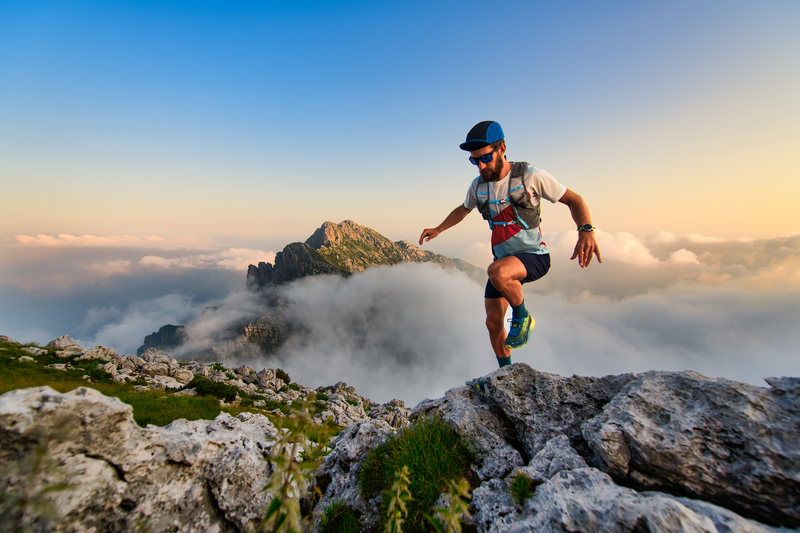
95% of researchers rate our articles as excellent or good
Learn more about the work of our research integrity team to safeguard the quality of each article we publish.
Find out more
ORIGINAL RESEARCH article
Front. Ecol. Evol. , 01 June 2023
Sec. Ecophysiology
Volume 11 - 2023 | https://doi.org/10.3389/fevo.2023.1053535
This article is part of the Research Topic New Frontiers in the Application of Stable Isotopes to Ecological and Ecophysiological Research View all 16 articles
Land-use conversion and resulting habitat fragmentation can affect the source(s) of primary productivity that fuels food webs and alter their structure in ways that leads to biodiversity loss. We investigated the effects of landscape modification on food webs in the Araguaia River floodplain in central Brazil using the top predator, and indicator species Caiman crocodilus (Crocodilia, Alligatoridae). We measured carbon (δ13C) and nitrogen (δ15N) isotope values of three tissues with different isotopic incorporation rates to evaluate spatial and temporal changes in caiman isotopic niche width with hierarchical Bayesian models that accounted for habitat use, intraspecific trait variation (sex and body size), and landscape attributes (composition and configuration). We also measured δ13C values of essential amino acids to assess if different primary producers are fueling aquatic food webs in natural and anthropogenic areas. Spatial analysis showed that caiman in agricultural areas had larger isotopic niche widths, which likely reflects some use of terrestrial resources in environments dominated by C4 plants. Patterns in δ13C values among essential amino acids were clearly different between natural and anthropogenic habitats. Overall, our findings suggest that caimans can persist in heterogeneous landscapes fueled by natural and agricultural energy sources of energy, which has implications for effectively managing such landscapes to maximize biodiversity.
Agribusiness requires extensive areas to meet human global food demand, compromising natural biodiversity and ecosystem processes (Phalan et al., 2013; Laurance et al., 2014). Floodplains provide fertile soils and water for agricultural activities but suffer intense habitat degradation and loss that impact terrestrial and aquatic ecosystems (Allan, 2004; Best, 2018). These changes occur in wetland ecosystems characterized by high complexity, productivity, and functionality that support unique and rich biodiversity and provide ecosystem goods and services (Millennium Ecosystem Assessment, 2005; Maltby and Baker, 2009). The middle Araguaia River floodplain in central Brazil, a region rich in natural communities and ecosystem processes, faces rapid conversion of native vegetation and waterbody management (pumping irrigation and damming) to supply agricultural demand and development primarily for soybean and rice production and livestock (Hunke et al., 2014; Companhia Nacional de Abastecimento [CONAB], 2015; Oliveira et al., 2015; Garcia et al., 2017; Araújo et al., 2019). Efforts to adopt sustainable practices in land and water management play an essential role in the ecological integrity of floodplain ecosystems like the Araguaia River, contributing to the conservation of biodiversity and ecosystem processes and reducing the negative impacts of anthropogenic alterations (Laurance et al., 2014; Leal et al., 2020).
Biodiversity patterns and food web dynamics are affected by the complexities of landscape modification (in the dimensions of extension, composition, and configuration) owing to the loss and fragmentation of natural habitats and alteration in the matrix of unsuitable habitats (Fischer and Lindenmayer, 2007; Haddad et al., 2015; Liao et al., 2017b). Landscape attributes, such as habitat configuration, size, and quality are determinants of population distribution and dynamics, and variation in these factors can even cause the local extinction of populations (Fahrig, 2003; Ewers and Didham, 2006). Matrix quality and type also play pivotal roles in population dynamics through factors such as permeability, hostility, disturbance, and resource availability (Quesnelle et al., 2015). However, matrix quality varies spatially and temporally for biodiversity (Driscoll et al., 2013). Because matrix and habitat quality are perceived at the species level, species traits (e.g., dispersal ability, habitat specialization, trophic level, and feeding behavior) are critically important for their persistence in fragmented landscapes (Ewers and Didham, 2006). Landscape simplification acts as an ecological filter and drives biotic homogenization of biodiversity abundance and richness, where restriction to habitat and resource availability favors species with ecological plasticity, whereas sensitive and specialist species are eliminated (Newbold et al., 2015; Le Provost et al., 2020). Such changes severely affect ecological processes such as productivity, functioning, stability, resilience, and resistance (Scheffer et al., 2001; Duffy et al., 2007; Hooper et al., 2012).
Stable isotope analysis (SIA) of consumer tissues offers an integrated analytical assessment of biochemical cycles, food web dynamics, and trophic niche information for organisms (Newsome et al., 2007; Crawford et al., 2008). Carbon isotope (δ13C) analysis can trace the basal carbon source(s) that fuel food webs while nitrogen isotope (δ15N) analysis is typically used to estimate trophic position and food chain length owing to predictable isotopic enrichment with each trophic transfer (Ben-David and Flaherty, 2012). An organism’s isotopic niche—a bidimensional δ-space (Newsome et al., 2007)—can be used as a proxy for niche variation related to ecological traits (e.g., body size and sex) that lead to differences in resource exploitation, ecosystem or habitat use, and trophic position (Marques et al., 2013; Nifong et al., 2015). In addition, SIA can reveal ecological responses to anthropogenic disturbances. For example, agricultural matrices (pastures or croplands) alter the nutrient dynamics and proportions of natural C3 autochthonous production and C4 allochthonous subsidies in aquatic food webs (Martinelli et al., 2007; Carvalho et al., 2015; Bentivoglio et al., 2016; Parreira de Castro et al., 2016). SIA can help link landscape modification with alterations in trophic dynamics that result in trophic downgrading, niche collapse, low niche redundancy, high niche overlap, homogenization of energy flow, and niche shifts (Layman et al., 2007; Resasco et al., 2017; Magioli et al., 2019; Price et al., 2019; Burdon et al., 2020).
Furthermore, spatial extension mediates the spatial heterogeneity of stable isotope ratios in ecosystems, including under small-scale and human influence (Zambrano et al., 2010; Doi et al., 2013; Merlo-Galeazzi and Zambrano, 2014) that can directly affect the isotopic niche (Ceia et al., 2014; Reddin et al., 2018). In general, landscape attributes are neglected in spatial food web models to elucidate the functioning mechanisms, especially in ongoing landscape modification worldwide (Pillai et al., 2011; Liao et al., 2017a). Ignoring spatial isotopic variability could lead to misinterpretation and potentially mask the impacts of anthropogenic disturbances. For example, SIA-based assessments of the impacts of anthropogenic disturbances in aquatic ecosystems often make inferences from categorical or disturbance gradient designs using dispersal-limited top predators, such as fishes (Carvalho et al., 2015; Price et al., 2019; Burdon et al., 2020). Such approaches are inappropriate for top predators in wetland ecosystems, which are often large-bodied and highly mobile species that utilize a generalist feeding behavior. For example, crocodilians explore all waterbody habitats and have population dynamics according to landscape attributes, exerting ecological influence on aquatic and adjacent terrestrial food webs (Somaweera et al., 2020). Thus, spatial analysis and landscape-level patterns (amount, composition, configuration of habitats, and matrix types) can integrate SIA data from top predators to provide critical information and context about spatial ecological processes in the floodplains (Wang et al., 2014; Riva and Nielsen, 2020).
Additionally, the limitations of studies using only bulk tissue SIA can make it challenging to identify the type(s) of primary production fueling aquatic or terrestrial food webs (Finlay and Kendall, 2008; Boecklen et al., 2011; Zaia Alves et al., 2017). The primary limitation is that algal-derived aquatic and plant-derived terrestrial primary producers often show overlap in their δ13C composition (Whiteman et al., 2019). An emerging alternative approach is measuring the δ13C values of essential amino acids. Plants and algae have distinct patterns in essential amino acid δ13C values (δ13CEAA) due to differences in the way(s) each producer type synthesizes these compounds (Larsen et al., 2009, 2013; Besser et al., 2022). Most eukaryotic consumers cannot synthesize essential amino acids de novo and must route these compounds directly from the protein in their food, leading to minimal isotope alteration of essential amino acids as they are passed up the food chain (McMahon et al., 2015; Whiteman et al., 2019; Manlick and Newsome, 2022). Applying this approach to top consumers can identify the basal source(s) of energy that fuels the food webs they rely on and examine potential human-induced shifts in energy sources (Thorp and Bowes, 2016; Bowes et al., 2019), especially in landscapes heavily influenced by agriculture.
Here, we combined landscape attributes, species intraspecific traits, and isotope-based estimates of the trophic ecology of Caiman crocodilus (spectacled caiman) to investigate the anthropogenic impacts of landscape modification on the food webs of the Araguaia floodplain using a spatially explicit approach. Caiman crocodilus (Crocodylia, Aligatoridae) is an indicator species and a model organism for detecting and monitoring environmental impacts in the Araguaia floodplain owing to its high detectability and seasonal and ontogenetic movements across a variety of terrestrial and aquatic habitats (Rosenblatt et al., 2013; Somaweera et al., 2020; Pereira and Colli, 2022). We assessed (1) the effects of intraspecific traits of sex, ontogeny, and habitat use based on the δ13C and δ15N isotopes values of C. crocodilus tissues; (2) the influence of landscape attributes (land-use composition and wetland configuration) on the isotopic niche of C. crocodilus; and (3) the influence of crop-derived (rice and soybean) energy on the food webs utilized by C. crocodilus in human-modified environments.
We conducted this study in the middle Araguaia River floodplain (Figure 1). The region is in a highly dynamic and complex Cerrado–Amazonia transitional zone in central Brazil (Marques E. Q. et al., 2020). The pronounced tropical wet-dry climate influences the flooding regime: the discharge increases from November to April (wet season) when the flood pulse can span approximately 88,000 km2 of surface area at maximum flood level and interconnects several waterbodies, and decreases during June and September (dry season), when waterbodies represent only 3.3% (2,930 km2) of the coverage area (Irion et al., 2016). The floodplain’s high spatial and temporal heterogeneity supports a rich and abundant biota, with many endemic and endangered species, sheltered in several protected areas and indigenous lands, including Bananal Island, RAMSAR site no. 624 (Ramsar Convention [RAMSAR], 2002). These protected areas are crucial in limiting the advance of fragmentation and land-use conversion (Carranza et al., 2014; Garcia et al., 2017).
Figure 1. Location of seven sampling landscapes in the middle Araguaia River floodplain, Central Brazil: (A) Canguçu; (B) Cristalândia; (C) Lagoa; (D) Bananal; (E) Xavante; (F) Coopergran; (G) Cooperformoso. Inset boxes ordered from North to South. Land use classification, hydrograph, and protected areas in the region are denoted. Red points represent the position of captured caimans in each sampling site. Red lines indicate the maximum spatial region that include a 3 km buffer for landscape attributes estimates. The 3 km buffers were merged when they overlapped.
However, this region is under sustained pressure from agricultural development funded by international and state programs because of the favorable topography and hydrology that has turned the floodplain into one of Brazil’s leading producers of irrigated rice (Fragoso et al., 2013; Companhia Nacional de Abastecimento [CONAB], 2015). The production is based on irrigated systems, where crops are cultivated according to the hydrological regime: rice in the wet season alternates with other crops (e.g., soybeans, beans, and watermelon) in the dry season (Oliveira et al., 2015). Similar to the entire Cerrado biome (Hunke et al., 2014; Dias et al., 2016), the Araguaia River Basin suffers sustained pressure from agricultural activities that have reduced native vegetation to less than 50% in the Upper Araguaia River (Ferreira et al., 2008; Coe et al., 2011) and changed the hydrogeomorphological dynamics owing to water damming, pumping, sedimentation, silting, erosion, and contamination (Latrubesse et al., 2009; Coe et al., 2011; Oliveira et al., 2015). A 26% reduction in native vegetation coverage was recorded between 1975 and 2013 in the middle Araguaia River floodplain, mainly driven by the expansion of pasturelands (Garcia et al., 2017). Currently, the land use pattern of the Cerrado is changing slowly from extensification to intensification of agricultural activities; however, pastureland coverage remains high (Dias et al., 2016).
We conducted fieldwork between July and September (dry season) in the years 2016 and 2018 in seven localities in five municipalities of Tocantins State (Figure 1), namely Bananal, Canguçu, Cristalândia, Cooperformoso, Coopergran, Xavante, and Lagoa (sampled in 2016). We sampled caimans in natural and anthropogenic habitats distributed across 32 sites under distinct land-use regimes, including inside and outside protected areas. Natural habitats include rivers and lakes derived from natural geomorphological processes in the Araguaia floodplain (Irion et al., 2016). Rivers comprise small and sizeable lotic water body tributaries of the Araguaia River, characterized by a sinuous water topology and natural riparian vegetation. Lakes include the small (0.5–5 ha) and large (>5 ha) lentic water bodies of diverse forms with riparian vegetation, but also large reservoirs created to supply water for agricultural activities. We defined anthropogenic habitats as waterbodies constructed for human activities within an agricultural matrix, such as artificial ponds (restricted to shallow waterbodies of <0.5 ha in area, created by soil excavation for livestock watering or fish farms and maintained by water complementation) and agricultural irrigation ditches—channelized drainages of permanent water flow inside agricultural fields—5–20 m wide and 0.5–2 m deep, with networks in a linear planform and right angles with vegetated or bare bank slopes (Davies et al., 2008; Herzon and Helenius, 2008; Biggs et al., 2016).
Migration and movement studies of South American crocodilians indicate a maximum movement distance of 20 km over 1–5 years (Gorzula, 1978; Ouboter and Nanhoe, 1988; Campos et al., 2006). Therefore, the sampling localities were at least 20 km apart, and sites within each locality were as far apart as possible. Therefore, we created circular buffer areas of 500 m, 1 km, and 3 km centered at each capture point to calculate the landscape metrics for the characterization, assessment, and measurement of human-modified landscapes. Based on home range studies, we considered buffer zones as a utilization area of caimans, which estimated ranges between 0.048 and 3.5 km2 (Ouboter and Nanhoe, 1988; Campos et al., 2006; Caut et al., 2019; Marques T. S. et al., 2020).
We assessed human land-use composition and wetland configuration through landscape metrics based on circular buffers of 500 m, 1 km, and 3 km centered at each animal in the site. First, we obtained land use/cover rasters from the MapBiomas Project (collection 4, 2016 and 2018).1 We grouped MapBiomas land-use classes into five categories: waterbody, forest, savanna (savanna, grassland, non-forest natural formation, and other non-forest natural formations), pasture (pasture and other non-vegetated areas), agriculture (annual and perennial crops), and urban (urban infrastructure). To improve water coverage assessment, we incorporated a hydrographic raster from a vector database acquired from Secretaria do Meio Ambiente e Recursos Hídricos of the State of Tocantins.2 Furthermore, we improved land use owing to differences between the supervised coverage in situ and the MapBiomas raster, reclassifying and redefining the topology guided by Landsat 8 satellite images for the same sampling period in 2016 and 2018 obtained from the Instituto Nacional de Pesquisas Espaciais–INPE (Brazilian Space Agency)3 using QGIS, version 3.12 (QGIS Development Team, 2020).
Second, we calculated the landscape metrics at the class and landscape levels for each buffer using the R package LANDSCAPEMETRICS (Hesselbarth et al., 2019). The landscape division index (LDI) was selected at the landscape level. At the class level, the metrics included only the proportion of classes (PCLASS) for all categories to describe the landscape composition. In contrast, the metrics at the patch level were restricted to water coverage to estimate wetland configuration: mean Euclidean nearest-neighbor distance (ENN), largest patch index (LPI), patch cohesion index (COHESION), and mean patch area (MPA). Such landscape metrics reflect the aspects of proportion, isolation, patch dominance, aggregation, physical connectivity, and landscape fragmentation (McGarigal and Marks, 1995; Jaeger, 2000).
Third, we minimized multicollinearity among landscape metrics using the variance inflation factor with a maximum of 4 in the R package USDM (Naimi et al., 2014), resulting in the retention of 14 metrics with a maximum correlation of r = 0.67 among them (Supplementary Table 1). We then calculated the mean values of landscape metrics at each site, applied a log (x + 1) transformation, and standardized the values around the mean with one standard deviation for posterior analyses.
We sampled each locality only once, staying between 4 and 7 days to perform captures, where we visited the sampled sites up to four times. We captured 275 caimans with sampling effort per site ranging from 6 to 14 animals and a sex ratio (male:female) of 2:1 (Supplementary Table 2). We captured caimans through nocturnal spotlight surveys with locking cable snares or by hand after locating the animals by eye reflection, with subsequent physical restraint of mouth and limbs with ropes and adhesive tape (Fitzgerald, 2012; Brien and Manolis, 2016). We recorded the snout-vent length (SVL; with a 0.1 cm precision tape), body mass (with 5, 10, or 50 kg spring scales, Scale Macro Line, Pesola Präzisionswaagen AG®, Schindellegi, Switzerland), and sex, determined by cloacal examination and palpation of the penis (Reed and Tucker, 2012). In addition, we individually marked C. crocodilus by notching tail scutes as a standardized numerical code and released them at the exact capture location after handling (Plummer and Ferner, 2012). We conducted this study under permits SISBIO #13324-6 and #57940-3 (issued by Instituto Chico Mendes de Conservação da Biodiversidade), FUNAI #08620.005147/2018-38 (Fundação Nacional do Índio), and CEUA-UnB #94/2017 (Comissão de Ética no Uso de Animais da Universidade de Brasília).
We collected claw (∼5 mm fragments), tail muscle (1 g), and whole blood (∼3 mL) samples from the captured animals for SIA (Beaupre et al., 2004; Fleming and Fontenot, 2015). Blood samples were obtained from the dorsal cervical sinus with a blood collection kit using 21G × 1” needles (25 × 8 mm) coupled to a 4 mL BD Vacutainer® with lithium heparin anticoagulant, which showed no significant isotopic effect on plasma and red blood cell samples within 3 h (Kim and Koch, 2012). Blood samples were then centrifuged at 1,370 g for 60 s (OMEGA, Laborline®, São Paulo, Brazil) to separate and collect the plasma samples. In the field, we stored claw samples in plastic, while other tissue samples were stored at -80°C in a cryogenic liquid nitrogen container until preparation in the laboratory.
In the laboratory, claw and muscle samples were cleaned, and lipids were extracted with a 2:1 ratio of chloroform:methanol solvent solution for three washes for 2 h each (Post et al., 2007). Samples were then dried at 50°C and ground into a homogenous powder. Plasma tissue samples were freeze-dried for 24 h (Mod. E-C MicroModulyo, E-C Apparatus®) and stored at 20°C. Additionally, we collected and prepared crop samples with seeds from the Coopergran locality (rice: n = 10; soybean: n = 10). The samples were dried in an oven (60°C for 48 h) and ground into a homogenous powder. Finally, we weighed approximately 1–2 mg of each caiman sample and 2.0–2.5 mg of each crop sample and placed them into a 3 × 5 mm tin capsules for δ13C and δ15N analysis.
Carbon (δ13C) and nitrogen (δ15N) isotope values were determined by combustion using a Carlo Erba, CHN-1100 elemental analyzer coupled with a Thermo Finnigan Delta Plus isotope ratio mass spectrometer at the Laboratory of Isotope Ecology of the “Centro de Energia Nuclear na Agricultura” (CENA/Universidade de São Paulo), Piracicaba, São Paulo, Brazil. Based on the internationally recognized standard, the results were expressed in delta notation (δ) in parts per thousand (‰). The following equation was used:
where Rsample and Rstandard represent the heavy and light isotope molar ratios (13C/12C or 15N/14N) of the sample and standard, respectively. The internationally accepted standards for δ13C and δ15N analysis is Vienna Pee Dee Belemnite (Vienna PDB; 13C/12C ratio = 0.01118) and atmospheric nitrogen (15N/14N ratio = 0.0036765), respectively. Internal reference materials (USGS-42 and sugarcane leaves) were routinely interspersed with unknown samples. The mean within-run analytical precision for the internal reference materials was 0.2‰ for both δ13C and δ15N.
We also measured each sample’s weight percent carbon:nitrogen concentrations (C:N). Most samples had mean (±SD) C:N values within acceptable limits in plasma (3.3 ± 0.2), muscle (3.3 ± 1.9), and claw (2.9 ± 0.1) (Post et al., 2007), indicating little presence of lipids. However, 17 muscle samples had C:N values >4.0, suggesting a high lipid content (Post et al., 2007; Logan et al., 2008). To address this problem, we imputed the δ13C values of these samples using the procedures described below in the Data Analysis subsection (Penone et al., 2014). We did not consider using lipid correction equations because such equations are species- and tissue-specific (Logan et al., 2008) and are not currently available for crocodilian tissues.
We evaluated resource use at multiple temporal and spatial scales using tissues with different isotopic incorporation rates that integrate diet over different periods (Crawford et al., 2008; Ben-David and Flaherty, 2012). Based on the tissue-specific isotopic incorporation rates available from a congener crocodilian species (Caiman latirostris), we assumed that plasma provides a relatively short timescale (∼90 days), muscle reflects an intermediate timescale (130–190 days), and claws represent a relatively long timescale integrating >1 year of ecological information (Caut, 2013; Marques et al., 2014; Vander Zanden et al., 2015).
We randomly selected muscle samples from 40 caimans for essential amino acid (AAESS) δ13C analysis. These samples were collected in both natural (lakes and river) and anthropogenic (ponds and ditches) habitats from four localities: 10 individuals from lakes (five from Canguçu and five from Bananal), 10 individuals from rivers (five from Canguçu and five from Bananal), 10 individuals from ponds (five from Coopergran and five from Cooperformoso), and 10 individuals from ditches (five from Coopergran and five from Cooperformoso). Descriptions of selected caiman populations are in Supplementary Table 3. Muscle samples were prepared for amino acid δ13C analysis at the University of New Mexico Center for Stable Isotopes (Albuquerque, NM). A ∼3–4 mg of lipid-extracted muscle sample was hydrolyzed to constituent amino acids in 1 ml of 6N HCl at 110°C for 20 h; tubes were flushed with N2 gas before sealing to prevent oxidation during hydrolysis. Amino acids were subsequently derivatized with 2-isopropanol and trifluoracetic acid (Silfer et al., 1991) and analyzed in duplicate to assess accuracy and precision. δ13C measurements were made on a Thermo Scientific Delta Plus IRMS (Bremen, Germany) after samples were separated on a 60 m BPX5 column (SGE Analytical Science, Ringwood, Victoria, Australia) in a Thermo Scientific Trace 1310 gas chromatograph (GC, Bremen, Germany) and underwent combustion to CO2 in a ceramic reactor set at 1,000°C in a Thermo Scientific IsoLink II (Bremen, Germany).
For amino acid δ13C measurements, we used a mixture of commercially available powdered amino acids (Sigma Aldrich, St. Louis, MO, USA) as a reference material derivatized and analyzed alongside each batch of unknown samples. All reference materials and unknown samples were processed and analyzed simultaneously with the same reagents and subject to the same protocols. δ13C values for each underivatized amino acid were previously measured with a Costech 4,010 elemental analyzer coupled to a Thermo Scientific Delta V Plus IRMS (Breman, Germany). Like bulk tissue results, amino acid isotope data are reported using the standard δ-notation using the Vienna Pee Dee Belemnite (V-PDB) scale. We measured δ13C values of six essential amino acids including threonine (Thr), valine (Val), leucine (Leu), isoleucine (Ile), phenylalanine (Phe), tyrosine (Tyr), and lysine (Lys). The average within-run standard deviation of δ13C values of the in-house amino acid reference material ranged from 0.2‰ (isoleucine) to 0.6‰ (lysine); mean analytical precision across all six AAESS was 0.4‰. We describe in Supplementary Appendix A the preparation and measurement procedures used for the AAESS δ13C analysis (Whiteman et al., 2019).
We treated the missing values (δ13C and δ15N from eight individuals for muscle sample; n = 16) and δ13C values for muscle samples with a C:N ratio > 4 (n = 17), representing 0.02% of all data (n = 1650), through imputation using the R package MISSFOREST (Stekhoven and Buühlmann, 2012). Imputation is a viable solution when missing data can introduce bias and lead to incorrect conclusions owing to the masking of biological patterns (Penone et al., 2014). MISSFOREST is a non-parametric method that relies on random forest algorithms to predict missing values (Stekhoven and Buühlmann, 2012). Performance is assessed using the normalized root mean squared error (NRMSE), where excellent performance leads to a value close to 0 (Stekhoven and Buühlmann, 2012). In our case, the NRMSE was 0.03%.
We estimated isotopic niche widths through the Bayesian standard ellipse area metric (SEAB; in ‰2) using the R package SIBER with its default settings (Jackson et al., 2011). SEAB estimates were quantified at the site level for each tissue type. We also selected landscape metrics relevant to isotopic niche width using the R package BORUTA (Kursa and Rudnicki, 2010), a random forest-based selection method that identifies all-relevant variables. We used a ntree of 2,000, maxRuns of 2,000, and the default settings for the other parameters. We retained the landscape attributes with mean and normalized importance values above zero (meanImp and normImp > 0), obtained through the function attStats (Supplementary Tables 2, 4).
We implemented a hierarchical Bayesian approach to model the spatial variation in the (i) isotopic composition (δ13C and δ15N) under the effects of intraspecific traits of sex, ontogeny, and habitat and (ii) the isotopic niche width of C. crocodilus under the effects of land-use composition and wetland configuration across landscapes in the Araguaia floodplain. Spatial hierarchical Bayesian models were structured through stochastic partial differential equations (SPDE) combined with the integrated nested Laplace approximation (INLA) algorithm using the R package R-INLA (Rue et al., 2009; Lindgren et al., 2011); thus, this approach accounted for the spatial dependency between sampling sites and the effects of selected predictors. We created models separately for each tissue, where the response variables were δ13C, δ15N, and isotopic niche width. The predictors were SVL, sex, habitat, and their interactions (for δ13C and δ15N models) or landscape attributes (for the isotopic niche width model). We applied backward stepwise procedures to INLA to obtain the best model using the INLAstep function in the R package INLAUTILS (Redding et al., 2017). We standardized the SVL around the mean with one standard deviation and applied an orthogonal contrast to categorical matrices using the model.matrix function.
For each model, we evaluated the performance of different mesh designs based on deviance information (DIC) and Watanabe-Akaike information (WAIC) criteria (Wang et al., 2018). We created five mesh designs using constrained refined Delaunay triangulation based on individual positions for the δ13C and δ15N models or sampling site locations for the isotopic niche width model by varying the sizes of triangles within and outside the sampled area (Supplementary Figure 1), attempting to minimize any boundary effects (Lindgren and Rue, 2015). Details about the models, representation of the spatial random fields, and descriptions of the posterior estimates of hyperparameters from the spatial hierarchical Bayesian approach are provided in Supplementary Appendix B.
We investigated differences in patterns of measured AAESS δ13C values (or fingerprints) of caiman collected across habitats or localities. We performed linear discriminant analysis (LDA) using the R package MASS (Venables and Ripley, 2002) to discriminate between habitats or localities. We applied and examined the reclassification error rates using the leave-one-out cross-validation approach (Larsen et al., 2013). In LDA, we plotted the 95% confidence interval ellipses for each habitat or location, and the dataset was not standardized. All statistical tests were performed using R, version 3.6.1 (R Development Core Team, 2021).
The hierarchical Bayesian approach demonstrated that the mesh design performed differently in the δ13C and δ15N models as indicated by DIC and WAIC (Supplementary Tables 5, 6). The spatial structure of mesh 1 was the best for all tissues in δ13C models, whereas mesh 1 (plasma and claw) and 5 (muscle) were the best for the δ15N models. The isotopic models had similar random fields among tissues, with reduced spatial uncertainty in the regions of the sampled points (Supplementary Figures 2, 3).
The spatial distribution of δ13C and δ15N values differed among tissues, i.e., the time window (Figures 2A, B). The predicted spatial distribution of δ13C showed the lowest value in plasma and the highest in claw. In contrast, the predicted spatial distribution of δ15N values showed little difference (∼0.5‰) among the tissues. The Xavante, Cooperformoso, and Coopergran localities had higher δ13C and δ15N values than other areas (Figures 2A, B and Supplementary Figures 4A, B).
Figure 2. Predicted values from spatial hierarchical Bayesian best models for (A) δ13C, (B) δ15N, (C) isotopic niche width of Caiman crocodilus according to tissue across landscapes in the Araguaia floodplain. Hydrograph was depicted in light blue color in the frames and white points represent each sampled caiman (A,B) or sampling sites (C). The colors indicate levels of mean δ13C (%), δ15N (%), isotopic niche width (%2) according to the associated legend. High values in bulk tissue δ13C and δ15N are related to anthropogenic habitats (e.g., irrigation systems) while surrounding natural habitats have lower values creating a spatial isotopic variability at landscape scales. Additionally, larger caiman isotopic niche widths were concentrated in the largest agricultural irrigation system and related to a greater proportion of pasture coverage. Some human-modified landscapes had similar niche widths as natural landscapes in the Araguaia floodplain, suggesting the same intensity of resource use in these populations across the landscape.
In the δ13C models, only habitat affected δ13C in the plasma model (short timescale), with ponds having higher values than other habitats (Table 1). Models for muscle (intermediate timescale) and claw (long timescale) showed similar results, with significant effects of habitat, SVL, and habitat: sex: SVL interaction; muscle and claw collected in ponds had higher δ13C values while ditches had lower δ13C values in comparison to other habitats. SVL negatively affected muscle and claw δ13C values, indicating that larger males positively influence the SVL–δ13C relationship.
Table 1. Posterior estimates (mean, SD, and 95% credibility interval) from spatial hierarchical Bayesian best models relating sex, snout-vent length (SVL), and habitat effects to δ13C values of Caiman crocodilus across landscapes in the Araguaia floodplain.
In the δ15N models, only habitat significantly affected plasma δ15N values, with ponds having lower values than other habitats (Table 2). Muscle δ15N differed among habitats, with lakes having lower values than other habitats. Furthermore, habitat and sex influenced the SVL–δ15N relationship, in which males in the ditch showed that δ15N values decreased with SVL while in the pond, males showed that δ15N values increased with SVL. Finally, claw δ15N was affected by the SVL–δ15N relationship owing to the habitat effect of the ditch, showing a negative trend. The same SVL–δ15N relationship in the ditch differed according to sex, with males having a negative effect.
Table 2. Posterior estimates (mean, SD, and 95% credibility interval) from spatial hierarchical Bayesian best models relating sex, snout-vent length (SVL), and habitat effects to δ15N values of Caiman crocodilus across landscapes in the Araguaia floodplain.
We found similar DIC and WAIC values among mesh designs within each tissue model, suggesting that the structures had similar spatial dependencies in the hierarchical Bayesian approach for isotopic niche width (Supplementary Table 7). However, we selected the mesh structure with the lowest DIC and WAIC values: mesh 2 for plasma, mesh 5 for muscle, and mesh 1 for the claw. The spatial random fields of caiman isotopic niche widths varied according to tissue type (Supplementary Figures 2C, 3C). Plasma and claw random fields had low spatial dependence and reduced uncertainty across the Araguaia floodplain, whereas the muscle random field had high dependence and uncertainty, especially in the north and south of the study area.
The Boruta and INLA stepwise selection procedures retained only the proportion of pasture coverage and the fragmentation index for models of isotopic niche width (Table 3). The proportion of pasture coverage in the 500 m buffer affected the caiman isotopic niche width, with a positive effect in the plasma. The remaining predictors in their respective tissue models did not affect the caiman isotopic niche widths. The predicted isotopic niche width showed spatial variability across sites with a large range in plasma, intermediate range in muscle, and small range in claw (Figure 2C and Supplementary Figure 4C). Plasma isotopic niche widths were remarkably high in the Cooperformoso and Coopergran regions. For muscle, the central region of the study area had small isotopic niche widths, whereas the Cooperformoso and Coopergran regions maintained high values. Overall, the spatial distribution of isotopic niche width in the claws was higher than in other tissues, but it was distributed homogeneously across the Araguaia floodplain.
Table 3. Posterior estimates (mean, SD, and 95% credibility interval) from spatial hierarchical Bayesian best models relating Boruta-selected landscape attributes to isotopic niche width (SEAB) of Caiman crocodilus across landscapes in the Araguaia floodplain.
Linear discriminant analysis showed that essential amino acid (AAESS) δ13C patterns differed among habitats with an overall successful reclassification rate of 65%. Successful reclassification was 50% for ponds, 60% for ditches, 70% for lakes, and 80% for rivers (Supplementary Appendix C). The linear discriminant axes explained 88% (LD1) and 9% (LD2) of the overall variation among habitats, and the most informative coefficients were phenylalanine, leucine, and lysine δ13C values. The LDA results showed a clear distinction between caiman sampled in natural (rivers and lakes) versus anthropogenic (ponds and ditches) habitats (Figure 3A).
Figure 3. Multivariate discrimination based on AAESS δ13C values of Caiman crocodilus according to habitat (A) and locality (B). Ellipses indicate the 95% confidence interval region for classified groups of C. crocodilus. There is a distinction in basal carbon sources fueling food webs in natural versus anthropogenic habitats utilized by caiman, indicating an anthropogenic influence on energy and nutrient flow.
Linear discriminant analysis also showed that AAESS δ13C patterns differed among localities (Figure 3B), with an overall correct reclassification rate of 85%. Successful reclassification varied between localities: Canguçu (70%), Coopergran (80%), Cooperformoso (90%), and Bananal (100%) (Supplementary Appendix D). LD1 and LD2 explained 80 and 10% of the variation, respectively, and the most informative coefficients were for phenylalanine, leucine, and lysine δ13C values.
We showed that human-induced landscape modifications affect wetland food webs in the Araguaia floodplain. Analysis of spatial isotopic patterns showed that high values of δ13C and δ15N values, as well as large isotopic niche widths of Caiman crocodilus were associated with agricultural areas. Pasture coverage was the principal landscape feature that affected caiman niche width, with changes resulting from land-use conversion, habitat alteration, and fragmentation. Moreover, AAESS δ13C analysis revealed that natural and anthropogenic habitats differed in basal carbon sources, indicating that crop-derived energy contributed to fuel caiman food webs in anthropogenic habitats.
Crocodilians are highly mobile top predators with opportunistic and generalist foraging strategies (Magnusson et al., 1987; Da Silveira and Magnusson, 1999; Somaweera et al., 2020). The diet varies with ontogeny, i.e., hatchlings feed primarily on aquatic and terrestrial invertebrates, whereas adults feed on vertebrates and fishes; flood pulse, with invertebrates predominating in the wet season and fishes in the dry season (Magnusson et al., 1987; Thorbjarnarson, 1993, 1997; Da Silveira and Magnusson, 1999); and sex, with mature females using different habitats and consumed resources during the nesting period for mature females (Barão-Nóbrega et al., 2016). Moreover, crocodilians can participate in aquatic and terrestrial food webs according to prey preferences and habitat use. Sympatric Amazonian crocodilians (Paleosuchus trigonatus, P. palpebrosus, Melanosuchus niger, and Caiman crocodilus) exhibit interspecific niche divergences based on the energy source, with more autochthonous sources in the floodplains over allochthonous inputs in the headwaters (Villamarín et al., 2017). We show that habitat, sex, and ontogeny regulate the strength and dynamics of their trophic interactions (Rosenblatt et al., 2013; Somaweera et al., 2020). Crocodilian studies show niche divergence through ontogenetic variation along stable isotopes or in the isotopic niches, which relates to reduced intraspecific competition (Radloff et al., 2012; Marques et al., 2013; Nifong et al., 2015; Caut et al., 2019). A previous study in the Araguaia region indicated that sex-related ontogenetic shifts drive isotopic niche partitioning in Caiman crocodilus that occupy similar habitats (Pereira et al., 2018). Such variations can be mediated by density-dependent mechanisms (such as social hierarchy and sexual dimorphism or nutritional and physiological requirements) to impose differences in the isotopic values, and niche segregation at the habitat and microhabitat level (Marques et al., 2013; Caut et al., 2019).
We observed marked landscape-scale spatial heterogeneity in δ13C and δ15N values of caimans from the Araguaia River floodplain. This variation is likely driven by spatial variation in the sources of primary production—aquatic versus terrestrial or natural versus agricultural across distinct habitats— and thus, to ecological processes and conditions across distinct habitats (Finlay and Kendall, 2008; Boecklen et al., 2011; Zaia Alves et al., 2017). Similar patterns in isotope variation across small spatial scales have been reported in artificial and natural freshwater ecosystems (Zambrano et al., 2010; Doi et al., 2013; Merlo-Galeazzi and Zambrano, 2014). Additionally, we found that caiman trophic niche width was influenced by pasture coverage in the Araguaia region, with high values clustered in the most extensive irrigation systems such as the Cooperformoso and Coopergran areas. The conversion of the floodplain to pasture changes the photosynthetic type of terrestrial production from C3 trees/shrubs to C4 grasses and alters soil hydro-physical properties that maximize the susceptibility of aquatic ecosystems to pasture inputs through erosion, sedimentation, and leaching processes, including a reduction in riparian vegetation (Latrubesse et al., 2009; Coe et al., 2011; Hunke et al., 2014). While (C4) grass fragments can enter the aquatic ecosystem and increase the δ13C composition of dissolved inorganic carbon and particulate organic matter at the base of aquatic food webs (Martinelli et al., 2007), such resources are not easily assimilated by aquatic consumers, who instead favor higher quality autochthonous (algae) or allochthonous (C3 terrestrial) resources (Wantzen et al., 2010; Thorp and Bowes, 2016). C4-derived carbon from pasture or savanna can be introduced into caiman tissues by consuming insectivorous-omnivorous fishes or terrestrial invertebrate or vertebrate grazer prey (Wantzen et al., 2010). Our results show that caimans that use artificial ponds are highly susceptible to that allochthonous subsidy (Jardine et al., 2017).
Basal autochthonous (particulate organic matter and algae) and allochthonous (C3 and C4 plants) resources that fuel aquatic food webs adjacent to pasturelands can have highly variable δ13C and δ15N values, which can be identified and monitored via analysis of consumer tissues (García et al., 2017). Thus, variation in the proportion of pasture coverage can drive considerable alterations in basal resources, feeding behaviors, and isotopic niche sizes of consumers. Large-scale conversion of wetlands to pasture can be an irreversible change (in the sense of intangible recovery of the previous state), disrupting ecological processes that define food web structure and function (Fischer and Lindenmayer, 2007; Tscharntke et al., 2012; Haddad et al., 2015). The relevant association of the fragmentation index with the caiman isotopic niche suggests a chronic effect of landscape modification and habitat disturbance on terrestrial and aquatic food webs through land-use conversion, expanding an agricultural matrix over natural vegetation. Overall, the Cerrado biome suffers from a historical and constant pressure of pasturelands and cropland expansion (Barretto et al., 2013; Dias et al., 2016), including in the Araguaia River Basin (Ferreira et al., 2008; Coe et al., 2011; Garcia et al., 2017). The favorable climate, topography, and soil physical properties in the Araguaia floodplain linked to government incentives through technological, mechanical, and financial support have converged this region into an agricultural frontier (Fragoso et al., 2013; Phalan et al., 2013; Araújo et al., 2019). Although future agribusiness expansion can be reduced by agriculture intensification and new protected areas (Barretto et al., 2013; Carranza et al., 2014; Dias et al., 2016; Garcia et al., 2017), areas of natural vegetation will still be fragmented and converted to pasturelands or croplands, with species in the Cerrado facing a considerable challenge to persist (Strassburg et al., 2017; Lemes et al., 2019).
Bulk tissue stable isotope analysis shows high isotopic variability and overlap of δ13C values between aquatic (algal) primary producers and the most common crops harvested in agricultural matrices (soybean and rice) in the Araguaia River floodplain, Figure 4 (Zaia Alves et al., 2017). However, essential amino acid δ13C data identified a clear distinction in basal carbon sources fueling food webs in natural versus anthropogenic habitats utilized by caiman, indicating an anthropogenic influence on energy and nutrient flow. We hypothesize that the distinct AAESS δ13C fingerprints between habitats or localities is driven by the incorporation of carbon from (C3) crops (soybeans and rice) which could have distinct AAESS δ13C fingerprints in comparison to natural (C3) vegetation. We acknowledge that this hypothesis has not been rigorously tested. Alternatively, a recent study shows that C3 and C4 plants have distinct AAESS δ13C fingerprints (Besser et al., 2022), so the patterns shown in Figure 3 could be primarily driven by greater incorporation of C4 resources caiman diets in anthropogenic habitats, ponds and ditches (Pereira et al., 2018; Pereira and Colli, 2022). Unfortunately, we cannot currently discriminate between these two explanations because AAESS δ13C data are unavailable for local primary producers.
Figure 4. δ13C and δ15N values (A) and associated means ± SD (B) of basal sources in the Araguaia River Basin, crop samples (rice and soybean), and all caiman samples collected in our study. Remarkable isotopic variability in the bulk δ13C and δ15N occurs in basal sources, including with agricultural samples and caimans. Basal source isotopic data for primary producers is from Zaia Alves et al. (2017) and includes periphyton, phytoplankton, particulate organic carbon (POC), C4 terrestrial grass, and C3 riparian vegetation.
The spatially explicit Bayesian models approach employed here helps explore the relationship between landscape attributes and species responses that consider intraspecific variations and avoid dichotomic/categorical landscape evaluations (e.g., Resasco et al., 2017; Magioli et al., 2019) that do not reflect spatial variations and mechanisms that moderate the landscape use by organisms (Tscharntke et al., 2012; Wang et al., 2014; Riva and Nielsen, 2020). Landscape configuration drives food web structure and trophic interactions (Rooney et al., 2008; Pillai et al., 2011; Liao et al., 2017b). Realistic ecological responses to landscape alteration arise from considering species traits (e.g., trophic level, feeding behavior, body size, and dispersal ability) and species-oriented habitat perception (Ewers and Didham, 2006). These traits interact with landscape characteristics modeling species’ sensitivity and tolerance in the face of disturbance and determining the persistence in human-modified landscapes (Villard et al., 2014).
Our findings support evidence that a mixture of natural and anthropogenic (agricultural) energy can support top predators in highly modified landscapes in the Araguaia River floodplain. However, previous studies found that changes in trophic structure occur and energy channels can be lost in anthropic landscapes, making it unfeasible for a food web to support top predators in the long term (Layman et al., 2007; Liao et al., 2017b), triggering a trophic cascade with pronounced impacts on ecosystem resilience and resistance to disturbances (Scheffer et al., 2001; Duffy et al., 2007; Hooper et al., 2012). Our results show that the diversification of energy pathways (or channels) may stabilize the structure of food webs in some human modified environments. The fragmentation threshold for species extinction depends on community and landscape contexts (Villard et al., 2014; Liao et al., 2017a). Understanding the potential of anthropogenic landscapes to support biodiversity and ecological and conservation values relies on evaluating the key attributes of species, food webs, and ecosystem processes in the spatial context of landscape properties (Rooney et al., 2008). Our results emphasize that landscape modification can be reflected in the trophic niche of a semi-aquatic top predator, and provides new insights into how landscape fragmentation affects food web dynamics in a human-modified floodplain. These results enhance our understanding and contributing critical information to environmental policies, conservation planning, and land use management.
The raw data supporting the conclusions of this article will be made available by the authors, without undue reservation.
This animal study was reviewed and approved by SISBIO #13324-6 and #57940-3 (issued by Instituto Chico Mendes de Conservação da Biodiversidade), FUNAI #08620.005147/2018-38 (Fundação Nacional do Índio), and CEUA-UnB #94/2017 (Comissão de Ética no Uso de Animais da Universidade de Brasília).
AP conceived the ideas together with GN and GC and designed the study. AP conducted fieldwork. AP, CM, and SN conducted laboratory analyzes of compound specific stable isotopes. AP and GC analyzed the data with help from SN. AP, GN, CM, and SN interpreted isotopic results. AP wrote the manuscript. All authors contributed to the drafts and gave final approval for publication.
This study received funding from Coordenação de Aperfeiçoamento de Pessoal de Nível Superior–CAPES (http://www.capes.gov.br), Finance Code: 001, Ph.D.; Programa de Doutorado Sanduíche no Exterior (PDSE)—CAPES, Process Number: 88881.357613/2019-01; Coordenação de Aperfeiçoamento de Pessoal de Nível Superior (CAPES), Edital CAPES 25/2014—Pró-Forenses, Project Number: 23038.006832/2014-11; Conselho Nacional de Desenvolvimento Científico e Tecnológico–CNPq (http://www.cnpq.br), Award Number: 140284/2018-4; The Rufford Foundation (https://www.rufford.org), Award Number: 23971-1; Programa de Pós-Graduação em Ecologia da Universidade de Brasília; Fundação de Apoio à Pesquisa do Distrito Federal (FAPDF), and the USAID’s PEER program under cooperative agreement AID-OAA-A-11-00012. The funders had no role in the study design, data collection, analysis, decision to publish, or manuscript preparation.
We thank all landowners and Conservation Units managers for permission and for being supportive during the development of fieldwork. We thank members of the SD, GN, and GC labs for significant comments, support, tips, and help during fieldwork, especially Bruno Araújo and Humberto Nappo. AP recognizes that Figure 1 was previously published in Pereira and Colli (2022).
The authors declare that the research was conducted in the absence of any commercial or financial relationships that could be construed as a potential conflict of interest.
All claims expressed in this article are solely those of the authors and do not necessarily represent those of their affiliated organizations, or those of the publisher, the editors and the reviewers. Any product that may be evaluated in this article, or claim that may be made by its manufacturer, is not guaranteed or endorsed by the publisher.
The Supplementary Material for this article can be found online at: https://www.frontiersin.org/articles/10.3389/fevo.2023.1053535/full#supplementary-material
Allan, J. D. (2004). Landscapes and riverscapes: The influence of land use on stream ecosystems. Annu. Rev. Ecol. Evol. Syst. 35, 257–284. doi: 10.1146/annurev.ecolsys.35.120202.110122
Araújo, M. L. S. D., Sano, E. E., Bolfe, ÉL., Santos, J. R. N., dos Santos, J. S., and Silva, F. B. (2019). Spatiotemporal dynamics of soybean crop in the Matopiba region, Brazil (1990–2015). Land Use Policy 80, 57–67. doi: 10.1016/j.landusepol.2018.09.040
Barão-Nóbrega, J. A. L., Marioni, B., Dutra-Araújo, D., Botero-Arias, R., Nogueira, A. J. A., Magnusson, W. E., et al. (2016). Nest attendance influences the diet of nesting female spectacled caiman (Caiman crocodilus) in Central Amazonia, Brazil. Herpetol. J. 26, 65–71.
Barretto, A. G., Berndes, G., Sparovek, G., and Wirsenius, S. (2013). Agricultural intensification in Brazil and its effects on land-use patterns: An analysis of the 1975-2006 period. Glob. Chang. Biol. 19, 1804–1815. doi: 10.1111/gcb.12174
Beaupre, S. J., Jacobson, E. R., Lillywhite, H. B., and Zamudio, K. (2004). Guidelines for use of live amphibians and reptiles in field and laboratory research. Norman: Herpetological Animal Care and Use Committee of the American Society of Ichthyologists and Herpetologist.
Ben-David, M., and Flaherty, E. A. (2012). Stable isotopes in mammalian research: A beginner’s guide. J. Mammal. 93, 312–328. doi: 10.1644/11-mamm-s-166.1
Bentivoglio, F., Calizza, E., Rossi, D., Carlino, P., Careddu, G., Rossi, L., et al. (2016). Site-scale isotopic variations along a river course help localize drainage basin influence on river food webs. Hydrobiologia 770, 257–272. doi: 10.1007/s10750-015-2597-2
Besser, A. C., Elliott Smith, E. A., and Newsome, S. D. (2022). Assessing the potential of amino acid δ13C and δ15N analysis in terrestrial and freshwater ecosystems. J. Ecol. 110, 935–950. doi: 10.1111/1365-2745.13853
Best, J. (2018). Anthropogenic stresses on the world’s big rivers. Nat. Geosci. 12, 7–21. doi: 10.1038/s41561-018-0262-x
Biggs, J., von Fumetti, S., and Kelly-Quinn, M. (2016). The importance of small waterbodies for biodiversity and ecosystem services: Implications for policy makers. Hydrobiologia 793, 3–39. doi: 10.1007/s10750-016-3007-0
Boecklen, W. J., Yarnes, C. T., Cook, B. A., and James, A. C. (2011). On the use of stable isotopes in trophic ecology. Annu. Rev. Ecol. Evol. Syst. 42, 411–440. doi: 10.1146/annurev-ecolsys-102209-144726
Bowes, R. E., Thorp, J. H., and Delong, M. D. (2019). Reweaving river food webs through time. Freshw. Biol. 65, 390–402. doi: 10.1111/fwb.13432
Brien, M., and Manolis, C. (2016). “Crocodilians,” in Reptile ecology and conservation, ed. C. K. Dodd (Oxford: Oxford University Press), 211–224.
Burdon, F. J., McIntosh, A. R., and Harding, J. (2020). Mechanisms of trophic niche compression: Evidence from landscape disturbance. J. Anim. Ecol. 89, 730–744. doi: 10.1111/1365-2656.13142
Campos, Z., Coutinho, M., Mourão, G., Bayliss, P., and Magnusson, W. E. (2006). Long distance movements by Caiman crocodilus yacare: Implications for management of the species in the Brazilian Pantanal. Herpetol. J. 16, 123–132.
Carranza, T., Balmford, A., Kapos, V., and Manica, A. (2014). Protected area effectiveness in reducing conversion in a rapidly vanishing ecosystem: The Brazilian Cerrado. Conserv. Lett. 7, 216–223. doi: 10.1111/conl.12049
Carvalho, D. R., Castro, D., Callisto, M., Moreira, M. Z., and Pompeu, P. S. (2015). Isotopic variation in five species of stream fishes under the influence of different land uses. J. Fish Biol. 87, 559–578. doi: 10.1111/jfb.12734
Caut, S. (2013). Isotope incorporation in broad-snouted caimans (crocodilians). Biol. Open 2, 629–634. doi: 10.1242/bio.20134945
Caut, S., Francois, V., Bacques, M., Guiral, D., Lemaire, J., Lepoint, G., et al. (2019). The dark side of the black caiman: Shedding light on species dietary ecology and movement in Agami Pond. French Guiana. PLoS One 14:e0217239. doi: 10.1371/journal.pone.0217239
Ceia, F. R., Paiva, V. H., Garthe, S., Marques, J. C., and Ramos, J. A. (2014). Can variations in the spatial distribution at sea and isotopic niche width be associated with consistency in the isotopic niche of a pelagic seabird species? Mar. Biol. 161, 1861–1872. doi: 10.1007/s00227-014-2468-9
Coe, M. T., Latrubesse, E. M., Ferreira, M. E., and Amsler, M. L. (2011). The effects of deforestation and climate variability on the streamflow of the Araguaia River, Brazil. Biogeochemistry 105, 119–131. doi: 10.1007/s10533-011-9582-2
Companhia Nacional de Abastecimento [CONAB] (2015). A cultura do arroz. Brasília: Companhia Nacional de Abastecimento.
Crawford, K., McDonald, R. A., and Bearhop, S. (2008). Applications of stable isotope techniques to the ecology of mammals. Mamm. Rev. 38, 87–107. doi: 10.1111/j.1365-2907.2008.00120.x
Da Silveira, R., and Magnusson, W. E. (1999). Diets of spectacled and black caiman in the Anavilhanas Archipelago, Central Amazonia, Brazil. J. Herpetol. 33, 181–192. doi: 10.2307/1565713
Davies, B. R., Biggs, J., Williams, P. J., Lee, J. T., and Thompson, S. (2008). A comparison of the catchment sizes of rivers, streams, ponds, ditches and lakes: Implications for protecting aquatic biodiversity in an agricultural landscape. Hydrobiologia 597, 7–17. doi: 10.1007/s10750-007-9227-6
Dias, L. C. P., Pimenta, F. M., Santos, A. B., Costa, M. H., and Ladle, R. J. (2016). Patterns of land use, extensification, and intensification of Brazilian agriculture. Glob. Chang. Biol. 22, 2887–2903. doi: 10.1111/gcb.13314
Doi, H., Zuykova, E. I., Shikano, S., Kikuchi, E., Ota, H., Yurlova, N. I., et al. (2013). Isotopic evidence for the spatial heterogeneity of the planktonic food webs in the transition zone between river and lake ecosystems. PeerJ 1:e222. doi: 10.7717/peerj.222
Driscoll, D. A., Banks, S. C., Barton, P. S., Lindenmayer, D. B., and Smith, A. L. (2013). Conceptual domain of the matrix in fragmented landscapes. Trends Ecol. Evol. 28, 605–613. doi: 10.1016/j.tree.2013.06.010
Duffy, J. E., Cardinale, B. J., France, K. E., McIntyre, P. B., Thébault, E., and Loreau, M. (2007). The functional role of biodiversity in ecosystems: Incorporating trophic complexity. Ecol. Lett. 10, 522–538. doi: 10.1111/j.1461-0248.2007.01037.x
Ewers, R. M., and Didham, R. K. (2006). Confounding factors in the detection of species responses to habitat fragmentation. Biol. Rev. 81, 117–142. doi: 10.1017/S1464793105006949
Fahrig, L. (2003). Effects of habitat fragmentation on biodiversity. Annu. Rev. Ecol. Evol. Syst. 34, 487–515. doi: 10.1146/annurev.ecolsys.34.011802.132419
Ferreira, M. E., Ferreira, L. G., Latrubesse, E. M., and Miziara, F. (2008). “High resolution remote sensing based quantification of the remnant vegetation cover in the Araguaia River Basin, Central Brazil,” in IGARSS 2008 - IEEE International Geoscience and Remote Sensing Symposium, (Piscataway: Institute of Electrical and Electronics Engineers), 739–741.
Finlay, J. C., and Kendall, C. (2008). “Stable isotope tracing of temporal and spatial variability in organic matter sources to freshwater ecosystems,” in Stable isotopes in ecology and environmental science, 2nd Edn, eds R. Michener and K. Lajtha (Oxford: Blackwell Publishing Ltd), 283–333.
Fischer, J., and Lindenmayer, D. B. (2007). Landscape modification and habitat fragmentation: A synthesis. Glob. Ecol. Biogeogr. 16, 265–280. doi: 10.1111/j.1466-8238.2007.00287.x
Fitzgerald, L. A. (2012). “Finding and capturing reptiles,” in Reptile biodiversity: Standard methods for inventory and monitoring, 1st Edn, eds R. W. McDiarmid, M. S. Foster, C. Guyer, J. W. Gibbons, and N. Chernoff (London: University of California Press), 77–80.
Fleming, G. J., and Fontenot, D. K. (2015). “Crocodilians (crocodiles, alligators, caiman, gharial),” in Fowler’s zoo and wild animal medicine, eds R. E. Miller and M. E. Fowler (St. Louis: Elsevier Inc), 38–49.
Fragoso, D. B., Cardoso, E. A., Souza, E. R., and Ferreira, C. M. (2013). Caracterização e diagnóstico da cadeia produtiva do arroz no Estado do Tocantins. Brasília: Embrapa Arroz e Feijão.
Garcia, A. S., Sawakuchi, H. O., Ferreira, M. E., and Ballester, M. V. R. (2017). Landscape changes in a neotropical forest-savanna ecotone zone in central Brazil: The role of protected areas in the maintenance of native vegetation. J. Environ. Manag. 187, 16–23. doi: 10.1016/j.jenvman.2016.11.010
García, L., Cross, W. F., Pardo, I., and Richardson, J. S. (2017). Effects of landuse intensification on stream basal resources and invertebrate communities. Freshw. Sci. 36, 609–625. doi: 10.1086/693457
Gorzula, S. J. (1978). An ecological study of Caiman crocodilus crocodilus inhabiting savanna lagoons in the Venezuelan Guayana. Oecologia 35, 21–34. doi: 10.1007/bf00345539
Haddad, N. M., Brudvig, L. A., Clobert, J., Davies, K. F., Gonzalez, A., Holt, R. D., et al. (2015). Habitat fragmentation and its lasting impact on Earth’s ecosystems. Sci. Adv. 1:e1500052. doi: 10.1126/sciadv.1500052
Herzon, I., and Helenius, J. (2008). Agricultural drainage ditches, their biological importance and functioning. Biol. Conserv. 141, 1171–1183. doi: 10.1016/j.biocon.2008.03.005
Hesselbarth, M. H. K., Sciaini, M., With, K. A., Wiegand, K., and Nowosad, J. (2019). landscapemetrics: An open-source R tool to calculate landscape metrics. Ecography 42, 1648–1657. doi: 10.1111/ecog.04617
Hooper, D. U., Adair, E. C., Cardinale, B. J., Byrnes, J. E., Hungate, B. A., Matulich, K. L., et al. (2012). A global synthesis reveals biodiversity loss as a major driver of ecosystem change. Nature 486, 105–108. doi: 10.1038/nature11118
Hunke, P., Mueller, E. N., Schroeder, B., and Zeilhofer, P. (2014). The Brazilian Cerrado: Assessment of water and soil degradation in catchments under intensive agricultural use. Ecohydrology 8, 1154–1180. doi: 10.1002/eco.1573
Irion, G., Nunes, G. M., Nunes-da-Cunha, C., de Arruda, E. C., Santos-Tambelini, M., Dias, A. P., et al. (2016). Araguaia River floodplain: Size, age, and mineral composition of a large tropical savanna wetland. Wetlands 36, 945–956. doi: 10.1007/s13157-016-0807-y
Jackson, A. L., Inger, R., Parnell, A. C., and Bearhop, S. (2011). Comparing isotopic niche widths among and within communities: SIBER - Stable Isotope Bayesian Ellipses in R. J. Anim. Ecol. 80, 595–602. doi: 10.1111/j.1365-2656.2011.01806.x
Jaeger, J. A. G. (2000). Landscape division, splitting index, and effective mesh size: New measures of landscape fragmentation. Landsc. Ecol. 15, 115–130. doi: 10.1023/A:1008129329289
Jardine, T. D., Rayner, T. S., Pettit, N. E., Valdez, D., Ward, D. P., Lindner, G., et al. (2017). Body size drives allochthony in food webs of tropical rivers. Oecologia 183, 505–517. doi: 10.1007/s00442-016-3786-z
Kim, S. L., and Koch, P. L. (2012). Methods to collect, preserve, and prepare elasmobranch tissues for stable isotope analysis. Environ. Biol. Fishes 95, 53–63. doi: 10.1007/s10641-011-9860-9
Kursa, M. B., and Rudnicki, W. R. (2010). Feature selection with the Boruta package. J. Stat. Softw. 36, 1–13. doi: 10.18637/jss.v036.i11
Larsen, T., Taylor, D. L., Leigh, M. B., and O’Brien, D. M. (2009). Stable isotope fingerprinting: A novel method for identifying plant, fungal, or bacterial origins of amino acids. Ecology 90, 3526–3535. doi: 10.1890/08-1695.1
Larsen, T., Ventura, M., Andersen, N., O’Brien, D. M., Piatkowski, U., and McCarthy, M. D. (2013). Tracing carbon sources through aquatic and terrestrial food webs using amino acid stable isotope fingerprinting. PLoS One 8:e73441. doi: 10.1371/journal.pone.0073441
Latrubesse, E. M., Amsler, M. L., de Morais, R. P., and Aquino, S. (2009). The geomorphologic response of a large pristine alluvial river to tremendous deforestation in the South American tropics: The case of the Araguaia River. Geomorphology 113, 239–252. doi: 10.1016/j.geomorph.2009.03.014
Laurance, W. F., Sayer, J., and Cassman, K. G. (2014). Agricultural expansion and its impacts on tropical nature. Trends Ecol. Evol. 29, 107–116. doi: 10.1016/j.tree.2013.12.001
Layman, C. A., Quattrochi, J. P., Peyer, C. M., Allgeier, J. E., and Suding, K. (2007). Niche width collapse in a resilient top predator following ecosystem fragmentation. Ecol. Lett. 10, 937–944. doi: 10.1111/j.1461-0248.2007.01087.x
Le Provost, G., Badenhausser, I., Le Bagousse-Pinguet, Y., Clough, Y., Henckel, L., Violle, C., et al. (2020). Land-use history impacts functional diversity across multiple trophic groups. Proc. Natl. Acad. Sci. U.S.A. 117, 1573–1579. doi: 10.1073/pnas.1910023117
Leal, C. G., Lennox, G. D., Ferraz, S. F. B., Ferreira, J., Gardner, T. A., Thomson, J. R., et al. (2020). Integrated terrestrial-freshwater planning doubles conservation of tropical aquatic species. Science 370, 117–121. doi: 10.1126/science.aba7580
Lemes, L., de Andrade, A. F. A., and Loyola, R. (2019). Spatial priorities for agricultural development in the Brazilian Cerrado: May economy and conservation coexist? Biodivers. Conserv. 29, 1683–1700. doi: 10.1007/s10531-019-01719-6
Liao, J., Bearup, D., and Blasius, B. (2017b). Food web persistence in fragmented landscapes. Proc. R. Soc. B-Biol. Sci. 284:1859. doi: 10.1098/rspb.2017.0350
Liao, J., Bearup, D., and Blasius, B. (2017a). Diverse responses of species to landscape fragmentation in a simple food chain. J. Anim. Ecol. 86, 1169–1178. doi: 10.1111/1365-2656.12702
Lindgren, F., and Rue, H. (2015). Bayesian spatial modelling with R-INLA. J. Stat. Softw. 63:25. doi: 10.18637/jss.v063.i19
Lindgren, F., Rue, H., and Lindström, J. (2011). An explicit link between Gaussian fields and Gaussian Markov random fields: The stochastic partial differential equation approach. J. R. Stat. Soc. Ser. B 73, 423–498. doi: 10.1111/j.1467-9868.2011.00777.x
Logan, J. M., Jardine, T. D., Miller, T. J., Bunn, S. E., Cunjak, R. A., and Lutcavage, M. E. (2008). Lipid corrections in carbon and nitrogen stable isotope analyses: Comparison of chemical extraction and modelling methods. J. Anim. Ecol. 77, 838–846. doi: 10.1111/j.1365-2656.2008.01394.x
Magioli, M., Moreira, M. Z., Fonseca, R. C. B., Ribeiro, M. C., Rodrigues, M. G., and Ferraz, K. (2019). Human-modified landscapes alter mammal resource and habitat use and trophic structure. Proc. Natl. Acad. Sci. U.S.A. 116, 18466–18472. doi: 10.1073/pnas.1904384116
Magnusson, W. E., Da Silva, E. V., and Lima, A. P. (1987). Diets of Amazonian crocodilians. J. Herpetol. 21, 85–95. doi: 10.2307/1564468
Manlick, P. J., and Newsome, S. D. (2022). Stable isotope fingerprinting traces essential amino acid assimilation and multichannel feeding in a vertebrate consumer. Methods Ecol. Evol. 13, 1819–1830. doi: 10.1111/2041-210X.13903
Marques, E. Q., Marimon-Junior, B. H., Marimon, B. S., Matricardi, E. A. T., Mews, H. A., and Colli, G. R. (2020). Redefining the cerrado-amazonia transition: Implications for conservation. Biodivers. Conserv. 29, 1501–1517. doi: 10.1007/s10531-019-01720-z
Marques, T. S., Bassetti, L. A. B., Lara, N. R. F., Portelinha, T. C. G., Piña, C. I., and Verdade, L. M. (2020). Home range and movement pattern of the broad-snouted caiman (Caiman latirostris) in a silviculture dominated landscape. South Am. J. Herpetol. 16, 16–25. doi: 10.2994/sajh-d-18-00052.1
Marques, T. S., Bassetti, L. A. B., Lara, N. R. F., Araujo, M. S., Piña, C. I., Camargo, P. B., et al. (2014). Isotopic discrimination factors (Δ15N and Δ13C) between tissues and diet of the broad-snouted caiman (Caiman latirostris). J. Herpetol. 48, 332–337. doi: 10.1670/12-274
Marques, T. S., Lara, N. R. F., Bassetti, L. A. B., Piña, C. I., Camargo, P. B., and Verdade, L. M. (2013). Intraspecific isotopic niche variation in broad-snouted caiman (Caiman latirostris). Isot. Environ. Health Stud. 49, 325–335. doi: 10.1080/10256016.2013.835309
Martinelli, L. A., Ometto, J. P. H. B., Ishida, F. Y., Domingues, T. F., Nardoto, G. B., Oliveira, R. S., et al. (2007). “The use of carbon and nitrogen stable isotopes to track effects of land-use changes in the Brazilian Amazon region,” in Terrestrial ecology, eds T. E. Dawson and R. T. W. Siegwolf (New York, NY: Elsevier), 301–318.
McGarigal, K., and Marks, B. J. (1995). “FRAGSTATS: Spatial pattern analysis program for quantifying landscape structure,” in USDA Forest Service General Technical Report PNW-GTR-351, ed. U.S. Department of Agriculture, Forest Service, Pacific Northwest Research Station (Portland, OR: U.S. Department of Agriculture, Forest Service, Pacific Northwest Research Station).
McMahon, K. W., Polito, M. J., Abel, S., McCarthy, M. D., and Thorrold, S. R. (2015). Carbon and nitrogen isotope fractionation of amino acids in an avian marine predator, the gentoo penguin (Pygoscelis papua). Ecol. Evol. 5, 1278–1290. doi: 10.1002/ece3.1437
Merlo-Galeazzi, A., and Zambrano, L. (2014). The effect of land use on isotope signatures of the detritus pathway in an urban wetland system. Wetlands 34, 1183–1190. doi: 10.1007/s13157-014-0577-3
Millennium Ecosystem Assessment (2005). Ecosystems and human well-being: Wetlands and water synthesis. Washington, DC: World Resources Institute.
Naimi, B., Hamm, N. A. S., Groen, T. A., Skidmore, A. K., and Toxopeus, A. G. (2014). Where is positional uncertainty a problem for species distribution modelling? Ecography 37, 191–203. doi: 10.1111/j.1600-0587.2013.00205.x
Newbold, T., Hudson, L. N., Hill, S. L., Contu, S., Lysenko, I., Senior, R. A., et al. (2015). Global effects of land use on local terrestrial biodiversity. Nature 520, 45–50. doi: 10.1038/nature14324
Newsome, S. D., Martínez del Rio, C., Bearhop, S., and Phillips, D. L. (2007). A niche for isotopic ecology. Front. Ecol. Environ. 5, 429–436. doi: 10.1890/060150.1
Nifong, J. C., Layman, C. A., and Silliman, B. R. (2015). Size, sex and individual-level behaviour drive intrapopulation variation in cross-ecosystem foraging of a top-predator. J. Anim. Ecol. 84, 35–48. doi: 10.1111/1365-2656.12306
Oliveira, T. A., Viola, M. R., Mello, C. R., Giongo, M., and Coelho, G. (2015). Natural vulnerability of water resources in the Formoso River Basin, Northern Brazil. Afr. J. Agric. Res. 10, 1107–1114. doi: 10.5897/ajar2014.9370
Ouboter, P. E., and Nanhoe, L. M. R. (1988). Habitat selection and migration of Caiman crocodilus crocodilus in a swamp and swamp-forest habitat in northern Suriname. J. Herpetol. 22, 283–294. doi: 10.2307/1564151
Parreira de Castro, D. M., Reis de Carvalho, D., Pompeu, P. D. S., Moreira, M. Z., Nardoto, G. B., and Callisto, M. (2016). Land use influences niche size and the assimilation of resources by benthic macroinvertebrates in tropical headwater streams. PLoS One 11:e0150527. doi: 10.1371/journal.pone.0150527
Penone, C., Davidson, A. D., Shoemaker, K. T., Di Marco, M., Rondinini, C., Brooks, T. M., et al. (2014). Imputation of missing data in life-history trait datasets: Which approach performs the best? Methods Ecol. Evol. 5, 961–970. doi: 10.1111/2041-210x.12232
Pereira, A. C., and Colli, G. R. (2022). Landscape features affect caiman body condition in the middle Araguaia River floodplain. Anim. Conserv. doi: 10.1111/acv.12841
Pereira, A. C., Nardoto, G. B., and Colli, G. R. (2018). “Intraspecific variation and spatial-temporal differences in the isotopic niche of Caiman crocodilus (spectacled caiman) in an agricultural landscape,” in Proceedings of the 25th working meeting of the IUCN-SSC Crocodile Specialist Group convened at Santa Fe, Argentina (Gland: IUCN), 72.
Phalan, B., Bertzky, M., Butchart, S. H., Donald, P. F., Scharlemann, J. P., Stattersfield, A. J., et al. (2013). Crop expansion and conservation priorities in tropical countries. PLoS One 8:e51759. doi: 10.1371/journal.pone.0051759
Pillai, P., Gonzalez, A., and Loreau, M. (2011). Metacommunity theory explains the emergence of food web complexity. Proc. Natl. Acad. Sci. U.S.A. 108, 19293–19298. doi: 10.1073/pnas.1106235108
Plummer, M. V., and Ferner, J. W. (2012). “Marking reptiles,” in Reptile biodiversity: Standard methods for inventory and monitoring, 1st Edn, eds R. W. McDiarmid, M. S. Foster, C. Guyer, J. W. Gibbons, and N. Chernoff (Berkeley, CA: University of California Press), 143–150.
Post, D. M., Layman, C. A., Arrington, D. A., Takimoto, G., Quattrochi, J., and Montaña, C. G. (2007). Getting to the fat of the matter: Models, methods and assumptions for dealing with lipids in stable isotope analyses. Oecologia 152, 179–189. doi: 10.1007/s00442-006-0630-x
Price, E. L., Sertic Peric, M., Romero, G. Q., and Kratina, P. (2019). Land use alters trophic redundancy and resource flow through stream food webs. J. Anim. Ecol. 88, 677–689. doi: 10.1111/1365-2656.12955
QGIS Development Team (2020). QGIS Geographic Information System”. 3, 12 Edn. Chicago, IL: Open Source Geospatial Foundation Project.
Quesnelle, P. E., Lindsay, K. E., and Fahrig, L. (2015). Relative effects of landscape-scale wetland amount and landscape matrix quality on wetland vertebrates: A meta-analysis. Ecol. Appl. 25, 812–825. doi: 10.1890/14-0362.1
R Development Core Team (2021). R: A language and environment for statistical computing, 4.1.0 Edn. Vienna: R Foundation for Statistical Computing.
Radloff, F. G., Hobson, K. A., and Leslie, A. J. (2012). Characterising ontogenetic niche shifts in Nile crocodile using stable isotope (δ13C, δ15N) analyses of scute keratin. Isot. Environ. Health Stud. 48, 439–456. doi: 10.1080/10256016.2012.667808
Ramsar Convention [RAMSAR] (2002). Ramsar sites information service - Ilha do Bananal no. 624. Gland: Ramsar Convention.
Reddin, C. J., Bothwell, J. H., O’Connor, N. E., Harrod, C., and Briones, M. J. (2018). The effects of spatial scale and isoscape on consumer isotopic niche width. Funct. Ecol. 32, 904–915. doi: 10.1111/1365-2435.13026
Redding, D. W., Lucas, T. C. D., Blackburn, T. M., and Jones, K. E. (2017). Evaluating Bayesian spatial methods for modelling species distributions with clumped and restricted occurrence data. PLoS One 12:e0187602. doi: 10.1371/journal.pone.0187602
Reed, R. N., and Tucker, A. D. (2012). “Determining age, sex, and reproductive condition,” in Reptile biodiversity: Standard methods for inventory and monitoring, eds R. W. McDiarmid, M. S. Foster, C. Guyer, J. W. Gibbons, and N. Chernoff (Berkeley,CA: University of California Press), 151–163.
Resasco, J., Tuff, K. T., Cunningham, S. A., Melbourne, B. A., Hicks, A. L., Newsome, S. D., et al. (2017). Generalist predator’s niche shifts reveal ecosystem changes in an experimentally fragmented landscape. Ecogrjaphy 41, 1209–1219. doi: 10.1111/ecog.03476
Riva, F., and Nielsen, S. E. (2020). Six key steps for functional landscape analyses of habitat change. Landsc. Ecol. 35, 1495–1504. doi: 10.1007/s10980-020-01048-y
Rooney, N., McCann, K. S., and Moore, J. C. (2008). A landscape theory for food web architecture. Ecol. Lett. 11, 867–881. doi: 10.1111/j.1461-0248.2008.01193.x
Rosenblatt, A. E., Heithaus, M. R., Mather, M. E., Matich, P., Nifong, J. C., Ripple, W. J., et al. (2013). The roles of large top predators in coastal ecosystems: New insights from long-term ecological research. Oceanography 26, 156–167. doi: 10.5670/oceanog.2013.59
Rue, H., Martino, S., and Chopin, N. (2009). Approximate Bayesian inference for latent Gaussian models by using integrated nested Laplace approximations. J. R. Stat. Soc. Ser. B 71, 319–392. doi: 10.1111/j.1467-9868.2008.00700.x
Scheffer, M., Carpenter, S., Foley, J. A., Folke, C., and Walker, B. (2001). Catastrophic shifts in ecosystems. Nature 413, 591–596.
Silfer, J. A., Engel, M. H., Macko, S. A., and Jumeau, E. J. (1991). Stable carbon isotope analysis of amino acid enantiomers by conventional isotope ratio mass spectrometry and combined gas chromatography/isotope ratio mass spectrometry. Anal. Chem. 63, 370–374. doi: 10.1021/ac00004a014
Somaweera, R., Nifong, J., Rosenblatt, A., Brien, M. L., Combrink, X., Elsey, R. M., et al. (2020). The ecological importance of crocodylians: Towards evidence-based justification for their conservation. Biol. Rev. 95, 936–959. doi: 10.1111/brv.12594
Stekhoven, D. J., and Buühlmann, P. (2012). MissForest – non-parametric missing value imputation for mixed-type data. Bioinformatics 28, 112–118. doi: 10.1093/bioinformatics/btr597
Strassburg, B. B. N., Brooks, T., Feltran-Barbieri, R., Iribarrem, A., Crouzeilles, R., Loyola, R., et al. (2017). Moment of truth for the Cerrado hotspot. Nat. Ecol. Evol. 1, 1–3. doi: 10.1038/s41559-017-0099
Thorbjarnarson, J. (1997). Are crocodilian sex ratios female biased? The data are equivocal. Copeia 1997, 451–455. doi: 10.2307/1447771
Thorbjarnarson, J. B. (1993). Diet of the spectacled caiman (Caiman crocodilus) in the central Venezuelan Llanos. Herpetologica 49, 108–117.
Thorp, J. H., and Bowes, R. E. (2016). Carbon sources in riverine food webs: New evidence from amino acid isotope techniques. Ecosystems 20, 1029–1041.
Tscharntke, T., Tylianakis, J. M., Rand, T. A., Didham, R. K., Fahrig, L., Batary, P., et al. (2012). Landscape moderation of biodiversity patterns and processes - eight hypotheses. Biol. Rev. 87, 661–685. doi: 10.1111/j.1469-185X.2011.00216.x
Vander Zanden, M. J., Clayton, M. K., Moody, E. K., Solomon, C. T., and Weidel, B. C. (2015). Stable isotope turnover and half-life in animal tissues: A literature synthesis. PLoS One 10:e0116182. doi: 10.1371/journal.pone.0116182
Venables, W. N., and Ripley, B. D. (2002). Modern applied statistics with S. New York, NY: Springer.
Villamarín, F., Jardine, T. D., Bunn, S. E., Marioni, B., and Magnusson, W. E. (2017). Opportunistic top predators partition food resources in a tropical freshwater ecosystem. Freshw. Biol. 62, 1389–1400. doi: 10.1111/fwb.12952
Villard, M.-A., Metzger, J. P., and Saura, S. (2014). Beyond the fragmentation debate: A conceptual model to predict when habitat configuration really matters. J. Appl. Ecol. 51, 309–318. doi: 10.1111/1365-2664.12190
Wang, X., Blanchet, F. G., and Koper, N. (2014). Measuring habitat fragmentation: An evaluation of landscape pattern metrics. Methods Ecol. Evol. 5, 634–646. doi: 10.1111/2041-210X.12198
Wang, X., Yue, Y. R., and Faraway, J. J. (2018). Bayesian regression modeling with INLA. New York, NY: CRC Press.
Wantzen, K. M., Fellerhoff, C., and Voss, M. (2010). “Stable isotope ecology of the food webs of the Pantanal,” in The Pantanal: Ecology, biodiversity and sustainable management of a large neotropical seasonal wetland, eds W. Junk, C. J. Silva, and C. Nunes da Cunha (Sofia: Pensoft Publishers), 597–616.
Whiteman, J. P., Smith, E. A. E., Besser, A. C., and Newsome, S. D. (2019). A guide to using compound-specific stable isotope analysis to study the fates of molecules in organisms and ecosystems. Diversity 11:18. doi: 10.3390/d11010008
Zaia Alves, G. H., Hoeinghaus, D. J., Manetta, G. I., and Benedito, E. (2017). Dry season limnological conditions and basin geology exhibit complex relationships with delta C-13 and delta N-15 of carbon sources in four Neotropical floodplains. PLoS One 12:e0174499. doi: 10.1371/journal.pone.0174499
Keywords: Araguaia floodplain, compound-specific stable isotope analysis, crocodilian, essential amino acids, hierarchical Bayesian modeling, pasture, spatial analysis
Citation: Pereira AC, Mancuso CJ, Newsome SD, Nardoto GB and Colli GR (2023) Agricultural input modifies trophic niche and basal energy source of a top predator across human-modified landscapes. Front. Ecol. Evol. 11:1053535. doi: 10.3389/fevo.2023.1053535
Received: 25 September 2022; Accepted: 05 May 2023;
Published: 01 June 2023.
Edited by:
Carlos Freitas, Federal University of Amazonas, BrazilReviewed by:
Jamerson Aguiar-Santos, Federal University of Maranhão, BrazilCopyright © 2023 Pereira, Mancuso, Newsome, Nardoto and Colli. This is an open-access article distributed under the terms of the Creative Commons Attribution License (CC BY). The use, distribution or reproduction in other forums is permitted, provided the original author(s) and the copyright owner(s) are credited and that the original publication in this journal is cited, in accordance with accepted academic practice. No use, distribution or reproduction is permitted which does not comply with these terms.
*Correspondence: André C. Pereira, cmFuY29wZXJAZ21haWwuY29t
Disclaimer: All claims expressed in this article are solely those of the authors and do not necessarily represent those of their affiliated organizations, or those of the publisher, the editors and the reviewers. Any product that may be evaluated in this article or claim that may be made by its manufacturer is not guaranteed or endorsed by the publisher.
Research integrity at Frontiers
Learn more about the work of our research integrity team to safeguard the quality of each article we publish.