- 1Environmental and Life Sciences Department, Trent University, Peterborough, ON, Canada
- 2Landscape Science and Technology Division, Environment and Climate Change Canada, Ottawa, ON, Canada
- 3Reproductive Sciences Unit, Toronto Zoo, Toronto, ON, Canada
- 4Saskatchewan Ministry of Environment, Regina, SK, Canada
Density is an important demographic parameter that is commonly overlooked in studies of wild populations. Here, we examined the effects of variable spatially explicit density on a range of demographic parameters in a wild population of a cryptic ungulate, boreal woodland caribou (Rangifer tarandus caribou). Using non-invasive genetic sampling, we applied spatial capture–recapture methods with landscape covariates to estimate the density of boreal woodland caribou across a 108,806 km2 study area. We then created a familial network from the reconstructed parent–offspring relationships to determine whether spatial density influenced sex-specific individual reproductive success, female pregnancy status, and dispersal distance. We found that animal density varied greatly in response to land cover types and disturbance; animal density was most influenced by landscape composition and distance to roads varying from 0 in areas with >20% deciduous cover to 270 caribou per 1,000 km2 in areas presenting contiguous older coniferous cover. We found that both male and female reproductive success varied with density, with males showing a higher probability of having offspring in higher-density areas, and the opposite for females. No differences were found in female pregnancy rates occurring in high- and low-density areas. Dispersal distances varied with density, with offspring moving shorter distances when parents were found in higher-density areas. Familial networks showed lower-closeness centrality and lower-degree centrality for females in higher-density areas, indicating that females found in higher-density areas tend to be less broadly associated with animals across the range. Although high-density areas do reflect good-quality caribou habitat, the observed decreased closeness and degree centrality measures, dispersal rates, and lower female recruitment rates suggest that remnant habitat patches across the landscape may create population sinks.
Introduction
In most vertebrate species, population density is heterogeneous across a species’ range, reflecting patterns resulting from an individual’s selective use of habitat resources to improve fitness (Morris, 2003), and in response to habitat disturbance (Tischendorf et al., 2005). Variations in habitat quality can influence population density, movement, and overall landscape connectivity by altering resource abundance and habitat structure (Łomnicki, 1980; Smith et al., 2016). Habitat fragmentation can also lead to an increase or decrease in population density in remnant habitat patches, shifting the balance between emigration and immigration (Bowers and Matter, 1997; Tischendorf et al., 2005).
Density dependence occurs when a population parameter (most often population dynamics such as population growth rate, vital rates, and reproduction) varies as a result of density (Hixon and Johnson, 2009). In ungulates, density dependence is observed through changes in vital rates, such that increased population density results in decreased female reproductive success through reduced pregnancy rate, decreased survival of young, and increased age of first reproduction (Gaillard et al., 2000; Bowyer et al., 2014). McLoughlin et al. (2006) found that the lifetime reproductive success of female red deer (Cervus elaphus) was inversely associated with local density, and reproductive benefits from selecting good-quality habitat decreased as density increased. McCullough (1999) has also shown that increased density can lead to increased reproductive success for males due to the greater number of available females, leading to greater individual variation in male reproductive success.
Understanding and accounting for individual heterogeneity in reproductive success is fundamental when making inferences about ecological patterns and processes, bearing in mind that inherent reproductive differences among individuals can lead to incorrect interpretations (Weladji et al., 2008; Badger et al., 2020). Individual differences in reproductive success are especially pronounced in long-lived species (Clutton-Brock and Sheldon, 2010), and this variation can have important consequences for population dynamics and demography (Clutton-Brock, 1988; Newton, 1989; Clutton-Brock and Sheldon, 2010). Accurately assessing individual variation in reproductive success and recruitment is critical for improving our understanding of mating systems and sexual selection (Bowler and Benton, 2005) and the impact of local conditions such as habitat composition and fragmentation on the species.
In addition to reproductive success, there is growing evidence that dispersal is a highly heterogeneous process (Bowler and Benton, 2005; Fronhofer et al., 2018), dependent on multiple factors such as resource availability and habitat quality (Aguillon and Duckworth, 2015), predation risk (Bestion et al., 2014), and density (Matthysen, 2005; Bitume et al., 2013). Density-dependent dispersal has been shown in many taxa and can be positive or negative depending on the driving mechanism (Matthysen, 2005). Positive density-dependent dispersal can be a result of local competition increasing the likelihood of individuals dispersing to improve fitness prospects by leaving high-density areas (Travis et al., 1999; Matthysen, 2005), while negative density-dependent dispersal can result from high densities reducing dispersal probability due to increased likelihood of safety from herding or mutual attraction (Matthysen, 2005). Most studies on density-dependent dispersal have shown that patches of high density resulting from habitat fragmentation result in increased dispersal (positive density dependence; Travis et al., 1999; Poethke and Hovestadt, 2002; Leturque and Rousset, 2003), with few studies showing decreased dispersal rates (negative density dependence; Matthysen, 2005).
Boreal woodland caribou (Rangifer tarandus caribou, hereafter referred to as boreal caribou) in Saskatchewan, Canada provides an ideal system for testing demographic density responses hypotheses as this population is sedentary, exhibiting relatively small-scale movements, and occur over large areas with no discrete populations (Ball et al., 2010; Priadka et al., 2019; McFarlane et al., 2021). They select large tracks of mature to old-growth coniferous forests that provide abundant lichens, or wetlands mixed with upland areas, and avoid early-stage, successional deciduous forests (Stuart-Smith et al., 1997; Environment Canada, 2012). Habitat alteration through anthropogenic disturbance leads to habitat loss for boreal caribou through the conversion of old-growth coniferous forests to early-stage deciduous forests (Polfus et al., 2011; Rudolph et al., 2017). Roads and linear features facilitate predator movement, affecting calf and adult survival (Dussault et al., 2012; Leblond et al., 2013). To investigate these factors on density and density response, we first used noninvasive genetic sampling and spatial capture–recapture (SCR) modeling to generate a spatially explicit caribou density raster layer using landscape covariates to create a heterogeneous density surface. Using the same genetic data, we constructed familial networks and calculated individual recruitment and dispersal rates along with a range of networks metrics to assess the contribution of individual boreal caribou to the population (McFarlane et al., 2021; Jones and Manseau, 2022). We have previously shown spatial variation in individual measures of network centrality (a node’s position within a network; Jones and Manseau, 2022) across Saskatchewan, with animals in the southern part of their range presenting higher numbers of parent–offspring relationships, along with connections to other highly connected individuals (McFarlane et al., 2021). In this study, we extend these analyses to examine the relationship between familial network metrics and density, a novel approach of testing the effects of density variation on population demographics.
Our hypothesis is that boreal caribou exhibit density dependence at the local level, with spatial variation in density leading to significant heterogeneity in demographic responses (Figure 1). Female reproductive success and pregnancy status are predicted to be negatively correlated with density, and male reproductive success is predicted to be positively correlated with density. The pregnancy rate was also measured from each sampled female to assess whether the lower reproductive success predicted in higher-density areas corresponds to lower pregnancy rates, which would suggest that females found in higher-density areas are in poor body condition or have limited access to food (Gerhart et al., 1996; Stewart et al., 2005). Dispersal is further predicted to be negatively correlated with density for both sexes, with a stronger influence on dispersal in females, as most polygynous mammal species have males as the dispersing sex and females as being philopatric (Greenwood, 1980; Wolff, 1997). Little is known about sex-specific dispersal in boreal caribou; radio-telemetry collaring is the most commonly used method for estimating the movement of caribou and males are rarely collared (e.g., van Oort et al., 2011). The aforementioned predictions have seldom been tested in wild populations of long-lived mammal species, but the increasing availability of genetic data and advanced analytical methods, applied in this study, are providing a unique opportunity to uncover fine-scale population demographic responses to landscape conditions.
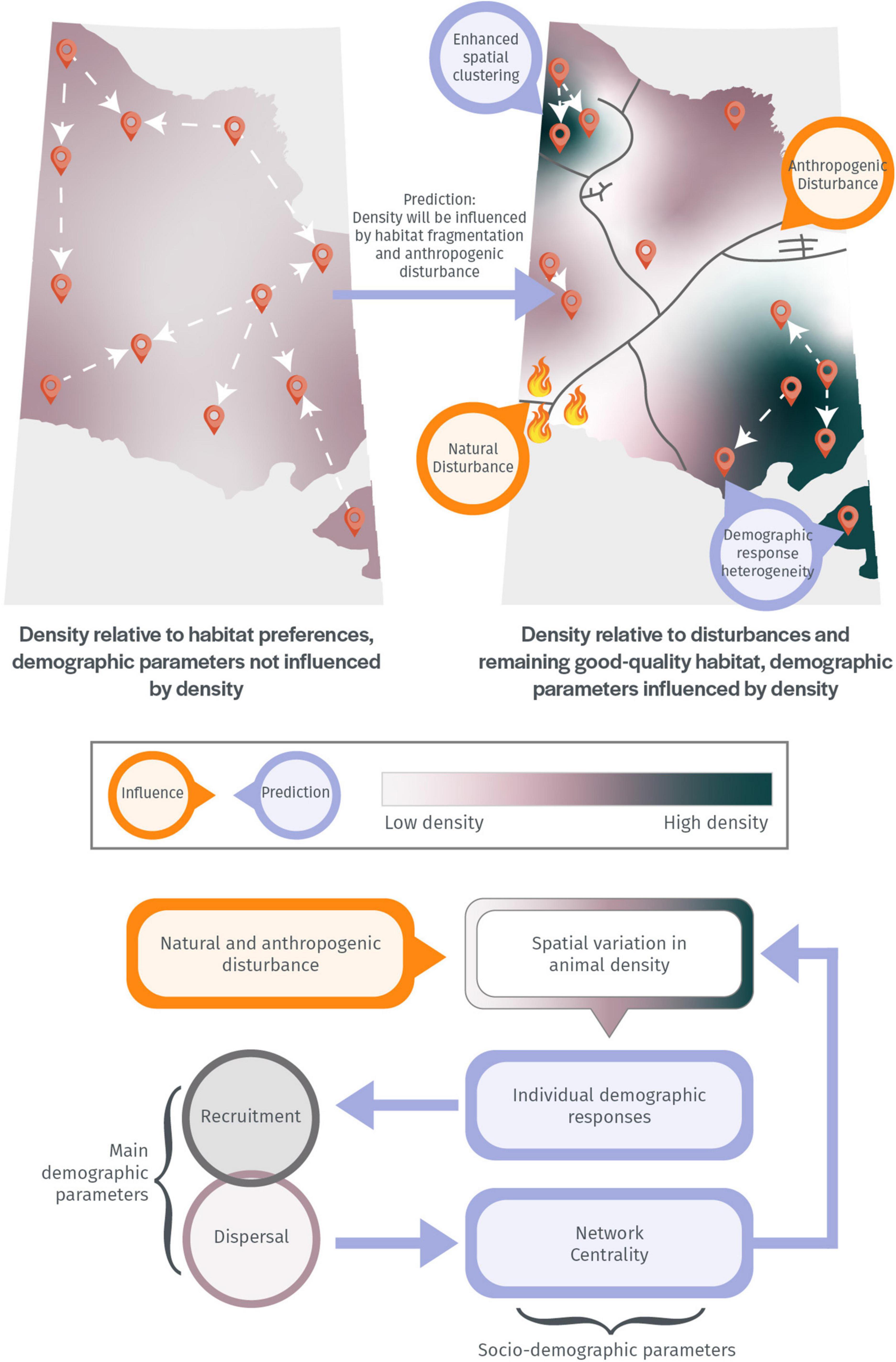
Figure 1. The effects of variable spatial density on demographic parameters. Points on the map represent individuals, and arrows represent the direction of movement of individuals.
Materials and methods
Study area
Data were collected from boreal caribou across the boreal plains ecozone in Saskatchewan, Canada (Supplementary Figure 1.1). The boreal plains are characterized by mixed-wood forests, lakes, and large areas of low-lying peatlands (Saskatchewan Ministry of Environment, 2019). Boreal caribou are part of the boreal caribou Designatable Unit (DU6) (COSEWIC, 2011); they are listed as Threatened under the Canadian Species at Risk Act (Government of Canada, 2002) and as Vulnerable in Saskatchewan (SKCDC, 2020). Due to relatively high levels of anthropogenic disturbance (Supplementary Figure 1.2), boreal caribou populations across Saskatchewan’s boreal plains are at a higher risk of decline and potential extirpation due to high levels of habitat loss and fragmentation, particularly along the southern range margin (Arsenault, 2003; Environment Canada, 2011). See Supplementary Appendix 1 for more details on the study area.
Data collection and analysis
Following the aerial survey protocol outlined in Hettinga et al. (2012), aerial transects were systematically flown at 3 km intervals across each survey area using rotary- or fixed-wing aircraft, or a combination of both, to locate boreal caribou feeding locations. Following the survey, feeding sites were accessed by rotary aircraft and sampled. Systematic surveys were conducted across different regions of the boreal plains for population genetic surveys between 2013 and 2016 (Table 1; Priadka et al., 2019). For the SK Central, SK East, and SK West areas (Supplementary Figure 1.1), two systematic surveys per winter were conducted to allow for the estimation of demographic parameters, with sampling occasions spaced approximately one month apart (Table 1). Fecal samples collected in the surveys were kept frozen at –20°C until DNA extraction was performed. We followed the DNA extraction protocol outlined by Ball et al. (2007) to generate individual-specific genetic profiles. To generate familial pedigree networks, we amplified samples from unique genotypes at 15 variable microsatellite loci (McFarlane et al., 2021).
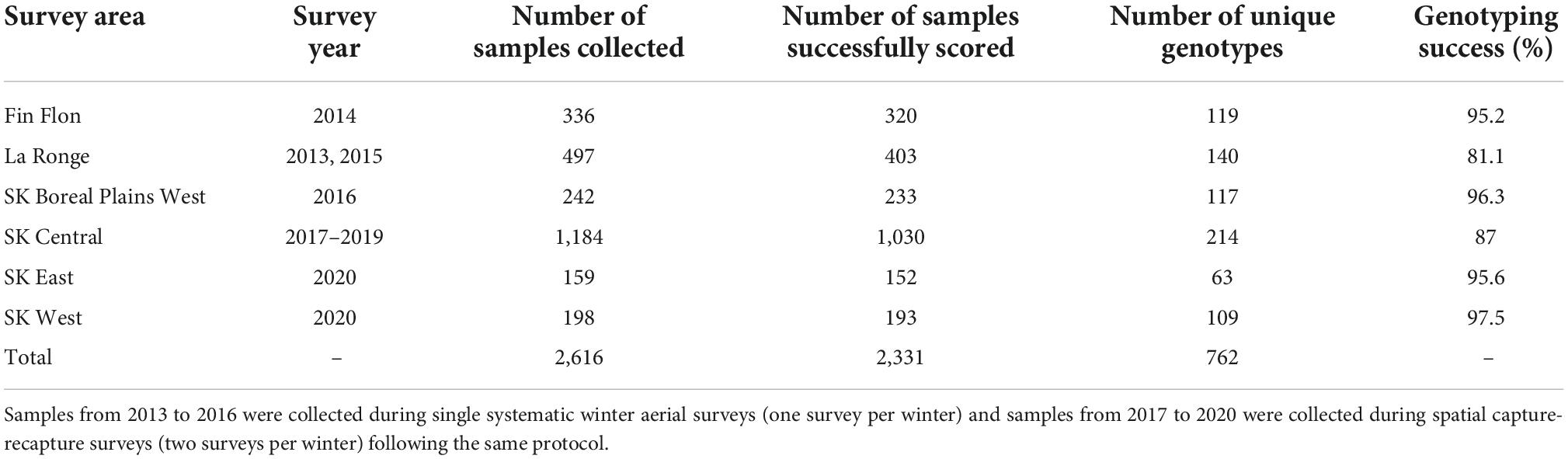
Table 1. Fecal pellet sampling data collected from boreal caribou across the boreal plains ecozone in Saskatchewan, Canada.
Analyses
Density
We used spatial capture–recapture (SCR) models to generate a spatially explicit density estimate of boreal caribou across the Saskatchewan boreal plains ecozone. We created a multi-session SCR model with one session for each survey area (SK West, SK Central, and SK East) to obtain a density estimate across survey areas. We used a maximum likelihood approach implemented in the R package secr (Efford, 2018; R Core Team, 2019). The density model allows for the input of spatial covariates to create a heterogeneous density surface across the study area. We used three groups of spatial covariates to investigate the factors that affect population density and to obtain a spatially explicit density surface: habitat (good and poor quality), distance to anthropogenic disturbance [as (1) all linear features including roads and (2) roads only], and habitat potential (Supplementary Appendix 1). Since the distance to linear features includes roads, we ran separate models that included either all linear features or roads only along with other spatial covariates. We computed Pearson’s correlation coefficient between all spatial covariates using the raster package (Hijmans, 2022) in R. Spatial covariates that were significantly correlated were not used in the same density model (Supplementary Appendix 1). Habitat data were extracted from the 2015 Land Cover of Canada dataset at 30 m resolution (Natural Resources Canada, 2020). We created a spatial layer representing the proportion of each land cover class at a spatial resolution of 1 km2 using a moving window. All proportions of land cover types ranged from 0 to 100%, and land cover classes were added together to develop good-quality and poor-quality habitat groupings. Based on results from resource selection analyses (Boyce and McDonald, 1999; Manly et al., 2002), we considered needleleaf forests as good-quality habitat, deciduous and mixed-wood forests (hereafter referred to as deciduous), and grasses and shrubs as poor-quality habitat. The linear feature layer included roads, railways, trails, seismic lines, protection structures (dikes, levees, etc.), pipelines, and power lines. We tested the effects of linear features by creating a distance to roads variable and a distance to all linear features variable. Finally, we used ranked boreal caribou habitat potential data to account for an ecosite’s potential to provide forage, refuge, and calving habitat (Saskatchewan Ministry of Environment, 2019). Ecosites were ranked by a panel of biologists with expertise in boreal caribou habitat use in Saskatchewan. We then calculated the median proportion of each land cover type and habitat potential within a 12 km radius of each detection location, based on the larger of the estimated home range radii for females (∼12 km) and males (∼6 km) calculated from the SK Central population density estimation without any covariates (S. McFarlane, unpublished data).
We estimated the parameters of the SCR detection function (g0 and σ) by maximizing the conditional likelihood and derived density from the top AICC-ranked models (Akaike, 1974; Anderson et al., 1994; Borchers and Efford, 2008). We used the hazard exponential form of the detection function, as area search data models the cumulative hazard of detection (Efford, 2011). Models assumed that, populations were demographically closed during sampling, and detections were independent, conditional on activity centers (Efford, 2004; Borchers and Efford, 2008). We first fit a series of detection models to the data using a homogeneous density surface (D∼1) with g0 and σ influenced by time (t), site-specific (k, site-learned response; site effectiveness changes once any animal is captured), and behavioral (bk, animal × site-learned response, and site-specific step change) responses (unpublished data). We used the top detection model (λ t + bk σ t + bk) in our subsequent density model runs. See Supplementary Appendix 2 for more information on density modeling and R code.
We used the relationships between density and spatial covariates from the top-ranked density model to create a spatial density surface and applied the density–covariate relationship across the entire boreal plains ecozone. We constrained this to the boreal plains ecozone as an ecological unit with distinct biotic and abiotic features (Marshall et al., 1999). We calculated the median population density (per ha) within a 12 km radius of each sampling location using the same method as mentioned previously for the spatial covariates. The resulting density surface was used to estimate the influence of density on sex-specific network metrics, dispersal distance, individual reproductive success, and female pregnancy status.
Reconstruction of familial relationships
We identified boreal caribou families by reconstructing parent–offspring relationships using COLONY v2.0.6.5 (Jones and Wang, 2010). COLONY uses a full-likelihood method for sibship inference and parentage assignment, assigning all sampled offspring to hypothetical maternal and paternal families (Wang, 2004). Input parameters were set to allow for female and male polygynous mating systems without inbreeding avoidance, and the probability of mothers or fathers being present in the sampled data set was set to 50% in the absence of other prior information. COLONY infers parental genotypes for missing parents; inferred parents are genotypes that are not included in the candidate parent samples, either because that individual was not captured during sampling, or that parent was no longer alive when sampling occurred, resulting in a family network with more individuals than were sampled. We used Cytoscape v3.7.2 (Shannon et al., 2003) to create a familial network from the reconstructed parent–offspring relationships identified by COLONY. Each individual has their parents and offspring identified by COLONY enabling a network to be created from the multigenerational relationships among individuals. We confirmed the direction of all parent–offspring dyads by comparing them to the full sibling and parent pair outputs provided by COLONY.
Network centrality
We used the protocol outlined by McFarlane et al. (2021) to identify individuals that are central to the network and used the R package CINNA (Ashtiani et al., 2018) to estimate individual node-based network centrality measures. Nodes represent individuals, while edges represent parent-to-offspring relationships. We quantified distinct aspects of network centrality using three node-based centrality measures: alpha, closeness, and degree centrality. Alpha centrality identifies individuals that are connected to other highly connected individuals, indicating indirect reproductive success, even for those with few direct connections (McFarlane et al., 2021). Reproductive output can be highly variable and asymmetrical (McFarlane et al., 2018), and alpha centrality can indicate if an individual is part of a large extended family and if they are connected to highly connected individuals. Degree centrality represents the number of edges connected to a node (Harary, 1969); in directed networks, degree centrality is defined based on the direction of the connection (in-degree and out-degree). For our familial networks, the measure reported includes both the in and out-degree centrality, which corresponds to the edges of the parents and the offspring of an individual (McFarlane et al., 2021). Closeness centrality has commonly been used to measure how fast information can spread from a given node to all other reachable nodes in a network (Latora and Marchiori, 2001). In familial networks, individuals with higher-closeness centrality come either from more connected families, or larger families with more generations captured during sampling. These three centrality measures capture different network characteristics and topology from the measure of individual influence in the alpha centrality, the direct familial connections in the degree centrality, and the well-connected nodes in the closeness centrality (Jones and Manseau, 2022).
Familial network and density
We ran sex-specific generalized linear models (GLMs) to determine the influence of density on the three individual network centrality measures using a Gaussian error structure. Each centrality measure was standardized between 0 and 1. To validate the familial network centrality measures used in these models (due to the lack of independence of data points present in network data), 10,000 random familial networks with a burn-in of 1,000 networks were generated using permutations (Jones and Manseau, 2022). Each network was created by swapping mothers or fathers and swapping offspring. As with the real data, each individual’s network metrics were recalculated using these permuted networks. All global models were recalculated for each of the 10,000 permuted networks. The sizes of the coefficients were then compared to the size of the global models based on the original data. A p-value was calculated as the proportion of times the observed value was more extreme than the permutated values (Farine, 2013). A network centrality measure effect was considered significant if this p-value was <0.05 and the coefficients’ confidence interval did not include zero.
Dispersal and density
To test for density-dependent dispersal, we ran sex-specific GLMs with a Gaussian error distribution to determine the influence of density at the parental site on offspring dispersal. Dispersal can be inferred when parents and offspring, reconstructed through sibship assignment, are found in different locations (e.g., Norman and Spong, 2015; Fountain et al., 2017). Although the actual dispersal route cannot be determined, recent gene flow and movement among locations can be inferred, and by applying this method to many pairs of relationships, the population’s dispersal patterns can be estimated (Escoda et al., 2017). We estimated dispersal as the distance between an offspring and their parents. We calculated Euclidean dispersal distances in the R package geosphere (Hijmans, 2019) for all parent–offspring dyads and calculated the difference between the minimum and maximum dispersal distances of parent–offspring dyads (Supplementary Figure 2.1), with parent and offspring locations defined as the location where their fecal pellets were collected during aerial surveys. We used the maximum dispersal distance between the parent–offspring dyads to represent offspring dispersal in the analyses. Using the maximum dispersal distance could potentially inflate dispersal estimates if both dispersal and home ranges are independent among individuals, and conversely could be underestimated if individuals had not yet dispersed.
Recruitment, pregnancy, and density
We ran sex-specific binomial GLMs to determine the influence of density on the probability of female and male boreal caribou having offspring using a binary classification of individual reproductive success (did or did not have offspring) calculated from the reconstructed parent–offspring relationships. To determine the pregnancy status of each female, we followed the protocol outlined by Flasko et al. (2017). A total of 460 samples were measured for fecal pregnane concentrations to determine the yearly female pregnancy status of 363 female caribou, using a threshold of 700 ng/g dry mass (Supplementary Table 5.1). We ran binomial GLMs to determine the influence of density on female pregnancy status.
Results
A total of 2,616 samples were collected and 2,331 were successfully scored (average success rate of 92.1%), resulting in the identification of 762 unique individuals (Table 1). In total, 455 females, 298 males, and nine individuals of unknown sex were identified. Overall, the average allele dropout rate was 0.0027%, and the average false allele rate was 0.01%.
Caribou density estimation
Recaptures, including recaptures within the same survey, were sufficient in all three study areas (ranging from 78 to 202 recaptures per study area; Table 2). Using a multi-session SCR model allowed us to increase the number of captures and recaptures used in the overall density model (Table 2). A total of 282 individual caribou were included in the multi-session SCR model with a total of 404 detections at 96 unique locations (Table 2). Median spatial recapture distances varied from 3.4 km in SK Central to 7.6 km in SK East (Supplementary Figure 2.2).
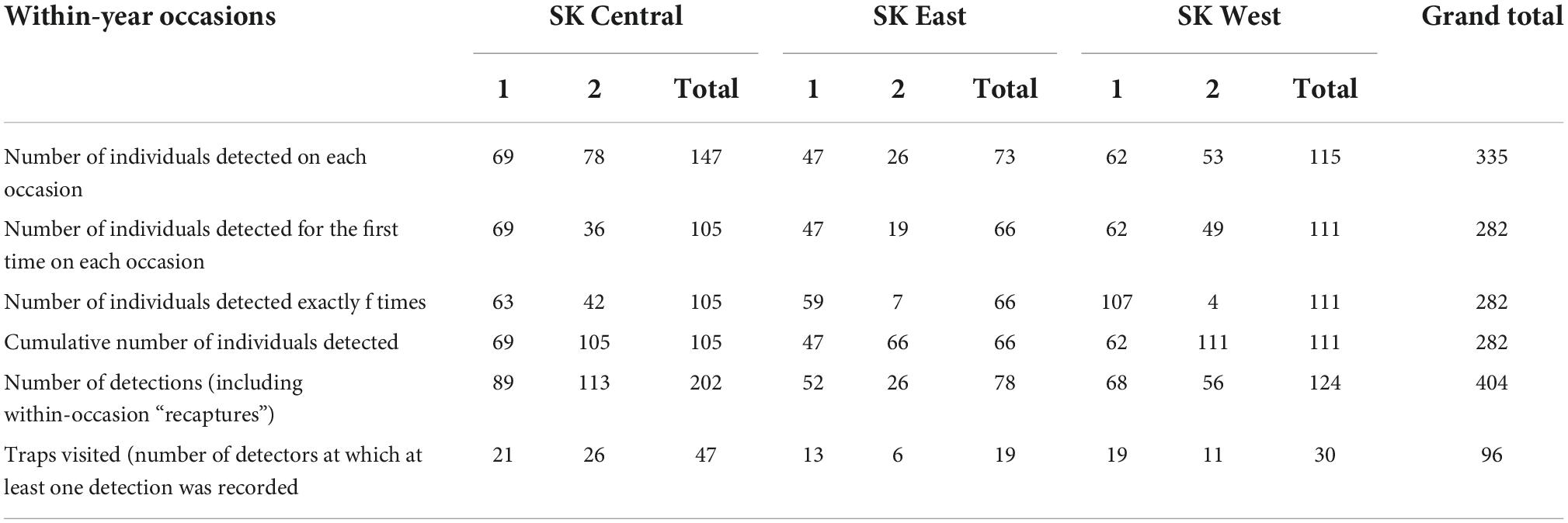
Table 2. Summary of spatial capture–recapture data used in the multi-spatial session model for estimating density.
Overall, our results show that boreal caribou densities are low and animals are spatially clustered, with an error below the 20% relative standard error threshold in all study areas (RSE = 11.5–13.9%), suggesting reasonably precise estimates. The top density model included a positive effect on good-quality habitat, a negative effect on poor-quality habitat, and a positive effect with greater distance to roads (Supplementary Table 2.2 and Figure 2). Although density was low overall, there was substantial variation across the range; ranging from 0 to 270 boreal caribou per 1,000 km2 (Figure 3). Distance to roads was a large contributor to the high variation in spatial density; large undisturbed areas far away from roads had the highest boreal caribou densities (Figures 2, 3). Poor-quality habitat was also a large driver of boreal caribou density, with density dropping to 0 in areas with >20% poor quality (deciduous) cover.
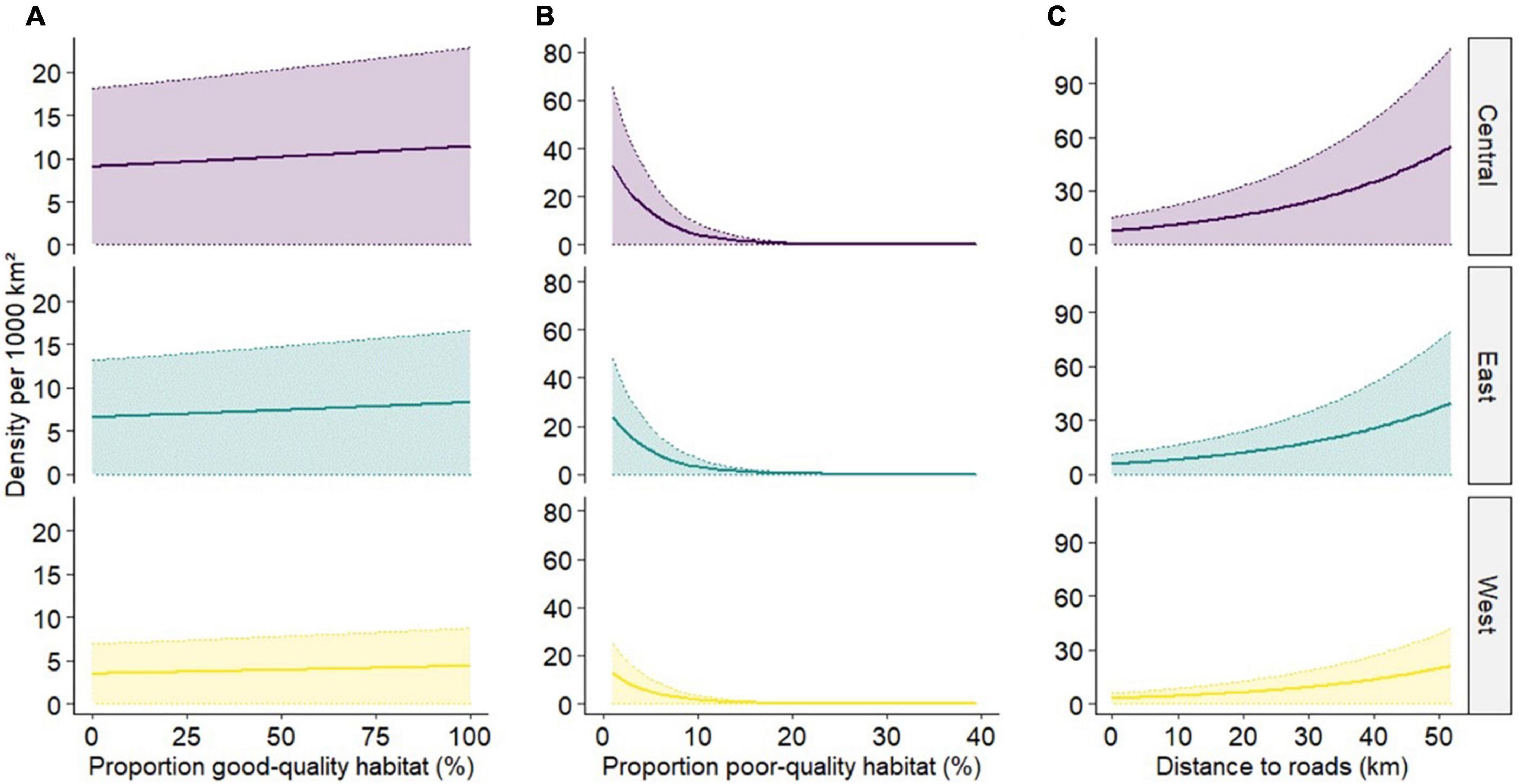
Figure 2. Predicted responses of the top density model showing the relationships between (A) good-quality habitat and caribou density, (B) poor-quality habitat and caribou density, and (C) distance to linear features and caribou density.
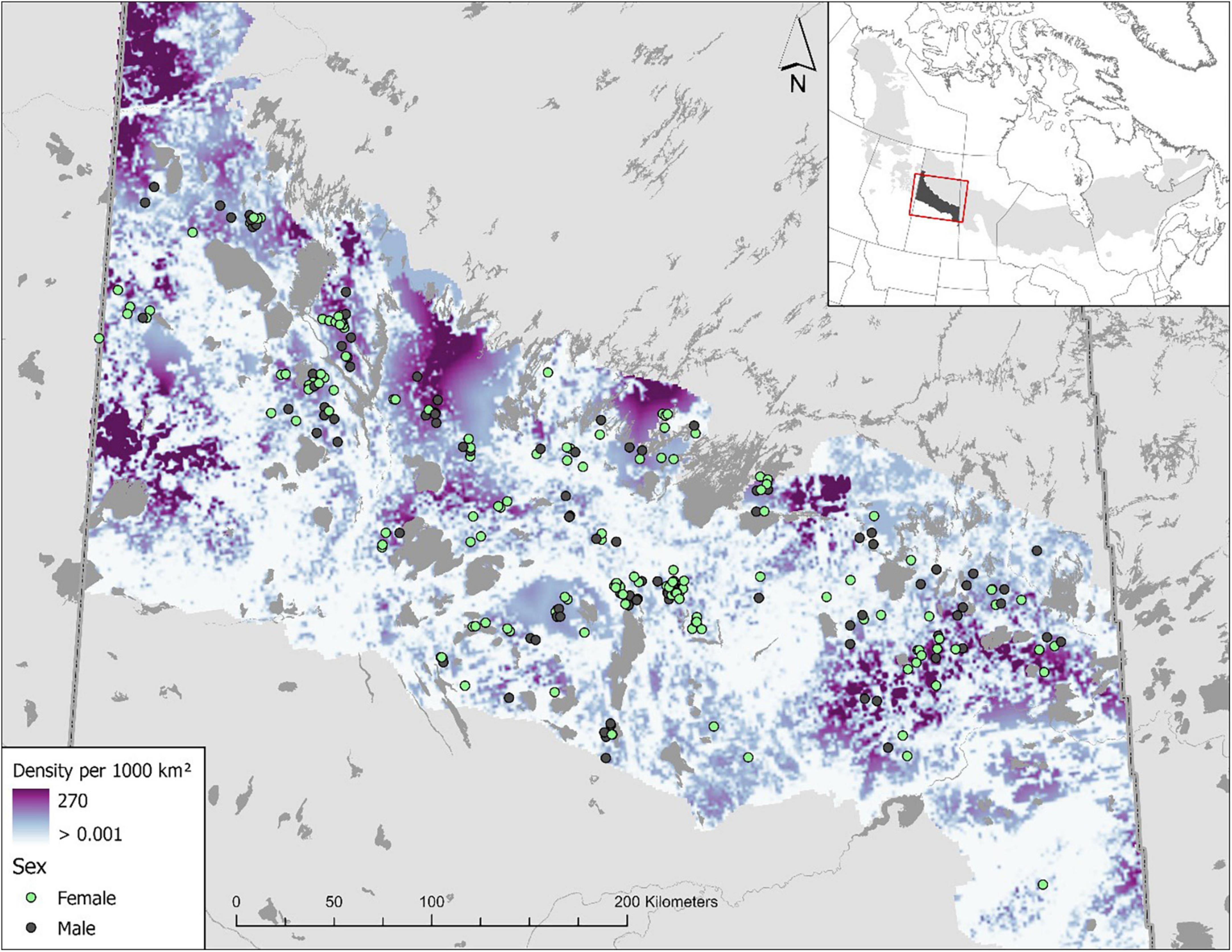
Figure 3. Spatially explicit boreal caribou density across the Saskatchewan boreal plains caribou range. Boreal caribou density was associated with good- and poor-quality habitat and linear disturbances. Densities are overall low and spatially clustered, greatest away from linear features and poor-quality habitat.
Familial network analysis
Pedigree reconstruction inferred an additional 227 females and 231 males, for a total familial network of 1,220 individuals (Supplementary Figure 3.1). In total, 305 females and 270 males were identified as parents (47% of all individuals). Comparison of the observed network to the 10,000 permuted networks indicated that females from lower-density areas have a higher-closeness centrality and higher-degree centrality than expected compared to a null model of random familial relationships (Supplementary Figure 4.1 and Table 3), indicating that females found in lower-density areas tend to be from larger families and more broadly associated to animals across the range. Conversely, we observed no pattern in degree and closeness centrality in response to density for males, and no pattern in alpha centrality in response to density for either males or females. Alpha centrality was not influenced by density for either sex. Alpha centrality represents an individual’s connection to other well-connected individuals, which gives an indirect indication of individual fitness, even if that individual does not have a lot of direct connections (offspring) themselves (McFarlane et al., 2021). Density, therefore, does not influence the connections of an individual to other highly connected individuals or large-extended family groups.
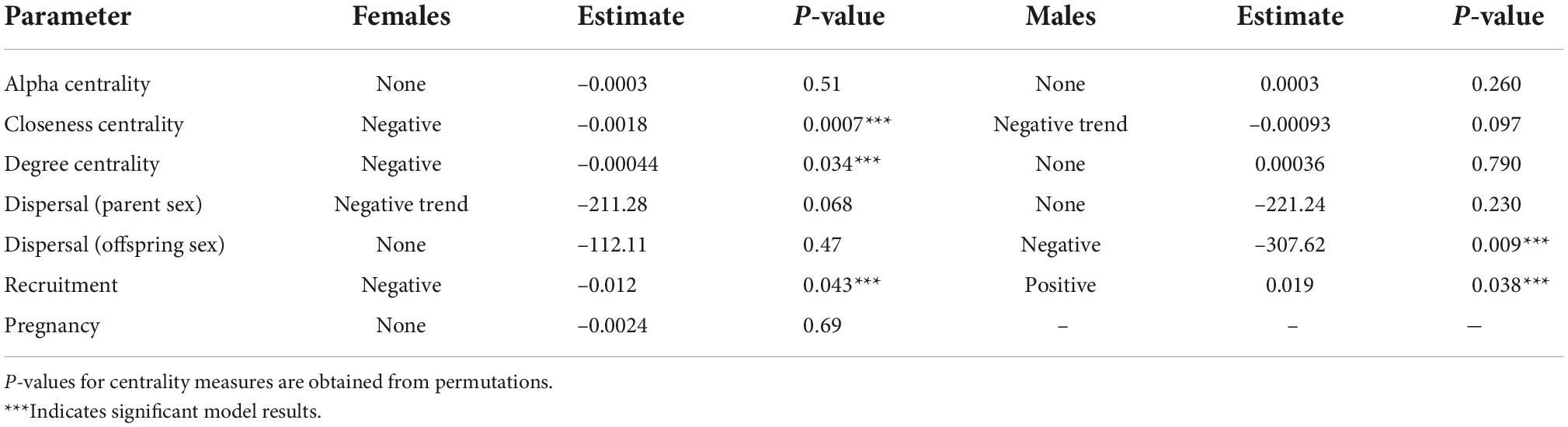
Table 3. Generalized linear model results for the effect of density on several demographic parameters.
Dispersal rates
In cases where an offspring was assigned a sampled mother or father, it was possible to infer offspring dispersal distance. Boreal caribou offspring dispersed primarily within short distances of their parents; 86% of offspring dispersal events were <40 km, and several offspring had a dispersal distance of 0 km, indicating that the offspring had not yet dispersed (Figure 4A). The largest dispersal distances were between fathers and their offspring (Figure 4B). The maximum dispersal distance between fathers and their offspring was 232 km with a mean of 22 km (±32 SD), while the maximum dispersal distance between mothers and their offspring was 152 km with a mean of 20 km (±25 SD). There were 35 parent–offspring pairs where both the parent and offspring were only located together, with 49% of these being mother–daughter pairs.
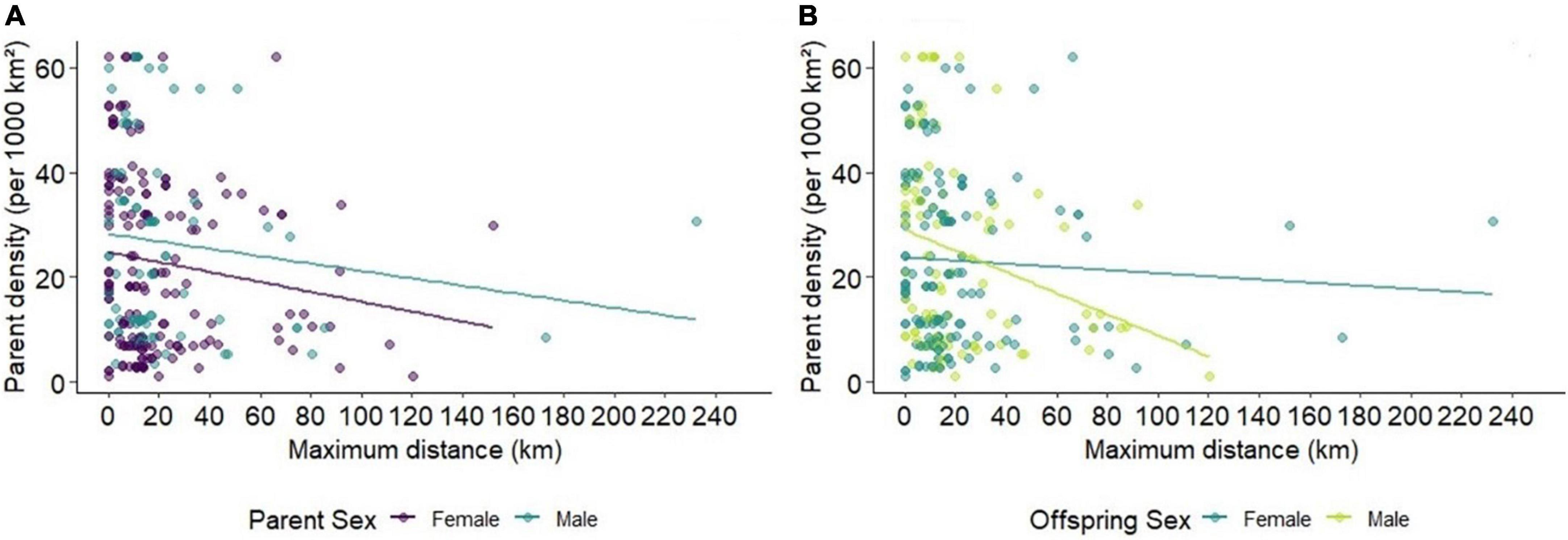
Figure 4. Frequency of maximum dispersal distances between parents and offspring by parent sex (A). Distribution of maximum dispersal distances versus density at the parent location by (A) parent sex and (B) offspring sex.
Caribou density at the parent location had no significant effect on dispersal when accounting for parental sex; however, a negative trend was identified for mothers and their offspring (p = 0.068; Table 3). When accounting for the sex of the dispersing offspring, the dispersal distance of male offspring was significantly and inversely related to parental density (Supplementary Table 3.1 and Figure 4B), while the dispersal of female offspring from their parents was not significantly related to parental density (Supplementary Table 3.1). Further investigation into sex differences in dispersal indicated that dispersal distance between mothers and male offspring was also significant (Supplementary Table 3.2), corresponding to the significant results for male offspring and the trend identified for mothers.
Recruitment and pregnancy rates
We identified large reproductive skews in both sexes, with 87% of sampled males and 83% of sampled females not successfully producing calves that survived until winter. As pregnancy was measured through hormone concentrations from the collected fecal pellets, we were able to determine the percentage of the females sampled who were pregnant. Through pedigree reconstruction, we were able to determine how many individuals have offspring in the population, as captured in the winter, approximately 8 months after being born, which was significantly lower than the number of females that were pregnant each year (only 17% of females successfully produced a calf that survived until winter, whereas pregnancy rates varied between 71 and 87% each year; Supplementary Table 5.1).
Density was significantly correlated with the reproductive success of both females and males (female p = 0.043, male p = 0.038; Table 3) but affected the sexes differently. For females, the probability of successfully having offspring was highest at lower densities, while for males it was highest at higher densities (Supplementary Figure 3.2). Density was not significantly correlated with the pregnancy status of females (Table 3).
Discussion
We used network analyses to measure density-dependent demographic responses in a large mammal population. As predicted, we detected density-dependent demographic responses in female and male boreal caribou and the use of familial networks allowed us to measure how density influences both direct and indirect reproductive success within a population. We found that boreal caribou density at the natal site covaried with male, but not female, offspring dispersal distance, and reproductive success of both males and females. Our results demonstrate that the density-dependence varies heterogeneously between sexes and by the association between animals, i.e., centrality, within the entire population (Table 3). The significantly higher-network centrality observed for females from low-density areas indicates that not only is the direct reproductive success of females in low-density areas higher but that they are also connected to family lines (i.e., parents or offspring) who also have higher reproductive success, compounding this effect.
Integrating familial network metrics allowed us to better capture complex demographic processes that may vary with density. By building a familial network from parent–offspring relationships, we were able to account for the contribution of non-reproducing individuals in the overall demographic structure of the population and assess how density dependence affects reproductive success. Boreal caribou mate polygynously, with mating occurring in loose harems (Thomas et al., 1989), and males defend access to mates such that the reproductive success of males is dominated by larger and older individuals (Hirotani, 1994). Familial network analyses indicated that only 13% of sampled males successfully sired a calf that survived until winter. Because the males with the highest reproductive success were found in higher-density areas, reproductive output was dominated by relatively few males. It may be beneficial for subordinate males to remain within these higher-density areas, remaining with a female group and waiting for the sexual activity of dominant males to wane, or for the possibility of gaining the dominant position and the associated reproductive benefits (Røed et al., 2002). At low densities, males may be more efficient at defending harems due to less competition from other males, leading to lower overall reproductive success in lower-density areas.
The reproductive success observed for females was low and varied with density. Our pregnancy results indicated that the overall pregnancy rate was 81%, yet our familial network analysis found that only 17% of sampled females successfully reproduced a calf that survived until winter, indicating that calf mortality is high and is a contributing factor to the low-observed recruitment rate. Although some of the non-reproducing females may be subadults not yet capable of breeding, this likely would not account for a large proportion of the population. Calf survival in caribou is low, with calf loss to predation highest during the first month of life (Stuart-Smith et al., 1997; Pinard et al., 2012); predation by black bears (Ursus americanus) and wolves (Canis lupus) can account for more than half of calf mortalities (Pinard et al., 2012). In our study, recruitment was inversely density-dependent, as calf survival was greater in lower-density populations, similar to what has been observed in a few other studies on ungulates (Toïgo et al., 2002; McLoughlin et al., 2006). Alpine ibex (Capra ibex) shows a greater variance of female reproductive success in a density-dependent population than in a population with no density dependence (Toïgo et al., 2002), and the lifetime reproductive success of female red deer was inversely density-dependent (McLoughlin et al., 2006).
There may be marked differences in individual reproductive quality among females (Byers, 1997; Weladji et al., 2006, 2008; Hamel et al., 2009), which may be exacerbated by population density (Bonenfant et al., 2009). Weladji et al. (2008) showed that female reindeer (Rangifer tarandus) that were successful breeders had higher subsequent reproductive success than non-breeders and unsuccessful breeders, and Weladji et al. (2006) showed that larger and older reindeer had higher lifetime reproductive success, possibly due to a higher social rank or ability to minimize high mortality risks. In pronghorns (Antilocapra americana), experienced breeding females had lower calf losses to predation (Byers, 1997), and in a longitudinal study of mountain goats (Oreamnos americanus), bighorn sheep (Ovis canadensis), and roe deer (Capreolus capreolus), higher quality females all had a higher probability of reproduction in all three species (Hamel et al., 2009). Network analysis can help identify both density-dependent and density-independent factors that affect female reproductive success leading to an overall better understanding of population dynamics. Our study is the first to assess how centrality within the familial network varies with density in a wild population.
Measuring dispersal in ungulate populations can be difficult due to long dispersal distances, sex-biased behaviors, differences in the detection probability of dispersers and non-dispersers, and multiple interactions (Moore et al., 2014). Here we successfully used network analyses to better understand what drives sex-specific dispersal. SCR modeling and the construction of familial networks from noninvasive genetic sampling allowed us to overcome some of these challenges. We found that male offspring stayed closer to their mothers when the mother was located in a high-density area (Supplementary Table 3.2 and Supplementary Figure 3.2). Because boreal caribou in our study are dispersing shorter distances at higher densities, and boreal caribou density was positively correlated with high-quality habitat availability and negatively correlated with distance to roads, individuals may become trapped within remnant patches of suitable habitat that can support higher densities. This suggests that anthropogenic disturbance can disrupt natal dispersal patterns, turning source populations into fragmented sinks (Fattebert et al., 2015). These results further emphasize the impact of landscape fragmentation on population demography and the importance for some species to maintain low population densities with high spatial connectivity throughout their range. Apart from caribou, variation in natal dispersal has been linked to population density and group characteristics in several species, including feral horses (Equus ferus caballus, Marjamäki et al., 2013) and African lions (Panthera leo, VanderWaal et al., 2009).
Boreal caribou density in our study area is influenced by landscape composition and fragmentation, resulting in patches of higher density that lead to individual heterogeneity in demographic responses. Driven, in part, by anthropogenic disturbances, density-dependent variation in demographic traits may be creating population sinks as both the reproductive success of females and the dispersal distances of male offspring were lower in higher-density areas. Network centrality metrics allowed us to improve the resolution of broad measures of demographic parameters to gain a better understanding of the density-dependent demographic processes of boreal caribou populations. Demographic processes, such as reproductive success, calf survival, and dispersal, can alter the network structure among individuals in a population by adding or removing an individual and its connections (Shizuka and Johnson, 2020). Although our conclusions depend on modeled density estimates, the extent of our data collection and SCR models used gave us high confidence in the estimates (Paterson et al., 2019; McFarlane et al., 2020). To our knowledge, this is the first study looking at the effects of variable density across the range of a wild ungulate population on individual-level measures of reproductive success and dispersal rates.
Data availability statement
The datasets presented in this study can be found in online repositories. The names of the repository/repositories and accession number(s) can be found below: https://doi.org/10.6084/m9.figshare.19905142.
Ethics statement
Ethical review and approval was not required for the animal study because non-invasively collected DNA samples with no handling or contact with animals.
Author contributions
MM and GP conceived and designed the study. SM implemented the analysis and wrote the article with help from MM and TJ. PW coordinated DNA extraction and analysis. DP coordinated and analyzed the spatial density covariate data. GM coordinated hormone extraction and analysis. All authors contributed to subsequent drafts and gave final approval for publication.
Funding
Government of Saskatchewan, Environment and Climate Change Canada, NSERC Collaborative Research & Development, Saskatchewan Power, and Weyerhaeuser (Grant number: CRDPJ 471003-14).
Acknowledgments
We thank Bridget Redquest and Austin Thompson at Trent University for the DNA extraction and analysis; Sonesinh Keobouasone at Environment and Climate Change Canada for data management; Government of Saskatchewan staff and consultants for planning and conducting the extensive field surveys and handling the samples; staff at the Toronto Zoo for doing the hormone analysis; Environment and Climate Change Canada for providing the land cover characterization; and John Wilmshurst for constructive reviews and editing.
Conflict of interest
The authors declare that the research was conducted in the absence of any commercial or financial relationships that could be construed as a potential conflict of interest.
Publisher’s note
All claims expressed in this article are solely those of the authors and do not necessarily represent those of their affiliated organizations, or those of the publisher, the editors and the reviewers. Any product that may be evaluated in this article, or claim that may be made by its manufacturer, is not guaranteed or endorsed by the publisher.
Supplementary material
The Supplementary Material for this article can be found online at: https://www.frontiersin.org/articles/10.3389/fevo.2022.956834/full#supplementary-material
References
Aguillon, S. M., and Duckworth, R. A. (2015). Kin aggression and resource availability influence phenotype-dependent dispersal in a passerine bird. Behav. Ecol. Sociobiol. 69, 625–633. doi: 10.1007/s00265-015-1873-5
Akaike, H. (1974). A new look at the statistical model identification. IEEE Trans. Automat. Contr. 19, 716–723. doi: 10.1109/tac.1974.1100705
Anderson, D. R., Burnham, K. B., and White, G. C. (1994). Model selection in overdispersed capture-recapture data. Ecology 75, 1780–1793. doi: 10.2307/1939637
Arsenault, A. A. (2003). Status and management of wildlife in Saskatchewan, 1999-2001. Portland: Fish and Wildlife Technical Report.
Ashtiani, M., Mirzaie, M., and Jafari, M. (2018). CINNA: An r/CRAN package to decipher central informative nodes in network analysis. Bioinformatics 35, 1436–1437. doi: 10.1093/bioinformatics/bty819
Badger, J. J., Bowen, W. D., den Heyer, C. E., and Breed, G. A. (2020). Variation in individual reproductive performance amplified with population size in a long-lived carnivore. Ecology 101:e03024. doi: 10.1002/ecy.3024
Ball, M. C., Finnegan, L., Manseau, M., and Wilson, P. (2010). Integrating multiple analytical approaches to spatially delineate and characterize genetic population structure: An application to boreal caribou (Rangifer tarandus caribou) in central Canada. Conserv. Genet. 11, 2131–2143. doi: 10.1007/s10592-010-0099-3
Ball, M. C., Pither, R., Manseau, M., Clark, J., Petersen, S. D., Kingston, S., et al. (2007). Characterization of target nuclear DNA from faeces reduces technical issues associated with the assumptions of low-quality and quantity template. Conserv. Genet. 8, 577–586. doi: 10.1007/s10592-006-9193-y
Bestion, E., Teyssier, A., Aubret, F., Clobert, J., and Cote, J. (2014). Maternal exposure to predator scents: Offspring phenotypic adjustment and dispersal. Proc. R Soc. B: Biol. Sci. 281:20140701. doi: 10.1098/rspb.2014.0701
Bitume, E. V., Bonte, D., Ronce, O., Bach, F., Flaven, E., Olivieri, I., et al. (2013). Density and genetic relatedness increase dispersal distance in a subsocial organism. Ecol. Lett. 16, 430–437. doi: 10.1111/ele.12057
Bonenfant, C., Gaillard, J. M., Coulson, T., Festa-Bianchet, M., Loison, A., Garel, M., et al. (2009). Empirical evidence of density-dependence in populations of large herbivores. Adv. Ecol. Res. 41, 313–357. doi: 10.1016/S0065-2504(09)00405-X/
Borchers, D. L., and Efford, M. G. (2008). Spatially explicit maximum likelihood methods for capture-recapture studies. Biometrics 64, 377–385. doi: 10.1111/j.1541-0420.2007.00927.x
Bowers, M. A., and Matter, S. F. (1997). Landscape ecology of mammals: Relationships between density and patch size. J. Mammal. 78, 999–1013. doi: 10.2307/1383044
Bowler, D. E., and Benton, T. G. (2005). Causes and consequences of animal dispersal strategies: Relating individual behaviour to spatial dynamics. Biol. Rev. 80, 205–225. doi: 10.1017/s1464793104006645
Bowyer, R. T., Bleich, V. C., Stewart, K. M., Whiting, J. C., and Monteith, K. L. (2014). Density dependence in ungulates: A review of causes, and concepts with some clarifications. Calif. Fish. Game. 100, 550–572.
Boyce, M. S., and McDonald, L. L. (1999). Relating populations to habitats using resource selection functions. Trends Ecol. Evol. 14, 268–272. doi: 10.1016/s0169-5347(99)01593-1
Byers, J. A. (1997). American pronghorn. Social adaptations and the ghosts of predators past. Chicago: University of Chicago Press.
Clutton-Brock, T. H. (1988). “Reproductive success,” in Reproductive Success: Studies of Individual Variation in Contrasting Breeding Systems, ed. T. H. Clutton-Brock (Chicago, IL: University of Chicago Press), 472–486.
Clutton-Brock, T. H., and Sheldon, B. C. (2010). Individuals and populations: the role of long-term, individual-based studies of animals in ecology and evolutionary biology. Trends Ecol. Evol. 25, 562–573. doi: 10.1016/j.tree.2010.08.002
COSEWIC (2011). Designatable units for caribou (Rangifer tarandus) in Canada. Ottawa: Committee on the Status of Endangered Wildlife in Canada, 88.
Dussault, C., Pinard, V., Ouellet, J.-P., Courtois, R., and Fortin, D. (2012). Avoidance of roads and selection for recent cutovers by threatened caribou: Fitness-rewarding or maladaptive behaviour? Proc. R Soc. B Biol. Sci. 279, 4481–4488. doi: 10.1098/rspb.2012.1700
Efford, M. (2004). Density estimation in live-trapping studies. Oikos 106, 598–610. doi: 10.1111/j.0030-1299.2004.13043.x
Efford, M. G. (2011). Estimation of population density by spatially explicit capture-recapture analysis of data from area searches. Ecology 92, 2202–2207. doi: 10.1890/11-0332.1
Efford, M. G. (2018). Secr: Spatially explicit capture-recapture models. Available Online at: https://cran.r-project.org/package=secr (accessed January 25, 2019).
Environment Canada. (2011). Scientific assessment to inform the identification of critical habitat for woodland caribou (Rangifer tarandus caribou), boreal population, in Canada: 2011 update. Ottawa: Environment Canada.
Environment Canada. (2012). Recovery strategy for the woodland caribou (Rangifer tarandus caribou), Boreal population, in Canada. Species at Risk Act Recovery Strategy Series. Ottawa: Environment Canada.
Escoda, L., González-Esteban, J., Gómez, A., and Castresana, J. (2017). Using relatedness networks to infer contemporary dispersal: Application to the endangered mammal galemys pyrenaicus. Mol. Ecol. 26, 3343–3357. doi: 10.1111/mec.14133
Farine, D. R. (2013). Animal social network inference and permutations for ecologists in R using asnipe. Methods Ecol. Evol. 4, 1187–1194. doi: 10.1111/2041-210x.12121
Fattebert, J., Balme, G., Dickerson, T., Slotow, R., and Hunter, L. (2015). Density-dependent natal dispersal patterns in a leopard population recovering from over-harvest. PLoS One 10:e0122355. doi: 10.1371/journal.pone.0122355
Flasko, A., Manseau, M., Mastromonaco, G., Bradley, M., Neufeld, L., and Wilson, P. (2017). Fecal DNA, hormones, and pellet morphometrics as a noninvasive method to estimate age class: An application to wild populations of central mountain and boreal woodland caribou (Rangifer tarandus caribou). Can. J. Zool. 95, 311–321. doi: 10.1139/cjz-2016-0070
Fountain, T., Husby, A., Nonaka, E., DiLeo, M. F., Korhonen, J. H., Rastas, P., et al. (2017). Inferring dispersal across a fragmented landscape using reconstructed families in the Glanville fritillary butterfly. Evol. Appl. 11, 287–297. doi: 10.1111/eva.12552
Fronhofer, E. A., Legrand, D., Altermatt, F., Ansart, A., Blanchet, S., Bonte, D., et al. (2018). Bottom-up and top-down control of dispersal across major organismal groups. Nat. Ecol. Evol. 2, 1859–1863. doi: 10.1038/s41559-018-0686-0
Gaillard, J. M., Festa-Bianchet, M., Yoccoz, N. G., Loison, A., and Toïgo, C. (2000). Temporal variation in fitness components and population dynamics of large herbivores. Annu. Rev. Ecol. Syst. 31, 367–393. doi: 10.1146/annurev.ecolsys.31.1.367
Gerhart, K. L., White, R. G., Cameron, R. D., and Russell, D. E. (1996). Estimating fat content of caribou from body condition scores. J. Wildl. Manag. 60, 713–718. doi: 10.2307/3802369
Government of Canada (2002). Species at risk act (S.C. 2002, c. 29). Available online at: https://laws-lois.justice.gc.ca/eng/acts/s-15.3/page-1.html
Greenwood, P. J. (1980). Mating systems, philopatry and dispersal in birds and mammals. Anim. Behav. 28, 1140–1162. doi: 10.1016/s0003-3472(80)80103-5
Hamel, S., Côté, S. D., Gaillard, J.-M., and Festa-Bianchet, M. (2009). Individual variation in reproductive costs of reproduction: High-quality females always do better. J. Anim. Ecol. 78, 143–151. doi: 10.1111/j.1365-2656.2008.01459.x
Hettinga, P. N., Arnason, A. N., Manseau, M., Cross, D., Whaley, K., and Wilson, P. (2012). Estimating size and trend of the North Interlake woodland caribou population using fecal-DNA and capture-recapture models. J. Wildl. Manag. 76, 1153–1164. doi: 10.1002/jwmg.380
Hijmans, R. J. (2019). Geosphere: Spherical trigonometry. Available Online at: https://cran.r-project.org/package=geosphere (accessed October 13, 2021).
Hijmans, R. J. (2022). Raster: Geographic data analysis and modeling. Available online at:https://cran.r-project.org/package=raster (accessed September 24, 2019).
Hirotani, A. (1994). Dominance rank, copulatory behaviour and estimated reproductive success in male reindeer. Anim. Behav. 48, 929–936. doi: 10.1006/anbe.1994.1318
Hixon, M. A., and Johnson, D. W. (2009). “Density dependence and independence,” in Encyclopedia of life sciences (ELS), ed. R. Johnson (Chichester: John Wiley & Sons, Ltd)
Jones, O. R., and Wang, J. (2010). COLONY: A program for parentage and sibship inference from multilocus genotype data. Mol. Ecol. Resour. 10, 551–555. doi: 10.1111/j.1755-0998.2009.02787.x
Jones, T. B., and Manseau, M. (2022). Genetic networks in ecology: A guide to population, relatedness, and pedigree networks and their applications in conservation biology. Biol. Cons. 267:109466. doi: 10.1016/j.biocon.2022.109466
Latora, V., and Marchiori, M. (2001). Efficient behavior of small-world networks. Phys. Rev. Lett. 87:198701. doi: 10.1103/physrevlett.87.198701
Leblond, M., Dussault, C., and Ouellet, J.-P. (2013). Avoidance of roads by large herbivores and its relation to disturbance intensity. J. Zool. 289, 32–40. doi: 10.1111/j.1469-7998.2012.00959.x
Leturque, H., and Rousset, F. (2003). Joint evolution of sex ratio and dispersal: Conditions for higher dispersal rates from good habitats. Evol. Ecol. 17, 67–84. doi: 10.1023/a:1022405415375
Łomnicki, A. (1980). Regulation of population density due to individual differences and patchy environment. Oikos 35, 185–193. doi: 10.2307/3544426
Manly, B. F. J., McDonald, L. L., Thomas, D. L., McDonald, T. L., and Erickson, W. P. (2002). Resource selection by animals: Statistical analysis and design for field studies. Dordrecht: Kluwer Academic Publishers.
Marjamäki, P. H., Contasti, A. L., Coulson, T. N., and McLoughlin, P. D. (2013). Local density and group size interacts with age and sex to determine direction and rate of social dispersal in a polygynous mammal. Ecol. Evol. 3, 3073–3082. doi: 10.1002/ece3.694
Marshall, I. B., Schut, P. H., and Ballard, M. (1999). Data from: A national ecological framework for Canada. Ottawa: State of the Environment Directorate.
Matthysen, E. (2005). Density-dependent dispersal in birds and mammals. Ecography 28, 403–416. doi: 10.1111/j.0906-7590.2005.04073.x
McCullough, D. R. (1999). Density dependence and life-history strategies of ungulates. J. Mammal. 80, 1130–1146. doi: 10.2307/1383164
McFarlane, S., Manseau, M., and Wilson, P. J. (2021). Spatial familial networks to infer demographic structure of wild populations. Ecol. Evol. 11, 4507–4519. doi: 10.1002/ece3.7345
McFarlane, S., Manseau, M., Flasko, A., Horn, R. L., Arnason, N., Neufeld, L., et al. (2018). Genetic influences on male and female variance in reproductive success and implications for the recovery of severely endangered mountain caribou. Glob. Ecol. Conserv. 16:e00451. doi: 10.1016/j.gecco.2018.e00451
McFarlane, S., Manseau, M., Steenweg, R., Hervieux, D., Hegel, T., Slater, S., et al. (2020). An assessment of sampling designs using SCR analyses to estimate abundance of boreal caribou. Ecol. Evol. 10, 11631–11642. doi: 10.1002/ece3.6797
McLoughlin, P. D., Boyce, M. S., Coulson, T., and Clutton-Brock, T. H. (2006). Lifetime reproductive success and density-dependent, multi-variable resource selection. Proc. R Soc. B Biol. Sci. 273, 1449–1454. doi: 10.1098/rspb.2006.3486
Moore, J. A., Draheim, H. M., Etter, D., Winterstein, S., and Scribner, K. T. (2014). Application of large-scale parentage analysis for investigating natal dispersal in highly vagile vertebrates: A case study of American black bears (Ursus americanus). PLoS One 9:e91168. doi: 10.1371/journal.pone.0091168
Morris, D. W. (2003). Toward an ecological synthesis: A case for habitat selection. Oecologia 136, 1–13. doi: 10.1007/s00442-003-1241-4
Natural Resources Canada (2020). Data from: 2015 land cover of Canada. Ottawa, ON: Natural Resources Canada.
Norman, A. J., and Spong, G. (2015). Single nucleotide polymorphism-based dispersal estimates using noninvasive sampling. Ecol. Evol. 5, 3056–3065. doi: 10.1002/ece3.1588
Paterson, J. T., Proffitt, K., Jimenez, B., Rotella, J., and Garrott, R. (2019). Simulation-based validation of spatial capture-recapture models: A case study using mountain lions. PLoS One 14:e0215458. doi: 10.1371/journal.pone.0215458
Pinard, V., Dussault, C., Ouellet, J.-P., Fortin, D., and Courtois, R. (2012). Calving rate, calf survival rate, and habitat selection of forest-dwelling caribou in a highly managed landscape. J. Wildl. Manag. 76, 189–199. doi: 10.1002/jwmg.217
Poethke, H. J., and Hovestadt, T. (2002). Evolution of density- and patch-size-dependent dispersal rates. Proc. R. Soc. B Biol. Sci. 269, 637–645. doi: 10.1098/rspb.2001.1936
Polfus, J. L., Hebblewhite, M., and Heinemeyer, K. (2011). Identifying indirect habitat loss and avoidance of human infrastructure by northern mountain woodland caribou. Biol. Conserv. 144, 2637–2646. doi: 10.1016/j.biocon.2011.07.023
Priadka, P., Manseau, M., Trottier, T., Hervieux, D., Galpern, P., McLoughlin, P. D., et al. (2019). Partitioning drivers of spatial genetic variation for a continuously distributed population of boreal caribou: Implications for management unit delineation. Ecol. Evol. 9, 141–153. doi: 10.1002/ece3.4682
R Core Team (2019). R: A language and environment for statistical computing. Vienna: R Foundation for Statistical Computing.
Røed, K. H., Holand, Ø, Smith, M. E., Gjøstein, H., Kumpula, J., and Nieminen, M. (2002). Reproductive success in reindeer males in a herd with varying sex ratio. Mol. Ecol 11, 1239–1243. doi: 10.1046/j.1365-294x.2002.01509.x
Rudolph, T. D., Drapeau, P., Imbeau, L., Brodeur, V., Legare, S., and St-Laurent, M.-H. (2017). Demographic responses of boreal caribou to cumulative disturbances highlight elasticity of range-specific tolerance thresholds. Biodivers. Conserv. 26, 1179–1198. doi: 10.1007/s10531-017-1292-1
Saskatchewan Ministry of Environment (2019). Range plan for woodland caribou in Saskatchewan: Boreal plain ecozone, SK2 Central Caribou Administrative Unit. Regina, SK: Saskatchewan Ministry of Environment.
Shannon, P., Markiel, A., Ozier, O., Baliga, N. S., Wang, J. T., Ramage, D., et al. (2003). Cytoscape: A software environment for integrated models of biomolecular interaction networks. Genome Res. 13, 2498–2504. doi: 10.1101/gr.1239303
Shizuka, D., and Johnson, A. E. J. (2020). How demographic processes shape animal social networks. Behav. Ecol. 31, 1–11. doi: 10.1093/beheco/arz083
SKCDC (2020). Data from: Species at risk in Saskatchewan. Saskatchewan conservation data centre (SKCDC), Fish, Wildlife; Lands Branch. Regina, SK: Saskatchewan Ministry of the Environment.
Smith, A. L., Landguth, E. L., Bull, C. M., Banks, S. C., Gardner, M. G., and Driscoll, D. A. (2016). Dispersal responses override density effects on genetic diversity during post-disturbance succession. Proc. R. Soc. B Biol. Sci. 283:20152934. doi: 10.1098/rspb.2015.2934
Stewart, K. M., Bowyer, R. T., Dick, B. L., Johnson, B. K., and Kie, J. G. (2005). Density-dependent effects on physical condition and reproduction in North American elk: an experimental test. Oecologia 143, 85–93. doi: 10.1007/s00442-004-1785-y
Stuart-Smith, A. K., Bradshaw, C. J. A., Boutin, S., Hebert, D. M., and Rippin, A. B. (1997). Woodland caribou relative to landscape patterns in northeastern Alberta. J. Wildl. Manag. 61, 622–633.
Thomas, D. C., Barry, S. J., and Kiliaan, H. P. (1989). Fetal sex ratios in caribou: Maternal age and condition effects. J. Wildl. Manag. 53, 885–890. doi: 10.2307/3809582
Tischendorf, L., Grez, A., Zaviezo, T., and Fahrig, L. (2005). Mechanisms affecting population density in fragmented habitat. Ecol. Soc. 10:7.
Toïgo, C., Gaillard, J.-M., Gauthier, D., Girard, I., Martinot, J.-P., and Michallet, J. (2002). Female reproductive success and costs in an alpine capital breeder under contrasting environments. Écoscience 9, 427–433. doi: 10.1080/11956860.2002.11682730
Travis, J. M. J., Murrell, D. J., and Dytham, C. (1999). The evolution of density-dependent dispersal. Proc. R Soc. B Biol. Sci. 266, 1837–1842. doi: 10.1098/rspb.1999.0854
van Oort, H., McLellan, B. N., and Serrouya, R. (2011). Fragmentation, dispersal and metapopulation function in remnant populations of endangered mountain caribou. Anim. Conserv. 14, 215–224. doi: 10.1111/j.1469-1795.2010.00423.x
VanderWaal, K. L., Mosser, A., and Packer, C. (2009). Optimal group size, dispersal decisions and postdispersal relationships in female African lions. Anim. Behav. 77, 949–954. doi: 10.1016/j.anbehav.2008.12.028
Wang, J. L. (2004). Sibship reconstruction from genetic data with typing errors. Genetics 166, 1963–1979. doi: 10.1534/genetics.166.4.1963
Weladji, R. B., Gaillard, J. M., Yoccoz, N. G., Holand, O., Mysterud, A., Loison, A., et al. (2006). Good reindeer mothers live longer and become better in raising offspring. Proc. R. Soc. B Biol. Sci. 273, 1239–1244. doi: 10.1098/rspb.2005.3393
Weladji, R. B., Loison, A., Gaillard, J.-M., Holand, Ø, Mysterud, A., Yoccoz, N. G., et al. (2008). Heterogeneity in individual quality overrides costs of reproduction in female reindeer. Oecologia 156, 237–247. doi: 10.1007/s00442-008-0961-x
Keywords: density dependence, dispersal, familial networks, individual fitness, pedigree, Rangifer tarandus, caribou
Citation: McFarlane S, Manseau M, Jones TB, Pouliot D, Mastromonaco G, Pittoello G and Wilson PJ (2022) Identification of familial networks reveals sex-specific density dependence in the dispersal and reproductive success of an endangered ungulate. Front. Ecol. Evol. 10:956834. doi: 10.3389/fevo.2022.956834
Received: 30 May 2022; Accepted: 21 November 2022;
Published: 14 December 2022.
Edited by:
Alexei B. Ryabov, University of Oldenburg, GermanyReviewed by:
Maria Cavedon, University of Calgary, CanadaJames McLaren, University of Oldenburg, Germany
Copyright © 2022 McFarlane, Manseau, Jones, Pouliot, Mastromonaco, Pittoello and Wilson. This is an open-access article distributed under the terms of the Creative Commons Attribution License (CC BY). The use, distribution or reproduction in other forums is permitted, provided the original author(s) and the copyright owner(s) are credited and that the original publication in this journal is cited, in accordance with accepted academic practice. No use, distribution or reproduction is permitted which does not comply with these terms.
*Correspondence: Micheline Manseau, bWljaGVsaW5lLm1hbnNlYXVAZWMuZ2MuY2E=