- 1Institute for Integrated Natural Sciences, Zoology, University of Koblenz-Landau, Landau, Germany
- 2Department of Zoology, Centre for Ecological Genomics and Wildlife Conservation, University of Johannesburg, Johannesburg, South Africa
- 3Endangered Wildlife Trust, Johannesburg, South Africa
- 4Institute of Zoology, Zoological Society of London, London, United Kingdom
- 5African Wildlife Conservation Fund, Chishakwe Ranch, Zimbabwe
- 6Department of Biology, University of Copenhagen, Copenhagen, Denmark
- 7Faculty of Sciences, Leiden University, Leiden, Netherlands
- 8Painted Dog Research Trust, Victoria Falls, Zimbabwe
- 9Department of Wildlife Management and Ecotourism, University of Namibia, Khomas, Namibia
- 10ISCED, Instituto Superior de Ciências de Educação da Huíla, Lubango, Angola
- 11CIBIO, Centro de Investigação em Biodiversidade e Recursos Genéticos, InBIO Laboratório Associado, Campus de Vairão, Universidade do Porto, Vairão, Portugal
- 12BIOPOLIS Program in Genomics, Biodiversity and Land Planning, CIBIO, Campus de Vairão, Vairão, Portugal
- 13Department of Biology, Faculty of Sciences, University of Porto, Porto, Portugal
Across much of Africa, decades of civil war, land reforms, and persecution by humans have decimated wildlife populations. African wild dogs (Lycaon pictus) have declined dramatically during the past decades, but have shown recent natural recolonisation of some areas. In Angola, they were rediscovered after almost five decades when no surveys were being conducted, and they have recolonised areas in southern Zimbabwe and northern South Africa. Wild dogs were also reintroduced to Mozambique, where only few individuals remained. Against this backdrop, understanding genetic structure and effective dispersal between fragmented populations is essential to ensure the best conservation approaches for the long-term survival of the species. Our study investigated population genetic diversity, differentiation and gene flow of wild dogs across southern Africa, to include areas where they have recently been rediscovered, reestablished or reintroduced. Our results point to four weakly differentiated genetic clusters, representing the lowveld of Zimbabwe/Limpopo, Kruger NP, Angola/KAZA-TFCA, and the managed metapopulation, counterbalanced by moderate levels of effective dispersal on a southern African scale. Our results suggest that if the human footprint and impact can be significantly minimized, natural dispersal of wild dogs could lead to the demographic recovery of the species in southern Africa.
Introduction
The loss of biodiversity is one of the most pressing issues we are faced with today, presenting a threat to both ecosystem functioning and human wellbeing (Ceballos et al., 2015). Biodiversity loss primarily results from habitat loss, degradation and fragmentation, over-exploitation, and the introduction of non-native invasive species (Dirzo and Raven, 2003). Across many areas of the world, civil unrest negatively impacts wildlife in many ways including the direct effects of mines, increased bushmeat hunting, and the exchange of high-value wildlife products for the purchase of arms (Gaynor et al., 2016). Furthermore, in times of conflict, fewer resources are available to protect biodiversity, such as anti-poaching units and education programmes (Kanyamibwa, 1998). Over the past 60 decades, armed conflict occurred in more than two-thirds of the world’s biodiversity hotspots, thereby presenting a larger threat to wildlife than is generally recognised (Hanson et al., 2009; Daskin and Pringle, 2018). Typical consequences of armed conflicts are a decline in forest cover and wildlife population numbers (Hanson et al., 2009), which results in the loss of genetic diversity at both the species and population level (Frankham et al., 2004). Because the loss of genetic diversity can substantially decrease a species’ evolutionary potential and survival success through inbreeding and genetic drift, it has become an important aspect of conservation efforts, set as one of the key components of biodiversity (Collen et al., 2011).
Africa holds the greatest abundance and diversity of large carnivores and large herbivores globally (Ripple et al., 2014). The greatest increase in human population numbers has also occurred in sub-Saharan Africa, which is projected to rise from 0.8 billion to 1.8 billion people within 40 years (Le Bel et al., 2011). The greatest occurrences of poverty also lie in Africa, which often results in competition for land, localised wars or even famine (Devereux, 2009). In Angola, a three-decades-long civil war, lasting from 1975 to 2002, displaced over four million people and decimated wildlife populations (Chase and Griffin, 2011). Similarly, in Mozambique many wildlife populations vanished during the civil war that lasted from 1977 to 1992 (Daskin and Pringle, 2018; Stalmans et al., 2019). Although not a civil war, the land reform programme in Zimbabwe after independence also resulted in a significant decline of wildlife populations, as natural habitat was transformed for agriculture (Lindsey et al., 2012). These conflicts have been the most important predictor of declines among large carnivores and herbivores in African protected areas (Daskin et al., 2016). Furthermore, due to the resulting poverty among local villagers, bushmeat hunting and wire snare poaching remain common threats to wildlife (Daskin et al., 2016). A study on African wild dogs (Lycaon pictus) in the Lower Zambezi, Zambia, found that snaring accounted for 54% of adult mortality, eventually contributing to the local extirpation (Leigh, 2005).
African wild dogs (hereafter wild dogs), originally known as Painted Lycaon (Smith, 1840), and latterly painted dogs or Cape hunting dogs, were once widely distributed across sub-Saharan Africa, but have declined drastically over the past decades due to landscape transformations and human activity (Woodroffe et al., 1997; Creel et al., 2020). Furthermore, they were classified as vermin in Zimbabwe between 1906 and 1975, and systematically persecuted as putative livestock predators (Childes, 1988; Rasmussen, 1999). In Rhodesia (now Zimbabwe), a 10 shilling bounty was even paid for the tail or head of a wild dog (Rasmussen, 1999). Their current distribution has become highly fragmented because savannah grasslands and woodlands are progressively threatened by livestock overgrazing and urbanisation (Riggio et al., 2013). As a result, wild dogs are listed as Endangered by the global IUCN Red List of Threatened Species (Woodroffe and Sillero-Zubiri, 2020). One population stronghold, consisting of approximately 17% of its historical range, still remains in southern Africa, where an estimated population of c. 4,400 wild dogs are distributed over reserves, with its widest extent found across Botswana and Namibia (IUCN/SSC, 2015).
In Angola, wild dog population numbers are ambiguous, and most of the country is currently classified as unknown range. However, recent records indicate the presence of wild dogs in the Luengue-Luiana National Park (NP) and Mavinga NP (Funston et al., 2017), Mucusso Reserve and Cuatir Conservation Area (Monterroso et al., 2020), the Cuanavale and Cuito river catchments (NGOWP, 2022), as well as Bicuar and Mupa national parks (Overton et al., 2020; Figure 1). Wild dogs have naturally recolonised private reserves in the lowveld of Zimbabwe, where population numbers have steadily increased (Funston et al., 2013). Free-roaming individuals have also naturally established in the Waterberg region and the Limpopo Province in northeastern South Africa (Nicholson et al., 2020). In Mozambique, wild dogs currently occur in the Tete region, Niassa National Reserve, Gile, the Catapu Concession, Maunge, Gorongosa and the Limpopo NP (IUCN/SSC, 2015). One post-civil war population remained in the densely forested Marromeu Complex in central Mozambique, although it is unclear whether individuals were transient, occurred at very low densities, or became absent (Tinley, 1977). In the Karingani Game Reserve and Gorongosa NP, three packs of wild dogs were translocated (2018/2019) from a managed metapopulation in South Africa to augment resident remnant populations (Bouley et al., 2021). In South Africa, c.350 wild dogs reside in Kruger NP, private reserves, and unprotected areas (WAG-SA, 2022). Following the collapse of the Kruger population, the outcome of a population viability analysis assessment (Mills et al., 1998) led to a managed metapopulation outside Kruger NP. The 66 founders were captive-bred or wild-caught around Kruger NP during human-conflict mitigation (Gusset et al., 2006), and the managed metapopulation is currently spread across 11 reserves (WAG-SA, 2022). Translocations are ongoing within these geographically isolated reserves to mimic natural dispersal (Tensen et al., 2019).
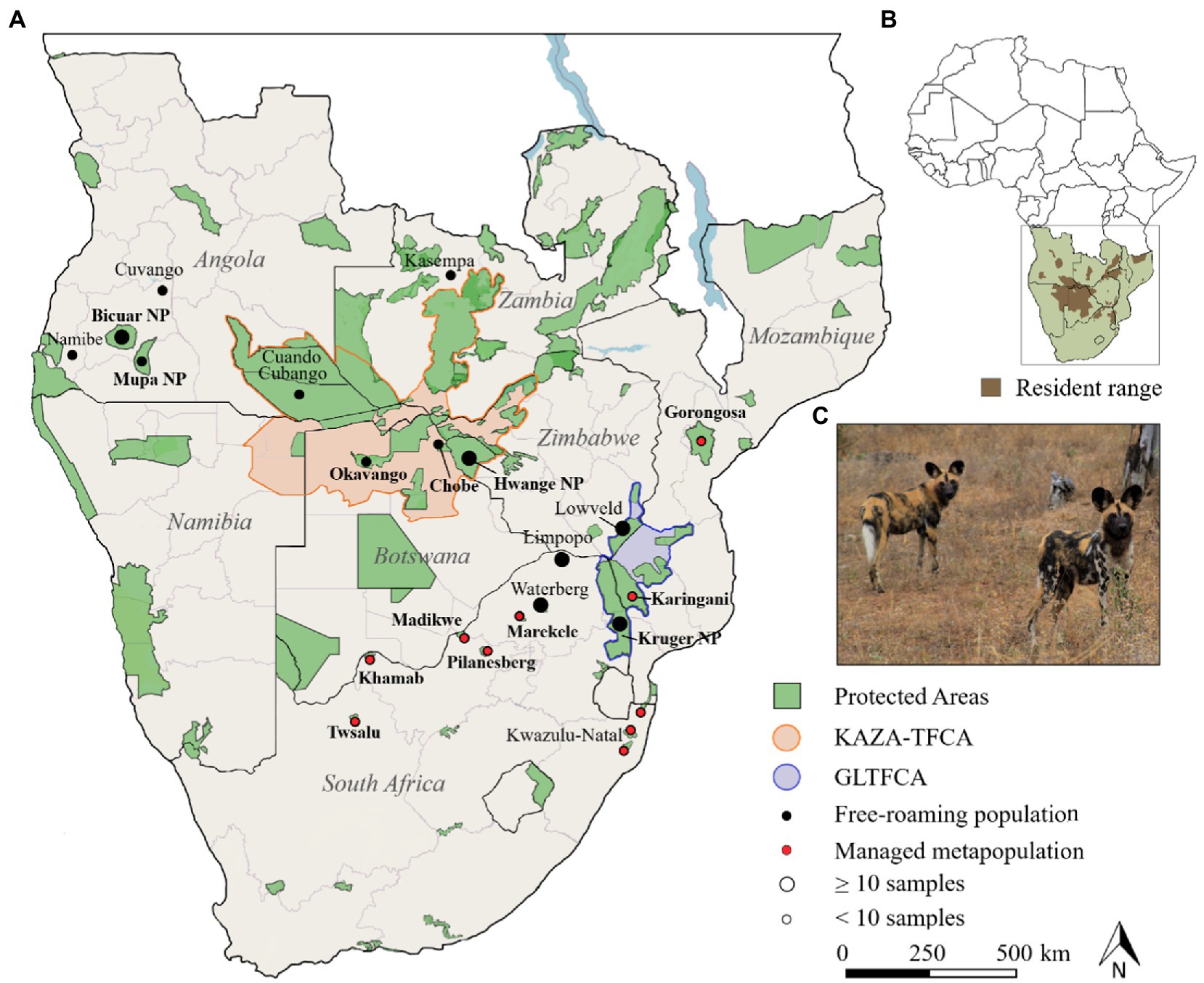
Figure 1. Wild dogs in southern Africa. (A). Map with sample locations of African wild dogs. Protected areas were adapted from (UNEP-WCMC and IUCN Protected Planet, 2022) and filtered for >1.000 km2. Highlighted are the Kavango-Zambezi Transfrontier Conservation Area (KAZA-TFCA), and Greater Limpopo Transfrontier Conservation Area (GLTFCA). Free-roaming populations naturally occur within and outside reserves, whereas the managed metapopulation originated from reintroductions and within fenced reserves. (B). Wild dog distribution range in southern Africa, adapted from IUCN/SSC (2015). (C). A picture of two African wild dogs in Savé Valley Conservancy, Zimbabwe (photo by L. Tensen).
Because wild dogs naturally occur at low densities, connectivity between reserves is extremely important to maintain gene flow and safeguard their long-term survival (Davies-Mostert et al., 2012; Tensen et al., 2019). Wild dogs are known to disperse over long distances, and they can cross hundreds of kilometres through unsuitable habitats and human-dominated landscapes (Rasmussen and Macdonald, 2012; Masenga et al., 2016). However, the survival success of dispersing individuals is often low, and the effective dispersal (i.e., reproduction after dispersal) between areas is compromised (Leigh et al., 2012; van der Meer et al., 2014). Genetic structuring, presumed to have resulted from anthropogenic habitat fragmentation, has already been illustrated in wild dogs, as well as a loss of genetic diversity across their range (Girman et al., 2001; Marsden et al., 2012). In southern Africa, natural dispersal appears ongoing in northern Botswana and western Zimbabwe (Cozzi et al., 2020; Hofmann et al., 2021) across an area that stretches from the Okavango Delta to Hwange NP (Figure 1). These reserves are part of the Kavango Zambezi Transfrontier Conservation Area (KAZA-TFCA), which was created to allow free movement of wildlife to enlarge effective distribution range and enhance metapopulation dynamics (Fynn and Bonyongo, 2011). Wild dog populations in the lowveld of Zimbabwe and Kruger NP in South Africa appear to be genetically isolated from other populations, which is likely associated with the Zimbabwean land reform programme in the 2000s and loss of natural habitat (Tensen et al., 2016). The lack of connectivity in this region has led to some of the lowest levels of genetic diversity recorded in Africa, based on mitochondrial DNA and microsatellites (Marsden et al., 2012). Furthermore, the eradication programme that lasted into the 1950s is thought to have influenced developmental stability, as a result of inbreeding and genetic drift (Edwards et al., 2013). The Greater Limpopo Transfrontier Conservation Area (GLTFCA) connects Zimbabwe, South Africa and Mozambique (Whande and Suich, 2012), and will hopefully help to restore connectivity and genetic diversity in the region.
Historic mitochondrial DNA suggests that wild dogs in Angola are related to Okavango and Hwange populations (Girman et al., 2001), although the genetic diversity and relationship of the Angolan population with other geographical regions remains unknown. The genetic background of the central Mozambique population is also unknown, although it is likely that this region formed a secondary contact zone between eastern and southern Africa, as it is positioned at the southern edge of the Great Rift Valley (Tinley, 1977; Bertola et al., 2022). Understanding demographic and genetic connectivity between fragmented populations is essential for the long-term survival of species, in particular for endangered species with marginal populations in their distribution area, such as African wild dogs. With this in mind, we aim to provide an overview of the spatial genetic diversity and population structure, as well as levels of gene flow, of wild dogs in southern Africa. We use comprehensive sampling including populations that have remained unknown so far, covering Angola, Botswana, Zambia, Zimbabwe, South Africa and Mozambique, and a large panel of microsatellite loci, to address the following questions: (i) have recent population declines led to reduced levels of genetic diversity in wild dogs, and if so, do marginal and recently rediscovered populations show different levels of diversity?; (ii) is there a consistent and spatially supported genetic structure between wild dog populations in southern Africa?; (iii) is there evidence of gene flow between different wild dog populations, and if so, do gene flow levels differ among regions?; and (vi) to which populations do free-roaming wild dogs in Angola and South Africa genetically relate? The results of this study will hopefully add to a broader understanding of metapopulation dynamics, by providing a genetic perspective on the demographic recovery and gene flow of the charismatic and threatened wild dog across southern Africa.
Materials and methods
Study area and sampling origin
For this study, we have used 162 samples from 21 areas in Angola, Botswana, Zambia, Zimbabwe, South Africa and Mozambique (Figure 1; Supplementary Table S1), including 148 modern (faecal, blood and muscle) and 12 historic (bone) samples. Modern samples were gathered in the scope of other studies (Rasmussen and Macdonald, 2012; Tensen et al., 2016, 2019; Bouley et al., 2021) or opportunistically collected from dead animals and scats. Four areas in Angola are part of this study, namely the Mupa NP, Bicuar NP, and Pediva area in the Namibe Province, and Luengue Luiana NP in the Cuando Cubango Province. In Botswana, samples were collected from the Okavango Delta and Chobe NP. The Okavango Delta (~28,000 km2) is located within the Ngamiland District, and extends into the Chobe NP (~12,000 km2) further east. Chobe NP is functionally connected through forest reserves to the Hwange NP (~15,000 km2), located in western Zimbabwe, and along with Okavango they support one of the largest continuous and stable wild dog populations in southern Africa (Woodroffe and Sillero-Zubiri, 2020). We also collected samples from the Kasempa district, Zambia.
In South Africa, samples originated from seven reserves that are part of the managed metapopulation, in the northern and eastern part of the country. Samples were also collected outside protected reserves, in northern Limpopo and the Waterberg area, and in the central and southern part of Kruger NP, which is the one of the largest protected areas in Africa (19,000 km2). Furthermore, samples were collected from the wild dogs that were translocated from South Africa (i.e., Khamab Kalahari Game Reserve and uMkhuze) to Gorongosa National Park, in central Mozambique. The Gorongosa-Marromeu area is managed as a contiguous complex of national parks, national reserves, buffer-zones, forest reserves and hunting concessions or “coutadas,” with a combined surface area of 20,000 km2 (Bouley et al., 2021).
Many study areas are part of the Kavango Zambezi (KAZA-TFCA) or Greater Limpopo (GLTFCA) Transfrontier Conservation Area. The KAZA-TFCA is the world’s largest transfrontier conservation area (520,000 km2) and forms Africa’s largest conservation landscape, serving as a stronghold for large herbivores and carnivores (Funston et al., 2017). The GLTFCA is a biodiversity hotspot that measures almost 100,000 km2 and links the Limpopo NP in Mozambique, Kruger NP in South Africa, and Gonarezhou NP in Zimbabwe (Whande and Suich, 2012).
DNA extraction, marker selection and amplification
DNA was extracted from blood and tissue samples using the DNAeasy Blood & Tissue Kit (Qiagen, Valencia, CA, United States) according to the manufacturer’s instructions at the Centre for Ecological Genomics and Wildlife Conservation at the University of Johannesburg. DNA was extracted from faecal and historic samples by a silica-based purification method, following an ancient DNA extraction protocol (Höss and Pääbo, 1993). For low quality DNA samples, all pre-PCR procedures were carried out in a dedicated laboratory for the manipulation of these samples under sterile conditions and positive air pressure at CIBIO Research Centre, University of Porto. Negative controls were included throughout the entire process to test for DNA contamination.
Individual multi-locus genotypes were generated using a suite of 40 autosomal microsatellites selected from across the genome, which were polymorphic in grey wolves (Canis lupus; Godinho et al., 2011, 2015). These markers amplified successfully in wild dogs using the same amplification conditions and multiplex sets described in Godinho et al. (2011, 2015). A subset of 20 microsatellites was selected for amplification of low quality DNA samples based on the number of alleles, amplification success, and compatible allelic ranges and melting temperatures for multiplexing. For faecal and museum samples, each locus was amplified and genotyped four times to monitor for genotyping errors. Amplifications were performed in four multiplex reactions using the QIAGEN Multiplex PCR Kit following the manufacturer’s instructions, and using a 10 μl final volume and negative controls to monitor possible contaminants (Multiplex sets and amplification conditions available in Supplementary Tables S2, S3, respectively). Fluorescent labelling of PCR fragments was accomplished following (Blacket et al., 2012). PCR products were separated by size on an ABI3130xl DNA analyser. Alleles were scored using GENEMAPPER 5.0 (Applied Biosystems) and checked manually by two observers. For non-invasive samples, the consensus genotypes over the four replicas were determined following the rules in (Godinho et al., 2015).
Statistical analysis
Micro-Checker v2.2.3 (Van Oosterhout et al., 2004) was used to test for genotype errors such as stuttering and large allele dropout, and to test whether loci were in Hardy–Weinberg equilibrium (HWE) based on 1,000 bootstraps and Bonferroni-adjustment (95%). Arlequin v3.5.2.2 (Excoffier and Lischer, 2010) was used to test for linkage disequilibrium (LD), using Bonferroni corrections for multiple comparisons (95%), and to measure genetic diversity, based on observed (HO) and expected (HE) heterozygosities for each locus at each population that had a sample size (N) > 10. We calculated allelic richness (AR) and private allelic richness (AP) using the rarefaction method in HP-Rare v1.0 (Kalinowski, 2005) to compensate for different sample sizes between populations, which standardizes to the smallest sample size (N = 10 for Bicuar/Mupa). Relatedness (r) between individuals within populations was calculated using ML-relate (Kalinowski et al., 2006) and one individual was removed from pairs of individuals with r > 0.4. Genetic diversity measures and relatedness were estimated using the 20 microsatellites available for all populations. To explore the demographic history and recent bottleneck events, we used BOTTLENECK v1.2.02 (Piry et al., 1999), using the infinite alleles (IAM), stepwise mutation (SMM), and two-phased (TPM) models. TPM tends to be the most appropriate mutation model for microsatellite loci (Di Rienzo et al., 1994), and we chose the proportions in favour of IAM (70% vs. 30% SMM) when applying this model. The computation was performed under the Wilcoxon method, and ran for 1,000,000 iterations and a variance set at 20. The second test done in BOTTLENECK was the allele frequency distribution test. Since rare alleles are rapidly lost during a bottleneck, the characteristic L-shaped distribution of allele proportions is not observed under a bottleneck event (Luikart et al., 1998). We additionally estimated demographic reductions using the Garza-Williamson index (or M ratio) implemented in Arlequin (Excoffier and Lischer, 2010). A M ratio below the critical value (< 0.68) is considered a signature of genetic bottleneck (Luikart et al., 1998). Only populations with a minimum sample size of 10 and the 20 microsatellites available for all populations were included for both demographic history analyses.
For identifying genetic clusters, we used STRUCTURE v2.3 (Pritchard et al., 2000), which applies a Bayesian framework for inferring population structure. We applied the admixture model for the ancestry of individuals, and assumed correlated allele frequencies. The program was run from K value 1 to 10 with 500,000 MCMC generations (discarding 20% as burn-in) and 20 iterations. The mean Ln(K) graph and rate of change of the likelihood distribution between successive K values (DeltaK) retrieved from Structure Harvester (Earl and Von Holdt, 2012) were used to determine the most likely number of genetic clusters. For population clustering analyses, all samples and 40 microsatellites (with missing data for samples with only 20 microsatellites genotyped) were used in the final dataset. Arlequin (Excoffier and Lischer, 2010) was used to estimate genetic differentiation between populations, within populations and among individuals through a hierarchical analysis of molecular variance (AMOVA), using Wright’s F-statistics. The same set of genotype data was used to perform a Principal Coordinate Analysis (PCoA) using GenAlEx 6.5 (Peakall and Smouse, 2006). PCoA is a non-parametric linear dimension reduction technique well suited to uncover population structure in admixed populations (Lee et al., 2009). However, because PCoA only describes the global diversity, it may possibly overlook differences between groups. Therefore, a Discriminant Analysis of Principal Components (DAPC) was performed using the adegenet 2.0.1 package (Jombart, 2008) in R 3.2.2 software (Team, 2015), which seeks differences between groups while minimising variation within clusters (Jombart, 2008).
To investigate the spatial arrangement of dispersal, we used the Estimated Effective Migration Surfaces method in the program EEMS (Petkova et al., 2016). EEMS identifies geographic regions where genetic dissimilarity decays more quickly than expected under a null model of isolation-by-distance. The program also estimates levels of effective genetic diversity across the landscape on the basis of expected genetic dissimilarity between pairs of individuals sampled from the same location. For each analysis, we ran three independent chains for 5,000,000 MCMC iterations, with a burn-in of 2,500,000 MCMC iterations and sampling every 10,000 iterations. We compared log posterior plots and performed a Gelman test to confirm convergence. Graphs were constructed using the rEEMSplots package in R (Petkova et al., 2016).
Results
All microsatellites were polymorphic with between two and 20 alleles per locus. We found no evidence for stuttering or large allele dropout in our microsatellite data, and no evidence of pairwise linkage disequilibrium between loci within individual populations or deviations from HWE after Bonferroni corrections (Supplementary Table S4). Due to high relatedness values (r > 0.40), we removed two samples from Bicuar NP, two from the lowveld, and four from Limpopo, resulting in a final sample size of N = 154 (Supplementary Table S1).
Genetic diversity
We found an average of 6.7 ± 3.8 alleles per locus across all populations (Supplementary Table S4). When applying the rarefaction method to standardize sample size, the allelic richness was on average 3.6 alleles per locus over all populations. The wild dog population in Hwange NP presented the highest values for the two allelic richness measures (AR = 4.35; PA = 0.55), revealing both higher diversity and uniqueness. The free-roaming population of Limpopo in South Africa presented the lowest allelic richness and no private alleles (AR = 2.76; PA = 0.00). Analysis also showed a heterozygote excess in the Limpopo population (Table 1), possibly indicating a recent decrease in its effective population size. In the other populations, the observed and expected heterozygosities were equivalent showing an overall mutation-drift equilibrium.
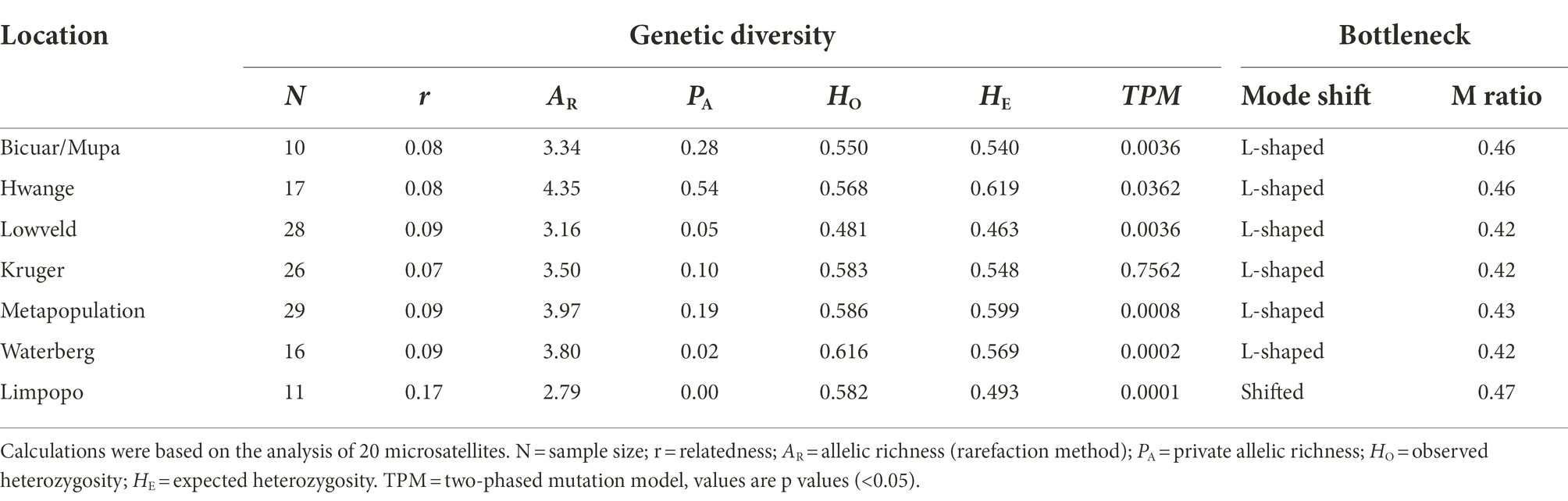
Table 1. Genetic diversity indices, mutation-drift equilibrium based on bottleneck analysis (Wilcoxon test), and the M ratio based on the Garza-Williamson Index, of wild dog populations (N > 10) in southern Africa.
Bottleneck analysis using the TPM model (see Supplementary Table S5 for IAM and SMM) detected significant signals of a recent bottleneck (Wilcoxon tests p > 0.05) in every population except for Kruger NP (Table 1). The allele distribution was found to be shifted only for the Limpopo population, illustrating a possibly more drastic recent bottleneck in this population. All other populations were found to be in mutation-drift equilibrium according to this test. The M ratios of all populations were below the critical value (M < 0.68) assumed as the threshold for detecting bottlenecks, indicating that all wild dog populations underwent recent demographic reductions (Table 1).
Population structure
The Bayesian clustering method for identifying the most probable K showed that two to four clusters best explained our data, using DeltaK and Ln(K) values, respectively, (Supplementary Figure S1). At K = 2, Angola, Zambia, Botswana, western Zimbabwe, Kruger NP, the metapopulation, and Mozambique grouped as one cluster. The lowveld of Zimbabwe and the free-roaming population in Limpopo differentiate themselves from the rest of southern Africa, although one individual in Kruger NP and several in the Waterberg free-roaming population are also assigned to the lowveld/Limpopo cluster. We note that wild dogs in the lowveld and Limpopo exhibited the lowest genetic diversity and strong signatures of a bottleneck, which may bias their assignment as the first cluster to differentiate in this analysis. When considering K = 3, we observe the clustering of Kruger individuals as a different population, and for K = 4 the metapopulation becomes a separate cluster as well (Figure 2). The variance between runs increases for K = 5 and beyond, and more than one solution is reached for the same K.
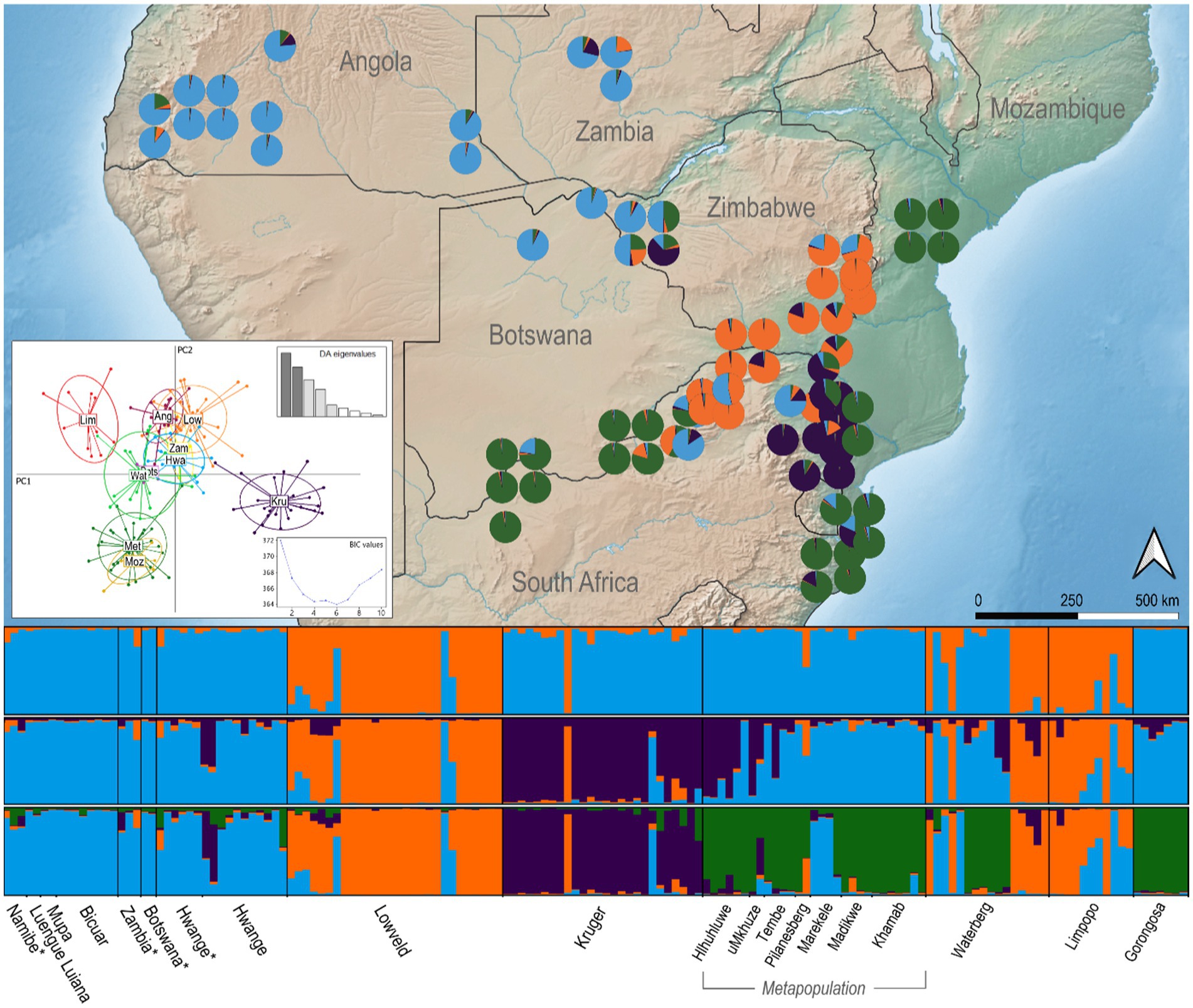
Figure 2. Spatial population structuring of African wild dogs sampled across southern Africa, including historic samples (1960–1970) highlighted with *. Pie charts are Bayesian estimates of individual assignment for each sample K = 4 and are positioned in sampling locations. Discriminant Analysis of Principal Components (DAPC) is illustrated in the scatter plot; Ovals are 95% inertia ellipses; Insets show the distribution of eigenvalues for all principal components and the Bayesian Information Criterion (BIC).
The historical samples from Angola, Zambia, Botswana and western Zimbabwe cluster together with contemporary samples from the same regions, showing consistency of wild dog population over time (Figure 2). The observed genetic clusters show some level of individual admixture, specially observed in Hwange NP, Limpopo and the metapopulation. Based on the clusters defined by STRUCTURE analysis, the wild dog population in Waterberg comprehends individuals from three clusters that did not exhibit admixture between them, namely the lowveld/Limpopo cluster, managed metapopulation, and Angola/KAZA-TFCA cluster. In the free-roaming population of northern Limpopo (South Africa), a few wild dogs clearly show a pattern of admixture between the lowveld/Limpopo cluster and Angola/KAZA-TFCA cluster. Additionally, two individuals in western Zimbabwe exhibit a signature of admixture with the Kruger cluster (Figure 2). The graphical representation of the genetic similarity between individuals observed in the PCoA plot illustrates a trend towards the clusters defined by STRUCTURE (Supplementary Figure S2). The first axis of PCoA explains 7.2% of the genetic variation and splits the lowveld and Limpopo from Angola and KAZA-TFCA sampling areas. The second coordinate, explaining 6.3% of variation, differentiates the metapopulation from most individuals from Limpopo, the lowveld, and Angola/KAZA-TFCA cluster. Individuals from Kruger and the Waterberg region are widely spread along coordinates 1 and 2, and their genetic affinity with the other populations is not explained by the two first coordinates in this analysis (Supplementary Figure S2). Unlike STRUCTURE and the PCoA, the DAPC clearly differentiates northern Limpopo from the lowveld in PC1, and Kruger NP is also well differentiated from all other populations in the same axis (Figure 2). Inspection of BIC values for the DAPC from 1 to 10 clusters identifies four to six as the optimal number of clusters present in southern African wild dogs.
The analysis of molecular variance (AMOVA) performed for the seven populations showed that 10% of the variation is accounted for by among-population differences (overall FST = 0.101; p < 0.05), and 92% by within-individual differences (Supplementary Table S6). Pairwise FST values were significant for all comparisons except for Hwange-Bicuar/Mupa. The highest genetic differentiation was observed between Bicuar/Mupa NP in Angola and the lowveld of Zimbabwe (FST = 0.243), and the lowest level of differentiation was observed between the Waterberg and the managed metapopulation (FST = 0.034). When performing an AMOVA based on the four genetic clusters as defined by STRUCTURE, we found that 7% of the variation is accounted for by genetic differences between clusters (FST = 0.066; p < 0.05), and 91% by within-individual differences. In this analysis, the lowest pairwise FST (0.042) was between Kruger and the managed metapopulation, and the highest FST (0.099) between the Angola/KAZA-TFCA and lowveld/Limpopo clusters (Supplementary Table S6). All comparisons tested significant.
Gene flow
The representation of effective dispersal and genetic diversity in wild dogs was investigated by using estimates of effective migration surfaces (EEMS) between the different sampling locations (Figure 3). Results suggested that there are no strong dispersal barriers across southern Africa (Figure 3A). Indeed, levels of effective dispersal among sampling areas in KAZA-TFCA are high, with a decreasing trend towards southwest Angola. A different gene flow scenario is revealed across eastern Zimbabwe and South Africa sampling areas, which are characterized by overall low levels of effective dispersal (Figure 3A). Estimates of effective genetic diversity surfaces for wild dogs inferred that sampling areas in Angola are the least diverse among Angola/KAZA-TFCA region, while a strong depauperation of genetic diversity is observed for southern Zimbabwe and northern South Africa corresponding to the lowveld and Limpopo Province. Interestingly, all other sampling areas in this region exhibit high levels of genetic diversity (Figure 3B).
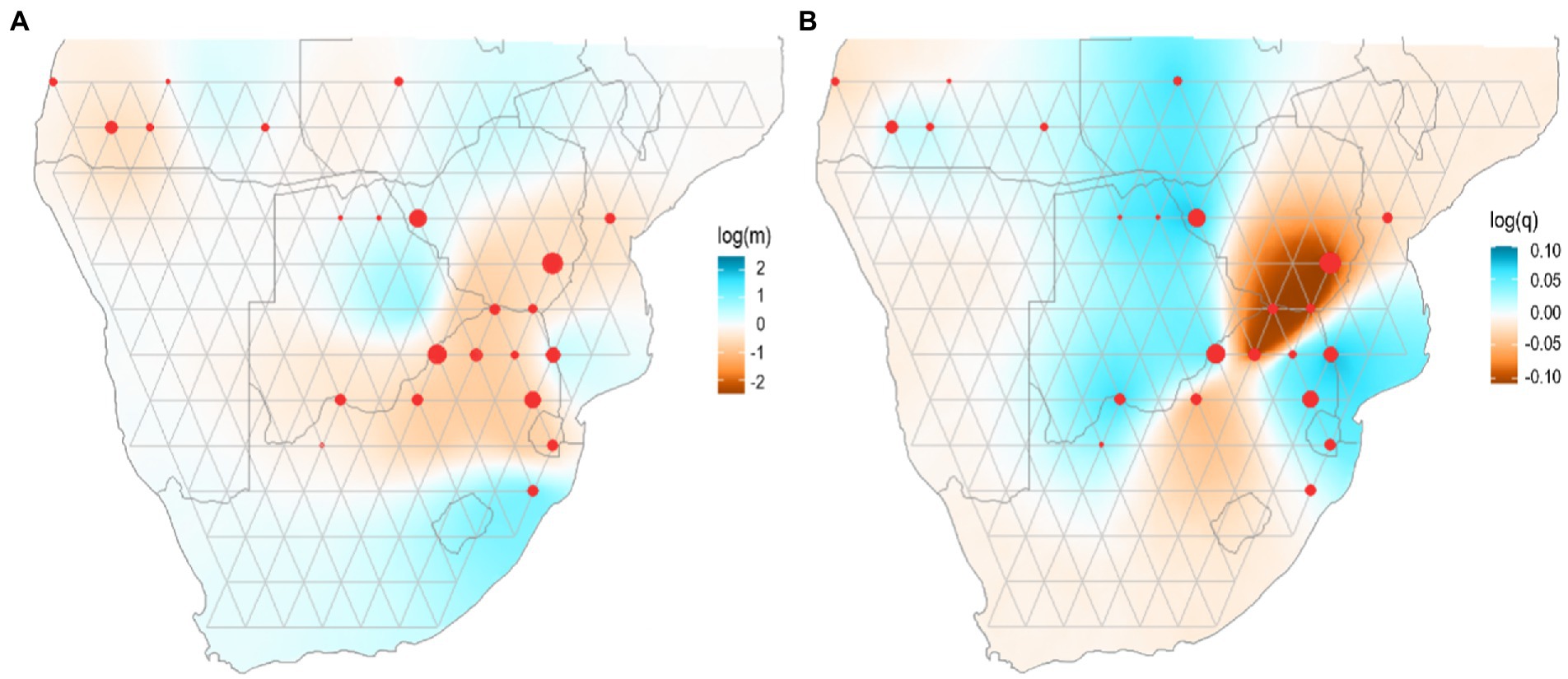
Figure 3. Estimates of effective migration and diversity surfaces inferred by EEMS for wild dog populations in southern Africa. (A) Blue colours represent estimated effective migration greater than would be expected under isolation-by-distance, and darker red colours represent increasingly lower migration. Log (m) is the posterior mean migration rate m (on a log10 scale). (B) Blue colours represent regions of elevated genetic diversity, and increasingly dark red colours represent regions of decreased genetic diversity. Log (q) is the posterior mean diversity rate q (on a log10 scale) Dots represent sampling localities.
Discussion
We investigated the population diversity, differentiation and connectivity of African wild dogs in southern Africa, including areas where they have recently been rediscovered, reestablished, or reintroduced. Our results point to four population clusters in southern Africa, exhibiting moderate levels of gene flow, and genetic diversity values that are comparable to those generally observed in large carnivores (Frankham, 1996; Ausband and Waits, 2020). We also found evidence of a few cases of long distance dispersal within southern Africa, which is supported by the absence of any impermeable dispersal barriers for wild dogs (Davies-Mostert et al., 2012) in an area that spans across roughly 2,260 km.
The first Bayesian-inferred split we observed in southern Africa identified wild dogs from the lowveld in Zimbabwe and the northern Limpopo Province (including part of the Waterberg) in South Africa as one cluster, and the remaining southern Africa wild dogs as a second cluster. The northern Limpopo area has recently been recolonized by wild dogs, very likely coming from the lowveld of Zimbabwe and eastern Botswana, as suggested by direct observation and radio-tracking data (D van der Merwe, personal communication), which support our results. Approximately 20 individuals reside in northern Limpopo (WAG-SA, 2022), exhibiting high relatedness (r = 0.17), low genetic diversity and a signature of bottleneck or of a founder effect, as expected in range expansions (Excoffier et al., 2009). It appears that dispersing wild dogs from the lowveld have also partially been the source of the current Waterberg population, which presented lower pairwise differentiation values with the lowveld than with Limpopo (FST = 0.05 and 0.07, respectively). Wild dogs in the lowveld and Limpopo showed the lowest values of genetic diversity (both expected heterozygosity and allelic richness), likely resulting from a reduced effective population size (Ne; Marsden et al., 2012). We believe that their signature of low diversity, low differentiation, and no deviations from formal genetic expectations may prevail in the Bayesian clustering analysis for the first split, which primarily groups individuals that best fit Hardy–Weinberg expectations (Pritchard et al., 2000). In contrast to our results, African wild dogs from the lowveld were previously identified as an admixed population between Kruger and Hwange/Okavango clusters, though based on a less comprehensive individual sampling in this region and a smaller set of markers (Marsden et al., 2012). On a finer-scale, previous population structuring identified the lowveld as a different cluster, but relatively well represented among Kruger individuals (Marsden et al., 2012).
The most geographically coherent number of genetic groups we found in this study is four, having high support from the estimated probability of data and low variance among runs. This partitioning of southern Africa wild dogs is consistent with the two main clusters in the region previously described (Marsden et al., 2012), i.e., Hwange/Okavango and Kruger, but elucidates for further structuring in populations that have not been considered before. In this respect, the managed metapopulation displayed a previously unknown signature of uniqueness, which was easily traced among the free-roaming wild dogs present in the Waterberg area (Tensen et al., 2019) and, not surprisingly, among individuals from the Gorongosa NP in Mozambique, as they have recently been successfully reintroduced into a large, unfenced landscape in the region (Bouley et al., 2021). Finally, the set of historic samples included in the Bayesian clustering analysis, from Angola, Zambia, Botswana and western Zimbabwe, assigned within the same cluster as all other individuals in this region, supporting a consistent spatial genetic structure of wild dogs across time. Although our sampling substantially secures the species’ range in southern Africa, we cannot disregard possible population nuclei of wild dogs harbouring genetic diversity and structure unsampled in our study.
Wild dogs are highly mobile animals that can implement fast and long dispersal movements, and are not limited by water bodies and unsuitable habitats (Davies-Mostert et al., 2012; Creel et al., 2020). Recent remarkable dispersal events reported for the species include a 345-km journey from Botswana to Zimbabwe in 9 days, and a 154-km journey from Botswana to Namibia in 5 days (Cozzi et al., 2020). Our results also illustrated a few likely events of long distance movements, namely (i) individuals assigned to the Angola/KAZA-TFCA cluster were sampled in the lowveld (N = 1), Limpopo (N = 1), the Waterberg area (N = 3) and Kruger, near the Shingwedzi rest camp (N = 1); and (ii) one individual assigned to the lowveld/Limpopo cluster was sampled in central Kruger, near Balule Nature Reserve. In the managed metapopulation, we observed individual assignments to the Angola/KAZA-TFCA cluster of wild dogs that were sampled in Marakele Game Reserve. This does not reflect dispersal events, however, but is rather the result of reintroductions that took place in 2003, as wild dogs in this area originated from five males that were caught in the Limpopo province and two females that were translocated from Botswana (SANParks, 2002). Based on field observations, it is also presumed that there has been natural immigration in and around the Madikwe and Khamab Kalahari game reserves of wild dogs that originate from Botswana (Bouley et al., 2021), which hopefully will lead to further genetic admixture in the area. The low genetic diversity in the lowveld of Zimbabwe warns for the benefit of possible mechanisms that allow natural dispersal within GLTFCA, which can counterbalance genetic drift and minimize possible stochastic effects (Alleaume-Benharira et al., 2006; Keller et al., 2014). In this regard, the observation of several individuals in the dataset that exhibit a genetic signature of admixture between different clusters (e.g., individuals in Hwange tracing back to the Kruger cluster; and in Limpopo tracing back to the Angola/KAZA-TFCA cluster) validates effective dispersal between wild dog genetic clusters and illustrates the maintenance of diversity even with limited gene flow.
The geographically coherent cluster revealed in the KAZA-TFCA region, which extends towards southwestern Angola, is highly concordant with independent results from telemetry data on the dispersal trajectories of wild dog coalitions, showing that no wild dogs were detected crossing the area just below the Okavango and Chobe NP (Cozzi et al., 2020). Human-populated landscapes and a matrix of agricultural fields and highways form an inhospitable landscape for the species and represent obstacles to dispersal in this region (Rasmussen, 1999; Cozzi et al., 2020). Despite these constraints, central Botswana and Zimbabwe still function as an effective dispersal area for wild dogs. Long-distance dispersal towards the southeast was observed in our genetic sampling and has been previously documented in this region (Davies-Mostert et al., 2012). However, the survival rate of dispersers is often very low (Creel and Creel, 2002), and gene flow resulting from dispersal may be reduced, also likely because of the wild dogs’ cooperative breeding system (Creel and Creel, 2002). Furthermore, low occupancy rates yield fewer opportunities for the formation of new packs (Hofmann et al., 2021). Altogether, this may cause the apparent decrease in effective gene flow towards the southeast.
After having suffered from civil unrest in Angola for almost four decades, wild dogs have recently been rediscovered in several different areas of the country (Monterroso et al., 2020). Nonetheless, war, urbanization, and persecution have severely limited population numbers in south central Angola. Additionally, major and heavily encroached rivers in the area, such as the Cunene and Cubango, could form limitations to dispersal (Overton et al., 2020), and limited prey across strongly disturbed landscapes could further restrict the movement of animals. Demographic declines are expected to be concurrent with anthropogenic habitat loss and persecution (Woodroffe et al., 1997), which are known to have repeatedly disturbed wild dog populations ubiquitously (Courchamp et al., 2000; Creel et al., 2004). Notwithstanding, we observed moderate to high estimates of effective migration within Angola, as well as comparable genetic diversity parameters for Bicuar/Mupa and Hwange, suggesting that wild dogs’ diversity in Angola may be maintained by gene flow with those in Zambia, Botswana and western Zimbabwe. Again, this is consistent with GPS data showing remarkable dispersal events across these countries (Cozzi et al., 2020; Hofmann et al., 2021).
In conclusion, we found that natural dispersal of wild dogs connects distant subpopulations across multiple countries in southern Africa. Some local genetic structuring and loss of diversity has occurred, which emphasizes the need to restore landscapes and maintain natural processes in human-dominated areas. Natural reestablishments and long-distance dispersal of wild dogs will hopefully aid a further demographic recovery in southern Africa. Furthermore, the wild dog managed metapopulation in South Africa has actively translocated wild dogs between private reserves every few years, to mimic gene flow and conserve genetic diversity (Tensen et al., 2019). Due to the success of the managed metapopulation, multiple wild dogs became available as founders for further range expansions (Bouley et al., 2021). Based on our results, there are no genetic constraints to moving wild dogs within southern Africa when the aim is to avoid admixing distinct evolutionary units (Bertola et al., 2022). However, it is increasingly being stressed that genetic metrics (such as inbreeding and relatedness) should be taken into account when planning management interventions such as translocations, to ensure the integrity of the recipient population (Becker et al., 2022). Ideally, individuals should be sourced from populations with high genetic diversity (Bertola et al., 2022), and geographic barriers or other biological factors that may drive ecological diversity should be considered (van Vuuren et al., 2017). The results of this study also illustrate the value of transfrontier conservation initiatives, like the Kavango-Zambezi and Greater Limpopo transfrontier conservation areas, in allowing the maintenance of large metapopulations of endangered fauna, and in particular of African wild dogs.
Benefit-sharing statement
A research collaboration was developed with scientists from the countries providing genetic samples, and all collaborators are included as co-authors. The results of this research will be shared with the provider communities and the broader scientific community. The research addresses a priority concern, in this case the conservation of African wild dogs.
Data availability statement
The raw data supporting the conclusions of this article will be made available by the authors, without undue reservation.
Ethics statement
The animal study was reviewed and approved by NSPCA, University of Johannesburg.
Author contributions
LT designed the research, conducted the literature and statistical analysis, and wrote the paper. RGo designed the research, provided samples and data, and contributed to the text and analytical tools. BJ advised on the research design, contributed analytical tools, and commented on the text. RGr provided samples, and commented on the statistical analyses and text. LB contributed to the genetic analysis. LB, HI, and GR contributed samples and commented on the statistical analyses and text. CP, HD-M, DM, FL, PM, GR, and FR contributed to acquisition of data. All authors contributed to the article and approved the submitted version.
Funding
LT worked under the UJ Global Excellence and Stature (UJ-GES) Fellowship (No. 2017337943) at the University of Johannesburg, and DFG funding (TE 1502/1-1) at the University of Koblenz-Landau. RG worked under a research contract from the Portuguese Foundation for Science and Technology (2021/00647/CEECIND).
Acknowledgments
The authors are deeply grateful to all the people who assisted in this study. We specifically thank the African Wildlife Conservation Funds and Endangered Wildlife Trust for their collaboration and willingness to share their samples with us. We also thank the Natural History Museum of Zimbabwe for allowing us to sample their collection, and Marjolein Schoe for her assistance during this process.
Conflict of interest
The authors declare that the research was conducted in the absence of any commercial or financial relationships that could be construed as a potential conflict of interest.
IS declared a shared affiliation with JT, BJ, and RG to the handling editor at time of review.
Publisher’s note
All claims expressed in this article are solely those of the authors and do not necessarily represent those of their affiliated organizations, or those of the publisher, the editors and the reviewers. Any product that may be evaluated in this article, or claim that may be made by its manufacturer, is not guaranteed or endorsed by the publisher.
Supplementary material
The Supplementary material for this article can be found online at: https://www.frontiersin.org/articles/10.3389/fevo.2022.992389/full#supplementary-material
References
Alleaume-Benharira, M., Pen, I. R., and Ronce, O. (2006). Geographical patterns of adaptation within a species’ range: interactions between drift and gene flow. J. Evol. Biol. 19, 203–215. doi: 10.1111/j.1420-9101.2005.00976.x
Ausband, D. E., and Waits, L. (2020). Does harvest affect genetic diversity in grey wolves? Mol. Ecol. 29, 3187–3195. doi: 10.1111/mec.15552
Becker, M. S., Almeida, J., Begg, C., Bertola, L., Breitenmoser, C., Breitenmoser, U., et al. (2022). Guidelines for evaluating the conservation value of African lion (Panthera leo) translocations. Front. Environ. Sci. 3:3. doi: 10.3389/fcosc.2022.963961
Bertola, L. D., Miller, S. M., Williams, V. L., Naude, V. N., Coals, P., Dures, S. G., et al. (2022). Genetic guidelines for translocations: maintaining intraspecific diversity in the lion (Panthera leo). Evol. Appl. 15, 22–39. doi: 10.1111/eva.13318
Blacket, M. J., Robin, C., Good, R. T., Lee, S. F., and Miller, A. D. (2012). Universal primers for fluorescent labelling of PCR fragments—an efficient and cost-effective approach to genotyping by fluorescence. Mol. Ecol. Res. 12, 456–463. doi: 10.1111/j.1755-0998.2011.03104.x
Bouley, P., Paulo, A., Angela, M., Du Plessis, C., and Marneweck, D. G. (2021). The successful reintroduction of African wild dogs (Lycaon pictus) to Gorongosa National Park, Mozambique. PloS One 16:e0249860. doi: 10.1371/journal.pone.0249860
Ceballos, G., Ehrlich, P. R., Barnosky, A. D., García, A., Pringle, R. M., and Palmer, T. M. (2015). Accelerated modern human–induced species losses: entering the sixth mass extinction. Sci. Adv. 1:e1400253. doi: 10.1126/sciadv.1400253
Chase, M. J., and Griffin, C. R. (2011). Elephants of south-East Angola in war and peace: their decline, re-colonization and recent status. Afr. J. Ecol. 49, 353–361. doi: 10.1111/j.1365-2028.2011.01272.x
Childes, S. L. (1988). The past history, present status and distribution of the hunting dog Lycaon pictus in Zimbabwe. Biol. Conserv. 44, 301–316. doi: 10.1016/0006-3207(88)90022-5
Collen, B., Turvey, S. T., Waterman, C., Meredith, H. M., Kuhn, T. S., Baillie, J. E., et al. (2011). Investing in evolutionary history: implementing a phylogenetic approach for mammal conservation. Philos. Trans. R. Soc. Lond. Ser. B Biol. Sci. 366, 2611–2622. doi: 10.1098/rstb.2011.0109
Courchamp, F., Clutton-Brock, T., and Grenfell, B. (2000). Multipack dynamics and the Allee effect in the African wild dog, Lycaon pictus. Anim. Conserv. 3, 277–285. doi: 10.1111/j.1469-1795.2000.tb00113.x
Cozzi, G., Behr, D. M., Webster, H. S., Claase, M., Bryce, C. M., Modise, B., et al. (2020). African wild dog dispersal and implications for management. J. Wildl. Managm. 84, 614–621. doi: 10.1002/jwmg.21841
Creel, S., and Creel, N. M. (2002). The African wild dog: Behavior, Ecology and Conservation. Princeton University Press, Princeton, UK.
Creel, S., Merkle, J., Mweetwa, T., Becker, M. S., Mwape, H., Simpamba, T., et al. (2020). Hidden Markov models reveal a clear human footprint on the movements of highly mobile African wild dogs. Sci. Rep. 10, 1–11. doi: 10.1038/s41598-020-74329-w
Creel, S., Mills, M. G., and McNutt, J. W. (2004). Demography and population dynamics of African wild dogs in three critical populations. Biology and conservation of wild canids. Oxford University Press, Oxford, UK. 337–350.
Daskin, J. H., and Pringle, R. M. (2018). Warfare and wildlife declines in Africa’s protected areas. Nature 553, 328–332. doi: 10.1038/nature25194
Daskin, J. H., Stalmans, M., and Pringle, R. M. (2016). Ecological legacies of civil war: 35-year increase in savanna tree cover following wholesale large-mammal declines. J. Ecol. 104, 79–89. doi: 10.1111/1365-2745.12483
Davies-Mostert, H. T., Kamler, J. F., Mills, M. G., Jackson, C. R., Rasmussen, G. S., Groom, R. J., et al. (2012). Long-distance transboundary dispersal of African wild dogs among protected areas in southern Africa. Afr. J. Ecol. 50, 500–506. doi: 10.1111/j.1365-2028.2012.01335.x
Devereux, S. (2009). Why does famine persist in Africa? Food security 1, 25–35. doi: 10.1007/s12571-008-0005-8
Di Rienzo, A., Peterson, A. C., Garza, J. C., Valdes, A. M., Slatkin, M., and Freimer, N. B. (1994). Mutational processes of simple-sequence repeat loci in human populations. Proc. Nat. Acad. Sci. 91, 3166–3170. doi: 10.1073/pnas.91.8.3166
Dirzo, R., and Raven, P. H. (2003). Global state of biodiversity and loss. Ann. Rev. Environm. Res. 28, 137–167. doi: 10.1146/annurev.energy.28.050302.105532
Earl, D. A., and Von Holdt, B. M. (2012). STRUCTURE HARVESTER: a website and program for visualizing STRUCTURE output and implementing the Evanno method. Conserv. Gen. Res. 4, 359–361. doi: 10.1007/s12686-011-9548-7
Edwards, C. T., Rasmussen, G. S., Riordan, P., Courchamp, F., and Macdonald, D. W. (2013). Non-adaptive phenotypic evolution of the endangered carnivore Lycaon pictus. PloS One 8:e73856. doi: 10.1371/journal.pone.0073856
Excoffier, L., Foll, M., and Petit, R. J. (2009). Genetic consequences of range expansions. Annu. Rev. Ecol. Evol. Syst. 40, 481–501. doi: 10.1146/annurev.ecolsys.39.110707.173414
Excoffier, L., and Lischer, H. E. (2010). Arlequin suite ver 3.5: a new series of programs to perform population genetics analyses under Linux and windows. Mol. Ecol Res. 10, 564–567. doi: 10.1111/j.1755-0998.2010.02847.x
Frankham, R. (1996). Relationship of genetic variation to population size in wildlife. Conserv. Biol. 10, 1500–1508. doi: 10.1046/j.1523-1739.1996.10061500.x
Frankham, R., Ballou, J. D., and Briscoe, D. A. (2004). A Primer of Conservation Genetics. Cambridge: Cambridge University Press.
Funston, P. J., Groom, R. J., and Lindsey, P. A. (2013). Insights into the management of large carnivores for profitable wildlife-based land uses in African savannas. PloS One 8:e59044. doi: 10.1371/journal.pone.0059044
Funston, P., Henschel, P., Petracca, L., Maclennan, S., Whitesell, C., Fabiano, E., et al. (2017). The distribution and status of lions and other large carnivores in Luengue-Luiana and Mavinga National Parks, Angola. KAZA TFCA Secretariat, Kasane, Botswana.
Fynn, R. W., and Bonyongo, M. C. (2011). Functional conservation areas and the future of Africa’s wildlife. Afr. J. Ecol. 49, 175–188. doi: 10.1111/j.1365-2028.2010.01245.x
Gaynor, K. M., Fiorella, K. J., Gregory, G. H., Kurz, D. J., Seto, K. L., Withey, L. S., et al. (2016). War and wildlife: linking armed conflict to conservation. Front. Ecol. Environm. 14, 533–542. doi: 10.1002/fee.1433
Girman, D. J., Vila, C., Geffen, E., Creel, S., Mills, M. G. L., McNutt, J. W., et al. (2001). Patterns of population subdivision, gene flow and genetic variability in the African wild dog (Lycaon pictus). Mol. Ecol. 10, 1703–1723. doi: 10.1046/j.0962-1083.2001.01302.x
Godinho, R., Llaneza, L., Blanco, J. C., Lopes, S., Alvares, F., Garcia, E. J., et al. (2011). Genetic evidence for multiple events of hybridization between wolves and domestic dogs in the Iberian Peninsula. Mol. Ecol. 20, 5154–5166. doi: 10.1111/j.1365-294X.2011.05345.x
Godinho, R., López-Bao, J. V., Castro, D., Llaneza, L., Lopes, S., Silva, P., et al. (2015). Real-time assessment of hybridization between wolves and dogs: combining noninvasive samples with ancestry informative markers. Mol. Ecol. Res. 15, 317–328. doi: 10.1111/1755-0998.12313
Gusset, M., Graf, J. A., and Somers, M. J. (2006). The re-introduction of endangered wild dogs into Hluhluwe-iMfolozi park, South Africa: an update on the first 25 years. Re-introduction NEWS 25, 31–33.
Hanson, T., Brooks, T. M., Da Fonseca, G. A., Hoffmann, M., Lamoreux, J. F., Machlis, G., et al. (2009). Warfare in biodiversity hotspots. Conserv. Biol. 23, 578–587. doi: 10.1111/j.1523-1739.2009.01166.x
Hofmann, D. D., Behr, D. M., McNutt, J. W., Ozgul, A., and Cozzi, G. (2021). Bound within boundaries: do protected areas cover movement corridors of their most mobile, protected species? J. Appl. Ecol. 58, 1133–1144. doi: 10.1111/1365-2664.13868
Höss, M., and Pääbo, S. (1993). DNA extraction from Pleistocene bones by a silica-based purification method. Nucl. Acids Res. 21, 3913–3914. doi: 10.1093/nar/21.16.3913
IUCN/SSC (2015). Review of the regional conservation strategy for the cheetah and African wild dogs in southern Africa. IUCN/SSC & Range Wide Conservation Program for Cheetah and African Wild Dogs, Gland, Switzerland. 2015. Available at: http://cheetahandwilddog.org
Jombart, T. (2008). Adegenet: a R package for the multivariate analysis of genetic markers. Bioinform. 24, 1403–1405. doi: 10.1093/bioinformatics/btn129
Kalinowski, S. T. (2005). Hp-rare 1.0: a computer program for performing rarefaction on measures of allelic richness. Mol. Ecol. Notes 5, 187–189. doi: 10.1111/j.1471-8286.2004.00845.x
Kalinowski, S. T., Wagner, A. P., and Taper, M. L. (2006). ML-relate: a computer program for maximum likelihood estimation of relatedness and relationship. Mol. Ecol. Notes 6, 576–579. doi: 10.1111/j.1471-8286.2006.01256.x
Kanyamibwa, S. (1998). Impact of war on conservation: Rwandan environment and wildlife in agony. Biodivers. Conserv. 7, 1399–1406. doi: 10.1023/A:1008880113990
Keller, S. R., Fields, P. D., Berardi, A. E., and Taylor, D. R. (2014). Recent admixture generates heterozygosity–fitness correlations during the range expansion of an invading species. J. Evol. Biol. 27, 616–627. doi: 10.1111/jeb.12330
Le Bel, S., Murwira, A., Mukamuri, B., Czudek, R., Taylor, R., and La Grange, M. (2011). Human wildlife conflicts in southern Africa: riding the whirl wind in Mozambique and in Zimbabwe. The importance of biological interactions in the study of biodiversity. ed. J. L. Pujol (IntechOpen), 283–322.
Lee, C., Abdool, A., and Huang, C. H. (2009). PCA-based population structure inference with generic clustering algorithms. BMC Bioinform. 10, 1–13. doi: 10.1186/1471-2105-10-S1-S73
Leigh, K. A. (2005). The ecology and conservation biology of the endangered African wild dog (Lycaon pictus). In the lower Zambezi, Zambia; PhD thesis.
Leigh, K. A., Zenger, K. R., Tammen, I., and Raadsma, H. W. (2012). Loss of genetic diversity in an outbreeding species: small population effects in the African wild dog (Lycaon pictus). Conserv. Genet. 13, 767–777. doi: 10.1007/s10592-012-0325-2
Lindsey, P., du Toit, R., Pole, A., and Romañach, S. (2012). “Savé Valley conservancy: a large-scale African experiment in cooperative wildlife management” in Evolution and innovation in wildlife conservation. eds. B. Child, H. Suich, and S. Anna (London: Routledge), 181–202.
Luikart, G., Allendorf, F. W., Cornuet, J. M., and Sherwin, W. B. (1998). Distortion of allele frequency distributions provides a test for recent population bottlenecks. J. Hered. 89, 238–247. doi: 10.1093/jhered/89.3.238
Marsden, C. D., Woodroffe, R., Mills, M. G., McNutt, J. W., Creel, S., Groom, R., et al. (2012). Spatial and temporal patterns of neutral and adaptive genetic variation in the endangered African wild dog (Lycaon pictus). Mol. Ecol. 21, 1379–1393. doi: 10.1111/j.1365-294X.2012.05477.x
Masenga, E. H., Jackson, C. R., Mjingo, E. E., Jacobson, A., Riggio, J., Lyamuya, R. D., et al. (2016). Insights into long-distance dispersal by African wild dogs in East Africa. Afr. J. Ecol. 54, 95–98. doi: 10.1111/aje.12244
Mills, M. G. L., Ellis, S., Woodroffe, R., Maddock, A., Stander, P., Rasmussen, G., et al. (1998). Population and habitat viability assessment for the African wild dog (Lycaon pictus) in southern Africa. Final workshop report. IUCN/SSC conservation breeding specialist group, apple Valley.
Monterroso, P., Rocha, F., van Wyk, S., António, T., Chicomo, M., Kosmas, S., et al. (2020). Updated ranges of the vulnerable cheetah and endangered African wild dog in Angola. Oryx 54, 851–853. doi: 10.1017/S0030605319000966
NGOWP (2022). National Geographic Okavango Wilderness Project. Okavango wilderness project|National Geographic Society. Accessed 2022 Apr.
Nicholson, S. K., Marneweck, D. G., Lindsey, P. A., Marnewick, K., and Davies-Mostert, H. T. (2020). A 20-year review of the status and distribution of African wild dogs (Lycaon pictus) in South Africa. Afr. J. Wildl. Res. 50, 8–19. doi: 10.3957/056.050.0008
Overton, J. M., Elizalde Castells, D., Figueira Fernandes Elizalde, S. R., Valério, H. M., Zumbo, A., Groom, R. J., et al. (2020). Endangered African wild dogs (Lycaon pictus Temm.) in Angola: filling a 50-year gap of knowledge with findings from two National Parks. Afr. J. Ecol. 58, 582–587. doi: 10.1111/aje.12715
Peakall, R. O. D., and Smouse, P. E. (2006). GENALEX 6: genetic analysis in excel. Population genetic software for teaching and research. Mol. Ecol. Notes 6, 288–295. doi: 10.1111/j.1471-8286.2005.01155.x
Petkova, D., Novembre, J., and Stephens, M. (2016). Visualizing spatial population structure with estimated effective migration surfaces. Nat. Gen. 48, 94–100. doi: 10.1038/ng.3464
Piry, S., Luikart, G., and Cornuet, J. M. (1999). Computer note. BOTTLENECK: a computer program for detecting recent reductions in the effective size using allele frequency data. J. Hered. 90, 502–503. doi: 10.1093/jhered/90.4.502
Pritchard, J. K., Stephens, M., and Donnelly, P. (2000). Inference of population structure using multilocus genotype data. Genetics 155, 945–959. doi: 10.1093/genetics/155.2.945
Rasmussen, G. S. A. (1999). Livestock predation by the painted hunting dog Lycaon pictus in a cattle ranching region of Zimbabwe: a case study. Biol. Conserv. 88, 133–139. doi: 10.1016/S0006-3207(98)00006-8
Rasmussen, G. S. A., and Macdonald, D. W. (2012). Masking of the zeitgeber: a frican wild dogs mitigate persecution by balancing time. J. Zool. 286, 232–242. doi: 10.1111/j.1469-7998.2011.00874.x
Riggio, J., Jacobson, A., Dollar, L., Bauer, H., Becker, M., Dickman, A., et al. (2013). The size of savannah Africa: a lion’s (Panthera leo) view. Biodivers. Conserv. 22, 17–35. doi: 10.1007/s10531-012-0381-4
Ripple, W. J., Estes, J. A., Beschta, R. L., Wilmers, C. C., Ritchie, E. G., Hebblewhite, M., et al. (2014). Status and ecological effects of the world’s largest carnivores. Science 343:1241484. doi: 10.1126/science.1241484
SANParks. (2002). Online article: Media release: Wild dog to Marakele National Park (http://sanparks.org).
Smith, C. H. (1840). The natural history of dogs: Canidæ or genus Canis of authors. Including also the genera Hyæna and Proteles. WH Lizars, S Highley, London; and W. Curry, jun. and Company Dublin.
Stalmans, M. E., Massad, T. J., Peel, M. J., Tarnita, C. E., and Pringle, R. M. (2019). War-induced collapse and asymmetric recovery of large-mammal populations in Gorongosa National Park. Mozambique. Plo S One 14:e0212864. doi: 10.1371/journal.pone.0212864
Team, R. C. (2015). R: A Language and Environment For Statistical Computing. Vienna, Austria. Available at: http://www.R-project.org
Tensen, L., Groom, R. J., Van Belkom, J., Davies-Mostert, H. T., Marnewick, K., and Jansen van Vuuren, B. (2016). Genetic diversity and spatial genetic structure of African wild dogs (Lycaon pictus) in the greater Limpopo transfrontier conservation area. Conserv. Gen. 17, 785–794. doi: 10.1007/s10592-016-0821-x
Tensen, L., van Vuuren, B. J., Du Plessis, C., and Marneweck, D. G. (2019). African wild dogs: genetic viability of translocated populations across South Africa. Biol. Conserv. 234, 131–139. doi: 10.1016/j.biocon.2019.03.033
Tinley, K. L. (1977). Framework of the Gorongosa ecosystem, Mozambique. Sc.(Ecology) Thesis University of Pretoria, Pretoria South Africa.
UNEP-WCMC and IUCN Protected Planet (2022). The world database on protected areas (WDPA) and world database on other effective area-based conservation measures (WD-OECM) [online], April 2022, Cambridge, UK. Available at: http://protectedplanet.net
van der Meer, E., Fritz, H., Blinston, P., and Rasmussen, G. S. (2014). Ecological trap in the buffer zone of a protected area: effects of indirect anthropogenic mortality on the African wild dog Lycaon pictus. Oryx 48, 285–293. doi: 10.1017/S0030605312001366
Van Oosterhout, C., Hutchinson, W. F., Wills, D. P., and Shipley, P. (2004). MICRO-CHECKER: software for identifying and correcting genotyping errors in microsatellite data. Mol. Ecol. Notes 4, 535–538. doi: 10.1111/j.1471-8286.2004.00684.x
van Vuuren, B. J., Rushworth, I., and Montgelard, C. (2017). Phylogeography of oribi antelope in South Africa: evolutionary versus anthropogenic panmixia. Afr. Zool. 52, 189–197. doi: 10.1080/15627020.2017.1386077
Whande, W., and Suich, H. (2012). “Transfrontier conservation initiatives in southern Africa: observations from the great Limpopo Transfrontier conservation area” in Evolution and innovation in wildlife conservation. eds. B. Child, H. Suich, and S. Anna (London: Routledge), 391–410.
Woodroffe, R., Ginsberg, J. R., and Macdonald, D. W. (1997). The African Wild Dog: Status Survey and Conservation Action Plan Iucn, IUCN, Gland, Switzerland and Cambridge, UK.
Keywords: African wild dog, effective dispersal, biodiversity conservation, Lycaon pictus, population genetic structure, transfrontier conservation areas
Citation: Tensen L, Jansen van Vuuren B, Groom R, Bertola LD, de Iongh H, Rasmussen G, Du Plessis C, Davies-Mostert H, van der Merwe D, Fabiano E, Lages F, Rocha F, Monterroso P and Godinho R (2022) Spatial genetic patterns in African wild dogs reveal signs of effective dispersal across southern Africa. Front. Ecol. Evol. 10:992389. doi: 10.3389/fevo.2022.992389
Edited by:
Alison G. Nazareno, Federal University of Minas Gerais, BrazilReviewed by:
Catherine Collins, University of Otago, New ZealandInnocent Sinthumule, University of Johannesburg, South Africa
Daniel M. Parker, University of Mpumalanga, South Africa
Copyright © 2022 Tensen, Jansen van Vuuren, Groom, Bertola, de Iongh, Rasmussen, Du Plessis, Davies-Mostert, van der Merwe, Fabiano, Lages, Rocha, Monterroso and Godinho. This is an open-access article distributed under the terms of the Creative Commons Attribution License (CC BY). The use, distribution or reproduction in other forums is permitted, provided the original author(s) and the copyright owner(s) are credited and that the original publication in this journal is cited, in accordance with accepted academic practice. No use, distribution or reproduction is permitted which does not comply with these terms.
*Correspondence: L. Tensen, tensen.laura@gmail.com; R. Godinho, rgodinho@cibio.up.pt