- 1Ecology and Evolutionary Biology, University of California Santa Cruz,Santa Cruz, CA, United States
- 2Fisheries Ecology Division, Southwest Fisheries Science Center, Santa Cruz, CA, United States
- 3Marine Science Center, Northeastern University, Nahant, MA, United States
- 4Marine and Environmental Biology, University of Southern California, Los Angeles, CA, United States
- 5Department of Ocean Sciences, University of California Santa Cruz, Santa Cruz, CA, United States
Although the concept of connectivity is ubiquitous in ecology and evolution, its definition is often inconsistent, particularly in interdisciplinary research. In an ecological context, population connectivity refers to the movement of individuals or species across a landscape. It is measured by locating organisms and tracking their occurrence across space and time. In an evolutionary context, connectivity is typically used to describe levels of current and past gene flow, calculated from the degree of genetic similarity between populations. Both connectivity definitions are useful in their specific contexts, but rarely are these two perspectives combined. Different definitions of connectivity could result in misunderstandings across subdisciplines. Here, we unite ecological and evolutionary perspectives into a single unifying framework by advocating for connectivity to be conceptualized as a generational continuum. Within this framework, connectivity can be subdivided into three timescales: (1) within a generation (e.g., movement), (2) across one parent-offspring generation (e.g., dispersal), and (3) across two or more generations (e.g., gene flow), with each timescale determining the relevant context and dictating whether the connectivity has ecological or evolutionary consequences. Applying our framework to real-world connectivity questions can help to identify sampling limitations associated with a particular methodology, further develop research questions and hypotheses, and investigate eco-evolutionary feedback interactions that span the connectivity continuum. We hope this framework will serve as a foundation for conducting and communicating research across subdisciplines, resulting in a more holistic understanding of connectivity in natural systems.
1. Introduction
A fundamental question in biology is how the connections between populations of organisms, particularly of the same species, impact their ecology and evolution. However, the nature of such connections, or “connectivity,” is characterized differently depending on the subdiscipline or research question (Lowe and Allendorf, 2010; Kool et al., 2013; Keeley et al., 2022). Ambiguity in definitions can lead to misunderstandings between scientists attempting to work across subdisciplines or manage different components of an ecosystem. Yet, integrative work is essential to the current practice of ecology and evolutionary biology (Habel et al., 2015), as well as the successful management of ecosystems under rapid environmental change (Vos et al., 2008). The nature of connections among populations and species have important implications for their present persistence and future adaptations. We present a unifying framework for the study of population connectivity in ecology and evolutionary biology to facilitate communication and collaboration between subdisciplines without requiring subdisciplines to adopt a universal vocabulary.
At its most basic level, connectivity describes the relationship between populations or groups of organisms. The degree of connectivity is “the outcome of dependencies between populations or individuals” (Kool et al., 2013). Broadly, connectivity might be defined as the movement of individuals between groups or species (UN CMS, 2020), the flow of genes between groups (Habel et al., 2015), or even indirect interactions between groups across a landscape (Sheaves, 2009). Population connectivity concerns the first two definitions – the flow of organisms or genes. For specific research questions, all of these connectivity definitions describe real phenomena and are therefore useful. However, inconsistent definitions pose problems when researchers attempt to collaborate or synthesize information across different fields (Lowe and Allendorf, 2010; Kool et al., 2013).
When attempting to work across disciplines or with new sources of data, inconsistent definitions create misunderstandings and unnecessary friction. The process of collaboration is “laden with semantic issues that at best slow down the process, and at worst, exclude some from the conversation” (Pennington, 2008). For example, many stock assessments assume that fish stocks (groups of fish captured within an explicit geographic area) are discrete, spatially circumscribed units exhibiting internally homogenous dynamics (Secor, 2005). Mismatch between the management definition of a stock and the ecological, demographic, and, especially, the genetic relationship among groups of fish caught within that area can result in suboptimal management (Lipcius et al., 2008; Cadrin et al., 2014; Spies and Punt, 2015; Kerr et al., 2017; Harrison et al., 2020). Similarly, disagreements surrounding the definition of “species” have led to conflict over listing species under the United States Endangered Species Act (Waples, 1991; Crifasi, 2007; Carolan, 2008; Levin et al., 2021). Outside of management applications, collaborations across disciplines are frequently characterized by circular conversations about terminology disagreements. This situation is particularly exacerbated when fields are highly interconnected with overlapping terminology, as is the case in the subdisciplines of ecology and evolutionary biology (Dewulf et al., 2007; Habel et al., 2015).
Here, we address the confusion resulting from inconsistent definitions of population connectivity by developing a single unifying framework. Rather than focusing on generating specific terminology or definitions, we instead focus on identifying the inferences we gain about connectivity when we view it from the different timescales used in various fields. By focusing on the inference rather than the terminology, we hope to facilitate collaborations and data integration efforts across subdisciplines. First, we summarize current definitions of population connectivity and provide a glossary of relevant terms. Next, we introduce our population connectivity framework, which focuses on the relationships between different measures of population connectivity and time, and highlight the specific inferences provided by each. Finally, we describe an example application, applying the connectivity framework to summer flounder, Paralichthys dentatus, to contextualize our framework.
2. Concepts of population connectivity
Though it is difficult to pinpoint the exact origin, the idea of connectivity between populations likely arose in patch dynamics theory beginning in the 1970s (e.g., Levins, 1969; Reddingius and den Boer, 1970; Levin and Paine, 1974; Levin, 1976; Roff, 1974). These early population models (e.g., Levins, 1969) recognized the interplay between migration and extinction in connected heterogenous landscapes, but migration was explicitly tied to the movement of organisms. Merriam (1984) introduced the concept of “landscape connectivity,” which noted that movement among habitat patches was not merely a function of an organism’s attributes, but also the quality of the landscape through which they moved. Formative debates within the field of landscape ecology concerned whether connectivity exists at the habitat patch-level definition, or whether connectivity is an emergent property at the landscape scale (Merriam, 1984; Taylor et al., 1993; Tischendorf and Fahrig, 2000; Moilanen and Hanski, 2001; Moilanen and Nieminen, 2002).
As early as the mid-20th century, geneticists described populations in terms of “allelic divergence” or “gene frequency” differences to better understand the evolutionary processes at play in a system (Wright, 1931, 1943; Speith, 1974; Allendorf and Phelps, 1981). Wright’s (1931, 1949) foundational ideas about the genetics of isolated populations are arguably an antecedent of metapopulation theory (Hastings and Harrison, 1994; Harding and McNamara, 2002), where populations are conceptualized as “populations of populations” or collections of population groups that interact via immigration and emigration (Levins, 1969). Interactions among metapopulations have implications for population persistence and evolution (Hanksi, 1994; Hanski and Gaggiotti, 2004; Hanski, 2011). During this period in evolutionary biology, the presumed movement of individuals between populations and their subsequent contribution to reproduction was often described as “gene flow” and quantified through F-statistics or their derivatives (see Glossary or Section 2.2). Recognizing the importance of the landscape for gene flow, some studies began to use “connectivity” to describe how physical barriers to dispersal led to genetic isolation and drift (Soulé and Simberloff, 1986; Simberloff and Cox, 1987; Green, 1994; Hastings and Harrison, 1994). Attempts to understand the scale of physical dispersal and biogeography readily took advantage of novel genetic tools for measuring genetic divergence (Hedgecock et al., 1988). For those using a genetics toolbox, the term “connectivity” came to describe past or present gene flow across space (Benzie and Ballment, 1994; Mills and Allendorf, 1996; Roberts, 1997).
With these conflicting terms, it became important to distinguish between different types of population connectivity (Waples and Gaggiotti, 2006; Lowe and Allendorf, 2010). Yet, delineating what constitutes a population is not always straightforward (Funk et al., 2005) and determining the “connectivity” of poorly defined units can be even more complicated. For example, estimates of connectivity using genetics have been used to design marine reserves and designate management units (Roberts, 1997; Palsbøll et al., 2007; Jenkins and Stevens, 2018). In many cases, landscape attributes or physical-biological models of dispersal correlated well with early genetic studies of marine fish species where high dispersal capacity manifested in high gene flow over large spatial scales (Rosenblatt and Waples, 1986; Shulman and Bermingham, 1995). Studies like these support the notion that “open” populations with high immigration and emigration rates can be genetically well-mixed. However, biological oceanographers using coupled biophysical models and fish behavior studies have found many fish species to be comprised of highly structured populations, even in the face of high dispersal (Cowen et al., 2000, 2006). As genetic tools were further refined, it was possible to detect genetic structure at fine scales, despite high dispersal capacity (Doherty et al., 1995; Shulman and Bermingham, 1995; Ruzzante et al., 2006). These studies revealed that populations may be more “closed” to demographic exchange than previously thought. These debates on whether populations are fundamentally “open” or “closed” indicate a rift between the gene flow and movement-based concepts of connectivity.
Attempts have been made to generate clear distinctions between conceptions of connectivity (famously Lowe and Allendorf, 2010), as genetic data alone provides little information on organism movements across a landscape (Lowe and Allendorf (2010) term this “demographic connectivity”). Tischendorf and Fahrig (2000) examined the usage of the term “landscape connectivity” in 33 different studies and identified three major areas of confusion: (1) functional connectivity based on organism movements vs. structural connectivity based on habitat contiguity, (2) patch isolation vs. landscape connectivity, and (3) corridors vs. connectivity. Calabrese and Fagan (2004) critiqued the “inconsistently defined concept” of connectivity and developed a framework for classifying connectivity metrics according to data requirements, spatial scales and information yield. Waples and Gaggiotti (2006) reviewed commonly used definitions of “population,” differentiating between the ecological paradigm, which emphasizes demographic cohesion, and the evolutionary paradigm, which emphasizes reproductive cohesion. Despite these attempts, the use of the ambiguous term “connectivity” in both ecological and genetic studies continues apace: As of the writing of this manuscript there are 710,000 studies with “ecology” and “connectivity” as keywords, 127,000 studies with “genetic connectivity” or “genomic connectivity,” and only 2,610 using “demographic connectivity” (via Google Scholar).
As there are already many definitions and multiple, previous efforts to generate a universal vocabulary, we instead focus this manuscript on distinguishing the different concepts of population connectivity by their underlying processes–ecology or evolution. For our purposes, we have divided conceptions of population connectivity into two groups: ecological population connectivity (hereafter “ecological connectivity”) and evolutionary population connectivity (hereafter “evolutionary connectivity”). The specific terms themselves are not important – indeed, evolutionary connectivity is synonymous with genetic connectivity in most senses. What is important is the process of interest and the questions motivating researchers. Below we outline ecological and evolutionary conceptions of connectivity by first describing the fields, questions, and techniques used to study connectivity, and we then show how these conceptions have essential differences related to time.
2.1. Estimating ecological population connectivity
Conservation ecology, behavioral ecology, and landscape ecology typically invoke connectivity as the movement of organisms across a landscape (Sheaves, 2009; UN CMS, 2020; Keeley et al., 2022). In this conception, groups of species are connected directly through interactions with one another or indirectly through interactions with the same processes and/or landscape. Terms in the literature that describe this conception include, “demographic connectivity,” and “migratory connectivity” (Webster et al., 2002; Lowe and Allendorf, 2010). Questions involving ecological population connectivity often measure aspects of resource use and availability, seasonal migration rates, or trophic structures (Sheaves, 2009; Fang et al., 2018). The spatial scales of these measurements are defined by the interactions themselves (Keeley et al., 2022), from insect interactions on a single plant to whale interactions in an ocean basin.
To understand ecological connectivity, researchers locate organisms within a landscape and measure their occurrence. Occurrence data quantify a target organism’s presence and absence within space and time. With occurrence data, researchers can analyze patterns of co-occurrence, such as whether species A is likely to occur in proximity to species (or resource) B. The two principal methods of acquiring occurrence data are through tracking and surveys. Tracking involves sampling the same individual organism through time, sometimes through the use of transmitting, or “active” devices such as satellite tags (Kool et al., 2013; Hussey et al., 2015; Kays et al., 2015). Surveys establish the presence of an individual or a group in a location, but do not necessarily track specific individuals across time (Burton et al., 2015; Supp et al., 2021; Hart et al., 2022). A single survey of whales, for example, may identify individuals at a location, but there is no guarantee the same individuals will be present to provide data on the next survey. Occurrence can also be determined through the use of so called “natural tags,” such as genetic samples taken from feces or fur, environmental DNA samples, isotope signatures identifiable to specific locations, or characteristic phenotypes endemic to a particular place (Kool et al., 2013; Schmidt et al., 2013; Azarian et al., 2021). In all these cases of ecological connectivity, the mode of determining connectivity relies on the co-occurrence, or overlap, of sampled organisms with other individuals, species, or processes. For a larger list of definitions and terms, see the Glossary (Table 1).
Ecological connectivity is the mixing of individuals in space and time and has implications for a variety of natural processes. This kind of connectivity could be a mechanism for evolutionary connectivity if breeding and gene flow occurs, but ecological connectivity itself does not require gene flow. When focused on connections within the same species (such as for conservation corridors), ecological connectivity often results in gene flow and impacts observed genetic population structure, with consequences for species or population persistence (Lowe and Allendorf, 2010; Kool et al., 2013). Because many animal populations have become increasingly fragmented, high levels of connectivity can help to ensure healthy population sizes (Lehnen et al., 2021; Fontoura et al., 2022). When focused on the relationships among different species, ecological connectivity impacts ecological processes such as competition and predation. Species with similar niches are expected to exhibit increased niche partitioning or behavioral avoidance when their occurrence overlaps (Brown and Wilson, 1956; Albrecht and Gotelli, 2001). Predator and prey species experience similar separations, with many prey species exhibiting predator avoidance as predator occurrence increases (Hampton, 2004; Sommers and Chesson, 2019). A variety of large-scale processes are mediated by ecological connectivity: herd concentrations can create characteristic patterns of nutrient flow [e.g., increased fecal deposits (Polis et al., 1997)] and the interactions between sea otters and sea urchin populations have trophic consequences for kelp forests (Smith et al., 2021). Ecological connectivity can also provide opportunities for cultural or disease transmission; a relevant context as we draft this manuscript in the midst of the COVID-19 pandemic (Tao et al., 2021).
2.2. Estimating evolutionary population connectivity
The fields of molecular ecology, evolutionary genetics, and population genetics generally use the term connectivity to describe the degree to which groups of individuals interbreed, thereby describing the degree of genetic homogeneity. These fields are chiefly concerned with how the interconnectedness of two or more groups impact the evolutionary processes of those groups, or the spatially-structured population as a whole. In this conception, the terms “population connectivity,” “genetic connectivity,” “number of migrants,” and “number of dispersers” are often used as a synonym for gene flow on both short (microevolutionary) and long (macroevolutionary) timescales. For the purposes of this paper, we will refer to gene flow across any timescale as “evolutionary connectivity.” Connections within or between populations are forged through reproductive events, which occur following some sort of interaction among individuals. However brief, active or passive these interactions might be, ones that result in successful reproduction will impact gene flow and may have downstream evolutionary consequences.
To understand evolutionary connectivity, researchers search for shared genetic material to estimate population structure and determine the degree of gene flow necessary to achieve such genetic structure. The degree of evolutionary population connectivity is inferred by assessing the frequency of individual genetic variants (called molecular tags or markers) across a populations. Early molecular markers included allozymes or a few mitochondrial or nuclear markers, but more recently, the use of microsatellites, large numbers of single nucleotide polymorphisms (SNPs; obtained from reduced representation or whole genome approaches), or haplotypes have become increasingly common (Mariani and Bekkevold, 2014; Dorant et al., 2019; D’Aloia et al., 2020). In all cases, the mode of determining connectivity seeks to estimate the proportion of shared genetic material among populations, often, but not always, of the same species. Many factors can affect estimates of gene flow, including dispersal rates among populations, effective population size of the source group, immigrant survival, and relative reproductive success (Cowen and Sponaugle, 2009; Lowe and Allendorf, 2010). For a larger list of definitions and terms, see the Glossary (Table 1).
Evolutionary connectivity influences the levels of genetic diversity within populations and species, which can impact the capacity for further evolution under new conditions (Forester et al., 2022). When levels of gene flow are low, populations can diverge genetically, which, given time and reproductive isolation, results in speciation (Mayr, 1942). In his seminal paper, Wright formalized F-statistics, including FST, which described how genetic variation in a biallelic system is partitioned among discrete populations (Wright, 1931). The smaller FST is, the more similar allele frequencies are among and within individual populations. Conversely, the larger FST is, allele frequencies among and within populations exhibit a greater degree of differentiation (for a comprehensive review of FST, see Holsinger and Weir, 2009). In this same paper, Wright also showed that the number of migrants could be indirectly approximated using FST, solidifying the relationship between observed patterns of genetic differentiation and gene flow, per se (Wright, 1931). This led to the “one migrant per generation” rule in conservation, in which a small amount of gene flow is enough to avoid the deleterious effects of genetic drift and inbreeding (Mills and Allendorf, 1996). However, it has since been recognized that this method for calculating the number of migrants may be too simplistic for real populations (Whitlock and Mccauley, 1999).
When detectable levels of genetic differentiation among populations are present, such differentiation can be used to assign individuals to their population of origin, which can be useful for conservation and management (Manel et al., 2005; Reid et al., 2018). In addition, spatially divergent selection, even in the presence of high gene flow, can result in the adaptation of populations to local environmental conditions (Hedrick et al., 1976; Kawecki and Ebert, 2004; Whitlock, 2015). Such local specialization can result in reduced genetic diversity at the scale of individual populations but may increase the overall genetic diversity of the metapopulation or species as a whole. Conversely, divergence followed by an increase in gene flow (e.g., secondary contact) can lead to hybridization and introgression among populations, which may increase genetic diversity but decrease the prevalence of local adaptations (Allendorf et al., 2001; Marques et al., 2019). This tradeoff occurs because increased connectivity and hybridization can lead to the homogenization of the gene pool and the loss of unique genetic combinations present only in isolated populations (Muhlfeld et al., 2017). On the other hand, introgressive hybridization has the potential to rapidly increase evolutionary innovation through increased standing genetic variation or the introduction of pre-adapted alleles into a population (Rieseberg et al., 2003; Jones et al., 2018; Oziolor et al., 2019). Gene flow can also increase genetic variation and the effective size of a population, which may help to buffer small populations from extinction through increased evolutionary potential (Holt and Gomulkiewicz, 1997). Parameters such as effective population size, the rate of population divergence, and introgression between populations are all mediated through evolutionary connectivity.
3. A generational framework for understanding population connectivity
Given the different ways of looking at population connectivity (i.e., ecologically and evolutionarily), how can we conceptualize the various connections among populations? Is there a framework we can use across subdisciplines which does not necessitate a universal vocabulary but can help synthesize inferences gained from different approaches? As described above, over the course of a lifetime, organisms will interact directly or indirectly with other individuals in a multitude of ways, from simply sharing space to consuming each other. Organisms may also interact through reproduction, thus passing genetic information across generations and maintaining gene flow between their populations. While the ecological or evolutionary processes resulting in population connectivity are continuous, we propose that focusing on the timescale of the interaction will result in more fruitful collaborations when working across biological subdisciplines. Therefore, we conceptualize population connectivity across discrete generational timescales, where the timescales together represent a time continuum. Just as a ripple propagates across a body of water from its point of origin, we can understand how biological interactions impact population connectivity within and across generations when we use this generational framework (Figure 1). Observations of the ripple near its point of origin encompass short generational timescales and ecological connectivity whereas observations of the ripple further away from its origin represent longer generational timescales and evolutionary connectivity. By focusing on a particular generational timescale, researchers may make inferences about specific interactions or processes, such as competition or reproduction, but observations over greater and greater generational timescales (and space in the case of a ripple) will result in a more holistic understanding of population connectivity.
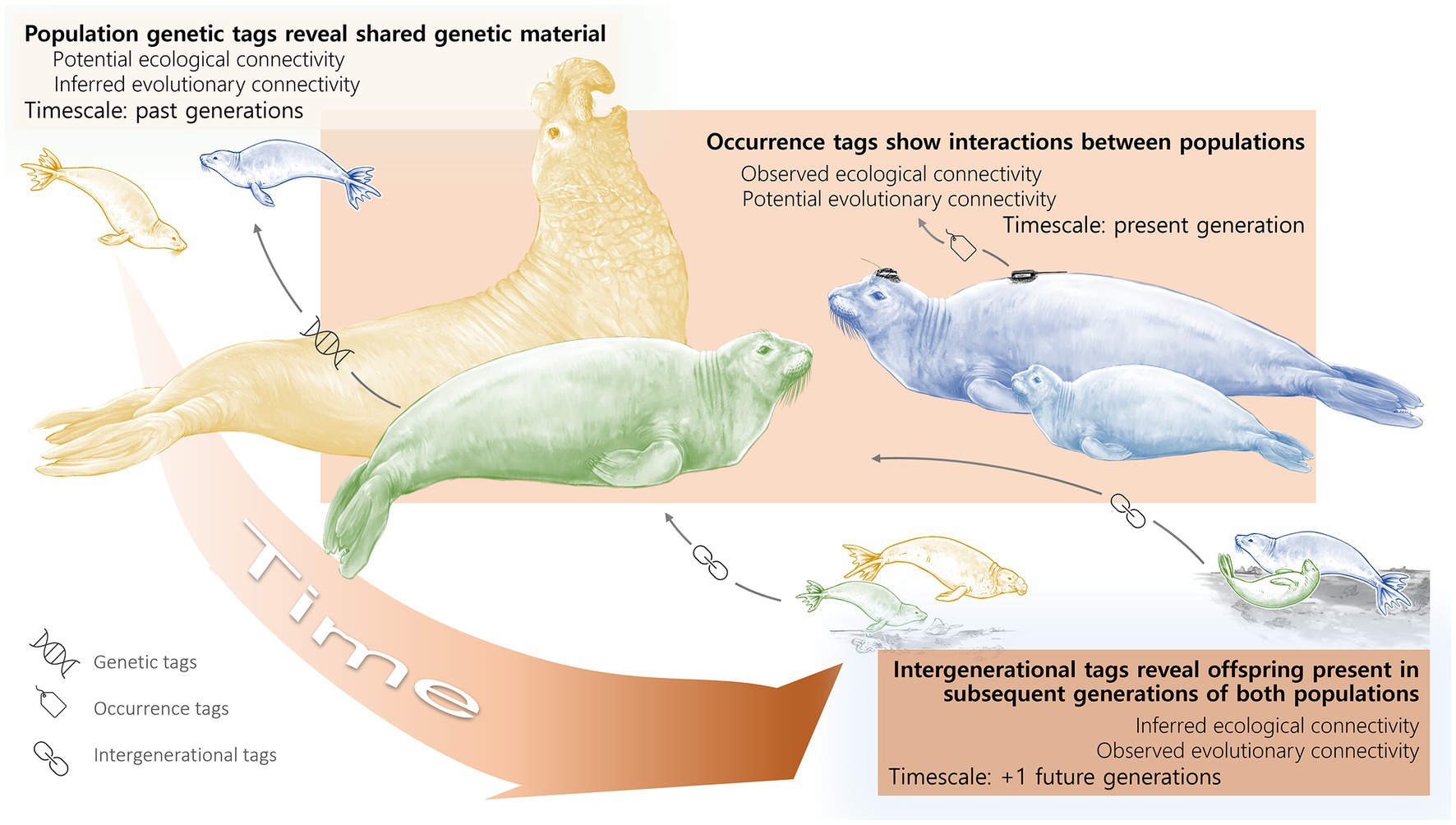
Figure 1. Timescales of Connectivity. Connectivity of two hypothetical parental populations from different locations (yellow and blue elephant seals) and an admixed population (green elephant seal) when viewed across the different generational timescales outlined in our framework. Time connects the elephant seal populations from the past (top left) to the present (center) to the future (bottom right), where the color saturation of each box corresponds with a discrete generational timescale along the time continuum. In this example, the elephant seal populations are connected, both ecologically and evolutionarily, yet the nature of the connectivity depends on the timescale of interest. In our framework, ecological connectivity encompasses direct and indirect interactions throughout an organism’s lifetime while evolutionary connectivity is gene flow among populations. Ecological connectivity occurs within the present generation. Evolutionary connectivity occurs across generations, whether looking back or forward in time. The timescales and processes about which we can make inferences vary depending on the sampling method and the generation to which it was applied (see bottom left icons and black arrows showing how sampling methods can reveal connectivity along the generational continuum). For example, population genetic data (“genetic tags”) sampled in the present generation of the green elephant seal population can reveal past connectivity between the yellow and blue populations, whereas “occurrence tags” in the present generation of the blue population track only the movement of individuals in the present generation. “Intergenerational tags” that sample individuals across a generation, whether through genetics or visual observations of individuals and their distinct markings, connect parents and offspring. In addition, genetic tags provide insight on reproduction over multiple generations, which, depending on the mode of reproduction may or may not have required organisms to overlap in space. Occurrence tags provide insights on spatial overlap, which may or may not coincide with mating. Intergenerational tags provide insight on reproduction from the proceeding generation and can be paired with additional data to indicate spatial overlap.
For example, the northern elephant seal (Mirounga angustirostris) population experienced an enormous decline along the eastern Pacific: less than 20 individuals remained by the late 1800s (Hoelzel et al., 1993). Determining the connectivity of this population today is dependent on the timescale of interest. From an evolutionary perspective, all elephant seals along the west coast of North America stem from one single, bottlenecked group. Over 100 years later, genetic estimates show there is essentially no genetic variability (Fst ~ 0) within the population (Abadía-Cardoso et al., 2017). Elephant seals tend to return to their natal beaches, but their long generation time (~8 years; estimate from LeBoeuf and Reiter, 1988) means there has not been enough time for any significant genetic divergence between them on a population scale. Genetic markers which focus on specific subpopulations might be able to distinguish between natal beaches, but differences between subpopulations are small (Abadía-Cardoso et al., 2017; as is the case with the blue and yellow seals on the left in Figure 1 despite the population as a whole being a single connected unit). Ecologically, however, the interactions that individual elephant seals have with conspecifics and other species vary depending on their natal beach location, as well as sex and age. For example, while adult elephant seals tend to remain near their breeding shore, juvenile elephant seals instead wander broadly, hauling out on beaches up and down the coast (this may be revealed by tagging data, as shown on the blue elephant seal in the center of Figure 1). The interactions between different elephant seal subpopulations therefore depend on when a researcher looks for a connection; competitive interactions, disease transmission, etc. could all happen between natal beaches during the juvenile phase, even if the adults remain largely isolated. The amount of “population connectivity” therefore depends on the timescale of interest (within or across generations) and the underlying process (ecological or evolutionary).
In our generational framework, connectivity can be subdivided into three timescales: (1) connectivity within a generation (center of Figure 1), (2) connectivity between parent and offspring (across one parent–offspring generation, right side of Figure 1), and (3) multigenerational connectivity (2+ generations, left side of Figure 1). Connectivity within a generation has ecological consequences (e.g., resource competition, habitat use, foraging, etc.) and may have implications for gene flow and evolutionary connectivity. Connectivity between parent and offspring can have ecological or evolutionary consequences. Finally, connectivity on longer timescales can have evolutionary consequences, which may ultimately have impacts on ecological processes (eco-evolutionary feedbacks).
While the degree to which populations are connected across space and time may change, connectivity at the shortest timescales (ecological scales) radiates outward to influence connectivity at longer timescales (evolutionary scales). Thus, population connectivity along the timescale continuum is nested, in that it both depends upon previous timesteps and may influence subsequent timesteps. But measures of connectivity at different timescales are not perfect reflections of each other. Populations can be both ecologically and evolutionarily connected at a given moment. Evolutionarily connected populations must have had some ecological connectivity in the past, but exactly when that occurred will vary. Ecologically connected populations of related species may have once interbred, but due to reduced gene flow and eventual speciation, may now be connected only through competition. Ecological connectivity occurs on a continuum, as individuals of a species persist throughout time. In contrast, evolutionary connectivity occurs at discrete time points; once gene flow occurs, the level of evolutionary connectivity cannot change until the next mating event. Direct observations of ecological connectivity are bound to the present while evidence of evolutionary connectivity can persist through time.
These three timescales of connectivity also capture how connectivity is measured. For instance, occurrence tags track individuals over part or all of their lives, and thus can only directly capture connectivity at the timescale of less than one generation – ecological connectivity. Parent–offspring connectivity (ecological and evolutionary connectivity) can be inferred from genetic parentage analysis, where genetic tags are characterized in the parents and “recovered” in the offspring. Parent–offspring relationships can also be deduced based on distinct visual markings and repeat observations (Bain, 1990). Both of these methods track connectivity across one generation of parent and offspring, thus functioning as “intergenerational tags.” Multigenerational connectivity is tackled by the broad field of population genetics, which provides insight into connectivity patterns on longer timescales than parent–offspring connectivity (i.e., 2+ generations, evolutionary connectivity). Assuming adequate sampling, each approach provides inferences about aspects of connectivity at different timescales, resulting in a more complete picture of connectivity.
This generational framework for population connectivity clarifies the limitations of different sampling approaches. Any method which samples within a generation makes several assumptions about connectivity across generations. For example, in addition to the common assumption that tagged individuals are reflective of an entire population, tracking studies often also assume that present behavior reflects past behavior. Similarly, sampling methods which target past generations (i.e., population genetic methods) are used to hypothesize on ecological connections that cause the observed patterns, but they may differ dramatically from present patterns. For example, is a genetic bottleneck a result of population reduction due to disease or physical separation from a larger population (i.e., a founder effect)? To understand connectivity from a more holistic perspective, multiple sampling approaches must be employed.
This generational framework allows us to ask synthetic questions about the causes and consequences of connectivity. By thinking of connectivity generationally, we can ask how specific ecological connectivity processes impact the mating pool of a single generation (Figure 2). Across generations, this mating pool effect has implications for the subsequent (i.e., F1 and following) generations, with eventual consequences for evolutionary connectivity. Processes that increase the availability of prospective mates should, all things being equal, forge new connections via gene flow and increase the genetic diversity within a population. These processes could be direct, such as new interactions with a population of the same species, or indirect, such as reduced competition leading to an increase in available mates. Over the long term, increased connectivity should decrease the prevalence of local phenotypes and their associated genotypes, and potentially increase the occurrence of hybridization (i.e., more overall genetic diversity, but less genetic adaptation to particular local environments). In contrast, processes which decrease the mating pool within a generation will generally reduce overall genetic diversity, but could possibly increase the prevalence of local adaptation, though the outcome of these various scenarios will depend on the interplay between natural selection and genetic drift. These processes could include the presence of a new predator or the reduction of available nutrients. Crucially, not all increases to the mating pool result in increased evolutionary connectivity – only new gene flow between distinct populations creates increased connectivity (defining what counts as a distinct population is a perpetual exercise and outside of the scope of this paper).
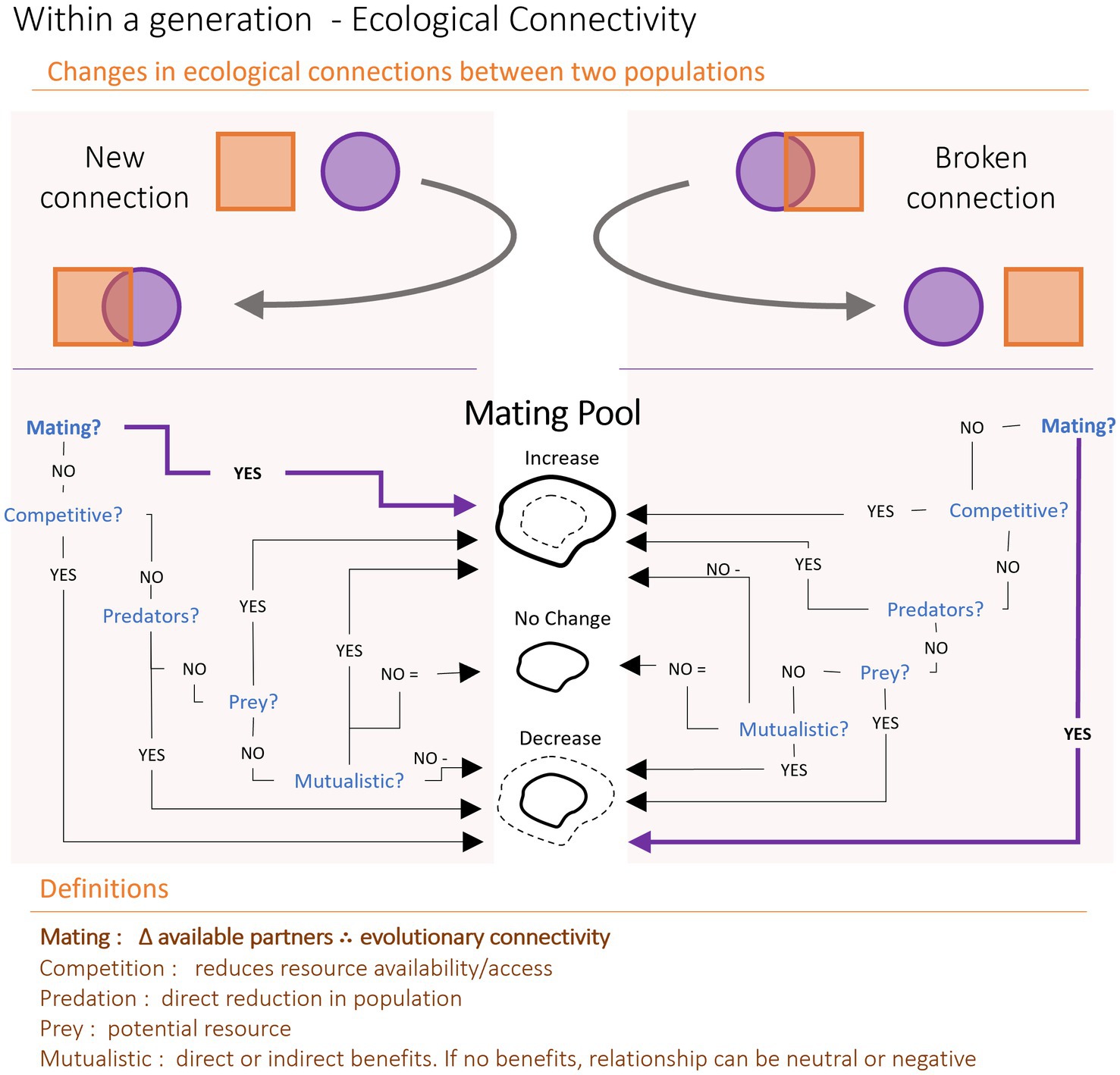
Figure 2. Impacts of ecological connectivity on evolutionary connectivity via the mating pool. Two populations, the purple circle and the orange squares, can form new (left side) or break old (right side) ecological connections. These connections have implications for the number of individuals in the mating pool. The only way to directly alter evolutionary connectivity is to change mating (in bold pathways); when new populations mate (i.e., same or closely related species) this results in gene flow. Similarly, when previously mating populations stop or lower reproduction, the change to the mating pool reduces gene flow. By looking explicitly at how ecology may impact a mating pool, we can generate predictions for how ecological connectivity impacts evolution. Given a population with a consistent mutation rate and no emigration (“ideal population” in population genetic parlance), a decreased mating pool via mechanisms such as predation can result in static or reduced genetic diversity and increased local adaptation. An increased mating pool from new immigrants or higher survivorship may lead to increased genetic diversity and hybridization, along with a decrease in local adaptations.
We can also use this generational perspective to examine how evolutionary connectivity may or may not impact an organism’s ecological connectivity (Figure 3). We know that evolutionarily connected populations must contain some shared genetic material and, within a focal population, this shared material may be maintained or removed through natural selection or random chance (i.e., genetic drift; Griffiths, 2005). Evolutionary connectivity has consequences for ecological connectivity if the presence of shared genetic material alters a species’ pattern of occurrence within the present generation. For this to occur, and assuming a strong enough selection coefficient and a large enough population size, the genetic material must first impact some trait which has ecological importance – if the trait is neutral, the impact will not be felt evolutionarily or ecologically (Mariani and Bekkevold, 2014; Xuereb et al., 2021). Secondly, trait impacts must be relevant for the present generation. For example, a trait expanding tolerance to cold may be evolutionarily important, but if current temperatures do not drop below a meaningful minimum temperature threshold, the trait is not expected to change the population’s current distribution. In contrast, if gene flow introduces variation in a trait relevant to current conditions, the distribution of a population within its range may shift, potentially altering the degree of direct or indirect contact between the focal population and its potential interactors. Thirdly, and relatedly, to impact ecological connectivity, a trait must inherently be under selection (i.e., the frequency of the trait is expected to change in subsequent generations). If all of this is true, specifically if introduced variation is functional, presently ecologically important, and under selection (i.e., not neutral), such evolutionary connectivity will impact ecological connectivity via altered occurrence, and therefore interactions, of the focal species.
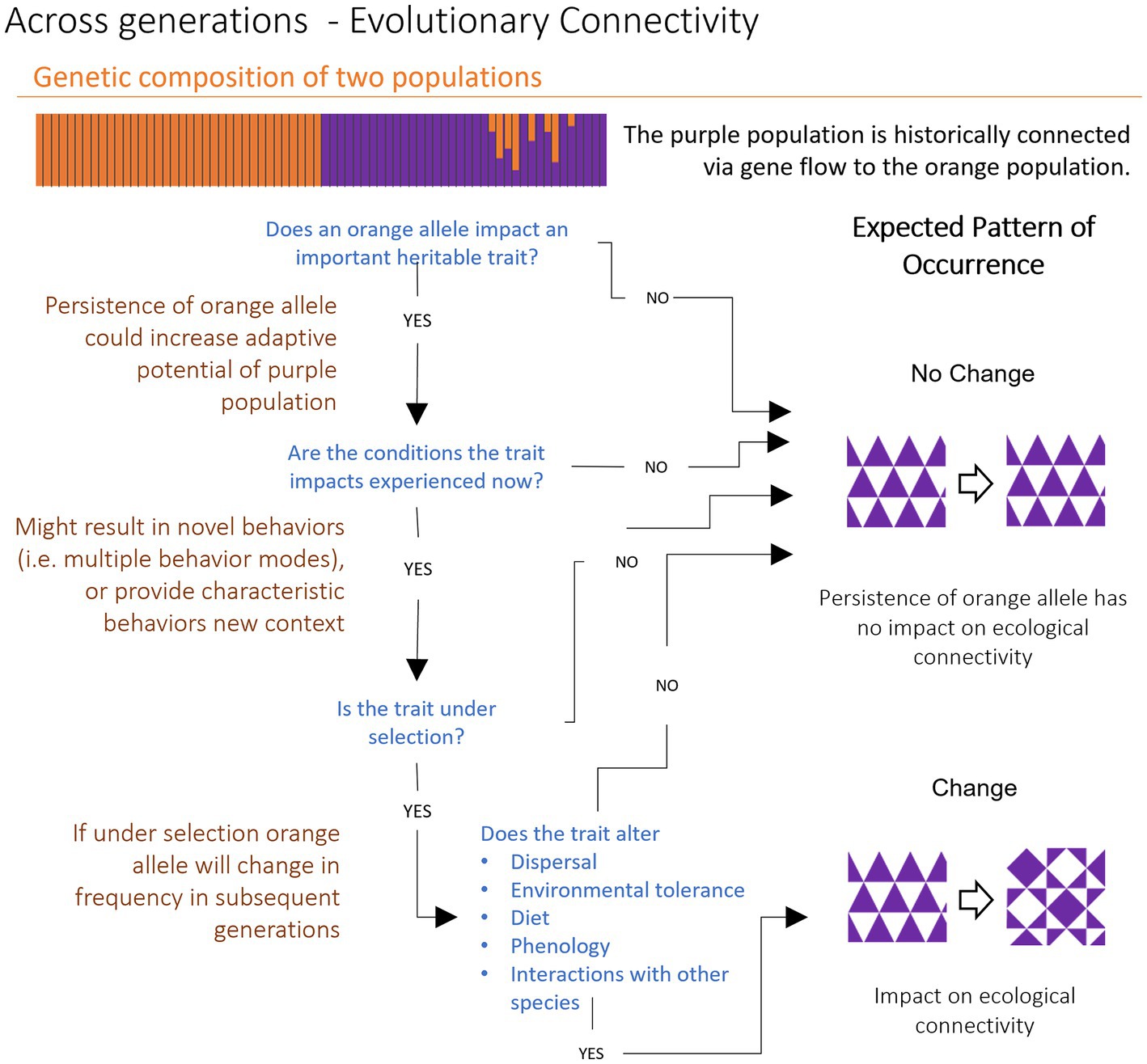
Figure 3. Impacts of evolutionary connectivity on ecological connectivity via a trait’s influence on occurrence. Population structure plot (large horizontal bar) of two populations, purple and orange. Each vertical bar represents an individual organism. The colors of the bars represent the proportion of an individual’s ancestry assigned to each of the two putative populations. There is an orange population on the left and a purple population on the right, where some individuals have mixed parentage (admixed) due to historical gene flow. To understand how evolutionary connectivity impacts ecological connectivity, it is necessary to look at the drivers of trait persistence. Historical gene flow between populations may not impact ecological connectivity, despite the persistence of admixed alleles. Historical connectivity can only alter present ecological connectivity if alleles are (1) changing in frequency and (2) the alleles impact traits that alter occurrence or other ecological interactions.
Like the mating pool in Figure 2, ecological connectivity reflects changes in the distribution of organisms in space and time (i.e., occurrence). Evolutionary connectivity that alters occurrence patterns within the present generation alters a species’ ecological connectivity. Of course, what precisely counts as the “present” generation depends on a species’ life history and timescale. Many species have overlapping generations, so evolutionary connectivity may alter occurrence patterns for a species’ offspring, which could then have consequences on the pattern of occurrence for older individuals in the population. It is important to remember that both ecological and evolutionary connectivity occur at the population level; individual patterns of occurrence or isolated hybridization do not, by themselves, indicate meaningful connections between populations.
4. The generational framework in action: connectivity in summer flounder, Paralichthys dentatus
In general, our generational framework aims to (1) explicitly identify the timescale of a research question, (2) identify data sources or points of view relevant to the research question, and (3) incorporate inferences gained from other conceptions of connectivity, while placing research findings within broader ecological and/or evolutionary contexts. As an example, we will use a research question focused on the population connectivity of summer flounder, Paralichthys dentatus.
4.1. Research question
Our research question is, “How connected are populations of summer flounder along the eastern seaboard of the United States?” From an evolutionary standpoint, genetic sequencing data will allow us to infer if sampled summer flounder come from the same population based on genetic differentiation (2+ generation timescale). Inferences from other generational timescales will let us understand how a population(s) uses and shares space (within generation timescale) and which parts of a population are successful at reproducing (short term, one generation timescale).
4.2. Background and summer flounder connectivity at different timescales
4.2.1. Connectivity within a generation
Summer flounder exhibit seasonal onshore–offshore movements and homing related to spawning (O’Brien et al., 1993, Packer, 1999). To understand these dynamics, we can look to a study by Sackett et al. (2007). They used ultrasonic telemetry tags from 2003 to 2005 to track juvenile and adult flounder movements in and out of the Mullica River–Great Bay estuary, in combination with 7 years of inner continental shelf surveys. They found that the majority of 70 tagged fish emigrated from the estuary July–September, but that the pattern varied between years. Some individuals immigrated/emigrated multiple times within a single season – returning to the estuary through December, before leaving again. Egress appeared to be associated with storm events and decreased dissolved oxygen. A proportion of tagged fish exhibited homing behavior (39% in the second year) and returned to the estuary March–June, providing further evidence that a significant portion of summer flounder home to their larval estuaries (green circle, Figure 4). This study examined aspects of ecological connectivity, as it was concerned with movement patterns and habitat use.
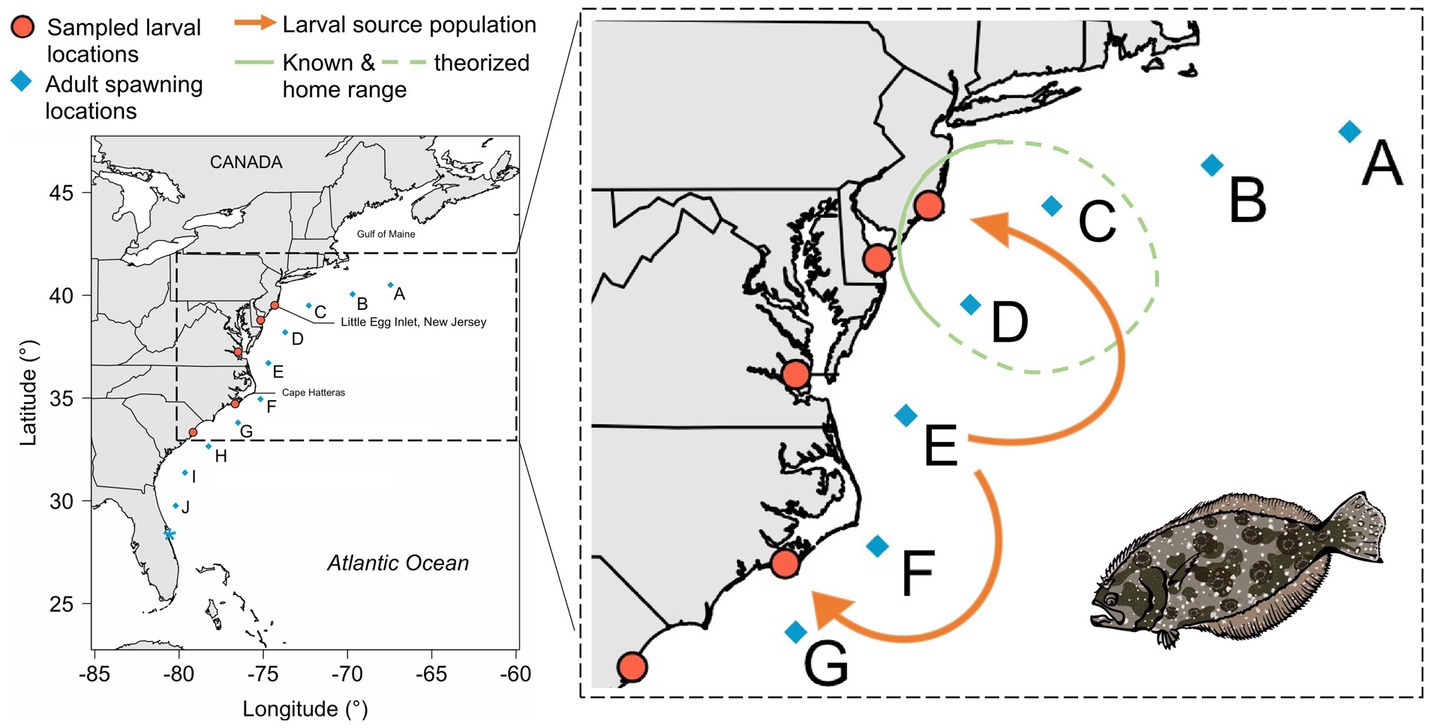
Figure 4. Synthesis of connectivity information for summer flounder. Insights from single generation and short term generational studies which contextualize panmixia. Orange-red dots and blue diamonds indicate larval and adult sampling locations, respectively (Hoey and Pinsky 2018, Hoey et al., 2020). Orange arrows indicate source location (E) of inshore larval flounder samples. Green circle indicates known and theorized home range, as determined from acoustic telemetry in Sackett et al. (2007).
4.2.2. Connectivity between parent and offspring
Seasonal movements from summer foraging grounds to winter breeding locations and homing in summer flounder could result in restricted dispersal. Although not truly between parent and offspring, Hoey et al. (2020) used SNPs and otolith microchemistry to reconstruct larval dispersal patterns through time. They used archived collections of larval summer flounder (n = 411) captured between 1989 and 2012 from five locations along the U.S. eastern seaboard (Figure 4). Combining inferences from two kinds of natural tags – multilocus genotypes and otolith microchemistry – they identified clusters of larvae (n = 3–33 larvae per cluster) with the same natal chemical signature at the otolith core, which allowed larval groups to be assigned to a source location (blue diamonds, Figure 4). The majority of summer flounder larvae (141 of 151; 93%) likely originated near the Cape Hatteras biogeographical break (E in Figure 4), and larvae appeared to be transported in both directions across this break (orange arrows, Figure 4). Hoey et al.’s (2020) results provide evidence for high connectivity in summer flounder populations where larval dispersal between offshore spawning grounds and inshore foraging grounds was considerable and geographically-extensive, and when examined over several generations, sustained over time. This study tackled both evolutionary and ecological connectivity, since it used SNP genetic markers to determine dispersal events and otolith microchemistry to identify intragenerational patterns of movement.
4.2.3. Multigenerational connectivity
Localized dispersal patterns could reflect highly localized breeding populations (i.e., subpopulations). To understand the number of distinct breeding populations in summer flounder, Hoey and Pinsky (2018) used genetic data (1,137 SNPs) from 232 adult summer flounder captured across much of the species range between Massachusetts and Florida, USA. They found that summer flounder are essentially a panmictic, or unstructured, single population. The probability of dispersal across the putative biogeographic barrier of Cape Hatteras, North Carolina was found to be high, and there was no evidence of isolation by distance across the study area. Although these lines of evidence suggest that summer flounder do not have distinct populations along the U.S. eastern seaboard (Hoey and Pinsky, 2018), a small number of loci were found to be associated with four environmental variables: distance along the coast, depth of sampling location, bottom temperature, and bottom salinity. Most of these gene regions (11 of 15) were correlated with bottom temperature. These findings suggest that spatially divergent environmental selection can result in adaptive divergence on regional scales, despite high levels of dispersal. This study focused on evolutionary connectivity since it looked for broad scale patterns in shared genetic material.
4.3. Synthesis using the generational connectivity framework
When interpreted independently, the studies described above provide informative snapshots of connectivity clearly connected to specific timescales and concepts. Synthesizing within our generational framework can further contextualize these findings, enabling a holistic understanding of the ecological and evolutionary connectivity in summer flounder.
4.3.1. Ecological connectivity within the context of evolutionary connectivity
On ecological timescales, tagging and survey data (within a generation) show that summer flounder “home” to specific estuaries. Thus, it might be expected that this kind of movement pattern would manifest in detectable genetic population structure on evolutionary timescales. However, intergenerational tags and population genetic data (one generation and 2+ generations) support widespread larval dispersal and a single, well-mixed adult population in summer flounder. How might we reconcile these seemingly contradictory results? By applying our generation framework and considering the timescale of connectivity, we argue that results can be better contextualized. In this example, homing behavior shows that flounder do have localized populations, at least spatially and within a generation. However, since larvae are not produced in the estuaries, but rather on the continental shelf, this would imply that flounder site fidelity to their estuaries must develop after larvae reach coastal habitats, perhaps in response to environmental filtering, as implied by Hoey and Pinsky (2018). By keeping evolutionary connectivity results in mind when interpreting connectivity on ecological timescales, we can better understand the interactions and processes occurring in a natural population and develop directions for follow up research.
4.3.2. Evolutionary connectivity within the context of ecological connectivity
When considering connectivity on evolutionary timescales, all the sampled adults in Hoey and Pinsky (2018) came from the same genetic population despite being sampled across a wide geographic area. However, genetic data used to investigate connectivity on ecological timescales suggest that most larvae originated from a particular offshore spawning region (Hoey et al., 2020). More specifically, spatially distinct patterns of variation for some genomic regions show that the majority of larvae sampled within estuaries along the U.S. East Coast likely came from the same region in the center of the species range – location E in Figure 4. When connectivity data on evolutionary and ecological timescales are considered together, these findings could indicate that adults mostly mate at the same location (E in Figure 4) or that spawning is widespread along the coast but only individuals spawning in particular locations contribute to the next generation (i.e., portfolio effect). The latter could occur if settling larvae are filtered out by environmental selection, due to factors such as oceanographic conditions, or if behavioral differences alter the timing of spawning. These findings could also be indicative of a form of “bet hedging,” where there is drastically differential survivorship from year to year and the majority of the population stems from a specific subset of locations most of the time, but “sink” populations may become successful when conditions change. By interpreting connectivity findings within our generational framework, we are better able to contextualize the biology of summer flounder.
4.3.3. Connectivity in both ecology and evolution
By considering connectivity along the generational timescale, these studies imply that either summer flounder generally mate in the same location somewhere offshore (E in Figure 4), effectively making each local estuary a branch with a single source, or summer flounder mate up and down the coast, operating as distinct subpopulations ecologically, yet environmental conditions favor specific mating groups over others, which then act as sources for the wider population on an evolutionary timescale. To differentiate between these two hypotheses, a next step would require ecological connectivity information within a single generation. Occurrence data, from approaches such as mark and recapture, would help to determine where adult summer flounder mate and which estuaries they come from.
5. Conclusion
Identifying the timescale of our inferences involving connectivity allows us to place our findings more clearly within the context of evolutionary and/or ecological processes. The generational framework introduced here serves as a tool to synthesize information about population connectivity. Understanding any natural process requires multiple points of view and no single definition of connectivity will adequately encompass the entirety of any complex natural system. Given this, our framework provides a structure to help researchers collaborate across subdisciplines without inventing new terminology. By allowing population connectivity to have context-specific meaning and focusing on the timescales of connectivity processes, it becomes easier to see which types of data and points of view are relevant for particular research questions.
Like any model, this framework serves as a starting place rather than an end point for understanding populations. Species interactions are complex. Conspecifics within the same population may have unexpected interactions (i.e., predation), challenging assumptions about ecological interactions and their evolutionary connectivity outcomes. For most species, multiple generations exist simultaneously, so the distinct “one generation” and “2+ generation” categories are not always clear. Ecological interactions between different generations may have complex evolutionary outcomes and vice versa. Investigating how discrete or overlapping generational structures may alter these inferences is one potential application of this generational framework.
While the framework discussed here is clearly grounded in population connectivity, population-level connections are not the only types of connections between organisms. Within ecology, connectivity can also refer to abiotic ecosystem components, including the transport of matter or energy within and between habitats (Pringle, 2001; Hillman et al., 2018; Hilty et al., 2020; Keeley et al., 2022). An oft-cited example of this is the influx of marine-derived nitrogen to upland riparian streams during salmon spawning (Helfield and Naiman, 2001). Many species coevolved with this seasonal influx of nutrients and shifts in salmon biomass have widespread ecological consequences (Carlson et al., 2011). The generational framework does not explicitly include these ecosystem connections, however, inferences from such studies might be incorporated if they were clearly assigned a particular timescale.
Only by integrating across many fields of study can we gain a holistic understanding of connectivity within natural systems. Although studies of population connectivity from multiple perspectives are rare (Cooke et al., 2008), the few studies that do exist have yielded important insights. Cross disciplinary collaborations can be challenging, but successful collaborations start with a shared set of ideas and goals (Knapp et al., 2015). We hope the generational framework presented here can serve as a foundation for conducting and communicating population connectivity research across subdisciplines.
Author contributions
AC, JH, TD, RG, JT, JC, EP, JG, and RB conceived and designed the manuscript content and scope. AC, JH, TD, RG, JC, and JT performed background research and framing. AC, JH, TD, RG, and JT wrote the manuscript. JG, EP, RB, TD, RG, and JT provided critical reviews. AC, JH, TD, and RG edited the manuscript. JG, EP, and RB supervised the project. All authors contributed to the article and approved the submitted version.
Funding
This project was funded by the Research Coordination Network for Evolution in Changing Seas (NSF-OCE 1764316).
Acknowledgments
We thank Peter Dudley and Joanna Kelley for initial feedback on the manuscript. We are grateful for the helpful feedback provided by the two reviewers and Michael Anteau. We also thank Abby Poray for designing the summer flounder graphic used in Figure 4.
Conflict of interest
The authors declare that the research was conducted in the absence of any commercial or financial relationships that could be construed as a potential conflict of interest.
Publisher’s note
All claims expressed in this article are solely those of the authors and do not necessarily represent those of their affiliated organizations, or those of the publisher, the editors and the reviewers. Any product that may be evaluated in this article, or claim that may be made by its manufacturer, is not guaranteed or endorsed by the publisher.
References
Abadía-Cardoso, A., Freimer, N. B., Deiner, K., and Garza, J. C. (2017). Molecular population genetics of the northern elephant seal Mirounga angustirostris. J. Hered. 108, 618–627. doi: 10.1093/jhered/esx053
Albrecht, M., and Gotelli, N. J. (2001). Spatial and temporal niche partitioning in grassland ants. Oecologia 126, 134–141. doi: 10.1007/s004420000494
Allendorf, F. W. (2017). Genetics and the conservation of natural populations: allozymes to genomes. Mol. Ecol. 26, 420–430. doi: 10.1111/mec.13948
Allendorf, F. W., Leary, R. F., Spruell, P., and Wenburg, J. K. (2001). The problems with hybrids: setting conservation guidelines. Trends Ecol. Evol. 16, 613–622. doi: 10.1016/S0169-5347(01)02290-X
Allendorf, F. W., Luikart, G., and Aitken, S. N. (2013). Conservation and the genetics of populations (2nd ed) Hoboken, NJ: John Wiley & Sons.
Azarian, C., Foster, S., Devloo-Delva, F., and Feutry, P. (2021). Population differentiation from environmental DNA: investigating the potential of haplotype presence/absence-based analysis of molecular variance. Environ. DNA 3, 541–552. doi: 10.1002/edn3.143
Allendorf, F. W., and Phelps, S. R. (1981). Use of allelic frequencies to describe population structure. Canadian Journal of Fisheries and Aquatic Sciences 38, 1507–1514. doi: 10.1139/f81-203
Bain, D. (1990). Examining the validity of inferences drawn from photo-identification data, with special reference to studies of the killer whale Orcinus orca in British Columbia. Rep. Int. Whaling Comm. 12, 93–100.
Benzie, J. A. H., and Ballment, E. (1994). Genetic differences among black-lipped pearl oyster (Pinctada margaritifera) populations in the western Pacific. Aquaculture 127, 145–156. doi: 10.1016/0044-8486(94)90421-9
Bierne, N., Gagnaire, P.-A., and David, P. (2013). The geography of introgression in a patchy environment and the thorn in the side of ecological speciation. Curr. Zoo. 59, 72–86. doi: 10.1093/czoolo/59.1.72
Børsting, C., and Morling, N. (2013). “Single-nucleotide polymorphisms” in Encyclopedia of forensic sciences eds. Jay A. Siegel, Pekka J. Saukko, Max M. Houc (Amsterdam: Elsevier), 233–238.
Brown, W. L., and Wilson, E. O. (1956). Character displacement. Syst. Zool. 5, 49–64. doi: 10.2307/2411924
Burton, A. C., Neilson, E., Moreira, D., Ladle, A., Steenweg, R., Fisher, J. T., et al. (2015). REVIEW: wildlife camera trapping: a review and recommendations for linking surveys to ecological processes. J. Appl. Ecol. 52, 675–685. doi: 10.1111/1365-2664.12432
Cadrin, S. X., Karr, L. A., and Mariani, S. (2014). “Chapter one - stock identification methods: an overview” in Stock identification methods. eds. S. X. Cadrin, L. A. Kerr, and S. Mariani. Second ed (Cambridge, MA: Academic Press), 1–5.
Cagnacci, F., Boitani, L., Powell, R. A., and Boyce, M. S. (2010). Animal ecology meets GPS-based radiotelemetry: A perfect storm of opportunities and challenges. Philos. Trans. R. Soc. B, Biol. Sci. 365, 2157–2162. doi: 10.1098/rstb.2010.0107
Calabrese, J. M., and Fagan, W. F. (2004). A comparison-shopper’s guide to connectivity metrics. Frontiers in Ecology and the Environment 2, 529–536. doi: 10.2307/3868383
Carlson, S. M., Quinn, T. P., and Hendry, A. P. (2011). Eco-evolutionary dynamics in Pacific salmon. Heredity 106, 438–447. doi: 10.1038/hdy.2010.163
Carolan, M. S. (2008). Is it a distinct subspecies? Preble’s mouse and the “best available science” mandate of the endangered species act. Soc. Nat. Resour. 21, 944–951. doi: 10.1080/08941920701681979
Carvalho, G. R., and Hauser, L. (1994). Molecular genetics and the stock concept in fisheries. Rev. Fish Biol. Fish. 4, 326–350. doi: 10.1007/BF00042908
Cooke, S. J., Hinch, S. G., Farrell, A. P., Patterson, D. A., Miller-Saunders, K., Welch, D. W., et al. (2008). Developing a mechanistic understanding of fish migrations by linking telemetry with physiology, behavior, genomics and experimental biology: an interdisciplinary case study on adult fraser river sockeye salmon. Fisheries 33, 321–339. doi: 10.1577/1548-8446-33.7.321
Cowen, R. K., and Sponaugle, S. (2009). Larval dispersal and marine population connectivity. Annu. Rev. Mar. Sci. 1, 443–466. doi: 10.1146/annurev.marine.010908.163757
Cowen, R. K., Lwiza, K. M. M., Sponaugle, S., Paris, C. B., and Olson, D. B. (2000). Connectivity of marine populations: open or closed? Science 287, 857–859. doi: 10.1126/science.287.5454.857
Cowen, R. K., Paris, C. B., and Srinivasan, A. (2006).Scaling of connectivity in marine populations. Science 311, 522–527. doi:doi: 10.1126/science.1122039
Crifasi, R. R. (2007). A subspecies no more? A mouse, its unstable taxonomy, and western riparian resource conflict. Cult. Geogr. 14, 511–535. doi: 10.1177/1474474007082292
Crossin, G. T., Heupel, M. R., Holbrook, C. M., Hussey, N. E., Lowerre-Barbieri, S. K., Nguyen, V. M., et al. (2017). Acoustic telemetry and fisheries management. Ecol. Appl. 27, 1031–1049. doi: 10.1002/eap.1533
D’Aloia, C. C., Andrés, J. A., Bogdanowicz, S. M., McCune, A. R., Harrison, R. G., and Buston, P. M. (2020). Unraveling hierarchical genetic structure in a marine metapopulation: a comparison of three high-throughput genotyping approaches. Mol. Ecol. 29, 2189–2203. doi: 10.1111/mec.15405
DeCelles, G. R., and Cadrin, S. X. (2010). Movement patterns of winter flounder (Pseudopleuronectes americanus) in the Southern Gulf of Maine: observations with the use of passive acoustic telemetry. Fish. Bull. 108, 408–419.
Dewulf, A., François, G., Pahl-Wostl, C., and Taillieu, T. (2007). A framing approach to cross-disciplinary research collaboration: experiences from a large-scale research project on adaptive water management. Ecol. Soc. 12. doi: 10.5751/ES-02142-120214
Doherty, P. J., Planes, S., and Mather, P. (1995). Gene flow and larval duration in seven species of fish from the great barrier reef. Ecology 76, 2373–2391. doi: 10.2307/2265814
Dorant, Y., Benestan, L., Rougemont, Q., Normandeau, E., Boyle, B., Rochette, R., et al. (2019). Comparing Pool-seq, rapture, and GBS genotyping for inferring weak population structure: the American lobster (Homarus americanus) as a case study. Ecol. Evol. 9, 6606–6623. doi: 10.1002/ece3.5240
Fang, X., Hou, X., Li, X., Hou, W., Nakaoka, M., and Yu, X. (2018). Ecological connectivity between land and sea: A review. Ecol. Res. 33, 51–61. doi: 10.1007/s11284-017-1549-x
Fontoura, L., D’Agata, S., Gamoyo, M., Barneche, D. R., Luiz, O. J., Madin, E. M. P., et al. (2022). Protecting connectivity promotes successful biodiversity and fisheries conservation. Science 375, 336–340. doi: 10.1126/science.abg4351
Forester, B. R., Beever, E. A., Darst, C., Szymanski, J., and Funk, W. C. (2022). Linking evolutionary potential to extinction risk: applications and future directions. Front. Ecol. Environ. 20, 507–515. doi: 10.1002/fee.2552
Frisk, M. G., Jordaan, A., and Miller, T. J. (2014). Moving beyond the current paradigm in marine population connectivity: are adults the missing link? Fish Fish. 15, 242–254. doi: 10.1111/faf.12014
Funk, W. C., Blouin, M. S., Corn, P. S., Maxell, B. A., Pilliod, D. S., Amish, S., et al. (2005). Population structure of Columbia spotted frogs (Rana luteiventris) is strongly affected by the landscape. Mol. Ecol. 14, 483–496. doi: 10.1111/j.1365-294X.2005.02426.x
Gilmour, J. S. L., and Heslop-Harrison, J. (1954). The deme terminology and the units of micro-evolutionary change. Genetica 27, 147–161.
Green, D. G. (1994). Connectivity and complexity in landscapes and ecosystems. Pac. Conserv. Biol. 1, 194–200. doi: 10.1071/PC940194
Griffiths, A. J. F. (2005). Introduction to genetic analysis. 8th Edn New York, NY: W.H. Freeman and Co.
Habel, J. C., Zachos, F. E., Dapporto, L., Rödder, D., Radespiel, U., Tellier, A., et al. (2015). Population genetics revisited – towards a multidisciplinary research field. Biol. J. Linn. Soc. 115, 1–12. doi: 10.1111/bij.12481
Hampton, S. E. (2004). Habitat overlap of enemies: temporal patterns and the role of spatial complexity. Oecologia 138, 475–484. doi: 10.1007/s00442-003-1446-6
Hanksi, I. (1994). A practical model of metapopulation dynamics. J. Anim. Ecol. 63, 151–162. doi: 10.2307/5591
Hanski, I. (2011). Eco-evolutionary spatial dynamics in the Glanville fritillary butterfly. Proc. Natl. Acad. Sci. 108, 14397–14404. doi: 10.1073/pnas.1110020108
Hanski, I., and Gaggiotti, O., eds. (2004). Ecology, genetics, and evolution of Metapopulations. Oxford, UK: Academic Press.
Harding, K. C., and McNamara, J. M. (2002). A unifying framework for metapopulation dynamics. Am. Nat. 160, 173–185. doi: 10.1086/341014
Hare, M. P., Nunney, L., Schwartz, M. K., Ruzzante, D. E., Burford, M., Waples, R. S., et al. (2011). Understanding and estimating effective population size for practical application in marine species management. Conserv. Biol. 25, 438–449. doi: 10.1111/j.1523-1739.2010.01637.x
Harrison, H. B., Bode, M., Williamson, D. H., Berumen, M. L., and Jones, G. P. (2020). A connectivity portfolio effect stabilizes marine reserve performance. Proc. Natl. Acad. Sci. 117, 25595–25600. doi: 10.1073/pnas.1920580117
Hart, A. G., Dawson, M., Fourie, R., MacTavish, L., and Goodenough, A. E. (2022). Comparing the effectiveness of camera trapping, driven transects and ad hoc records for surveying nocturnal mammals against a known species assemblage. Commun. Ecol. 23, 27–39. doi: 10.1007/s42974-021-00070-7
Hastings, A., and Harrison, S. (1994). Metapopulation dynamics and genetics. Annu. Rev. Ecol. Syst. 25, 167–188. doi: 10.1146/annurev.es.25.110194.001123
Hedgecock, D., Nelson, K., and Lopez-Lemus, L. G. (1988). Biochemical genetic and morphological divergence among three species of thread herring (Opisthonema) in northwest México. CalCOFI Reports 29, 110–121.
Hedgecock, D., Barber, P. H., and Edmands, S. (2007). Genetic approaches to measuring connectivity. Oceanography 20, 70–79. doi: 10.5670/oceanog.2007.30
Hedrick, P. W., Ginevan, M. E., and Ewing, E. P. (1976). Genetic polymorphism in heterogeneous environments. Annu. Rev. Ecol. Evol. Syst. 7, 1–32. doi: 10.1146/annurev.es.07.110176.000245
Helfield, J. M., and Naiman, R. J. (2001). Effects of salmon-derived nitrogen on riparian forest growth and implications for stream productivity. Ecology 82, 2403–2409. doi: 10.1890/0012-9658(2001)082[2403:EOSDNO]2.0.CO;2
Heupel, M., Semmens, J., and Hobday, A. (2006). Automated acoustic tracking of aquatic animals: scales, design and development of listening station arrays. Mar. Freshw. Res. 57:1. doi: 10.1071/MF05091
Heupel, M. R., and Webber, D. M. (2012). Trends in acoustic tracking: where are the fish going and how will we follow them? Am. Fish. Soc. Symp., 76, 219–231. doi: 10.47886/9781934874271.ch15
Hill, W. G. (1981). Estimation of effective population size from data on linkage disequilibrium. Genet. Res. 38, 209–216. doi: 10.1017/S0016672300020553
Hillman, J. R., Lundquist, C. J., and Thrush, S. F. (2018). The challenges associated with connectivity in ecosystem processes. Front. Mar. Sci. 5:364. doi: 10.3389/fmars.2018.00364
Hilty, J., Worboys, G. L., Keeley, A., Woodley, S., Lausche, B. J., Locke, H., et al. (2020). Guidelines for conserving connectivity through ecological networks and corridors. IUCN xiv:122. doi: 10.2305/IUCN.CH.2020.PAG.30.en
Hodel, R. G. J., Segovia-Salcedo, M. C., Landis, J. B., Crowl, A. A., Sun, M., Liu, X., et al. (2016). The report of my death was an exaggeration: A review for researchers using microsatellites in the 21st century. Appl. Plant Sci. 4:1600025. doi: 10.3732/apps.1600025
Hoelzel, A., Halley, J., O'Brien, S. J., Campagna, C., Arnborm, T., Le Boeuf, B., et al. (1993). Elephant seal genetic variation and the use of simulation models to investigate historical population bottlenecks. J. Hered. 84, 443–449. doi: 10.1093/oxfordjournals.jhered.a111370
Hoey, J. A., Fodrie, F. J., Walker, Q. A., Hilton, E. J., Kellison, G. T., Targett, T. E., et al. (2020). Using multiple natural tags provides evidence for extensive larval dispersal across space and through time in summer flounder. Mol. Ecol. 29, 1421–1435. doi: 10.1111/mec.15414
Hoey, J. A., and Pinsky, M. L. (2018). Genomic signatures of environmental selection despite near-panmixia in summer flounder. Evol. Appl. 11, 1732–1747. doi: 10.1111/eva.12676
Holsinger, K. E., and Weir, B. S. (2009). Genetics in geographically structured populations: defining, estimating and interpreting FST. Nat. Rev. Genet. 10, 639–650. doi: 10.1038/nrg2611
Holt, R. D., and Gomulkiewicz, R. (1997). How does immigration influence local adaptation? A reexamination of a familiar paradigm. Am. Nat. 149, 563–572. doi: 10.1086/286005
Hussey, N. E., Kessel, S. T., Aarestrup, K., Cooke, S. J., Cowley, P. D., Fisk, A. T., et al. (2015). Aquatic animal telemetry: A panoramic window into the underwater world. Science 348:1255642. doi: 10.1126/science.1255642
Jenkins, T. L., and Stevens, J. R. (2018). Assessing connectivity between MPAs: Selecting taxa and translating genetic data to inform policy. Marine Policy 94, 165–173. doi: 10.1016/j.marpol.2018.04.022
Jones, M. R., Mills, L. S., Alves, P. C., Callahan, C. M., Alves, J. M., Lafferty, D. J. R., et al. (2018). Adaptive introgression underlies polymorphic seasonal camouflage in snowshoe hares. Science 360, 1355–1358. doi: 10.1126/science.aar5273
Kawecki, T. J., and Ebert, D. (2004). Conceptual issues in local adaptation. Ecol. Lett. 7, 1225–1241. doi: 10.1111/j.1461-0248.2004.00684.x
Kays, R., Crofoot, M. C., Jetz, W., and Wikelski, M. (2015). Terrestrial animal tracking as an eye on life and planet. Science 348:aaa2478. doi: 10.1126/science.aaa2478
Keeley, A. T. H., Fremier, A. K., Goertler, P. A. L., Huber, P. R., Sturrock, A. M., Bashevkin, S. M., et al. (2022). Governing ecological connectivity in cross-scale dependent systems. Bioscience 72, 372–386. doi: 10.1093/biosci/biab140
Kerr, L. A., Hintzen, N. T., Cadrin, S. X., Clausen, L. W., Dickey-Collas, M., Goethel, D. R., et al. (2017). Lessons learned from practical approaches to reconcile mismatches between biological population structure and stock units of marine fish. ICES J. Mar. Sci. 74, 1708–1722. doi: 10.1093/icesjms/fsw188
Knapp, B., Bardenet, R., Bernabeu, M. O., Bordas, R., Bruna, M., Calderhead, B., et al. (2015). Ten simple rules for a successful cross-disciplinary collaboration. PLoS Comput. Biol. 11:e1004214. doi: 10.1371/journal.pcbi.1004214
Kool, J. T., Moilanen, A., and Treml, E. A. (2013). Population connectivity: recent advances and new perspectives. Landsc. Ecol. 28, 165–185. doi: 10.1007/s10980-012-9819-z
LeBoeuf, B. J., and Reiter, J. (1988). “Life time reproductive success in the northern elephant seal” in Reproductive success. ed. H. T. Clutton-Brock (Chicago (IL): University of Chicago Press), 344–362.
Lehnen, S. E., Sternberg, M. A., Swarts, H. M., and Sesnie, S. E. (2021). Evaluating population connectivity and targeting conservation action for an endangered cat. Ecosphere 12:e03367. doi: 10.1002/ecs2.3367
Levin, S. A., and Paine, R. T. (1974). Disturbance, Patch Formation, and Community Structure. Proceedings of the National Academy of Sciences 71, 2744–2747. doi: 10.1073/pnas.71.7.2744
Levin, S. A. (1976). Population dynamic models in heterogeneous environments. Annu. Rev. Ecol. Syst. 7, 287–310. doi: 10.1146/annurev.es.07.110176.001443
Levin, P. S., Gray, S. A., Möllmann, C., and Stier, A. C. (2021). Perception and conflict in conservation: the rashomon effect. Bioscience 71, 64–72. doi: 10.1093/biosci/biaa117
Levins, R. (1969). Some demographic and genetic consequences of environmental heterogeneity for biological control. Bull. Entomol. Soc. Am. 15, 237–240. doi: 10.1093/besa/15.3.237
Lipcius, R. N., Eggleston, D. B., Schreiber, S. J., Seitz, R. D., Shen, J., Sisson, M., et al. (2008). Importance of metapopulation connectivity to restocking and restoration of marine species. Rev. Fish. Sci. 16, 101–110. doi: 10.1080/10641260701812574
Lowe, W. H., and Allendorf, F. W. (2010). What can genetics tell us about population connectivity? Mol. Ecol. 19, 3038–3051. doi: 10.1111/j.1365-294X.2010.04688.x
Lowerre-Barbieri, S. K., Kays, R., Thorson, J. T., and Wikelski, M. (2019). The ocean’s movescape: fisheries management in the bio-logging decade (2018–2028). ICES J. Mar. Sci. 76, 477–488. doi: 10.1093/icesjms/fsy211
Manel, S., Gaggiotti, O. E., and Waples, R. S. (2005). Assignment methods: matching biological questions with appropriate techniques. Trends Ecol. Evol. 20, 136–142. doi: 10.1016/j.tree.2004.12.004
Mariani, S., and Bekkevold, D. (2014). “Chapter fourteen - the nuclear genome: neutral and adaptive markers in fisheries science” in Stock identification methods. eds. S. X. Cadrin, L. A. Kerr, and S. Mariani. Second ed (Cambridge, MA: Academic Press), 297–327.
Marques, D. A., Meier, J. I., and Seehausen, O. (2019). A combinatorial view on speciation and adaptive radiation. Trends Ecol. Evol. 34, 531–544. doi: 10.1016/j.tree.2019.02.008
McMahon, K. W., Hamady, L. L., and Thorrold, S. R. (2013). A review of ecogeochemistry approaches to estimating movements of marine animals. Limnol. Oceanogr. 58, 697–714. doi: 10.4319/lo.2013.58.2.0697
Merriam, G. (1984). “Connectivity: a fundamental ecological characteristic of landscape pattern” in Proc. first international seminar on methodology in landscape ecological research and planning. eds. J. Brandt and P. Agger, vol. 1 (Roskilde, Denmark: Roskilde Universitetsforlag GeoRuc), 5–15.
Mills, L. S., and Allendorf, F. W. (1996). The one-migrant-per-generation rule in conservation and management. Conserv. Biol. 10, 1509–1518. doi: 10.1046/j.1523-1739.1996.10061509.x
Moilanen, A., and Hanski, I. (2001). On the use of connectivity measures in spatial ecology. Oikos 95, 147–151. doi: 10.1034/j.1600-0706.2001.950116.x
Moilanen, A., and Nieminen, M. (2002). Simple connectivity measures in spatial ecology. Ecology 83, 1131–1145. doi: 10.1890/0012-9658(2002)083[1131:SCMISE]2.0.CO;2
Muhlfeld, C. C., Kovach, R. P., Al-Chokhachy, R., Amish, S. J., Kershner, J. L., Leary, R. F., et al. (2017). Legacy introductions and climatic variation explain spatiotemporal patterns of invasive hybridization in a native trout. Glob. Chang. Biol. 23, 4663–4674. doi: 10.1111/gcb.13681
O’Brien, L., Burnett, J., and Mayo, R. K. (1993). Maturation of Nineteen Species of Finfish off the Northeast Coast of the United States, 1985–1990. Available at: http://aquaticcommons.org/id/eprint/2702, https://aquadocs.org/handle/1834/20494 (Accessed January 2021)
Oziolor, E. M., Reid, N. M., Yair, S., Lee, K. M., VerPloeg, S. G., Bruns, P. C., et al. (2019). Adaptive introgression enables evolutionary rescue from extreme environmental pollution. Science 364, 455–457. doi: 10.1126/science.aav4155
Packer, D. (1999). Essential fish habitat source document. Summer flounder, Paralichthys dentatus, life history and habitat characteristics (NOAA technical memorandum NMFS-NE-151; essential fish habitat source document). National Oceanic and Atmospheric Administration. Available at: https://www.semanticscholar.org/paper/Essential-fish-habitat-source-document.-Summer-life-Packer/386be3a0a4b8b96a3edf42b99ac7b8dc4fdd9d32 (Accessed September 2019)
Palsbøll, P. J., Bérubé, M., and Allendorf, F. W. (2007). Identification of management units using population genetic data. Trends in ecology & evolution 22, 11–16. doi: 10.1016/j.tree.2006.09.003
Parker, S. P. (Ed.) (1989). McGraw-Hill dictionary of scientific and technical terms. 4th Edn New York, NY: McGraw-Hill Book Co.
Pennington, D. D. (2008). Cross-disciplinary collaboration and learning. Ecol. Soc. 13:art8. doi: 10.5751/ES-02520-130208
Polis, G. A., Anderson, W. B., and Holt, R. D. (1997). Toward an integration of landscape and food web ecology: the dynamics of spatially subsidized food webs. Annu. Rev. Ecol. Syst. 28, 289–316. doi: 10.1146/annurev.ecolsys.28.1.289
Pringle, C. M. (2001). Hydrologic connectivity and the management of biological reserves: a global perspective. Ecol. Appl. 11, 981–998. doi: 10.1890/1051-0761(2001)011[0981:HCATMO]2.0.CO;2
Reddingius, J., and den Boer, P. J. (1970). Simulation experiments illustrating stabilization of animal numbers by spreading of risk. Oecologia 5, 240–284. doi: 10.1007/BF00344886
Reid, K., Palkovacs, E. P., Hasselman, D. J., Baetscher, D., Kibele, J., Gahagan, B., et al. (2018). Comprehensive evaluation of genetic population structure for anadromous river herring with single nucleotide polymorphism data. Fish. Res. 206, 247–258. doi: 10.1016/j.fishres.2018.04.014
Rieseberg, L. H., Raymond, O., Rosenthal, D. M., Lai, Z., Livingstone, K., Nakazato, T., et al. (2003). Major ecological transitions in wild sunflowers facilitated by hybridization. Science 301, 1211–1216. doi: 10.1126/science.1086949
Rius, M., and Darling, J. A. (2014). How important is intraspecific genetic admixture to the success of colonising populations? Trends Ecol. Evol. 29, 233–242. doi: 10.1016/j.tree.2014.02.003
Roff, D. A. (1974). The analysis of a population model demonstrating the importance of dispersal in a heterogeneous environment. Oecologia 15, 259–275. doi: 10.1007/BF00345182
Rosenblatt, R. H., and Waples, R. S. (1986). A genetic comparison of allopatric populations of shore fish species from the Eastern and Central Pacific Ocean: Dispersal or Vicariance? Copeia 2, 275–284. doi: 10.2307/1444988
Ruzzante, D. E., Mariani, S., Bekkevold, D., André, C., Mosegaard, H., Clausen, L. A. W., et al. (2006). Biocomplexity in a highly migratory pelagic marine fish, Atlantic herring. Proceedings of the Royal Society B: Biological Sciences 273, 1459–1464. doi: 10.1098/rspb.2005.3463
Roberts, C. M. (1997). Connectivity and management of Caribbean coral reefs. Science 278, 1454–1457.
Secor, D. H. (2005). “Chapter 3 – fish migration and the unit stock: three formative debates” in Stock identification methods (Cambridge, MA: Academic Press), 17–44.
Sackett, D. K., Able, K. W., and Grothues, T. M. (2007). Dynamics of summer flounder, Paralichthys dentatus, seasonal migrations based on ultrasonic telemetry. Estuar. Coast. Shelf Sci. 74, 119–130. doi: 10.1016/j.ecss.2007.03.027
Schmidt, B. R., Kéry, M., Ursenbacher, S., Hyman, O. J., and Collins, J. P. (2013). Site occupancy models in the analysis of environmental DNA presence/absence surveys: A case study of an emerging amphibian pathogen. Methods Ecol. Evol. 4, 646–653. doi: 10.1111/2041-210X.12052
Sheaves, M. (2009). Consequences of ecological connectivity: the coastal ecosystem mosaic. Mar. Ecol. Prog. Ser. 391, 107–115. doi: 10.3354/meps08121
Simberloff, D., and Cox, J. (1987). Consequences and costs of conservation corridors. Conserv. Biol. 1, 63–71. doi: 10.1111/j.1523-1739.1987.tb00010.x
Smith, J. G., Tomoleoni, J., Staedler, M., Lyon, S., Fujii, J., and Tinker, M. T. (2021). Behavioral responses across a mosaic of ecosystem states restructure a sea otter–urchin trophic cascade. Proc. Natl. Acad. Sci. 118:e2012493118. doi: 10.1073/pnas.2012493118
Sommers, P., and Chesson, P. (2019). Effects of predator avoidance behavior on the coexistence of competing prey. Am. Nat. 193, E132–E148. doi: 10.1086/701780
Soulé, M. E., and Simberloff, D. (1986). What do genetics and ecology tell us about the design of nature reserves? Biol. Conserv. 35, 19–40. doi: 10.1016/0006-3207(86)90025-X
Spies, I., and Punt, A. E. (2015). The utility of genetics in marine fisheries management: a simulation study based on Pacific cod off Alaska. Can. J. Fish. Aquat. Sci. 72, 1415–1432. doi: 10.1139/cjfas-2014-0050
Speith, H. T. (1974). Courtship behavior in Drosophila. Annual Review of Entomology 19, 385–405. doi: 10.1146/annurev.en.19.010174.002125
Supp, S. R., Bohrer, G., Fieberg, J., and La Sorte, F. A. (2021). Estimating the movements of terrestrial animal populations using broad-scale occurrence data. Movement Ecology 9:60. doi: 10.1186/s40462-021-00294-2
Shulman, M. J., and Bermingham, E. (1995). Early life histories, ocean currents, and the population genetics of Caribbean reef fishes. Evolution 49, 897–910. doi: 10.2307/2410412
Tao, Y., Hite, J. L., Lafferty, K. D., Earn, D. J. D., and Bharti, N. (2021). Transient disease dynamics across ecological scales. Theor. Ecol. 14, 625–640. doi: 10.1007/s12080-021-00514-w
Taylor, P. D., Fahrig, L., Henein, K., and Merriam, G. (1993). Connectivity is a vital element of landscape structure. Oikos 68, 571–573. doi: 10.2307/3544927
Tischendorf, L., and Fahrig, L. (2000). On the usage and measurement of landscape connectivity. Oikos 90, 7–19. doi: 10.1034/j.1600-0706.2000.900102.x
Turner, S. M., Limburg, K. E., and Palkovacs, E. P. (2015). Can different combinations of natural tags identify river herring natal origin at different levels of stock structure? Can. J. Fish. Aquat. Sci. 72, 845–854. doi: 10.1139/cjfas-2014-0403
UN CMS (2020). R. 12. 26. Improving ways of addressing connectivity in the conservation of migratory species (Convention on the Conservation of Migratory Species of Wild Animals). Available at: https://www.cms.int/sites/default/files/document/cms_cop13_res.12.26_rev.cop13_e.pdf (Accessed January 2022)
Vos, C. C., Berry, P., Opdam, P., Baveco, H., Nijhof, B., O’Hanley, J., et al. (2008). Adapting landscapes to climate change: examples of climate-proof ecosystem networks and priority adaptation zones. J. Appl. Ecol. 45, 1722–1731. doi: 10.1111/j.1365-2664.2008.01569.x
Wang, J., and Whitlock, M. C. (2003). Estimating effective population size and migration rates from genetic samples over space and time. Genetics 163, 429–446. doi: 10.1093/genetics/163.1.429
Waples, R. S. (1991). Pacific Salmon, Oncorhynchus spp., and the definition of “species” under the endangered species act. Mar. Fish. Rev. 53:11.
Waples, R. S., and Gaggiotti, O. (2006). What is a population? An empirical evaluation of some genetic methods for identifying the number of gene pools and their degree of connectivity. Mol. Ecol. 15, 1419–1439. doi: 10.1111/j.1365-294X.2006.02890.x
Webster, M. S., Marra, P. P., Haig, S. M., Bensch, S., and Holmes, R. T. (2002). Links between worlds: unraveling migratory connectivity. Trends Ecol. Evol. 17, 76–83. doi: 10.1016/S0169-5347(01)02380-1
Whitlock, M. C., and McCauley, D. E. (1999). Indirect measures of gene flow and migration: FST not equal to 1/(4Nm+1). Heredity 82, 117–125. doi: 10.1038/sj.hdy.6884960
Winsor, M. P. (2000). Species, demes, and the omega taxonomy: Gilmour and The NewSystematics. Biology & Philosophy 15, 349–388. doi: 10.1023/A:1006774217770
Whitlock, M. C. (2015). Modern approaches to local adaptation. Am. Nat. 186, S1–S4. doi: 10.1086/682933
Wright, S. (1931). Evolution in Mendelian populations. Genetics 16, 97–159. doi: 10.1093/genetics/16.2.97
Wright, S. (1949). The genetical structure of populations. Ann. Hum. Genet. 15, 323–354. doi: 10.1111/j.1469-1809.1949.tb02451.x
Wright, S. (1965). The interpretation of population structure by F-statistics with special regard to systems of mating. Evolution 19, 395–420. doi: 10.2307/2406450
Keywords: connectivity, population, genetic, markers, spatial, tracking, generations
Citation: Cramer AN, Hoey JA, Dolan TE, Gatins R, Toy JA, Chancellor JL, Palkovacs EP, Garza JC and Beltran RS (2023) A unifying framework for understanding ecological and evolutionary population connectivity. Front. Ecol. Evol. 11:1072825. doi: 10.3389/fevo.2023.1072825
Edited by:
Michael J. Anteau, United States Department of the Interior, United StatesReviewed by:
Guinevere O. U. Wogan, Oklahoma State University, United StatesSarah Sonsthagen, University of Nebraska-Lincoln, United States
Copyright © 2023 Cramer, Hoey, Dolan, Gatins, Toy, Chancellor, Palkovacs, Garza and Beltran. This is an open-access article distributed under the terms of the Creative Commons Attribution License (CC BY). The use, distribution or reproduction in other forums is permitted, provided the original author(s) and the copyright owner(s) are credited and that the original publication in this journal is cited, in accordance with accepted academic practice. No use, distribution or reproduction is permitted which does not comply with these terms.
*Correspondence: Alli N. Cramer, ancramer@uw.edu; Jennifer A. Hoey, jhoey@calacademy.org
†These authors have contributed equally to this work and share first authorship
‡Present addresses: Alli N. Cramer, Friday Harbor Laboratories, University of Washington, Friday Harbor, WA, United States
Jennifer A. Hoey, California Academy of Sciences, San Francisco, CA, United States
Tara E. Dolan, Massachusetts Division of Marine Fisheries, Salem, MA, United States