- 1Henan Key Laboratory of Germplasm Innovation and Utilization of Eco-economic Woody Plant, Pingdingshan University, Pingdingshan, China
- 2Key Laboratory for Value Realization of Ecological Products of Mountains-Rivers-Forests-Farmlands-Lakes-Grasslands in Pingdingshan City, Pingdingshan University, Pingdingshan, China
- 3Department of Human Resources Management, School of Business and Management, Jilin University, Changchun, China
- 4College of Horticulture and Plant Protection, Henan University of Science and Technology, Luoyang, China
- 5School of Soil and Water Conservation, Beijing Forestry University, Beijing, China
To evoke positive human emotions is a critical goal of blue spaces in urban wetland parks. However, information is still scarce on how people self-express across the spatiotemporal spectrum when they come across wetlands which include varying levels of elevation in a single landscape and microclimate. In this study, 30 urban wetland parks were selected from 17 cities in Central China, where a total of 1,184 portrait photos of visitors were obtained from a social media platform (Sina Weibo) to analyze their expressed sentiments by rating facial expression scores of happy and sad emotions and net positive emotion index (NPE; happy-score minus sad-score) in 2020. Landscape metrics were remotely evaluated for every wetland park, and microclimatic factors were obtained for the days when the photos were taken. Based on regressions of park-level data, blue-space areas could be perceived as a positive driver to trigger happiness in spring (regression coefficient [RC] of 0.20), but it triggered negative emotions in autumn (RC of −2.98). The higher elevation areas triggered positive emotions in summer and autumn (RC of 1.35 × 10−3), but extreme daily temperature, air humidity, and wind velocity together triggered sadness (RC of 0.11, 0.03, and 0.51, respectively). Mapped distribution of the area and corresponding emotions showed that visiting blue space evoked more smiles in wetland parks of northern Hunan, southern Hubei, and eastern Anhui in spring. Blue spaces in Shanxi and northwestern Hebei evoked better moods in autumn. Smaller blue spaces in wetlands located at higher elevations were recommended for nature enthusiasts in warm seasons to overcome the prevalent sadness characteristic of that time of the year and location.
Introduction
Wetlands have many important ecosystem uses, such as sequestering carbon and biodiversity conservation (Hansson et al., 2005; Han et al., 2012). Urban wetlands are special types of ecological infrastructures (EIs) that attract the public due to their unique function across terrestrial and aquatic ecosystems (Childers et al., 2019). Biodiversity, greenery, and microclimates together account for a synthesized trigger that makes people perceive a sense of mental restoration and wellbeing in urban wetlands (Fuller et al., 2007; Dallimer et al., 2012; Carrus et al., 2015; Wei et al., 2020b, 2021a,b). In the UN Agenda 2030 for sustainable development, the benefits of wetlands are projected to contribute to achieving specific sustainable development goal (SDG) targets such as “basic human needs”, “sustainable tourism”, and “improving and conserving the environment” (Jaramillo et al., 2019). However, wetland ecosystems and the benefits accrued from them are being reduced to an extent that they may not be able to help achieve these SDG targets (Darrah et al., 2019; Jaramillo et al., 2019; Ishtiaque et al., 2020). Global reserves of natural wetlands have declined on an average by 35% in the past four decades (Darrah et al., 2019) due to ecosystem degradation, soil erosion, drainage, and mostly, urbanization (Gunderson et al., 1995; Ishtiaque et al., 2020). As a nature-based solution (NBS), however, human-made urban wetlands have increased by 233% during the same period (Darrah et al., 2019). Given that over four billion people are living in cities that are full of stressors (WHO, 2022), more conceptual frameworks are needed to guide the planning of wet-landscapes in urban centers to improve human wellbeing.
Urban blue space is a critical element of a city's environmental infrastructure, where wetlands account for a large proportion (Tuofu et al., 2021). People can perceive landscape metrics in a blue space as drivers to evoke positive emotions and post them online (Akpinar, 2016; Wei et al., 2019; Zhang et al., 2021; Li et al., 2022a,b; Yu et al., 2022) (Table 1). In coastal urban wetlands, it was reported that the proximity to a blue space was a key precondition to evoking positive self-affirmation in people (White et al., 2016; Freeman et al., 2022). Generally, a distance of 1 km to 5 km to a blue space in urban wetlands had an impact on a person's emotions (de Vries et al., 2003; White et al., 2014). However, a distance closer than 1 km from a wetland park failed to emulate a similar feeling as it lacked an association with the perceived mental wellbeing (Triguero-Mas et al., 2015). Studies of this type used data from self-reported scores on questionnaires, which may suffer from human errors that cause more fluctuations than expected (Wei et al., 2022). The WHO suggests that perceived mental states reported by participants (in questionnaires) are seldom reliable (WHO, 1994). Meanwhile, recent studies also suggest that psychological responses to experiences in wet landscapes can be assessed through big data of posted emotions on social media networks (Zhang et al., 2021; Li et al., 2022a). The higher prevalence of blue spaces in urban wetland parks in coastal cities can be presumed to promote positive emotions in these places (Li et al., 2022a). On the other hand, it has been reported that coming across blue spaces in a wetland located in an urban forest park in temperate cities evoked sad emotions (Zhang et al., 2021). Furthermore, blue spaces in elevated wetlands induced more sadness in people (Zhang et al., 2021; Li et al., 2022a). However, these studies were undertaken in parks at the local level, and a study of perceived emotions in blue spaces among a larger regional distribution may be different from areas covered just by parks. Remote sensing-based research has been a major advancement in assessing the function of wetlands while monitoring the progress of SDGs related to human interactions (Ishtiaque et al., 2020). Using the remote-sensing approach, people's perceptions of their experiences in parks can be extended to other places by predicting values across a regional area. To the best of our knowledge, the prediction of the perceived emotions of people in blue spaces of wetlands has not been mapped at the regional level.
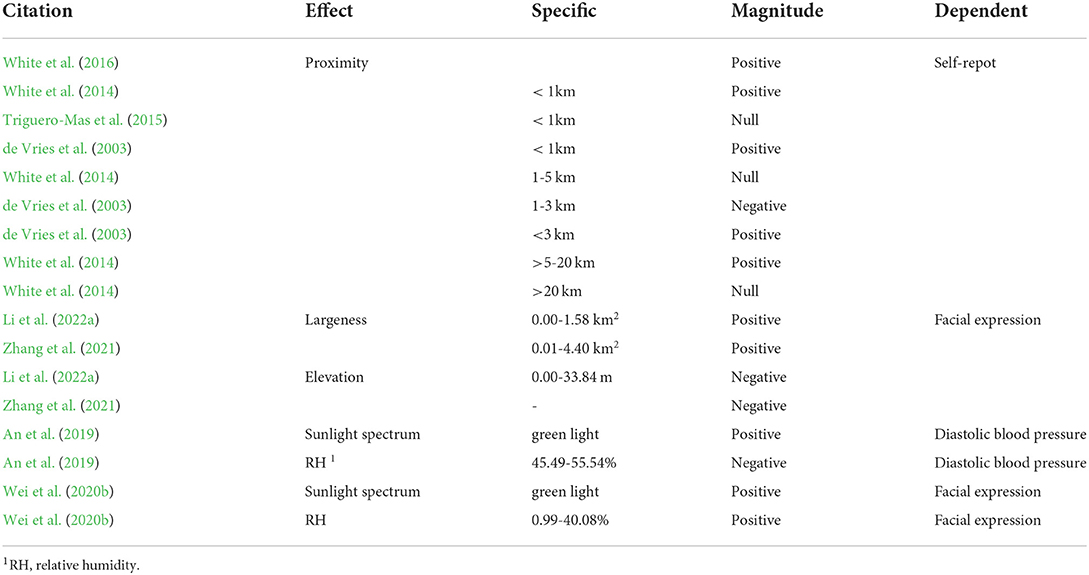
Table 1. Summary of emotional and psychological responses to experiences in blue spaces with varied metrics.
The microclimate within EIs plays a significant role. Wetlands comprise green and blue spaces in a synthesis of terrestrial and aquatic environments (Gascon et al., 2015; Childers et al., 2019; Zhang et al., 2021; Li et al., 2022a) (Table 1). Transpiration in the vegetation can mitigate the heat-island effect and induce thermal comforts (Abd Latif et al., 2012; Klemm et al., 2015; Liu et al., 2021c; Priya and Senthil, 2021). The intensity of sunlight that is transmitted and understory RH (~53.29%) are factors that enhance the physiological and psychological wellbeing of people (An et al., 2019; Wei et al., 2020b). Mild winds at a moderate velocity (~5.36 m s−1) in green spaces can evoke a sense of better mood (Mao et al., 2022). Large-scale water bodies in blue spaces of wetlands can prevent thermal evaporation and replace it with cold gases (Nipoti and Binney, 2007). Temperatures in the range of 17.5–22.3°C are considered optimum to induce people to exhibit positive emotions (Li et al., 2022b). The experiences in wetlands during plant-growing seasons can improve human comfort through its cooling effect (Zhang et al., 2016; Wong et al., 2017). However, if the duration is extended beyond the season to a year, the moist environment fails to evoke the same reaction (Li et al., 2022b). Again, these findings of meteorological effects on people's perceptions were obtained from wetlands in single parks at scattered locations. Observations of the emotional impacts of wetland microclimates at a larger scale are still lacking. In addition, knowledge about the combined effects of landscapes and microclimates on the mental status of people is lacking and has not attracted enough attention. The use of remote sensing and monitoring can facilitate mapping regional landscapes and their function for further analysis.
The achievement of environmental-related SDG targets depends on decisions made to secure the resources and manage wetlands in a sustainable manner (Weise et al., 2020). Mapping the water-related ecosystem is a fundamental requirement for managing landscapes in a large scale (Fitoka et al., 2020). Landscape metrics are directly identifiable from surface features on the earth that reflect light in different spectral bands making it easy to map and assess their spatial distribution. Wetlands, on the other hand, are not direct metrics but contribute to a critical part of the management (Rapinel et al., 2016). Determining the relationship between landscape metrics and their function is an available approach to predicting the outcome of the interaction using remotely monitored parameters in a group of single parks (Liu et al., 2021a; Zhang et al., 2021; Li et al., 2022b). The regressed models can be further used to predict functional services for people coming across blue spaces in regional wetlands. To the best of our knowledge, the wetland function of promoting people's mental wellbeing has not been fully mapped at a regional scale. This might be due to the lack of predictions of mental states regressed against landscape metrics remotely evaluated at the regional scale.
The use of facial expressions posted on social media has been established in studies detecting mental wellbeing in urban green and blue spaces (Wei et al., 2019; Liu et al., 2021c; Mao et al., 2022). This approach has the merit of using online big data from social media networks that can process the variety of expressions expressed by people while at the same time managing human errors in the process (Wei et al., 2020a; Evers and Phoenix, 2022). Conventional assessments depending on questionnaires are more likely to suffer bias from human errors when used for evaluating mental status (Yang et al., 2018). On the other hand, facial expressions are closer to the reflections of true sentiments of people who have posted them online (Sun et al., 2019, 2021). However, the collection of facial expressions largely depends on the check-in location records of visitors in EIs. This may not be a challenge when collecting records from a single park where people are prone to visit and likely to post selfies. However, when collating spatially distributed facial expressions from local parks, especially when extending it to a regional scale with several wetlands, the geographical gaps in the data, due to a lack in the desired number of visits in a given time, need to be filled up by predicted values which had been regressed against landscape metrics in single parks. As far as we are aware, only a few studies have focused on this issue and made a preliminary attempt at mapping facial expressions (Yu et al., 2022). However, the results have been far from satisfactory given the limitations of small geographical scale and insufficient numbers of parks and landscape metrics used during regression analysis compared to landscape metrics in single parks.
Constructed wetlands are major urban catchments that mitigate flood events (Kumar et al., 2021). Inland cities located on plain terrains are more vulnerable to floods than those in coastal cities (Shin et al., 2019; Koyama and Yamada, 2020). This is because, following an extreme rainstorm, water flows with a lower probability to arrive at the closest catchment area of inland cities where watersheds are generally smaller compared to those along the shore. Many regions in Central China have been adapted to facilitate the diversification and production of agricultural crops (Zeng et al., 2020). However, these areas are at a high risk of flooding given more frequent rainstorms due to climate change (Huo et al., 2013; Kong and Iop, 2019). For example, in July 2021, Zhengzhou had a rainstorm with 617 mm of rain over a 3-day period which led to disastrous flooding (China Today, 2021). Constructing more wetlands is an urgent necessity to cope with further flooding risk in this area, but equally missing is an understanding of the different perceptions of people when exposed to different wetlands. This gains importance against the background of SDG targets relating to human wellbeing, including mental wellbeing. Mapping the spatial distribution of emotional perceptions can provide a theoretical framework to guide the sustainable management of blue-space landscapes and waterbodies, which are urgently needed in the context of flooding in Central China.
In this study, facial expressions that were posted online were rated for visitors' perceptions of urban wetlands in cities in Central China. The spatial distribution was mapped according to daily microclimatic records and seasonal variations. Landscape metrics of blue spaces in wetlands were characterized as independent variables to regress facial expressions. Predicted facial expressions were extended to all blue spaces in the whole study area according to regressed dependences of joint landscape and microclimates. We aimed to map the spatiotemporal distributions of emotional perceptions of people experiencing wetlands and their associations with landscape metrics and microclimates. We hypothesized that: (i) at the regional scale, people will pose more positive emotions in wetland blue spaces with a large area and low elevation based on current findings in cities of East China (Zhang et al., 2021; Li et al., 2022a), and (ii) a combination of high temperature and low RH microclimates evoke more smiles in online photos as surmised by the findings related to experiences in urban green spaces of North China (Zhang et al., 2021; Li et al., 2022b; Mao et al., 2022) and blue spaces of coastal cities of East China (Li et al., 2022b). Our results will contribute to a significant and novel approach that predicts and maps visitors' perceptions of urban blue spaces at a regional scale utilizing the independent data of joint landscape metrics and microclimates.
Materials and methods
Study area and regional characteristics
In this study, undertaken in 2020, a total of 30 wetlands from urban parks in 17 cities of Central China were included as study sites (Figure 1; Table 2). The parks were chosen through a basic requirement that no fewer than 40 visitors per park had to post their photos (with facial expressions) on Sina Weibo during the study period (Sina Weibo, 2021) (alternatively, Sina Micro-blog or China Twitter) (Liu et al., 2021c; Zhu et al., 2021). Most parks were constructed in cities on plain terrains. According to The Yearbook of China's Cities (2020 edition) (Hong, 2020), the chosen cities had a population ranging from 78.20 × 104 (Zhangjiajie) to 668.10 × 104 (Wuhan), with a built-up area of 37.00 km2 (Xinzhou) to 2,661.93 km2 (Handan), and per capita GDP from 3.16 × 104 yuan per person (Xinzhou) to 15.68 × 104 yuan per person (Changsha). The study area had a continental monsoon climate across temperate and subtropical climatic zones. The local climate was characterized by a yearly rainfall of 6.61 mm, RH of 59.06%, an average daily temperature of 15.80°C, AveW of 3.39 m s−1, and sunshine duration of 6.80 h (National Standard of China, 2017).
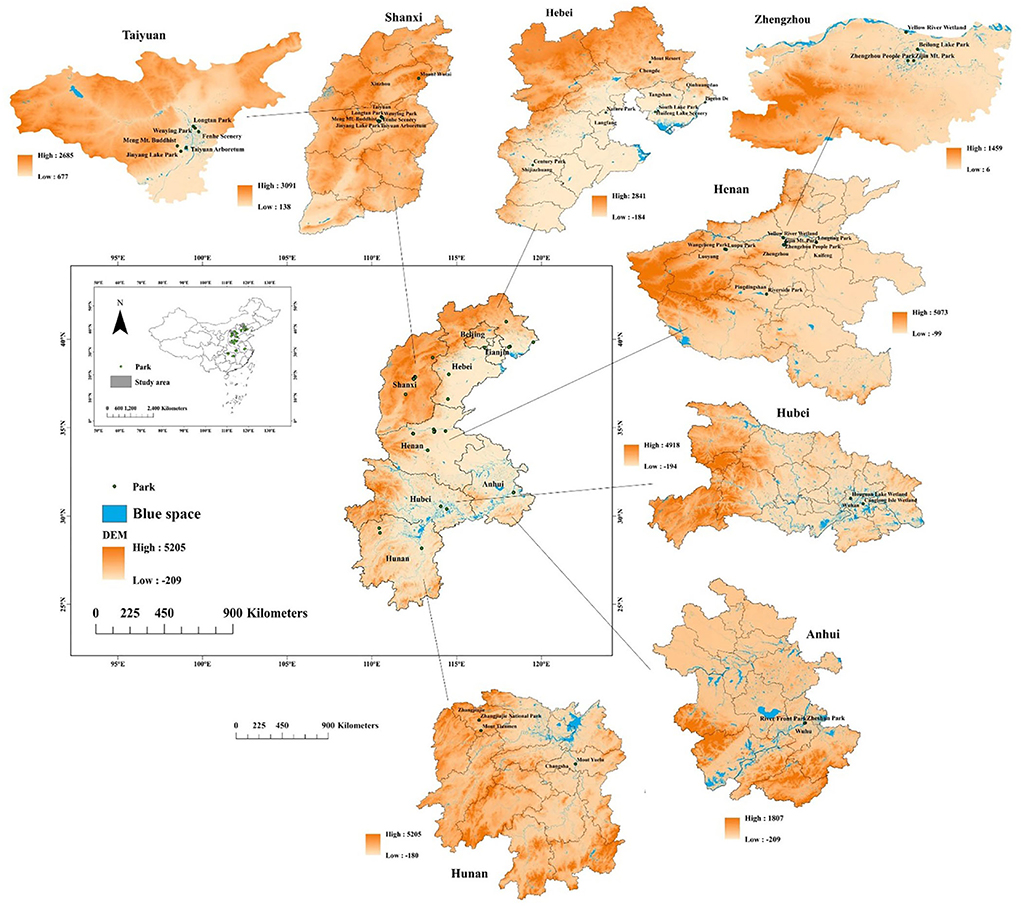
Figure 1. Spatial distribution of urban wetland parks for data collection in cities of Central China.
Experimental design and study layout
The processes of the experiment design and layout are shown in Figure 2. We employed parks as places (Table 2) from where data were collected about posted facial expressions, landscape metrics, and regional microclimates. All data were mapped to reveal their spatial distributions with scattered park locations. Facial expression scores were regressed against the landscape and climate data and regression models were established using park-level data. Using remote-sensing techniques, regional blue spaces were mapped in all regions of the study area, and respective climatic conditions were obtained using data from the nearest meteorological stations. Facial expression scores were then regressed against joint landscape metrics and climates. Thereafter, spatial distributions of independent variables (landscape and climate) with significant influence on facial expression scores were used as sources of data; thereafter facial expressions could be predicted and mapped in all blue spaces based on mapping these independent variables. Therefore, results would also reveal blue spaces that individuals may have not visited in local wetland ecosystems but might evoke similar positive emotions.
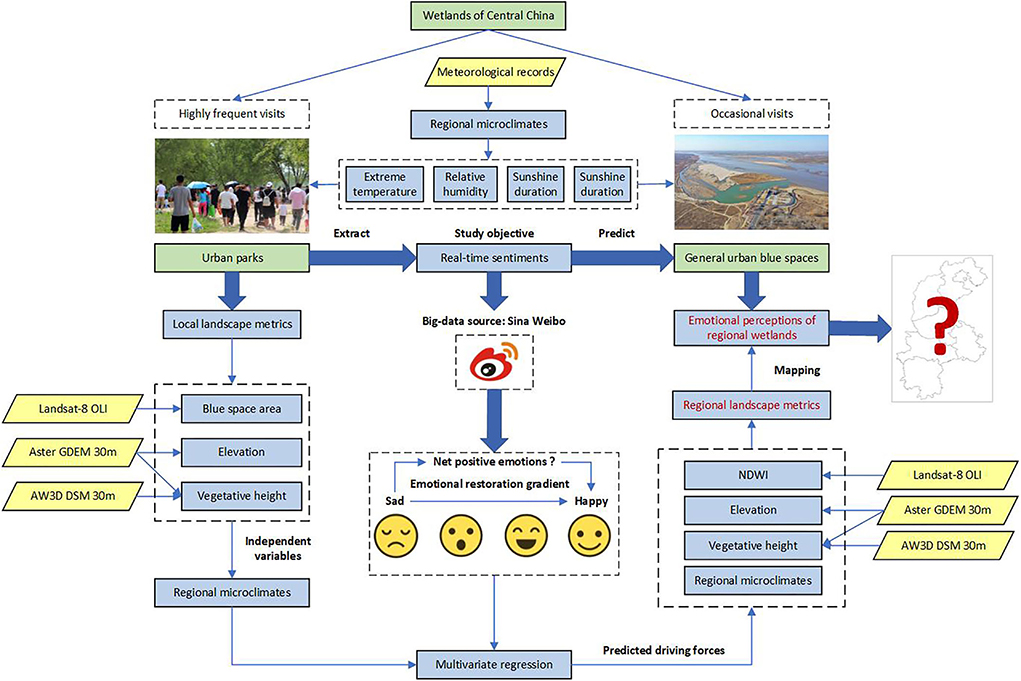
Figure 2. The layout of the experiment design and study arrangement in data collection, statistical analysis, spatial characterization, and geographical interpolation.
Landscape metrics and regional climates
Landsat-8 OLI (30-m resolution) was used as the source of the geographical data (USGS, 2021). Satellite images were obtained from plant growing seasons in May-August 2020 for days with the coverage rate of clouds lower than 10%. The geographical range of every park was outlined through manual and digital mapping of locations in Baidu maps as references (Baidu Map, 2022). Blue space was remotely evaluated by normalized difference water index (NDWI), which was assessed according to the method using spectral bands from Landsat-8 OLI (McFeeters, 2013):
where, Green is the green light reflectance (band 3) and NIR is the near-infrared red light reflectance (band 5) (Guha et al., 2020). Regions with NDWI values in a range of 0–1 were extracted from wetlands in parks and areas of blue spaces were calculated as the surface area using ArcGIS v. 10.2 (Esri-China, Shanghai, China). The elevation of every wetland was evaluated by digital elevation model (DEM) data using the ASTER GDEM 30 m map-data product (NASA EarthData, 2021). Vegetative height of plant communities in wetlands was evaluated by the difference between digital surface model (DSM) data and DEM data in the geographical ranges of wetlands (Schirpke et al., 2013). DSM data were obtained from the AW3D30 DSM map (Japan Aerospace Exploration Agency, 2021).
Microclimates were determined from meteorological factors of daily rainfall, extreme temperature (MaxT and MinT), average RH, SunH (National Standard of China, 2017), and AveW from records of the closest meteorological stations of the identified parks (Climatic Data Centre, 2021). Meteorological stations that supplied climatic records had to be located with adjacent wetland parks in the same city. If no meteorological station was established in the same city, climatic records were obtained from adjacent meteorological stations in neighboring cities at a distance <10 km from the identified wetland park. Meteorological factors were obtained from daily records when wetlands in the identified parks were visited by individuals who shared their selfies or photos with their faces on Sina Weibo and locationally “checked in” at the identified parks. Microclimates gathered over a timeframe of a year were divided into four seasonal periods spring (from March to May) (Xu et al., 2021), summer (from June to August) (Yu et al., 2020), autumn (from September to November) (Sui et al., 2013), and winter (from December to following February).
Photos of faces and rating expressions
Photos with facial expressions were obtained from micro-blogs during the time of 2020 with check-in locations at the wetlands listed in Table 2. The selfies were uploaded to Sina Weibo by visitors, and their numbers had to be at least 40 individuals per park. This was the basic requirement of the number of photos that may be needed for a study to rate facial expressions among different locations (Li et al., 2022b; Mao et al., 2022; Wei et al., 2022; Yu et al., 2022). To facilitate further refinement to determine facial expressions from photos, we required the portrait of just one person in each photo, and photos that contained more than one person was cropped. The facial area of a photographed person had to be exposed without any covers, e.g., hat-edge, hair, sunglass, or digital decoration. Screened photos were rotated to make the nose vertical to the horizontal line. This was a basic requirement that secured the desired accuracy for the facial recognition (Guan et al., 2021; Wei et al., 2021b). Processed photos were saved in local hardware and uploaded to the instrument for analysis. Photos were processed for facial expressions and their scores were rated by FireFACE v. 1.0 software (Zhilunpudao Agric. S&T Inc., Changchun, China). Photos were uploaded to the software by tagging them under relevant categories so that they could be recognized by the machine by selecting the required category. While most photos could thus be tagged and categorized for analysis, there were some photos where the facial expression could not be accurately categorized and therefore the final number of selfies per park that could be used for analysis may be fewer than 40 (Table 2). Scores of happy and sad emotions were rated in percentages of people visiting selected wetlands; therefore, the NPE (in percentage) could be calculated as the difference between happy scores minus sad scores (Wei et al., 2021a,b). The parameter of NPE was initially used for evaluating the taste of salts (Kerrihard et al., 2017) and later adapted to studies on the emotional perceptions of people exposed to urban green spaces (Wei et al., 2019; Yu et al., 2022).
Statistical analysis and mapping
Facial expression scores and microclimatic factors were mapped along with their seasonal variations across four seasons in 2020. Data were transformed to logarithms to facilitate ANOVA and Pearson correlation (Zhang et al., 2021; Mao et al., 2022). SAS software (ver. 9.4) (SAS Statistics Inc., New York, USA) was used as the instrument for data analysis. Seasonal variations of facial expressions and microclimates were analyzed by two-way ANOVA using mixed models to compare wetlands in repeated measures across the four seasons (Wei et al., 2020b). Duncan test was used to detect significant differences in parameters among locations with uneven numbers of replicated photos per wetland (Guan et al., 2021; Liu et al., 2021c; Mao et al., 2022; Yu et al., 2022). Landscape metrics were mapped in the study area and analyzed by Pearson correlations with microclimates. An arc diagram was prepared to reveal relationships between pairs of landscape and microclimatic parameters. Multivariable linear regression was used to predict facial expression scores against landscape metrics and seasonal microclimates. The regressed results were further used to predict potential facial expressions on the projected area of wetland blue spaces that were recognized by NDWI values.
Results
Spatial distribution of facial expression scores among parks
The happy scores showed a significant difference among parks principally during spring, compared to the other three seasons (Table 3). In spring, the happy score was generally higher in northern regions (Hebei and Shanxi) than in the south (Anhui and Hunan) (Figure 3A). For example, spring happy scores in South Lake Park of Tangshan (79.44 ± 29.45%) and Fenhe Scenery of Taiyuan (69.31 ± 39.24%) were higher than those in River Front Park of Wuhu (5.11 ± 8.68%) and Mount Yuelu of Changsha (3.27 ± 3.47%). In summer and winter, happy scores tended to be higher in regions near the boundary, but in autumn happy scores turned out to be alternatively high and low across the study area (Figures 3B–D).
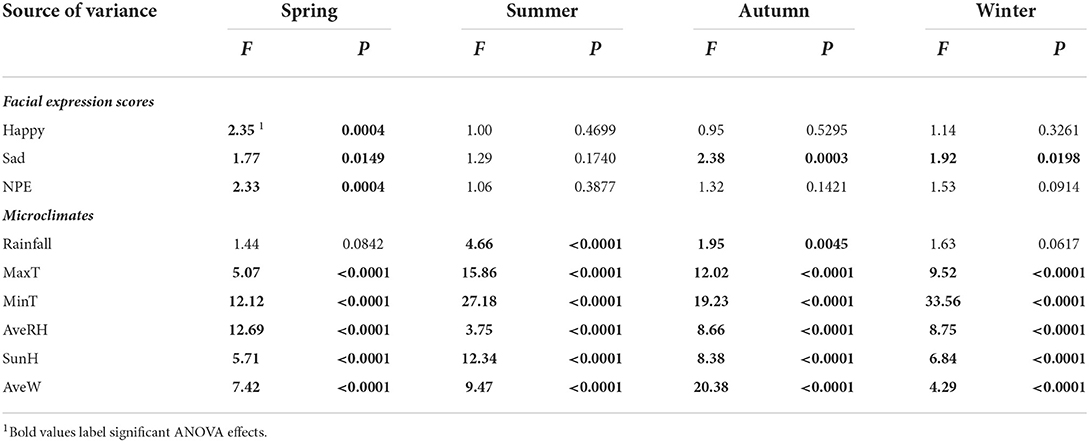
Table 3. ANOVA of different park variations on facial expression scores and microclimates in urban wetlands in cities of China.
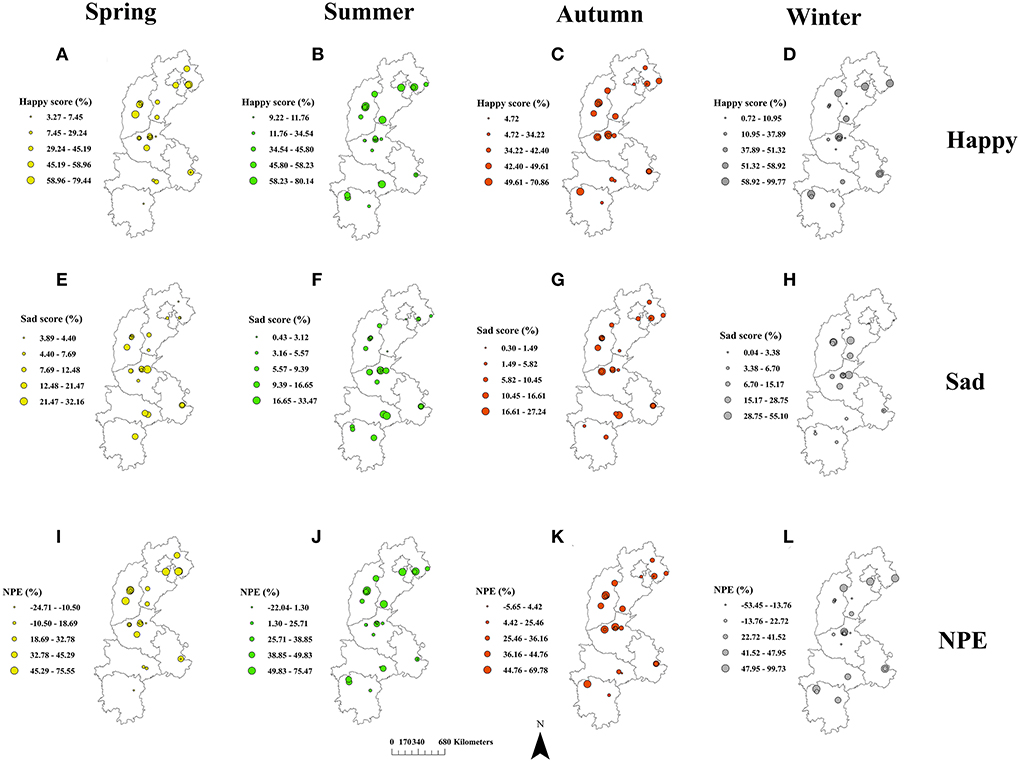
Figure 3. Spatial distributions of facial expression scores (%) for happy (A–D) and sad (E–H) emotions and net positive emotion index (NPE) (I–L) of visitors in urban wetland parks in cities of Central China across four seasons of spring, summer, autumn, and winter of 2020.
Spatial variation had a significant effect on sad scores in most seasons of the year except in summer (Table 3). In spring, more parks attracted people with higher sad scores in the south than in the north (Figure 3E). People showed higher sad scores in Longting Park of Kaifeng (32.16 ± 11.99%) and Canglong Isle Wetland of Wuhan (21.47 ± 18.04%) compared to Mount Resort of Chengde (4.37 ± 6.75%) and Fenhe Scenery of Taiyuan (4.40 ± 5.50%). Summer sad score continued the distribution pattern with higher scores in the southern areas but the difference between park locations was not statistically different (Figure 3F). In autumn, parks with high and low sad scores were distributed in a more heterogeneous pattern, but, again, the Canglong Isle Wetland in the south (27.24 ± 20.60%) showed higher sad scores than that in Mount Resort (7.98 ± 8.08%), Huifeng Lake Scenery (0.30 ± 0.00%), Longtan Park (1.49 ± 1.63%), and Wenying Park (1.09 ± 2.64%) in the south (Figure 3G). In winter, high sad scores were concentrated in the central part of the study area (Figure 3H). Sad scores in winter were higher in Longting Park of Kaifeng (55.10 ± 0.00%) relative to that in Pigeon Dove Park of Qinhuangdao (0.28 ± 0.00%) and Mount Yuelu of Changsha (4.94 ± 5.20%).
The net positive emotion index (NPE) also showed a significant response to spatial distribution but was restricted only to the spring period of the year (Table 3). In contrast to results relating to sad score distribution, spring NPE tended to be higher in southern regions than in the north (Figure 3I). For example, NPE was higher in South Lake Park of Tangshan (75.55 ± 35.56%) compared to Longting Park of Kaifeng (−24.71 ± 18.13%), River Front Park of Wuhu (−12.29 ± 26.14%), and Mount Yuelu of Changsha (−10.50 ± 8.07%). In summer, autumn, and winter, NPE showed heterogeneous distribution patterns with alternative high and low values in scattered regions of the study area (Figures 3J–L).
Spatial distribution of microclimates among parks
Rainfall showed a significant spatial distribution among parks in summer and autumn (Table 3). Summer rainfall was higher in regions along the central parts of the study area compared to regions near the boundary. For example, rainfall was higher in Mount Wutai of Xinzhou (17.62 ± 14.75 mm) than in Mount Resort of Chengde (1.46 ± 1.49 mm) and Mount Yuelu of Changsha (6.03 ± 10.82 mm). The autumn rainfall was higher in Wenying Park of Taiyuan (3.71 ± 5.92 mm) compared to the wetland parks of Hebei, e.g., in Pigeon Dove Park of Qinhuangdao (0.07 ± 0.22 mm). Rainfall in spring ranged from 0.00–5.30 mm and 0.00–3.40 mm in winter (Figures 4A,D).
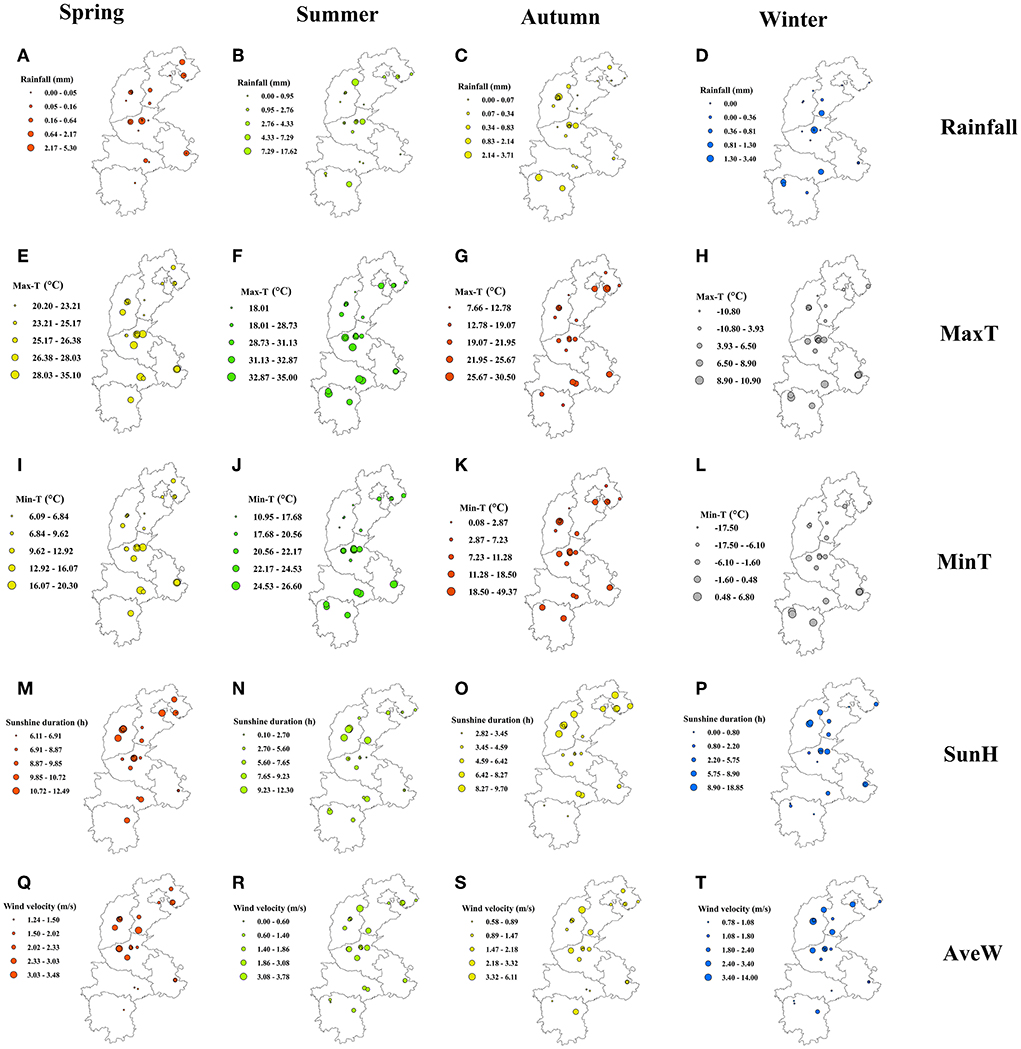
Figure 4. Spatial distributions of microclimates on days when Sina Weibo users visited wetland parks and posted their facial photos in cities of Central China across four seasons of spring, summer, autumn, and winter of 2020. Microclimatic factors: rainfall, (A–D); maximum daily temperature (MaxT), (E–H); minimum daily temperature (MinT), (I–L); sunshine duration (SunH), (M–P); average wind velocity every 2 min (AveW), (Q–T).
Rainfall was generally higher in wetlands in southern cities than in northern ones (Figure 3B). Rainfall in wetland parks in Beijing city ranged between 0.06–0.07 mm, which was lower than in Jiulong lake wetland park in Nanchang city (8.62 mm) and Huayang lake wetland park in Dongguan city (10.97 mm) (Table 4). However, dissimilar rainfall records were also observed in regionally adjacent wetland parks (Figure 3B). For example, in Nanchang city, rainfall in the Jiulong lake wetland park (8.62 mm) was higher than in Aixi lake park (0.76 mm) (Table 4). Similarly, in Dongguan city, rainfall in Huayang lake was higher than in Daguan (0.76 mm) and Nansha (4.76 mm) wetland parks in Guangzhou city.
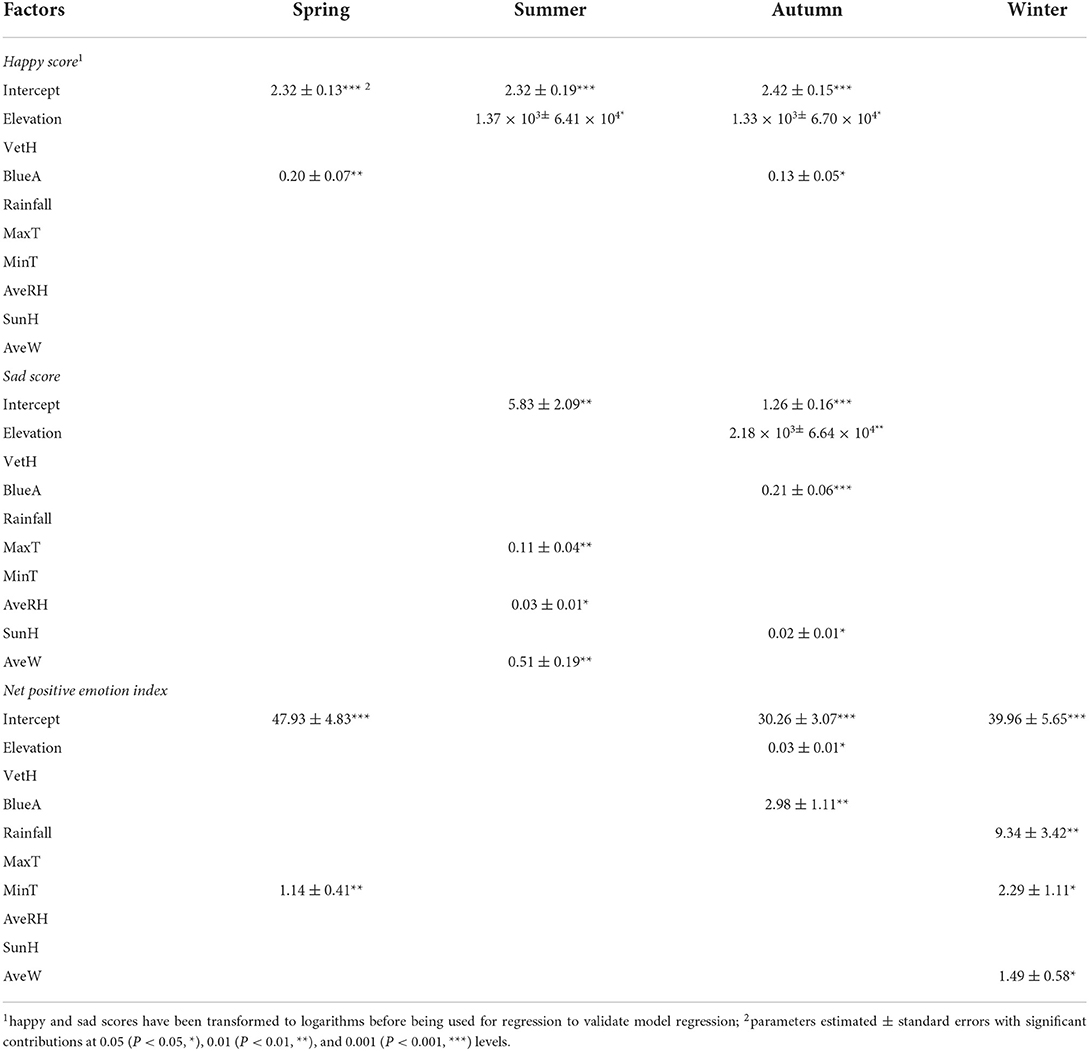
Table 4. Parameter estimates from multivariable linear regressions of factors of landscape metrics and microclimates on facial expression scores of people experiencing urban wetland parks across four seasons.
Both maximum daily temperature (MaxT) and minimum daily temperature (MinT) significantly varied between different park locations (Table 3). In spring, both MaxT and MinT were higher in the southern parts than in the north (Figures 4E,I). For example, in spring Zhengzhou People Park of Zhengzhou had higher MaxT (35.10 ± 0.00°C) and MinT (19.50 ± 0.00°C) than in parks in Hebei and Shanxi. In summer, Mount Tianmen of Zhangjiajie showed higher MaxT (35.00 ± 0.00°C) compared to parks in the north, and Zheshan Park of Wuhan showed higher MinT (26.60 ± 0.00°C) (Figures 4F,J). In autumn, more parks in the north showed temperature records to similar extents as those in the south (Figures 4G,K). In winter, MaxT and MinT were higher in Canglong Isle Wetland of Wuhan (10.9 ± 0.20°C) and Mount Yuelu of Changsha (6.80 ± 0.87°C) compared to the northern parks, respectively (Figures 4H,L).
Sunshine duration (SunH) was distributed at different levels in different parks across the four seasons (Table 3). More parks in the north had longer SunH values than in the south (Figures 4M–P). In spring, SunH was longer in Wenying Park (12.49 ± 0.74h) than in the parks of Anhui and Hubei (Figure 4M). Summer SunH was longer in Pigeon Dove Park (5.01 ± 2.59h) than in the parks of Henan, Anhui, Hubei, and Hunan (Figure 4N). In autumn, SunH was generally longer in Hebei and Shanxi parks compared to Henan and Hunan parks (Figure 4O). Similarly, winter SunH was longer in regions of Shanxi, Hebei, and Henan (Figure 4P). AveW was mostly higher in parks around the central part of the study area, i.e., in Hebei and Shanxi compared to other regions, especially in the southern regions of Hubei and Hunan (Figures 4Q–T).
Spatial distribution of landscape metrics among parks
The parks in northern Hebei, central Shanxi, and northern Hunan were at a higher elevation level (Figure 5A). The total area of blue spaces was larger in parks near Tianjin, central Shanxi, and northern Henan (Figure 5B). Vegetative height was generally higher in parks in western regions than in the east (Figure 5C).
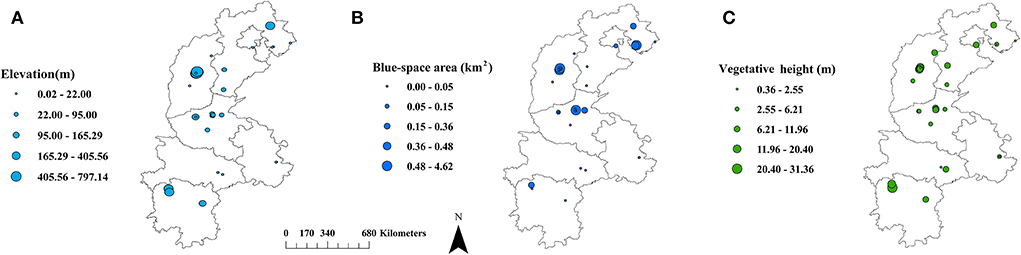
Figure 5. Spatial distributions of landscape metrics in elevation (A), blue-space area (B), and vegetative height (C) for urban wetland parks in Central China.
Elevation had a positive relationship with rainfall, which further showed a positive relationship with AveW (Figure 6). Elevation also had a negative relationship with MinT, which was positively correlated with MaxT. The total area of blue space had a negative relationship with AveRH, which further showed negative relationships with SunH and AveW. Vegetative height had negative relationships with MaxT and MinT, which both further showed negative relationships with AveW.
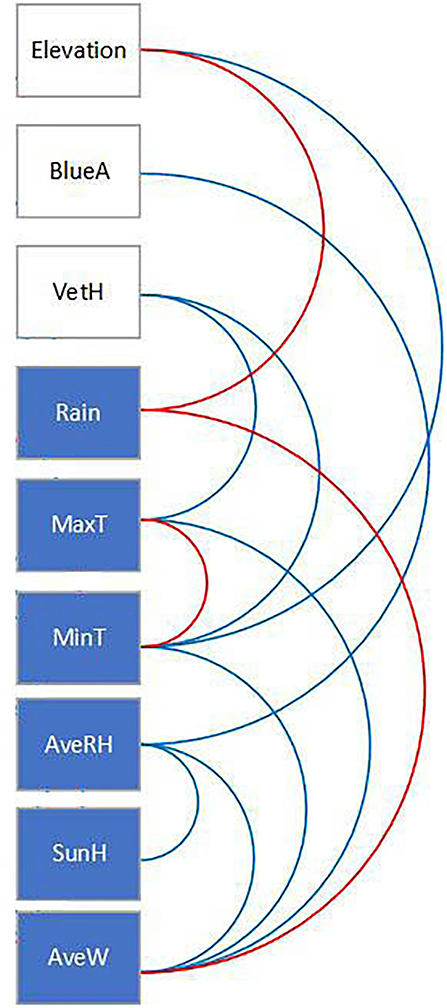
Figure 6. Arc-diagram of correlations between parameters about landscape metrics and microclimatic factors. Lines in red link two parameters that had a positive relationship and those in blue link two parameters with a negative relationship. BlueA, blue-space area; VegH, vegetative height; Rain, rainfall; MaxT, maximum daily temperature; MinT, minimum daily temperature; AveRH, average RH; SunH, sunshine duration; AveW, average wind velocity every 2 min.
Regression of facial expression against landscape metrics and microclimatic factors
Happy scores were regressed in most seasons of the year, except for winter (Table 4). The total area of blue spaces had a contribution (estimated parameter of 0.20) to happy scores in spring, but elevation levels had a smaller contribution (estimated parameter of 1.37 × 10−3) to happy scores in summer. In autumn, both elevation and area of blue-space showed significant contributions (estimated parameters of 1.33 × 10−3 and −0.13, respectively) to happy score but just from blue-space areas alone, it was negative and weak (estimated parameter of −0.13).
Sad scores were regressed only in summer and autumn (Table 4). The MaxT, AveRH, and AveW together showed positive contributions (estimated parameters of 0.11, 0.03, and 0.51, respectively) to sad scores in summer. In autumn, blue-space areas induced a small positive contribution (estimated parameter of 0.21) to sad scores, while contributions from elevation and SunH were negative (estimated parameters of −2.18 × 10−3 and −0.02, respectively).
The net positive emotion index regressed in spring, autumn, and winter (Table 4). In spring, MinT showed a negative contribution (estimated parameter of −1.14) to NPE. In autumn, elevation had a small positive contribution (estimated parameter of 0.03) to NPE, but blue-space areas showed a strong negative contribution (estimated parameter of −2.98). In winter, the contribution of MinT to NPE was reversed to be positive (estimated parameter of 2.29), while contributions from rainfall and AveW were negative (estimated parameters of −9.34 and −1.49, respectively).
Prediction of emotional responses to potential experiences in blue spaces
Based on the regression results presented in Table 4, experiences in blue spaces were interpolated in spring, summer, and autumn (Figures 7A–C). In spring, it is predicted that visiting blue spaces can evoke happy emotions in four regions. The first, in order of level of happiness, is predicted in regions of Hebei near Beijing; the second is predicted in common regions across Shanxi and Henan; the third is in eastern Anhui, and the last along the large area from north Hunan to southern Hubei (Figure 7A). In summer and autumn, visits to blue spaces in the western regions of the study area are predicted to evoke happy emotions, which continued in connected blue spaces from northern Hebei to western Hunan (Figures 7B,C).
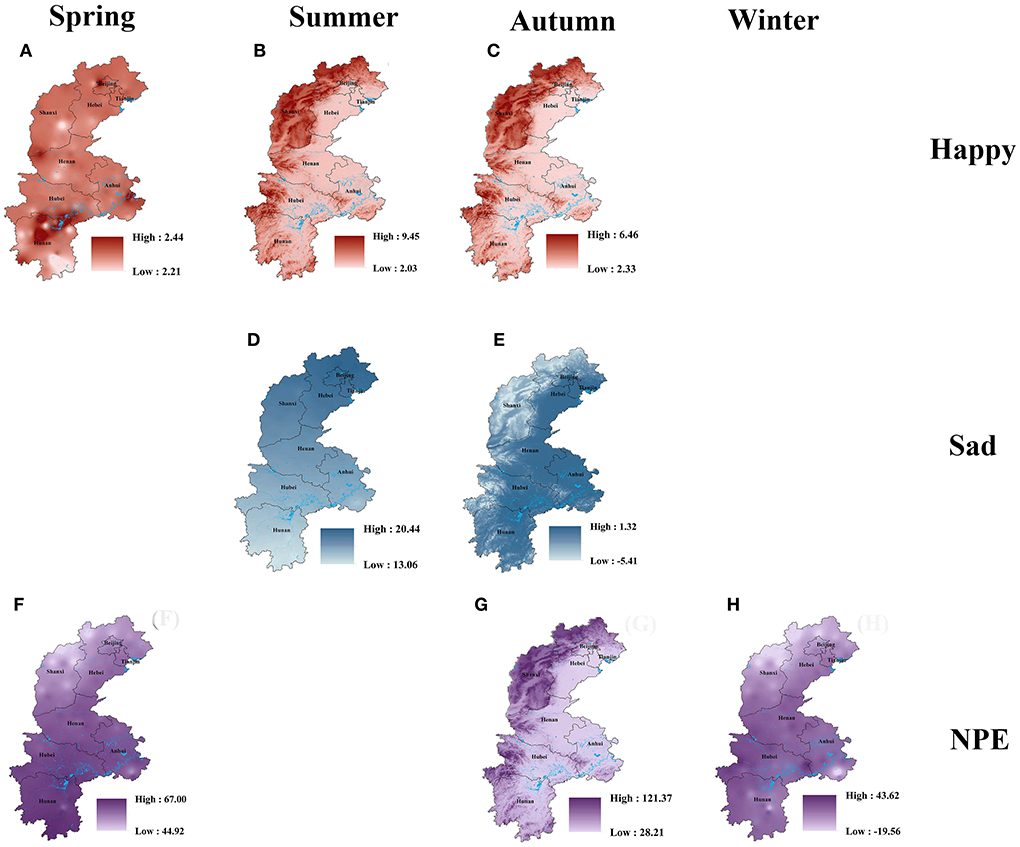
Figure 7. Seasonal distributions of predicted facial expression scores for happy and sad emotions and net positive emotion index (NPE) from potential experiences of visitors in blue spaces in regions of Central China. Regions in the blue color mark blue spaces that have regressed characteristics in landscape and microclimate from selected urban wetland parks. Regional distributions are interpolated by regression results adapted from Table 4. (A–C) Predicted happy scores in spring, summer, and autumn, respectively; (D,E) Predicted sad scores in summer and autumn, respectively; (F–H) Predicted NPE in spring, autumn, and winter, respectively.
The sad scores could be predicted in summer and autumn (Figures 7D,E). In summer, it was predicted that visiting blue spaces in the northern parts of the study area would induce sad emotions (Figure 7D), while in autumn, blue spaces in most regions of the study area were predicted to evoke sad emotions except for visitors who visited blue spaces in Shanxi, western and northern Hebei, and western regions of the other provinces (Figure 7E).
In spring, NPE was predicted to be higher in the southern parts of the study area, especially in the wetlands of Hunan, Hubei, and Anhui (Figure 7F). No regression was found in summer, but in autumn, the large areas of western parts were predicted to induce NPE for blue space visitors (Figure 7G). In winter, blue spaces in northern Hebei, southern Anhui, and some central places in Hunan were predicted to mildly induce higher scores of NPE (Figure 7H). However, blue spaces in the common area across Hubei and Anhui evoked high NPE scores.
Discussion
Spatial distributions of facial expressions of people visiting urban wetland parks
It was surprising to find that the variations in wetland parks generated differences in the posted photos of smiles among wetland park visitors only in spring. In this season when the temperature starts to rise to a mild level, more positive emotions were expressed on the faces of people in the northern parks in contrast to those in the south. Our findings do not concur with those found by Li et al. (2022b), where the emotions of visitors did not vary across the spatial distribution patterns among wetland parks in eastern coastal cities in spring. Wetland parks in our study were chosen from inland cities, where the spring atmosphere is quite different from coastal cities (White et al., 2013). The geographical trend in spring disappeared in other seasons when positive and negative emotions were alternatively exposed on the faces of people in wetland parks along the geographical gradient.
Our results did not reveal any geographical trend of emotional change for urban wetland park visitors. For urban green space visitors, however, it was reported that the frequency with which people were exposed to positive emotions reduced along the latitudinal gradient from the south to the north in eastern cities (Liu et al., 2021c). The changes in expressed emotions of people visiting green spaces mirrored the changes in temperature and wind velocity (Mao et al., 2022). In wetland settings, the variations in the temperature did not generate any direct effects that could be perceived by visitors (Li et al., 2022b). According to the regression results in our study, more microclimatic factors were involved to jointly contribute to the expressed emotions. Therefore, the complexity of simulated settings resulted in a null response in positive emotions relating to the geographical distribution of wetland parks in seasons excluding spring. However, people posted more sad faces online in northern parks in colder climates, especially in winter (Figures 3G,H). These results are in line with the findings regarding expressed emotions of visitors to urban forests in Liu et al. (2021c), which are explained by the low temperature in the north. These results suggest that urban visitors to natural parks may be sensitive to colder temperatures, which is evident by the negative emotions expressed on their faces as soon as the temperature drops. These are temporary surmises which are discussed further below.
Landscape and microclimates perceived as emotional drivers by urban wetland park visitors
The large size of an urban landscape is believed to lower mental stress and restore it to normal levels according to stress recovery theory (SRT) (Ulrich et al., 1991). The acceptance of SRT is quite prevalent and people generally consider a larger area of urban nature to mean a higher dose of nature which can relieve mental stress. Recent studies are establishing through evidence that experiences in larger blue spaces of urban wetland parks did result in a higher rate of smiles and positive emotions on visitors' faces (Li et al., 2022a). Studies on urban green spaces also support the close relationship between experiencing large areas of forest parks and the frequency of smiles (Liu et al., 2021b; Zhang et al., 2021). In our study, although blue spaces evoked smiles in spring, the vastness of the landscape triggered sadness in autumn.
To bring about smiles, blue spaces were the sole contributor, but for triggering sadness blue spaces had to be accompanied by higher elevation (Table 4). In eastern coastal cities, the elevation of an urban wetland park was perceived as a driver of bad moods (Li et al., 2022a). Nevertheless, elevation-induced sadness had an opposite force that countered it by way of blue spaces in autumn. Compared to the coefficient of the estimated parameter for blue spaces, it was relatively smaller for elevation. These results suggest that the antagonistic effect of elevation was relatively smaller when a bad mood was triggered by blue spaces. Therefore, our first hypothesis is not valid because our results indicate that people are more likely to expose positive emotions in wetland parks that are smaller in size and have high elevation.
Warm temperatures were reported to be the main driver of expressed smiles in urban green spaces in autumn for cities of East China (Liu et al., 2021c). However, average daily temperatures (AveT) were also reported to be an indirect effect on smiles both in green (Mao et al., 2022) and blue spaces (Li et al., 2022b). These results suggest contrasting conclusions about the AveT effect on happy scores which were determined by “U-shape” curves under a maximum temperature record of 6.05°C in green spaces (Mao et al., 2022) and a minimum of 11.5°C in blue space (Li et al., 2022b). To study this contradiction, we used extreme temperature records per day instead of the average value. We found that the perception of daily MaxT induced sadness in summer, and the MinT contributed to smiles in spring and sadness in winter (Table 4). In the central part of China, spring is a mild season when high temperatures are preferred. Since winter is harsh, any lowering of the MinT is acutely felt (and disliked) by local people. Negative contributions of rainfall and wind to NPE in winter may be because they decrease the MinT. Therefore, our results do not provide any clear indication that high temperatures are a driver of positive emotions for visitors to urban wetlands of inland cities.
No NPE was found in summer, suggesting that in summer the MaxT effect on sadness was offset by smiles. The effect of MaxT in summer was accompanied by the effects of AveRH and AveW, which together contributed to expressing sad faces. The negative effect of experiencing humidity on posted emotions was also reported in wetland parks in East China (Li et al., 2022b). Therefore, people do not enjoy their time in urban wetlands in hot weather with humidity. In urban green spaces, however, studies demonstrated that RH at the understory layer can lower mental stress by reducing blood pressure (An et al., 2019) and inducing positive emotions (Park et al., 2011; Wei et al., 2020b). Relative to settings in green spaces, those in wetlands were closer to the waters, which may result in people feeling uncomfortable with high levels of humidity and high temperatures. The contribution of AveW to express sadness disagreed with the results in Li et al. (2022b), where the wind was perceived as a stronger diver to evoke smiles than sadness. We believe stronger winds in wetland parks should have alleviated the perceived bad mood caused by humidity. However, in our study, strong winds in elevated landscapes resulted in high scores of sadness instead of the perception of sorrow. Average wind velocity has a negative relationship with MaxT. This could be a key reason why NPE had a null response to spatial variations in summer because strong winds in highlands triggered sad faces, whereas it should have been a positive driver to encourage smiles. Anyway, our second hypothesis was also invalid because neither temperature nor humidity generated any positive impact to induce posting faces with positive emotions when visiting urban wetlands.
Mapping spatiotemporal distributions of predicted exposed emotions from potential visits to blue spaces
Based on regression results obtained in urban wetland parks, all blue spaces in the whole study area were predicted for their potential associations with posted facial expressions although some places may have not attracted any visits. Spring was a recommended season because no blue spaces were indicated to trigger sadness. A large area of connected blue spaces from northern Hunan, across southern Hubei, to the eastern Anhui, was recommended for visits in spring and winter when experiences can evoke positive emotions with a big number of posted smiles. In this area, urban wetland parks fall within the recommended range in the cities of Changsha, Wuhan, and Wuhu (Tuofu et al., 2021; Ye and Qiu, 2021). Ye and Qiu (2021) employed geographically weighted regression models that also revealed that visits to urban wetland parks in Wuhan can bring self-reported wellbeing to residents in Wuhan. Tuofu et al. (2021) found that urban blue spaces attracted people with higher wellbeing resulting in a stronger will to pay for low-priced houses.
In the growing seasons of summer and autumn, wetlands in northern Hebei, Shanxi, and western regions of Henan, Hubei, and Hunan were predicted to have visitors with a potentially high frequency to evoke positive emotions. In summer, wetlands in Hebei and Shanxi were also predicted to carry a high risk of inducing sad moods when no NPE was predicted. These results concur with our surmises in preceding discussions that the impulse to express smiles in these regions was offset by the impulse to post sad faces. In autumn, however, NPE was also predicted to be high in wetlands nearly overlapping the distribution of happiness. In Shanxi, although surface mining greatly impacted local ecosystem services to evoke wellbeing (Xiang et al., 2021), the local environment combined with wind strength at low velocity ( ≤ 5.36 m s−1) and temperature around 6.05°C was suggested to encourage smiles during urban forest visits (Mao et al., 2022). Our regression results suggest that the small-sized areas of blue spaces in these regions, including Shanxi, should be visited more often, especially the wetlands at high elevations.
Limits of the current study
The main drawback of using images from social media networks is an ongoing one and critics believe facial expressions in posts are mostly posed and self-screened before being uploaded to the internet. We used the posted facial photos as a source of data for facial expressions like others did in previous studies (Wei et al., 2019, 2020a, 2022; Li et al., 2022b). We agree with the argument that photos with spontaneous facial expressions would result in more reliable data that reflects the subconscious emotions of people when experiencing nature in urban landscapes (Wei et al., 2020b, 2021a,b). Thus far, we do not have a solution on how to collect a large number of photos from people that captures their subconscious emotions and generate a big-data pool of facial expressions. This is the first limitation of this study and hopefully, future research can address this issue with some cutting-edge technology.
Secondly, we predicted the spatial distribution of posted emotions of people as potential visitors to all blue spaces in our study area in Central China. The original data we used for regression were derived from selected urban wetland parks, therefore the predicted distribution of blue spaces was expected to have these landscape and microclimatic characteristics hence they can be good sceneries for visits with better moods. We did not interpolate our data to all urban wetland parks, which may result in some errors in the baseline of blue space distribution because some blue spaces other than wetlands may have been involved in spatial interpolation as well. Further studies are suggested to extend data obtained in parks to all areas of urban wetland parks, which will enhance the accuracy of targeting specific areas with blue spaces that have the recommended topographic characteristics.
Thirdly, our results are limited to spatiotemporal distribution that currently exists as a result of years of urbanization; most wetland parks are developed from natural remnants or newly introduced blue spaces. The location of the wetland ecosystem may be subject to projected land use or planned land transformation, which may both greatly impact resident visitors and influence their experiences when visiting blue spaces. China is a country with a record of rapid urbanization in recent decades. Hence it is important to factor in the coefficient of urbanization in the regression model that predicts people's emotions when visiting blue spaces.
Finally, we did not calibrate our mapping results which limits the accuracy of the spatial distribution of predicted emotions. Further work is suggested to validate results by recruiting participants and placing them in random places in the mapped region. If the recruited participants showed similar trends in emotions to blue spaces that were predicted by the mapping, then their accuracy can be deemed validated, and calibration is successfully undertaken.
Conclusions
Our study confirms an overall argument that people can display emotions through facial expressions in a spatiotemporal distribution pattern in different places of urban wetland parks. This was shaped by visitors' perceptions of joint landscape metrics and microclimates at different places across four seasons of the year. In this study, taking Central China cities as an example, we recommend which blue spaces to visit and at which season to get the maximum benefit of positive emotions. In spring and winter, it was recommended to visit blue spaces in urban wetlands in northern Hunan, southern Hubei, and eastern Anhui (positive emotion expressed in 40–60%). In autumn, northern wetlands in Shanxi and northern and western Hebei were recommended to be visited (positive emotion expressed in 20–100%). The size of blue spaces in wetland parks should be small (e.g., ~0.05 km2), and the elevation should be high (up to 790 m). Visitors may suffer from bad moods from their experiences in blue spaces if during their visit they encounter also a high temperature and strong wind.
Future studies can continue the scope of our study and extend its science in several aspects. Firstly, flood events are a force that can change the blue space landscape, which was not considered in this study. The post-flood landscape can be taken as a major factor that impacts people's perceptions of re-shaped blue spaces. Secondly, our study area was limited to a region that, although a large area in size, had a relatively homogenous terrain; it had plains in the central part and was surrounded by big mountains. Future work can be extended to other landscapes with alternatively high and low elevations across the whole area which may trigger different perceptions in people. Finally, demographics is a significant factor that distinguishes perceptions of people even when they share the same experience. Further work should consider studying people drawn from different races, genders, and ages to detect their perceptions of regional blue spaces.
Data availability statement
The datasets presented in this article are not readily available because the authors do not have the right to disclose raw data about facial expressions on any platform. Requests to access the datasets should be directed to the corresponding author.
Ethics statement
The studies involving human participants were reviewed and approved by Ethic Board Committee of Department of Human Resources Management, School of Business and Management, Jilin University. Written informed consent for participation was not required for this study in accordance with the national legislation and the institutional requirements. Written informed consent was not obtained from the individual(s) for the publication of any potentially identifiable images or data included in this article.
Author contributions
YL and YS: conceptualization and software. YZ and YS: methodology and writing—review and editing. YL, YZ, and YS: validation and project administration. YL and SC: formal analysis and funding acquisition. YZ and YW: investigation. YW: resources. YL: data curation and writing—original draft preparation. YL and YW: visualization. SC: supervision. All authors have read and agreed to the published version of the manuscript.
Funding
This research was funded by Key Scientific Research Projects of Colleges and Universities in Henan Province (Grant Nos.: 22B180011; 18A180026).
Acknowledgments
We acknowledge the editor and the reviewers for their contributions and assistance in developing the manuscript to its current form.
Conflict of interest
The authors declare that the research was conducted in the absence of any commercial or financial relationships that could be construed as a potential conflict of interest.
Publisher's note
All claims expressed in this article are solely those of the authors and do not necessarily represent those of their affiliated organizations, or those of the publisher, the editors and the reviewers. Any product that may be evaluated in this article, or claim that may be made by its manufacturer, is not guaranteed or endorsed by the publisher.
Abbreviations
EI, ecological infrastructure; SDG, sustainable development goal; NBS, nature-based solution; WHO, World Health Organization; AveRH, average relative humidity; AveW, average wind velocity every 2 min; NDWI, normalized difference water index; DEM, digital elevation model; DSM, digital surface model; MaxT, maximum daily temperature; MinT, minimum daily temperature; SunH, sunshine duration; NPE, net positive emotion index; ANOVA, analysis of variance; VetH, vegetative height; BlueA, blue space area; SRT, stress recovery theory.
References
Abd Latif, Z., Nasir, R. A., and Mohd, W. (2012). “Diurnal microclimate variations in tropical rainforest: Case study of Kuala Tahan, Pahang”, in: IEEE Colloquium on Humanities, Science and Engineering Research (CHUSER). doi: 10.1109/CHUSER.2012.6504356
Akpinar, A. (2016). How is quality of urban green spaces associated with physical activity and health? Urban Forest. Urban Green. 16, 76–83. doi: 10.1016/j.ufug.2016.01.011
An, B. Y., Wang, D., Liu, X. J., Guan, H. M., Wei, H. X., and Ren, Z. B. (2019). The effect of environmental factors in urban forests on blood pressure and heart rate in university students. J. Forest Res. 24, 27–34. doi: 10.1080/13416979.2018.1540144
Baidu Map (2022). 2022 Baidu - GS (2021) No. 6026. Beijing: Baidu. Available online at: https://map.baidu.com (accessed March 3, 2022).
Carrus, G., Scopelliti, M., Lafortezza, R., Colangelo, G., Ferrini, F., Salbitano, F., et al. (2015). Go greener, feel better? The positive effects of biodiversity on the wellbeing of individuals visiting, urban and peri-urban green areas. Landscape Urban Plann. 134, 221–228. doi: 10.1016/j.landurbplan.2014.10.022
Childers, D. L., Bois, P., Hartnett, H. E., McPhearson, T., Metson, G. S., and Sanchez, C. A. (2019). Urban Ecological Infrastructure: An inclusive concept for the non-built urban environment. Elementa-Sci. Anthrop. 7, 46. doi: 10.1525/elementa.385
China Today (2021). China launches massive rescue efforts after Henan rainstorms [Online]. Available online at: http://www.chinatoday.com.cn/ctenglish/2018/ttxw/202107/t20210722_800253833.html. (accessed April 11, 2022).
Climatic Data Centre (2021). Meteorological Data Centre of China Meteorological Administration [Online]. Available online at: http://data.cma.cn. (accessed September 13, 2021).
Dallimer, M., Irvine, K. N., Skinner, A. M. J., Davies, Z. G., Rouquette, J. R., Maltby, L. L., et al. (2012). Biodiversity and the feel-good factor: understanding associations between self-reported human wellbeing and species richness. Bioscience 62, 47–55. doi: 10.1525/bio.2012.62.1.9
Darrah, S. E., Shennan-Farpon, Y., Loh, J., Davidson, N. C., Finlayson, C. M., Gardner, R. C., et al. (2019). Improvements to the Wetland Extent Trends (WET) index as a tool for monitoring natural and human-made wetlands. Ecol. Indic. 99, 294–298. doi: 10.1016/j.ecolind.2018.12.032
de Vries, S., Verheij, R. A., Groenewegen, P. P., and Spreeuwenberg, P. (2003). Natural environments - healthy environments? An exploratory analysis of the relationship between greenspace and health. Environ. Plan. A 35, 1717–1731. doi: 10.1068/a35111
Evers, C., and Phoenix, C. (2022). Relationships between recreation and pollution when striving for wellbeing in blue spaces. Int. J. Environ. Res. Public Health 19, 4170. doi: 10.3390/ijerph19074170
Fitoka, E., Tompoulidou, M., Hatziiordanou, L., Apostolakis, A., Hofer, R., Weise, K., et al. (2020). Water-related ecosystems' mapping and assessment based on remote sensing techniques and geospatial analysis: The SWOS national service case of the Greek Ramsar sites and their catchments. Rem. Sens. Environ. 245, 111795. doi: 10.1016/j.rse.2020.111795
Freeman, C., Buttery, Y., and van Heezik, Y. (2022). Nature exposure and use of open spaces in three generation families: implications for planning. J. Environ. Plan. Manage. 65, 562–582. doi: 10.1080/09640568.2021.1891870
Fuller, R. A., Irvine, K. N., Devine-Wright, P., Warren, P. H., and Gaston, K. J. (2007). Psychological benefits of greenspace increase with biodiversity. Commun. Ecol. 3, 390–394. doi: 10.1098/rsbl.2007.0149
Gascon, M., Triguero-Mas, M., Martínez, D., Dadvand, P., Forns, J., Plasència, A., et al. (2015). Mental health benefits of long-term exposure to residential green and blue spaces: a systematic review. Int. J. Environ. Res. Public Health 12, 4354–4379. doi: 10.3390/ijerph120404354
Guan, H. M., Wei, H. X., Hauer, R. J., and Liu, P. (2021). Facial expressions of Asian people exposed to constructed urban forests: Accuracy validation and variation assessment. Plos ONE 16, e0253141. doi: 10.1371/journal.pone.0253141
Guha, S., Govil, H., and Besoya, M. (2020). An investigation on seasonal variability between LST and NDWI in an urban environment using Landsat satellite data. Geom. Nat. Hazards Risk. 11, 1319–1345. doi: 10.1080/19475705.2020.1789762
Gunderson, L. H., Light, S. S., and Holling, C. S. (1995). Lessons from the Everglades: Learning in a turbulent system. BioScience 45, S-66–S-73. doi: 10.2307/1312447
Han, Q. C., Kang, J. C., Yan, G. D., Wang, G. D., Lin, H., Zhu, J., et al. (2012). “Advances in the Study on the Relationship between Climate Change and Wetland Ecosystem”, in: 1st International Conference on Energy and Environment Protection (ICEEP 2012), p. 4953–4960. doi: 10.4028/www.scientific.net/AMR.518-523.4953
Hansson, L. A., Bronmark, C., Nilsson, P. A., and Abjornsson, K. (2005). Conflicting demands on wetland ecosystem services: nutrient retention, biodiversity or both? Freshwater Biol. 50, 705–714. doi: 10.1111/j.1365-2427.2005.01352.x
Hong, F. (2020). The Yearbook of China' Cities. Beijing, China: Press of The Yearbook of China' Cities.
Huo, Z. G., Wen, Q. P., Ma, Z. F., Xiao, J. J., and Zhang, L. (2013). “Assessment of rainstorm climate risk and rainstorm-induced agricultural disaster risk in east-central China,” in: 5th International Conference on Flood Management (ICFM5), p. 405.
Ishtiaque, A., Masrur, A., Rabby, Y. W., Jerin, T., and Dewan, A. (2020). Remote sensing-based research for monitoring progress towards sdg 15 in Bangladesh: a review. Rem. Sens. 12, 691. doi: 10.3390/rs12040691
Japan Aerospace Exploration Agency (2021). ALOS World 3D - 30m. Distributed by OpenTopography. (accessed October 28, 2021).
Jaramillo, F., Desormeaux, A., Hedlund, J., Jawitz, J. W., Clerici, N., Piemontese, L., et al. (2019). Priorities and interactions of sustainable development goals (SDGs) with focus on wetlands. Water 11, 619. doi: 10.3390/w11030619
Kerrihard, A. L., Khair, M. B., Blumberg, R., Feldman, C. H., and Wunderlich, S. M. (2017). The effects of acclimation to the United States and other demographic factors on responses to salt levels in foods: An examination utilizing face reader technology. Appetite 116, 315–322. doi: 10.1016/j.appet.2017.05.004
Klemm, W., Heusinkveld, B. G., Lenzholzer, S., Jacobs, M. H., and Van Hove, B. (2015). Psychological and physical impact of urban green spaces on outdoor thermal comfort during summertime in The Netherlands. Build. Environ. 83, 120–128. doi: 10.1016/j.buildenv.2014.05.013
Kong, F. (2019). Iop “Study on the contribution rate of urbanization and air-sea circulation factors to the increase of rainstorm in China”, in: 5th International Conference on Advances in Energy Resources and Environment Engineering (ICAESEE).
Koyama, N., and Yamada, T. (2020). A proposed simultaneous calculation method for flood by river water, inland flood, and storm surge at tidal rivers of metropolitan cities: a case study of katabira river in Japan. Water 12, 1769. doi: 10.3390/w12061769
Kumar, S., Agarwal, A., Villuri, V. G. K., Pasupuleti, S., Kumar, D., Kaushal, D. R., et al. (2021). Constructed wetland management in urban catchments for mitigating floods. Stochastic Environ. Res. Risk Assess. 35, 2105–2124. doi: 10.1007/s00477-021-02004-1
Li, H., Peng, J., Jiao, Y., and Ai, S. (2022a). Experiencing urban green and blue spaces in urban wetlands as a nature-based solution to promote positive emotions. Forests 13, 473. doi: 10.3390/f13030473
Li, H., Wang, X., Wei, H., Xia, T., Liu, M., and Ai, S. (2022b). Geographical distribution and driving meteorological forces of facial expressions of visitors in urban wetland parks in Eastern China. Front. Earth Sci. 10, 781204. doi: 10.3389/feart.2022.781204
Liu, P., Cao, B., Wang, Y. T., Wei, Z. P., Ye, J. F., and Wei, H. X. (2021a). Spectral effect of streetlamps on urban trees: A simulated study on tissue water, nitrogen, and carbohydrate contents in maple and oak. PLoS ONE 16, e0248463. doi: 10.1371/journal.pone.0248463
Liu, P., Liu, M., Xia, T., Wang, Y., and Guo, P. (2021b). The relationship between landscape metrics and facial expressions in 18 urban forest parks of northern China. Forests 12, 12. doi: 10.3390/f12121619
Liu, P., Liu, M. N., Xia, T. T., Wang, Y. T., and Wei, H. X. (2021c). Can urban forest settings evoke positive emotion? Evidence on facial expressions and detection of driving factors. Sustainability 13, 8687. doi: 10.3390/su13168687
Mao, B., Liang, F., Li, Z., and Zheng, W. (2022). Microclimates potentially shape spatial distribution of facial expressions for urban forest visitors: a regional study of 30 parks in North China. Sustainability 14, 1648. doi: 10.3390/su14031648
McFeeters, S. K. (2013). Using the normalized difference water index (NDWI) within a geographic information system to detect swimming pools for mosquito abatement: a practical approach. Rem. Sens. 5, 3544–3561. doi: 10.3390/rs5073544
NASA EarthData (2021). NASA EarthData [Online]. Available online at: https://search.earthdata.nasa.gov/search/?ac=trueandm=0.0703125!0!2!1!0!0%2C2. (accessed October 27, 2021).
National Standard of China (2017). Specifications for Surface Meteorological Observation-Sunshine. Beijing: Standardization Administration of China.
Nipoti, C., and Binney, J. (2007). The role of thermal evaporation in galaxy formation. Monthly Notices of the Royal Astronom. Society. 382, 1481–1493. doi: 10.1111/j.1365-2966.2007.12505.x
Park, B. J., Furuya, K., Kasetani, T., Takayama, N., Kagawa, T., and Miyazaki, Y. (2011). Relationship between psychological responses and physical environments in forest settings. Landscape Urban Plann. 102, 24–32. doi: 10.1016/j.landurbplan.2011.03.005
Priya, U. K., and Senthil, R. (2021). A review of the impact of the green landscape interventions on the urban microclimate of tropical areas. Build. Environ. 205, 108190. doi: 10.1016/j.buildenv.2021.108190
Rapinel, S., Hubert-Moy, L., Clement, B., and Maltby, E. (2016). Mapping wetland functions using Earth observation data and multi-criteria analysis. Environ. Monitor. Assess. 188, 641. doi: 10.1007/s10661-016-5644-1
Schirpke, U., Tasser, E., and Tappeiner, U. (2013). Predicting scenic beauty of mountain regions. Landscape Urban Plann. 111, 1–12. doi: 10.1016/j.landurbplan.2012.11.010
Shin, E. T., Shin, J., Rhee, D. S., Kim, H. J., and Song, C. G. (2019). Integrated inundation modeling of flooded water in coastal cities. Appl. Sci. Basel 9, 1313. doi: 10.3390/app9071313
Sina Weibo (2021). Weibo Reports Third Quarter 2021 Unaudited Financial Results. IR, Sina Weibo. Available online at: http://ir.weibo.com/news-releases/news-release-details/weibo-reports-third-quarter-2021-unaudited-financial-results (accessed March 2, 2022).
Sui, Y., Jiang, D. B., and Tian, Z. P. (2013). Latest update of the climatology and changes in the seasonal distribution of precipitation over China. Theor. Appl. Climatol. 113, 599–610. doi: 10.1007/s00704-012-0810-z
Sun, X., Yang, W. T., Sun, T., and Wang, Y. P. (2019). Negative Emotion under Haze: An Investigation Based on the Microblog and Weather Records of Tianjin, China. Int. J. Environ. Res. Public Health 16, 86. doi: 10.3390/ijerph16010086
Sun, Y., Ji, M., Jin, F. X., and Wang, H. M. (2021). Public Responses to Air Pollution in Shandong Province Using the Online Complaint Data. Isprs Int. J. Geo-Inf. 10, 126. doi: 10.3390/ijgi10030126
Triguero-Mas, M., Dadvand, P., Cirach, M., Martinez, D., Medina, A., Mompart, A., et al. (2015). Natural outdoor environments and mental and physical health: Relationships and mechanisms. Environ. Int. 77, 35–41. doi: 10.1016/j.envint.2015.01.012
Tuofu, H., Qingyun, H., Dongxiao, Y., and Xiao, O. Y. (2021). Evaluating the impact of urban blue space accessibility on housing price: a spatial quantile regression approach applied in Changsha, China. Front. Environ. Sci. 9, 696626. doi: 10.3389/fenvs.2021.696626
Ulrich, R. S., Simons, R. F., Losito, B. D., Fiorito, E., Miles, M. A., and Zelson, M. (1991). Stress recovery during exposure to natural and urban environments. J. Environ. Psychol. 11, 201–230. doi: 10.1016/S0272-4944(05)80184-7
USGS (2021). USGS EarthExplorer [Online]. Available online at: https://earthexplorer.usgs.gov/ (accessed October 21, 2021).
Wei, H., Hauer, R. J., and Guo, S. (2021a). Daytime dynamic of spontaneous expressions of pedestrians in an urban forest park. Urban Forestry Urban Green. 65, 127326. doi: 10.1016/j.ufug.2021.127326
Wei, H., Zhang, J., Xu, Z., Hui, T., Guo, P., and Sun, Y. (2022). The association between plant diversity and perceived emotions for visitors in urban forests: A pilot study across 49 parks in China. Urban Forestry Urban Green. 73, 127613. doi: 10.1016/j.ufug.2022.127613
Wei, H. X., Hauer, R. J., Chen, X., and He, X. Y. (2019). Facial expressions of visitors in forests along the urbanization gradient: what can we learn from selfies on social networking services? Forests 10, 1049. doi: 10.3390/f10121049
Wei, H. X., Hauer, R. J., and He, X. Y. (2021b). A forest experience does not always evoke positive emotion: A pilot study on unconscious facial expressions using the face reading technology. Forest Policy Econ. 123, 102365. doi: 10.1016/j.forpol.2020.102365
Wei, H. X., Hauer, R. J., and Zhai, X. Q. (2020a). The relationship between the facial expression of people in university campus and host-city variables. Appl. Sci. Basel 10, 1474. doi: 10.3390/app10041474
Wei, H. X., Ma, B. Q., Hauer, R. J., Liu, C. Y., Chen, X., and He, X. Y. (2020b). Relationship between environmental factors and facial expressions of visitors during the urban forest experience. Urban Forest. Urban Green. 53, 126699. doi: 10.1016/j.ufug.2020.126699
Weise, K., Hofer, R., Franke, J., Guelmami, A., Simonson, W., Muro, J., et al. (2020). Wetland extent tools for SDG 6.6.1 reporting from the Satellite-based Wetland Observation Service (SWOS). Rem. Sens. Environ. 247, 111892. doi: 10.1016/j.rse.2020.111892
White, M. P., Alcock, I., Wheeler, B. W., and Depledge, M. H. (2013). Coastal proximity, health and wellbeing: Results from a longitudinal panel survey. Health Place 23, 97–103. doi: 10.1016/j.healthplace.2013.05.006
White, M. P., Pahl, S., Wheeler, B. W., Fleming, L. E. F., and Depledge, M. H. (2016). The 'Blue Gym': What can blue space do for you and what can you do for blue space? J. Marine Biol. Assoc. United Kingdom 96, 5–12. doi: 10.1017/S0025315415002209
White, M. P., Wheeler, B. W., Herbert, S., Alcock, I., and Depledge, M. H. (2014). Coastal proximity and physical activity: Is the coast an under-appreciated public health resource? Prevent. Med. 69, 135–140. doi: 10.1016/j.ypmed.2014.09.016
WHO (1994). A User's Guide to the Self Reporting Questionnaire (SRQ). Division of Mental Health, World Health Organization.
WHO (2022). Urban Health [Online]. Available online at: https://www.who.int/health-topics/urban-health#tab=tab_1 (accessed April 11, 2022).
Wong, C. P., Jiang, B., Bohn, T. J., Lee, K. N., Lettenmaier, D. P., Ma, D. C., et al. (2017). Lake and wetland ecosystem services measuring water storage and local climate regulation. Water Resour. Res. 53, 3197–3223. doi: 10.1002/2016WR019445
Xiang, H. X., Wang, Z. M., Mao, D. H., Zhang, J., Zhao, D., Zeng, Y., et al. (2021). Surface mining caused multiple ecosystem service losses in China. J. Environ. Manage. 290, 112618. doi: 10.1016/j.jenvman.2021.112618
Xu, B., Li, G., Gao, C., Yan, H., Wang, Z., Li, Y., et al. (2021). Asymmetric Effect of El Niño—Southern Oscillation on the Spring Precipitation over South China. Atmosphere 12, 391. doi: 10.3390/atmos12030391
Yang, J. X., Zhou, Q., Liu, X. Y., Liu, M. M., Qu, S., and Bi, J. (2018). Biased perception misguided by affect: How does emotional experience lead to incorrect judgments about environmental quality? Global Environ. Change-Human Policy Dimen. 53, 104–113. doi: 10.1016/j.gloenvcha.2018.09.007
Ye, Y., and Qiu, H. F. (2021). Environmental and social benefits, and their coupling coordination in urban wetland parks. Urban Forestry Urban Green. 60, 127043. doi: 10.1016/j.ufug.2021.127043
Yu, F., Deng, J., Ding, X., and Ma, H. (2022). Interpolated stand properties of urban forest parks account for posted facial expressions of visitors. Sustainability 14, 3817. doi: 10.3390/su14073817
Yu, L., Liu, Y., Liu, T., and Yan, F. (2020). Impact of recent vegetation greening on temperature and precipitation over China. Agric. Forest Meteorol. 295, 108197. doi: 10.1016/j.agrformet.2020.108197
Zeng, L. L., Li, X. Y., and Ruiz-Menjivar, J. (2020). The effect of crop diversity on agricultural eco-efficiency in China: A blessing or a curse? J. Cleaner Product. 276, 124243. doi: 10.1016/j.jclepro.2020.124243
Zhang, J., Yang, Z., Chen, Z., Guo, M., and Guo, P. (2021). Optimizing urban forest landscape for better perceptions of positive emotions. Forests 12, 1691. doi: 10.3390/f12121691
Zhang, W., Zhu, Y. B., and Jiang, J. G. (2016). Effect of the urbanization of wetlands on microclimate: a case study of xixi wetland, Hangzhou, China. Sustainability 8, 885. doi: 10.3390/su8090885
Keywords: urban wetland, blue space service, perceived restoration, mental wellbeing, sustainable development goal
Citation: Li Y, Sun Y, Zhao Y, Wang Y and Cheng S (2022) Mapping seasonal sentiments of people visiting blue spaces in urban wetlands: A pilot study on inland cities of China. Front. Ecol. Evol. 10:969538. doi: 10.3389/fevo.2022.969538
Received: 15 June 2022; Accepted: 25 July 2022;
Published: 06 October 2022.
Edited by:
Md Rejaur Rahman, University of Rajshahi, BangladeshReviewed by:
Ramalingam Senthil, SRM Institute of Science and Technology, IndiaPir Mohammad, Hong Kong Polytechnic University, Hong Kong SAR, China
Copyright © 2022 Li, Sun, Zhao, Wang and Cheng. This is an open-access article distributed under the terms of the Creative Commons Attribution License (CC BY). The use, distribution or reproduction in other forums is permitted, provided the original author(s) and the copyright owner(s) are credited and that the original publication in this journal is cited, in accordance with accepted academic practice. No use, distribution or reproduction is permitted which does not comply with these terms.
*Correspondence: Yuxiang Sun, sunyuxiang@jlu.edu.cn; Yan Zhao, zhaoyanvip2008@163.com