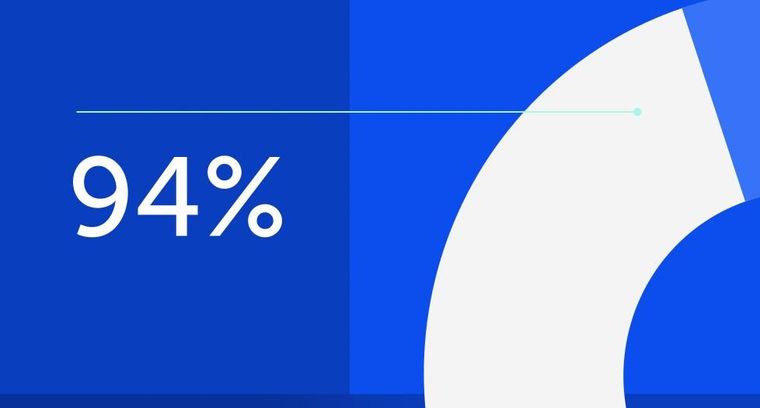
94% of researchers rate our articles as excellent or good
Learn more about the work of our research integrity team to safeguard the quality of each article we publish.
Find out more
ORIGINAL RESEARCH article
Front. Ecol. Evol., 08 September 2022
Sec. Evolutionary and Population Genetics
Volume 10 - 2022 | https://doi.org/10.3389/fevo.2022.970249
This article is part of the Research TopicRecent Advances in Museomics: Revolutionizing Biodiversity ResearchView all 16 articles
Advances in the field of museomics have promoted a high sampling demand for natural history collections (NHCs), eventually resulting in damage to invaluable resources to understand historical biodiversity. It is thus essential to achieve a consensus about which historical tissues present the best sources of DNA. In this study, we evaluated the performance of different historical tissues from Iberian wolf NHCs in genome-wide assessments. We targeted three tissues—bone (jaw and femur), maxilloturbinal bone, and skin—that have been favored by traditional taxidermy practices for mammalian carnivores. Specifically, we performed shotgun sequencing and target capture enrichment for 100,000 single nucleotide polymorphisms (SNPs) selected from the commercial Canine HD BeadChip across 103 specimens from 1912 to 2005. The performance of the different tissues was assessed using metrics based on endogenous DNA content, uniquely high-quality mapped reads after capture, and enrichment proportions. All samples succeeded as DNA sources, regardless of their collection year or sample type. Skin samples yielded significantly higher amounts of endogenous DNA compared to both bone types, which yielded equivalent amounts. There was no evidence for a direct effect of tissue type on capture efficiency; however, the number of genotyped SNPs was strictly associated with the starting amount of endogenous DNA. Evaluation of genotyping accuracy for distinct minimum read depths across tissue types showed a consistent overall low genotyping error rate (<7%), even at low (3x) coverage. We recommend the use of skins as reliable and minimally destructive sources of endogenous DNA for whole-genome and target enrichment approaches in mammalian carnivores. In addition, we provide a new 100,000 SNP capture array validated for historical DNA (hDNA) compatible to the Canine HD BeadChip for high-quality DNA. The increasing demand for NHCs as DNA sources should encourage the generation of genomic datasets comparable among studies.
Natural history collections (NHCs) have been gathered since the seventeenth century, motivated by human curiosity about our planet’s biodiversity and the breakthrough in preserving perishable material (Farrington, 1915). Currently, national, regional, and private collections worldwide own irreplaceable natural resources, offering wide perspectives across distinct temporal and spatial scales that inspire research in many scientific areas (Casas-Marce et al., 2012; Tsangaras and Greenwood, 2012; Lopez et al., 2020; Pearson et al., 2020). Such collections provide unique overviews of historical biodiversity, from both extinct and extant species, and are also essential resources for addressing questions about species that have sampling limitations due to financial, bureaucratic, or conservation constraints (Burrell et al., 2015). Advances in molecular genetics and sequencing technology have promoted the use of the naturally fragmented DNA of historical specimens, transforming NHCs into invaluable sources of material for investigating genetics-related questions among different fields, including phylogenetics, biogeography, and conservation (Suarez and Tsutsui, 2004; Holmes et al., 2016; Bi et al., 2019).
Whereas a growing body of studies using historical DNA (hDNA) illustrate the potential of NHCs in genetic research, their regular use still poses different challenges. Sampling of genetic material is often destructive, eventually compromising the integrity of specimens and jeopardizing their future use. Therefore, because sampling techniques that minimize damages are prioritized (Pálsdóttir et al., 2019), the amount of genetic material collected is often limited (Horváth et al., 2005). Additionally, traditional taxidermy practices, which commonly use hazardous chemicals, and general carelessness in protecting specimens from environmental damage, do not favor DNA preservation. Thus, historical samples often yield limited and highly degraded DNA (Raxworthy and Smith, 2021) that presents major challenges during laboratory and analytical procedures (Allentoft et al., 2012; Dabney et al., 2013). Moreover, hDNA extracts can contain a non-negligible proportion of exogenous DNA from pre- or post-mortem sources, frequently in overwhelming ratios (Weiß et al., 2016; McDonough et al., 2018; Eisenhofer et al., 2019). These factors may explain why genetic studies using hDNA have often relied on the amplification of short nuclear or mitochondrial fragments (e.g., Schwartz et al., 2007; Maebe et al., 2016; Lonsinger et al., 2019). However, the use of hDNA is nowadays facilitated by high-throughput sequencing and by recent developments in molecular methods (e.g., Rowe et al., 2011; Staats et al., 2013; Hung et al., 2014). Methods like sequence capture of target loci, which limits the representation of the genome to specific loci, are among the most used in genome-wide studies of low-quality DNA to achieve large and cost-effective datasets (Jones and Good, 2016; McCormack et al., 2016; Derkarabetian et al., 2019). Furthermore, despite requiring a priori availability of the target genome to design specific baits, target enrichment has been shown to be successful using bait designs based on closely related species (Vallender, 2011).
Mammals have been traditionally preserved in NHCs by archiving skins, bones, teeth, or mounted specimens (Rowe et al., 2011). It is thus not surprising that most of the available genetic studies using hDNA rely on these tissues (Raxworthy and Smith, 2021). However, DNA yields may vary greatly among different tissues and be dependent on curation history (Burrell et al., 2015). Hard tissues, such as teeth and bones, were at first thought to provide higher-quality DNA (Wandeler et al., 2007; Casas-Marce et al., 2010), encouraging proposals to use hard tissues assumed to minimize sampling damage, such as maxilloturbinal bone (Wisely et al., 2004). Yet, recent studies have shown conflicting results (Rowe et al., 2011; Lonsinger et al., 2019; Tsai et al., 2020), revealing soft tissues to be good sources of hDNA when preserved appropriately (Burrell et al., 2015). To date, few studies have implemented genomic resources to assess differences in the performance of distinct tissues, and those tackling this question are often based on very low sample sizes that hamper reliable statistical comparisons (e.g., Rowe et al., 2011; McDonough et al., 2018). Thus, the tissue of choice for increasing the quality of genomic data, while sampling mammal NHCs with minimal damaging, remains an open question (Raxworthy and Smith, 2021).
In this work, we sought to evaluate the performance of different mammalian carnivore tissues generally available at NHCs in genome-wide assessments. Using the Iberian wolf (Canis lupus signatus) as a case study, we first collected bones (jaw and femur), maxilloturbinal bones, and skins from 103 historical specimens (Figure 1A). Then, we performed a shotgun sequencing of these samples to characterize endogenous DNA content. Third, we developed and tested a capture array of 100,000 regions overlapping single nucleotide polymorphisms (SNPs) contained in the commercial Illumina Canine HD BeadChip, ensuring compatibility between datasets generated with both low- and high-quality DNA. We intended to answer three main questions: Does endogenous DNA content differ across historical tissues? How is capture efficiency affected by historical tissue type? What is the effect of read depth on SNP genotyping error rates from hDNA?
Figure 1. Characterization of samples and hDNA used in this work. (A) Photographs illustrating the specimens used for the collection of each tissue type (Image credits Raquel Godinho). (B,C) Correlation (r2) between DNA yield (ng) following extraction and the proportion of endogenous DNA content with the original collection date of the specimens. Each dot represents a historical sample and inset boxplots display the median (central line) and distribution per tissue type. Significant differences (p < 0.05) between tissues are identified with an asterisk. Colors depict the three different tissue types used in this study: orange—bones (jaw and femur); yellow—maxilloturbinal (nasal) bones; and, green—skins.
We collected 103 samples from Iberian wolf specimens housed at the three largest museum collections in the Iberian Peninsula and at 15 private NHCs, consisting of 43 bones (jaw and femur), 31 maxilloturbinal bones (hereafter nasal bones), and 29 skins. The original collection years for these specimens ranged from 1912 to 1990, except for two samples from 2004 and 2005 (Figure 1, Supplementary Table 1). All our samples conform to the definition of historical samples by Raxworthy and Smith (2021), which restricts hDNA to that fortuitously obtained from traditional museum specimens not intended to serve as sources of DNA. Jaw and femur bones were sampled by drilling ca. 1 g of bone powder with a Dremel tool (Dremel, WI, United States; Supplementary Figure 1). Femurs were sampled in the patellar surface region, whereas jaws were sampled in the posterior lower region of the mandibula (see Supplementary Figure 1 for examples of the drilling location in each bone type). Nasal bones were collected following Wisely et al. (2004), by inserting sterilized forceps into the nasal cavity of the skull to extract the bones. Nasal bone material was posteriorly crushed into small fragments. Bones were not bleached prior to DNA extraction. Skin samples were collected from pelts or mounted specimens by extracting a patch of approximately 2 cm2. Collecting tools, including drill bits, were cleaned with bleach, and flamed with 96% ethanol between samples to minimize cross contamination.
We prepared DNA extracts using 50 mg of sample following Dabney and Meyer (2019). For skins, we favored the inner layer for DNA extraction and discarded hairs to minimize contamination with external DNA sources. DNA concentration was measured using the Qubit fluorometer dsDNA HS Assay Kit (Thermo Fisher Scientific, MA, United States). We used 100–300 ng of DNA to prepare blunt-end dual-indexed DNA sequencing libraries using a full-uracil-DNA-glycosylase treatment following Meyer and Kircher’s (2010) protocol with the modifications described in Kircher et al. (2012). To limit DNA contamination, DNA extractions and library preparations were conducted in dedicated rooms under sterile conditions and positive air pressure, and negative controls were used alongside the procedures. DNA libraries were diluted based on concentration measurements obtained with the Qubit fluorometer dsDNA HS Assay Kit, and library size ranges were characterized using a Bioanalyzer 2100 with High Sensitivity DNA kits (Agilent Technologies, CA, United States). To characterize the endogenous DNA content of each sample, we performed a shotgun sequencing run using one lane of an Illumina HiSeq X instrument (Illumina, CA, United States) in PE 150 bp mode. For this, we selected libraries with concentrations > 15 ng/μl (N = 79; Nbone = 29; Nnasal bone = 30; Nskin = 20) to ensure that enough library material remained for the following steps (based on the required starting amount for target capture enrichment of 14–72 ng/μl).
We also generated genomic information from two contemporary wolf muscle samples to be used as positive controls to validate the implemented approach. No animals were killed or injured for this study. We isolated DNA from these samples using the DNeasy Blood and Tissue Kit (Qiagen, Hilden, Germany). DNA quantification and library preparation were performed following the same procedures as described above for historical samples but with two modifications during library preparation: (i) a shearing step using a Bioruptor Pico sonication device (Diagenode, NJ, United States) was performed to obtain fragments of ∼250 bp, and (ii) the USER enzyme treatment was not performed. These two samples were genotyped using two different approaches: the Illumina CanineHD BeadChip (Illumina, CA, United States; ∼170,000 SNPs) and the capture array of 100,000 regions developed in this work (see next sections).
We targeted a set of autosomal genome-wide SNPs whose positions were defined based on the coordinates available on the CanineHD BeadChip. With this experimental design, we ensured the compatibility of historical datasets with other datasets generated using the same SNP chip. From the ∼170,000 SNPs in the CanineHD BeadChip, only uniquely mapped autosomal SNPs were considered for probe design. Based on this list of putative SNPs, we custom designed a sequence capture panel containing a final set of 100,000 SNPs distributed across the wolf genome using MYbaits Target Capture technology (Arbor Biosciences, Ann Arbor, MI, United States). The implemented methods for probe design followed the strategy described by Haak et al. (2015) and Cruz-Dávalos et al. (2017), in which four probes of 60 bp each were designed to target fragments of 120 bp per SNP: one upstream of the SNP, one downstream, and one for each possible allele, with the allele positioned at the center of the probe. RNA probes were designed and synthesized by Arbor Biosciences (product code #302016). The selection of the final set of MYbaits RNA probes was based on their capture efficiency (stringent criteria ≤ 25% repeat masked). A FASTA file providing the sequences of all 400,000 synthesized probes is available online.
We performed target enrichment using the MYbaits Custom Target Capture Kit following the manufacturer protocol (v.3.02). DNA libraries with similar endogenous DNA content were pooled in equimolar sets of 8–10 samples per capture reaction (600 ng in the final pool). Hybridization between RNA probes and the DNA library occurred at 65°C for 40 h. Real-time PCRs were performed to determine the number of amplification cycles required to obtain sufficient molarity for sequencing. Post-enrichment libraries were amplified using KAPA HiFi Hotstart Ready Mix (KAPA Biosystems, MA, United States), following the manufacturer recommendations, with an annealing temperature of 60°C. The amplified enriched DNA libraries were purified in 20 μl of EB buffer using the MinElute PCR Purification kit (Qiagen, Hilden, Germany) and quantified by the Qubit dsDNA HS Assay Kit. Library size ranges were characterized using a Bioanalyzer 2100 with High Sensitivity DNA kits and pooled in equimolar ratios. Pooled libraries were then sequenced using 2 lanes of an Illumina HiSeq X instrument in PE 150 bp mode.
Raw sequences from shotgun sequencing and target enrichment were processed similarly in different time periods. First, sequence reads were demultiplexed and quality assessments were done using FastQC (Andrews, 2018) and MultiQC (Ewels et al., 2016). Sequence reads were then processed and aligned using the PALEOMIX v.1.2.13.2 BAM pipeline (Schubert et al., 2014). Briefly, the pipeline consisted of the following steps: (i) adapter sequences were removed, low-quality and N bases were trimmed, and overlapping read pairs were collapsed using AdapterRemoval v.2.2.2 (Schubert et al., 2016) with the default parameters (on average, 96 and 95% of reads were collapsed for shotgun and capture sequencing, respectively); (ii) all sequence reads were then aligned against the CanFam3.1 dog reference genome (Lindblad-Toh et al., 2005) using BWA-MEM v.0.7.17 (Heng, 2013); and (iii) PCR duplicates for each library were marked by the “paleomix rmdup_collapsed” tool but were not used for the following steps. The final alignment file was subjected to local realignment around indels using the GATK v.3.8 IndelRealigner tool (DePristo et al., 2011). The endogenous content was determined by the ratio of unique reads (no duplicates) that mapped to the dog reference genome with mapping quality above 20 (MQ > 20) to the total number of available reads (also referred as library complexity by some authors; Dehasque et al., 2022). For the target enrichment experiment, the number of reads mapping on target was defined as the total number of unique high-quality (MQ > 20) reads overlapping at least 1 base of the 120 bp target region. Enrichment success was determined by the ratio of reads on target in relation to (i) the total number of available reads and (ii) the total number of uniquely and high-quality mapped reads. These metrics were retrieved from the PALEOMIX summary report and, additionally, with the help of SAMtools v.1.9 (Li et al., 2009). Fold enrichment was determined by the ratio of the number of on-target reads to the total number of uniquely and high-quality mapped reads, divided by the expected representation of the target regions without enrichment (i.e., the ratio of genome length, 2.4 Gb, to target length, 100,000 × 120 bp, corresponding to 0.5%).
Following target enrichment, genotypes were called using BCFtools v.1.10.2 (Li, 2011) mpileup/call -m tools, with minimum Phred-scaled thresholds of 20 for base quality and read mapping quality. At the end, to evaluate the effect of sequencing depth on genotyping quality rates, we considered genotypes supported by at least three (DP ≥ 3), four (DP ≥ 4), or more reads (DP ≥ 5) using the custom python script gtvalues2plink.py, which was also used to convert the final VCF file to plink format. The distribution and density of SNPs in our dataset (using DP ≥ 4) was visualized using the R/Bioconductor package karyoploteR (Gel and Serra, 2017) in R (R Development Core Team, 2017).
Genotypes were ultimately validated by estimating their concordance rates with the genotypes obtained from the control samples using the Canine HD BeadChip. In this last approach, genotype calling was performed using GenomeStudio software (Illumina), following Illumina’s recommendations. Sex-chromosome-related SNPs and non-uniquely mapped SNPs (SNPs with multiple positions attributed) were removed from the dataset using PLINK v.1.9 (Purcell et al., 2007), resulting in a final dataset of ∼ 121,000 SNPs. Concordance rates were calculated for the entire set of genome-wide SNPs obtained across the three depth thresholds for each control sample, using a second custom script, SNP_concordance.py. We also calculated concordance rates for SNPs called exclusively with 3x and 4x coverage in each control sample and estimated the associated error rates. Additionally, to assess the genotype quality across all historical samples, we estimated the potential genotyping error rate associated with low read depth using the ErrorCount.sh script from the dDocent pipeline (Puritz et al., 2014). Briefly, this script reports a low range based on a 50% binomial probability of observing the second allele in a heterozygote and a high range based on a 25% probability. All genotyped SNPs were considered for this analysis without any filter for missing data.
To determine whether the amount of endogenous DNA and capture efficiency were influenced by sample type (bone, nasal bone, and skin), we implemented a set of generalized linear models (GLMs). We ran four different GLMs with the following dependent variables: (i) proportion of endogenous DNA, i.e., number of reads that mapped uniquely with MQ > 20 in relation to the total number of available reads, following shotgun sequencing; (ii) mapped reads after capture, i.e., proportion of the number of reads that mapped uniquely and with MQ > 20 to the total number of reads available after target enrichment; (iii) reads on target (all), i.e., number of unique and high-quality (MQ > 20) reads that mapped on target regions in relation to the total number of reads available; and (iv) reads on target (mapped), i.e., the same as above, but in relation to the total number of mapped reads. All GLMs were fitted with a binomial error distribution and a logit link. The fit of each model was further assessed using the Pearson’s χ2 residuals, which test whether any significant patterns remain in the residuals. Given the unavailability of shotgun sequencing data for all the samples, we tested levels of correlation between the proportion of endogenous DNA and the proportion of reads mapping after capture to understand if the latter could be interpreted as a proxy for endogenous content. We also tested correlation coefficients using the following variables: original collection year, DNA yield (ng) following extraction, endogenous DNA proportion, fragment length (average length of filtered reads from shotgun sequencing), and mapping length (average length of mapped and unique reads, with MQ > 20). The effect of sample type on DNA concentration was also evaluated using a Kruskal-Wallis test. All the previously mentioned tests were performed in R, and all the plots were constructed using the R package ggpubr (Kassambara, 2020).
We successfully obtained DNA extracts for all 103 historical samples, with an average DNA yield of 469.91 ± 63.18 (s.e.) ng; (range 84–3,812 ng). DNA yield was not correlated with the original specimen collection year (r2 = 0.15) nor with the sample type (Kruskal-Wallis test, p = 0.256; Figure 1B). Nevertheless, even using the same quantity of starting material for DNA extraction, we cannot rule out greater effects due to histological differences between hard and soft tissues. The initial sample characterization by shotgun sequencing resulted in an average fragment length of 97 bp (range 42–145 bp). Endogenous DNA content across all samples varied from 0.05 to 76.35% (mean: 14.48 ± 2.47%; Figure 1C) and was not correlated with the fragment length (r2 = −0.42; Supplementary Figure 2A) nor with the original collection year (r2 = −0.24; Figure 1C). The average mapping length across all samples was 75 bp (range 40–116 bp) and was not correlated with specimen original collection year (r2 = 0.15; Supplementary Figure 2B). Among sample types, skin samples retrieved the highest proportion of endogenous DNA (43.49 ± 4.76%, p = 0.008; Figure 1C and Supplementary Tables 2, 3) in relation to bones (8.14 ± 2.66%) and nasal bones (1.28 ± 0.22%).
After capture enrichment, the proportion of reads mapping to the reference genome ranged from 0.13 to 78.26% (mean: 19.89 ± 2.59%; Supplementary Table 2). Consistently, skin samples presented a significantly higher proportion of mapped reads (49.01 ± 4.69%, p = 0.001; Figure 2 and Supplementary Tables 2, 4) relative to bones (12.57 ± 2.99%) and nasal bones (2.79 ± 0.64%). Across contemporary control samples, the average proportion of reads mapping after capture was 32.85 ± 0.56% (Supplementary Table 5), with 65.4% of filtered reads being duplicates. Endogenous DNA content and the proportion of reads mapping to the reference genome following capture were highly correlated (r2 = 0.93; Supplementary Figure 3A).
Figure 2. Genomic metrics assessed from capture enrichment for each tissue type: bones (orange), maxilloturbinal (nasal) bones (yellow), and skins (green). Boxplots display the proportion of reads mapping after capture enrichment and reads mapping on target region in relation to all or mapped reads for each tissue. Within boxplots, dots represent historical samples, and the central line indicates the median value. Significant differences (p < 0.05) between tissues for each metric are identified with an asterisk.
Following capture enrichment, the proportion of reads mapping on target regions ranged from 0.01 to 27.79% (mean: 4.75 ± 0.73%; Supplementary Table 2) in relation to all available reads and from 1 to 37% (mean: 19.82 ± 0.84%; Supplementary Table 2) in relation to all mapped reads. The average fold enrichment was 39.64x, with 91% of all samples presenting an enrichment > 10x. Among sample types, skin samples showed the highest proportion of reads mapping on target regardless of the metric used (12.35 ± 1.58% of all reads; 22.49 ± 1.65% of mapped reads; Figure 2). Bones (2.68 ± 0.78% of all reads; 18.13 ± 1.41% of mapped reads) and nasal bones (0.50 ± 0.11% of all reads; 19.68 ± 1.18% of mapped reads) worked less successfully; however, differences were not significant among the three sample types (Figure 2 and Supplementary Tables 6, 7). For the two contemporary control samples, the proportion of reads mapping on target regions was 17.54 ± 0.01% of all available reads and 53.42 ± 0.69% of mapped reads (Supplementary Table 5).
We were able to generate genotypes for a panel of 99,982 genome-wide SNPs (Supplementary Figure 4) using the target enrichment approach, with distinct levels of missing data across samples. For contemporary control samples, concordance rates between genotypes obtained from the capture array developed in this study and those from the Canine HD BeadChip were above 99%. Error rates for the two control samples (i.e., rates of genotype discordance) were almost negligible but increased with decreasing coverage at the target sites (0.64 and 0.91% error rate, at coverage ≥ 5x and ≥ 3x, respectively; Figure 3A and Supplementary Table 8). When calculating the genotype concordance rates using SNPs called exclusively with 3x and 4x, we found a decrease to 94.1 and 96.4%, respectively (Supplementary Table 8). The most common error found in genotypes called with the lowest coverage (3x) was the dropout of a second allele (miscalled homozygous in relation to the SNP chip), corresponding to 79.5% of the observed discordances. The number of loci obtained in contemporary control samples increased as the coverage decreased; for example, the average number of SNPs obtained across both samples declined from 93,948 to 88,173 at coverage ≥ 3x and ≥ 5x, respectively (Figure 3B and Supplementary Table 9).
Figure 3. Genotyping performance of hDNA from three sample types. (A) Interval of potential genotyping error rate estimates (%) for the three hDNA sample types, and average empirical genotyping error rate for control samples, considering the three minimum depth levels. (B) Percentage of genotyped SNPs (N = 99,982) considering a minimum depth of 3x, 4x, or 5x per SNP across 103 samples. Each dot represents a sample.
We found the same pattern across historical samples, with increased estimates of potential genotyping error rates associated with SNPs called with decreasing coverage (Figure 3A). Nevertheless, the highest error rate estimate for coverage ≥ 3x did not reach 7%. Concordantly, we also found an increase in the average number of SNPs across all samples for lower read depths (24,663 and 18,523 SNPs at coverage ≥ 3x and ≥ 5x; Figure 3B and Supplementary Table 9). Overall, the average number of SNPs obtained across historical samples was substantially lower than in contemporary control samples. Still, the highest genotyping success rates were found among three historical samples, which presented very similar rates (>95%) to those found in the contemporary samples (Figure 3B and Supplementary Table 9). Skin samples presented the highest average numbers of SNPs genotyped (Figure 3B). Genotyping success rate (number of genotyped SNPs) was positively correlated with the proportion of reads mapping to the genome after capture (r2 = 0.85; Supplementary Figure 3B).
In this study, we evaluated the performance of different historical tissues—bones, nasal bones, and skins—across a population sampling of Iberian wolf NHCs, in whole-genome sequencing and target enrichment. Specifically, we analyzed metrics based on endogenous DNA content, uniquely high-quality mapped reads after capture, and enrichment proportions.
Bones, nasal bones, and skins all succeeded as DNA sources but differed in endogenous DNA content. Based on equal starting amounts of DNA for shotgun sequencing, skins yielded significantly higher amounts of endogenous DNA than did the other sample types. This concords with other studies that have previously shown that soft tissues of mammals (van der Valk et al., 2017) and birds (Tsai et al., 2020) provide higher endogenous DNA content than hard tissues, although these are based on less comprehensive sample sizes than the one used here. Although nasal bones were initially recommended for presenting higher genotyping success rates than other bones (Wisely et al., 2004), a recent study showed conflicting results (Lonsinger et al., 2019). Here, we assessed for the first time the proportion of endogenous DNA retrieved from nasal bones, showing that this bone type provides lower content than other commonly used bones. Despite the observed significant effect of tissue type on endogenous DNA, we cannot disregard the impact of distinct collection histories in this result (Raxworthy and Smith, 2021). We did not observe significant associations between endogenous DNA content, or its mapping length, and the original collection year, suggesting no substantial DNA degradation across the period under evaluation.
There was no direct effect of tissue type on capture efficiency, with most samples presenting an enrichment > 10x. This result emphasizes the efficiency of target capture as a powerful method for building genomic datasets from hDNA and is in line with other studies using capture approaches (Carpenter et al., 2013; van der Valk et al., 2017). An a priori understanding of the importance of endogenous DNA content in the success of a capture experiment (Hernandez-Rodriguez et al., 2018) has driven our decision to perform shotgun sequencing prior to capture enrichment. Using this approach, we were able to demonstrate a perfect association between the number of resulting SNPs and the initial amount of endogenous DNA, emphasizing that this is a practical and cost-effective way to select samples prior to capture experiments. Furthermore, knowing the endogenous DNA content across samples also allows to minimize variation within each sequencing experiment, ensuring low rates of index hopping and reducing possible bias in downstream analysis (van der Valk et al., 2020). Our SNP genotyping results demonstrate that historical samples with high amounts of endogenous DNA can behave similarly to, or even better than, contemporary samples in capture procedures, further supporting the use of capture enrichment to genotype thousands of genome-wide SNPs in hDNA samples (Bi et al., 2013; Smith et al., 2014; Harvey et al., 2016; Lim and Braun, 2016).
The final number of SNPs in a dataset can be a trade-off between coverage and genotyping error rates: relaxing the minimum read depth to increase the number of SNPs is accompanied by higher uncertainty in genotype calling. Still, we were able to generate an average of ca. 25,000 genome-wide SNPs per sample, called with ≥ 3x coverage and with low potential error rates (<7%). Reducing coverage led to an increase in the number of SNPs, mostly across samples with intermediate genotyping success, i.e., those where SNPs were captured but read depth was generally low. In such cases, decreasing the coverage from ≥ 4x to ≥ 3x represented an increase of ∼11,000 SNPs. Regardless of the threshold used for minimum coverage, genotypes obtained for the contemporary control samples were confirmed and validated against the SNP chip genotypes. The average error rates found in the controls were within, or very close to, the potential error rate intervals estimated for the historical dataset. This overlap suggests that the use of such an analytical approach (Puritz et al., 2014) can be a reliable alternative to estimate potential error rates when no control samples are available. Observed error rates in our work for ≥ 5x coverage, which is a widely accepted threshold for SNP calling (Yi et al., 2010), were much lower than those reported by Fountain et al. (2016) at the same coverage for fresh tissue samples.
The most common error found among control samples was the dropout of a second allele after target enrichment. Given the overall low error rates, we predict minimal impact associated with allelic dropouts in downstream analysis. However, even low rates of genotyping error tend to overestimate genetic variation and can affect population genetic studies in different ways, or, to a greater extent linkage and association studies (Pompanon et al., 2005; Gautier et al., 2013). Since most studies do not have control samples available to calculate empirical concordance rates and validate genotypes (Fountain et al., 2016), predicting the effects of errors might be difficult, and in this case higher minimal depth thresholds (>5x) may be considered. Choosing between increasing the number of loci or having high reliability in the genotypes should be considered on a case-by-case basis to ensure compatibility with downstream applications. Alternatively, historical datasets can be analyzed based on genotype likelihoods instead of genotypes, in order to take the inherent uncertainty of the genotypes into account (Nielsen et al., 2011).
Skin samples were revealed to be a good source of endogenous DNA while still being minimally destructive to specimens, as small patches can be easily sampled from non-unique morphological features of hides or skin mounts. Bone sampling is generally more destructive and does not necessarily translate into higher endogenous DNA content. This applies in particular to nasal bones, which yield the lowest endogenous DNA content while relying on a quite destructive sampling process in which a large part of the structure is removed. Although we acknowledge that the consumptive sampling of nasal bones does not compromise the utility of a specimen for morphometric or character studies (Wisely et al., 2004), our results discourage their sampling for genomic studies of mammalian carnivores.
As the demand for NHCs in molecular studies increases, conscious sampling of specimens should now become routine to not compromise their future use. The drilling procedure we used for bone sampling left a small hole in the bone tissue (Figure 1A and Supplementary Figure 1) but did not hamper future morphometric studies. This sampling approach is less damaging than cutting bone fragments (Supplementary Figure 1) and still ensures that enough material is collected for genomic analysis without further manipulation. Sampling the petrous bone, a recognized excellent source of endogenous DNA (Pinhasi et al., 2015), would only be recommendable for NHC specimens if entailing the use of damaged skulls, as its sampling is highly destructive to the skull (Charlton et al., 2019). The starting amount of endogenous DNA can also be maximized through wet lab procedures by performing several rounds of DNA extraction, creating multiple and differentially indexed DNA libraries to increase complexity levels, and/or captures per sample (Hernandez-Rodriguez et al., 2018; White et al., 2019; Fontsere et al., 2021; von Seth et al., 2021). Understanding that a reduced fragment of the appropriate tissue is enough to successfully recover molecular data without compromising the reusability of the specimen is of remarkable importance for managing NHCs.
In contrast to current practices for preserving biological material in controlled environments and using sophisticated resources that prevent DNA damage, older traditional methods did not prioritize DNA integrity (Hall et al., 1997; Burrell et al., 2015; Card et al., 2021). Thus, these preservation techniques and storage conditions can greatly impact the quality and quantity of DNA. In this work, we used wolf specimens from three museums and 15 private collections distributed across a 90-year period, where most samples (∼60%) were collected between 1960 and 1980. These samples likely have different collection histories, particularly those in private collections, where less-standardized preservation methods can be expected. Such heterogeneity may explain the observed levels of variability in endogenous DNA content, although our sample size hampers a statistical evaluation of these metrics across sampling origins. We acknowledge that it would be relevant to assess the effects of different preservation techniques on DNA degradation; unfortunately, information about the method applied to each specimen used for this study was not available, as is often the case in NHCs.
One of the major challenges the museum community currently faces is improving the documentation of collection histories for individual specimens and to make it accessible to the scientific community. Global initiatives have been promoting the digitization of specimens’ metadata and digital images to make them available in electronic databases that can be easily accessed (e.g., The Global Information Facility; Robertson et al., 2014). As this process evolves, it is essential that researchers working in the field of museomics synergize genomic data with NHCs metadata to enhance the scientific impact and traceability of their studies by, for example, always providing the catalog numbers of specimens used (Card et al., 2021).
To our knowledge, this study uses the most comprehensive dataset to date—in terms of sample size and genome representation—to test the performance of three wolf tissues as sources of hDNA. Based on our findings, we recommend the use of skins for sampling mammalian carnivore specimens, as these are reliable and minimally destructive sources of endogenous DNA suitable for whole-genome and target enrichment approaches. This study should also encourage future research with the same aims but targeting different vertebrate and invertebrate groups. In addition, we provide a validated genome-wide SNP tool (i.e., probe design) that allows for direct comparison between historical and contemporary data. Although the enrichment approach presented here was based on canid genomes, its conceptual design can be implemented in any species for which SNP chips are available. We believe that the increasing demand for NHCs as DNA sources, and the requirements for minimal damage to the specimens, should encourage the generation of genomic datasets comparable among studies.
Individual SNP genotypes and the sequence of the 400,000 RNA probes are available from the OSF repository: https://osf.io/j3r7x/?view_only=a747acf5297c49309bbb89f2e7414104. Raw sequence reads from whole-genome resequencing are available on NCBI (accession SRA PRJNA860381): https://www.ncbi.nlm.nih.gov/sra/PRJNA860381. Custom python scripts (gtvalues2plink.py and SNP_concordance.py) used in this study are available at https://github.com/pdroslva84/SNPcap.
RG coordinated the project. RG, CP, DL, and PS designed the study. RG, JL-B, and FÁ coordinated historical sample collection efforts. RG, JL-B, EG, FÁ, JL, and DL collected samples from historical specimens. CP, DL, and DC conducted laboratory work. CP and DL performed data analysis under the guidance of RG and PS. PS did the bioinformatic scripting. CP, DL, and RG wrote the manuscript with input from all the other authors. All authors contributed to the article and approved the submitted version.
This work was co-funded by the Portuguese Foundation for Science and Technology (FCT) under the project PTDC/BIA-EVF/2460/2014 and the project NORTE-01-0246-FEDER-000063, the Norte Portugal Regional Operational Programme (NORTE2020), under the PORTUGAL 2020 Partnership Agreement, through the European Regional Development Fund (ERDF). DL, CP, RG, and PS were supported by the FCT (PD/BD/132403/2017, PD/BD/135026/2017, 2021/00647/CEECIND, and PTDC/BIA-EVL/31902/2017, respectively). JL-B was supported by the Spanish Ministry of Economy, Industry and Competitiveness (RYC-2015-18932), and the GRUPIN research grant from the Regional Government of Asturias (IDI/2021/000075).
We acknowledge the Museu Nacional de História Natural e da Ciência (Lisbon, Portugal), Museo Nacional de Ciencias Naturales (Madrid, Spain), Estación Biológica de Doñana (Seville, Spain) and all owners of private collections who shared historical wolf samples. The two contemporary tissue samples were provided by the Portuguese Institute for Nature Conservation and Forestry (ICNF) and the Consejería de Medio Ambiente de la Junta de Castilla y León. We are grateful to R. Pedra and S. Roque for helping collecting samples, and to S. Mourão and M. Magalhães for lab assistance.
JL was employed by Consultores en Iniciativas Ambientales, S.L.
The remaining authors declare that the research was conducted in the absence of any commercial or financial relationships that could be construed as a potential conflict of interest.
All claims expressed in this article are solely those of the authors and do not necessarily represent those of their affiliated organizations, or those of the publisher, the editors and the reviewers. Any product that may be evaluated in this article, or claim that may be made by its manufacturer, is not guaranteed or endorsed by the publisher.
The Supplementary Material for this article can be found online at: https://www.frontiersin.org/articles/10.3389/fevo.2022.970249/full#supplementary-material
Allentoft, M. E., Collins, M., Harker, D., Haile, J., Oskam, C. L., Hale, M. L., et al. (2012). The half-life of DNA in bone: Measuring decay kinetics in 158 dated fossils. Proc. R. Soc. B Biol. Sci. 279, 4724–4733. doi: 10.1098/rspb.2012.1745
Andrews, S. (2018). FastQC: A quality control tool for high throughput sequence data (v. 0.11.7). Berlin: ScienceOpen, Inc.
Bi, K., Linderoth, T., Singhal, S., Vanderpool, D., Patton, J. L., Nielsen, R., et al. (2019). Temporal genomic contrasts reveal rapid evolutionary responses in an alpine mammal during recent climate change. PLoS Genet. 15:e1008119. doi: 10.1371/journal.pgen.1008119
Bi, K., Linderoth, T., Vanderpool, D., Good, J. M., Nielsen, R., and Moritz, C. (2013). Unlocking the vault: Next-generation museum population genomics. Mol. Ecol. 22, 6018–6032. doi: 10.1111/mec.12516
Burrell, A. S., Disotell, T. R., and Bergey, C. M. (2015). The use of museum specimens with high-throughput DNA sequencers. J. Hum. Evol. 79, 35–44. doi: 10.1016/j.jhevol.2014.10.015
Card, D. C., Shapiro, B., Giribet, G., Moritz, C., and Edwards, S. V. (2021). Museum Genomics. Annu. Rev. Genet. 55, 633–659. doi: 10.1146/annurev-genet-071719-020506
Carpenter, M. L., Buenrostro, J. D., Valdiosera, C., Schroeder, H., Allentoft, M. E., Sikora, M., et al. (2013). Pulling out the 1%: Whole-Genome capture for the targeted enrichment of ancient dna sequencing libraries. Am. J. Hum. Genet. 93, 852–864. doi: 10.1016/j.ajhg.2013.10.002
Casas-Marce, M., Revilla, E., and Godoy, J. A. (2010). Searching for DNA in museum specimens: A comparison of sources in a mammal species. Mol. Ecol. Resour. 10, 502–507. doi: 10.1111/j.1755-0998.2009.02784.x
Casas-Marce, M., Revilla, E., Fernandes, M., Rodriguez, A., Delibes, M., and Godoy, J. A. (2012). The value of hidden scientific resources: Preserved animal specimens from private collections and small museums. Bioscience 62, 1077–1082. doi: 10.1525/bio.2012.62.12.9
Charlton, S., Booth, T., and Barnes, I. (2019). The problem with petrous? A consideration of the potential biases in the utilization of pars petrosa for ancient DNA analysis. World Archaeol. 51, 574–585. doi: 10.1080/00438243.2019.1694062
Cruz-Dávalos, D. I., Llamas, B., Gaunitz, C., Fages, A., Gamba, C., Soubrier, J., et al. (2017). Experimental conditions improving in-solution target enrichment for ancient DNA. Mol. Ecol. Resour. 17, 508–522. doi: 10.1111/1755-0998.12595
Dabney, J., and Meyer, M. (2019). Extraction of highly degraded DNA from ancient bones and teeth. Methods Mol. Biol. 1963, 25–29. doi: 10.1007/978-1-4939-9176-1_4
Dabney, J., Knapp, M., Glocke, I., Gansauge, M. T., Weihmann, A., Nickel, B., et al. (2013). Complete mitochondrial genome sequence of a Middle Pleistocene cave bear reconstructed from ultrashort DNA fragments. Proc. Natl. Acad. Sci. U. S. A. 110, 15758–15763. doi: 10.1073/pnas.1314445110
Dehasque, M., Pečnerová, P., Kempe Lagerholm, V., Ersmark, E., Danilov, G. K., Mortensen, P., et al. (2022). Development and Optimization of a Silica Column-Based Extraction Protocol for Ancient DNA. Genes 13:687. doi: 10.3390/genes13040687
DePristo, M. A., Banks, E., Poplin, R., Garimella, K. V., Maguire, J. R., Hartl, C., et al. (2011). A framework for variation discovery and genotyping using next-generation DNA sequencing data. Nat. Genet. 43, 491–498. doi: 10.1038/ng.806
Derkarabetian, S., Benavides, L. R., and Giribet, G. (2019). Sequence capture phylogenomics of historical ethanol-preserved museum specimens: Unlocking the rest of the vault. Mol. Ecol. Resour. 19, 1531–1544. doi: 10.1111/1755-0998.13072
Eisenhofer, R., Minich, J. J., Marotz, C., Cooper, A., Knight, R., and Weyrich, L. S. (2019). Contamination in Low Microbial Biomass Microbiome Studies: Issues and Recommendations. Trends Microbiol. 27, 105–117. doi: 10.1016/j.tim.2018.11.003
Ewels, P., Magnusson, M., Lundin, S., and Käller, M. (2016). MultiQC: Summarize analysis results for multiple tools and samples in a single report. Bioinformatics 32, 3047–3048. doi: 10.1093/bioinformatics/btw354
Farrington, O. C. (1915). The rise of Natural History Museums. Science 42, 197–208. doi: 10.1126/science.42.1076.197
Fontsere, C., Alvarez-Estape, M., Lester, J., Arandjelovic, M., Kuhlwilm, M., Dieguez, P., et al. (2021). Maximizing the acquisition of unique reads in noninvasive capture sequencing experiments. Mol. Ecol. Resour. 21, 745–761. doi: 10.1111/1755-0998.13300
Fountain, E. D., Pauli, J. N., Reid, B. N., Palsbøll, P. J., and Peery, M. Z. (2016). Finding the right coverage: The impact of coverage and sequence quality on single nucleotide polymorphism genotyping error rates. Mol. Ecol. Resour. 16, 966–978. doi: 10.1111/1755-0998.12519
Gautier, M., Gharbi, K., Cezard, T., Foucaud, J., Kerdelhué, C., Pudlo, P., et al. (2013). The effect of RAD allele dropout on the estimation of genetic variation within and between populations. Mol. Ecol. 22, 3165–3178. doi: 10.1111/mec.12089
Gel, B., and Serra, E. (2017). KaryoploteR: An R/Bioconductor package to plot customizable genomes displaying arbitrary data. Bioinformatics 33, 3088–3090. doi: 10.1093/bioinformatics/btx346
Haak, W., Lazaridis, I., Patterson, N., Rohland, N., Mallick, S., Llamas, B., et al. (2015). Massive migration from the steppe was a source for Indo-European languages in Europe. Nature 522, 207–211. doi: 10.1038/nature14317
Hall, L. M., Willcox, M. S., and Jones, D. S. (1997). Association of enzyme inhibition with methods of museum skin preparation. Biotechniques 22, 928–934. doi: 10.2144/97225st07
Harvey, M. G., Smith, B. T., Glenn, T. C., Faircloth, B. C., and Brumfield, R. T. (2016). Sequence Capture versus Restriction Site Associated DNA Sequencing for Shallow Systematics. Syst. Biol. 65, 910–924. doi: 10.1093/sysbio/syw036
Heng, L. (2013). Aligning sequence reads, clone sequences and assembly contigs with BWA-MEM*. arXiv [Preprint]. doi: 10.48550/arXiv.1303.3997
Hernandez-Rodriguez, J., Arandjelovic, M., Lester, J., de Filippo, C., Weihmann, A., Meyer, M., et al. (2018). The impact of endogenous content, replicates and pooling on genome capture from faecal samples. Mol. Ecol. Resour. 18, 319–333. doi: 10.1111/1755-0998.12728
Holmes, M. W., Hammond, T. T., Wogan, G. O. U., Walsh, R. E., Labarbera, K., Wommack, E. A., et al. (2016). Natural history collections as windows on evolutionary processes. Mol. Ecol. 25, 864–881. doi: 10.1111/mec.13529
Horváth, M. B., Martínez-Cruz, B., Negro, J. J., Kalmár, L., and Godoy, J. A. (2005). An overlooked DNA source for non-invasive genetic analysis in birds. J. Avian Biol. 36, 84–88. doi: 10.1111/j.0908-8857.2005.03370.x
Hung, C. M., Shaner, P. J. L., Zink, R. M., Liu, W. C., Chu, T. C., Huang, W. S., et al. (2014). Drastic population fluctuations explain the rapid extinction of the passenger pigeon. Proc. Natl. Acad. Sci. U. S. A. 111, 10636–10641. doi: 10.1073/pnas.1401526111
Jones, M. R., and Good, J. M. (2016). Targeted capture in evolutionary and ecological genomics. Mol. Ecol. 25, 185–202. doi: 10.1111/mec.13304
Kassambara, A. (2020). ggpubr R Package: Ggplot2-Based Publication Ready Plots. Available online at: https://rpkgs.datanovia.com/ggpubr/ (accessed July, 2021).
Kircher, M., Sawyer, S., and Meyer, M. (2012). Double indexing overcomes inaccuracies in multiplex sequencing on the Illumina platform. Nucleic Acids Res. 40:e3. doi: 10.1093/nar/gkr771
Li, H. (2011). A statistical framework for SNP calling, mutation discovery, association mapping and population genetical parameter estimation from sequencing data. Bioinformatics 27, 2987–2993. doi: 10.1093/bioinformatics/btr509
Li, H., Handsaker, B., Wysoker, A., Fennell, T., Ruan, J., Homer, N., et al. (2009). The Sequence Alignment/Map format and SAMtools. Bioinformatics 25, 2078–2079. doi: 10.1093/bioinformatics/btp352
Lim, H. C., and Braun, M. J. (2016). High-throughput SNP genotyping of historical and modern samples of five bird species via sequence capture of ultraconserved elements. Mol. Ecol. Resour. 16, 1204–1223. doi: 10.1111/1755-0998.12568
Lindblad-Toh, K., Wade, C. M., Mikkelsen, T. S., Karlsson, E. K., Jaffe, D. B., Kamal, M., et al. (2005). Genome sequence, comparative analysis and haplotype structure of the domestic dog. Nature 438, 803–819. doi: 10.1038/nature04338
Lonsinger, R. C., Daniel, D., Adams, J. R., and Waits, L. P. (2019). Consideration of sample source for establishing reliable genetic microsatellite data from mammalian carnivore specimens held in natural history collections. J. Mammal. 100, 1678–1689. doi: 10.1093/jmammal/gyz112
Lopez, L., Turner, K. G., Bellis, E. S., and Lasky, J. R. (2020). Genomics of natural history collections for understanding evolution in the wild. Mol. Ecol. Resour. 20, 1153–1160. doi: 10.1111/1755-0998.13245
Maebe, K., Meeus, I., Vray, S., Claeys, T., Dekoninck, W., Boevé, J. L., et al. (2016). A century of temporal stability of genetic diversity in wild bumblebees. Sci. Rep. 6:38289. doi: 10.1038/srep38289
McCormack, J. E., Tsai, W. L. E., and Faircloth, B. C. (2016). Sequence capture of ultraconserved elements from bird museum specimens. Mol. Ecol. Resour. 16, 1189–1203. doi: 10.1111/1755-0998.12466
McDonough, M. M., Parker, L. D., McInerney, N. R., Campana, M. G., and Maldonado, J. E. (2018). Performance of commonly requested destructive museum samples for mammalian genomic studies. J. Mammal. 99, 789–802. doi: 10.1093/jmammal/gyy080
Meyer, M., and Kircher, M. (2010). Illumina sequencing library preparation for highly multiplexed target capture and sequencing. Cold Spring Harb. Protoc. 2010:db.rot5448. doi: 10.1101/pdb.prot5448
Nielsen, R., Paul, J. S., Albrechtsen, A., and Song, Y. S. (2011). Genotype and SNP calling from next-generation sequencing data. Nat. Rev. Genet. 12, 443–451. doi: 10.1038/nrg2986
Pálsdóttir, A. H., Bläuer, A., Rannamäe, E., Boessenkool, S., and Hallsson, J. H. (2019). Not a limitless resource: Ethics and guidelines for destructive sampling of archaeofaunal remains. R. Soc. Open Sci. 6:191059. doi: 10.1098/rsos.191059
Pearson, K. D., Nelson, G., Aronson, M. F. J., Bonnet, P., Brenskelle, L., Davis, C. C., et al. (2020). Machine learning using digitized herbarium specimens to advance phenological research. Bioscience 70, 610–620. doi: 10.1093/biosci/biaa044
Pinhasi, R., Fernandes, D., Sirak, K., Novak, M., Connell, S., Alpaslan-Roodenberg, S., et al. (2015). Optimal ancient DNA yields from the inner ear part of the human petrous bone. PLoS One 10:e0129102. doi: 10.1371/journal.pone.0129102
Pompanon, F., Bonin, A., Bellemain, E., and Taberlet, P. (2005). Genotyping errors: Causes, consequences and solutions. Nat. Rev. Genet. 6, 847–859. doi: 10.1038/nrg1707
Purcell, S., Neale, B., Todd-Brown, K., Thomas, L., Ferreira, M. A. R., Bender, D., et al. (2007). PLINK: A Tool Set for Whole-Genome Association and Population-Based Linkage Analyses. Am. J. Hum. Genet. 81, 559–575. doi: 10.1086/519795
Puritz, J. B., Hollenbeck, C. M., and Gold, J. R. (2014). dDocent: A RADseq, variant-calling pipeline designed for population genomics of non-model organisms. PeerJ 2014:e431. doi: 10.7717/peerj.431
R Development Core Team (2017). R: A language and environment for statistical computing. Vienna: R Foundation for Statistical Computing.
Raxworthy, C. J., and Smith, B. T. (2021). Mining museums for historical DNA: Advances and challenges in museomics. Trends Ecol. Evol. 36, 1049–1060. doi: 10.1016/j.tree.2021.07.009
Robertson, T., Döring, M., Guralnick, R., Bloom, D., Wieczorek, J., Braak, K., et al. (2014). The GBIF Integrated Publishing Toolkit: Facilitating the Efficient Publishing of Biodiversity Data on the Internet. PLoS One 9:e102623. doi: 10.1371/journal.pone.0102623
Rowe, K. C., Singhal, S., Macmanes, M. D., Ayroles, J. F., Morelli, T. L., Rubidge, E. M., et al. (2011). Museum genomics: Low-cost and high-accuracy genetic data from historical specimens. Mol. Ecol. Resour. 11, 1082–1092. doi: 10.1111/j.1755-0998.2011.03052.x
Schubert, M., Ermini, L., Der Sarkissian, C., Jónsson, H., Ginolhac, A., Schaefer, R., et al. (2014). Characterization of ancient and modern genomes by SNP detection and phylogenomic and metagenomic analysis using PALEOMIX. Nat. Protoc. 9, 1056–1082. doi: 10.1038/nprot.2014.063
Schubert, M., Lindgreen, S., and Orlando, L. (2016). AdapterRemoval v2: Rapid adapter trimming, identification, and read merging. BMC Res. Notes 9:88. doi: 10.1186/s13104-016-1900-2
Schwartz, M. K., Luikart, G., and Waples, R. S. (2007). Genetic monitoring as a promising tool for conservation and management. Trends Ecol. Evol. 22, 25–33. doi: 10.1016/j.tree.2006.08.009
Smith, B. T., Harvey, M. G., Faircloth, B. C., Glenn, T. C., and Brumfield, R. T. (2014). Target Capture and Massively Parallel Sequencing of Ultraconserved Elements for Comparative Studies at Shallow Evolutionary Time Scales. Syst. Biol. 63, 83–95. doi: 10.1093/sysbio/syt061
Staats, M., Erkens, R. H. J., Vossenberg, B., van de Wieringa, J. J., Kraaijeveld, K., Stielow, B., et al. (2013). Genomic Treasure Troves: Complete Genome Sequencing of Herbarium and Insect Museum Specimens. PLoS One 8:e69189. doi: 10.1371/JOURNAL.PONE.0069189
Suarez, A., and Tsutsui, N. (2004). The Value of Museum Collections for Research and Society. Bioscience 54, 66–74. doi: 10.1641/0006-35682004054
Tsai, W. L. E., Schedl, M. E., Maley, J. M., and McCormack, J. E. (2020). More than skin and bones: Comparing extraction methods and alternative sources of DNA from avian museum specimens. Mol. Ecol. Resour. 20, 1220–1227. doi: 10.1111/1755-0998.13077
Tsangaras, K., and Greenwood, A. D. (2012). Museums and disease: Using tissue archive and museum samples to study pathogens. Ann. Anat. 194, 58–73. doi: 10.1016/j.aanat.2011.04.003
Vallender, E. J. (2011). Expanding whole exome resequencing into non-human primates. Genome Biol. 12:R87. doi: 10.1186/GB-2011-12-9-R87
van der Valk, T., Lona Durazo, F., Dalén, L., and Guschanski, K. (2017). Whole mitochondrial genome capture from faecal samples and museum-preserved specimens. Mol. Ecol. Resour. 17, e111–e121. doi: 10.1111/1755-0998.12699
van der Valk, T., Vezzi, F., Ormestad, M., Dalén, L., and Guschanski, K. (2020). Index hopping on the Illumina HiseqX platform and its consequences for ancient DNA studies. Mol. Ecol. Resour. 20, 1171–1181. doi: 10.1111/1755-0998.13009
von Seth, J., Dussex, N., Díez-del-Molino, D., van der Valk, T., Kutschera, V. E., Kierczak, M., et al. (2021). Genomic insights into the conservation status of the world’s last remaining Sumatran rhinoceros populations. Nat. Commun. 12:2393. doi: 10.1038/s41467-021-22386-8
Wandeler, P., Hoeck, P. E. A., and Keller, L. F. (2007). Back to the future: Museum specimens in population genetics. Trends Ecol. Evol. 22, 634–642. doi: 10.1016/j.tree.2007.08.017
Weiß, C. L., Schuenemann, V. J., Devos, J., Shirsekar, G., Reiter, E., Gould, B. A., et al. (2016). Temporal patterns of damage and decay kinetics of dna retrieved from plant herbarium specimens. R. Soc. Open Sci. 3:160239. doi: 10.1098/rsos.160239
White, L. C., Fontsere, C., Lizano, E., Hughes, D. A., Angedakin, S., Arandjelovic, M., et al. (2019). A roadmap for high-throughput sequencing studies of wild animal populations using noninvasive samples and hybridization capture. Mol. Ecol. Resour. 19, 609–622. doi: 10.1111/1755-0998.12993
Wisely, S. M., Maldonado, J. E., and Fleischer, R. C. (2004). A technique for sampling ancient DNA that minimizes damage to museum specimens. Conserv. Genet. 5, 105–107. doi: 10.1023/B:COGE.0000014061.04963.da
Keywords: endogenous DNA, historical DNA, museomics, natural history collections, SNP genotyping errors, target enrichment
Citation: Pacheco C, Lobo D, Silva P, Álvares F, García EJ, Castro D, Layna JF, López-Bao JV and Godinho R (2022) Assessing the performance of historical skins and bones for museomics using wolf specimens as a case study. Front. Ecol. Evol. 10:970249. doi: 10.3389/fevo.2022.970249
Received: 15 June 2022; Accepted: 11 August 2022;
Published: 08 September 2022.
Edited by:
Jimmy McGuire, University of California, Berkeley, United StatesCopyright © 2022 Pacheco, Lobo, Silva, Álvares, García, Castro, Layna, López-Bao and Godinho. This is an open-access article distributed under the terms of the Creative Commons Attribution License (CC BY). The use, distribution or reproduction in other forums is permitted, provided the original author(s) and the copyright owner(s) are credited and that the original publication in this journal is cited, in accordance with accepted academic practice. No use, distribution or reproduction is permitted which does not comply with these terms.
*Correspondence: Raquel Godinho, cmdvZGluaG9AY2liaW8udXAucHQ=
†These authors have contributed equally to this work and share first authorship
Disclaimer: All claims expressed in this article are solely those of the authors and do not necessarily represent those of their affiliated organizations, or those of the publisher, the editors and the reviewers. Any product that may be evaluated in this article or claim that may be made by its manufacturer is not guaranteed or endorsed by the publisher.
Research integrity at Frontiers
Learn more about the work of our research integrity team to safeguard the quality of each article we publish.