between the overall probability connectivity (PC) and the dispersal distance, in addition to using community analysis. This method can effectively avoid the adverse effects of excessive connectivity. Since the field data of wild boar dispersal was not recorded, the connectivity distance threshold of edges can be evaluated by using information sourced from like published literature and naturalist association records, but which may produce an excessive connectivity, promoting the spread of pests, diseases or wildfire among populations (Saura et al., 2018; Tang et al., 2021).
As presence–absence data of wild boar are available, which can be directly embedded in the definition of patches from an species distribution model SDM or another output of suitability modeling, following procedures synthesized in Duflot et al. (2018). For instance, the MaxEnt model can provided a reliable predictive map of species habitat and the environmental predictor effects fitted well with the known ecology of the species (Brown et al., 2018).
The habitat suitability data were inverted to provide a resistance map, however habitat suitability does not represent species movement per se. Inferring resistance maps from HS presumes that mobility behavior follows the same criteria as habitat selection, which is not necessarily the case (Zeller et al., 2012). HSI derived from presence points may be a decent surrogate for these processes, and employing inverted HSI as resistance is likely a solid choice in this regard (Blazquez-Cabrera et al., 2016). A further possibility is to combine expert opinion with HSM-derived resistance maps.
The priority of the forest is determined indirectly by determining the priority area in the landscape. This may theoretically improve the reliability of forest priority analyses and the way they are carried out. To be reliable, this approach should preferably be supplemented by a validation process with genetic and telemetry data (Zeller et al., 2018).
In this study, the suitable area was evaluated by the habitat suitability (HS) model, and the prediction accuracy of HS outputs was evaluated by the infrared monitoring data of wild boar. The networks combined with the connectivity index of and were utilized to assess the priority patches and corridors, respectively, and the forest priority is consistent with the priority of the landscape area it belongs to. The results show that not only the forests in the NNR, but also a part of the forests around the NNR are of great significance to maintain the habitat connectivity of wild boar in and around Hupingshan–Houhe NNR. Results of our study are informative for wild boar habitat conservation, corridor establishment, and land use planning of study area in the future. Our study indicated that determining the forest priority through evaluating the landscape priority was an effective approach for forest hotspot selection, and this method can be applied for other areas or animals to identify the priority of forests in the purpose of biodiversity conservation.
The raw data supporting the conclusions of this article will be made available by the authors, without undue reservation.
TT completed the draft. JL obtained projects and provided financial support. JV reviewed and edited the paper. All authors contributed to the article and approved the submitted version.
The National Natural Science Foundation of China (31470642) and the Scientific Research Project of the Education Department of Hunan Province (22B0252) provided financial support for this study.
We gratefully acknowledge the reviewers and editors for their insightful remarks and recommendations.
The authors declare that the research was conducted in the absence of any commercial or financial relationships that could be construed as a potential conflict of interest.
All claims expressed in this article are solely those of the authors and do not necessarily represent those of their affiliated organizations, or those of the publisher, the editors and the reviewers. Any product that may be evaluated in this article, or claim that may be made by its manufacturer, is not guaranteed or endorsed by the publisher.
The Supplementary Material for this article can be found online at: https://www.frontiersin.org/articles/10.3389/fevo.2023.1085272/full#supplementary-material
Avon C., Bergès L. (2016). Prioritization of habitat patches for landscape connectivity conservation differs between least-cost and resistance distances. Landsc. Ecol. 31, 1551–1565. doi: 10.1007/s10980-015-0336-8
Baranyi G., Saura S., Podani J., Jordán F. (2011). Contribution of habitat patches to network connectivity: redundancy and uniqueness of topological indices. Ecol. Indic. 11, 1301–1310. doi: 10.1016/j.ecolind.2011.02.003
Battisti C., Poeta G., Fanelli G. (2016). The concept of disturbance. Environ. Sci. Eng., 7–12. doi: 10.1007/978-3-319-32476-0_2
Bennett V. J., Beard M., Zollner P. A., Fernández-Juricic E., Westphal L., LeBlanc C. L. (2009). Understanding wildlife responses to human disturbance through simulation modelling: a management tool. Ecol. Complex. 6, 113–134. doi: 10.1016/j.ecocom.2008.08.002
Blazquez-Cabrera S., Gastón A., Beier P., Garrote G., Simón M.Á., Saura S. (2016). Influence of separating home range and dispersal movements on characterizing corridors and effective distances. Landsc. Ecol. 31, 2355–2366. doi: 10.1007/s10980-016-0407-5
Bodin Ö., Saura S. (2010). Ranking individual habitat patches as connectivity providers: integrating network analysis and patch removal experiments. Ecol. Modell. 221, 2393–2405. doi: 10.1016/j.ecolmodel.2010.06.017
Brown V. R., Bowen R. A., Bosco-Lauth A. M., Torretta E., Serafini M., Imbert C., et al. (2018). Effects of habitat and landscape characteristics on medium and large mammal species richness and composition in northern Uruguay. Eur. J. Wildl. Res. 8, 1–11. doi: 10.1371/journal.pone.0135254
Burge R. (1999). Riding the tiger: tiger conservation in human-dominated landscapes (Cambridge University Press).
Cabarga-Varona A., Arroyo N. L., Nogués S. (2016). The function of plantation forestry in landscape connectivity. Appl. Ecol. Environ. Res. 14, 527–542. doi: 10.15666/aeer/1402_527542
Campbell Grant E. H., Lowe W. H., Fagan W. F. (2007). Living in the branches: population dynamics and ecological processes in dendritic networks. Ecol. Lett. 10, 165–175. doi: 10.1111/j.1461-0248.2006.01007.x
Duflot R., Avon C., Roche P., Bergés L. (2018). Combining habitat suitability models and spatial graphs for more effective landscape conservation planning: an applied methodological framework and a species case study. J. Nat. Conserv. 46, 38–47. doi: 10.1016/j.jnc.2018.08.005
Dutta T., Sharma S., DeFries R. (2018). Targeting restoration sites to improve connectivity in a tiger conservation landscape in India. PeerJ 2018, 1–22. doi: 10.7717/peerj.5587
Foltête J. C., Clauzel C., Vuidel G. (2012). A software tool dedicated to the modelling of landscape networks. Environ. Model. Software 38, 316–327. doi: 10.1016/j.envsoft.2012.07.002
Foltête J. C., Savary P., Clauzel C., Bourgeois M., Girardet X., Sahraoui Y., et al. (2020). Coupling landscape graph modeling and biological data: a review. Landsc. Ecol. 35, 1035–1052. doi: 10.1007/s10980-020-00998-7
Froese J. G., Smith C. S., Durr P. A., McAlpine C. A., van Klinken R. D. (2017). Modelling seasonal habitat suitability for wide-ranging species: invasive wild pigs in northern Australia. PloS One 12 (5), e0177018. doi: 10.1371/journal.pone.0177018
Gil-Tena A., Brotons L., Fortin M. J., Burel F., Saura S. (2013). Assessing the role of landscape connectivity in recent woodpecker range expansion in Mediterranean Europe: forest management implications. Eur. J. For. Res. 132, 181–194. doi: 10.1007/s10342-012-0666-x
Hebblewhite M., Miquelle D. G., Murzin A. A., Aramilev V. V., Pikunov D. G. (2011). Predicting potential habitat and population size for reintroduction of the far Eastern leopards in the Russian far East. Biol. Conserv. 144, 2403–2413. doi: 10.1016/j.biocon.2011.03.020
Hebblewhite M., Miquelle D. G., Robinson H., Pikunov D. G., Dunishenko Y. M., Aramilev V. V., et al. (2014). Including biotic interactions with ungulate prey and humans improves habitat conservation modeling for endangered amur tigers in the Russian far East. Biol. Conserv. 178, 50–64. doi: 10.1016/j.biocon.2014.07.013
Hernando A., Velázquez J., Valbuena R., Legrand M., García-Abril A. (2017). Influence of the resolution of forest cover maps in evaluating fragmentation and connectivity to assess habitat conservation status. Ecol. Indic. 79, 295–302. doi: 10.1016/j.ecolind.2017.04.031
Jie C., Guofu L., Shengyan D. (2012). Landscape connectivity analysis for the forest landscape restoration:a case study of gongyi city. Acta Ecol. Sin. 32, 3773–3781. doi: 10.5846/stxb201108171210
Kafley H., Gompper M. E., Sharma M., Lamichane B. R., Maharjan R. (2016). Tigers (Panthera tigris) respond to fine spatial-scale habitat factors: occupancy-based habitat association of tigers in chitwan national park, Nepal. Wildl. Res. 43, 398–410. doi: 10.1071/WR16012
Karanth K. U., Nichols J. D. (2010). Non-invasive survey methods for assessing tiger populations. 2nd ed. (Elsevier Inc). doi: 10.1016/B978-0-8155-1570-8.00018-9
Karanth K. U., Nichols J. D., Kumar N. S., Link W. A., Hines J. E. (2004). Tigers and their prey: predicting carnivore densities from prey abundance. Proc. Natl. Acad. Sci. U. S. A. 101, 4854–4858. doi: 10.1073/pnas.0306210101
Karanth K. U., Sunquist M. E. (1995). Prey selection by tiger, leopard and dhole in tropical forests. J. Anim. Ecol. 64, 439. doi: 10.2307/5647
Karanth K. U., Sunquist M. E. (2000). Behavioural correlates of predation by tiger (Panthera tigris), leopard (Panthera pardus) and dhole (Cuon alpinus) in nagarahole, India. J. Zool. 250, 255–265. doi: 10.1017/S0952836900002119
Liu S., Yin Y., Li J., Cheng F., Dong S., Zhang Y. (2017). Using cross-scale landscape connectivity indices to identify key habitat resource patches for Asian elephants in xishuangbanna, China. Landsc. Urban Plan. 171, 80–87. doi: 10.1016/j.landurbplan.2017.09.017
Lõhmus A., Leivits M., Pēterhofs E., Zizas R., Hofmanis H., Ojaste I., et al. (2017). The capercaillie (Tetrao urogallus): an iconic focal species for knowledge-based integrative management and conservation of Baltic forests. Biodivers. Conserv. 26, 1–21. doi: 10.1007/s10531-016-1223-6
Martin A. B., Ruppert J. L. W., Gunn E. A., Martell D. L. (2017). A replanning approach for maximizing woodland caribou habitat alongside timber production. Can. J. For. Res. 47, 901–909. doi: 10.1139/cjfr-2016-0254
Miquelle D. G., Smirnov E. N., Quigley H. B., Hornocker M. G., Nikolaev I. G., Matyushkin E. n. (1996). Food habits of amur tigers in sikhote-alin zapovednik and the Russian far East, and implications for conservation. J. Wildl. Res. 1, 138–147.
Préau C., Grandjean F., Sellier Y., Gailledrat M., Bertrand R., Isselin-Nondedeu F. (2020). Habitat patches for newts in the face of climate change: local scale assessment combining niche modelling and graph theory. Sci. Rep. 10, 1–13. doi: 10.1038/s41598-020-60479-4
Qin Y., Nyhus P. J., Larson C. L., Carroll C. J. W., Muntifering J., Dahmer T. D., et al. (2015). An assessment of south China tiger reintroduction potential in hupingshan and houhe national nature reserves, China. Biol. Conserv. 182, 72–86. doi: 10.1016/j.biocon.2014.10.036
Ruppert J. L. W., Fortin M. J., Gunn E. A., Martell D. L. (2016). Conserving woodland caribou habitat while maintaining timber yield: a graph theory approach. Can. J. For. Res. 46, 914–923. doi: 10.1139/cjfr-2015-0431
Saura S., Bertzky B., Bastin L., Battistella L., Mandrici A., Dubois G. (2018). Protected area connectivity: shortfalls in global targets and country-level priorities. Biol. Conserv. 219, 53–67. doi: 10.1016/j.biocon.2017.12.020
Saura S., Pascual-Hortal L. (2007). A new habitat availability index to integrate connectivity in landscape conservation planning: comparison with existing indices and application to a case study. Landsc. Urban Plan. 83, 91–103. doi: 10.1016/j.landurbplan.2007.03.005
Saura S., Rubio L. (2010). A common currency for the different ways in which patches and links can contribute to habitat availability and connectivity in the landscape. Ecography (Cop.). 33, 523–537. doi: 10.1111/j.1600-0587.2009.05760.x
Saura S., Torné J. (2009). Conefor sensinode 2.2: a software package for quantifying the importance of habitat patches for landscape connectivity. Environ. Model. Software 24, 135–139. doi: 10.1016/j.envsoft.2008.05.005
Saura S., Vogt P., Velázquez J., Hernando A., Tejera R. (2011). Key structural forest connectors can be identified by combining landscape spatial pattern and network analyses. For. Ecol. Manage 262 (2), 150–160. doi: 10.1016/j.foreco.2011.03.017
Shu-Rong T., Cun-Cun Y., Bo-Wen J., Jing D. U., Qing-Yi L., Di-Qiang L. I. (2019). Spatial and temporal patterns of amphibian and reptile diversity in hupingshan national nature reserve. Chin. J. Zool. 54, 825–834. doi: 10.13859/j.cjz.201906009
Shurong T., Hewen L. I., Bowen J., Yiming C., Xin L. I., Diqiang L. I. (2020). Spatial and temporal pattern of mammal diversity in hupingshan national nature reserve. Acta Theriol. Sin. 40, 87–95. doi: 10.16829/j.slxb.150295
Shurong T., Mingxu W., Guangshun J., Li L. I., Zhujie K., Taifu H., et al. (2022). Population viability analysis on reintroduction of south China tiger (Panthera tigris amoyensis) at East wuling mountain range. Chin. J. Wildl. 43, 5–16. doi: 10.19711/j.cnki.issn2310-1490.2022.01.001
Smith A. T., Xie Y., Hoffmann R. S., Lunde D., MacKinnon J., Wilson D. E. (2008). A guide to the mammals of China. Princeton, NJ: Princeton University Press.
Soille P., Vogt P. (2009). Morphological segmentation of binary patterns. Pattern Recognit. Lett. 30, 456–459. doi: 10.1016/j.patrec.2008.10.015
Sunquist M. E (1981). The social organization of tigers (Panthera tigris) in royal chitawan national park, Nepal. Smithson. Contrib. to Zool, 1–98. doi: 10.5479/si.00810282.336
Sunquist M. E. (1981). The social organization of tigers (Panthera tigris) in royal chitawan national park, Nepal. Smithson. Contrib. to Zool, 1–98. doi: 10.5479/si.00810282.336
Tang T., Li J., Sun H. (2023). Integrated approach considering seasonal variability and habitat uncertainty to map habitat for the prey of south China tiger. Ecol. Indic. 148, 110082. 148, 0–2. doi: 10.1016/j.ecolind.2023.110082
Tang T., Li J., Sun H., Deng C. (2021). Priority areas identified through spatial habitat suitability index and network analysis: wild boar populations as proxies for tigers in and around the hupingshan and houhe national nature reserves. Sci. Total Environ. 774, 145067. doi: 10.1016/j.scitotenv.2021.145067
Urban D. L., Minor S., Treml E. A., Schick R. S. (2009). Graph models of habitat mosaics. Ecol. Lett. 82, 1205–1218. doi: 10.1890/0012-9658(2001)082[1205:LCAGTP]2.0.CO;2
Urban D., Keitt T., Ecology S., May N. (2001). Landscape connectivity: a graph-theoretic perspective. America (NY). 82, 1205–1218. doi: 10.1890/0012-9658(2001)082[1205:LCAGTP]2.0.CO;2
Velázquez J., Gutiérrez J., García-Abril A., Hernando A., Aparicio M., Sánchez B. (2019). Structural connectivity as an indicator of species richness and landscape diversity in castilla y león (Spain). For. Ecol. Manage. 432, 286–297. doi: 10.1016/j.foreco.2018.09.035
Velázquez J., Gutiérrez J., Hernando A., García-Abril A. (2017). Evaluating landscape connectivity in fragmented habitats: cantabrian capercaillie (Tetrao urogallus cantabricus) in northern Spain. For. Ecol. Manage. 389, 59–67. doi: 10.1016/j.foreco.2016.12.006
Wei L. I., Xiahui Y. A. N., Qiang Z., Jinbo Z., Wenwu D., Ecological C., et al. (2020). Research Status of South China Tiger. J. Econ. Anim. 24 (2), 5–9. doi: 10.13326/j.jea.2019.1383
Yu G., Kang Z., Li M. S., Chen Z. F., Deng Z. C. (2018). Preliminary survey using infrared camera reveals fauna and avifauna diversity at hupingshan national nature reserve, hunan, China. Acta Theriologica Sinica. 38 (1), 104–112. doi: 10.16829/j.slxb.150137
Zeller K. A., Jennings M. K., Vickers T. W., Ernest H. B., Cushman S. A., Boyce W. M. (2018). Are all data types and connectivity models created equal? validating common connectivity approaches with dispersal data. Divers. Distrib. 24, 868–879. doi: 10.1111/ddi.12742
Keywords: forest, priority level, habitat suitability, network analysis, Sus scrofa
Citation: Tang T, Li J and Velázquez J (2023) Determination of forest priority levels for wild boar by analysis of habitat suitability and landscape connectivity. Front. Ecol. Evol. 11:1085272. doi: 10.3389/fevo.2023.1085272
Received: 31 October 2022; Accepted: 27 June 2023;
Published: 25 July 2023.
Edited by:
Matthew Grainger, Norwegian Institute for Nature Research (NINA), NorwayReviewed by:
Corrado Battisti, Roma Tre University, ItalyCopyright © 2023 Tang, Li and Velázquez. This is an open-access article distributed under the terms of the Creative Commons Attribution License (CC BY). The use, distribution or reproduction in other forums is permitted, provided the original author(s) and the copyright owner(s) are credited and that the original publication in this journal is cited, in accordance with accepted academic practice. No use, distribution or reproduction is permitted which does not comply with these terms.
*Correspondence: Jiping Li, bGlqaXBpbmcxNjAyQDE2My5jb20=
†ORCID: Javier Velázquez, orcid.org/0000-0002-9188-3827
Jiping Li, orcid.org/0000-0003-0875-1784
Tao Tang, orcid.org/0000-0002-4975-0828
Disclaimer: All claims expressed in this article are solely those of the authors and do not necessarily represent those of their affiliated organizations, or those of the publisher, the editors and the reviewers. Any product that may be evaluated in this article or claim that may be made by its manufacturer is not guaranteed or endorsed by the publisher.
Research integrity at Frontiers
Learn more about the work of our research integrity team to safeguard the quality of each article we publish.