- 1Centre of Excellence for Biosecurity Risk Analysis, The University of Melbourne, Parkville, VIC, Australia
- 2Centre for Environmental and Economic Research, The University of Melbourne, Parkville, VIC, Australia
- 3Victorian Department of Jobs, Precincts and Regions, Melbourne, VIC, Australia
Participants in the grains industry undertake general surveillance monitoring of grain crops for early detection of pests and diseases. Evaluating the adequacy of monitoring to ensure successful early detection relies on understanding the probability of detection of the relevant exotic crop pests and diseases. Empirical data on probability of detection is often not available. Our aim was to both gain a better understanding of how agronomists undertake visual crop surveillance, and use this insight to help inform structured expert judgments about the probability of early detection of various exotic grain pests and diseases. In our study we surveyed agronomists under a state funded program to identify survey methods used to undertake visual inspection of grain crops, and their confidence in detecting pests and diseases using the associated methods. We then elicited expert judgments on the probabilities of visual detection by agronomists of key exotic pests and diseases, and compared these estimates with the self-assessments of confidence made by agronomists. Results showed that agronomists used a systematic approach to visual crop inspection but that they were not confident in detecting exotic pests and diseases, with the exception of pest and diseases that affect leaves. They were most confident in visually detecting Barley stripe rust and Russian wheat aphid; however, confidence in detecting the latter was influenced by recent training. Expert judgments on the ability of agronomists to visually detect exotic pests and diseases early was in accordance with agronomists’ self-rated confidence of detection but highlighted uncertainty around the ability of agronomists in detecting non-leaf pests and diseases. The outcomes of the study demonstrated the utility of structured expert elicitation as a cost-effective tool for reducing knowledge gaps around the sensitivity of general surveillance for early detection, which in turn improves area freedom estimates.
Introduction
Some pests and diseases pose a significant threat to biosecurity because of their expected damage to environmental, economic, and social assets. Early detection surveillance aims to detect pests and diseases when their populations are small and geographically confined (Kalaris et al., 2014). If an incursion or outbreak is detected early, chances of containment or eradication are high, and long-term costs to governments and industry and damages to assets can be minimized (Leung et al., 2002; Magarey et al., 2009; Anderson et al., 2017; Epanchin-Niell, 2017; Hester and Cacho, 2017). However, surveillance activities cannot detect every biosecurity threat with absolute certainty, and pre-border and border activities cannot reduce the risk of incursions to zero. It is therefore important to quantify detection rates and probabilities to understand what inferences can reliably be made from surveillance data. Biosecurity managers are then able to use detection rates and probabilities to inform optimal surveillance design and allocation to ongoing monitoring efforts (Hauser et al., 2015).
Data quality is a critical consideration in judging how robust the evidence is to support decisions around pest absence. Data from general surveillance (i.e., obtained through non-specialist observations) is perceived as less authoritative and of lower quality than data from targeted surveillance where visual inspections are done by specialists (Whittle et al., 2013). The “surveillance continuum” developed by Hester and Cacho (2017) offers a clear distinction between four surveillance activities that differ in reporting source and purpose, and potentially, data quality. At one end, members of the public report fortuitous sightings of pests and diseases through passive surveillance. Further along the continuum, community groups, farmers, orchardists, agronomists, vets and laboratories participate in more structured approaches–Citizen science and general surveillance programs–and report detections that affect their agricultural industry or local community. At the other end of the continuum, pest and disease management agencies conduct deliberate, targeted and coordinated searches as part of active surveillance (Hester and Cacho, 2017). General and passive surveillance leverage the expertise of members of the public and industry participants and can be a powerful and cost-saving tool for the reporting of new pest incursions (Cacho et al., 2012; Hester and Cacho, 2012; Kalaris et al., 2014), but data quality needs to be explored. Confidence in active surveillance data is greater, but the costs of deploying trained field specialists can be prohibitive on the large scales required for surveillance for early detection.
Irrespective of the type of surveillance, the sensitivity of a surveillance system, or the probability of detection, is integral to the calculation of the likelihood of pest or disease absence. International and domestic trading partners require pest status information of a country, or a region within a country, as assurance that the products they import meet quarantine standards (PHA, 2013). According to the International Plant Protection Convention, a “pest free area is an area in which a specific pest does not occur as demonstrated by scientific evidence” (IPPC, 2017). The design prevalence is an important threshold in this context. It determines at what level of infestation a survey is expected to detect a pest or disease. Thus, freedom means that a pest or disease may be present, but at a level that is lower than the chosen design prevalence (Cameron and Baldock, 1998; Martin et al., 2007; Hester et al., 2015; Martin, 2017). To avoid trade restrictions or to gain market access, exporting countries provide trading partners with evidence of absence of a pest, referred to as “area freedom” claims. Area freedom, the statistical quantification of the likelihood of pest absence, can be calculated using different methods and often has a complex mix of inputs of quantitative and qualitative components from a diversity of sources (Kalaris et al., 2014; Hester et al., 2017) including targeted and general surveillance (Martin, 2017).
Probability of detection is difficult to estimate with confidence because it is influenced by a range of decisions and factors. For example, the decisions biosecurity managers make about surveillance effort (number of surveys, survey time, and area) and human resources (observer experience) (Bailey et al., 2004; Garrard et al., 2008, 2013; Epanchin-Niell et al., 2014; Hauser et al., 2015). Abiotic and biotic factors, such as species traits, site and weather conditions and the local abundance of the species are also influential (Bailey et al., 2004; Garrard et al., 2013; Hauser et al., 2015). The calculation of the likelihood of absence requires further inputs, depending on the chosen approach for estimating it. The null hypothesis approach requires knowledge of the design prevalence and the amount of surveillance effort, and the Bayesian approach calls for a “prior,” the belief a pest or disease is present at a location (Camac et al., 2019).
Little is known about the visual detection component of general surveillance systems for early detection of exotic grain pests and diseases (but see Hammond et al., 2016). Though detection probability can be estimated using different approaches, including routine surveillance activities, the most reliable method for estimating detection probability is direct experimentation because it reduces the gap between the perceived and the true efficacy of detection. However, detection experiments are expensive because they are resource intensive (Hauser et al., 2015). Under constrained government budgets, direct experimentation for each target pest and disease may therefore not always be possible, especially if these threats have never been detected in an area. When empirical data are not available, narrow in scope or difficult to acquire, elicitation of expert opinion can be another source of data (Gustafson et al., 2013). Structured expert elicitation protocols can help provide accurate and well-calibrated data (Hemming et al., 2018b), and can be obtained at much lower cost than detection experiments. Research also suggests that judgments of groups outperform those of individuals (Hemming et al., 2018b). The use of expert opinion is an established method for gathering knowledge in natural resource management (Yamada et al., 2003; Runge et al., 2011; Adams-Hosking et al., 2016) and biosecurity (Wittmann et al., 2014; Barry et al., 2015).
In this study we aimed to generate improved estimates of the probability of detection of exotic grain pests and diseases for a government funded visual surveillance program led by grain agronomists in Australia. Our study set out to enhance the current understanding of visual inspection methods applied in the grains industry in Victoria, and to improve the confidence of biosecurity managers in using general surveillance data for area freedom calculations. The approach taken in our study can easily be transferred to other countries and plant production industries. We surveyed agronomists to better understand how they undertake crop health surveillance and to obtain an estimate of their self-rated confidence in detecting exotic grain pests and diseases early. Then, using this insight, we conducted a structured expert elicitation with experts from the grains industry on the probability of early detection of various exotic grain pests and diseases by agronomists. We further explore the uncertainty around the ability of agronomists to detect different types of pests and diseases and the implications for area freedom calculations.
Materials and methods
Case study–Crop safe program
Crop Safe is a state funded general surveillance program in Victoria, Australia, that coordinates collection of crop health information through a network of 180 industry grain agronomists, who undertake extensive and repeated observations of grain crops to provide early warning of incursions of 15 (since the incursion of Russian wheat aphid in June 2016 only 14) exotic grain pests and diseases. During 2018, agronomists surveyed 25,276 paddocks of wheat, oats, barley, canola, field peas, chickpeas, faba beans, lupins, lentils, vetch, and triticale. Agronomists visited paddocks 4–7 times a growing season, totaling more than 118,656 individual crop inspections over an area of 1.9 million hectares (more than 65% of the total area of grain crops in Victoria). Agronomists access annual workshops designed to familiarize them with common and exotic pests and diseases of cereal and pulse crops. On average, 90–100 suspect samples are submitted to a Crop Safe diagnostic laboratory annually to diagnose unknown plant disease symptoms or pests. The laboratory provides a diagnosis and feedback, improving agronomist skills while ensuring accuracy of the surveillance data. If the Crop Safe laboratory still suspects and exotic pest has been found, the sample is submitted to a reference diagnostic laboratory for verification.
Apart from providing early warning assurance, the information gathered under the Crop Safe program can also be used to inform area freedom claims (Hammond et al., 2016; PHA, 2020) at a lesser cost than targeted surveillance. Calculating the likelihood of pest absence requires knowledge of the sensitivity of the components of the surveillance system. Sensitivity means that visual inspection will detect a pest or disease if it is present in the surveyed unit. The Crop Safe program is one component of the overall surveillance system in Victoria for the detection of exotic grain pests and diseases. From here on, we will refer to the Crop Safe component of the Victorian surveillance system as “surveillance system” or “surveillance program.” The probability of agronomists detecting pest and diseases on visual inspection is an important factor in the calculation of the sensitivity of the surveillance system, as the higher the probability of detection by agronomists, the greater the sensitivity of the surveillance system (Froessling et al., 2013; Wright et al., 2016). Specificity, the proportion of truly negative detections, may be lower for root diseases and stem pests because signs and symptoms can be similar. However, specificity is assumed to be perfect in our context, following the reasoning by Martin et al. (2007), because any false positives detected at visual inspection would be resolved through follow-up testing in the laboratory.
Representation of the surveillance system as a scenario tree
The surveillance system consists of a sequence of events that can be depicted by a scenario tree (Figure 1). The tree has one infection node. It represents the proportion of crops, the paddocks of farmers or growers participating in the surveillance program across the region, that are infected, PrCrop. This is the design prevalence set by the Crop Safe managers. The next event is detection at visual inspection by a Crop Safe agronomist, at a probability of PrVis. The unit of inspection is discrete paddocks. Prior to our study, probability of detection at visual inspection in the surveillance system was set at 0.6–0.8 based on evidence from detection experiments with Lupin anthracnose, an exotic crop disease that affects above-ground plant parts. This estimate was applied to all other crop pests and diseases surveyed under Crop Safe. Following visual detection by an agronomist, two stages of Crop Safe diagnosis follow. First, a sample of affected crop is sent to a regional laboratory for preliminary testing. The sample tests positive with a probability of PrLDCC, which is the sensitivity of the test at that regional laboratory–The probability that the test result is positive given the pest or disease is present. If the first laboratory test is positive, the sample is sent to crop health services for definitive testing. The probability of a positive result, again, is the sensitivity of the test, depicted as PrLab in the scenario tree. The sensitivity of the surveillance system is the product of the conditional probabilities associated with each positive node. The focus of this study is on the second node Detected at visual inspection, on the probability of visual detection by an agronomist in the field.
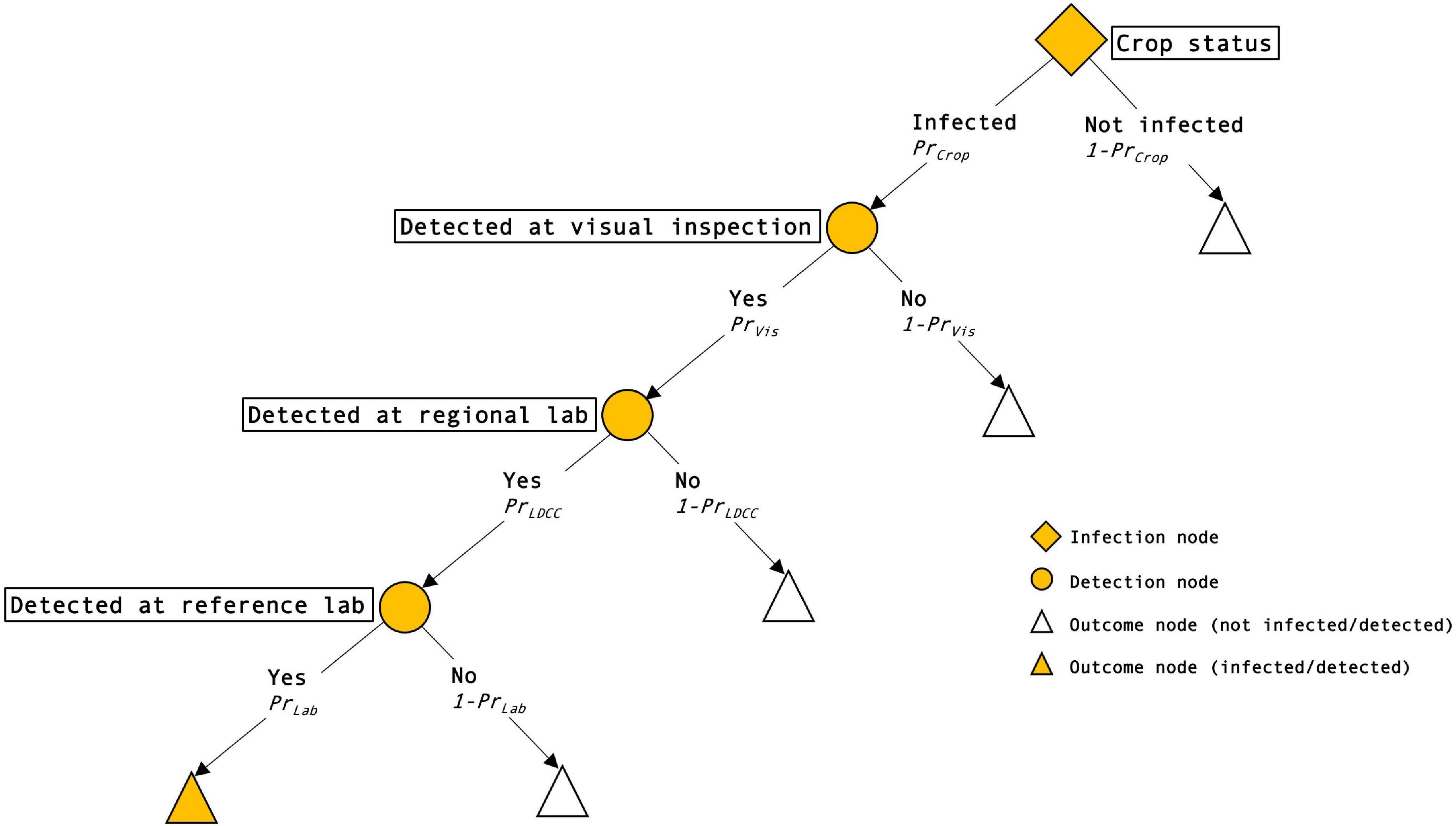
Figure 1. Representation of the surveillance system as a scenario tree. In sequence of events, the surveillance system consists of infection node or crop status (filled diamond), detection nodes where visual inspection and laboratory testing is undertaken (filled circles), and outcome nodes (triangles). Outcome nodes represent infected/detected crop proportions (filled triangles) and not infected/detected crop proportions (empty triangles).
Online survey
In the first part of this study, we developed an online survey Qualtrics software (Qualtrics, Provo, UT, USA) to improve current understanding of methods applied by agronomists for surveying crop health and of their level of confidence in early detection of exotic grain pests and diseases. The survey was anonymous and asked participants about: (i) personal information (gender, age, and work experience in agronomy), (ii) inspection approach (including time spent and frequency of inspections), (iii) confidence in identifying different crop problems, (iv) triggers for investigating a problem further in terms of its severity and extent, (v) frequency of using laboratory diagnostic facilities, (vi) confidence in detecting exotic pests and diseases in early stages of infestation, (vii) Crop Safe training for identifying Russian wheat aphids, (viii) use of specific manuals and awareness of others, and (ix) their opinion on the surveillance program in general (strengths and weaknesses). Here, we will only report survey results related to participant demographics, inspection approaches and confidence in detecting exotic pests and diseases.
Crop Safe staff distributed a link of the survey to 157 agronomists in the grains industry in June 2016. The survey remained open for 1 week and offered a non-monetary incentive to encourage participation. Survey participant responses were collated and analyzed using the open source software R Statistical Software [version 3.5.2 (2018-12-20)–“Eggshell Igloo,” R Core Team, 2018, Vienna, Austria]. For time spent on specific inspection methods and agronomists’ self-rated confidence of detection, the mode is used for describing survey results. We present the mode, the category (in percent of the time), that was selected most often, and the percentage of respondents that have selected this category.
Russian wheat aphid incursion and training
On 9 June 2016, the presence of Russian wheat aphid was confirmed in South Australia and western Victoria (IPPC, 2016). As a response to this incursion a Crop Safe agronomist workshop was held in Horsham, Victoria on 10 June 2016 to train agronomists in the detection of Russian wheat aphid. This allowed us to include a question about training attendance in the survey and to divide the responses of survey participants into two groups depending on whether they had attended the training course or not. A fifth (20%) of survey participants attended the training, 4 days before the survey was sent out.
Expert elicitation workshop
The second part of our study involved the use of an expert panel in an elicitation workshop on 29 June 2016 to quantify experts’ perception of the probability of early detection of exotic pests and diseases by agronomists. The panel consisted of nine experts with a professional background in biosecurity, such as diagnosticians and other technical experts. The panel participants were selected based on their active engagement in entomological and plant pathological field research of broad acre crops, as well as training of Crop Safe agronomists in pest and disease identification. At the start of the day, results from the online survey were introduced to workshop participants, except for self-rated confidence in detection. The survey results were shared so that participants gained a better understanding of what field survey methods agronomists applied. Prior to the elicitation, participants assigned the fifteen exotic pests and diseases of interest to the surveillance program to seven groups (one to four species per group), considering different aspects of grain morphology, plant life cycle and visual detectability of infestations (Table 1). This was done to reduce the elicitation burden on participants, but also allows a more generalized approach to assessing detectability, i.e., the judgments could be applied to different pests and diseases, as long as they fall within the seven groups. Workshop participants decided to exclude Khapra beetle from the elicitation exercise as it is a post-harvest pest of stored grain and usually absent from crops.
The same elicitation question “What is the probability of early detection by Crop Safe agronomists according to effort?” was applied to the seven groups using a structured elicitation procedure called the IDEA protocol (Hemming et al., 2018a). We applied the four-step format of the IDEA protocol for eliciting quantitative estimates from experts, with uncertainty (Hanea et al., 2017). During the first part of the elicitation process, experts provided their initial individual estimates of the probability of early detection, basing their judgments on an incursion level (design prevalence) of 1% of a 100 ha hypothetical crop. We standardized interval estimates to 80% confidence levels across all experts using linear extrapolation (described in detail in Hemming et al., 2018b). This allowed viewing of credible intervals on a consistent scale and facilitated comparisons during the following group discussion phase. The results were de-identified, aggregated and presented to the group. Facilitators revealed agronomists’ self-rated confidence in early detection of exotic grain pests and diseases at this stage to prevent introducing anchoring bias, the tendency to hold onto a previously considered number when making numerical estimates (Burgman, 2015). Following a group discussion based on first round estimates and agronomists’ self-rated confidence, experts submitted their revised and final individual estimates.
Aggregating expert judgments
Quantile aggregation, where the arithmetic mean of experts’ estimates is calculated for the lower, best, and upper estimates is used frequently in applications of the IDEA protocol. It is a fast and uncomplicated approach that is easily understood by participants, but there is indication that the quantile aggregation method performs poorly when compared to aggregating fitted distributions (Hemming et al., 2018a). The scaling to 80% intervals does not correspond to any standard distributional assumptions. Because estimates of detection probability were to be used in a scenario tree model (Figure 1) we aggregated the elicited data using Beta distributions as the underlying model for probability of detection during visual inspection. Our approach was to assume that the elicited data from each expert were summary statistics from a Beta distribution, θk∼ Beta (αk,βk), from which we estimated the parameters (αk,βk). The aggregated expert density can then be estimated as θ′∼ Beta (α′,β′), where αk,βk are the parameters of the k = 1, …, K Beta distributions for experts’ estimates of probability of detection, and B is the Beta function, and ψ is the digamma function.
The Kullback–Leibler divergence, DKL (θ′,θk), represents the amount of information lost by assuming that the distribution is θk∼ Beta (αk,βk), when in fact it is really θ′ Beta (α′,β′). We aggregated and plotted expert judgments in R Statistical Software [version 3.5.2 (2018-12-20)–“Eggshell Igloo,” R Core Team, 2018, Vienna, Austria].
Statistical analysis
Statistical differences between aggregated final estimates of the probability of visual detection were determined by fitting beta distributions to the data, sampling from the distributions, and then comparing the samples using the Two-Sample Kolmogorov–Smirnov Test. Results are presented in the Supplementary Appendix Table A1. Analysis was conducted in MATLAB R2021a, The MathWorks, Inc., Natick, MA, USA.
Results
Out of 157 targeted Crop Safe agronomists, a total of 89 completed the survey with a valid response, resulting in a participation rate of 57%. Survey respondents were diverse in age (22–67 years, median 37), and work experience in agronomy (1–45 years, median 14 years), but not gender (76% male).
Time spent on specific inspection methods
The online survey (refer to Supplementary material), asked agronomists about their approach for inspecting crop health at a site, including how much time they usually spend on eight particular inspection methods (Figure 2). Survey results showed that Crop Safe agronomists invested considerable time in visually inspecting grain crops and that they applied a systematic and detailed approach. Most agronomists obtained an overview of both healthy looking and weak patches in an area first and closely examined individual plants from good and bad patches (mode 60–80% of the time, 47% of responses) or only from weak patches (mode 60–80% of the time, 36% of responses). They often applied zigzag inspection patterns (mode 80–100% of the time, 34% of responses), and examined areas that the farmer has identified to them (mode 60–80% of the time, 33% of responses). It was not the norm among agronomists to drive around the perimeter of a paddock (mode 0–20% of the time, 48% of responses) or focus on seemingly bad patches (mode 0–20% of the time, 36% of responses). Drones were rarely used at the time of the survey. A high proportion of respondents (92%), reported that they rarely (0–20% of the time), examined a crop area by drone first before attending to problem areas.
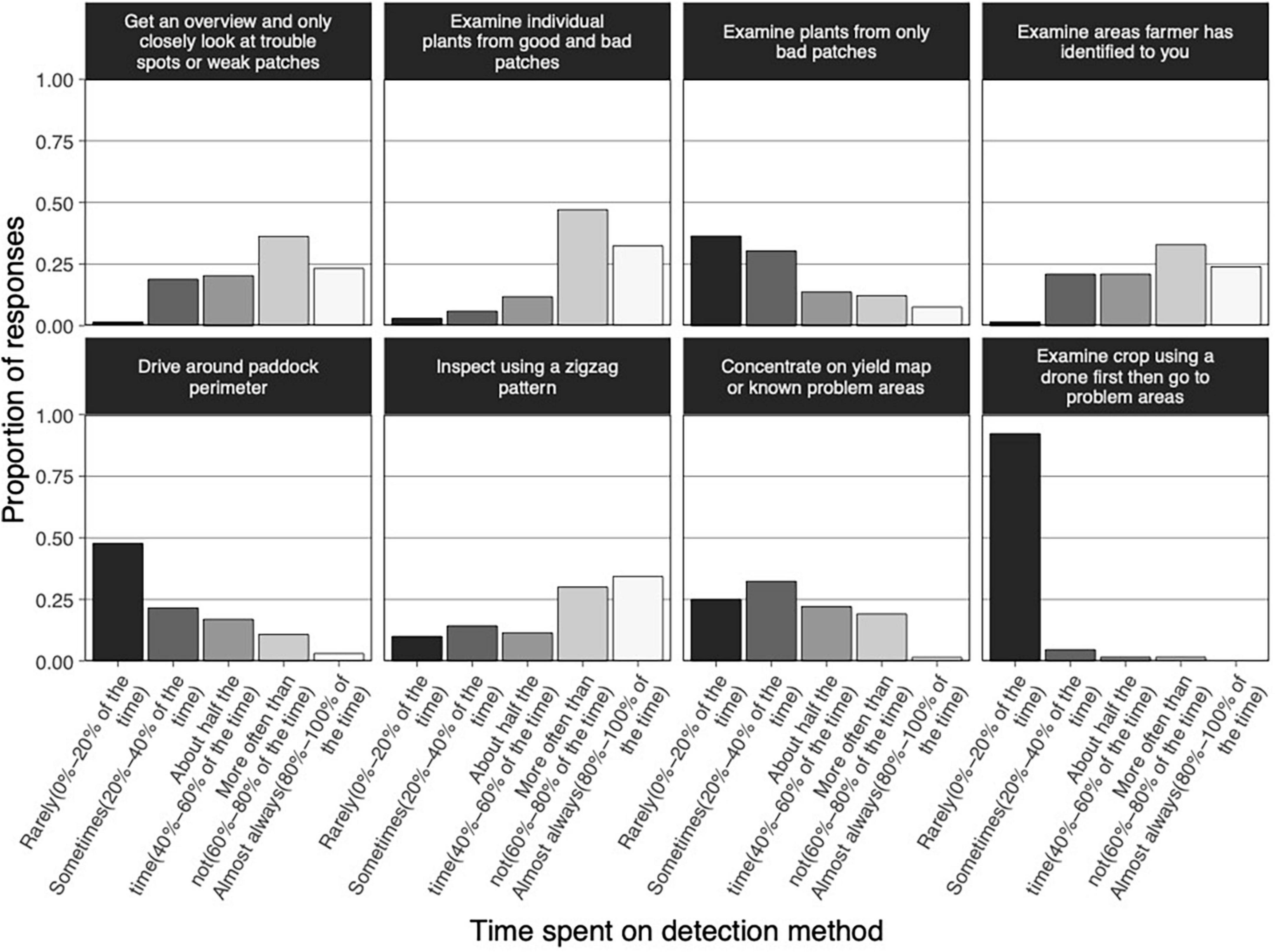
Figure 2. Proportion of time spent by agronomists on eight different crop inspection methods. Vertical bars represent the proportion of responses. Bar gray scaling indicates differences in frequency of method application, ranging from “rarely” to “almost always.”
To provide more context around inspection approach, the time spent inspecting a 100 ha crop varied from 15 to 30 min and agronomists reportedly visited each site 4–7 times per growing season.
Agronomists’ self-rated confidence of detection for each pest or disease
Self-rated confidence of detection among respondents varied with each crop pest and disease (Figure 3). Crop Safe agronomists were most confident in detecting Barley stripe rust and Russian wheat aphid (mode 90–100% of the time–highly confident, 46 and 41% of responses, respectively). Crop agronomists rated their confidence of detection as very low (mode 0% of the time–not at all confident) for most of the pests and diseases, including Maize leafhopper (67% of responses), American serpentine leaf miner (65% of responses), Cabbage seedpod weevil (62% of responses), European wheat stem sawfly (62% of responses), Barley stem gall midge (55% of responses), Canola verticillium wilt (52% of responses), Khapra beetle (51% of responses), Turnip moth (47% of responses), Fusarium wilt of chickpea (38% of responses), Karnal bunt (33% of responses), Lentil anthracnose (33% of responses), and Fusarium wilt of canola (32% of responses). Lentil rust and Lupin anthracnose had a balanced distribution of responses (Figure 3). Agronomists who had undertaken the Russian wheat aphid training were more confident in detecting this particular pest. More specifically, 57% of training participants were “highly confident” in identifying Russian wheat aphid compared to 38% of agronomists who had not attended the training.
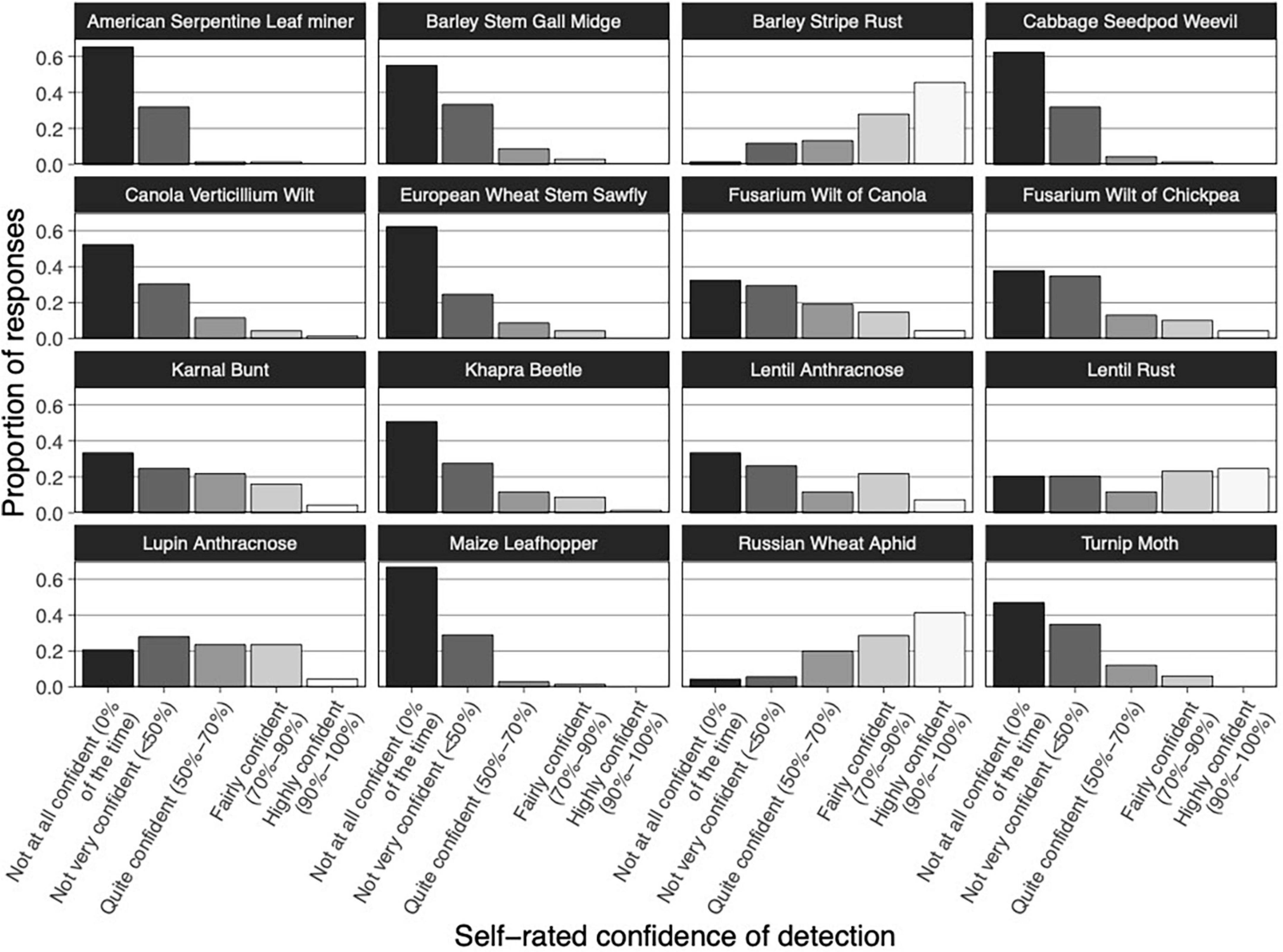
Figure 3. Crop Safe agronomists’ self-rated confidence of detection of exotic pests and diseases at visual inspection. Vertical bars represent the proportion of responses. Bar gray scaling indicates differences in self-rated confidence of detection, ranging from “not at all confident” to “highly confident.”
We found no relationship between field experience and confidence in detection (data not shown). This was probably due to the high level of field experience among survey respondents. Most respondents had more than 5 years (30 out of 73 valid responses for this question), and more than 15 years (34 out of 73 valid responses) of field experience. Nine respondents indicated that they had less than 5 years of field experience and sixteen did not answer this question or provided an invalid response.
Agronomists’ self-rated confidence of detection for each pest/disease group
Self-rated confidence in visually detecting pests and diseases was low (mode 0% of the time–not at all confident ranging from 35 to 59% of responses, Figure 4) across the seven pest/disease groups, with one exception. Overall, self-rated confidence of detection was greater for pests and diseases that affect leaves. Agronomists were most confident in detecting diseases in the leaf disease 1 group (mode 90–100% of the time, 25% of responses). The groups leaf disease 2 and leaf pest had marginally better ratings than the other pest/disease groups. Agronomists were least confident in detecting grain and stem pests (mode 0% of the time–not at all confident, 57 and 59% of responses, respectively).
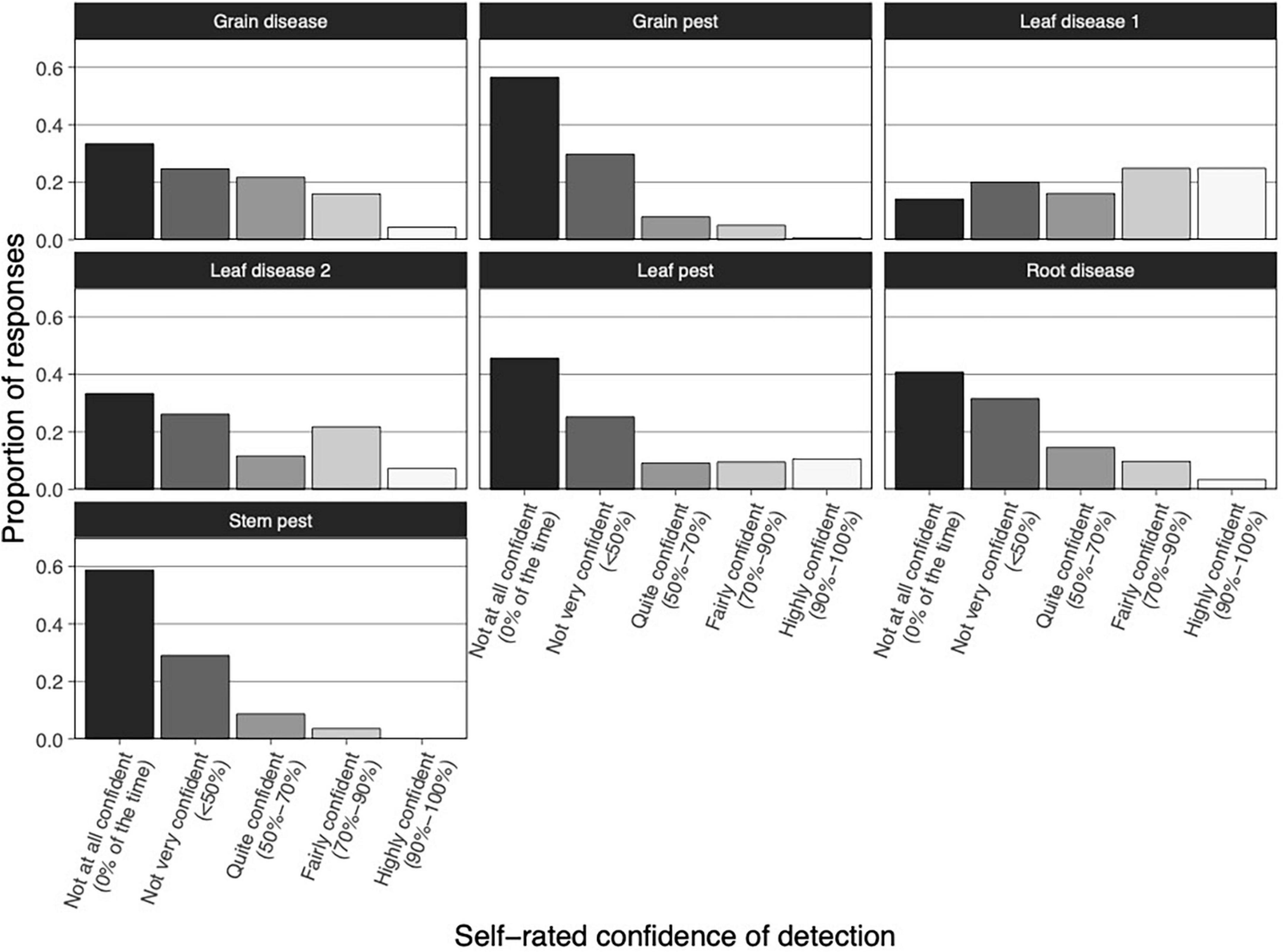
Figure 4. Crop Safe agronomists’ self-rated confidence of detection of exotic pests and diseases at visual inspection. Pests and diseases are divided into seven groups. Vertical bars represent the proportion of responses. Bar gray scaling indicates differences in self-rated confidence of detection, ranging from “not at all confident” to “highly confident.”
Estimation of visual probability of detection by experts
The aggregated 80% intervals for the probability of visual detection during inspection (Figure 5) showed that experts rated the detectability of leaf diseases (group 1 and 2) and pests as higher (best guesses 0.76, 0.37, and 0.57 after discussion) than that of grain pests and diseases (best guesses 0.25 and 0.30), root diseases (best guess 0.32), and stem pests (best guess 0.30). Pairwise comparisons showed that estimates for leaf diseases and pests were significantly higher (null hypothesis rejected 95–100% of the time, Supplementary Appendix Table A1), than those of the other groups, with a less pronounced result for the comparison between leaf disease 2 and root diseases (null hypothesis rejected 68% of the time). These results are consistent with the broad detectability ratings (Table 1), which were based on the ease of visual detection and considered characteristics such as visual distinctiveness, which part of the plant is affected and the stage of infestation or infection at which symptoms occur. Results are also consistent with agronomists’ self-rated confidence.
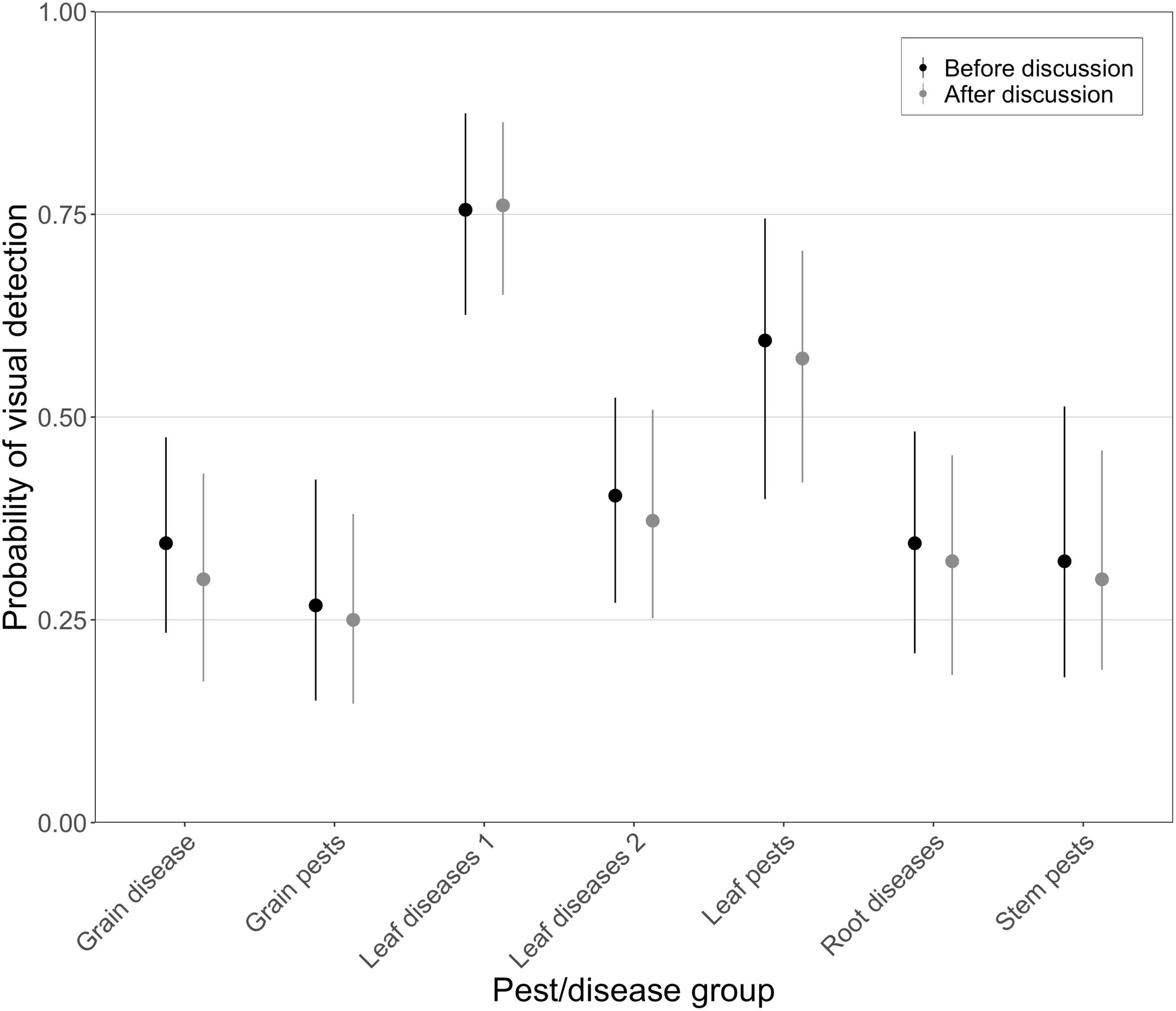
Figure 5. Aggregated 80% intervals for the probability of detection of exotic pests and diseases during visual inspection. Pests and diseases are divided into seven groups. Best estimates (means), with 80% upper and lower credible intervals, are shown for the first and second round of expert elicitation. Black circles represent best estimates before discussion (initial estimates) and gray circles represent best estimates after discussion (final estimates).
Representation of expert opinion using Beta distributions showed that individual experts rated detectability of pests and diseases differently and with varying degrees of confidence (Figure 6). There was greater uncertainty among experts about the probability of visual detection by agronomists of grain diseases, stem pests, and Lentil anthracnose (leaf disease 2 group). While experts’ opinions were more aligned for other pest and disease groups, some of those groups included outliers. For example, one expert estimated the probability of detection of leaf pests to be very low (0.16) while the aggregated group estimate showed that most experts were fairly confident (0.63) that agronomists would detect leaf pests at visual inspection. Similarly, group estimates of detection probability of pests and diseases in the leaf disease 1 group were high (0.82) with a number of confident experts (high and narrow peaks). Two experts, however, estimated the probability of detection to be much lower (0.50 and 0.65).
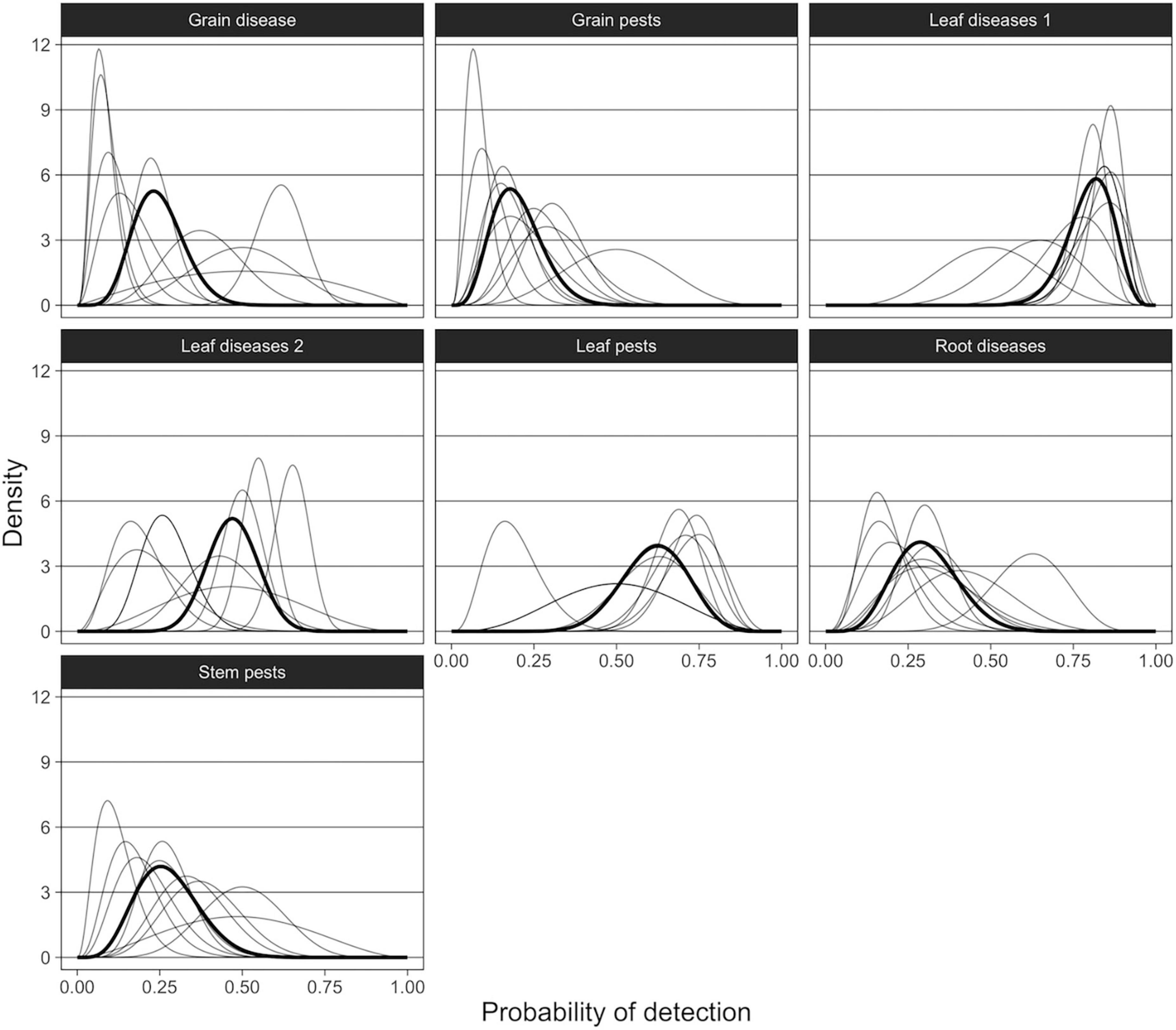
Figure 6. Probability density functions of the aggregated Beta distributions, showing the probability of detection during visual inspection for each pest/disease grouping as estimated by the experts. Thin lines represent individual experts’ estimates and bold lines represent aggregated densities by minimizing Kullback–Leibler divergence.
Discussion
The self-rated confidence of Crop Safe agronomists in the probability of visually detecting exotic crop pests and diseases early was generally low. Not surprisingly, confidence in detection was highest for pests and diseases that affect leaves. Signs and symptoms on leaves are more visible and easier to detect than belowground symptoms caused by root diseases such as Fusarium wilt of canola and chickpea, caused by a fungus that infects plants through the root system. Agronomists were most confident in detecting Barley stripe rust, a fungal leaf disease that develops distinct yellow stripes of rust pustules between the veins of leaves (PHA, 2019a). Agronomists were also highly confident in detecting Russian wheat aphid, a small soft bodied insect with a distinct double structure at the rear that feeds mostly on wheat and barley (PHA, 2019b). A recent incursion of Russian wheat aphid allowed us to compare confidence ratings of agronomists who had attended emergency response training with ratings of agronomists who had not attended. Survey participants who attended the training were clearly more confident than participants who did not attend, demonstrating that targeted, face-to-face training of agronomists was effective in increasing their confidence in early detection. In contrast, some methods of information transfer, such as fact sheets, other reading material and information booths at local field days appear to be less effective (Wright et al., 2016). Training courses can increase the probability of detection and reporting of pests and diseases, but they often do not reach relevant end users. For instance, in the Western Australian grains industry, agricultural consultants reported that they had not attended any training to improve their knowledge of high priority pests and diseases (Hammond et al., 2016).
Surveys of the Australian and Western Australian grains industries covered four of the pests and diseases included in our survey. Both these studies asked participants to self-rate the likelihood of detection and/or to correctly identify symptoms associated with crop pests and diseases (Hammond et al., 2016; Wright et al., 2016). The findings were similar, in that respondents in both surveys had more confidence in detecting plant diseases (Barley stripe rust and Karnal bunt) than insect pests (Khapra beetle and Russian wheat aphid). Our survey, however, revealed that Crop Safe agronomists were much more confident in detecting Barley stripe rust and Russian wheat aphid than Khapra beetle and Karnal bunt. In contrast to Hammond et al. (2016), who reported low confidence in the likelihood of detection by growers, agricultural consultants, and government staff (mode 0–10% likelihood), Victorian agronomists were highly confident in detecting Russian wheat aphid, including many of the respondents who had not attended the Crop Safe training workshop. The high confidence reported by agronomists may have been an effect of the incursion of this pest in South Australia and western Victoria shortly before our survey was sent out. Incursions usually trigger communication activities such as the dissemination of information material on pest identification and early detection.
When pests and diseases are exotic to an area, they have never been detected there, or, they have been absent for a long time. A consequence of no previous history of observed detections is that no empirical data on detection probability is available. Eliciting expert judgments can mitigate gaps in knowledge, especially when applying a structured elicitation protocol, which improves transparency, accountability and quality of these judgments (Hemming et al., 2018b). The elicited probability estimates of visual detection, by pest/disease group, were consistent with both the broad detectability ratings given by participants before the expert elicitation and the self-rated confidence by Crop Safe agronomists. Experts also estimated a greater probability of detecting pests and diseases that affect leaves than the probability of detecting those that affect roots, stems, and grains.
Crop Safe managers have replaced their previous estimates of probability of detection at visual inspection for all 14 exotic pests and diseases with the results from this study, but our results also indicate potential areas where Crop Safe managers could direct future efforts to improve confidence in the sensitivity of the surveillance system. Experts were in strong agreement with each other and with agronomists about early visual detection of leaf pests and diseases, confirming the use of agronomists as a cost effective and reliable method to survey crop health in relation to leaf pests and diseases. Experts were, however, uncertain about detection probability of non-leaf pests and diseases.
To further reduce the uncertainty around visual detectability of pests and diseases that affect roots, stems and grains, biosecurity managers have different options. They can invest in direct detection experiments in the field, in targeted training or active surveillance. Direct detection experiments cannot be conducted easily with pests and diseases that are absent in all of Australia but could be conducted using pests and diseases that are similar to their exotic counterparts. In the case of Russian wheat aphid, which was detected in South Australia and Victoria a short time prior to our study, direct field detection experiments could be developed to better estimate the probability of visual detection. This would be useful information for surveillance initiatives in other Australian jurisdictions where no infestation of Russian wheat aphid has been detected to date. Targeted training increased the confidence of agronomists in detecting Russian wheat aphid early and could be used to reduce the uncertainties of detecting pest and diseases affecting roots, stems, and grains. However, training may not improve visual detection probability for diseases that affect roots because they are usually difficult to detect at early stages of infection and above ground symptoms can be confused with those that are responses to physiological stresses such as drought (Jiménez-Díaz et al., 2015). Active surveillance, a more formal and structured approach, could increase the probability of detection through an increased surveillance effort. In the case of pests and diseases that are hard to detect visually, a more targeted surveillance strategy would have to be supported by bespoke sampling protocols and diagnostic resources.
Overlaying individual estimates of the probability of detection with the aggregated group estimate showed that expert opinion varied depending on the pest/disease group. For example, the leaf pest group had a difference of 0.47 between the aggregated group estimate and one individual’s estimate. This difference is distinct and exemplifies the risk of relying on the judgment of a single person that may be considered the most credentialed expert. We can use this example to illustrate the consequences of relying on a single expert. The probability of visual detection of leaf pests is an input into the calculation of the sensitivity of the surveillance system for this group of pests as depicted in the scenario tree representation of the surveillance system (Figure 1). Because of the positive relationship between the variables, a greater probability of detection will result in a greater sensitivity of the surveillance system (Froessling et al., 2013; Wright et al., 2016). Decision makers use the sensitivity of a surveillance system for estimating the probability that a pest or disease is absent, because they are interested in quantitative evidence of absence for trade negotiations. Two fundamental approaches are available: the likelihood of pest absence can be inferred from confidence of detection (Null hypothesis testing) or it can be explicitly estimated using the Bayes’ theorem (see Martin et al., 2007; Camac et al., 2019). To illustrate, using the Bayes approach, an underestimated sensitivity of the surveillance system, based on poor judgment of a single expert, would result in a less favorable posterior estimate of probability of freedom. Overestimating the sensitivity of the surveillance system, on the other hand, inflates the posterior estimate of probability of freedom. Empirical evidence shows that aggregated judgments of a group of individuals outperform judgments of individuals (Surowiecki, 2004; Budescu and Chen, 2015; Hemming et al., 2018b).
Looking at the use of technology for crop inspections, the online survey revealed that respondent agronomists applied a systematic and detailed approach to visual inspection of crop health. But at the time of the survey, they were not using drones regularly to examine wider crop areas. The efficacy of drones in monitoring crop health has been demonstrated elsewhere (e.g., Stehr, 2015; Puri et al., 2017; Mogili and Deepak, 2018). Veroustraete (2015), for example, reported that the main application of drones in agriculture is for crop health inspections using sensors that can detect visible light and near-infrared wavelengths. Low uptake of this new technology may partly be due to strict regulations around the use of drones that only permit holders of specific licenses to operate them outside the visual line of sight (CASA, 2018). Another limitation on the use of drones is that they are not suitable for detecting the early stages of infection of diseases that affect plant roots. This limitation also holds true for remote sensing technologies carried on satellites. However, high-resolution multispectral satellite imagery can be useful in detecting some plant pests and diseases (Raza et al., 2020). If the grains industry in Victoria would adopt the use of drones or satellite imagery as a routine method for monitoring crop health in the future, the probability of detection for crop pests and diseases might change, as well as the way how surveillance is done in general.
Conclusion
This study combined a comprehensive survey of Crop Safe agronomists, who undertake general surveillance for early detection of exotic grain pests and diseases, with an elicitation expert workshop to compare agronomists’ self-rated confidence in detecting pests and diseases with the perception of detection probability in the grains industry. Our work improves the current understanding of visual inspection methods applied in the grains industry in Victoria. It also provides quantitative estimates of visual detection probabilities that biosecurity managers have adopted in their estimates of pest status of exotic grain pests and diseases.
The structured IDEA protocol applied in this study proved effective and practical in reducing an important knowledge gap around the sensitivity of the surveillance program. It was a cost-effective alternative to resource intensive detection experiments which cannot be conducted easily with pests and diseases that are absent in Australia.
Data availability statement
The raw data supporting the conclusions of this article will be made available by the authors, without undue reservation.
Ethics statement
Ethical review and approval was not required for the study on human participants in accordance with the local legislation and institutional requirements. The patients/participants provided their written informed consent to participate in this study.
Author contributions
TK, MM, LR, and SB contributed to conception and design of the study. SL performed the statistical analysis. EA wrote the first draft of the manuscript. All authors contributed to manuscript revision, read, and approved the submitted version.
Funding
The Australian Government Department of Agriculture, Water and the Environment and the Victorian Department of Jobs, Precincts and Regions jointly funded this project.
Acknowledgments
We would like to thank the Australian Government Department of Agriculture, Water and the Environment and the Victorian Department of Jobs, Precincts and Regions for funding this project. We would also like to thank Crop Safe coordinators Kellyanne Harris, Dale Gray, and Dale Boyd, diagnostician Luise Fanning and agronomists who responded to the survey and the group of experts who participated in the expert elicitation workshop. We are also grateful to Anca Hanea for her input into the statistical analysis of workshop results.
Conflict of interest
The authors declare that the research was conducted in the absence of any commercial or financial relationships that could be construed as a potential conflict of interest.
Publisher’s note
All claims expressed in this article are solely those of the authors and do not necessarily represent those of their affiliated organizations, or those of the publisher, the editors and the reviewers. Any product that may be evaluated in this article, or claim that may be made by its manufacturer, is not guaranteed or endorsed by the publisher.
Supplementary material
The Supplementary Material for this article can be found online at: https://www.frontiersin.org/articles/10.3389/fevo.2022.968436/full#supplementary-material
References
Adams-Hosking, C., McBride, M. F., Baxter, G., Burgman, M., de Villiers, D., Kavanagh, R., et al. (2016). Use of expert knowledge to elicit population trends for the koala (Phascolarctos cinereus). Divers. Distrib. 22, 249–262. doi: 10.1111/ddi.12400
Anderson, C., Low-Choy, S., Whittle, P., Taylor, S., Gambley, C., Smith, L., et al. (2017). Australian plant biosecurity surveillance systems. Crop Prot. 100, 8–20. doi: 10.1016/j.cropro.2017.05.023
Bailey, L. L., Simons, T. R., and Pollock, K. H. (2004). Estimating site occupancy and species detection probability parameters for terrestrial salamanders. Ecol. Appl. 14, 692–702. doi: 10.1890/03-5012
Barry, S. C., Caley, P., Liu, S., Paini, D. R., Carey, J., and Clark, G. (2015). Development of an Expert-Based Model for Improved Biofouling Risk Assessment. Canberra: CSIRO.
Budescu, D. V., and Chen, E. (2015). Identifying expertise to extract the wisdom of crowds. Manag. Sci. 61, 267–280. doi: 10.1287/mnsc.2014.1909
Burgman, M. (2015). “What leads experts astray?,” in Trusting Judgements: How to Get the Best Out of Experts, ed. M. A. Burgman (Cambridge, MA: Cambridge University Press), 44–86. doi: 10.1017/CBO9781316282472.004
Cacho, O., Reeve, I., Tramell, J., and Hester, S. (2012). Valuing Community Engagement in Biosecurity Surveillance. Melbourne: Australian Centre of Excellence for Risk Analysis.
Camac, J., Clarke, S., Niranjane, A., and de Majnik, J. (2019). Estimating Probabilities of Pest Absence Across Geographic Space. Parkville: The University of Melbourne.
Cameron, A. R., and Baldock, C. F. (1998). A new probability formula for surveys to substantiate freedom from disease. Prev. Vet. Med. 34, 1–17. doi: 10.1016/S0167-5877(97)00081-0
Epanchin-Niell, R. S. (2017). Economics of invasive species policy and management. Biol. Invasions 19, 3333–3354. doi: 10.1007/s10530-017-1406-4
Epanchin-Niell, R. S., Brockerhoff, E. G., Kean, J. M., and Turner, J. A. (2014). Designing cost-efficient surveillance for early detection and control of multiple biological invaders. Ecol. Appl. 24, 1258–1274. doi: 10.1890/13-1331.1
Froessling, J., Wahlstroem, H., Agren, E. C. C., Cameron, A., Lindberg, A., and Lewerin, S. S. (2013). Surveillance system sensitivities and probability of freedom from (Mycobacterium avium) subsp. (paratuberculosis) infection in Swedish cattle. Prev. Vet. Med. 108, 47–62. doi: 10.1016/j.prevetmed.2012.07.010
Garrard, G. E., Bekessy, S. A., McCarthy, M. A., and Wintle, B. A. (2008). When have we looked hard enough? A novel method for setting minimum survey effort protocols for flora surveys. Austral Ecol. 33, 986–998. doi: 10.1111/j.1442-9993.2008.01869.x
Garrard, G. E., McCarthy, M. A., Williams, N. S. G., Bekessy, S. A., and Wintle, B. A. (2013). A general model of detectability using species traits. Methods Ecol. Evol. 4, 45–52. doi: 10.1111/j.2041-210x.2012.00257.x
Gustafson, L. L., Gustafson, D. H., Antognoli, M. C., and Remmenga, M. D. (2013). Integrating expert judgement in veterinary epidemiology: example guidance for disease freedom surveillance. Prev. Vet. Med. 109, 1–9. doi: 10.1016/j.prevetmed.2012.11.019
Hammond, N. E. B., Hardie, D., Hauser, C. E., and Reid, S. A. (2016). Can general surveillance detect high priority pests in the Western Australian grains industry? Crop Prot. 79, 8–14. doi: 10.1016/j.cropro.2015.10.004
Hanea, A. M., McBride, M. F., Burgman, M. A., Wintle, B. C., Fidler, F., Flander, L., et al. (2017). Investigate discuss estimate aggregate for structured expert judgement. Int. J. Forecast. 33, 267–279. doi: 10.1016/j.ijforecast.2016.02.008
Hauser, C. E., Garrard, G. E., and Moore, J. L. (2015). “Estimating detection rates and probabilities,” in Biosecurity surveillance. Quantitative approaches, eds F. Jarrad, S. Low-Choy, and K. Mengersen (Oxfordshire: CAB International), 151–166. doi: 10.1079/9781780643595.0151
Hemming, V., Walshe, T. V., Hanea, A. M., Fidler, F., and Burgman, M. A. (2018b). Eliciting improved quantitative judgements using the IDEA protocol: a case study in natural resource management. PLoS One 13:e0198468. doi: 10.1371/journal.pone.0198468
Hemming, V., Burgman, M. A., Hanea, A. M., McBride, M. F., and Wintle, B. C. (2018a). A practical guide to structured expert elicitation using the IDEA protocol. Methods Ecol. Evol. 9, 169–180. doi: 10.1111/2041-210X.12857
Hester, S., and Cacho, O. (2012). Optimization of search strategies in managing biological invasions: a simulation approach. Hum. Ecol. Risk Assess. 18, 181–199. doi: 10.1080/10807039.2012.632307
Hester, S., Sergeant, E., Robinson, A. P., and Schultz, G. (2015). “Animal, vegetable, or.? A case study in using animal-health monitoring design tools to solve a plant-health surveillance problem,” in Biosecurity Surveillance. Quantitative Approaches, eds F. Jarrad, S. Low-Choy, and K. Mengersen (Oxfordshire: CAB International), 313–333. doi: 10.1079/9781780643595.0313
Hester, S. M., and Cacho, O. J. (2017). The contribution of passive surveillance to invasive species management. Biol. Invasions 19, 737–748. doi: 10.1007/s10530-016-1362-4
Hester, S. M., Hauser, C. E., and Kean, J. M. (2017). “Tools for designing and evaluating post-border surveillance systems,” in Invasive species. Risk Assessment and Management, eds A. P. Robinson, T. Walshe, M. A. Burgman, and M. Nunn (Cambridge, MA: Cambridge University Press). doi: 10.1017/9781139019606.003
IPPC (2016). Detection of Russian Wheat Aphid (Diuraphis noxia) in South Australia and Victoria. Rome: International Plant Protection Convention.
IPPC (2017). International Standard for Phytosanitary Measures (ISPM) 4. Requirements for the Establishment of Pest Free Areas. Rome: International Plant Protection Convention.
Jiménez-Díaz, R. M., Castillo, P., del Mar Jiménez-Gasco, M., Landa, B. B., and Navas-Cortés, J. A. (2015). Fusarium wilt of chickpeas: biology, ecology and management. Crop Prot. 73, 16–27. doi: 10.1016/j.cropro.2015.02.023
Kalaris, T., Fieselmann, D., Magarey, R., Colunga-Garcia, M., Roda, A., Hardie, D., et al. (2014). “The role of surveillance methods and technologies in plant biosecurity,” in The Handbook of Plant Biosecurity, eds G. Gordh and S. McKirdy (Dordrecht: Springer), 309–337. doi: 10.1007/978-94-007-7365-3_11
Leung, B., Lodge, D. M., Finnoff, D., Shogren, J. F., Lewis, M. A., and Lamberti, G. (2002). An ounce of prevention or a pound of cure: bioeconomic risk analysis of invasive species. Proc. R. Soc. B Biol. Sci. 269, 2407–2413. doi: 10.1098/rspb.2002.2179
Magarey, R. D., Colunga-Garcia, M., and Fieselmann, D. A. (2009). Plant biosecurity in the United States: roles, responsibilities, and information needs. BioScience 59, 875–884. doi: 10.1525/bio.2009.59.10.9
Martin, P. A. J., Cameron, A. R., and Greiner, M. (2007). Demonstrating freedom from disease using multiple complex data sources 1: a new methodology based on scenario trees. Prev. Vet. Med. 79, 71–97. doi: 10.1016/j.prevetmed.2006.09.008
Martin, T. (2017). “Surveillance for detection of pests and diseases: how sure can we be of their absence?,” in Invasive Species. Risk Assessment and Management, eds A. P. Robinson, T. Walshe, M. A. Burgman, and M. Nunn (Cambridge, MA: Cambridge University Press), 348–384. doi: 10.1017/9781139019606.018
Mogili, R. U. M., and Deepak, B. B. V. L. (2018). Review on application of drone systems in precision agriculture. Proc. Comput. Sci. 133, 502–509. doi: 10.1016/j.procs.2018.07.063
PHA (2013). National Plant Biosecurity Surveillance Strategy 2013-2020. Version 1.0 May 2013. Canberra: Plant Health Australia.
PHA (2020). Government and Plant Industry Cost Sharing Deed in Respect of Emergency Plant Pest Responses. Canberra: Plant Health Australia.
Puri, V., Nayyar, A., and Raja, L. (2017). Agriculture drones: a modern breakthrough in precision agriculture. J. Stat. Manag. Syst. 20, 507–518. doi: 10.1080/09720510.2017.1395171
R Core Team (2018). R: A Language and Environment for Statistical Computing. Vienna: R Foundation for Statistical Computing.
Raza, M. M., Harding, C., Liebman, M., and Leandro, L. F. (2020). Exploring the potential of high-resolution satellite imagery for the detection of soybean sudden death syndrome. Remote Sens. 12:1213. doi: 10.3390/rs12071213
Runge, M. C., Converse, S. J., and Lyons, J. E. (2011). Which uncertainty? Using expert elicitation and expected value of information to design an adaptive program. Biol. Conserv. 144, 1214–1223. doi: 10.1016/j.biocon.2010.12.020
Stehr, N. J. (2015). Drones: the newest technology for precision agriculture. Nat. Sci. Educ. 44, 89–91. doi: 10.4195/nse2015.04.0772
Surowiecki, J. (2004). The Wisdom of Crowds: Why the Many are Smarter Than the Few and How Collective Wisdom Shapes Business, Economies, Societies, and Nations. London: Little Brown.
Whittle, P., Jarrad, F., and Mengersen, K. (2013). Design of the quarantine surveillance for non-indigenous species of invertebrates on Barrow Island. Rec. West. Aust. Mus. Suppl. 83, 113–130. doi: 10.18195/issn.0313-122x.83.2013.113-130
Wittmann, M. E., Cooke, R. M., Rothlisberger, J. D., Rutherford, E. S., Zhang, H., Mason, D. M., et al. (2014). Use of structured expert judgment to forecast invasions by bighead and silver carp in Lake Erie. Conserv. Biol. 29, 187–197. doi: 10.1111/cobi.12369
Wright, D., MacLeod, B., Hammond, N., and Longnecker, N. (2016). Can grain growers and agronomists identify common leaf diseases and biosecurity threats in grain crops? An Australian example. Crop Prot. 89, 78–88. doi: 10.1016/j.cropro.2016.07.005
Keywords: general surveillance, detection probability, biosecurity, expert judgment, grain agronomists
Citation: Arndt E, Rumpff L, Lane S, Bau S, Mebalds M and Kompas T (2022) Estimating probability of visual detection of exotic pests and diseases in the grains industry—An expert elicitation approach. Front. Ecol. Evol. 10:968436. doi: 10.3389/fevo.2022.968436
Received: 14 June 2022; Accepted: 01 November 2022;
Published: 18 November 2022.
Edited by:
Heleen Kruger, Department of Agriculture, Water and the Environment, AustraliaReviewed by:
Samniqueka J. Halsey, University of Missouri System, United StatesMarta Rodríguez-Rey, University of Alcalá, Spain
Copyright © 2022 Arndt, Rumpff, Lane, Bau, Mebalds and Kompas. This is an open-access article distributed under the terms of the Creative Commons Attribution License (CC BY). The use, distribution or reproduction in other forums is permitted, provided the original author(s) and the copyright owner(s) are credited and that the original publication in this journal is cited, in accordance with accepted academic practice. No use, distribution or reproduction is permitted which does not comply with these terms.
*Correspondence: Edith Arndt, edith.arndt@unimelb.edu.au
†ORCID: Edith Arndt, orcid/0000-0002-1850-2063; Libby Rumpff, orcid/0000-0001-9400-8086; Stephen Lane, orcid/0000-0002-4848-613X; Sana Bau, orcid/0000-0002-7586-0959; Martin Mebalds, orcid/0000-0002-5894-6865; Tom Kompas, orcid/0000-0002-0665-0966