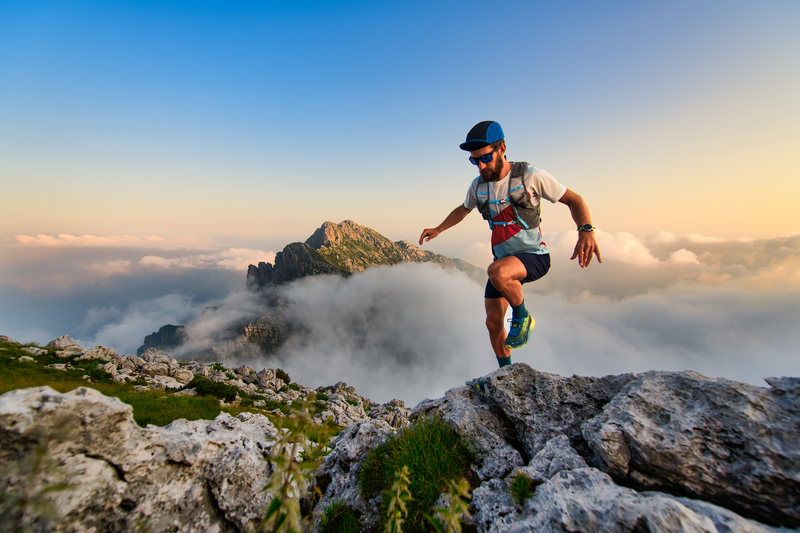
94% of researchers rate our articles as excellent or good
Learn more about the work of our research integrity team to safeguard the quality of each article we publish.
Find out more
ORIGINAL RESEARCH article
Front. Ecol. Evol. , 11 October 2022
Sec. Conservation and Restoration Ecology
Volume 10 - 2022 | https://doi.org/10.3389/fevo.2022.990835
This article is part of the Research Topic Biodiversity Conservation and Ecological Function Restoration in Freshwater Ecosystems View all 16 articles
The biodiversity-stability relationship is a fundamental subject of ecological research. Considerable evidence demonstrates that biodiversity can either increase or decrease stability. Most relevant research mainly focuses on grassland and forest ecosystems. The biodiversity-stability relationship in aquatic ecosystems and the underlying mechanisms remain poorly understood. To fill the gap, we conducted a year-long study on the phytoplankton of reservoir ecosystems in the Xiangxi Bay of Three Gorges Reservoir (TGR) to test the following hypotheses: (H1) phytoplankton species richness and functional diversity directly reduce phytoplankton community stability in reservoir ecosystems; (H2) nutrient enrichment and water temperature increasing directly reduce phytoplankton community stability; and (H3) nutrients and water temperature indirectly reduce phytoplankton community stability via biodiversity. The structural equation model (SEM) found that functional diversity (community-weighted means of traits and functional divergence) had significant negative correlations with phytoplankton community stability (p < 0.05), while the species diversity had no significant correlation with phytoplankton community stability (p > 0.05). This finding partially supported the hypothesis H1, which suggested that the functional diversity had a closer tie with stability than the species diversity. SEM did not find any direct effect of environmental factors on phytoplankton community stability, which rejected our hypothesis H2. Instead, SEM found that water temperature and phosphate decreased phytoplankton community stability by increasing the first principal component of the community-weighted means of traits (CWM_PC1), which supported hypothesis H3. Further analysis showed that the increased water temperature and phosphate concentration can promote “r-strategists” species (larger CWM_PC1), which are less resistant to environmental disturbances, therefore decreasing the phytoplankton community stability. Our study highlights the importance of functional diversity in maintaining the relationship between biodiversity and stability in the phytoplankton community, which may provide a mechanistic understanding of the biodiversity-stability relationships in aquatic ecosystems.
The relationship between biodiversity and ecosystem functioning is a central topic in ecological research (Ptacnik et al., 2008; Filstrup et al., 2014; Ye et al., 2019). A particularly pressing scientific challenge in the context of global change is to understand and predict the impact of biodiversity loss to ecosystem functions, because biodiversity is being lost at an unprecedented rate (Diaz et al., 2007; de Mazancourt et al., 2013; Leps et al., 2018). As one of the fundamental ecosystem functions, ecosystem stability is defined as the ability of a community to restore its original structure and functional state after disturbance (Loreau and de Mazancourt, 2013). The ecosystems that have higher stability are generally supposed to have a high ability to buffer the effects of environmental disturbances (de Bello et al., 2021). Therefore, understanding the drivers that promote ecosystem stability is essential to maintain biodiversity and ecosystem stability, and mitigate the adverse impacts of global change on ecosystems and biodiversity (de Bello et al., 2021; Van Meerbeek et al., 2021).
The relationship between biodiversity and stability is one of the long-term research interests for ecologists (MacArthur, 1955; McCann, 2000; de Bello et al., 2021). Both theoretical and empirical studies have shown that species diversity can either increase or decrease stability, depending on different intrinsic mechanisms (McCann, 2000; Ives and Carpenter, 2007). For example, Craven et al. (2018) reported that grassland community stability increases with grassland diversity increasing due to compensatory dynamics across species. Yet, for the ecosystems in which compensatory dynamics is not the mechanism for diversity dependence, a negative biodiversity-community stability relationship was also observed (Pfisterer and Schmid, 2002). So far, most knowledge on the biodiversity-stability relationship comes from grassland ecosystems and forest ecosystems (Craven et al., 2018; Bongers et al., 2021; Schnabel et al., 2021). Diffusion barriers in aquatic ecosystems are usually weak and energy and material flow within and between habitats are relatively rapid (Giller et al., 2004). Thus, it is usually considered that the dispersal rates of plankton in aquatic ecosystems are faster than other species in terrestrial ecosystems (Ptacnik et al., 2010), implying that aquatic organisms have a higher community turnover rate and may have different biodiversity-stability relationships comparing to terrestrial ecosystems. Exploring the biodiversity-stability relationships in aquatic ecosystems can help us deeper understand the mechanism of community maintenance in aquatic ecosystems, which is crucial for the future development of conservation strategies for aquatic ecosystems (Baert et al., 2016; Danet et al., 2021; Xu et al., 2021).
Beyond taxonomic diversity, there is a growing interest in investigating the relationship between traits-based functional diversity and ecosystem functioning (Ye et al., 2013, 2019; Gross et al., 2017; Bongers et al., 2021). Functional diversity is based on the various traits of species and their adaptations and responses to environmental change. Compared to taxonomic diversity, trait-based functional diversity can better reflect species resource acquisition and niche complementarity (Mason et al., 2005; Poos et al., 2009). Recently, ecologists have begun to focus on the effects of functional diversity and traits on stability (Mouillot et al., 2013; Oliver et al., 2015; de Bello et al., 2021). Theoretically, the dominant species effect, compensatory dynamics, and insurance effect are three of the major mechanisms explaining biodiversity-stability relationships (de Bello et al., 2021). Specifically, the dominant species effect is generally used to explain the relationships between stability and functional diversity; while, the theories of compensatory dynamics and insurance effect are applicable for explaining the relationships between stability and taxonomic and functional diversity (de Bello et al., 2021). The theory of the dominant species effect is derived from the mass-ratio theory (Grime, 1998) and holds that the resistance and recovery of community biomass after disturbance is related to the traits and functional roles of dominant species in the community, rather than to species diversity itself. The compensatory dynamics theories hypothesized that community species have countervailing responses to environmental change (Gonzalez and Loreau, 2009). While the theory of insurance effect emphasizes that the species with the same of similar effects on ecosystem functions have functional redundancy to maintain the community stability (Yachi and Loreau, 1999). Therefore, an interesting question is whether taxonomic or functional diversity is more closely related to the stability in aquatic ecosystems and the mechanism behind it.
Recently, several studies have shown the significance of functional trait-based life history strategies in understanding the functional diversity-stability relationships (Craven et al., 2018; Li et al., 2021; Schnabel et al., 2021). It is generally accepted that “K-strategists” species with larger individuals and more conservative resource acquisition show greater resistance (ability to withstand perturbation) but less resilience (recovery from perturbation) to a disturbance (MacGillivray et al., 1995; Karlowsky et al., 2018). On the contrary, “r-strategists” species with smaller size and rapid resource uptake can recover rapidly after a disturbance and show greater resilience. However, “r-strategists” species are less resistant in the face of environmental disturbances (Pianka, 1970). The “r-K strategists” continuum based on phytoplankton traits in freshwater ecosystems was proposed by Wentzky et al. (2020), who argued that a trait-based rather than taxonomic approach to classifying phytoplankton into “r-K strategists” species is more helpful in the understanding of how phytoplankton communities respond to changes in environmental conditions. Specifically, the “r-strategists” species were characterized by smaller cell size, stronger motility and clustering tendency, ability to take mixed nutrients, silicon-free access capability, and the “K-strategists” species were characterized by larger cell size, weaker motility and clustering tendency, lack of ability to take mixed nutrients, ability to obtain silicon. Previous studies on the phytoplankton “r-K strategists” continuum generally focus on how phytoplankton community structures in response to environmental factors (Wentzky et al., 2020). In this study, we try to investigate the mechanisms underlying the relationship between phytoplankton functional diversity and community stability from the conception of the phytoplankton “r-K strategists” continuum.
Phytoplankton is the basis of food webs in aquatic ecosystems (Litchman and Klausmeier, 2008), accounting for about 50% of total global primary production (Field et al., 1998). The phytoplankton diversity and dynamics are closely related to the biogeochemical cycling processes of elements (Falkowski et al., 2004). Therefore, studying the phytoplankton diversity-community stability relationship is essential for the development of conservation strategies in aquatic ecosystems. Most current research on phytoplankton diversity-community stability relationships is based on the compensatory and insurance effect (Ptacnik et al., 2008; Tian et al., 2017a,b). For example, Tian et al., 2017a,b reported that phytoplankton taxonomic diversity increases phytoplankton community stability due to compensatory effects. On the contrary, the dominant species effect in maintaining the phytoplankton diversity-stability relationship was seldom reported yet. Actually, in some dynamic ecosystems (e.g., reservoir ecosystems), the small-size phytoplankton likely dominate the community (Wang et al., 2011b; Pȩczuła et al., 2014). In this case, the dominant species effect may be the main mechanism explaining the relationship of phytoplankton diversity-stability. Therefore, following the theory of the dominant species effect, we hypothesized that phytoplankton diversity directly reduces phytoplankton community stability in reservoir ecosystems because of the dominance of small-size phytoplankton.
In contrast, the impact of changes in environmental factors on biodiversity-stability relationships has received extensive attention from scholars (De Laender et al., 2016; Craven et al., 2018; Li et al., 2021; Schnabel et al., 2021). Phytoplankton community stability is not only affected by diversity but also directly affected by the environment (Hillebrand et al., 2018; Polazzo and Rico, 2021). Research showed that warming will increase the proportion of small-size species in aquatic ecosystems (Daufresne et al., 2009). In the light of this research, we hypothesized that water temperature increase will result in the dominance of small-size phytoplankton species (“r-strategists” species), therefore reducing the phytoplankton community stability. Meanwhile, nutrient enrichment has been recognized as the major factor causing cyanobacterial blooms (Lurling et al., 2018), which was generally constituted by small body-size cyanobacteria (e.g., Microcystis sp.) (Filstrup et al., 2014). Therefore, we also hypothesized that nutrient enrichment would reduce the phytoplankton community stability because of the increment of the proportion of small body-size cyanobacteria.
We conducted a year-long study on the phytoplankton of natural aquatic ecosystems in the Xiangxi Bay of Three Gorges Reservoir (TGR). Using the weekly dynamic time series of phytoplankton communities and environmental variables, the causal network between environmental variables, biodiversity, and community stability was reconstructed using piecewise structural equation modeling, which examines the relationship between environmental variables, biodiversity, and community stability. Specifically, we tested the following hypotheses:
H1: Phytoplankton species richness and functional diversity directly reduce phytoplankton community stability in reservoir ecosystems.
H2: Nutrient enrichment and water temperature increase directly reduce phytoplankton community stability.
H3: Nutrients and water temperature indirectly reduce phytoplankton community stability via biodiversity.
The field sampling site was set up in the middle region of Xiangxi Bay of the TGR (Figure 1). The TGR is located in Chongqing and Hubei Province in the upper reaches of the Yangtze River, with a total water surface area of 1,080 square kilometers and a total storage capacity of 39.3 billion m3 (Ye et al., 2022). The TGR is the most giant strategic reservoir of freshwater resources in China and has huge benefits for water resource allocation (Ye et al., 2016). Xiangxi Bay is the largest tributary of the TGR in Hubei Province. After the TGR was impounded, the downstream section of the river for dozens of kilometers became a lake-like slow-flowing water body, called Xiangxi Bay (Ye et al., 2007). We choose Xiangxi Bay as the study site because the previous study showed that small-size species dominate the phytoplankton community (Wang et al., 2011b), which provides an excellent natural study site to test our research hypotheses. The local climate is subtropical monsoon, characterized by warm winters and hot summers, cold early spring and autumn, cloudy and breezy, high humidity, heavy rain, and little frost (Wang et al., 2011a).
Figure 1. Geographical location of sampling site in the Xiangxi Bay of Three Gorges Reservoir (TGR).
The phytoplankton and related environmental data used in this study were obtained from the Xiangxi Bay Ecosystem Station of TGR. Specifically, weekly data from 29 February 2008 to 3 April 2009, for the site in the middle of Xiangxi Bay (Figure 1) was selected to test our research hypotheses. During the study period, the weather in the area was typical, with no unusual occurrences of hotter or wetter weather than the annual average (Wang et al., 2011a). The details for the field sampling, phytoplankton, and environmental factors analyses can be found in Ye et al. (2007) and Wang et al. (2011b). In simple terms, the water temperature (WT) was measured in situ by a multi-parameter water quality sonde (YSI 6600, YSI Inc., Yellow Springs, OH, United States). The water samples were collected at the surface layer (0.5 m depth) for water quality analyses and phytoplankton identification and counting. The water quality variables, including dissolved inorganic nitrogen (DIN, the sum of the ammonia nitrogen, nitrite nitrogen, and nitrate nitrogen), phosphate (PO4-P), dissolved silicate (DSi), were analyzed by the segmented flow analyzer (Skalar SAN++, Netherlands) in the lab. Phytoplankton was counted in the Fuchs–Rosenthal chamber by a microscope (Olympus CX21, Japan) at 10 × 40 magnification. The phytoplankton species identification was referred to by John et al. (2002) and Hu and Wei (2006). The summary statistics of the above environmental variables including nutrients and water temperature could be found in (Supplementary Table 1) in the supporting information.
The diversity indices include, species richness (SR), community-weighted means (CWM) of traits (Lavorel et al., 2008), and functional divergence (FDiv) (Villeger et al., 2008) were used to investigate the biodiversity-stability relationship. CWM represents the effect of dominant species in a community on ecosystem stability (de Bello et al., 2021). FDiv represents the degree of ecological niche complementarity among community species (Lavorel et al., 2008). The CWM and FDiv are selected to investigate whether the dominant species effect or the insurance effect is the mechanism determining the relationship between phytoplankton diversity and stability in our study.
Following Weithoff (2003) and Ye et al. (2019), the functional diversity indices were calculated based on five functional traits, including (1) motility, (2) cell size, (3) silica use, (4) mixotrophy, and (5) chains or colonies. Specifically, Motility is classified as 0, no motility; 0.5, motility regulated by air bubbles in the body; and 1, flagellated and able to move actively in water. The cell size takes values ranging from 0.1 to 1. The last three functional traits are binary variables (0, 1), with 1 for having the ability and 0 for not having the ability (Ye et al., 2019).
To reduce the dimension of community-level traits, we performed principal component analysis (PCA) on the community-weighted means eigen values CWM of these five traits. The first principal component explained 73.41% of the total variation of the community-weighted means eigen values of the five traits (CWM_PC1) (Craven et al., 2018). The Bi-plot of principal component analysis (Supplementary Figure 1) and its loadings of community weight mean of five functional traits (Supplementary Table 2) suggest an “r-K strategists” continuum in life history. Specifically, a larger value of CWM_PC1 corresponds to “r-strategists” species, characterized by smaller cell size, stronger motility and clustering tendency, ability to take mixed nutrients, and silicon-free access capability. Whereas a smaller value of CWM_PC1 corresponds to “K-strategists” species, characterized by larger cell size, weaker motility and clustering tendency, lack of ability to take mixed nutrients, and ability to obtain silicon (Wentzky et al., 2020). PCA was performed with the “PCA” function in the “FactoMineR” package (Lê et al., 2008). Calculation of functional diversity using the “FD” package (Laliberté and Legendre, 2010; Laliberté et al., 2014).
The temporal stability of phytoplankton is calculated as the Bray–Curtis similarity (BC) between phytoplankton community samples sampled in two adjacent weeks (Ptacnik et al., 2008; Filstrup et al., 2014; Tian et al., 2017b). The values of BC were calculated by Equation 1. Specifically, in Equation 1, Wi1 and Wi2 are the biomass of phytoplankton species i in the two samples; n is the total number of phytoplankton species present in the two sampling samples. The numerator of the above equation represents the summed variation of biomass; the denominator represents the total biomass. Exploring the changes in the numerator and denominator can provide further insight into the mechanisms of community stability changes (Tian et al., 2017b). The BC value is ranged from 0 to 1, and a higher BC value represents higher community stability (Filstrup et al., 2014).
To test our hypotheses, we used piecewise structural equation models (SEMs) to analyze the direct and indirect causal relationships between community stability and environmental and biotic variables. SEM is widely used in the field of ecology to investigate direct or indirect relationships between study variables (Schnabel et al., 2021; Li et al., 2022). Following Craven et al. (2018) and Schnabel et al. (2021), we fitted the piecewise SEM using the linear regression models. Based on prior knowledge (Ptacnik et al., 2008; Xu et al., 2021), we hypothesized that biodiversity diversity directly improved community stability and its components (i.e., total biomass and summed variation of biomass) and that these indicators are regulated by environmental variables. In other words, environmental variables can affect the stability of the phytoplankton community, either directly or indirectly, by influencing biodiversity. We built our SEMs using the “piecewiseSEM” package (Lefcheck, 2016).
The relative importance of the two components of stability (i.e., summed variation of biomass and total biomass) in explaining community stability was investigated using linear regression and variance partitioning analysis (VPA). VPA was performed with 999 permutation tests to obtain significance. VPA used the “varpart” function in the “vegan” package (Oksanen et al., 2019). All data analysis was performed on R 4.1.2 (R Core Team, 2021). In all analyses, environmental factors, biodiversity (except CWM_PC1), community stability and phytoplankton biomass were log2 transformed to meet normality assumptions (Craven et al., 2018; Schnabel et al., 2019).
The weekly dynamics of phytoplankton community stability calculated by the Bray–Curtis similarity were presented in Figure 2A. It can be seen from the figure that though there was substantial month-to-month variation, community stability was generally lower from March 2008 to October 2008, compared to November 2008 to January 2009. And the community was most stable on 6 March 2009, and the community stability decreased from 6 March 2009, onward. This suggests that the phytoplankton community is more stable from late autumn to early winter compared to spring and summer, but longer-term testing would be needed to confirm this. Components of community stability whose weekly dynamics of summed variation of biomass trended in the opposite direction to that of community stability (Figure 2B). That is, both summed variation of biomass and total biomass were significantly and negatively correlated with phytoplankton community stability (Figures 3A,B), suggesting there was a correlation between high community stability and lower total phytoplankton biomass variation from the points of 3 October 2008, 5 December 2008, and 6 March 2009. Summed variation of biomass and total biomass explained 51 and 23% of the phytoplankton community stability, respectively, and together they explained 14% of the variation in community stability (Figure 3C).
Figure 2. Weekly dynamics of phytoplankton community stability (Bray–Curtis similarity) (A) and the components of Bray–Curtis similarity shown in panels (A,B) in the Xiangxi Bay of Three Gorges Reservoir (TGR).
Figure 3. Linear relationships between phytoplankton community stability (Bray–Curtis similarity) and summed variation of biomass (A) and total biomass (B). (C) Shows the variance partitioning result for phytoplankton community stability. *p < 0.05, **p < 0.01, and ***p < 0.001.
Our structural equation modeling provides insight into the relationship between biodiversity, environmental factors, and phytoplankton community stability, which provides strong evidence that biodiversity is a key mediator regulating the environmental factor-phytoplankton community stability relationship, and that biodiversity is driven by environmental factors. Overall, our model achieved an acceptable fitness (Fisher’s C = 3.661, and p-value = 0.454) (Figure 4A). All variables together explained 33% of the variation in community stability (R2 = 0.33). The pathway with the greatest impact on community stability was (standardized path coefficient of direct effect = −0.50) CWM_PC1, which directly reduced community stability, and FDiv had a similarly strong negative effect on community stability (standardized path coefficient of direct effect = −0.37). Direct pathways of SR effects on community stability were not significant. Furthermore, we found that PO4-P and WT affected stability through indirect pathways, i.e., WT and PO4-P enhanced CWM_PC1 to reduce community stability. The direct pathways of PO4-P, WT, DIN, and DSi on community stability were not significant (Figure 4A).
Figure 4. Piecewise structural equation model (SEM) depicts the relationships between the environment variables, biodiversity and community stability (A). Overall, the model fits the data well: Fisher’s C = 3.661, AIC = 59.661, and p-value = 0.454. Piecewise SEM depicts the relationships between the environment variables, biodiversity and community stability components (B). The model fit the data well: Fisher’s C = 3.661, AIC = 79.661, and p-value = 0.454. The solid and dashed arrows represent positive and negative relationships, respectively, and the light-gray arrows represent non-significant relationships (p > 0.05). Dissolved silicate (DSi), phosphate (PO4-P), dissolved inorganic nitrogen (DIN), water temperature (WT), phytoplankton species richness (SR), the first principal component of the community-weighted means of traits (CWM_PC1), functional divergence (FDiv), Bray–Curtis similarity between phytoplankton community samples sampled in two adjacent weeks (Bray–Curtis similarity), the total biomass of phytoplankton samples from two adjacent weeks (Total biomass), summed variation of biomass of phytoplankton samples from two adjacent weeks (Summed variation of biomass).
Further, we investigated how environmental factors and diversity affect the community stability for a deeper understanding of the mechanisms behind the biodiversity-stability relationship (Figure 4B). All variables together explained 40% of the total biomass (R2 = 0.40) and 44% of the summed variation of biomass (R2 = 0.44). We can see that all the paths that affect the total biomass are not significant (Figure 4B), and the path that has the greatest impact on the summed variation of biomass (standardized path coefficient of direct effect = 0.48) is that CWM_PC1 directly increases the summed variation of biomass, which indicates that CWM_PC1 decreases community stability by increasing summed variation of biomass. Similar to CWM_PC1, species richness also improved the summed variation of biomass (standardized path coefficient of direct effect = 0.47). Environmental variables affect the summed variation of biomass through direct or indirect pathways, WT increases the summed variation of biomass by promoting CWM_PC1 and species richness, thereby reducing the community stability. PO4-P positively acting on CWM_PC1 increased the summed variation of biomass, while negatively acting on SR decreased the summed variation of biomass. DIN can reduce the summed variation of biomass directly or by reducing SR (Figure 4B).
We found that phytoplankton community stability had no significant correlation with the species richness but had a significant negative correlation with CWM_PC1 (Figure 4A). This finding revealed that CWM_PC1 is the most important biotic factor driving phytoplankton community stability (Figure 4A) and supported our hypothesis that functional diversity directly reduced phytoplankton community stability in reservoir ecosystems. In our case, larger values of CWM_PC1 indicated that the phytoplankton community was more unstable because the community was dominated by “r-strategists” species (characterized by smaller cell size, stronger motility and clustering tendency, ability to take mixed nutrients, and silicon-free access capability). Conversely, smaller values of CWM_PC1 indicated that the phytoplankton community dominated by “K-strategists” species (characterized by larger cell size, weaker motility and clustering tendency, lack of ability to take mixed nutrients, and ability to obtain silicon), which can maintain a more stable community. We also found that CWM_PC1 reduced community stability by increasing summed variation of biomass (Figure 4B). When communities were dominated by “r-strategists” species, they were less resistant to environmental stresses and thus showed greater variation in community biomass, which reduced community stability (Donohue et al., 2013).
Our study is consistent with a recent study conducted in grassland ecosystems (Craven et al., 2018). Craven et al. (2018) found that communities dominated by “slow” species (low specific leaf area, low leaf N and P, and high leaf dry matter content) are more stable than those dominated by “fast” species (high specific leaf area, high leaf N and P, and low leaf dry matter content) because “slow” species can enhance ecosystem stability (the ratio of the mean and standard deviation of biomass) by increasing mean above-ground biomass production. When considering different disturbances, communities species dominated by “r-strategists” species can maintain community stability when a system is subjected to persistent environmental fluctuations (environmental stochasticity) due to stochastic factors in the biotic or abiotic environment due to the ability to recover quickly, while communities dominated by “K-strategists” species may maintain community stability in the face of pulse perturbation (Donohue et al., 2016; Kefi et al., 2019). The “r-strategists” species should be more resilient, while the “K-strategists” species should be more resistant (Kefi et al., 2019). Previous studies showed that water level fluctuations will affect the dynamic of phytoplankton in the TGR (Wang et al., 2011a,b; Ye et al., 2022). Therefore, how water level fluctuations influence the phytoplankton diversity-stability relationships is an interesting research question and should be explored in future studies.
Structural equation model found a significant negative relationship between FDiv and community stability (Figure 4A). This is contrary to some previous studies on the phytoplankton diversity-stability relationships (Ptacnik et al., 2008; Tian et al., 2016, 2017b). However, the negative correlation between phytoplankton biodiversity (evenness) and phytoplankton community stability was supported by Filstrup et al. (2014). Filstrup et al. (2014) studied the lakes are eutrophic and the phytoplankton communities were dominated by a few dominant species of the cyanobacteria, especially the Microcystis sp., limiting the growth and reproduction of other phytoplankton species. The reduction in phytoplankton community turnover in cyanobacteria-dominated ecosystems indicates an “inhibition model” of succession (Connell and Slatyer, 1977), similar to the formation of closed canopy shrubs in terrestrial ecosystems, and inhibits the growth of trees. Microcystis blooms can also reduce weekly community turnover by competitively excluding or inhibiting other taxa through the efficient use of TP supply (Reynolds, 2006) or the chemosensory effects of secondary metabolites (Pflugmacher, 2002). Previous studies showed that Xiangxi Bay is facing a serious eutrophication problem because of the pollutants in its watershed and the associated phytoplankton bloom in the bay (Wang et al., 2011b; Ye et al., 2014, 2022). Specifically, in our study, cyanobacterial biomass accounted for 51% of the total phytoplankton community biomass, and the cyanobacterial was dominated by Microcystis sp. and Anabaena sp., which is consistent with the study reported by Filstrup et al. (2014), suggesting the biodiversity-stability relationship was also determined by the dominant species effect.
We note that CWM_PC1 has a significant negative effect on FDiv (standardized path coefficient of direct effect = −0.73) (Figure 4A). FDiv describes the formation of species clusters in the character space, where a higher degree of divergence indicates that species groups are distributed at the edges of the character space (Mason et al., 2005; Villeger et al., 2008). For example, divergence is lower when the most abundant species have functional traits close to the center of the functional trait range; conversely, divergence is higher when the most abundant species have extreme functional trait values. In multivariate environments, functional divergence is related to the distribution of abundance within the functional trait space occupied by the species (Villeger et al., 2008). CWM is defined as the average of the values present in the community, weighted by the relative abundance of taxa with each value (Lavorel et al., 2008), Lavorel et al. (2008) argue that both CWM and FDiv measures require characterization of taxa and information on their relative abundance, and thus both CWM and FDiv depend on the characterization values among the more abundant species within the community distribution of the two indices, and there is some similarity in the properties of these two indices. We also found that an increase in the CWM_PC1 index caused a decrease in the FDiv index, i.e., the dominance of “r-strategists” species led to a decrease in FDiv, and since FDiv has a negative effect on stability, CWM_PC1 indirectly maintained phytoplankton community stability by decreasing FDiv.
Our study has not found a significant relationship between species richness and phytoplankton community stability (Figure 4A). Functional diversity reflects the differentiation of functional traits of community species, and trait-based functional diversity better explains the variation of phytoplankton community stability through ecological niche differentiation (Mouillot et al., 2013; de Bello et al., 2021). The functional diversity index was suggested to be superior to species diversity in explaining the stability of the phytoplankton community.
Our SEM found that water temperature and phosphate indirectly reduced phytoplankton community stability via functional diversity, which supports hypothesis H3. The direct effects of the four environmental factors on phytoplankton community stability were not significant. It is widely considered that warming will affect the size structure of organisms in ecosystems (Daufresne et al., 2009) and thereafter changes ecosystem function directly or indirectly (Burgmer and Hillebrand, 2011; Yvon-Durocher et al., 2015). Our study shows that WT increases summed variation of biomass by increasing CWM_PC1 values (Figures 4A,B) (i.e., increasing temperature promotes the growth of “r-strategists” species and suppresses the growth of “K-strategists” species), thus reducing community stability. At the same time, our study is consistent with previous findings that elevated temperatures affect biodiversity, which disrupts ecosystems and increases the volatility of phytoplankton community biomass (Beninca et al., 2011; Bestion et al., 2021). Wentzky et al. (2020) found that spring phytoplankton communities with lower temperatures were dominated by “r-strategists” species, and summer phytoplankton communities with higher temperatures were dominated by “K-strategists” species. Our results differ from the study of Wentzky et al. (2020), and further comparisons show that the lakes studied by Wentzky et al. (2020) differed not only in temperature but also in nutrients between spring and summer, i.e., higher nutrient content in spring and lower nutrient content in summer lakes. Differences in nutrient content between seasons are also factors to be considered in causing a species shift in the “r-K strategists.”
PO4-P was positively correlated with CWM_PC1, indicating that PO4-P promoted the growth of “r-strategists” species and increased summed variation of biomass, thus reducing community stability (Figures 4A,B). According to previous studies in Xiangxi Bay, phosphorus was found to be the main limiting nutrient for algal growth and blooms in this bay (Huang et al., 2020). According to the Redfield ratio (Redfield, 1958), the stoichiometric ratio of 16N: 1P (in atoms) is a widely used criterion in determining nutrient limitation in aquatic ecosystems. According to our study, we found that the N/P ratio was greater than 16 in 42 of 57 samples, indicating that the phytoplankton in Xiangxi Bay may be limited by phosphorus resources. Nutrient inputs are considered to be important drivers of species turnover (Jochimsen et al., 2013) and diversity (Lehtinen et al., 2017), suggesting that diversity may be a necessary mediator of nutrient effects on stability. The study by Jochimsen et al. (2013) also reported that community changes were closely related to changes in phosphorus, i.e., as nutrients increased, increases in some taxa were offset by decreases in others, thus altering the resilience of phytoplankton community biomass. Therefore, the increase in effective phosphorus concentration in Xiangxi Bay may increase the risk of autumn blooms, suggesting that management strategies need to pay special attention to phosphorus loading and control.
In this study, we investigated how environmental factors, species and functional diversity affect phytoplankton community stability. We found that the functional diversity indices, community-weighted means of traits and functional divergence, had significant negative correlations with phytoplankton community stability (p < 0.05), while the species richness had no significant relationship with the phytoplankton community stability (p > 0.05). This indicated that functional diversity can better explain phytoplankton diversity and community stability than species richness. Meanwhile, we found that the environmental factors have no direct impact on stability, but indirectly reduce phytoplankton community stability through changing functional diversity. Specifically, water temperature and phosphate are the most important environmental factors indirectly affecting phytoplankton community stability. We found that increasing water temperature and PO4-P will promote the first principal component of the community-weighted means of traits (CWM_PC1), and thereafter decrease phytoplankton community stability. Further analysis found that the increased water temperature and PO4-P concentration promoted the growth of “r-strategists” species and inhibited the growth of “K-strategists” species, thereby reducing phytoplankton community stability. Our study suggests that global warming and eutrophication will bring significant negative effects on phytoplankton community stability and highlights the important role of functional diversity and traits in maintaining the stability of aquatic ecosystems.
The datasets used and/or analyzed during the current study are available from the corresponding author upon reasonable request.
XZ was responsible for the analyses and interpretation of the data and wrote the original draft. QC was responsible for the conception. LT provided the methodology and conducted the sample analysis. LY was responsible for the administration and supervision of the project and writing—review and editing and contributed to the conception. All authors read and approved the final manuscript.
This study was supported by the National Key R&D Program of China (2018YFD0900902) and the National Natural Science Foundation of China (31670534 and 31300392).
This manuscript was inspired by discussions with ecologists in the field of ecology, we are grateful to Zhouyuan Li (Beijing Forestry University) and Wang Tian (North China Electric Power University) for providing manuscript ideas and theoretical support. We also thank two reviewers for their valuable comments and suggestion in improving this manuscript.
The authors declare that the research was conducted in the absence of any commercial or financial relationships that could be construed as a potential conflict of interest.
All claims expressed in this article are solely those of the authors and do not necessarily represent those of their affiliated organizations, or those of the publisher, the editors and the reviewers. Any product that may be evaluated in this article, or claim that may be made by its manufacturer, is not guaranteed or endorsed by the publisher.
The Supplementary Material for this article can be found online at: https://www.frontiersin.org/articles/10.3389/fevo.2022.990835/full#supplementary-material
Baert, J. M., De Laender, F., Sabbe, K., and Janssen, C. R. (2016). Biodiversity increases functional and compositional resistance, but decreases resilience in phytoplankton communities. Ecology 97, 3433–3440. doi: 10.1002/ecy.1601
Beninca, E., Dakos, V., Van Nes, E. H., Huisman, J., and Scheffer, M. (2011). Resonance of plankton communities with temperature fluctuations. Am. Nat. 178, E85–E95. doi: 10.1086/661902
Bestion, E., Haegeman, B., Codesal, S. A., Garreau, A., Huet, M., Barton, S., et al. (2021). Phytoplankton biodiversity is more important for ecosystem functioning in highly variable thermal environments. Proc. Natl. Acad. Sci. U.S.A. 35:118. doi: 10.1073/pnas.2019591118
Bongers, F. J., Schmid, B., Bruelheide, H., Bongers, F., Li, S., von Oheimb, G., et al. (2021). Functional diversity effects on productivity increase with age in a forest biodiversity experiment. Nat. Ecol. Evol. 5, 1594–1603. doi: 10.1038/s41559-021-01564-3
Burgmer, T., and Hillebrand, H. (2011). Temperature mean and variance alter phytoplankton biomass and biodiversity in a long-term microcosm experiment. Oikos 120, 922–933. doi: 10.1111/j.1600-0706.2010.19301.x
Connell, J. H., and Slatyer, R. O. (1977). Mechanisms of succession in natural communities and their role in community stability and organization. Am. Nat. 111, 1119–1144. doi: 10.1086/283241
Craven, D., Eisenhauer, N., Pearse, W. D., Hautier, Y., Isbell, F., Roscher, C., et al. (2018). Multiple facets of biodiversity drive the diversity-stability relationship. Nat. Ecol. Evol. 2, 1579–1587. doi: 10.1038/s41559-018-0647-7
Danet, A., Mouchet, M., Bonnaffe, W., Thebault, E., and Fontaine, C. (2021). Species richness and food-web structure jointly drive community biomass and its temporal stability in fish communities. Ecol. Lett. 24, 2364–2377. doi: 10.1111/ele.13857
Daufresne, M., Lengfellner, K., and Sommer, U. (2009). Global warming benefits the small in aquatic ecosystems. Proc. Natl. Acad. Sci. U.S.A. 106, 12788–12793. doi: 10.1073/pnas.0902080106
de Bello, F., Lavorel, S., Hallett, L. M., Valencia, E., Garnier, E., Roscher, C., et al. (2021). Functional trait effects on ecosystem stability: assembling the jigsaw puzzle. Trends Ecol. Evol. 36, 822–836. doi: 10.1016/j.tree.2021.05.001
De Laender, F., Rohr, J. R., Ashauer, R., Baird, D. J., Berger, U., Eisenhauer, N., et al. (2016). Reintroducing environmental change drivers in biodiversity-ecosystem functioning research. Trends Ecol. Evol. 31, 905–915. doi: 10.1016/j.tree.2016.09.007
de Mazancourt, C., Isbell, F., Larocque, A., Berendse, F., De Luca, E., Grace, J. B., et al. (2013). Predicting ecosystem stability from community composition and biodiversity. Ecol. Lett. 16, 617–625. doi: 10.1111/ele.12088
Diaz, S., Lavorel, S., de Bello, F., Quetier, F., Grigulis, K., and Robson, M. (2007). Incorporating plant functional diversity effects in ecosystem service assessments. Proc. Natl. Acad. Sci. U.S.A. 104, 20684–20689. doi: 10.1073/pnas.0704716104
Donohue, I., Hillebrand, H., Montoya, J. M., Petchey, O. L., Pimm, S. L., Fowler, M. S., et al. (2016). Navigating the complexity of ecological stability. Ecol. Lett. 19, 1172–1185. doi: 10.1111/ele.12648
Donohue, I., Petchey, O. L., Montoya, J. M., Jackson, A. L., McNally, L., Viana, M., et al. (2013). On the dimensionality of ecological stability. Ecol. Lett. 16, 421–429. doi: 10.1111/ele.12086
Falkowski, P. G., Katz, M. E., Knoll, A. H., Quigg, A., Raven, J. A., Schofield, O., et al. (2004). The evolution of modern eukaryotic phytoplankton. Science 305, 354–360. doi: 10.1126/science.1095964
Field, C. B., Behrenfeld, M. J., Randerson, J. T., and Falkowski, P. (1998). Primary production of the biosphere: Integrating terrestrial and oceanic components. Science 281, 237–240. doi: 10.1126/science.281.5374.237
Filstrup, C. T., Hillebrand, H., Heathcote, A. J., Harpole, W. S., and Downing, J. A. (2014). Cyanobacteria dominance influences resource use efficiency and community turnover in phytoplankton and zooplankton communities. Ecol. Lett. 17, 464–474. doi: 10.1111/ele.12246
Giller, P. S., Hillebrand, H., Berninger, U. G., Gessner, M. O., Hawkins, S., Inchausti, P., et al. (2004). Biodiversity effects on ecosystem functioning: emerging issues and their experimental test in aquatic environments. Oikos 104, 423–436. doi: 10.1111/j.0030-1299.2004.13253.x
Gonzalez, A., and Loreau, M. (2009). The causes and consequences of compensatory dynamics in ecological communities. Annu. Rev. Ecol. Evol. Syst. 40, 393–414. doi: 10.1146/annurev.ecolsys.39.110707.173349
Grime, J. P. (1998). Benefits of plant diversity to ecosystems: immediate, filter and founder effects. J. Ecol. 86, 902–910. doi: 10.1046/j.1365-2745.1998.00306.x
Gross, N., Le Bagousse-Pinguet, Y., Liancourt, P., Berdugo, M., Gotelli, N. J., and Maestre, F. T. (2017). Functional trait diversity maximizes ecosystem multifunctionality. Nat. Ecol. Evol. 1:0132. doi: 10.1038/s41559-017-0132
Hillebrand, H., Langenheder, S., Lebret, K., Lindstrom, E., Oestman, O., and Striebel, M. (2018). Decomposing multiple dimensions of stability in global change experiments. Ecol. Lett. 21, 21–30. doi: 10.1111/ele.12867
Hu, H., and Wei, Y. (2006). The freshwater algae of china systematics, taxonomy and ecology. Beijing: Science Press.
Huang, Y. N., Li, Y. P., Ji, D. B., Nwankwegu, A. S., Lai, Q. Y., Yang, Z. J., et al. (2020). Study on nutrient limitation of phytoplankton growth in Xiangxi Bay of the Three Gorges Reservoir, China. Sci. Total Environ. 723:138062. doi: 10.1016/j.scitotenv.2020.138062
Ives, A. R., and Carpenter, S. R. (2007). Stability and diversity of ecosystems. Science 317, 58–62. doi: 10.1126/science.1133258
Jochimsen, M. C., Kummerlin, R., and Straile, D. (2013). Compensatory dynamics and the stability of phytoplankton biomass during four decades of eutrophication and oligotrophication. Ecol. Lett. 16, 81–89. doi: 10.1111/ele.12018
John, D., Whitton, B., and Brook, A. (2002). The freshwater algal flora of the british isles: an identification guide to freshwater and terrestrial algae. Cambridge: Cambridge University Press.
Karlowsky, S., Augusti, A., Ingrisch, J., Hasibeder, R., Lange, M., Lavorel, S., et al. (2018). Land use in mountain grasslands alters drought response and recovery of carbon allocation and plant-microbial interactions. J. Ecol. 106, 1230–1243. doi: 10.1111/1365-2745.12910
Kefi, S., Dominguez-Garcia, V., Donohue, I., Fontaine, C., Thebault, E., and Dakos, V. (2019). Advancing our understanding of ecological stability. Ecol. Lett. 22, 1349–1356. doi: 10.1111/ele.13340
Laliberté, E., Legendre, P., and Shipley, B. (2014). FD: measuring functional diversity from multiple traits, and other tools for functional ecology. R package version 1.0-12.1.
Laliberté, E., and Legendre, P. (2010). A distance-based framework for measuring functional diversity from multiple traits. Ecology 91, 299–305. doi: 10.1890/08-2244.1
Lavorel, S., Grigulis, K., McIntyre, S., Williams, N. S. G., Garden, D., Dorrough, J., et al. (2008). Assessing functional diversity in the field - methodology matters!. Funct. Ecol. 22, 134–147. doi: 10.1111/j.1365-2435.2007.01339.x
Lefcheck, J. S. (2016). PIECEWISESEM: Piecewise structural equation modeling in R for ecology, evolution, and systematics. Methods Ecol. Evol. 7, 573–579. doi: 10.1111/2041-210x.12512
Lehtinen, S., Tamminen, T., Ptacnik, R., and Andersen, T. (2017). Phytoplankton species richness, evenness, and production in relation to nutrient availability and imbalance. Limnol. Oceanogr. 62, 1393–1408. doi: 10.1002/lno.10506
Lê, S., Josse, J., and Husson, F. (2008). FactoMineR: An R package for multivariate analysis. J. Stat. Softw. 25, 1–18. doi: 10.18637/js.v025.i01
Leps, J., Majekova, M., Vitova, A., Dolezal, J., and de Bello, F. (2018). Stabilizing effects in temporal fluctuations: management, traits, and species richness in high-diversity communities. Ecology 99, 360–371. doi: 10.1002/ecy.2065
Li, H., Gu, Y., Cai, Q., Dong, X., and Ye, L. (2022). Zooplankton size structure in relation to environmental factors in the Xiangxi Bay of Three Gorges Reservoir, China. Front. Ecol. Evol. 10:800025. doi: 10.3389/fevo.2022.800025
Li, Z. Y., Zhang, H., Xu, Y. J., and Wang, S. P. (2021). Composition of ‘fast-slow’ traits drives avian community stability over North America. Funct. Ecol. 35, 2831–2840. doi: 10.1111/1365-2435.13909
Litchman, E., and Klausmeier, C. A. (2008). Trait-based community ecology of phytoplankton. Annu. Rev. Ecol. Evol. Syst. 39, 615–639. doi: 10.1146/annurev.ecolsys.39.110707.173549
Loreau, M., and de Mazancourt, C. (2013). Biodiversity and ecosystem stability: a synthesis of underlying mechanisms. Ecol. Lett. 16, 106–115. doi: 10.1111/ele.12073
Lurling, M., Mendes, E., Mello, M., van Oosterhout, F., Domis, L. D. S., and Marinho, M. M. (2018). Response of natural cyanobacteria and algae assemblages to a nutrient pulse and elevated temperature. Front. Microbiol. 9:1851. doi: 10.3389/fmicb.2018.01851
MacArthur, R. (1955). Fluctuations of animal populations and a measure of community stability. Ecology 36, 533–536. doi: 10.2307/1929601
MacGillivray, C. W., and Grime, J. P., The Integrated, and Screening Programme, T. (1995). Testing predictions of the resistance and resilience of vegetation subjected to extreme events. Funct. Ecol. 9, 640–649. doi: 10.2307/2390156
Mason, N. W. H., Mouillot, D., Lee, W. G., and Wilson, J. B. (2005). Functional richness, functional evenness and functional divergence: the primary components of functional diversity. Oikos 111, 112–118. doi: 10.1111/j.0030-1299.2005.13886.x
Mouillot, D., Graham, N. A. J., Villeger, S., Mason, N. W. H., and Bellwood, D. R. (2013). A functional approach reveals community responses to disturbances. Trends Ecol. Evol. 28, 167–177. doi: 10.1016/j.tree.2012.10.004
Oksanen, J., Blanchet, F. G., Friendly, M., Kindt, R., Legendre, P., McGlinn, D., et al. (2019). vegan: Community Ecology Package. R Package Version 2.5-6.
Oliver, T. H., Heard, M. S., Isaac, N. J. B., Roy, D. B., Procter, D., Eigenbrod, F., et al. (2015). Biodiversity and Resilience of Ecosystem Functions. Trends Ecol. Evol. 30, 673–684. doi: 10.1016/j.tree.2015.08.009
Pȩczuła, W., Szczurowska, A., and Poniewozik, M. (2014). Phytoplankton community in early stages of reservoir development - a case study from the newly formed, colored, and episodic lake of mining-subsidence genesis. Pol. J. Environ. Stud. 23, 585–591.
Pfisterer, A. B., and Schmid, B. (2002). Diversity-dependent production can decrease the stability of ecosystem functioning. Nature 416, 84–86. doi: 10.1038/416084a
Pflugmacher, S. (2002). Possible allelopathic effects of cyanotoxins, with reference to microcystin-LR, in aquatic ecosystems. Environ. Toxicol. 17, 407–413. doi: 10.1002/tox.10071
Polazzo, F., and Rico, A. (2021). Effects of multiple stressors on the dimensionality of ecological stability. Ecol. Lett. 24, 1594–1606. doi: 10.1111/ele.13770
Poos, M. S., Walker, S. C., and Jackson, D. A. (2009). Functional-diversity indices can be driven by methodological choices and species richness. Ecology 90, 341–347. doi: 10.1890/08-1638.1
Ptacnik, R., Moorthi, S. D., and Hillebrand, H. (2010). Hutchinson reversed, or why there need to be so many species. Adv. Ecol. Res. 43, 1–43. doi: 10.1016/S0065-2504(10)43001-9
Ptacnik, R., Solimini, A. G., Andersen, T., Tamminen, T., Brettum, P., Lepisto, L., et al. (2008). Diversity predicts stability and resource use efficiency in natural phytoplankton communities. Proc. Natl. Acad. Sci. U.S.A. 105, 5134–5138. doi: 10.1073/pnas.0708328105
Redfield, A. C. (1958). The biological control of chemical factors in the environment. Am. Sci. 46, 205–221.
Schnabel, F., Liu, X., Kunz, M., Barry, K. E., Bongers, F. J., Bruelheide, H., et al. (2021). Species richness stabilizes productivity via asynchrony and drought-tolerance diversity in a large-scale tree biodiversity experiment. Sci. Adv. 7:eabk1643. doi: 10.1126/sciadv.abk1643
Schnabel, F., Schwarz, J. A., Danescu, A., Fichtner, A., Nock, C. A., Bauhus, J., et al. (2019). Drivers of productivity and its temporal stability in a tropical tree diversity experiment. Glob. Chang. Biol. 25, 4257–4272. doi: 10.1111/gcb.14792
Tian, W., Zhang, H., Zhang, J., Zhao, L., Miao, M., and Huang, H. (2017a). Biodiversity effects on resource use efficiency and community turnover of plankton in Lake Nansihu, China. Environ. Sci. Pollut. Res. 24, 11279–11288. doi: 10.1007/s11356-017-8758-2
Tian, W., Zhang, H., Zhao, L., Xu, X., and Huang, H. (2016). Plankton community stability and its relationship with phytoplankton species richness in Lake Nansihu, China. Water 8:454. doi: 10.3390/w8100454
Tian, W., Zhang, H., Zhao, L., Zhang, F., and Huang, H. (2017b). Phytoplankton diversity effects on community biomass and stability along nutrient gradients in a eutrophic lake. Int. J. Environ. Res. Public Health 14:95. doi: 10.3390/ijerph14010095
Van Meerbeek, K., Jucker, T., and Svenning, J.-C. (2021). Unifying the concepts of stability and resilience in ecology. J. Ecol. 109, 3114–3132. doi: 10.1111/1365-2745.13651
Villeger, S., Mason, N. W. H., and Mouillot, D. (2008). New multidimensional functional diversity indices for a multifaceted framework in functional ecology. Ecology 89, 2290–2301. doi: 10.1890/07-1206.1
Wang, L., Cai, Q. H., Xu, Y. Y., Kong, L. H., Tan, L., and Zhang, M. (2011a). Weekly dynamics of phytoplankton functional groups under high water level fluctuations in a subtropical reservoir-bay. Aquat. Ecol. 45, 197–212. doi: 10.1007/s10452-010-9346-4
Wang, L., Cai, Q., Zhang, M., Tan, L., and Kong, L. (2011b). Longitudinal patterns of phytoplankton distribution in a tributary bay under reservoir operation. Quat. Int. 244, 280–288. doi: 10.1016/j.quaint.2010.09.012
Weithoff, G. (2003). The concepts of ‘plant functional types’ and ‘functional diversity’ in lake phytoplankton - a new understanding of phytoplankton ecology? Freshw. Biol. 48, 1669–1675. doi: 10.1046/j.1365-2427.2003.01116.x
Wentzky, V. C., Tittel, J., Jager, C. G., Bruggeman, J., and Rinke, K. (2020). Seasonal succession of functional traits in phytoplankton communities and their interaction with trophic state. J. Ecol. 108, 1649–1663. doi: 10.1111/1365-2745.13395
Xu, Q., Yang, X., Yan, Y., Wang, S., Loreau, M., and Jiang, L. (2021). Consistently positive effect of species diversity on ecosystem, but not population, temporal stability. Ecol. Lett. 24, 2256–2266. doi: 10.1111/ele.13777
Yachi, S., and Loreau, M. (1999). Biodiversity and ecosystem productivity in a fluctuating environment: The insurance hypothesis. Proc. Natl. Acad. Sci. U.S.A. 96, 1463–1468. doi: 10.1073/pnas.96.4.1463
Ye, L., Cai, Q., Zhang, M., and Tan, L. (2014). Real-time observation, early warning and forecasting phytoplankton blooms by integrating in situ automated online sondes and hybrid evolutionary algorithms. Ecol. Inf. 22, 44–51. doi: 10.1016/j.ecoinf.2014.04.001
Ye, L., Cai, Q., Zhang, M., Tan, L., and Shen, H. (2016). Ecosystem metabolism and the driving factors in Xiangxi Bay of Three Gorges Reservoir. China Freshw. Sci. 35, 826–833. doi: 10.1086/687205
Ye, L., Chang, C. W., Matsuzaki, S. I. S., Takamura, N., Widdicombe, C. E., Hsieh, C. H., et al. (2019). Functional diversity promotes phytoplankton resource use efficiency. J. Ecol. 107, 2353–2363. doi: 10.1111/1365-2745.13192
Ye, L., Chang, C.-Y., Garcia-Comas, C., Gong, G.-C., and Hsieh, C.-H. (2013). Increasing zooplankton size diversity enhances the strength of top-down control on phytoplankton through diet niche partitioning. J. Anim. Ecol. 82, 1052–1060. doi: 10.1111/1365-2656.12067
Ye, L., Han, X. Q., Xu, Y. Y., and Cai, Q. H. (2007). Spatial analysis for spring bloom and nutrient limitation in Xiangxi bay of three Gorges Reservoir. Environ. Monit. Assess. 127, 135–145. doi: 10.1007/s10661-006-9267-9
Ye, L., Tan, L., Wu, X., Cai, Q., and Li, B. L. (2022). Nonlinear causal analysis reveals an effective water level regulation approach for phytoplankton blooms controlling in reservoirs. Sci. Total Environ. 806:150948. doi: 10.1016/j.scitotenv.2021.150948
Keywords: biodiversity, community-weighted means of traits, functional diversity, ecosystem function, community stability, Three Gorges Reservoir
Citation: Zhang X, Tan L, Cai Q and Ye L (2022) Environmental factors indirectly reduce phytoplankton community stability via functional diversity. Front. Ecol. Evol. 10:990835. doi: 10.3389/fevo.2022.990835
Received: 10 July 2022; Accepted: 20 September 2022;
Published: 11 October 2022.
Edited by:
Min Zhang, China Institute of Water Resources and Hydropower Research, ChinaReviewed by:
Zhaofeng Guo, Key Laboratory of Urban Environment and Health, Institute of Urban Environment (CAS), ChinaCopyright © 2022 Zhang, Tan, Cai and Ye. This is an open-access article distributed under the terms of the Creative Commons Attribution License (CC BY). The use, distribution or reproduction in other forums is permitted, provided the original author(s) and the copyright owner(s) are credited and that the original publication in this journal is cited, in accordance with accepted academic practice. No use, distribution or reproduction is permitted which does not comply with these terms.
*Correspondence: Lin Ye, eWVsaW5AaWhiLmFjLmNu
Disclaimer: All claims expressed in this article are solely those of the authors and do not necessarily represent those of their affiliated organizations, or those of the publisher, the editors and the reviewers. Any product that may be evaluated in this article or claim that may be made by its manufacturer is not guaranteed or endorsed by the publisher.
Research integrity at Frontiers
Learn more about the work of our research integrity team to safeguard the quality of each article we publish.