- CSIRO, Ecosciences Precinct, Brisbane, QLD, Australia
Spatially explicit population dynamic models have been successfully used to explore management scenarios in terms of pest suppression across a wide range of systems. However, the economic implications of pest management, particularly in the case of biological control and non-crop management strategies, have not been well considered. A bioeconomic spatially explicit simulation model was developed, that integrates models of pest population dynamics, pest movement and economics of management. The utility of the model is demonstrated here using Nysius vinitor, a pest of grain crops in Australia. The model estimates the short- and long-term economic benefits of three pest management strategies: (1) in-field pesticide spray; (2) pest suppression through weed management in non-crop habitat; and (3) bolstering biocontrol through revegetation with, or maintenance of, native vegetation. Across all management types, high yield and low relative management cost resulted in a greater chance of a gross profit. The impacts of the pests themselves were shown to be non-linear, with an intermediate level of pest pressure maximizing the economic gain from management. Pest dispersal capacity influenced the profitability of management of non-crop vegetation, with lower pest dispersal resulting in a greater likelihood of benefit, as benefits from non-crop management are localized (e.g., increased beneficial insect populations). In an intensively cropped landscape, pesticide management was most profitable over the short-term. Once a 10-year horizon was reached, then the profitability of revegetation was greater and continued to increase. While weeding requirements are low, it is likely to always be profitable in the long-term to maintain or restore native vegetation in good condition to control this pest in an intensively cropped landscape. Using pesticide alongside revegetation gave some short-term gain, but the negative impact of pesticide on beneficials outweighed the benefit and in the long-term it is less profitable. These results do not hold in a low production landscape, due to increased pest pressure and costs of managing non-crop habitat. In summary, when quantified over a 10–20 year time horizon, revegetation or conserving native remnants in good (i.e., non-weedy) condition could be economically more beneficial to control an insect pest than ongoing pesticide use, in intensively cropped landscapes.
Introduction
Conservation biocontrol is a “soft” approach to insect pest control, that aims to enhance abundance or activity of the existing natural enemies in the landscape through local farm management practices (Chaplin-Kramer et al., 2019). In broadacre agriculture there remains a reluctance of growers to shift to such “soft options” for pest management or to practice more integrated approaches; in general this is because growers are highly risk adverse and have become reliant on pesticides, with an average of 6% of total on-farm costs Australia-wide are attributed to pesticide use (Adamson et al., 2014). In Australia, insecticides are cheap and readily available, however, they are facing increasing regulation and issues of resistance which is providing some motivation for industry to consider how best to integrate conservation biocontrol into practice, and to support more judicious use of pesticides (Hunt et al., 2021). Economic studies conducted in Europe to consider the impacts of national policies for reduced pesticide use have mostly not considered possible changes in production techniques (such as conservation biological control) to compensate for a reduction in pesticide; this has led to potentially erroneous conclusions that regulation of pesticide use will have dramatic negative consequences for farm economies and supply of products (Jacquet et al., 2011). For growers and landholders to invest in the development of alternate practices like conservation biological control they require a clearer picture of the economic costs and benefits over longer time frames.
Multiple ecological studies in the past have shown that non-crop habitat may suppress pests and boost beneficial insects in crops if well managed (see reviews by Bianchi et al., 2006; Chaplin-Kramer et al., 2011; Veres et al., 2013). Beneficials are “bolstered” by the continuous resources available (Schellhorn et al., 2015a) and many will move into the crop to provide ecosystem services (Macfadyen et al., 2015). Simulation models for multiple species have also been developed to show the benefits of biological control in terms of pest suppression under varying climatic or landscape conditions (e.g., Li et al., 2019; Barton et al., 2021). It has been recognized for some time that such models could form the basis of valuable decision tools for pest management incorporating biological control (Waage and Barlow, 1993). However, scientific studies rarely go so far as to estimate the economic implications (e.g., prevention of yield loss) of conservation biological control, instead stopping short with a focus on the process of pest suppression rather than profit outcomes (Kleijn et al., 2019). In contrast, several economic studies have sought to quantify economic thresholds for control (e.g., Wise and Lamb, 1998), where economic threshold is defined as “the density at which control measures should be determined to prevent an increasing pest population from reaching…the lowest population density that will cause economic damage” (Stern et al., 1959). These studies relate almost exclusively to insecticide application, and do not incorporate functions for biological control (Naranjo et al., 2015). Economic threshold estimates for insecticide spray exist for a number of pest species and crops (e.g., Miles, 1997), and there is significant research into IPM that is applied for some crops like cotton in Australia that incorporates a detailed consideration of beneficials (Wilson et al., 2018). However, for many broadacre grains crops in Australia, these thresholds are usually a “static” single value and largely based on expert opinion, which is also likely to be biased in favor of chemical applications given the risk-adverse nature of pest control. Such thresholds take a narrow view of management options available for pest suppression to growers, and do not consider how such thresholds will be influenced by factors such as the surrounding landscape, potential crop yield and the efficacy of the management itself, nor explore options for conservation biological control as part of integrated pest management (IPM). They also do not assess management costs and benefits over the long term.
At present, on-farm pest management decision-making in Australia rarely considers the wider landscape effect on pest and beneficial populations, and how non-crop habitats might be managed to give greater economic returns. This is perhaps due to the limited amount of research conducted, and thus limited evidence of, the effect of non-crop habitats on pest control within Australian crop fields, changes in insecticide use, crop damage and yield (Gagic et al., 2018). It is also a complex question, with many factors that can influence the economic outcome (Naranjo et al., 2015). As an “ecosystem service,” Boerema et al. (2017) found only six measures of economic value for biological control in an international literature review. In addition, the ecological benefit of the service is often quantified separately to the socio-economic benefit (Boerema et al., 2017). For example, a review of the benefits of flower strips for farmers found that only around 6% of studies explicitly considered benefits to the farmer, only one study was carried out over a time horizon beyond 4 years, and many papers focused on ecological process, not profit outcomes (Uyttenbroeck et al., 2016). For decision support to be more practical and encompassing of biocontrol, it should integrate ecological and economic information in relation to economic threshold decisions (Chaplin-Kramer et al., 2019). To date, a means to move beyond nominal, rule-of-thumb approaches to determine more dynamic thresholds (or “multi-dimensional thresholds,” see Waage and Barlow, 1993) has not been presented. There is also a lack of understanding of what key ecological and economic data are required in order to calculate dynamic thresholds, and how best to efficiently obtain this data, but see Macfadyen et al. (2014) for an attempt to do so in Australia. The ecosystem services accounting literature is taking some steps to address this, including some of the complexities around accounting for stocks of natural capital and the flow of the ecosystem service (Vira and Adams, 2009). Overall, in order to take a softer management approach (such as so-called “ecological intensification” or to implement so-called “dynamic economic thresholds”) “more knowledge is needed, particularly on the quantification of the costs and benefits … using variables that are relevant to farmers (e.g., crop yield [loss] and profits at the farm level), and the effectiveness of different ecological intensification practices, alone and in combination with other practices, over longer periods of time” (Kleijn et al., 2019, pp. 10). Reconciling temporal and spatial scales is complex, but quantifying the interplay between in-field decision-making and the landscape context is critical (e.g., Furlong et al., 2018).
A means to achieve this quantification is through a bioeconomic simulation modeling approach, based on knowledge gained empirically from multiple ecological and economic studies. As pest management decisions are primarily made at the farm level, it is necessary that the model focuses on private costs and benefits (Waterfield and Zilberman, 2012). Bioeconomic model have their origins, and have been mostly applied, in fisheries research where this term refers to particular models and approaches (e.g., Seijo et al., 1998), but for a recent relevant example where a spatially explicit bioeconomic model was developed for weed management in cereals see González-Díaz et al. (2015). There are limited examples of such an approach applied to insect pest management besides the “natural enemy-adjusted economic threshold” study of Zhang and Swinton (2009). Although their model is not spatially explicit and does not incorporate management practices other than pesticide, it does address some of the important economic trade-offs between pest density, pesticide spray, natural enemy densities, yield and returns. They further developed this model into a tool that assists with the assessment of pesticide application thresholds depending on the number of natural enemies per plant, as well as derived a value for ecosystem services of soybean aphid biocontrol for some US States (Zhang and Swinton, 2012). Other studies have quantified a marginal value of natural enemies at the farm scale, for example in cotton in China (Huang et al., 2018).
Overall, the gap in the scientific literature and lack of tools available to determine the economic value of natural enemies has contributed to little up-take by the agricultural sector of ecologically intensive farming practices (Huang et al., 2018; Kleijn et al., 2019). As Zhang and Swinton (2012) concluded, “future research should move beyond pesticide use thresholds to develop landscape-scale guidelines for explicit management of habitat for the natural enemies of agricultural pests” (pp. 15). Here, a bioeconomic simulation model was developed to explore the complex system of insect pest and beneficial dynamics in relation to non-crop vegetation management across a landscape and in-crop insecticide application at the farm landscape scale. The model was then applied to estimate under what conditions growers may expect a profitable return from managing non-crop habitat either by weed management or with revegetation, compared to conventional use of insecticides.
Materials and methods
Purpose
The purpose of the model is to estimate, on a per field and landscape-level basis, the economic return expected from three different pest management action scenarios over time. This includes both pesticide applications and non-crop vegetation management, across multiple insect species. The estimation of the economic return considers many aspects of the complex agro-ecological system of broadacre grain growing in Australia.
At present, the model focuses on the management of a single pest, Rutherglen bug (Nysius vinitor). Rutherglen bug is a pest of increasing importance in a range of grain and horticulture crops, as well as cotton. Here, we focus on the management of the pest in canola. It can be highly mobile and is known to be supported by multiple hosts in the Australian landscape, so habitat management is important (see Parry et al., 2019). Many other pests may also be impacted by similar non-crop management practices in Australia (see Parry et al., 2015; Downes et al., 2017), so although the focus here is on a single species the findings have broader relevance.
The model is object-oriented and coded in Java. The code makes use of the Repast Simphony 2.4 toolkit for agent-based modeling for its spatial visualization, input/output handling and batch run capabilities, and treats agricultural fields as “agents” (objects). Thus the model is described below following the “ODD” (Overview, Design concepts, and Details) protocol for describing agent-based models (Grimm et al., 2006, 2010).
Entities, state variables and scales
The model has several drivers that ultimately influence the estimated economic return from a given pest management scenario (Figure 1). In terms of management scenarios, the economic benefits (gross profit) can be realized in three ways: (i) pesticide spray reduces pest populations, reducing crop yield loss; (ii) weeding of non-crop habitat reduces pest populations, also reducing crop yield loss; and (iii) revegetation of remnants with native plant species increases beneficials that reduce pest populations, reducing crop yield loss. The model is also able to account for negative feedbacks attributed to pesticide use with negative effects on beneficials. There are several agronomic parameters including the value of the crop and yield, and ecological parameters relating to pest population pressure and impact.
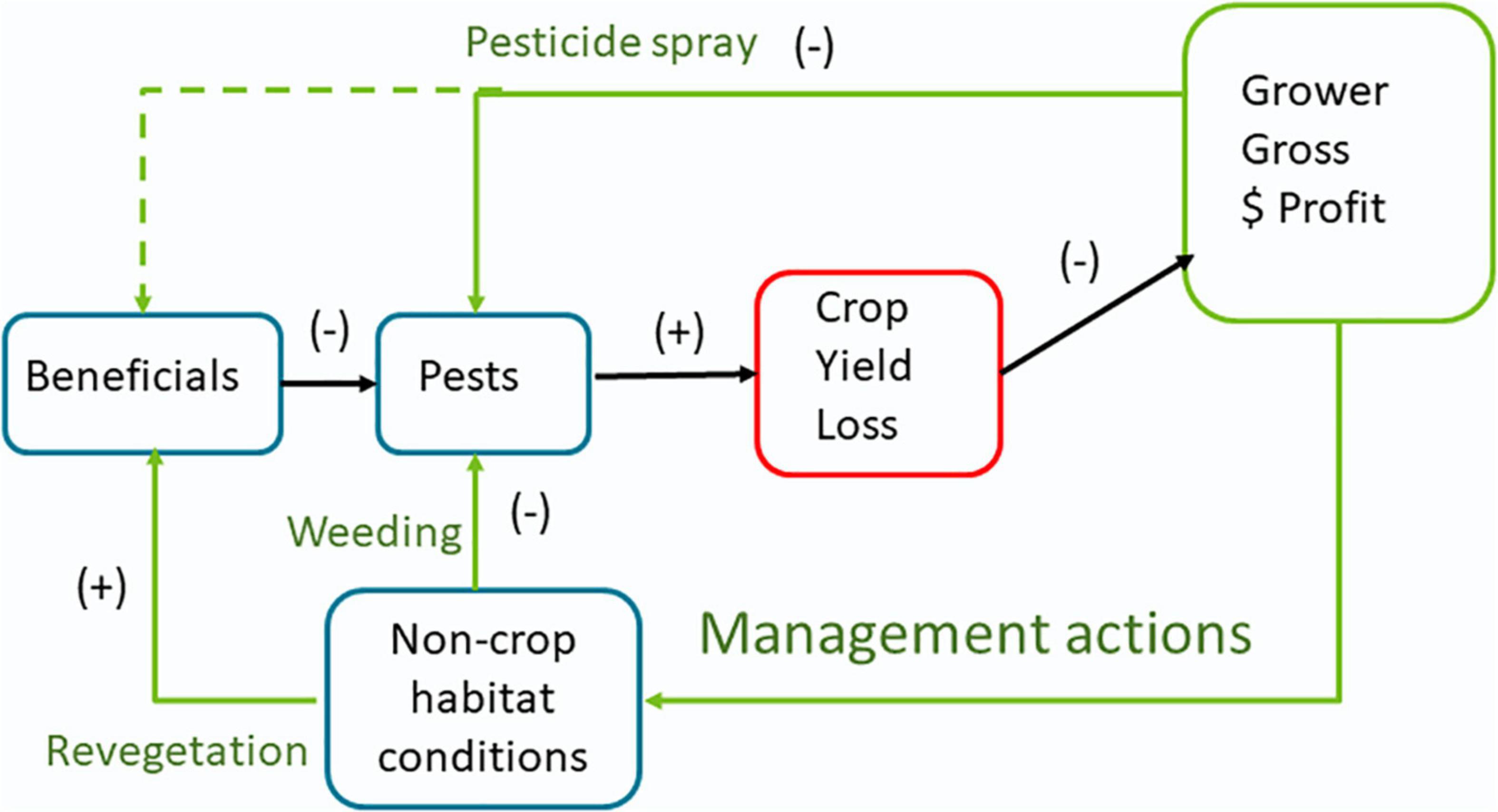
Figure 1. Bioeconomic framework indicating the key factors included in the model and how they relate to one another: (–) negative impact (+) positive impact.
The model is spatially explicit, and simulates management across a landscape, with the key entities the habitat patches in that landscape: crop (divided into crop types) and non-crop (pasture and remnant vegetation). Each crop patch may contain a pest population and the dynamics of this population are modeled at the patch scale, along with the impacts of management on that population. The model runs on a monthly basis, reflecting the data availability for crops and pest populations in the non-crop habitat in the study region. The model simulates the agronomic management of the grain growing study region and can be run across multiple years (necessary in order to estimate effects of long-term management benefits of non-crop habitat). The model currently runs on 12 months of digitized landscape data for NSW, Australia [−34.714, 147.726 (lat, lon) Supplementary Figure 1], but could easily be applied in other similar locations at different scales, if spatial data are available. An intensively cropped landscape (Supplementary Figure 1) is compared with that of a landscape containing a high proportion of semi-natural vegetation in the same region [−34.383, 148.434 (lat, lon)], that contains a much higher proportion of native vegetation remnant and pasture (see Supplementary Figure 2). Multiple years are simulated in the model in this study by simply repeating the spatial data for each month. It is assumed that the landscape structure in terms of crop vs. non-crop remains constant, i.e., it is assumed that no productive land is revegetated, or that there is no opportunity available to convert native remnants into production land. It is assumed that the other grain crops in the landscape (Supplementary Figure 1) are such poor hosts that they can be modeled as “non-hosts” (see Parry et al., 2019 for data to support). The landscape is modeled as a “closed” landscape, i.e., with reflective boundaries, as opposed to a torus: this is due to the irregular shape. This has some minor implications for population densities at the edge (in general somewhat lower due to reduced connectivity to other patches). Immigration from further afield is not included in this model, although this can be an important but irregular driver of pest pressure for this pest (McDonald and Farrow, 1988).
Management
Three types of management with pest-suppression benefits in the model were implemented:
1. Pesticide spray application (varies by month applied and number of sprays)
2. Weeding of non-crop habitat (remnant vegetation or pasture)
3. Revegetation of remnant habitat with native vegetation – incorporates weeding effect and cost of weeding in year 1.
The model was also used to explore the combined effects of management scenarios, and the economic benefits gained by preserving existing native vegetation in good (i.e., non-weedy) condition. When combining management, a function is added to the model to account for the negative feedback of pesticide application on top of revegetation, as this is likely to negate the predation function that is a benefit of native vegetation.
The way in which management actions are implemented in space and time is central to the functionality of the model. There are several assumptions that underlie the management models. For the management of non-crop habitat:
1. Management takes place equally across non-crop habitat in the whole landscape.
2. The cost of the non-crop vegetation management is shared equally amongst all crop fields that may benefit (i.e., crop in which the pest is known to be an issue) in the landscape.
For the management of the crop (pesticide spray):
1. Management takes place equally across crops of the same type in the whole landscape, regardless of pest density.
2. The cost per field is a function of the extent of the crop field multiplied by the spray cost per ha (including labor costs).
Process overview and scheduling
Each management scenario requires a different set of processes and scheduling in a monthly timestep (Figure 2). In all cases, pest and beneficial populations are simulated in the non-crop and then transfer to the crop (see sub-models below), based on findings from previous (field) studies (Macfadyen et al., 2015; Parry et al., 2015). In all simulations the “null” model is run: pest impact on the crop is simulated and then the yield under no management is recorded in order to calculate management benefits (below). Management scenarios are parameterized (as below) and run independently. Pesticide management is applied equally to the crop fields at a user-defined rate and date, and the pest population reduced according to the key parameters of number of sprays, timing of spray and effectiveness of the spray (below).
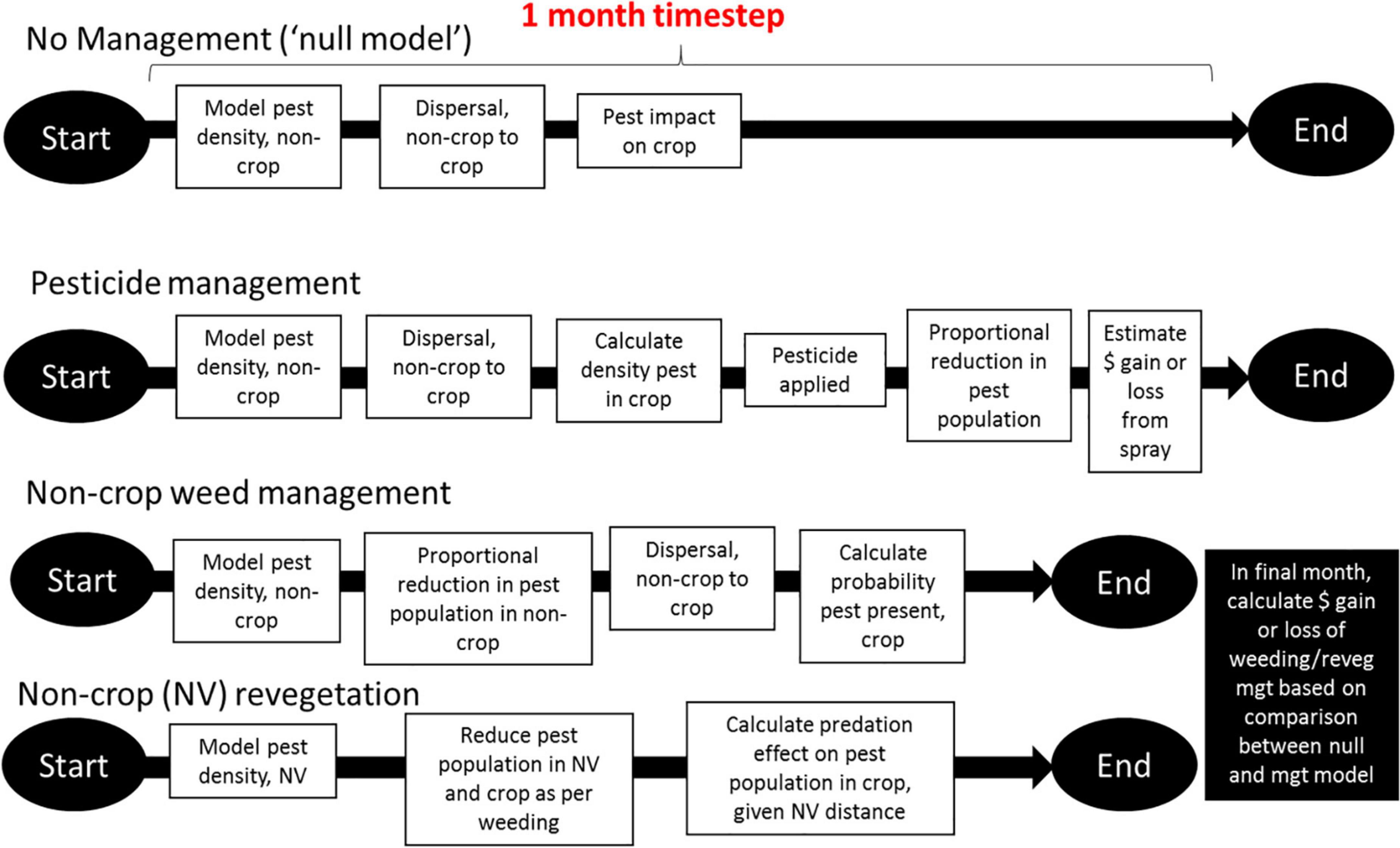
Figure 2. Flow diagram showing, for each monthly timestep during the year, how non-crop weed management simulation processes and scheduling differ from pesticide management and no management. Revegetation management [remnant vegetation (NV) only] effects are calculated in a similar manner to non-crop weed management.
Non-crop weed management is applied in the non-crop habitat, both remnant vegetation and pasture [with the costs transferred to the area of crop that benefits, in this case, canola; note that the cost of weed management given in Table 1 is per month; it is assumed that this applies over 6 months of the year to give the annual total cost (Butts and Lamb, 1991)]. The population of pests in the non-crop habitat is reduced by 90% (based on findings in Parry et al., 2015, 2019) to represent the effect of weeding. The simulation then proceeds as per the null model, but the immigration into crops is reduced and thus so is the impact of the pest on yield.
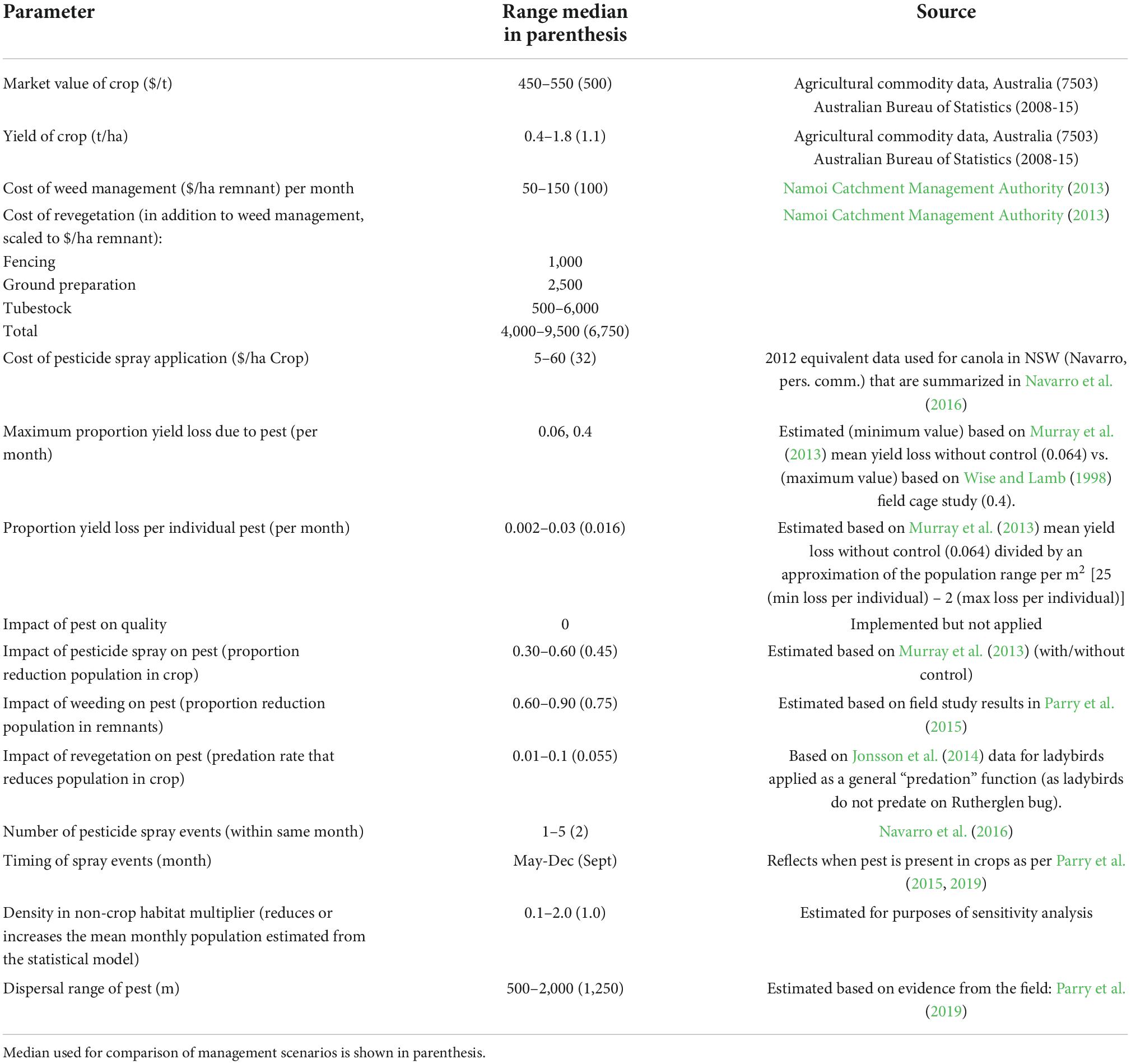
Table 1. Parameter input value ranges (user input) for model sensitivity analysis for N. vinitor and canola based on data/literature as indicated at 2012–2013 prices.
The revegetation of non-crop remnant habitat incorporates the effects of weeding, but has an additional function that represents an immediate increase in beneficial insects (predators) that then move into the crop. This “predation effect” (natural regulation of pests by predators) is based on similar principles to Jonsson et al. (2014) and is set as a fixed factor that reduces crop pest populations (the predation rate) on a monthly basis (see Table 1), but is applied only within a distance of 1,500 m of remnant vegetation. Thus, for each crop field, this function is only applied if the proximity of remnant vegetation to the crop field is within this range under the revegetation scenario. This distance is fairly arbitrary, with disagreement in the literature on the scale of response to non-crop structures of predators and parasitoids in canola (Zaller et al., 2009).
When modeling pesticide application in addition to revegetation, negative feedback between pesticide spray and the benefit of revegetation is taken into account by reducing the “predation effect” by a factor equivalent to the impact of pesticide spray on the pest, see Table 1 (the reduction in pest populations in remnant vegetation due to “weeding,” or the absence of weeds, is still effective).
The model can be run via a graphical user interface, Supplementary Figure 3, or in batch-mode (for exploring a parameter space, as presented in the sensitivity analysis).
Initialization
The model is initialized with a monthly spatial dataset of cropping for the region of interest and the pest population (in this case Rutherglen bug, Nysius vinitor) is calculated for the remnant vegetation and pasture from the statistical population dynamic model (see sub-models). The spatial data for each field/habitat patch is stored as an object in memory, including the land use for each month and the area of the field as well as the initial pest population.
Input data
In addition to the monthly spatial data, input as an ArcGIS shapefile, the model has multiple parameter inputs that apply to the simulation, depending on the scenario (Table 1). A screenshot of the model Graphical User Interface (GUI) is shown in Supplementary Figure 3.
Output data
The output of the model, like the input, is spatially explicit. Output occurs at the end of the year (mid-summer). The efficacy of management may vary in space, due to the limitations of dispersal of both the pest and the predation function in relation to the non-crop habitat. An example of the output to the Graphical User Interface (GUI) that monitors whether there is an economic gain or loss for a particular year is shown in Figure 3.
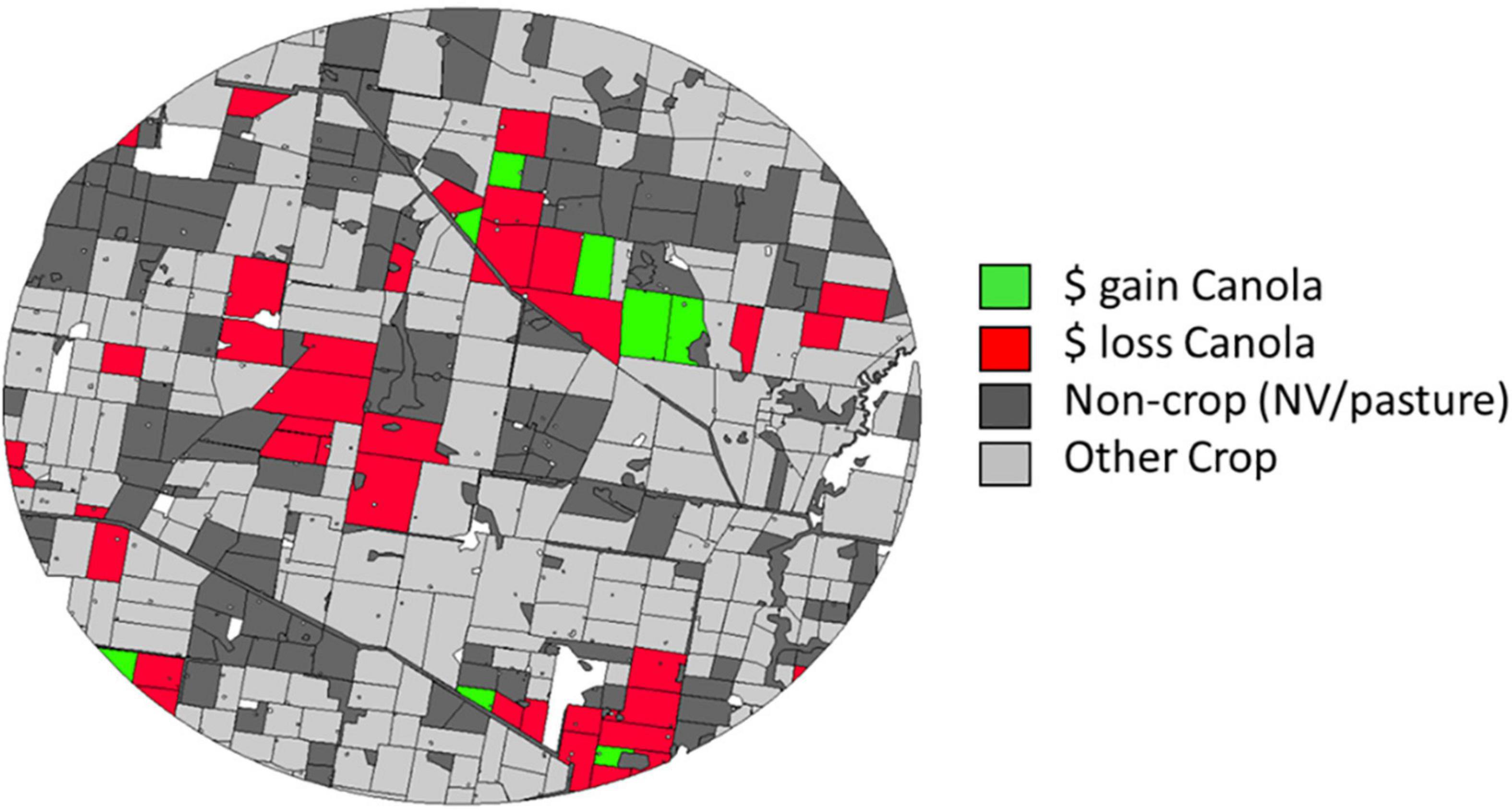
Figure 3. Example Graphical User Interface (GUI) output illustrating the spatial variation in $ gain or loss per canola crop field.
The model outputs to file at the end of each year (i.e., after 12 time steps) for each crop field, capturing the parameterization for the scenario modeled, the crop pest population, annual yield, profit (benefit), cost and revenue over time.
Submodels
Statistical model of the density of pests in non-crop habitat
A statistical model of the population dynamic of N. vinitor was fit to the data collected in NSW in 2010 for both pasture (Eq. 1) and grasses/weeds (Eq. 2). This model was fit using GLM in R using a quasi-poisson distribution with a sinusoidal function (see Parry et al., 2019 for details). The equations were then applied in the model to deterministically represent the non-crop population dynamics, and the sensitivity of this dynamic explored by multiplying by a factor between 0.1 and 2.0.
Where Np = the density of N. vinitor adults per m2 pasture; Nw = the density of N. vinitor adults per m2 weeds/grasses in remnant vegetation; m = month converted to radians: .
Although a single pest case study is presented here for simplicity, other key pests (Armyworm and European Earwig populations) in non-crop habitat can be modeled statistically from the available data in future, and these pests could be simulated by the model either individually or in combination with Rutherglen bug.
Connectivity of patches and movement of the pest from the native vegetation or pasture habitat into the crop
A simple measure of connectivity is the distance of a patch to the nearest neighbor. However, this doesn’t account for the number of neighbors or patch size. An alternate metric is that which accounts for the size and proximity of neighboring patches within a local neighborhood: Whitcomb et al. (1981) “isolation index” and Gustafson and Parker (1992) “proximity index.”
In this model, patches are assumed to connect to one another if they are within the (user-specified) maximum local dispersal range of the pest (Table 1).
The probability of leaving a non-crop patch, Pleave, is fixed at 0.5. In reality this would vary depending on habitat and environmental conditions, and this could be refined if functions derived from available data were incorporated into the model (Moradi-Vajargah and Parry, 2017).
A transfer probability (the likelihood of moving from a focal patch to another patch) for each non-crop patch is calculated, that is inversely proportional to the number of “connected patches” (n) within the movement range of a focal patch, with a maximum of the leaving probability (above) when there is connection to only one other patch (Eq. 3).
A connectivity probability for each non-crop patch is calculated as the sum of all transfer probabilities for the connected non-crop patches (i). If the result is > 1 this becomes 1 (Eq. 4).
An isolation weighting is then applied to the focal non-crop patch, calculated by multiplying the connectivity probability with the mean density from the statistical population model (as above). The result is that more isolated and smaller patches will have a lower density (Supplementary Figure 4).
To calculate movement of the pest from non-crop habitat into crop habitat (Supplementary Figure 5), and subsequent movement from crop patch to crop patch (or back to non-crop), the above metrics are applied to each patch in the landscape. The transfer probability is used to directly estimate the transfer of pests between habitat patches. The transfer probability is multiplied by the focal patch population to calculate the population to transfer to the connected patch(es) from the focal patch. When more than one patch is connected to a focal patch, the transferring population is divided between the connected patches.
Benefits and costs of management actions
In the following some standard economic assumptions are made. Firstly, that canola prices are a constant as given or determined by the market (the sensitivity of pricing is explored). Second, that pest impacts are small and don’t affect the supply curve of the industry (i.e., feedbacks from pest impacts are not taken into account). The farmer is assumed to be a profit-maximizer and revenue should be positive. So when costs associated with pest injury (as calculated by the model) exceed benefit this is no longer optimal and results in economic loss.
Table 2 summarizes the key attributes of each management actions in terms of their spatio-temporal scale, both at which they are implemented and at which their cost per ha crop is calculated.
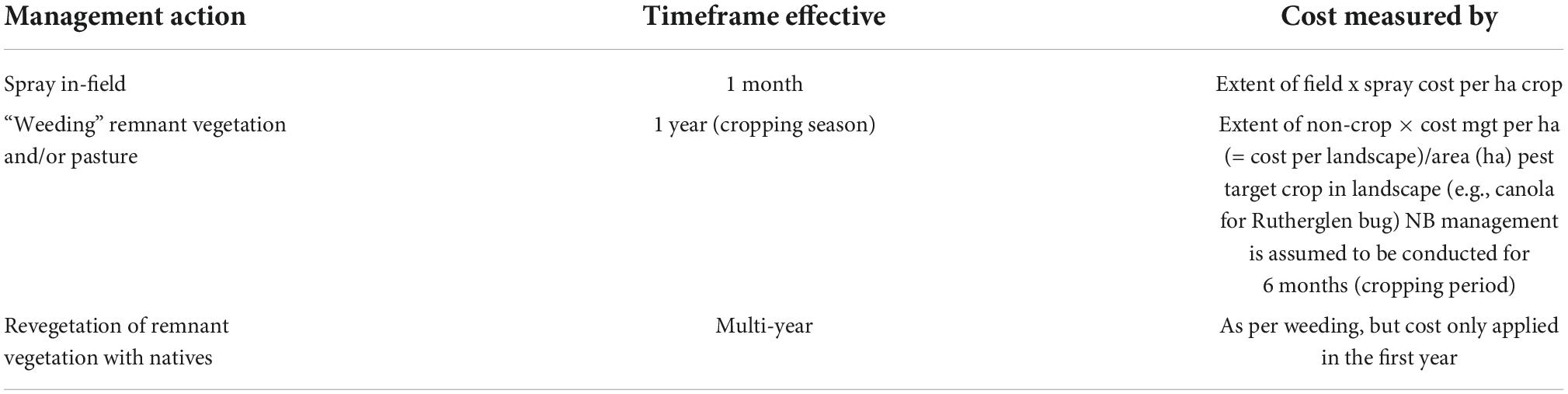
Table 2. Spatio-temporal scales at which costs and benefits of management actions are measured in the model.
The benefit ($/ha crop) of a management action (i.e., the marginal revenue gained by the action) is evaluated as follows (Eq. 5):
Where Y = yield; P = price per unit of yield; s = level of pest injury; a = control action; [s(a)] = level of injury as modified by the control action (Southwood and Norton, 1973; cited by Pedigo et al., 1986).
In order to evaluate future benefits and costs in today’s terms, a simple assumption of nominal prices and costs of management P (price per unit of yield) and C(a) (cost of management action) over the modeled 20-year time horizon is applied. In order to convert future benefit inflows into present values, discounting is applied to the future benefit inflows across all future years i (where current year = 0) to a maximum projected time horizon T, so that the benefit for year i is calculated as follows (Eq. 6):
Where r is the discount rate. Results are presented showing the variation due to the discount rate applied: a minimum of 2%, mean of 4% and maximum of 7%, which approximate the interest rate in Australia (nominal interest rate minus inflation). This produces BPV, the present value ($/ha crop) a management action in year i up to time T will generate. The future cost outflows CPV(a) are converted into present values using the same discounting method.
Gross profit G(a) ($/ha crop) of the management action is calculated (Eq. 7):
Cost C(a) $/ha crop (or crop equivalent if non-crop management – see Table 2) of a management action is within an estimated range as given in Table 1.
Yield Y[s(a)], dependent on the level of injury as modified by the control action is calculated (Eq. 8):
Where Y = potential yield, %loss = Proportion of yield loss due to pests (see below), %control = the proportion of reduction in pest injury due to the control action.
Yield without control, Ys, is simply the potential yield minus the yield accounting for loss due to total pests with no control (Eq. 9):
Price per unit of yield, P[s(a)], dependent on the level of injury as modified by the control action, is calculated (Eq. 10):
Where MV is the market value of the crop.
Price without control, Ps, is simply the potential market value minus the market value accounting for quality loss due to total pests with no control (Eq. 11):
The above is quite straightforward to evaluate for pesticide control, as the management action occurs within the same spatial context as the effect in the crop and assumed to remain in effect for just a single timestep of the model (1 month). Effectiveness of the spray varies, as per Table 1 (impact of pesticide spray on the pest). However, for non-crop vegetation management, there is a spatial off-set between the spatial context of the management action (i.e., non-crop habitat) and the spatial context of the effect (i.e., crop fields), and the effect of non-crop management is realized over multiple months. This is addressed using a simulation approach, where a null model is run alongside the non-crop management scenario model, and the area under the curve (AUC) for crop pest population is recorded for the year in both cases. The proportion of pest control in the crop (NV% control) due to the non-crop management is calculated (Eq. 12):
The benefit flow due to the non-crop management, B(a), is then calculated as above per ha crop, per annum. The differences between the calculations and the simulation approach is shown graphically in Figure 2.
The expected yield loss proportion (%loss) due to pest density is calculated as a function based on Zhang and Swinton (2009), which in turn was based on Cousens (1985) model for yield loss as a function of weed density (Eq. 13):
The proportion of yield loss is a function of:
1. The pest population density in that month (St)
2. The proportion of yield lost per unit of pest density in month t (μt)
3. The maximum proportion yield loss to pest damage (0 ≤ θ ≤ 1) in month t (θt)
The above is calculated for both the scenario under management and the null model on a monthly basis. The proportion loss for the year, %lossy, is calculated (Eq. 14):
Where n ∈ [1, 12].
Early tests with the parameters in this equation show it to be highly sensitive to both the maximum impact per individual pest (μt) and the maximum proportion of yield loss due to pest damage (θt). Due to the high level of uncertainty and sensitivity in these key parameters (Supplementary Figure 6), the model sensitivity was tested by fixing μ and varying θ, and two scenarios of maximum yield loss were tested: θ = 0.06 and θ = 0.4. This range is supported by a study of Lygus bugs on canola in Canada (Butts and Lamb, 1991), which found 6% of canola seed in some regions was destroyed by Lygus bugs, on average, and that in some fields > 20% of the seed was destroyed. When Lygus bugs were controlled with insecticide at the early pod stage, yield increased by 11–35% in five tests (Butts and Lamb, 1991).
This equation appears to represent well the function of the impact of the pest population on yield when modeled with the higher parameter values of θ = 0.4, μ = 0.03, when compared to an empirical (field cage) study of a Lygus spp. in canola in Canada (Wise and Lamb, 1998), and so the value of θ = 0.4 is used throughout the study. The sensitivity analysis (Supplementary Figures 7A–C) indicates that if θ is reduced to a much lower value (0.06) then control is nearly always uneconomic; thus the economic threshold for control is likely to be much higher than a 6% pest impact on yield, but it will also depend on other factors.
Results
Key factors driving gross profit for each management scenario
The mean pest population dynamic in the crop vs. the non-crop habitat across the year for the different pest control scenarios (in comparison with the null model of no control) is shown in Supplementary Figures 8A–D. The sensitivity analysis (Supplementary Figures 7A–C) and analysis of the mean parameters that resulted in profitable outcomes after 4 years (Table 3) indicated the key factors driving profitability for each management type. Across all management types, crop yield and the cost of management were drivers: high yield (> ∼1.2 t/ha) and low relative cost of management would result in a greater chance of a gross profit (it is assumed there is not a correlation between variables).
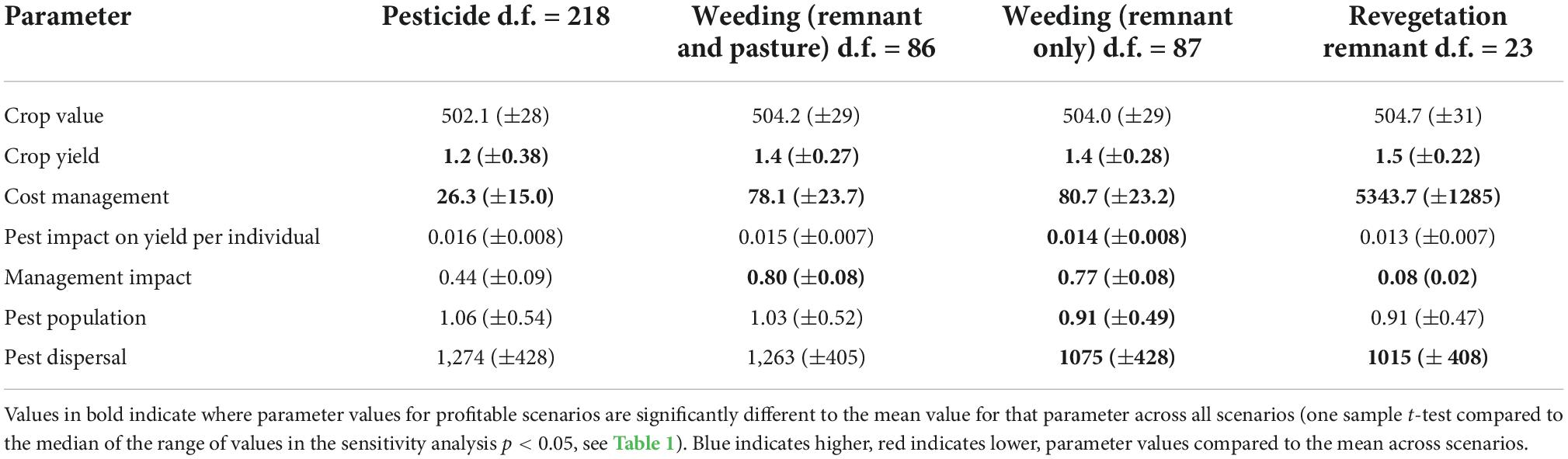
Table 3. Mean values (and standard deviation) of each variable for scenarios that achieved a gross cumulative profit at year 4.
Across the landscape, pest pressure in crops varied spatially according to the landscape configuration and pest model. Therefore field-scale benefits varied spatially and there are likely differences between the overall benefit (as reported here) and individual field scale benefits (with some likely negative even if the overall benefit is positive), see Figure 3.
The impacts of the pest per se was not a big factor in determining a gross profit (Eq. 7), either the individual pest impact on yield or the pest population itself. However, pest dispersal was important when the scenario was focused on the management of remnant vegetation. This is because the effects of the management of remnant vegetation (reduced pest populations in the case of weeding and also increased predation in the case of revegetation) have a scale of effect relative to the location of the native vegetation (i.e., how far the pests disperse, and the limited range of the predation function). Thus, when pest dispersal is relatively low there is more likely to be success of remnant vegetation management practices in the model.
The impact of the spray application itself (management impact) when varied across the parameter range of uncertainty (Table 1, impact of pesticide spray on pest) was not important for pesticide management profit, but when and how many sprays were applied was important, as they multiply the management impact: only 2 sprays and early spray (in June) were the most common parameters in spray management scenarios that returned a gross profit (Supplementary Figures 7A–C).
Effects of management and pest pressure on yield
When comparing the yield under management (a scenario of a single application of pesticide early season) against the null model (no control), the efficacy of management in terms of benefit in dollars varies in a logical manner (Figure 4A). Under low pest pressure the economics of control may result in only a relatively low economic benefit, but as pest pressure increases the benefit of control reaches an optimum, after which it declines again as pest pressure becomes greater than the benefit from increasing control (Figure 4B).
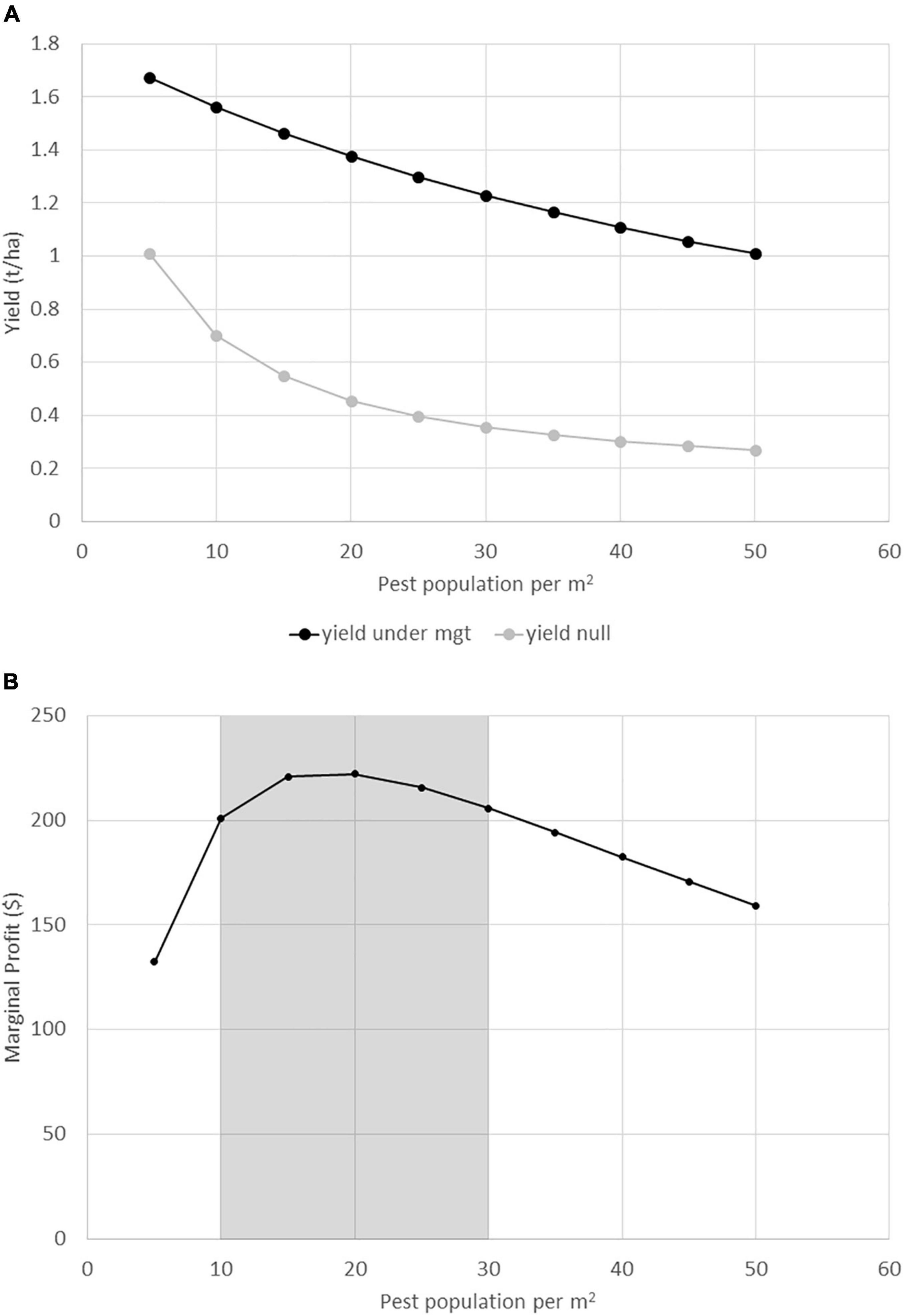
Figure 4. Comparison of yield between pesticide management and non-management scenarios (A) and the marginal profit from management (B) under increasing pest pressure. Gray shading indicates the zone of optimal profit gain.
Gross profits for management scenarios over time
Under conditions where mean values were used for all parameters (Table 1), the annual gross profit, measured in today’s values, is modified by a discount rate of 4% on the benefit [costs are assumed to remain at present (nominal) value]. Considering benefits alone (without costs), the greatest benefit in comparison to the null model, i.e., without management, is to weed pasture (Figure 5). This means that the greatest reduction in pest effects on yield is achieved by controlling the largest population source in the landscape (pasture) before pest outbreaks occur. Weeding of native vegetation remnants (NV) is of much less benefit as this is a less significant source (smaller area in the landscape). Revegetation, which bolsters beneficial populations in NV remnants, is more beneficial in comparison to weeding NV, and only marginally less beneficial than spraying with pesticide. Variance is higher between fields when assessing the benefits of non-crop management options compared to pesticide management (Figure 5), as the benefits may vary spatially depending on the location of the crop in relation to the non-crop habitat.
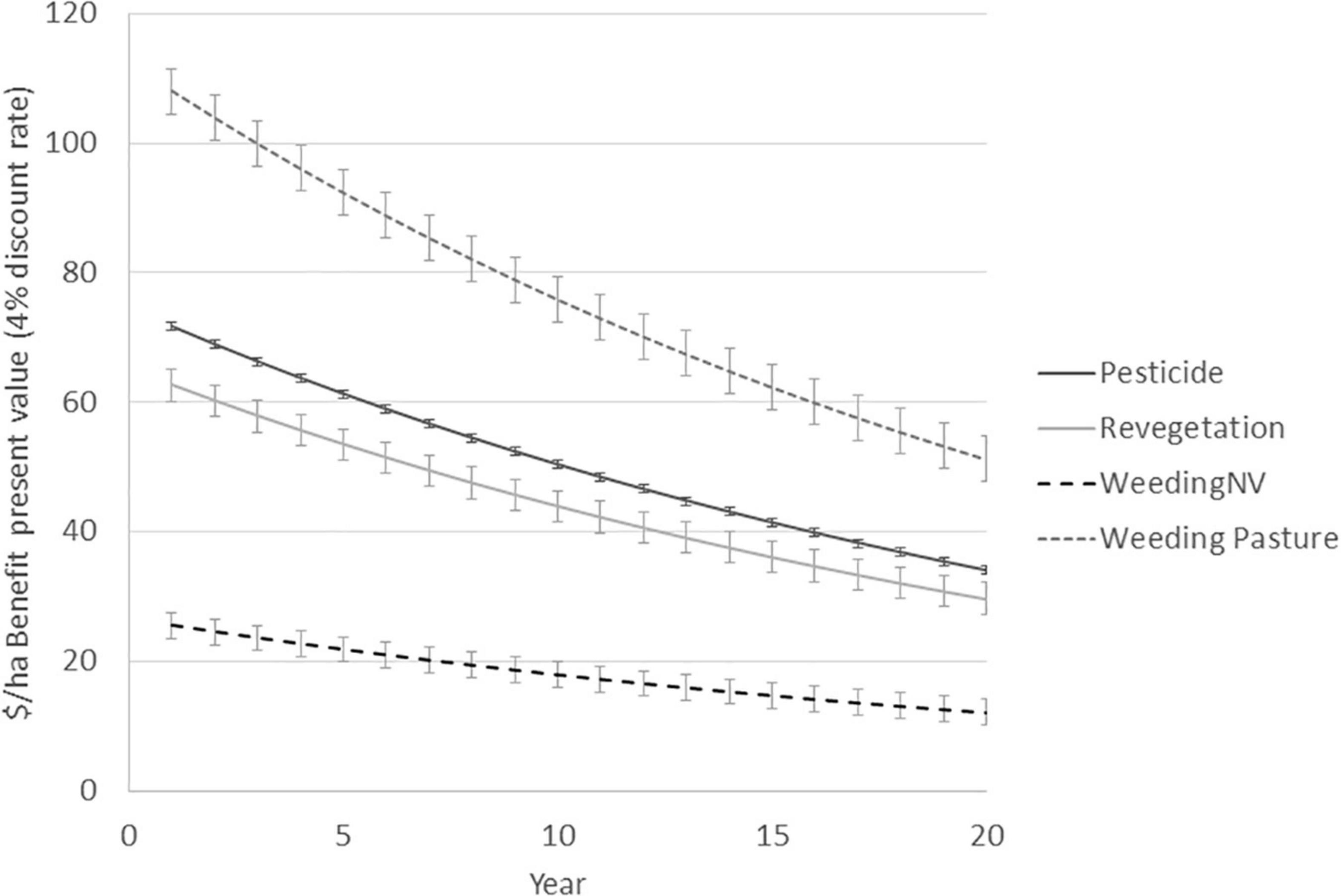
Figure 5. Mean annual benefit ($) in present value across the landscape for mean parameter values of each management scenario over time in comparison to the null (no management) model, including an annual discount rate of 4% on the benefit [error bars indicate standard error of benefit ($) between canola fields in the landscape].
The model estimated that the gross profit for pesticide application in the first year is $7.6 per ha; for weeding (remnant NV) it is a loss of $18.2 per ha; for weeding (pasture) it is a loss of $109.1 per ha; and for revegetation it is a loss of $429.7 per ha due to the large up-front cost. The benefits of revegetation occur over a long timeframe, but all costs are at the beginning (assuming weeding costs are negligible except in the first year). Whereas, although pesticide management has a low cost, it is ongoing. However, by discounting future benefits with an assumption that costs will remain at present (nominal) values for the next 20 years, then the cumulative benefit of pesticide becomes finite over time in this scenario (Figure 6). On the other hand, at this point the cumulative profit of revegetation is continuing to increase with no future costs and, even with a discounted future benefit value, after 10 years it becomes profitable. Therefore, in the medium- to long- term, revegetation is likely more profitable than ongoing pesticide use even when uncertainty due to discount rates is applied (Figure 6).
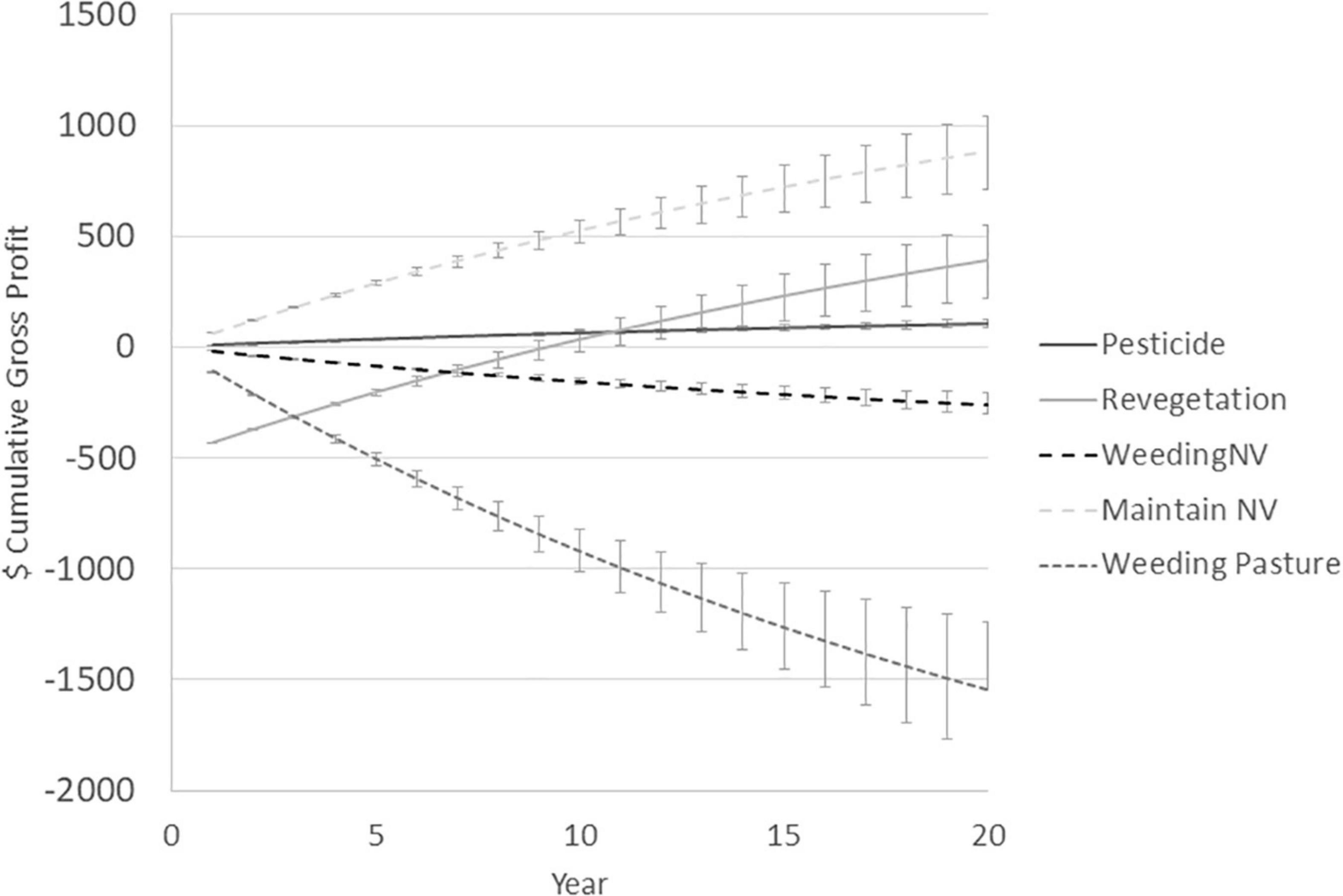
Figure 6. Mean cumulative gross profit for canola across the landscape for mean parameter values of each management scenario over time, including a discount rate of 4% on the benefit and cost (error bars indicate a discount rate of 2 and 7%).
Native vegetation management benefits
Of course, if there is no cost to revegetate (i.e., there is existing native vegetation in good condition), then there will be 100% profit in maintaining good native vegetation, and this can be quantified as pest control benefits in comparison to a situation where such vegetation is not present, realizing a substantial cumulative gross profit (Figure 7). In reality, there is likely to be a need for some level of ongoing weed management of existing native vegetation to maintain condition, which will reduce the cumulative gross profit.
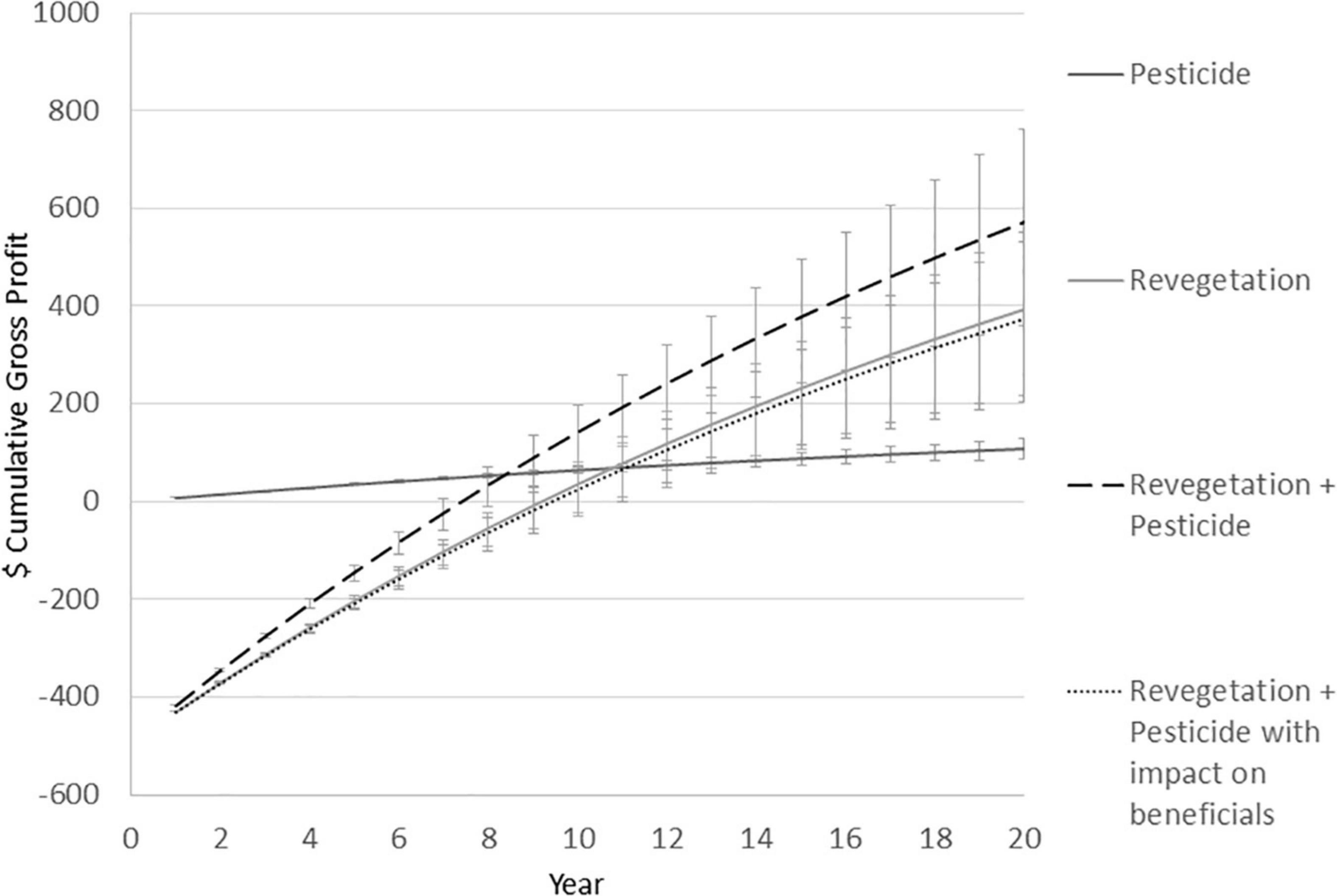
Figure 7. Mean cumulative gross profit for canola achieved over time by independent management scenarios of pesticide and revegetation, compared to a scenario of revegetation with pesticide application where the pesticide has a negative impact on beneficials. Including a discount rate of 4% on the benefit and cost (error bars indicate a discount rate of 2 and 7%).
When modeling the combined effects of management practices, it is important to consider potential negative feedbacks. In the case of pesticides, it is known they can negatively impact on beneficial insects (Pullen et al., 1992; Thompson, 2001; Franzmann et al., 2008), and the management of Rutherglen bug is particularly reliant on broad spectrum chemicals. A comparison between the revegetation scenario and a scenario with the addition of pesticide (both with and without a negative impact on the beneficials) shows that pesticides may enhance benefits achieved by revegetation only in the short-term, and in the long-term, particularly with negative impacts on beneficials, the continued uneconomic use of pesticides undermines the benefits of revegetation (Figure 7).
Comparing landscape context: Low intensity vs. high intensity cropping landscape
In addition, the model was run under a landscape scenario of low cropping intensity (high native vegetation and pasture). Due to the much larger area of non-crop habitat to manage, there is a much higher cost of management across the landscape for revegetation and weeding. In all cases, management of the pest in this landscape scenario was not likely to be profitable, even with pesticide (as pest pressure is greater) (Supplementary Figure 9).
Discussion
A novel model for simulating pest population dynamics in time and space and exploring the economics of multiple management practices for pest control has been developed. The model incorporates the benefits of managing non-crop habitat for integrated pest management and biological control, in addition to pesticide. This presents a leap forward in quantifying the economics of biological control in agro-ecosystems alongside pesticides, something that has been acknowledged in terms of the economics of pest control: “Biological control is an underlying pillar of integrated pest management, yet little focus has been placed on assigning economic value to this key ecosystem service” (Naranjo et al., 2015, pp. 621).
Although management of non-crop systems have been a focus for conservation biological control research, this is very rarely valued as part of economic assessments. Rudimentary relationships between biodiversity in the landscape and crop yield loss have not been quantified, although there is much observational evidence of a relationship between the two (Zaller et al., 2008, 2009; Naranjo et al., 2015). The challenges to achieving this with the model were both spatial and temporal, and thus a spatially explicit simulation approach has proved valuable. This has allowed for comparisons between management of a limited spatial and temporal extent (pesticide), with management that may be actioned in one location but have a “benefit” in another (non-crop vegetation management) and where the economic benefit is not realized immediately but becomes evident over time. The quantitative modeling of the mechanisms by which “biodiversity” in the landscape can lead to crop pest suppression is important to the ability to model this relationship: The impact of non-crop vegetation management was simulated in terms of pest population suppression at source (weed removal) and increased predation in the crop due to a boost in beneficials (revegetation) based on field studies (Parry et al., 2015, 2019). Such relatively simple ecological management changes that target non-crop landscape features are thought to have a greater appeal to farmers than in-field solutions, according to a recent review (Kleijn et al., 2019). However, there are limited examples in the literature of the approach taken here, despite a clear need to align the spatial scale of economic analysis with the scale of ecological interactions (Adamson et al., 2014; Schellhorn et al., 2015b). A similar, spatially explicit, “bioeconomic” approach was taken to evaluate weed management strategies in cereals by González-Díaz et al. (2015), where a weed population dynamic model was combined with a weed-crop competition model and economic sub-model, however, in that case the weed management component was simpler in that it occurred only in-field (i.e., without a spatial separation between management actions outside the crop and in-field benefits, as modeled here).
A case study comparison of the management scenarios for Rutherglen bug under mean parameter conditions demonstrates some of the complexity that the model can explore in terms of spatial and temporal scales of management economics. The comparison of the cumulative gross profit over time (with discounted future costs and benefits) shows that for management practices with ongoing costs (pesticide and weed management) the margin is not favorable, and declines over time for pesticide. Although initially the gross margin for revegetation was very poor due to the upfront cost, this rapidly increased and a positive economic return on investment could be expected after 10 years with cumulative gross profits continuing to increase. The model estimates an annual return of $30/ha after 20 years of revegetation. Combining treatments of pesticide and revegetation doesn’t give an additive effect: the model estimates that the additional control benefit that might be expected by spraying is in effect halved, due to the impact of pesticide on the predation function associated with native vegetation. Recently, empirical evidence of this negative interaction has shown that semi-natural margins benefit pest control only in unsprayed fields (Gagic et al., 2019). Further exploration of the interactions between these two management practices could help optimize pesticide applications to minimize the negative effects on revegetation benefits (c.f. Yang et al., 2016). In all cases, revegetating with, or conserving, native vegetation in this landscape is likely to be economically beneficial in terms of pest control, if viewed as a long-term investment. Other relevant approaches to model combined methods of pest control are given in Barclay (1992).
The model is sensitive to several parameters, in particular crop yield, the management cost, the management impact (or timing/number of sprays in the case of pesticide), and pest dispersal if the management action is in remnant vegetation. Relevant data may not be available for other species; for example, here data from a Canadian study for a similar species in canola (Wise and Lamb, 1998) was used to calibrate the pest damage function, as this was not available for N. vinitor in Australia. If some key parameters change, like the pest pressure/damage function and yield (and thus value of the crop) increases, then a lot more might be gained from any management of the pest, but in particular modification to the general populations of the pest and natural enemies that is achieved by non-crop habitat manipulation (cf Stern et al., 1959). The finding that low maximum yield loss due to pest damage results in control not reaching an economic return (no matter what form of control) aligns with estimations of economic damage thresholds that are in the order of 20% yield loss: for sorghum this is attributed to 20 or more individuals per head at the flowering or soft dough stage (Miles, 2012). A similar recommendation is made for canola (10 adults or 20 nymphs per plant, Miles, 1997), however, the corresponding yield loss is not given. Importantly, the sensitivity analysis of the model presented here illustrates that pest damage is only one factor that determines whether control is likely to give an economic return; even when the maximum pest damage is relatively high other factors such as the yield itself, the cost of the management and the impact of the management are also important to the economic outcome. The model presented here shows that the effect of pest pressure on economic returns from control is likely to be non-linear, with optimal gain from implementing control at an intermediate level of pest pressure (cf. Mumford and Norton, 1984; Waterfield and Zilberman, 2012; Macfadyen et al., 2014). This will also vary spatially depending on dispersal of both the pest and natural enemies: here a simplistic estimate of movement range is applied in the model, as few studies have quantified the spillover of populations of both natural enemies and pests from non-crop habitat (but see Macfadyen et al., 2015; Samaranayake and Costamagna, 2018; Boetzl et al., 2019). These factors pose the question as to whether a single static “economic threshold” for pest management can ever suffice to quantify economic returns from management across multiple regions and management conditions and indicates the need for a more dynamic approach. This modeling tool can form the basis of such a dynamic approach.
There are several assumptions and simplifications in the model (including the focus on a single pest). One assumption is that the provision of pest control services is immediate when revegetation occurs, however, it is likely that it might take some time to build these services and a time-lag may occur between the management action and the benefit (Kleijn et al., 2019). However, the model shows quite strongly that profit is likely from revegetation over the long term in the intensively cropped landscape; even with a lag in benefit that result is unlikely to change. The simulation model also provides a framework in which this lag effect, and the subsequent implications for incentives for non-crop management for pest control, could be tested. Likewise, the efficacy of pesticide may decline over time (particularly if used at low dosage but high frequency) as pests develop resistance to the chemicals, or secondary pest problems develop due to the impacts of pesticides on natural enemies (Hill et al., 2017): this would have a negative impact on the economic gains that can be achieved with ongoing pesticide use. Combined with increasingly stringent regulation of pesticides and the external costs of pesticide use that accrue to society such as water pollution, human health impacts and reduced biodiversity (not included in the present model), the alternative non-crop management scenarios presented here are likely to be even more appealing (Griffiths et al., 2008), particularly if they were incentivized through subsidies (or if pesticides were highly taxed) (Jacquet et al., 2011). At the same time, there are some simplifications made in this study to the economics that may influence the results, in particular the use of “nominal prices” and ignoring inflation may not give a clear picture of the dynamics of the economic return over time. By considering only the direct effects, the gross margin approach fails to account for feedbacks (e.g., market adjustments and dynamic supply and demand trends, which are central in economic evaluations). For example, the study may overestimate long-term economic impacts of a pest that impacts canola yield when there exists a suitable substitute commodity (e.g., winter wheat) – however, taking such complex feedbacks into account was beyond the scope of this study.
Currently, the population dynamic of the pest in the non-crop habitat is modeled deterministically, based on data at a particular location. The “predation function” is even more simplified as a fixed factor that doesn’t consider predator population dynamics. Both could be altered to a mechanistic, process-based population dynamic model driven by climatic conditions, which would allow for the model to translate more easily to other regions but would also introduce significant complexity. This would likely necessitate a finer scale timestep in the model (currently monthly), to better reflect pest generation times. The model can also implement multiple pest species and consider all crops in the landscape; this would help us consider whether management of non-crop habitat will provide multiple economic benefits, and thus provide an “ecological intensification” that will be more attractive to farmers (Kleijn et al., 2019). This could be overlaid with a model of the social interactions in the landscape, perhaps similar to that of Cong et al. (2014), to allow for a consideration of incentives in coordinated area-wide management that may be necessary for non-crop management for pest control to function in practice. In order to apply this model in another context, such as Europe, it would be necessary to consider the evidence for how the role of non-crop habitat may differ in relation to pests and beneficials in canola (Zaller et al., 2008, 2009).
Importantly, the landscape itself impacts the results. For example, in a landscape with a much higher proportion of non-crop habitat (either native vegetation remnants or pasture) there is a greater potential source of pests in the non-crop area requiring control if the vegetation is not well managed. The results here show that, although not economical, the best outcome in terms of pest suppression and prevention of yield loss would be to weed pastures, as they are the largest area that provides a source of pests (see also Nagy et al., 2020). This means that the economics of management of non-crop areas may not be favorable, and the value of the native vegetation remnants for pest management may be low (cf. Polyakov et al., 2015, “native vegetation exhibits diminishing marginal benefits as its proportion of a property increases.” See also Griffiths et al., 2008). However, although the area of non-crop habitat might be larger, it may not be necessary to manage the whole area to achieve a benefit: there may be some trade-off between management intensity and extent. Indeed, coordination of actions at the landscape scale between landholders are likely to be important, and area-wide management incentives may be necessary as the landscape as presented here would likely be divided into separate properties in reality. A consideration of what should be managed, where and when to gain the most benefit both for individual growers and across a region is a challenging question, that could be addressed by further explorations with this model. A comparable bioeconomic modeling approach was recently taken to explore trade-offs for ecosystem services of pollination when field sizes increase (Kirchweger et al., 2020). Ecosystem services such as pest control will be influenced by the landscape configuration and distance between the crops and natural habitats that provide these services (Kremen, 2005). Here, a framework is provided to quantify the benefits and costs of different pest management strategies that can be further refined to explore what solutions may be most economically viable (e.g., flower strips) that can still provide benefits in high native vegetation landscapes.
In summary, this novel model quantified and compared, within the bounds of uncertainty, farm landscape-scale economic benefits of both pesticide and softer management approaches to insect pest control in an intensively cropped agricultural landscape. Further incentive to shift to more ecological approaches may be provided by increasing cost of chemicals (and reduced availability), resistance, policy interventions and societal acceptance of the environmental costs of high-input farming (Waterfield and Zilberman, 2012; Kleijn et al., 2019). Accounting for external benefits to society of reduced pesticide usage is beyond the scope of this study, as well as costs (negative externalities) to others due to unintended consequences, e.g., pesticide use by one farmer may impose negative externality to other adjunct farms through pollination decline. Such accounting would further support the adoption of softer management approaches. However, even if the evidence provided by the model is accepted, this is no guarantee that there will be a change in practice. A range of landscape and local factors may influence the choices of farmers in insect pest management (as first identified by Mumford and Norton, 1984), and to-date decisions have been made largely based only on the evidence of short-term economic benefits, even for the adoption of novel biodiversity-enhancing practices. Agronomic decisions are unfortunately highly influenced by the conflicted interests of agricultural consultants who work for chemical companies (Kleijn et al., 2019). However, it is hoped that the quantification of the private benefit that can be gained from the management of non-crop vegetation to suppress pests and support beneficials, as presented here, may be of value to begin to shift farmers in intensively cropped farming landscapes to consider more ecological pest management practices as economically beneficial.
Data availability statement
Publicly available datasets were analyzed in this study. This data can be found here: https://data.csiro.au/collection/csiro:55021.
Author contributions
The author confirms being the sole contributor of this work and has approved it for publication.
Funding
This work was supported primarily by the Australian Grains Research and Development Corporation (GRDC) project CSA00045 (Linkage), and relates to ongoing project CSP2104-007RTX (Improved Management of Rutherglen bug in the Northern Region). It used data collected in a past GRDC project CSE00051 (Pest Suppressive Landscapes) – see data availability statement.
Acknowledgments
The author thanks my colleagues who collaborated on the projects listed above, particularly with respect to field study design and data collection, which provided valuable information for the modeling work presented here and is published elsewhere: both projects were led by Dr. Nancy Schellhorn (CSIRO), and the following contributed to the data collection and/or study design: Dr. Svetlana Micic, Dr. Art Diggle, Mr. Mike Grimm, Mr. Tony Dore (DAFWA), Dr. Paul Yeoh, Mr. Andrew Hulthen, Mr. Mick Neave, Dr. Sarina Macfadyen, Dr. Felix Bianchi (CSIRO), Ms. Zara Hall, Mr. Adam Quade, Mr. Matt Davis, and Dr. Jamie Hopkinson (QDAF). Many thanks to Dr. Paul Mwebaze (CSIRO) for his advice on, and early review of, the economic aspect of the modeling, as well as Dr. Sarina Macfadyen and Dr. Abebayehu Geffersa (CSIRO).
Conflict of interest
The author declares that the research was conducted in the absence of any commercial or financial relationships that could be construed as a potential conflict of interest.
Publisher’s note
All claims expressed in this article are solely those of the authors and do not necessarily represent those of their affiliated organizations, or those of the publisher, the editors and the reviewers. Any product that may be evaluated in this article, or claim that may be made by its manufacturer, is not guaranteed or endorsed by the publisher.
Supplementary material
The Supplementary Material for this article can be found online at: https://www.frontiersin.org/articles/10.3389/fevo.2022.970624/full#supplementary-material
References
Adamson, D., Zalucki, M. P., and Furlong, M. J. (2014). “Pesticides and integrated pest management practice, practicality and policy in Australia,” in Integrated pest management: Experiences with implementation, global overview, Vol. 4, eds R. Peshin and D. Pimentel (New York, NY: Springer), 387–411.
Barclay, H. J. (1992). Combining methods of insect pest control: Partitioning mortality and predicting complementarity. Res. Popul. Ecol. 34, 91–107.
Barton, M., Parry, H., Ward, S., Hoffmann, A. A., Umina, P. A., van Helden, M., et al. (2021). Forecasting impacts of biological control under future climates: Mechanistic modelling of an aphid pest and a parasitic wasp. Ecol. Modell. 457:109679. doi: 10.1016/j.ecolmodel.2021.109679
Bianchi, F. J. J. A., Booij, C. J. H., and Tscharntke, T. (2006). Sustainable pest regulation in agricultural landscapes: A review on landscape composition, biodiversity and natural pest control. Proc. R. Soc. B Biol. Sci. 273, 1715–1727. doi: 10.1098/rspb.2006.3530
Boerema, A., Rebelo, A. J., Bodi, M. B., Esler, K. J., and Meire, P. (2017). Are ecosystem services adequately quantified? J. Appl. Ecol. 54, 358–370. doi: 10.1111/1365-2664.12696
Boetzl, F. A., Krimmer, E., Krauss, J., and Steffan-Dewenter, I. (2019). Agri-environmental schemes promote ground-dwelling predators in adjacent oilseed rape fields: Diversity, species traits and distance-decay functions. J. Appl. Ecol. 56, 10–20. doi: 10.1111/1365-2664.13162
Butts, R. A., and Lamb, R. J. (1991). Pest status of Lygus bugs (Hemiptera, Miridae) in oilseed brassica crops. J. Econ. Entomol. 84, 1591–1596. doi: 10.1093/jee/84.5.1591
Chaplin-Kramer, R., O’Rourke, M. E., Blitzer, E. J., and Kremen, C. (2011). A meta-analysis of crop pest and natural enemy response to landscape complexity. Ecol. Lett. 14, 922–932. doi: 10.1111/j.1461-0248.2011.01642.x
Chaplin-Kramer, R., O’Rourke, M. E., Schellhorn, N., Zhang, W., Robinson, B. E., Gratton, C., et al. (2019). Measuring what matters: Actionable information for conservation biocontrol in multifunctional landscapes. Front. Sustain. Food Syst. 3:60. doi: 10.3389/fsufs.2019.00060
Cong, R.-G., Smith, H. G., Olsson, O., and Brady, M. (2014). Managing ecosystem services for agriculture: Will landscape-scale management pay? Ecol. Econ. 99, 53–62. doi: 10.1016/j.ecolecon.2014.01.007
Cousens, R. (1985). A simple model relating yield loss to weed density. Ann. Appl. Biol. 107, 239–252. doi: 10.1111/j.1744-7348.1985.tb01567.x
Downes, S., Kriticos, D., Parry, H., Paull, C., Schellhorn, N., and Zalucki, M. P. (2017). A perspective on management of Helicoverpa armigera: Transgenic Bt cotton, IPM, and landscapes. Pest Manag. Sci. 73, 485–492. doi: 10.1002/ps.4461
Franzmann, B. A., Hardy, A. T., Murray, D. A. H., and Henzell, R. G. (2008). Host-plant resistance and biopesticides: Ingredients for successful integrated pest management (IPM) in Australian sorghum production. Aust. J. Exp. Agric. 48, 1594–1600. doi: 10.1071/EA08071
Furlong, M. J., Ang, G. C. K., Silva, R., and Zalucki, M. P. (2018). Bringing ecology back: How can the chemistry of indirect plant defenses against herbivory be manipulated to improve pest management? Front. Plant Sci. 9:1436. doi: 10.3389/fpls.2018.01436
Gagic, V., Hulthen, A. D., Marcora, A., Wang, X., Jones, L., and Schellhorn, N. A. (2019). Biocontrol in insecticide sprayed crops does not benefit from semi-natural habitats and recovers slowly after spraying. J. Appl. Ecol. 56, 2176–2185. doi: 10.1111/1365-2664.13452
Gagic, V., Paull, C., and Schellhorn, N. A. (2018). Ecosystem service of biological pest control in Australia: The role of non-crop habitats within landscapes. Aust. Entomol. 57, 194–206. doi: 10.1111/aen.12328
González-Díaz, L., Blanco-Moreno, J. M., and González-Andújar, J. L. (2015). Spatially explicit bioeconomic model for weed management in cereals: Validation and evaluation of management strategies. J. Appl. Ecol. 52, 240–249. doi: 10.1111/1365-2664.12359
Griffiths, G. J. K., Holland, J. M., Bailey, A., and Thomas, M. B. (2008). Efficacy and economics of shelter habitats for conservation biological control. Biol. Control 45, 200–209. doi: 10.1016/j.biocontrol.2007.09.002
Grimm, V., Berger, U., Bastiansen, F., Eliassen, S., Ginot, V., Giske, J., et al. (2006). A standard protocol for describing individual-based and agent-based models. Ecol. Modell. 198, 115–126. doi: 10.1016/j.ecolmodel.2006.04.023
Grimm, V., Berger, U., DeAngelis, D. L., Polhill, J. G., Giske, J., and Railsback, S. F. (2010). The ODD protocol A review and first update. Ecol. Modell. 221, 2760–2768. doi: 10.1016/j.ecolmodel.2010.08.019
Gustafson, E. J., and Parker, G. R. (1992). Relationships between landcover proportion and indices of landscape spatial pattern. Landsc. Ecol. 7, 101–110. doi: 10.1007/BF02418941
Hill, M. P., Macfadyen, S., and Nash, M. A. (2017). Broad spectrum pesticide application alters natural enemy communities and may facilitate secondary pest outbreaks. PeerJ 5:e4179. doi: 10.7717/peerj.4179
Huang, J., Zhou, K., Zhang, W., Deng, X., van der Werf, W., Lu, Y., et al. (2018). Uncovering the economic value of natural enemies and true costs of chemical insecticides to cotton farmers in China. Environ. Res. Lett. 13:064027. doi: 10.1088/1748-9326/aabfb0
Hunt, P., Thatcher, L., Downes, S., Binns, M., Gardinier, D., Vadakattu, G., et al. (2021). Overcoming a chemically limited future for weed, pest and disease control in agriculture. ECOS 278. Available online at: https://ecos.csiro.au/a-chemically-limited-future/
Jacquet, F., Butault, J.-P., and Guichard, L. (2011). An economic analysis of the possibility of reducing pesticides in French field crops. Ecol. Econ. 70, 1638–1648. doi: 10.1016/j.ecolecon.2011.04.003
Jonsson, M., Bommarco, R., Ekbom, B., Smith, H. G., Bengtsson, J., Caballero-Lopez, B., et al. (2014). Ecological production functions for biological control services in agricultural landscapes. Methods Ecol. Evol. 5, 243–252. doi: 10.1111/2041-210X.12149
Kirchweger, S., Clough, Y., Kapfer, M., Steffan-Dewenter, I., and Kantelhardt, J. (2020). Do improved pollination services outweigh farm-economic disadvantages of working in small-structured agricultural landscapes?–Development and application of a bio-economic model. Ecol. Econ. 169:106535. doi: 10.1016/j.ecolecon.2019.106535
Kleijn, D., Bommarco, R., Fijen, T. P. M., Garibaldi, L. A., Potts, S. G., and van der Putten, W. H. (2019). Ecological intensification: Bridging the gap between science and practice. Trends Ecol. Evol. 34, 154–166. doi: 10.1016/j.tree.2018.11.002
Kremen, C. (2005). Managing ecosystem services: What do we need to know about their ecology? Ecol. Lett. 8, 468–479. doi: 10.1111/j.1461-0248.2005.00751.x
Li, Z., Furlong, M. J., Yonow, T., Kriticos, D. J., Bao, H. L., Yin, F., et al. (2019). Management and population dynamics of diamondback moth (Plutella xylostella): Planting regimes, crop hygiene, biological control and timing of interventions. Bull. Entomol. Res. 109, 257–265. doi: 10.1017/S0007485318000500
Macfadyen, S., Hardie, D. C., Fagan, L., Stefanova, K., Perry, K. D., DeGraaf, H. E., et al. (2014). Reducing insecticide use in broad-acre grains production: An Australian study. PLoS One 9:e89119. doi: 10.1371/journal.pone.0089119
Macfadyen, S., Hopkinson, J., Parry, H., Neave, M. J., Bianchi, F. J. J. A., Zalucki, M. P., et al. (2015). Early-season movement dynamics of phytophagous pest and natural enemies across a native vegetation-crop ecotone. Agric. Ecosyst. Environ. 200, 110–118. doi: 10.1016/j.agee.2014.11.012
McDonald, G., and Farrow, R. A. (1988). Migration and dispersal of the Rutherglen bug, Nysius vinitor Bergroth (Hemiptera: Lygaeidae), in eastern Australia. Bull. Entomol. Res. 78, 493–509. doi: 10.1017/S0007485300013249
Miles, M. (1997). Control thresholds and sampling recommendations for insect pests of field crops and pasture. Horsham: Vic DPI AgNote.
Miles, M. (2012). Management of Rutherglen bug in seedling crops, sorghum and sunflower - updated. Los Angeles, CA: The Beat sheet.
Moradi-Vajargah, M., and Parry, H. R. (2017). Environmental and biological drivers of flight initiation in a sporadic pest, Rutherglen bug, Nysius vinitor Bergroth (Hemiptera: Orsillidae). Aust. Entomol. 56, 225–234. doi: 10.1111/aen.12225
Mumford, J. D., and Norton, G. A. (1984). Economics of decision making in pest management. Annu. Rev. Entomol. 29, 157–174. doi: 10.1146/annurev.en.29.010184.001105
Murray, D. A. H., Clarke, M. B., and Ronning, D. A. (2013). Estimating invertebrate pest losses in six major Australian grain crops. Aust. J. Entomol. 52, 227–241. doi: 10.1111/aen.12017
Nagy, R., Bell, L. W., Schellhorn, N. A., and Zalucki, M. P. (2020). Role of grasslands in pest suppressive landscapes: How green are my pastures? Aust. Entomol. 59, 227–237. doi: 10.1111/aen.12464
Namoi Catchment Management Authority (2013). Planting native trees on farms: A guide to revegetation in the Namoi catchment. Gunnedah: Namoi Catchment Management Authority.
Naranjo, S., Ellsworth, P., and Frisvold, G. (2015). Economic value of biological control in integrated pest management of managed plant systems. Annu. Rev. Entomol. 60, 621–645. doi: 10.1146/annurev-ento-010814-021005
Navarro, J., Bryan, B. A., Marinoni, O., Eady, S., and Halog, A. (2016). Mapping agriculture’s impact by combining farm management handbooks, life-cycle assessment and search engine science. Environ. Model. Softw. 80, 54–65. doi: 10.1016/j.envsoft.2016.02.020
Parry, H., Marcora, A., Macfadyen, S., Hopkinson, J., Hulthen, A. D., Neave, M., et al. (2019). A native with a taste for the exotic: Weeds and pasture provide year-round habitat for Nysius vinitor Bergroth (Hemiptera: Orsillidae). Aust. Entomol. 58, 237–247. doi: 10.1111/aen.12391
Parry, H. R., Macfadyen, S., Hopkinson, J. E., Bianchi, F. J. J. A., Zalucki, M. P., Bourne, A., et al. (2015). Plant composition modulates arthropod pest and predator abundance: Evidence for culling exotics and planting natives. Basic Appl. Ecol. 16, 531–543. doi: 10.1016/j.baae.2015.05.005
Pedigo, L., Hutchins, S., and Higley, L. (1986). Economic injury levels in theory and practice. Annu. Rev. Entomol. 31, 341–368. doi: 10.1146/annurev.en.31.010186.002013
Polyakov, M., Pannell, D. J., Pandit, R., Tapsuwan, S., and Park, G. (2015). Capitalized amenity value of native vegetation in a multifunctional rural landscape. Am. J. Agric. Econ. 97, 299–314. doi: 10.1093/ajae/aau053
Pullen, A. J., Jepson, P. C., and Sotherton, N. W. (1992). Terrestrial non-target invertebrates and the autumn application of synthetic pyrethroids: Experimental methodology and the trade-off between replication and plot size. Arch. Environ. Contam. Toxicol. 23, 246–258. doi: 10.1007/BF00212283
Samaranayake, K. G. L. I., and Costamagna, A. C. (2018). Levels of predator movement between crop and neighboring habitats explain pest suppression in soybean across a gradient of agricultural landscape complexity. Agric. Ecosyst. Environ. 259, 135–146. doi: 10.1016/j.agee.2018.03.009
Schellhorn, N. A., Gagic, V., and Bommarco, R. (2015a). Time will tell: Resource continuity bolsters ecosystem services. Trends Ecol. Evol. 30, 524–530. doi: 10.1016/j.tree.2015.06.007
Schellhorn, N. A., Parry, H. R., Macfadyen, S., Wang, Y., and Zalucki, M. P. (2015b). Connecting scales: Achieving in-field pest control from areawide and landscape ecology studies. Insect Sci. 22, 35–51. doi: 10.1111/1744-7917.12161
Seijo, J. C., Defeo, O., and Salas, S. (1998). Fisheries bioeconomics: Theory, modelling and management. FAO Fisheries Technical Paper No. 368. Rome: FAO.
Southwood, T. R. E., and Norton, G. A. (1973). “Economic aspects of pest management strategies and decisions,” in Insects: Studies in population management, eds P. W. Geier, L. R. Clark, D. J. Anderson, and H. A. Nix (Canberra: Ecological Society of Australia), 68–84.
Stern, V. M., Smith, R. F., van den Bosch, R., and Hagen, K. (1959). The integrated control concept. Hilgardia 29, 81–101. doi: 10.3733/hilg.v29n02p081
Thompson, H. M. (2001). Assessing the exposure and toxicity of pesticides to bumblebees (Bombus sp.). Apidologie 32, 305–321. doi: 10.1051/apido:2001131
Uyttenbroeck, R., Hatt, S., Paul, A., Boeraeve, F., Piqueray, J., Francis, F., et al. (2016). Pros and cons of flowers strips for farmers. A review. Biotechnol. Agron. Soc. Environ. 20, 225–235. doi: 10.25518/1780-4507.12961
Veres, A., Petit, S., Conord, C., and Lavigne, C. (2013). Does landscape composition affect pest abundance and their control by natural enemies? A review. Agric. Ecosyst. Environ. 166, 110–117. doi: 10.1016/j.agee.2011.05.027
Vira, B., and Adams, W. M. (2009). Ecosystem services and conservation strategy: Beware the silver bullet. Conserv. Lett. 2, 158–162. doi: 10.1111/j.1755-263X.2009.00063.x
Waage, J. K., and Barlow, N. D. (1993). “Decision tools for biological control,” in Decision tools for pest management, eds G. A. Norton and J. D. Mumford (Wallingford: CAB International), 229–245.
Waterfield, G., and Zilberman, D. (2012). Pest management in food systems: An economic perspective. Annu. Rev. Environ. Resour. 37, 223–245. doi: 10.1146/annurev-environ-040911-105628
Whitcomb, R. F., Robbins, C. S., Lynch, J. F., Whitcomb, B. L., Klimkiewicz, M. K., and Bystrak, D. (1981). “Effects of forest fragmentation on avifauna of the eastern deciduous forest,” in Forest island dynamics in man-dominated landscapes, eds R. L. Burgess and D. M. Sharpe (New York, NY: Springer-Verlag), 125–205. doi: 10.1007/978-1-4612-5936-7_8
Wilson, L. J., Whitehouse, M. E. A., and Herron, G. A. (2018). The management of insect pests in Australian cotton: An evolving story. Annu. Rev. Entomol. 63, 215–237. doi: 10.1146/annurev-ento-020117-043432
Wise, I. L., and Lamb, R. J. (1998). Economic threshold for plant bugs, Lygus spp. (Heteroptera: Miridae), in canola. Can. Entomol. 130, 825–836. doi: 10.4039/Ent130825-6
Yang, L., Elbakidze, L., Marsh, T., and McIntosh, C. (2016). Primary and secondary pest management in agriculture: Balancing pesticides and natural enemies in potato production. Agric. Econ. 47, 609–619. doi: 10.1111/agec.12259
Zaller, J. G., Moser, D., Drapela, T., Schmöger, C., and Frank, T. (2008). Insect pests in winter oilseed rape affected by field and landscape characteristics. Basic Appl. Ecol. 9, 682–690. doi: 10.1016/j.baae.2007.10.004
Zaller, J. G., Moser, D., Drapela, T., Schmöger, C., and Frank, T. (2009). Parasitism of stem weevils and pollen beetles in winter oilseed rape is differentially affected by crop management and landscape characteristics. BioControl 54, 505–514. doi: 10.1007/s10526-009-9212-2
Zhang, W., and Swinton, S. M. (2009). Incorporating natural enemies in an economic threshold for dynamically optimal pest management. Ecol. Modell. 220, 1315–1324. doi: 10.1016/j.ecolmodel.2009.01.027
Keywords: ecological intensification, integrated pest management (IPM), spatial simulation, Rutherglen bug, canola, ecosystem services, regenerative agriculture
Citation: Parry H (2022) Economic benefits of conservation biocontrol: A spatially explicit bioeconomic model for insect pest management in agricultural landscapes. Front. Ecol. Evol. 10:970624. doi: 10.3389/fevo.2022.970624
Received: 16 June 2022; Accepted: 20 September 2022;
Published: 09 December 2022.
Edited by:
Michael J. Furlong, The University of Queensland, AustraliaReviewed by:
Prof Myron Zalucki, The University of Queensland, AustraliaPascal Querner, Natural History Museum Vienna, Austria
Copyright © 2022 Parry. This is an open-access article distributed under the terms of the Creative Commons Attribution License (CC BY). The use, distribution or reproduction in other forums is permitted, provided the original author(s) and the copyright owner(s) are credited and that the original publication in this journal is cited, in accordance with accepted academic practice. No use, distribution or reproduction is permitted which does not comply with these terms.
*Correspondence: Hazel Parry, hazel.parry@csiro.au