- 1Laboratory of Biocorrosion and Biotechnology, Corporación para la Investigación de la Corrosión (CIC), Piedecuesta, Colombia
- 2Research Group in Industrial Biotechnology and Molecular Biology – CINBIN, Escuela de Biología, Facultad de Ciencias, Universidad Industrial de Santander (UIS), Bucaramanga, Colombia
- 3Department of Entomology, National Coffee Research Center (Cenicafé), Manizales, Colombia
A country’s biodiversity is a key resource for the development of a sustainable bioeconomy. However, often the most biodiverse countries on the planet hardly profit from their biological diversity. On the contrary, occasionally components of that biodiversity become a threat to society and its food sustainability. That is the case of the sugarcane borer Diatraea saccharalis. Here, the analysis of the bacteria associated with the digestive tract of D. saccharalis reveals a rich and diverse microbiota. Two types of diets were analyzed under laboratory conditions. The metataxonomic analysis revealed a number of taxa common to most of the larval pools analyzed with relative abundances exceeding 5%, and five families of bacteria which have also been reported in the gut of another Lepidoptera. A large fraction of microorganisms detected by amplicon sequencing were considered to be rare and difficult to cultivate. However, among the cultivable microorganisms, 12 strains with relevant biotechnological features were identified. The strain that showed the highest cellulolytic activity (GCEP-101) was genome sequenced. The analysis of the GCEP-101 complete genome revealed that the values of 16S rRNA identity, the Average Nucleotide Identity, and the digital DNA–DNA hybridization place the strain as a candidate for a new species within the genus Pseudomonas. Moreover, the genome annotation of the putative new species evidenced the presence of genes associated with cellulose degradation, revealing the hidden potential of the pest as a reservoir of biotechnologically relevant microorganisms.
1. Introduction
The class Insecta comprises a vast group of organisms with remarkable adaptive capabilities that have favored their colonization of the biosphere. The evolutionary success of insects largely relies on the degree of specialization of their gut microbiota (Poveda, 2019). The number of microorganisms present in an insect’s intestinal tract is estimated to be up to 10 times larger than the number of insect cells. This translates into an estimate of 100 times more microbial genes than animal genes within one organism (Rajagopal, 2009; Engel and Moran, 2013; Gurung et al., 2019; Gupta and Nair, 2020). Apart for being main pollinators, several insect species and their microbiomes are considered major threats to several crops that are crucial to humans. Sugarcane, being one of the most cultivated crops globally, is vulnerable to pests such as Diatraea saccharalis, commonly known as the sugarcane borer (Beuzelin et al., 2014; Joyce et al., 2014; Daquila et al., 2019). In its larval stage, D. saccharalis efficiently penetrates deep into the stalks establishing galleries in which it continues its life cycle, thereby causing large economic losses (Vargas and Gómez, 2005; Lopes et al., 2014; Simões et al., 2015).
Notwithstanding the impact of D. saccharalis, to our knowledge there are no metagenomics or metataxonomics reports describing its gut microbiome. Most of the existing research is based on the characterization of cultivable microbes made while scouting for organisms capable of exerting biological control over the pest itself (Tan et al., 2011; Mahmoud et al., 2012; Davolos et al., 2015). Still, some authors have been interested in D. saccharalis as a source of microorganisms with biotechnological relevance (Barbosa et al., 2020; da Silva et al., 2022). Among the potentially relevant microbes are those involved in the copious degradation of cellulose within D. saccharalis’ gut. The latter since there is a global need for biomass processing as a means to both reduce the environmental impact of some industrial and agricultural processes and diversify the sources for energy generation (Dantur et al., 2015). In this work, we describe the first insights into the gut microbiome of D. saccharalis by using 16S rRNA gene analysis and culturing techniques. This approach represents an opportunity for the discovery of previously undescribed microorganisms bearing catalytic activities of industrial interest.
2. Materials and methods
2.1. Obtention and processing of Diatraea saccharalis
Eggs of D. saccharalis were purchased from the Colombian Agricultural Research Corporation (AGROSAVIA – Bogotá, Colombia). These were separated into two groups and incubated until hatching in hermetically closed containers at room temperature. Upon hatching one group was fed with an artificial diet provided by AGROSAVIA containing per liter: 8 g of wheat germ, 11.25 g of milled corn, 8 g of casein, 40 g of beer yeast, 20 g of pulverized sugar, 40 g of milled carrot, 80 g of pulverized sugarcane sheath, and 3.75 g of agar. The second group of larvae was fed with natural non-processed sugarcane pieces obtained from a local farm in the municipality of Piedecuesta, Santander, Colombia.
After 10 to 15 days at room temperature, the complete digestive tract of in average 80 larvae from each incubation was dissected under sterile conditions. In each case, approximately 60 tracts were placed into 1.5 mL centrifuge tubes and preserved at −80 °C for DNA extraction, while the remaining 20 were placed in sterile PBS for microbial cultivation.
2.2. Microbial enrichment and isolation
Approximately 20 digestive tracts from each incubation were macerated with a sterile swab until a homogeneous solution was obtained. The macerated material was then inoculated in liquid minimal media suitable for recovering uncommon slow-growing microorganisms (Kato et al., 2018; Chaudhary et al., 2019; Kurm et al., 2019). The base medium used was M9 as described by Reasoner and Geldreich, 1985; and modified as follows: 6.05 mM K2HPO4, 3.95 mM KH2PO4, 0.855 mM NaCl, 10 mM NH4Cl, 1 mM MgSO4, 0.3 mM CaCl2, 0.134 mM EDTA, 0.311 mM FeCl3, 6.2 μM ZnSO4, 0.76 μM CuSO4, 1.62 μM H3BO3, 1.65 μM Na2MoO4, 0.6 μM KI, and 0.1 g/L of yeast extract. All chemicals were purchased from Merck, Germany. The pH was adjusted to 7.2 ± 0.2 before autoclaving at 121 °C for 20 min. To favor the enrichment of microorganisms with cellulolytic activities, the medium was supplemented with a separately autoclaved carboxymethylcellulose (CMC) solution added to a concentration of 2 g/L as only carbon source. The 50 mL liquid enrichments were incubated for 15 days at room temperature and then transferred to solid modified M9 medium. Cellulose-degrading colonies were identified and isolated after showing a surrounding halo when stained with a Congo red solution (McDonald et al., 2012; Dantur et al., 2015). In addition, isolated colonies were tested for growth on solid M9 medium with 2 g/L of sawdust as only carbon source.
2.3. DNA extraction and sequencing
Metagenomic DNA was extracted from the dissected digestive tracts with the DNeasy® PowerSoil® - Qiagen kit following the manufacturer’s instructions. Subsequently, the DNA samples were sent to Novogene Corporation Inc. (Stockton Blvd, California, United States) for partially sequencing the 16S rRNA gene with 250 bp paired-end reads using the primers 341F (5’-CCTAYGGGRBGCASCAG) and 806R (5’-GGACTACNNGGGTATCTAAT) with the Illumina NovaSeq 6000 platform (Illumina, United States).
Genomic DNA of pure isolates was extracted as described by Martín-Platero et al. (2007). Sequencing of the 16S rRNA gene of all isolates was carried out with a MinION Mk1C device (Oxford Nanopore Technologies Inc., United Kingdom), using the 16S Barcoding Sequencing Kit 1–24 (SQK-16S024) and FLO-MIN106 (R9.4.1) flow cells. The obtained sequences were compared with the GenBank DNA database release 251.0 (Benson et al., 2013) and the Ribosomal Database Project release Taxonomy 18 (Cole et al., 2014). In addition, a combination of Illumina NovaSeq 6000 (PE150) and Nanopore MinION platforms was used to sequence the genome of the cellulolytic strain GCEP-101. The latter was performed by using the Ligation Sequencing Kit (SQK-LSK109). MinION data was gathered and basecalled with MinKNOW 21.10.8.
2.4. Bioinformatic analysis
2.4.1. Metataxonomic composition of the intestines with the amplicon 16S rRNA
Demultiplexed Illumina FASTQ sequences were analyzed using QIIME2 v.2020.8 (Bolyen et al., 2019). First, paired-end read sequences were filtered by quality, denoised, truncated at 220 bases and clustered using DADA2 (Callahan et al., 2016). The Amplicon Sequence Variants (ASV) were classified at taxonomic level using a Naive Bayes classifier built with the SILVA 138_2 database (Robeson et al., 2020). ASVs identified as chloroplast or mitochondria were removed from the representative sequences and excluded from further analyses. The output tables for ASV abundance and taxonomy were processed with R v.4.2.1 (Team, R C, 2022) using phyloseq v.1.3.20 (McMurdie and Holmes, 2013). Alpha-diversity was measured as Shannon (H′) and “Chao1” indexes. Statistical differences between diets were assessed with Mann–Whitney U test. Beta-diversity was measured using Bray-Curtis distance. Statistical differences for beta diversity between diets was assessed with the permutational multivariate analysis of variance (PERMANOVA) and the analysis of similarities (ANOSIM).
2.4.2. Whole genome assembly and annotation
The raw reads from both sequencing platforms were quality filtered with FastQC v.0.11.9 (Andrews, 2010). High-quality reads were hybrid-assembled de novo with Unicycler v 0.4.8 (Wick et al., 2017). Subsequently, the functional annotation of the genome was performed initially with Prokka v. 1.14.6 (Seemann, 2014), RAST v. 2.0 (Aziz et al., 2008) and PATRIC v. 3.6.12 (Davis et al., 2020). Finally, BlastKOALA annotation tool V2.2 was used to predict genes involved in cellulose degradation. The KEGG Orthology assignments were made against the KEGG prokaryotes gene database at the genus level (Kanehisa et al., 2016).
2.4.3. Genome-based phylogenetic analysis and in-silico species delineation
The genomic similarity between the strain GCEP-101 and the closest strains from the NCBI database were calculated using the Orthologous Average Nucleotide Identity (OrthoANI) Tool (OAT v. 0.93.1; Lee et al., 2016). To explore the existence of a putative new species, the Genome-to-Genome Distance Calculator (GGDC) and the Type (Strain) Genome Server (TYGS) provided by the German Collection of Microorganisms and Cell Cultures (DSMZ) was used (Meier-Kolthoff and Göker, 2019). The determination of the closest type strain genomes was carried out in two complementary ways: First, the GCEP-101 genome was compared against all type strains available in the TYGS database with the MASH algorithm (Ondov et al., 2016), from which the 10 type strains with the smallest MASH distances were chosen. Secondly, the 16S rDNA sequences were extracted from the GCEP-101 genome using RNAmmer (Lagesen et al., 2007) and BLASTed (Camacho et al., 2009) against the 16S rRNA gene sequences of the 16,976 type strains in the TYGS database (as for Jul.22.22). The 10 closest type strains were identified using the Genome BLAST Distance Phylogeny approach (GBDP) (Meier-Kolthoff et al., 2013). Digital DNA–DNA hybridization (dDDH) values and confidence intervals were calculated using the recommended settings of the GGDC 3.0 (Meier-Kolthoff et al., 2013, 2022). The genome and closest relatives were visualized using BLAST Ring Image Generator (BRIG v. 0.95; Alikhan et al., 2011). In addition, intergenomic distances were used to infer a balanced minimum evolution tree with branch support via FASTME 2.1.6.1 including SPR postprocessing (Lefort et al., 2015). Branch support was inferred from 100 pseudobootstrap replicates. The tree was rooted (Farris, 1972) and visualized with PhyD3 (Kreft et al., 2017).
3. Results
3.1. Diversity and composition of the gut microbiota of Diatraea saccharalis
We profiled the gut microbiota of 388 D. saccharalis individuals fed with two distinct diets. In total 231,943 reads from the V3-V4 region of the 16S rRNA gene were analyzed. Nine hundred and twenty-nine amplicon sequence variants (ASVs) with an average length of 395 bp were identified. After removing sequences considered artifacts (ASVs present in only 1 sample), 361 ASVs were selected for taxonomic distribution and diversity analysis. The saturation of the rarefaction curves indicated that most of the bacterial biodiversity was sampled during sequencing (Supplementary Figure S1). In seven of the larval pools Proteobacteria were the most abundant phylum (Figure 1A). The exception was one sample fed with an artificial diet where the most abundant phylum was Firmicutes. At the order level, representatives from Rhizobiales and Micrococcales were present throughout all analyzed samples. Two of the sugarcane diet microbiomes showed dominance of organisms within the order Rickettsiales (Figure 1B). At family and genus level, representatives of Rhizobiaceae and Wolbachia dominated in several samples, accompanied by a large number of taxa present in less than 1% of the sequences (Figures 1C,D). Alpha diversity for D. saccharalis gut microbiota was similar when insects were reared on artificial or natural diets. Species richness (Chao1, Mann–Whitney value of p = 0.0714) and species diversity (Shannon, Mann–Whitney value of p = 0.3929) did not resulted in statistically significant differences between diets. Community structure of gut microbiome among diet treatments also was stable and did not result in statistical differences as measured by PERMANOVA (Bray-Curtis distance; F-value = 1.105; R-square = 0.16324; value of p = 0.215) and ANOSIM (Bray-Curtis distance; R = 0.10769; value of p <0.231).
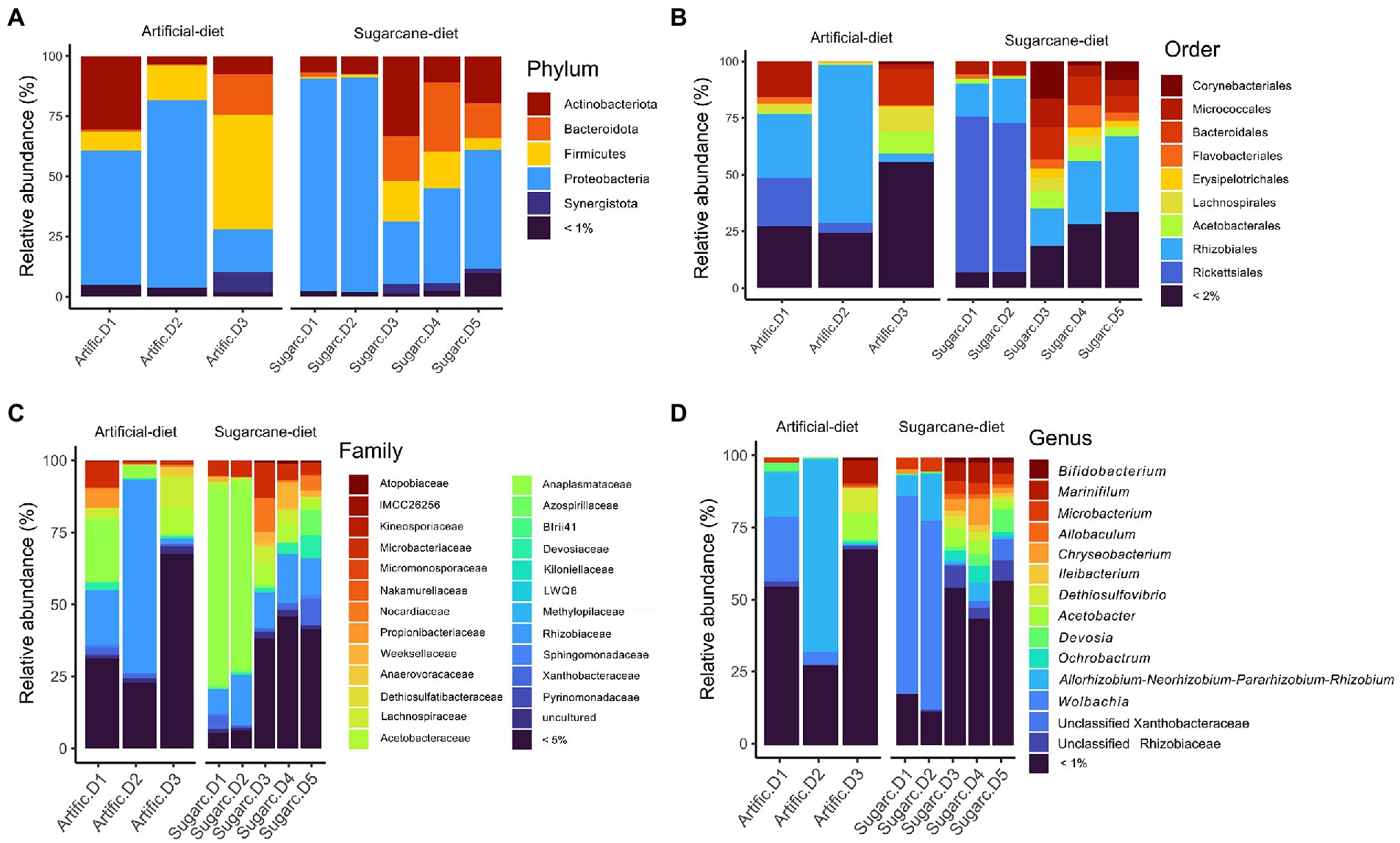
Figure 1. Relative abundances of bacterial taxa at (A) phylum, (B) order, (C) family and (D) genus level found in digestive tracts of Diatraea saccharalis.
3.2. Bioprospecting of a putative new bacterial species with cellulosic activity
In order to target microorganisms with cellulolytic activity, approximately 160 digestive tracts were aseptically dissected from D. saccharalis fifth instar larvae grown on the sugarcane and artificial diet set-ups. Once homogenized in PBS, the biological material was inoculated into modified M9 medium. In total, 12 bacterial colonies were isolated showing cellulose degrading activity on CMC-supplemented plates (Supplementary Table S1). Upon macroscopical and microscopical inspection, four morphologically distinct isolates were selected for whole 16S rRNA gene sequencing. Isolates GCEP-92 and GCEP-94 showed to be closely related to Klebsiella variicola while isolate GCEP-95 affiliated to Pseudomonas nitroreducens (Supplementary Table S2). The isolate that showed the highest apparent cellulolytic activity on CMC plates (GCEP-101), had as closest hit a bacterium within the genus Pseudomonas with no species-level assignment (Pseudomonas sp. HS-18). Consequently, a hybrid assembly using Illumina and Oxford Nanopore reads was prepared reaching a 304-fold coverage and producing a single circular contig of 6,229,841 bp (Figure 2; GenBank Acc. N°. CP104011). The genome has an overall GC content of 66.34%. In total, 5,510 genes were predicted, including 70 tRNAs, 16 rRNAs, 1 tmRNA, 3,400 genes encoding proteins with predicted functions, and 2,023 genes encoding hypothetical proteins. The BlastKOALA pipeline assigned at least one GO term to 3,247 of the predicted proteins (59%), while 2,392 were assigned to at least one KEGG pathway. A total of 269 pathways were predicted, including 8 proteins related to cellulose degradation. These were identified within pathways for carbohydrate metabolism (Supplementary Table S3; Supplementary Figure S2). Regarding the Average Nucleotide Identity (ANI), strain GCEP-101 showed the highest nucleotide-level similarity with Pseudomonas nicosulfuronedens LAM1902T (ANI: 90.27%; Figure 3). In addition, the in-silico DNA–DNA hybridization showed maximum d4 and d6 values of 41.5 and 68.2% with members of P. nicosulfuronedens, respectively (Supplementary Table S4). In all cases such values are lower than the 70% hybridization threshold used commonly to assign closely related strains to the same species (Wayne et al., 1987). A phylogenetic tree (Supplementary Figure S3) was calculated using the genomes of 14 microorganisms. Pseudomonas sp. GCEP-101 was located in an independent branch within the same cluster than the recently described P. nicosulfuronedens, and two species known by their metabolism of nitrogenated compounds: P. nitroreducens and P. nitritireducens. Finally, the analysis of the genes within GCEP-101 genome revealed the presence of genes encoding enzymes related to the degradation of plant biomass, as well as chitobiases and β-hexosaminidases.
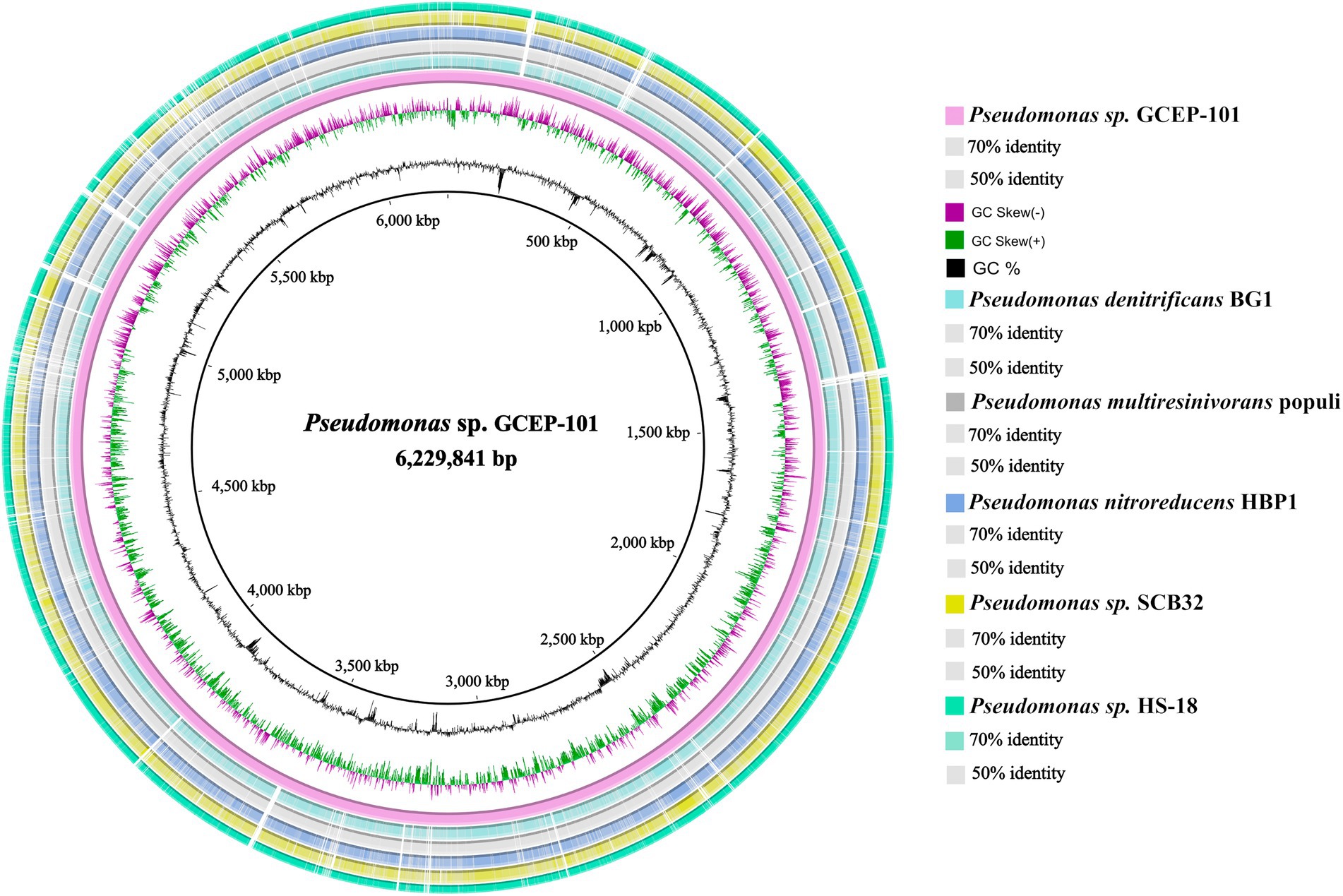
Figure 2. Map of the bacterial chromosome of isolate GCEP-101 (purple inner ring). In the five outer concentric rings, the chromosomes of closely related species are aligned. Their nucleotide identities to GCEP-101 are depicted in color gradients. The middle circle indicates de GC skew [(G−C)/(G + C)] positive (green) and negative (purple). The inner most circle (black) indicates the % GC content.
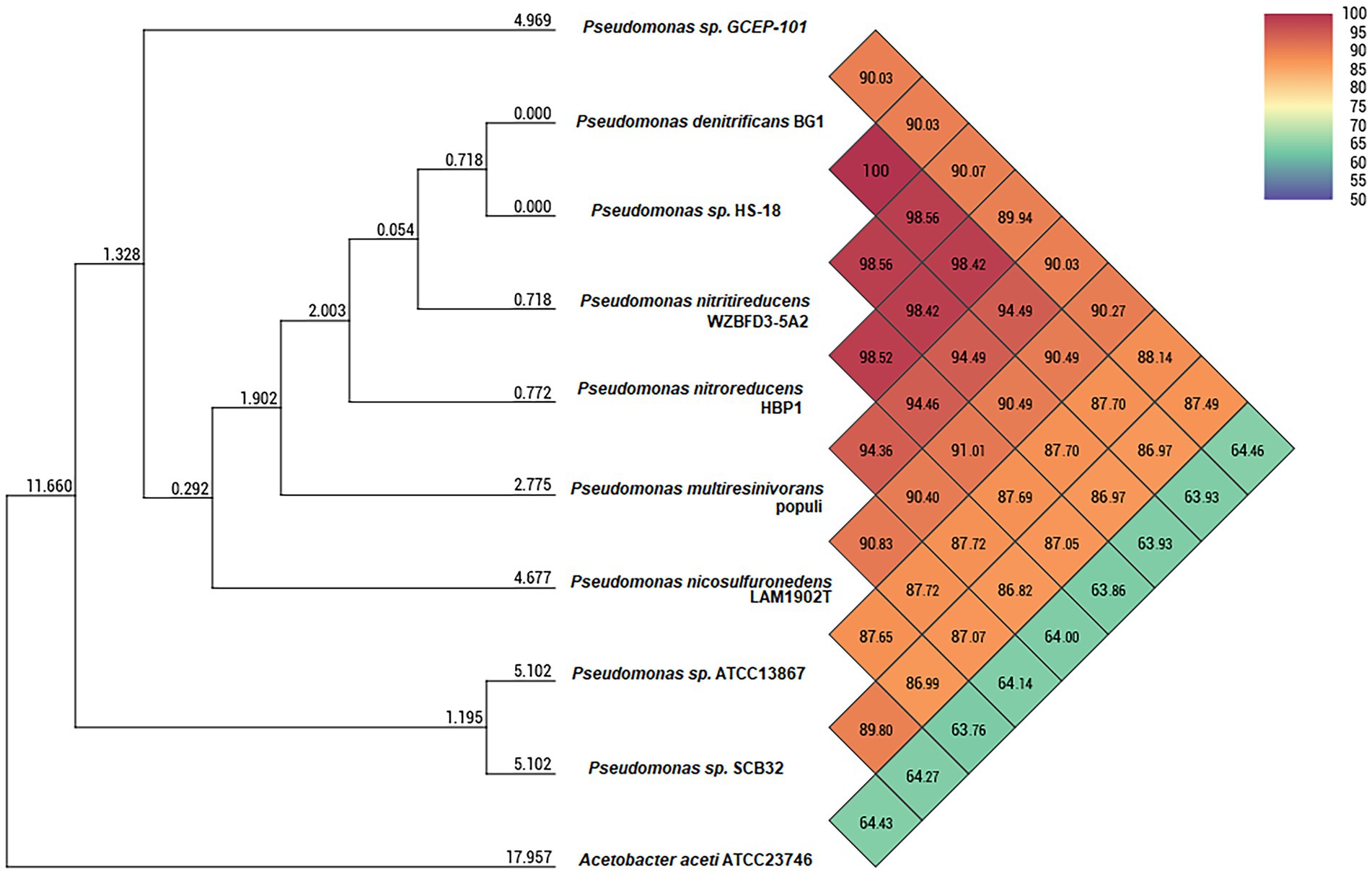
Figure 3. Heatmap of Orthologous Average Nucleotide Identity (OrthoANI) between strain GCEP-101 and closely related relatives. Values in color scale indicate the similarity percentage among the genomes. The numerical values on the branches represent the calculated intergenomic genetic distance calculated by the Orthologous Average Nucleotide Identity Tool (OAT) software.
4. Discussion
Lepidoptera is one of the most widely distributed insect orders in nature (Van Nieukerken et al., 2011). The larval stages of numerous Lepidoptera, including D. saccharalis, have a detrimental impact on society since they feed mainly on living plants. During the arms race over the course of evolution, both plants and insects have evolved mechanisms to thrive in their respective ecosystems (Mello and Silva-Filho, 2002; Zunjarrao et al., 2020). Insects in particular rely on the adaptability of their gut microbiome for the utilization of plants and plant material for the completion of their life cycle (Gupta and Nair, 2020). Here, the analysis of the composition and structure of the bacteriome associated with the digestive tract of D. saccharalis reveals a rich and diverse microbiota. A number of taxa are common to most of the larval pools analyzed in both diet types and were detected with relative abundances exceeding 5%. In this study, a high diversity of microorganisms with low relative abundances was evidenced (Figure 1). Other reports on Lepidoptera and additional insect orders that characterized gut microbiomes from wild specimens have documented significantly lower relative abundances of rare taxa (Pinto-Tomás et al., 2011; Mejía-Alvarado et al., 2021). Interestingly, the microbiome of D. saccharalis exhibited five families of bacteria (Actinomycetaceae, Microbacteriaceae, Rhizobiaceae, Propionobacteriaceae, and Lachnospiraceae; Figure 1) that have also been reported in the gut of at least other 30 species of Lepidoptera (Voirol et al., 2018). Normally the lepidopteran microbiome is affected by diverse factors, including diet, environment, and the physiology of the host (Colman et al., 2012). This study pursued to determine community changes influenced by the type of diet. However, no statistical difference was detected by comparing the treatments. Our findings will help the subsequent research on the topic to decide the real value of using natural or artificial diets in this type of studies (Hammer et al., 2017).
Among the Proteobacteria, the families Xanthobacteraceae and Rhizobiaceae were common in all analyzed samples (Figure 2). Both families are widely known to be capable of N2 fixation into ammonia which is then assimilated by gut endosymbionts that biosynthesize vitamins and amino acids needed for insect development (Indiragandhi et al., 2008; González-Cortés et al., 2022). At genus level, similar to the other taxonomic levels examined, a large number of taxa with relative abundances of less than 1% were observed. This category represents the largest number of genera in most of the groups fed with artificial diet and in three out of five of the larval pools nourished with sugarcane. A prominent genus present in samples from both the sugarcane and the artificial diet was Wolbachia, an α-proteobacterium endosymbiont that is widespread among nematodes and arthropods (Werren et al., 2008; Lefoulon et al., 2020). Some of the ecological interactions of Wolbachia with its hosts include mutualism and in certain cases parasitic manipulation. A meta-analysis conducted to determine the frequency and structure of Wolbachia infection in butterflies and moths demonstrated that this bacterial genus is present in approximately 80% of the lepidopteran species analyzed (Ahmed et al., 2015). This high distribution has been of interest since it is considered that the bacterium could bear the potential for the development of environmentally friendly strategies for the control of pests.
Culturing bacteria from the digestive system of insects is challenging since often these harbor highly specialized microorganisms. Some of them require the specific physicochemical conditions of their host’s gut for their development. In the case of D. saccharalis, most of the taxa found by amplicon sequencing were considered rare and complex to culture. Still, considering the remarkable capacity of D. saccharalis for degrading plant biomass, in this work, we focused on the isolation of microorganisms with cellulose degradation capacity. We isolated representatives of low abundance genera found by amplicon sequencing. Other studies have reported cellulolytic activity in the gut microbiota of insects. For instance, among the bacteria capable of degrading CMC in Coleoptera are Bacillus, Enterobacter, Klebsiella, and Paenibacillus (Rinke et al., 2011; Show et al., 2022).
Different microorganisms with cellulolytic capacity have been reported for D. saccharalis, including Klebsiella, Bacillus, Stenotrophomonas, Microbacterium, Pseudomonas and Enterococcus (Dantur et al., 2015; Barbosa et al., 2020). In this study, 12 strains capable of degrading cellulose on solid culture media were isolated. Based on phenotypic features and the taxonomic classification after 16S rRNA sequencing, the strain GCEP-101 was genome sequenced using two complementary sequencing technologies (Figure 2). Properly done prokaryotic systematics is based on the application of the so-called polyphasic approach (Schleifer, 2009). It comprises phenotypic characteristics, chemotaxonomy and genotypic and phylogenetic data. As part of these features, genomic profiling plays a fundamental role during the first stages of systematic determination of prokaryotes. This has been influenced by the major developments in genome sequencing in the last decades (Stackebrandt et al., 2002). In addition, alternatives to the classical DNA–DNA hybridization method have been introduced, since it demands significant laboratory work and it is prone to experimental biases (Richter and Rosselló-Móra, 2009). These approaches include dDDH and ANI and its derivatives such as OrthoANI, JSpecies (ANIb and ANIm) and gANI (Chun and Rainey, 2014; Chun et al., 2018).
The genus Pseudomonas is one of the most complex bacterial genera in terms of systematics. The number of species in the genus increases yearly (Aidan, 2014). In this specific case, the cellulase activity and the results from the 16S rRNA gene showing a close association with an undefined strain of Pseudomonas, motivated further steps for a better systematic determination. According to the criteria proposed by the ad hoc committee for the evaluation of species definition in bacteriology, both the adoption of ANI and the application of a technique with high correlation with conventional DDH, i.e., dDDH, are necessary for a species definition in prokaryotes (Wayne et al., 1987; Stackebrandt et al., 2002). Consequently, in this study both the OrthoANI values (90.27%) and dDDH (<70% for d4 and d6) strongly indicate the existence of a new species within the genus Pseudomonas derived from the gut microbiome of D. saccharalis. Nonetheless, the description of the potential new species requires further biological evidence. The results of this work certainly demonstrate the importance of studying in depth the cultivable and non-cultivable components of the sugarcane borer microbiome. The former is especially true when it is considered that the genome of GCEP-101 revealed the presence of enzymes involved in the hydrolysis of both labile carbohydrates (Supplementary Figure S2) and recalcitrant biomass such as the beta-xylosidase (EC3.2.1.52) and chitobiose (EC3.2.1; Naraian and Gautam, 2017). This study on the one hand expands the understanding of the microbiomes associated with an important lepidopteran pest affecting one major source of food and income at global scale. On the other hand, it contributes on the identification of potential biocatalysts to be used in industrial processes. In this regard, the microbiomes of D. saccharalis and similar pests are still unexplored potential sources for relevant biomolecules.
Data availability statement
The datasets presented in this study can be found in online repositories. The names of the repository/repositories and accession number(s) can be found below: https://www.ncbi.nlm.nih.gov/, PRJNA870473.
Author contributions
EP-C, GC-V, VT-P, and MA-B conceived the experiments. MA-B and VT-P conducted laboratory work. EP-C, GC-V, LN-E, and JH-T outlined the manuscript. GC-V, EP-C, MA-B, VT-P, LN-E, and JH-T analyzed the data and prepared the figures and tables. All authors contributed to the manuscript preparation and approved the submitted version.
Funding
This project (SIGP 75043) was funded through the FFJC by the Colombian Ministry of Science, Technology and Innovation – MINCIENCIAS and its program Colombia-BIO with contract number 80740-532-2020. The sampling and processing of biological material were granted by the contract ARG-167-2017, addendum N°1.
Acknowledgments
The authors want to extensively thank Miss Julieth Camila Maldonado-Pava for her support in the bioinformatics analyses and the construction of the figures.
Conflict of interest
The authors declare that the research was conducted in the absence of any commercial or financial relationships that could be construed as a potential conflict of interest.
Publisher’s note
All claims expressed in this article are solely those of the authors and do not necessarily represent those of their affiliated organizations, or those of the publisher, the editors and the reviewers. Any product that may be evaluated in this article, or claim that may be made by its manufacturer, is not guaranteed or endorsed by the publisher.
Supplementary material
The Supplementary material for this article can be found online at: https://www.frontiersin.org/articles/10.3389/fevo.2023.1027527/full#supplementary-material
References
Ahmed, M. Z., Araujo-Jnr, E. V., Welch, J. J., and Kawahara, A. Y. (2015). Wolbachia in butterflies and moths: geographic structure in infection frequency. Front. Zool. 12:16. doi: 10.1186/s12983-015-0107-z
Aidan, C. P. (2014). LPSN - list of prokaryotic names with standing in nomenclature. Nucleic Acids Res. 42, D613–D616. doi: 10.1093/nar/gkt1111
Alikhan, N.-F., Petty, N. K., Ben Zakour, N. L., and Beatson, S. A. (2011). BLAST ring image generator (BRIG): simple prokaryote genome comparisons. BMC Genomics 12:402. doi: 10.1186/1471-2164-12-402
Andrews, S. (2010). FastQC. A quality control tool for high throughput sequence data. Available at: https://www.bioinformatics.babraham.ac.uk/projects/fastqc/ (Accessed July 12, 2022).
Aziz, R. K., Bartels, D., Best, A. A., DeJongh, M., Disz, T., Edwards, R. A., et al. (2008). The RAST server: rapid annotations using subsystems technology. BMC Genomics 9:75. doi: 10.1186/1471-2164-9-75
Barbosa, K. L., Malta, V. R. D. S., Machado, S. S., Leal Junior, G. A., Da Silva, A. P. V., Almeida, R. M. R. G., et al. (2020). Bacterial cellulase from the intestinal tract of the sugarcane borer. Int. J. Biol. Macromol. 161, 441–448. doi: 10.1016/j.ijbiomac.2020.06.042
Benson, D. A., Cavanaugh, M., Clark, K., Karsch-Mizrachi, I., Lipman, D. J., Ostell, J., et al. (2013). GenBank. Nucleic Acids Res. 41, D36–D42. doi: 10.1093/nar/gks1195
Beuzelin, J., Huang, F., Reagan, T. E., and Stout, M. J. (2014). Diatraea saccharalis (Sugarcane Stalk Borer) CABI Compendium. CABI International.
Bolyen, E., Rideout, J. R., Dillon, M. R., Bokulich, N. A., Abnet, C. C., Al-Ghalith, G. A., et al. (2019). Reproducible, interactive, scalable and extensible microbiome data science using QIIME 2. Nat. Biotechnol. 37, 852–857. doi: 10.1038/s41587-019-0209-9
Callahan, B. J., McMurdie, P. J., Rosen, M. J., Han, A. W., Johnson, A. J. A., and Holmes, S. P. (2016). DADA2: high-resolution sample inference from Illumina amplicon data. Nat. Methods 13, 581–583. doi: 10.1038/nmeth.3869
Camacho, C., Coulouris, G., Avagyan, V., Ma, N., Papadopoulos, J., Bealer, K., et al. (2009). BLAST+: architecture and applications. BMC Bioinformatics 10:421. doi: 10.1186/1471-2105-10-421
Chaudhary, D. K., Khulan, A., and Kim, J. (2019). Development of a novel cultivation technique for uncultured soil bacteria. Sci. Rep. 9, 6666–6611. doi: 10.1038/s41598-019-43182-x
Chun, J., Oren, A., Ventosa, A., Christensen, H., Arahal, D. R., da Costa, M. S., et al. (2018). Proposed minimal standards for the use of genome data for the taxonomy of prokaryotes. Int. J. Syst. Evol. Microbiol. 68, 461–466. doi: 10.1099/ijsem.0.002516
Chun, J., and Rainey, F. A. (2014). Integrating genomics into the taxonomy and systematics of the bacteria and Archaea. Int. J. Syst. Evol. Microbiol. 64, 316–324. doi: 10.1099/ijs.0.054171-0
Cole, J. R., Wang, Q., Fish, J. A., Chai, B., McGarrell, D. M., Sun, Y., et al. (2014). Ribosomal database project: data and tools for high throughput rRNA analysis. Nucleic Acids Res. 42, D633–D642. doi: 10.1093/nar/gkt1244
Colman, D. R., Toolson, E. C., and Takacs-Vesbach, C. D. (2012). Do diet and taxonomy influence insect gut bacterial communities? Mol. Ecol. 21, 5124–5137. doi: 10.1111/j.1365-294X.2012.05752.x
da Silva, L. C. D., Ferreira, F. I. P., Dezoti, L. A., Nascimento, C. T., Orikasa, C., Takita, M. A., et al. (2022). Diatraea saccharalis harbors microorganisms that can affect growth of sugarcane stalk-dwelling fungi. Brazilian J. Microbiol. 53, 255–265. doi: 10.1007/s42770-021-00647-4
Dantur, K. I., Enrique, R., Welin, B., and Castagnaro, A. P. (2015). Isolation of cellulolytic bacteria from the intestine of Diatraea saccharalis larvae and evaluation of their capacity to degrade sugarcane biomass. AMB Express 5:15. doi: 10.1186/s13568-015-0101-z
Daquila, B. V., Scudeler, E. L., Dossi, F. C. A., Moreira, D. R., Pamphile, J. A., and Conte, H. (2019). Action of bacillus thuringiensis (Bacillales: Bacillaceae) in the midgut of the sugarcane borer Diatraea saccharalis (Fabricius, 1794) (Lepidoptera: Crambidae). Ecotoxicol. Environ. Saf. 184:109642. doi: 10.1016/j.ecoenv.2019.109642
Davis, J. J., Wattam, A. R., Aziz, R. K., Brettin, T., Butler, R., Butler, R. M., et al. (2020). The PATRIC bioinformatics resource center: expanding data and analysis capabilities. Nucleic Acids Res. 48, D606–D612. doi: 10.1093/nar/gkz943
Davolos, C. C., Hernández-Martinez, P., Crialesi-Legori, P. C., Desidério, J. A., Ferré, J., Escriche, B., et al. (2015). Binding analysis of bacillus thuringiensis Cry1 proteins in the sugarcane borer, Diatraea saccharalis (Lepidoptera: Crambidae). J. Invertebr. Pathol. 127, 32–34. doi: 10.1016/j.jip.2015.01.013
Engel, P., and Moran, N. A. (2013). The gut microbiota of insects – diversity in structure and function. FEMS Microbiol. Rev. 37, 699–735. doi: 10.1111/1574-6976.12025
Farris, J. S. (1972). Estimating phylogenetic trees from distance matrices. Am. Nat. 106, 645–668. doi: 10.1086/282802
González-Cortés, J. J., Valle, A., Ramírez, M., and Cantero, D. (2022). Characterization of bacterial and Archaeal communities by DGGE and next generation sequencing (NGS) of nitrification bioreactors using two different intermediate landfill leachates as ammonium substrate. Waste Biomass Valorization 13, 3753–3766. doi: 10.1007/s12649-022-01759-0
Gupta, A., and Nair, S. (2020). Dynamics of insect–microbiome interaction influence host and microbial Symbiont. Front. Microbiol. 11:1357. doi: 10.3389/fmicb.2020.01357
Gurung, K., Wertheim, B., and Falcao Salles, J. (2019). The microbiome of pest insects: it is not just bacteria. Entomol. Exp. Appl. 167, 156–170. doi: 10.1111/eea.12768
Hammer, T. J., Janzen, D. H., Hallwachs, W., Jaffe, S. P., and Fierer, N. (2017). Caterpillars lack a resident gut microbiome. Proc. Natl. Acad. Sci. U. S. A. 114, 9641–9646. doi: 10.1073/pnas.1707186114
Indiragandhi, P., Anandham, R., Madhaiyan, M., and Sa, T. M. (2008). Characterization of plant growth-promoting traits of bacteria isolated from larval guts of diamondback moth Plutella xylostella (lepidoptera: plutellidae). Curr. Microbiol. 56, 327–333. doi: 10.1007/s00284-007-9086-4
Joyce, A. L., White, W. H., Nuessly, G. S., Solis, M. A., Scheffer, S. J., Lewis, M. L., et al. (2014). Geographic population structure of the sugarcane borer, Diatraea saccharalis (F.) (Lepidoptera: Crambidae), in the southern United States. PLoS One 9:e110036. doi: 10.1371/journal.pone.0110036
Kanehisa, M., Sato, Y., and Morishima, K. (2016). BlastKOALA and GhostKOALA: KEGG tools for functional characterization of genome and Metagenome sequences. J. Mol. Biol. 428, 726–731. doi: 10.1016/j.jmb.2015.11.006
Kato, S., Yamagishi, A., Daimon, S., Kawasaki, K., Tamaki, H., Kitagawa, W., et al. (2018). Isolation of previously uncultured slowgrowing bacteria by using a simple modification in the preparation of agar media. Appl. Environ. Microbiol. 84, 1–22. doi: 10.1128/AEM.00807-18
Kreft, L., Botzki, A., Coppens, F., Vandepoele, K., and Van Bel, M. (2017). PhyD3: a phylogenetic tree viewer with extended phyloXML support for functional genomics data visualization. Bioinformatics 33, 2946–2947. doi: 10.1093/bioinformatics/btx324
Kurm, V., Putten, W. H.Van Der, and Hol, W. H. G. (2019). Cultivation-success of rare soil bacteria is not influenced by incubation time and growth medium. PLoS One, 14, 1–14. doi: 10.1371/journal.pone.0210073
Lagesen, K., Hallin, P., Rødland, E. A., Stærfeldt, H. H., Rognes, T., and Ussery, D. W. (2007). RNAmmer: consistent and rapid annotation of ribosomal RNA genes. Nucleic Acids Res. 35, 3100–3108. doi: 10.1093/nar/gkm160
Lee, I., Ouk Kim, Y., Park, S.-C., and Chun, J. (2016). OrthoANI: an improved algorithm and software for calculating average nucleotide identity. Int. J. Syst. Evol. Microbiol. 66, 1100–1103. doi: 10.1099/ijsem.0.000760
Lefort, V., Desper, R., and Gascuel, O. (2015). FastME 2.0: a comprehensive, accurate, and fast distance-based phylogeny inference program. Mol. Biol. Evol. 32, 2798–2800. doi: 10.1093/molbev/msv150
Lefoulon, E., Foster, J. M., Truchon, A., Carlow, C. K. S., and Slatko, B. E. (2020). “The Wolbachia Symbiont: here, there and everywhere” in BT - Symbiosis: Cellular, Molecular, Medical and Evolutionary Aspects. ed. M. Kloc (Cham: Springer International Publishing), 423–451.
Lopes, D., Cantagalli, L., Stuchi, A., Mangolin, C., and Ruvolo-Takasusuki, M. C. (2014). Population genetics of the sugarcane borer Diatraea saccharalis (Fabr.) (Lepidoptera: Crambidae). Acta Sci. Agron. 36, 189–194. doi: 10.4025/actasciagron.v36i2.16211
Mahmoud, A. M. A., De Luna-Santillana, E. J., Guo, X., Reyes-Villanueva, F., and Rodríguez-Pérez, M. A. (2012). Development of the braconid wasp Cotesia flavipes in two Crambids, Diatraea saccharalis and Eoreuma loftini: evidence of host developmental disruption. J. Asia Pac. Entomol. 15, 63–68. doi: 10.1016/j.aspen.2011.07.007
Martín-Platero, A. M., Valdivia, E., Maqueda, M., and Martínez-Bueno, M. (2007). Fast, convenient, and economical method for isolating genomic DNA from lactic acid bacteria using a modification of the protein “salting-out” procedure. Anal. Biochem. 366, 102–104. doi: 10.1016/j.ab.2007.03.010
McDonald, J. E., Rooks, D. J., and McCarthy, A. J. (2012). “Chapter nineteen - methods for the isolation of cellulose-degrading microorganisms” in Cellulases. ed. H. Gilbert (Cambridge, MA: Academic Press), 349–374.
McMurdie, P. J., and Holmes, S. (2013). Phyloseq: an R package for reproducible interactive analysis and graphics of microbiome census data. PLoS One 8:e61217. doi: 10.1371/journal.pone.0061217
Meier-Kolthoff, J. P., Auch, A. F., Klenk, H.-P., and Göker, M. (2013). Genome sequence-based species delimitation with confidence intervals and improved distance functions. BMC Bioinformatics 14:60. doi: 10.1186/1471-2105-14-60
Meier-Kolthoff, J. P., Carbasse, J. S., Peinado-Olarte, R. L., and Göker, M. (2022). TYGS and LPSN: a database tandem for fast and reliable genome-based classification and nomenclature of prokaryotes. Nucleic Acids Res. 50, D801–D807. doi: 10.1093/nar/gkab902
Meier-Kolthoff, J. P., and Göker, M. (2019). TYGS is an automated high-throughput platform for state-of-the-art genome-based taxonomy. Nat. Commun. 10:2182. doi: 10.1038/s41467-019-10210-3
Mejía-Alvarado, F. S., Ghneim-Herrera, T., Góngora, C. E., Benavides, P., and Navarro-Escalante, L. (2021). Structure and dynamics of the gut bacterial community across the developmental stages of the coffee berry borer, Hypothenemus hampei. Front. Microbiol. 12:639868. doi: 10.3389/fmicb.2021.639868
Mello, M. O., and Silva-Filho, M. C. (2002). Plant-insect interactions: an evolutionary arms race between two distinct defense mechanisms. J. Plant Physiol. 14, 71–81. doi: 10.1590/S1677-04202002000200001
Naraian, R., and Gautam, R. L. (2017). “Penicillium enzymes for the saccharification of lignocellulosic feedstocks” in New and Future Developments in Microbial Biotechnology and Bioengineering: Penicillium System Properties and Applications. eds. V. K. Gupta and S. Rodriguez-Couto (Amsterdam: Elsevier), 121–136.
Ondov, B. D., Treangen, T. J., Melsted, P., Mallonee, A. B., Bergman, N. H., Koren, S., et al. (2016). Mash: fast genome and metagenome distance estimation using MinHash. Genome Biol. 17:132. doi: 10.1186/s13059-016-0997-x
Pinto-Tomás, A. A., Sittenfeld, A., Uribe-Lorío, L., Chavarría, F., Mora, M., Janzen, D. H., et al. (2011). Comparison of Midgut bacterial diversity in tropical caterpillars (Lepidoptera: Saturniidae) fed on different diets. Environ. Entomol. 40, 1111–1122. doi: 10.1603/EN11083
Poveda, J. (2019). Los microorganismos asociados a los insectos y su aplicación en la agricultura. Rev. Digit. Univ. 20, 1–15. doi: 10.22201/codeic.16076079e.2019.v20n1.a2
Rajagopal, R. (2009). Beneficial interactions between insects and gut bacteria. Indian J. Microbiol. 49, 114–119. doi: 10.1007/s12088-009-0023-z
Reasoner, D. J., and Geldreich, E. E. (1985). A new medium for the enumeration and subculture of bacteria from potable water. Appl. Environ. Microbiol. 49, 1–7. doi: 10.1128/aem.49.1.1-7.1985
Richter, M., and Rosselló-Móra, R. (2009). Shifting the genomic gold standard for the prokaryotic species definition. PNAS 106, 19126–19131. doi: 10.1073/pnas.0906412106
Rinke, R., Costa, A. S., Fonseca, F. P. P., Almeida, L. C., Delalibera, I., and Henrique-Silva, F. (2011). Microbial diversity in the larval gut of field and laboratory populations of the sugarcane weevil Sphenophorus levis (Coleoptera, Curculionidae). Genet. Mol. Res. 10, 2679–2691. doi: 10.4238/2011.November.1.1
Robeson, M., O’Rourke, D., Kaehler, B., Ziemski, M., Dillon, M., Foster, J., et al. (2020). RESCRIPt: Reproducible sequence taxonomy reference database management for the masses. PLoS Comput. Biol. 17:e1009581. doi: 10.1101/2020.10.05.326504
Schleifer, K. H. (2009). Classification of bacteria and Archaea: past, present and future. Syst. Appl. Microbiol. 32, 533–542. doi: 10.1016/j.syapm.2009.09.002
Seemann, T. (2014). Prokka: rapid prokaryotic genome annotation. Bioinformatics 30, 2068–2069. doi: 10.1093/bioinformatics/btu153
Show, B., Banerjee, S., Banerjee, A., Ghosh Thakur, R., Hazra, A. K., Mandal, N. C., et al. (2022). Insect gut bacteria: a promising tool for enhanced biogas production. Rev. Environ. Sci. Bio. Technol. 21, 1–25. doi: 10.1007/s11157-021-09607-8
Simões, R. A., Feliciano, J. R., Solter, L. F., and Delalibera, I. (2015). Impacts of Nosema sp. (Microsporidia: Nosematidae) on the sugarcane borer, Diatraea saccharalis (Lepidoptera: Crambidae). J. Invertebr. Pathol. 129, 7–12. doi: 10.1016/j.jip.2015.05.006
Stackebrandt, E., Frederiksen, W., Garrity, G. M., Grimont, P. A. D., Kämpfer, P., Maiden, M. C. J., et al. (2002). Report of the ad hoc committee for the re-evaluation of the species definition in bacteriology. Int. J. Syst. Evol. Microbiol. 52, 1043–1047. doi: 10.1099/ijs.0.02360-0
Tan, S. Y., Cayabyab, B. F., Alcantara, E. P., Ibrahim, Y. B., Huang, F., Blankenship, E. E., et al. (2011). Comparative susceptibility of Ostrinia furnacalis, Ostrinia nubilalis and Diatraea saccharalis (Lepidoptera: Crambidae) to Bacillus thuringiensis Cry1 toxins. Crop Prot. 30, 1184–1189. doi: 10.1016/j.cropro.2011.05.009
Team, R C. (2022). A language and environment for statistical computing. R Found. Stat. Comput. Available at: https://www.r-project.org/ (Accessed August 2, 2022).
Van Nieukerken, E. J., Kaila, L., Kitching, I. J., Kristensen, N. P., Lees, D. C., Minet, J., et al. (2011). “Order Lepidoptera Linnaeus, 1758” in Animal Biodiversity: An Outline of Higher-Level Classification and Survey of Taxonomic Richness. ed. Z.-Q. Zhang (Auckland: Zootaxa), 212–221.
Vargas, G., and Gómez, L. (2005). La evaluación del daño causado por Diatraea sp. en caña de azúcar y su manejo en el valle del río Cauca. Cenicaña 9:8.
Voirol, L. R. P., Frago, E., Kaltenpoth, M., Hilker, M., and Fatouros, N. E. (2018). Bacterial symbionts in lepidoptera: their diversity, transmission, and impact on the host. Front. Microbiol. 9:556. doi: 10.3389/fmicb.2018.00556
Wayne, L. G., Brenner, D. J., Colwell, R. R., Grimont, P. A. D., Kandler, O., Krichevsky, M. I., et al. (1987). Report of the ad hoc committee on reconciliation of approaches to bacterial systematics. Int. J. Syst. Evol. Microbiol. 37, 463–464. doi: 10.1099/00207713-37-4-463
Werren, J. H., Baldo, L., and Clark, M. E. (2008). Wolbachia: master manipulators of invertebrate biology. Nat. Rev. Microbiol. 6, 741–751. doi: 10.1038/nrmicro1969
Wick, R. R., Judd, L. M., Gorrie, C. L., and Holt, K. E. (2017). Unicycler: Resolving bacterial genome assemblies from short and long sequencing reads. PLOS Comput. Biol. 13:e1005595. doi: 10.1371/journal.pcbi.1005595
Keywords: Diatraea saccharalis, biocatalysts and bioprocesses, biodiversity, microbiome, sugarcane
Citation: Puentes-Cala E, Atehortúa-Bueno M, Tapia-Perdomo V, Navarro-Escalante L, Hernández-Torres J and Castillo-Villamizar G (2023) First insights into the gut microbiome of Diatraea saccharalis: From a sugarcane pest to a reservoir of new bacteria with biotechnological potential. Front. Ecol. Evol. 11:1027527. doi: 10.3389/fevo.2023.1027527
Edited by:
Zheng Wang, Yale University, United StatesReviewed by:
Rob DeSalle, American Museum of Natural History, United StatesRoland Wohlgemuth, Lodz University of Technology, Poland
Copyright © 2023 Puentes-Cala, Atehortúa-Bueno, Tapia-Perdomo, Navarro-Escalante, Hernández-Torres and Castillo-Villamizar. This is an open-access article distributed under the terms of the Creative Commons Attribution License (CC BY). The use, distribution or reproduction in other forums is permitted, provided the original author(s) and the copyright owner(s) are credited and that the original publication in this journal is cited, in accordance with accepted academic practice. No use, distribution or reproduction is permitted which does not comply with these terms.
*Correspondence: Genis Castillo-Villamizar, ✉ Z2Nhc3RpbGxvQGNvcnJvc2lvbmNpYy5jb20=
†ORCID: Edinson Puentes-Cala https://orcid.org/0000-0003-0587-2177
Jorge Hernández-Torres https://orcid.org/0000-0003-1867-9800
Genis Castillo-Villamizar https://orcid.org/0000-0001-6730-6889