- 1Department of Neurobiology, Physiology and Behavior, University of California, Davis, Davis, CA, United States
- 2Department of Fisheries, Wildlife, and Conservation Science, Oregon State University, Corvallis, OR, United States
The framework of allostasis, allostatic load and overload (i.e., stability through change) attempts to combine homeostasis processes in day-to-day responses of physiology and behavior. These include predictive changes in environment such as seasons, and facultative responses to perturbations. The latter can be severe, occur at any time, and may present considerable additional challenges to homeostasis. Hormonal cascades, such as the hypothalamo-pituitary-adrenal cortex (HPA) axis, play a key role in responses to perturbations across vertebrate taxa. Glucocorticoids have been implicated in these processes in relation to energy balance that plays a role in determining responses to energetic demand (allostatic load) and influencing subsequent physiology and behavior associated with coping. Circulating glucocorticoid levels are likely regulated in part based on an individual’s proximity to energetic crisis, identified as the perturbation resistance potential (PRP). In the model of allostatic load, PRP is quantified as the difference between available resources and all energetic costs of allostatic load such as daily routines, life history stages (breeding, migration, molt and so on), and the impact of environmental perturbations. PRP can change gradually or abruptly and may be reflected by spikes in blood hormone levels. The pattern of individual responsiveness to PRP may vary and has specific implications for the activation of mineralocorticoid vs glucocorticoid-type receptors, hormone metabolizing enzymes and other downstream factors in target tissues. However, PRP is a difficult metric to measure. Here, we examine the variety of cues that animals may use to inform them about the status of their PRP and probability of energetic crisis. We consider (1) elevation in glucocorticoids as an endocrine “decision,” and (2) error management strategies in evaluating responsiveness to cues that may reflect or predict an impending energetic crisis. The potential for differential receptor activation as well as further integrative “decisions” to determine the diverse and sometimes contradictory effects of receptor activation and its downstream actions are important to the consideration of error management. This perspective offers insight into the basis of intra- and inter-individual variability in responsiveness and opens an avenue toward improving compatibility of the allostasis model with more classical views on “stress”.
Introduction
Coping with environmental change is an essential component of an organism’s life cycle. These changes can be predictable – environmental cues such as day length, light intensity, temperature and food availability – that are used to time life history stages and homeostatic adjustments (Gwinner, 1996; Wingfield, 2004; Hau et al., 2008). Additionally, all organisms must cope with unpredictable events – social disruptions, predators, extreme weather events and human disturbance. However, there is no clear distinction between coping with predictable and unpredictable events. Indeed, there is much overlap in how organisms cope which varies as a function of individual body condition, social status, age and sex. The term “stress” is used (recursively) to describe many environmental changes that may result in the organism modifying its behavior or physiology to mitigate the impacts of the “stressor.” Predictable changes, and the life history stages best adapted to deal with them, may be energetically demanding, but an individual can prepare by using predictive cues to anticipate onset of a life history stage rather than respond facultatively. A key point is that an environmental situation (including both predictable and unpredictable) might be “stressful” to one individual but not to another. How we assess this kind of contextual variation and explore how behavioral and physiological coping mechanisms are regulated is critically important to teasing apart the confusing and often contradictory narratives about stress. Allostasis, i.e., stability through change, is one theoretical framework that integrates the demands of predictable and unpredictable environments (allostatic load) according to individual condition (McEwen and Wingfield, 2003). Twenty years from its inception, we feel it is time to revisit the concept of allostasis in the light of recent developments and research.
Stability and change: Allostasis and allostatic load
The term “allostasis” was initially advanced by Sterling and Eyer (1988). They observed that many human pathologies appeared to result from damaging shifts in physiological set-points, such as high blood pressure, as opposed to simple homeostatic failures. Their goal, as clarified by Sterling (2004), was specifically to reject and replace the term “homeostasis,” which was the preferred term to describe most or all regulatory processes in the body since its introduction by Walter Cannon (1932). The root “homeo,” Sterling and Eyer (1988) argue, is almost always inaccurate, as biology is dynamic and constancy in set points is rare. Furthermore, shifts often occur in anticipation of demands, a notion very much at odds with the reactive, thermostat-like model of homeostasis presented in most textbooks (Sterling and Eyer, 1988; Sterling, 2004, 2020).
Dallman (1993) interpreted Sterling and Eyer’s (1988) examples of stable shifts in physiology as “new operating conditions” in response to “occasionally hostile conditions.” In a subsequent paper, McEwen and Stellar (1993) featured “allostasis” centrally. However, they focused on set-point-shifts as the core of their definition, and explicitly retained homeostasis as a “tried and useful concept” that, while inadequate, did not merit outright replacement. McEwen (2004) later clarified that they felt “homeostasis should be reserved for parameters that are essential to maintain for survival.” McEwen and Stellar (1993) aimed to identify and name the consequences of such shifts i.e., “the strain on the body produced by repeated ups and downs of physiologic response, as well as by the elevated activity of physiologic systems under challenge, changes in metabolism (see also Jimeno et al., 2018) and the impact of wear and tear on a number of organs and tissues.” For this, they coined the term, “allostatic load” (McEwen and Stellar, 1993).
The definition of allostatic load developed by McEwen and Stellar (1993) and elaborated upon by McEwen (1998) referred to specific pathologies associated with chronic stress, and particularly chronic exposure to elevated glucocorticoid hormones in humans and laboratory animals. Much of the biomedical literature continues to treat allostatic load in this way, using metrics like blood pressure, human low density lipoprotein (HLDL) cholesterol, and glycosylated hemoglobin to generate a score estimating wear-and-tear on the body. In the course of elaborating upon allostatic load, words like “price,” “cost” and “demand” began to recur. For instance, McEwen (1998) describes “the price of adaptation that promotes pathophysiology.” Koob and Le Moal (2001), described allostatic load as “the cost or the price the body may have to pay for being forced to adapt to an adverse or deleterious physiological or physical situation.”
McEwen and Wingfield (2003) took this recurring notion of “cost” and developed it into an energetics-based (E) framework for allostatic load. In brief, allostatic load is a function of Ee (basal existence) + Ei (routine activity) + Eo (unpredictable perturbations; Table 1; McEwen and Wingfield, 2003). Glucocorticoid levels are predicted to change in parallel with allostatic load. Pathophysiology, in this model, is reserved for two types of allostatic overload: Type I occurs when allostatic load exceeds the energetic resources available in the environment (Table 1 and Figure 1B), and Type II occurs when allostatic load remains high without exceeding basal energy available. The latter is defined as Eg (food available in the environment) and Ecr which while including Eg also recognizes endogenous energy reserves of the individual and access to Eg as a result of predation risk, social status and so on (Table 1). Type II allostatic overload results in pathology associated with chronically elevated glucocorticoids (Figure 1B; McEwen and Wingfield, 2003).
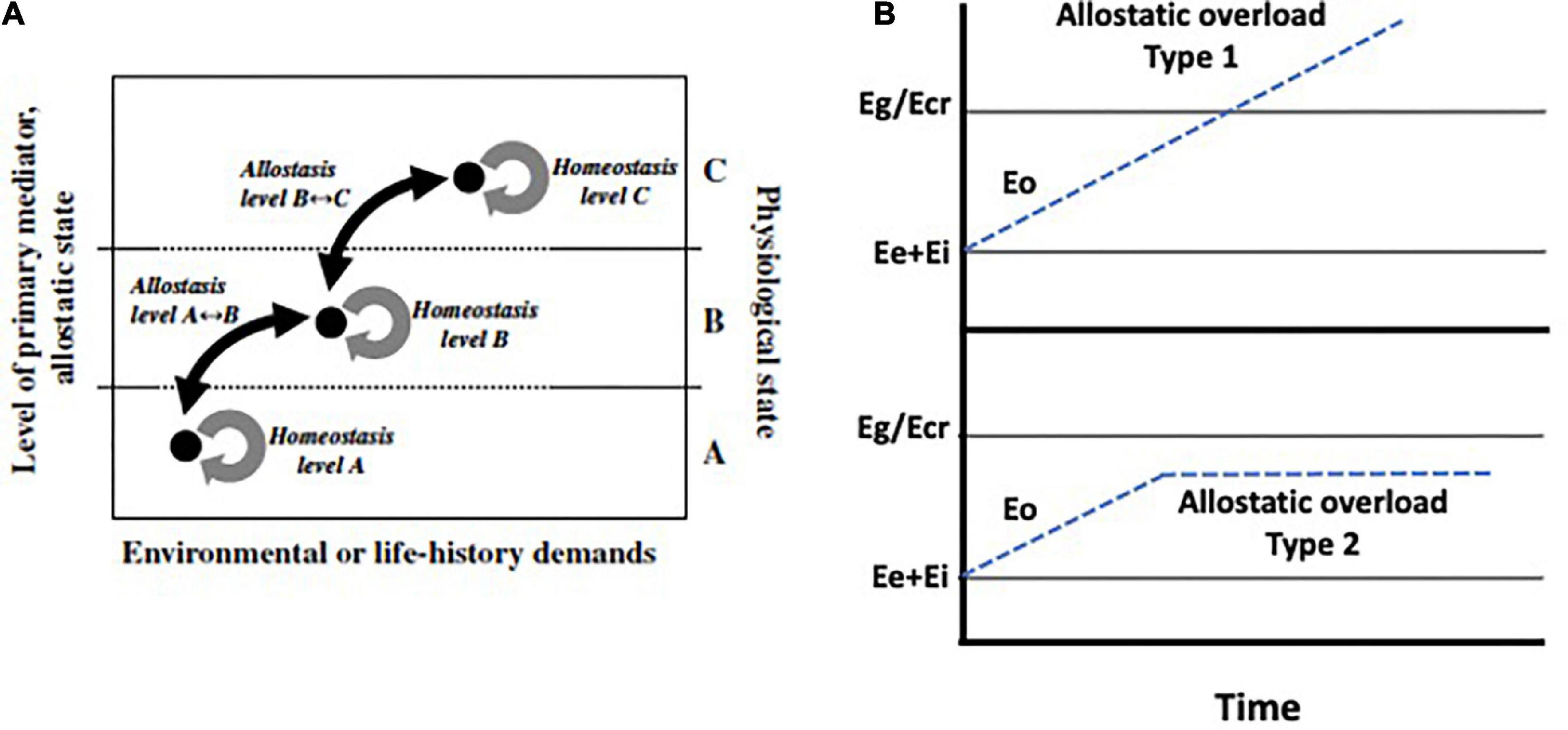
Figure 1. (A) An explanation of the terms: “homeostasis,” “allostasis,” and “physiological state.” Action of primary mediators (i.e., action of hormones, elements of the immune system, and/or neural responses; defined as an allostatic state) tune critical internal variables to specific set points as appropriate to existing environmental conditions and life-history demands (McEwen and Wingfield, 2003). “Allostasis” is defined as the process of adjusting critical internal variables among such set points. In contrast, we refer to “homeostasis” as the process of maintaining internal variables within a given set point. The physiological and behavioral characteristics of a specific set point can be described by three increasingly complex physiological states – A, B, and C. Physiological state A is characterized by basic physiological and behavioral processes fundamental for simple existence. Physiological state B is representative of processes associated with increased, but predictable or manageable demands in the environment or in life history. Physiological state C is characterized by facultative responses (e.g., the emergency life-history stage) associated with unpredictable and life-threatening events. The physiological states A, B, and C are paralleled by allostatic states that maintain processes at given set points through the action of primary mediators. From Landys et al. (2006) with permission, Elsevier. (B) A schematic representation of energy available to obtain in the habitat (Eg) or total resources including stored fat (Ecr), existence energy (Ee) and energy required to go about daily routines (E1). Eo (dashed blue line) is the additional energy resulting from a perturbation or changed weather conditions that increases allostatic load (for example increased foraging effort). Allostatic overload type 1 occurs when Ee + Ei + Eo exceeds Eg/Ecr precipitating negative energy balance (top panel) and triggers the emergency life history stage (ELHS). In the bottom panel, Eo increases and remains permanently elevated but below Eg/Ecr. This is allostatic overload type 2.
This inclusion of energetics in the definition of allostatic load has gained traction since its introduction such that there now exists a spectrum of definitions for allostatic load. These definitions range from those that retain the original focus on specific pathologies or damage (e.g., Picard et al., 2014; Juster and McEwen, 2015) to definitions that describe a more all-inclusive energetic quantity (Lattin and Romero, 2014; Monaghan and Spencer, 2014). While these differing definitions are generally assumed to be equivalent, or at least not in conflict, their congruity has not been assessed. In part, this is because although animal energetics is an ancient and well-developed field, very little research has been done that can be used to assess its relationship with other hallmarks of allostatic load, such as circulating glucocorticoid levels (McEwen and Wingfield, 2003).
Here, we will break down the allostatic load framework into its component parts and contrast it with a complementary model that has arisen since its inception, Reactive Scope (Romero et al., 2009). Both models incorporate the concept of a threshold, albeit differently. It is proposed that a framework focused on the endocrine “decision” to cross a functional threshold may be a better fit. We will explore conserved and variable aspects of glucocorticoid signaling to characterize the nature of this threshold. Finally, we will discuss ways of interpreting variation to inform future modeling efforts.
Breaking down the model
As we engage with an update of the framework of allostatic load presented by McEwen and Wingfield (2003), it is useful to first break it down into its component parts. After discussing the basis for our proposed updates, we will return to these to note relevant changes.
1. Allostatic load is an energetic quantity or Eload. This quantity may be subdivided into its component parts: basal existence, routine, and perturbation-related costs, dubbed Ee, Ei, and Eo, respectively (Table 1). These are served by physiological state levels of activity – A, B, and C in Figures 1, 2. Ei can vary seasonally as life-history stage and individual needs change, for example as occurs during breeding, migration, molt, etc. Eo is inherently unpredictable and includes costs of injury, disease, and unexpected wear-and-tear as well as responses to habitat loss or degradation, predation risk, major weather events and so on. Wear-and-tear are included in Ee and Ei to the extent that they incur significant costs; thus, these ideas are not necessarily mutually exclusive (Figures 1, 2 and Table 1).
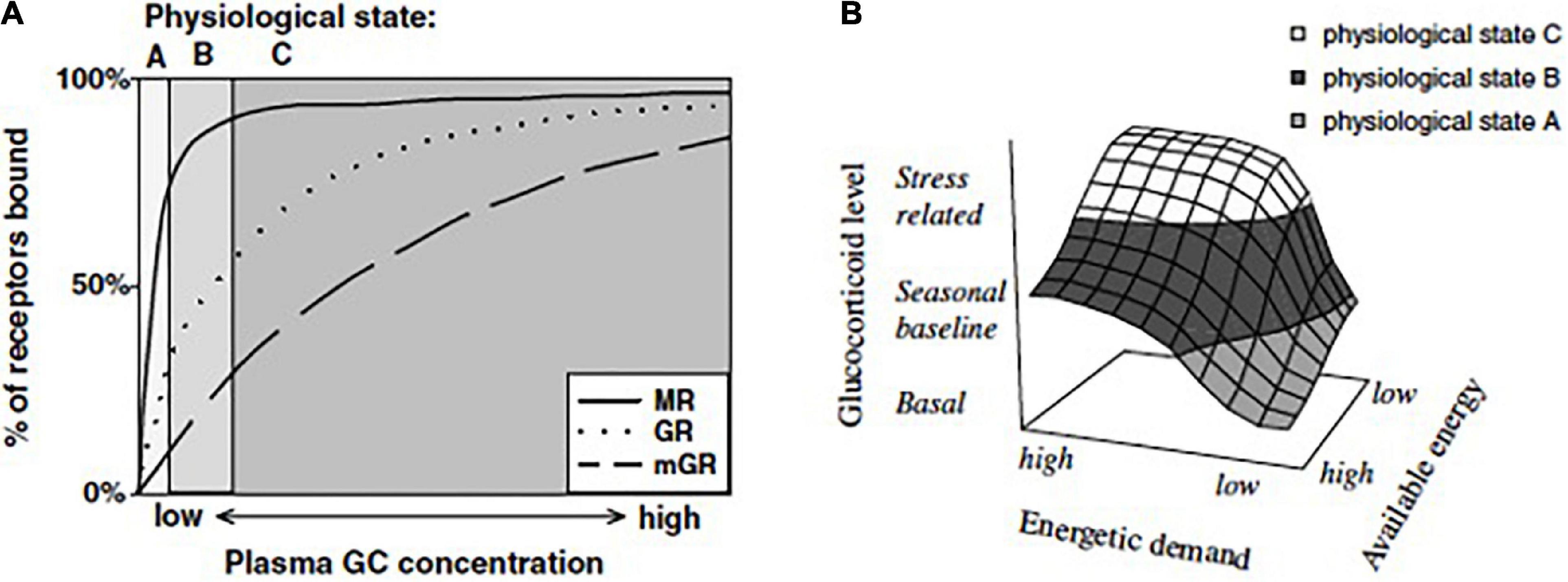
Figure 2. (A) Hypothetical binding curves of the three known adrenal steroid receptors: the genomic mineralocorticoid receptor (MR), the genomic glucocorticoid receptor (GR), and the non-genomic membrane-associated glucocorticoid receptor (mGR). The MR binds glucocorticoids (GCs) with a higher affinity than does the GR. At least in birds, the mGR displays the lowest affinity for GCs (Breuner and Orchinik, 2009). These in vitro receptor properties suggest that low GC concentrations primarily bind the MR, whereas elevated levels bind the GR and may begin to bind the mGR. Thus, differences in the proportion of bound receptor types may provide a mechanism whereby different GC concentrations are able to produce specific physiological states. Low GC levels that predominantly activate the MR may promote physiological state A. Seasonal elevations in plasma GCs may support physiological state B through partial binding to the GR and the mGR. As GCs increase above the seasonal baseline, increased binding of all three receptor types may stimulate the physiological state C. (B) Relationship between energetic demand (allostatic load), available energy, and glucocorticoid (GC) level in the promotion of physiological states. Energetic demand can change with daily activity patterns or with life-history stage and may also increase as a consequence of challenging perturbations in the environment. Although animals respond to energetic demand by secreting GCs, this response may be attenuated by high energy availability, i.e., abundant food resources and/or internal energy depots. For example, even when energy demand is high, animals that have access to sufficient energy stores may remain within the reaction norm for a particular life-history stage and may not increase plasma GCs above a seasonal baseline. Within seasonal baseline levels, GCs may promote processes representative of physiological state B. However, as energy stores become unavailable, GCs may increase to stress-related concentrations at which point they support increasingly complex physiological states to allow an individual to cope. From Landys et al. (2006) with permission courtesy of Elsevier.
2. Variation in allostatic load is correlated with mediators such as plasma glucocorticoid levels. This component (Figures 1, 2) has been tested in birds and tentatively rejected based on Word et al. (2022). Nonetheless, glucocorticoids clearly have a central role to play, the nature of which will be considered further in this paper.
3. Resource availability is designated Eg. It represents a limit to the energy that can be expended without negative energy balance; hence, it must be considered to have the same units as allostatic load (Table 1). This has been extended to Ecr which includes Eg as well as endogenous energy reserves, and degree of access to Eg because of social status, predation risk and so on (see Table 1).
4. Allostatic overload Type 1 occurs when an animal is in negative energy balance. Specifically, this occurs when the sum of all types of allostatic load (Figure 1B) exceeds Eg. When this type of allostatic overload occurs, an “emergency life-history stage” (ELHS; Wingfield et al., 1998) will be triggered, redirecting physiology and behavior to individual survival (Figure 1B and Table 1). It often interrupts normal life history stages, depending on the intensity and duration of the perturbation, and individual trade-off decisions.
5. The ELHS is induced by elevated secretion of glucocorticoid hormones. Glucocorticoid fluctuations that occur routinely, for example, with time of day, season, or life-history stage, are distinguished from abnormally high levels associated with Eo (Landys et al., 2006; Romero and Wingfield, 2016). According to that description, ELHS activation (“physiological state level C”) occurs when glucocorticoid concentrations are consistently elevated above the seasonal norm for the life-history stage (“physiological state level B”). Effects at level C are proposed to be mediated primarily through the low-affinity glucocorticoid receptor (GR, Figure 2A).
6. Allostatic overload Type 2 occurs when allostatic load is chronically high, but an animal is not in negative energy balance. An ELHS is not triggered in this case. The precise point at which this occurs is not defined, as it is for Type 1 overload. Glucocorticoid levels are suggested to be chronically elevated (see #3 above), probably at the interfaces of levels B and C (Landys et al., 2006; Figures 1, 2B), although this has yet to be clarified. It appears that chronically elevated glucocorticoid levels may cause imbalances in sympathetic and parasympathetic tone as well as other hormones associated with homeostasis, food intake and reproduction (McEwen, 1998). These imbalances may be reflected in hypertension, insulin resistance, etc. (McEwen, 1998). Allostatic overload Type 2 would thereby include the concepts of wear-and-tear, metabolic syndrome, chronic stress, and so on, that are more typical in their biomedical usage.
7. Perturbation resistance potential, or PRP has been advanced as an important quantity in the framework of allostasis. It describes the difference between allostatic load and resource availability, Eg – (Ee + Ei), see Figure 3, and specifically reflects the vulnerability of an individual to the potential for allostatic overload Type 1 should additional perturbations or resource limitations occur (Table 1 and Figure 3). However, as Eo progresses overall allostatic load increases and PRP decreases. As such an individual must assess PRP as Eo changes.
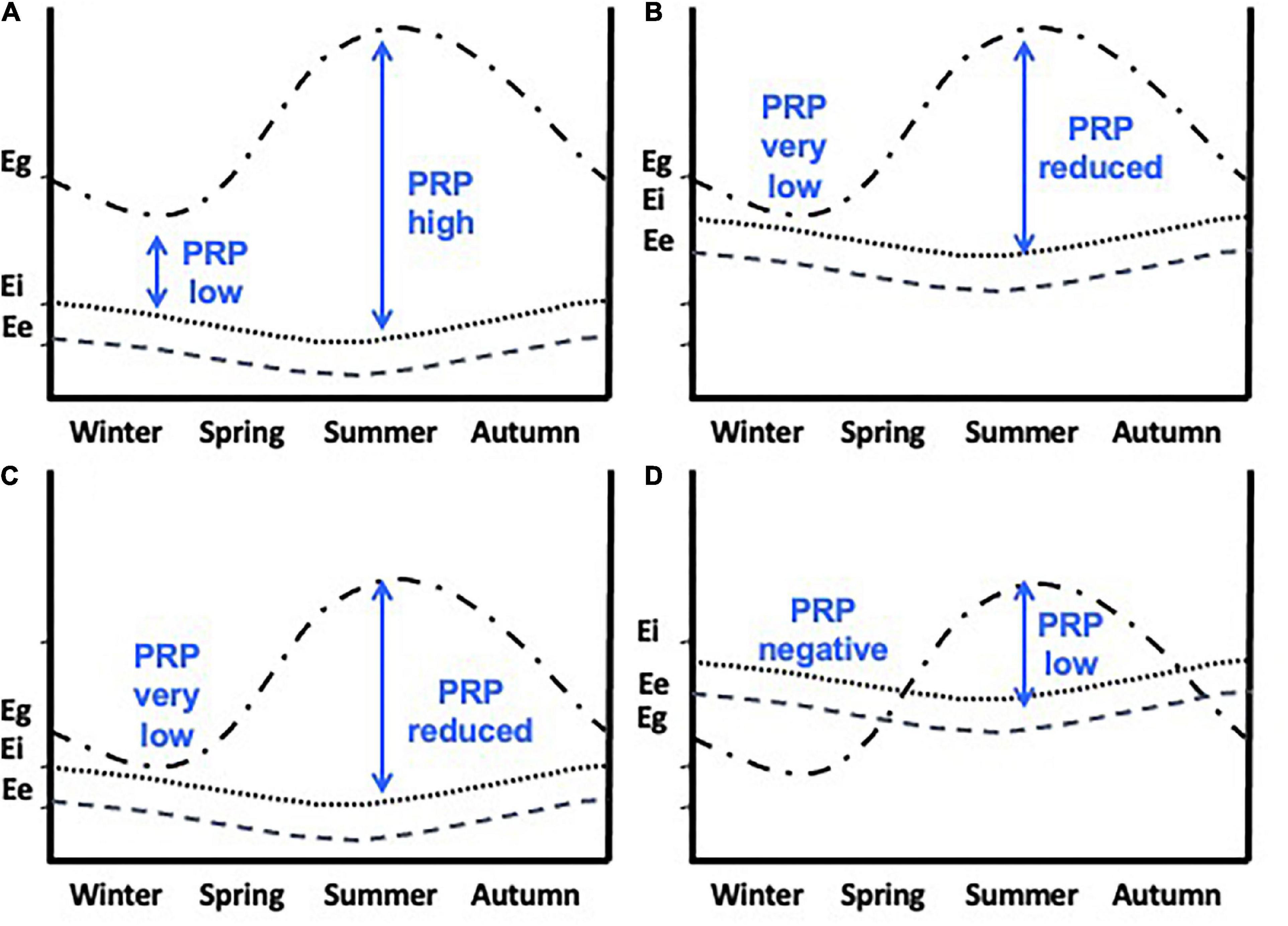
Figure 3. Perturbation resistance potential (PRP) in different seasons. Eg (also called Ecr – cumulative resources) is the cumulative energetic resources available to an individual. Ee represents the existence energy and Ei is the additional energy required to go about daily routines. PRP, Eg - Ee + Ei (vertical blue arrows). (A) PRP is higher in summer allowing more energetically demanding life-history stages such as breeding, molt and migrations to occur. (B) Ee + Ei are higher and because Eg remains unchanged then PRP is very low in winter and reduced in spring and summer. (C) Ee + Ei remain the same as in (a) but Eg is greatly reduced resulting in very low PRP. The worst-case scenario is shown in (D) where Ee + Ei are increased and Eg is reduced. Now PRP is negative in winter and greatly reduced in spring and summer. When PRP is low then extreme climatic events could result in allostatic overload type 1. From Wingfield et al. (2017). Courtesy of the Royal Society, UK.
While all these points are presented as a unified whole in the original model of allostatic load (with the exception of #7), many of them may stand (or fall) independently with variable effects on the coherence of the framework overall. These will be considered later, based on current evidence as well as arguments derived from other frameworks and concepts.
A complementary model: Reactive scope
The Reactive Scope model is an alternative to allostatic load, which was first detailed in an eponymous paper by Romero et al. (2009). It does not include Eg or PRP and the Reactive Scope model focuses more on the physiological actions of endocrine mediators, including the mechanisms by which adaptive responses indicate and generate physiological damage. Again, while the model itself may be summarized graphically (Figure 4), it is useful here to focus on the model’s components and how they relate to allostasis and predict changes in hormonal mediators such as corticosterone.
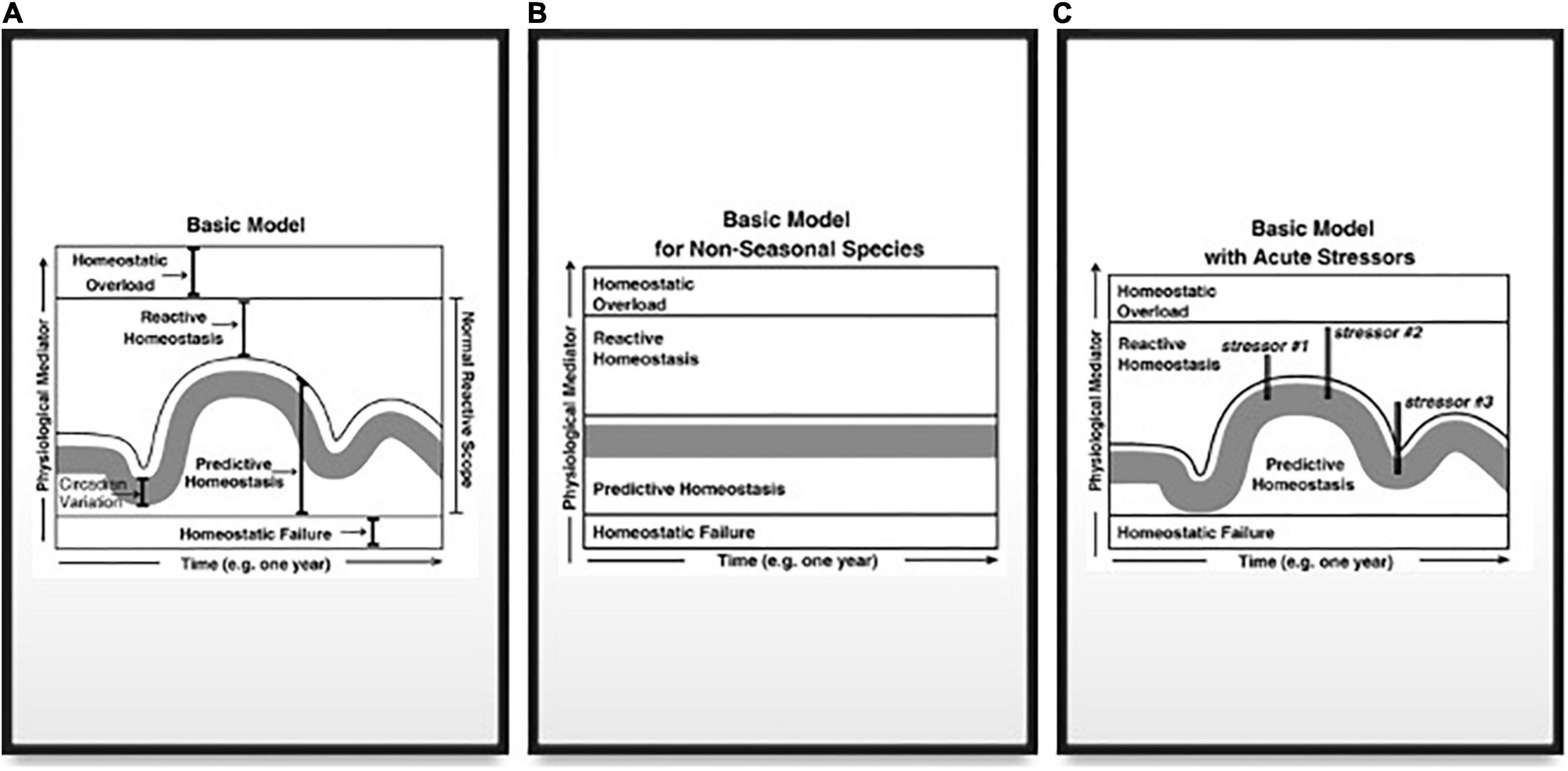
Figure 4. (A) Graphical model of the concentrations of different physiological mediators on the y-axis vs. time. The range of concentrations or levels of physiological mediators is broken into four ranges. The lowest range depicts concentrations/levels that are too low to maintain homeostasis and is termed Homeostatic Failure. The minimum required concentration/level forms a threshold that does not change over time. Above this threshold is the Predictive Homeostasis range that varies according to predictable life-history changes. The circadian variation in concentrations/levels is depicted as a gray bar (with the bottom being the circadian nadir and the top being the circadian peak). The range of Predictive Homeostasis varies depending upon life-history demands, and thus changes seasonally. The Predictive Range extends slightly above the circadian peak in each season to encompass predictable daily events such as foraging. Above the Predictive Homeostasis range is the Reactive Homeostasis range, which represents concentrations/levels of the physiological mediator necessary to maintain homeostasis following an unpredictable event that threatens homeostasis. The Predictive and Reactive Homeostasis ranges form the normal reactive scope for that physiological mediator. The upper limit to the Reactive Homeostasis range is the concentration/level where the mediator itself starts to cause damage, and the range above this threshold is termed Homeostatic Overload. The threshold between Reactive Homeostasis and Homeostatic Overload is presumed to not change on a daily or seasonal basis. (B) A simplified version of the graphical model presented in (A) in a non-seasonal species such as humans. (C) A graphical depiction of the response to stressors. Each vertical line represents both a rapid spike of the mediator into the Reactive Homeostasis range in order to maintain homeostasis in the face of a stressor and a rapid decrease in the mediator once the stressor has ended. Stressor #2 is a stronger stressor than #1 and thus requires a stronger response to maintain homeostasis. Stressors #2 and #3 are of equivalent strength but occur at different times of year. Consequently, the mediator is at different concentrations/levels in the Predictive Homeostasis range so that stressor #3 is less likely to elicit a response from the mediator that extends into the Homeostatic Overload range. From Romero et al. (2009) with permission and courtesy of Elsevier.
1. Predictive homeostasis (Figure 4) is the normal range (scope) of a mediator (such as glucocorticoids) that occurs in response to reasonably predictable events such as circadian changes, reproduction, or migration (as components of Ee and Eo).
2. Reactive homeostasis is the range of a circulating mediator (Figure 4) above predictive homeostasis, up to, but not including, the level where exposure to that mediator becomes damaging (wear and tear; Figure 4). Reactive homeostasis is the expected range when a healthy animal responds to, for example, an unpredictable perturbation. The point at which reactive homeostasis occurs is subject to change and is not easily predicted by the model.
3. Homeostatic overload occurs when high blood levels of a mediator cause damage (i.e., above the range of reactive homeostasis, Figure 4).
4. The reactive scope of blood levels of a mediator thus spans predictive homeostasis and reactive homeostasis ranges (Figure 4). It is the range of hormone concentrations extending from the minimum to maintain homeostasis up to the point where levels of a mediator cause harm.
Romero and Wingfield (2016) have discussed these two models together noting that “homeostatic overload” and “allostatic overload” are related but not identical – much as the different types of allostatic overload are not identical. Homeostatic overload is analogous to the “level C” regulatory range described in Landys et al. (2006; Figures 1, 2). It is most analogous to Type 2 allostatic overload where prolonged high hormone levels are damaging but an ELHS is not triggered. ELHS activation in Type 1 overload may lead to homeostatic overload, but not always. If ELHS activation does not cause damage (i.e., if it is short-lived), it would then fall in the range of reactive homeostasis and would be within the reactive scope because it is a normal and adaptive response to an abnormal perturbation. In those cases, Type 1 allostatic overload would be distinct from homeostatic overload. Some animals may rely on prolonged energy imbalance to trigger the ELHS.
It is interesting that when the range of predictive homeostasis is high in a particular life history stage, the extent that hormones may be elevated without inducing homeostatic overload may be smaller. If the point at which damage occurs does not change, this would result in a narrowed range for reactive homeostasis (Figure 4). In this way, reactive homeostasis may be considered an endocrine analog to the energetic PRP under routine conditions, i.e., the difference between the maximum that can be accommodated (or in this case, tolerated), and seasonally normal levels.
The relevance of time is another interesting difference between these two models. With allostatic load, it is necessary to select an appropriate time interval over which to average energy expenditure because large moment-to-moment changes in energetic quantities should not trigger overload. For example, animals are expected to exhibit circadian patterns in activity (Morton, 1967) with periods of active energy expenditure and periods of restfulness. Even within active periods, activity is often exhibited in irregular bursts. These routine fluctuations do not reflect the resource demand like total energy expenditure over a period of a day or longer does. This difference is implicitly recognized by the use of time-energy budgets (Pearson, 1954) and other integrated averages in the study of field energetics. Hence, some level of averaging is necessary for energy expenditure to reflect allostatic load. The most useful time interval for averaging is likely to vary by species, sex and season, based on variability in the duration over which an animal can tolerate negative energy balance as part of its daily or seasonal routine.
Integration of signal over time also makes the significance of an acute stress response unclear with regard to allostatic load. Among the sub-categories of allostatic load, Eo is meant to represent the cost of responding to “labile perturbation factors” (LPFs). LPFs occur over varying time frames and may be classified as direct – necessitating a shift to the ELHS – or indirect– where they may be disruptive and costly but do not initiate overload (Wingfield, 2005; Romero and Wingfield, 2016). However, where energetic and endocrine signals are integrated over time, there may be no measurable effect of the most acute LPFs such as escape from a predator, on either allostatic load or circulating glucocorticoid levels. It is possible that with a truly integrated measure of hormone levels (such as via fecal metabolite sampling) that recurrent acute events might contribute to measurable outcomes. However, the model does not specifically predict any cumulative consequences of such events. Following exposure to an LPF, and once allostatic load has returned to normal levels, the mediator control system essentially resets as if the acute event had never happened.
For reactive scope, acute responses fit into the model and are visualized as spikes in the reactive homeostasis range (Figure 4). Both frequency and duration of acute responses are expected to influence (reduce) the threshold at which homeostatic overload will occur (Romero et al., 2009). The extent that this happens is not predicted and may not be predictable because it requires information about the precise point where damage is caused by excess glucocorticoids. The target tissues that are potentially subject to damage are diverse. “Damage” and potential to affect fitness must be distinguished from standard metabolic consequences of activity, e.g., elevation in reactive oxygen species (Costantini et al., 2011; Wada, 2019), in order for such a point to be determined. Nonetheless, acute perturbations are known to influence subsequent responsiveness (Sapolsky et al., 2000) and may cumulatively contribute toward activation of the ELHS (Busch et al., 2008a,b). Such events, once passed, may not contribute to the energetic quantities of allostatic load or to the PRP, but they may be integrated into an animal’s perception of risk relative to the PRP, and may induce changes in feedback or other mechanisms that mediate the hormonal response to future energetic challenges.
While the emphasis of this section is on each model’s utility in understanding the function of glucocorticoid hormones, the reactive scope model is designed to more broadly consider the function and dysfunction of diverse mediators (Romero et al., 2009). Nonetheless, glucocorticoid physiology tends to dominate discussions of this model, thus far (Blas and Fairhurst, 2022). The basis for this, and the evolutionary history of glucocorticoid physiology, will be discussed in the next section.
Focus on glucocorticoids
Hormones are the primary mediators of allostasis because of their broad spatial and temporal reach compared with neural or paracrine signaling. Glucocorticoids, in particular, are believed to have originated in function as coordinators of environmental responsiveness (Baker et al., 2015).
Almost all modern vertebrates have two types of corticosteroid receptors, the so-called mineralocorticoid receptors (MR) and the glucocorticoid receptors (GR). MRs bind glucocorticoids in addition to mineralocorticoids while the lower affinity GRs bind exclusively to glucocorticoid hormones and are nearly ubiquitously expressed (Baker et al., 2013). MR and GR are homologous, with estimated duplication and divergence some 450 million years ago. This divergence occurred around the time when bony fish and cartilaginous fish diverged (Baker et al., 2013, 2015). Many modern fish have a third subtype from a more recent duplication of the GR gene. The ancestral corticosteroid receptor (CR) has been characterized as promiscuous based on its inferred structure (Ortlund et al., 2007). While the functionality of ancestral CR can only be surmised, it is interesting to note that lampreys (one of two extant Agnathan groups) express a single CR and utilize only one active corticosteroid hormone (11-deoxycortisol) to accomplish both ion regulation and reproductive inhibition (Close et al., 2010). Thus, two of the functions divided between mineralocorticoid and glucocorticoid hormones in modern vertebrates may have originally been performed by a single ancestral CR.
Linking organismal response to salinity with reproductive regulation in an anadromous lamprey may have been adaptive (Close et al., 2010). It is straightforward to imagine a need to segregate such responses, as evolving generations pioneered new environments with diverse osmotic conditions. Comparative sequence analysis suggests that GR rapidly diminished its hormone binding affinity after divergence (Carroll et al., 2011). The difference in sensitivity between GR and MR allows some segregation of function via amplitude of signal (Figure 2A) even in animals that employ only one corticosteroid (Carroll et al., 2008, 2011).
Tissue-level regulation of responsiveness is also likely ancestral and fundamental. Various modern elasmobranchs possess both receptor types, use corticosterone and 1α-hydroxycorticosterone (though not exclusively) as potential agonists, and express 11β-hydroxysteroid dehydrogenase (HSD) type 2 enzymes (Carroll et al., 2008; Baker et al., 2013). 11β-HSD2 breaks down corticosterone and cortisol thereby protecting MR-expressing tissues from glucocorticoid activation. These components likely co-evolved to generate a well-segregated dual-function corticosteroid response system (Baker et al., 2015).
The ubiquity of this organizational arrangement among a promiscuous family of steroid receptors and enzymes suggests that the segregation of corticosteroid function may be an important correlate of vertebrate evolution. However, it is not only this segregation that seems to be highly conserved. A suite of functional responses for which glucocorticoids are known in terrestrial vertebrates is also evident in more ancestral lineages like elasmobranchs. These responses include mobilization of energy substrates like glucose and free fatty acids, inhibition of growth, and modulation of immune responsiveness (reviewed in Carroll et al., 2008). It should be emphasized that components of the HPA axis leading to release of glucocorticoids and the effects downstream (binding protein transport, receptors and target tissue enzymes and other factors regulating glucocorticoid function) are conserved, but how they are involved in activation and modulation of the adrenocortical response to stress are variable across species as well as among and within individuals (Romero and Gormally, 2019). However, despite the high degree of variability in specific functionality across species, there is reason to believe that the multifaceted role of corticosteroids is conserved, and thus, conducive to theoretical generalizations. It is useful to keep this ubiquity across vertebrates in mind when faced with frustratingly contradictory examples in how this role is manifested. Despite these contradictions, generalizations should be possible and practical in such a well-conserved system.
Focus on the threshold
Both allostasis and reactive scope frameworks describe changes in mediator (glucocorticoid) levels across the full spectrum of possible concentrations. While a continuum between baseline and peak concentrations necessarily exists, MR and GR (including their putative membrane-bound forms) have very different binding characteristics (Prager et al., 2010), which are widely expected to be the basis for the discrete functions of different hormone concentrations. Nuclear MR has high affinity but low capacity and becomes quickly saturated at lower concentrations whereas nuclear GR has a binding affinity an order of magnitude lower or more than MR and remains minimally occupied until hormone levels are elevated. Membrane-bound receptors may be almost entirely inactive at the lowest physiological concentrations, at least in birds (Breuner and Orchinik, 2009). Sapolsky et al. (2000) attribute a categorical difference in function to GR and MR, with permissive roles being effected at low hormone concentrations through the MR and suppressive/stimulating/preparative roles enacted by “stress-induced” GR-effected concentrations.
Such distinction in binding affinity and function is broadly supportive of a threshold-based activation model. However, the threshold at which the ELHS is believed to be activated is not clearly defined by receptor occupancy. Receptor activation has been discussed in the context of allostasis by Landys et al. (2006, Figure 2). They suggest that the basal concentrations at which MR occupancy occurs constitutes level A (Figure 2), where glucocorticoid levels are low and binding of MRs occur at an exponential rate. However, this basal regulatory level is rarely observed (Landys et al., 2006). As plasma glucocorticoids increase, MR occupancy reaches its peak and asymptotes while the rate of GR occupancy occurs more slowly (physiological state levels B and C, Figure 2). The difference between levels B and C (intermediate and maximal) in this model seems to be a matter of degree of occupancy of the GR and membrane receptors (Figure 2).
This leads to the question, what is the nature of the threshold? Both allostasis and reactive scope models designate a point at which “overload” results from elevated mediator levels, which in turn, leads variously to activation of the ELHS and/or damage. However, the mechanism by which such a threshold might be effected is unknown and likely varies according to context of the response, seasons, sex and so on (Romero and Gormally, 2019). It could arise based on a critical occupancy of certain receptors, by a ratio of occupancy of different receptors or by accumulation of products over time. It could even be determined by some feature independent of receptor binding, e.g., displacement of other steroids from their ligands or actions of peptides that occur elsewhere in the HPA cascade such as CRF (corticotropin-releasing factor) or ACTH (adrenocorticotropin hormone).
Thus, despite the impressive conservation of this signaling system among vertebrates, the complex ways in which they activate and modulate the response makes it difficult to hypothesize a complete, stimulus-to-response mechanism (Romero and Gormally, 2019) by which either the ELHS might be activated or damage might occur. This problem has frustrated attempts to understand glucocorticoid physiology as a whole. However, the activation of a well-conserved system with a threshold-based outcome – even if the proximate mechanism is incompletely understood – may still be clarified based on the outcome itself and the proximate and ultimate causes related to its occurrence. Because we have satisfied the condition that the mechanism, whatever it may be, is broadly similar among animals, we may focus on the causes and consequences of its functionality.
Thresholds that are defined by “damage” (such as Type II allostatic and homeostatic overload) are difficult to pinpoint especially when damage accumulation and repair mechanisms are also involved (see Wada, 2019). The ultimate adaptive value of damage thresholds is unclear and should only arise by constraint. Type II allostatic overload is thought to be rare in the wild. However, when the threshold is an allostatic shift, as in Type I allostatic overload with activation of the ELHS, both the nature of the threshold and its ultimate function are clear. In response to a challenge, an adaptive hormonal response that drives physiology toward the ELHS should be driven by proximity to the energetic threshold at which activation of extreme conservation measures becomes necessary. In the allostasis model, that threshold is defined by a narrowing scope between energetic requirements and resource availability, i.e., by PRP (Figure 3).
The original model of allostasis predicted that blood glucocorticoid levels should change with allostatic load. The PRP, in part, is determined by allostatic load. If resource availability is constant, then change in allostatic load should constitute change in PRP, but change in allostatic load may not reflect proximity to its threshold. In cases where Eg is very high, for example, changes in allostatic load may constitute proportionally minor changes in the PRP. This could explain the absence of clear correlation between allostatic load and glucocorticoid levels in previous studies (Word et al., 2022). Similarly, changes in resource availability should play little role when resources are higher than energetic requirements. Another possibility is that change in PRP – either by change in allostatic load or change in resource availability or both – induces change in glucocorticoid levels only when PRP becomes critically low. If that is the case, there remains a question as to how low and how to empirically test it.
If the ELHS is the functional threshold where glucocorticoid elevation drives physiology, and if PRP = 0 (i.e., there is no difference between allostatic load and resource availability) and is the point at which the ELHS should be activated then there are two possible endocrine strategies (shown in Figure 5). In the case of strategy 1, glucocorticoids gradually elevate in direct (inverse) correlation with PRP when it is low (near 0) and reaches the threshold that the ELHS is activated only when PRP = 0. Alternatively, an animal may maintain glucocorticoid levels in the region of a seasonal baseline (across life history stages, Figure 6) until and unless PRP is critically low (strategy 2, Figure 5). In strategy 2, only negative energy balance results in a rise in glucocorticoid levels that trigger the ELHS. In this case, no correlation between PRP and glucocorticoid levels would be observed under most conditions.
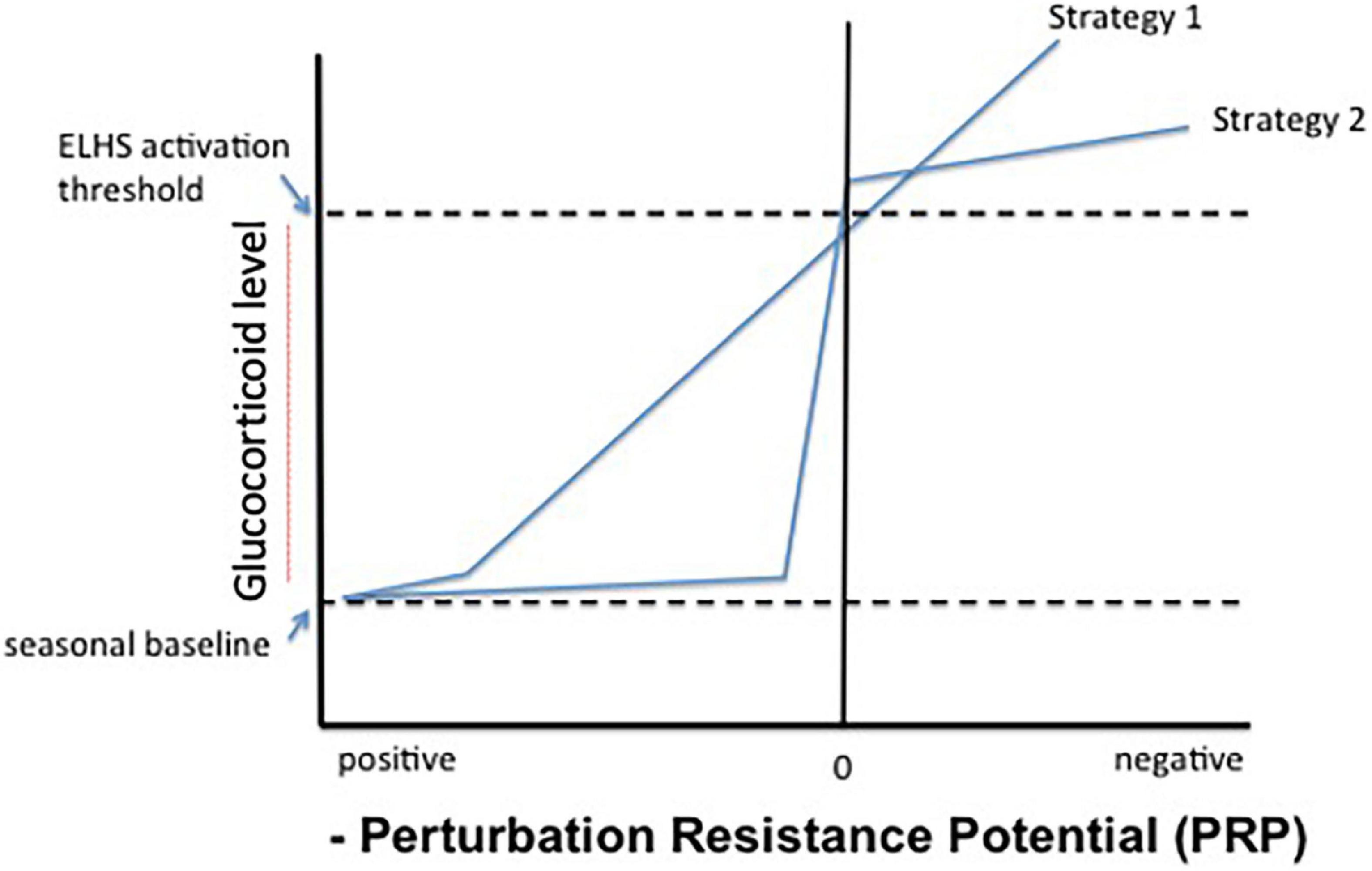
Figure 5. Possible relationships between integrated perturbation resistance potential (PRP) and circulating glucocorticoid levels. Strategy 1 shows a continuous and linear relationship between PRP and corticosterone, effectively substituting PRP for allostatic load in previous versions of the model. In strategy 2, only negative energy balance, or acute perception thereof (PRP ≤ 0) results in sharply elevated corticosterone levels that promptly trigger an emergency life-history stage (ELHS).
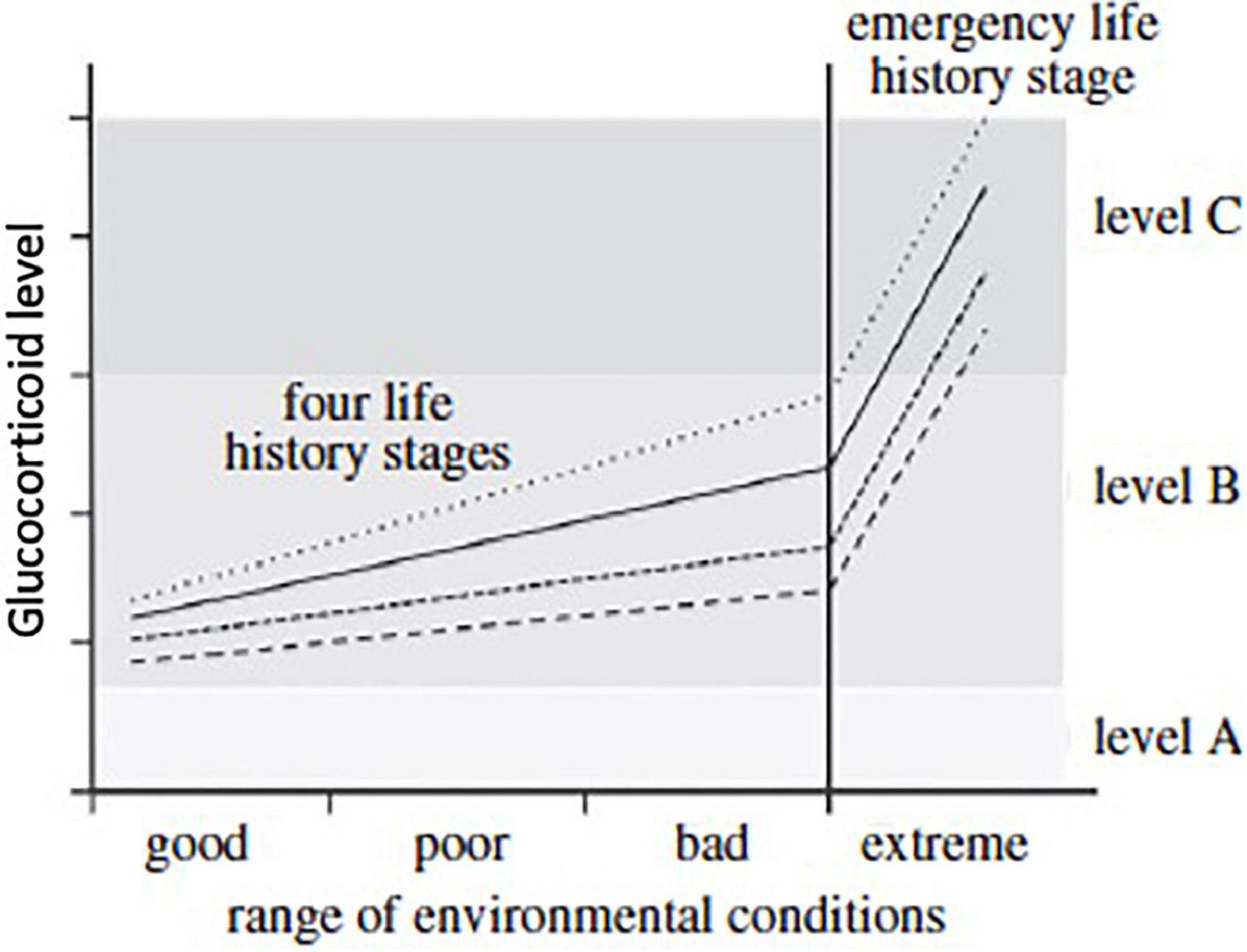
Figure 6. The hypothetical relationships of circulating glucocorticoids and the range of environmental conditions expected when a specific life-history stage is expressed (strategy 2, Figure 5). Environmental conditions can be expected to be good, poor or bad for each life-history stage. Baseline glucocorticoid levels in blood tend to increase slowly as conditions deteriorate and increase allostatic load. These concentrations rise above level A (homeostatic level, lightest shade of gray) and within level B (reactive scope for that life-history stage, middle shade of gray). When conditions become extreme (right hand vertical line) then glucocorticoid levels increase rapidly to level C as a result of allostatic overload; that then triggers facultative responses such as the emergency life-history stage (darkest shade of gray). The slope of the lines for circulating corticosteroids will vary among life-history stages. Four are presented here with one showing low baseline and rate of increase as well as maximum level. Such variation in the dynamics of glucocorticoid responses to deteriorating weather events and stress in general are common. From Wingfield et al. (2017) courtesy of the Royal Society.
If intermediate hormone concentrations promote sub-threshold physiological changes in a dose-responsive fashion that facilitate coping under conditions of reduced, but not critical, PRP, then strategy 1 might be expected. This scenario is depicted in physiological state level B of Figure 2 (Landys et al., 2006). Because it is the expectation and assumption upon which the current model is constructed, then elevated glucocorticoid levels may also increase the probability that additional perturbations will lead to ELHS activation. Some animals may be more likely to exhibit strategy 2, keeping responsiveness suppressed until PRP approaches 0. The point at which an animal responds to changing PRP with an elevation in glucocorticoid levels may tell us something about the potential trade-off presented by ELHS activation in the particular species and context under examination.
Scenarios resembling Strategy 2 have been further explored in Wingfield et al. (2017). The hypothetical relationships of circulating glucocorticoids and the range of environmental conditions expected when a specific life-history stage is expressed (strategy 2, Figure 5) are given in Figure 6. Environmental conditions can be good, poor or bad for each life-history stage. Baseline glucocorticoid levels in blood tend to increase slowly as conditions deteriorate and increase allostatic load (strategy 2). Glucocorticoid concentrations rise above level A (homeostatic level, lightest shade of gray) and within level B (reactive scope for that life-history stage, middle shade of gray). When conditions become extreme (right hand vertical line) then glucocorticoid levels increase rapidly to level C as a result of allostatic overload, which then triggers facultative responses such as the ELHS (darkest shade of gray). The slope of the lines for circulating corticosteroids will vary among life-history stages. Four are presented (in Figure 6) with one showing low baseline and rate of increase as well as maximum level. Such variation in the dynamics of glucocorticoid responses to deteriorating weather events and stress in general are widespread.
Crossing the threshold: Glucocorticoids, perturbation resistance potential, and starvation physiology
For the PRP to be a useful metric, it must be measurable. This is true for the scientist/modeler but also for the organism that is expected to respond to the quantity in question. Resource availability may be estimated via existing research methods, but these approaches require extensive knowledge about resource utilization, are imprecise, and are generally unable to account for variability in access to resources (Goymann and Wingfield, 2004). Consequently, it may be more accurate, and more interesting, to consider the means an animal might be expected to employ to detect a strong enough decline in PRP to merit an endocrine response.
To achieve this goal, we must define what it means for the PRP to equal zero. One complication of PRP near zero is how we assess the expected role of routinely accessed body reserves like glycogen and fat. Type I allostatic overload is often described as if it were synonymous with negative energy balance, but some animals routinely burn fat during the night (Hatchwell et al., 2009) and they are not in allostatic overload. Based on the role assigned to fat reserves in other iterations of the model, negative energy balance actually occurs prior to allostatic overload. According to Wingfield (2004), fat acts as a buffer against overload by providing an energy source to supplement Eg. Fat depot has specifically been modeled as a subtraction from the cost of perturbation, Eo, but it may be more reasonable to consider body reserves as included in the energy availability metric (Wingfield, 2004). An alternate term to Eg is Ecr (i.e., the cumulative available resources), which has more recently been applied (Wingfield et al., 2017; Figure 1B and Table 1).
Aligning the expected glucocorticoid response with studies of the endocrine response to starvation requires adjusting the model to include fat reserves in the energetic term such that PRP = 0 is the point where fat is exhausted or insufficient to fulfill metabolic demands. This resolves an apparent contradiction that has led to some contention in development of the model (Romero et al., 2009; McEwen and Wingfield, 2010; Romero and Wingfield, 2016). Starvation occurs in three physiological phases: Phase I: dietary energy and carbohydrate stores are first exhausted, Phase II: fat stores are utilized while protein is spared and metabolism is depressed and Phase III where protein oxidation (deamination) is added to remaining lipid metabolism at the expense of functionality in protein-rich tissues (Goodman et al., 1980; see also Romero and Wingfield, 2016). In most species, glucocorticoids are elevated during both Phase I and Phase III starvation, but many species actually display low levels of glucocorticoids during Phase II (Cherel et al., 1988). The suppression of glucocorticoids in Phase II has been cited as an argument against the applicability of the allostatic load model (Romero et al., 2009) and against the relevance of starvation (as opposed to energetic demand) in defining the term (McEwen and Wingfield, 2010). However, if Ecr replaces Eg in the model, meaning allostatic overload does not occur until Phase III starvation, it satisfies the requirement that overload occurs concurrently with elevated glucocorticoid levels. Glucocorticoid suppression during Phase II starvation suggests a non-linear increase which is, perhaps, more consistent with strategy 2 in Figure 5 where glucocorticoids increase exponentially near PRP ≤ 0 and the ELHS activation threshold is surpassed. Alternatively, it may suggest that strategy 1, where glucocorticoids increase more linearly in response to changes in PRP may be more complex, with acute suppression preceding full activation.
In considering a non-linear approach to ELHS activation, it is interesting to note that a variety of acute changes in hormone levels could still approximate strategy 2 (Figure 5) if they are integrated over time. Endocrine responsiveness to frequency of activation, as well as magnitude, is established in a number of systems. This potential for complex signal structure is particularly notable with regard to glucocorticoid regulation in mammals where significant effects are induced in response to changes in pulsatility (McMaster et al., 2011). Even in birds, repeated acute stressors have been found to induce symptoms of chronic stress (Busch et al., 2008a,b), which suggests that such events could cumulatively lead to ELHS activation. Indeed, that animals do respond to non-starvation “stressors” via acute elevation of glucocorticoid hormones indicates that there is more that goes into ELHS activation than just internal sensation of substrate biochemistry. Further research will clarify these issues or point in other directions.
Crossing the threshold: A neuroendocrine decision
Why respond to cues that do not directly reflect starvation? In most cases, an optimal response will occur before PRP < 0, but when PRP is in decline substantially (strategy 1, Figure 5). “Indirect” LPFs do not constitute a direct threat to energy balance, because they tend to be brief (such as a predation attempt, social challenge or sudden extreme weather event) but often involve an energetic cost and may elicit a glucocorticoid response potentially contributing to ELHS activation. In important ways, the elevation of glucocorticoids – acutely or chronically – toward the threshold of ELHS activation may be functionally similar to behavioral decisions that animals make. In the absence of a quantitative metric of PRP, animals must rely on cues that reflect both their internal energetic status and a potentially diverse and changing array of cues that provide information about Ecr. To understand and model ELHS activation further, we must make use of studies addressing the methods by which animals use information from their environment to make survival-related decisions of other kinds.
A decision to elevate glucocorticoid levels to threshold and engage the ELHS, resembles the decision that prey makes in response to a perceived predation threat. When a predator is approaching, an animal does not necessarily flee automatically, but instead initiates flight at the point where the risk of remaining outweighs the cost of surrendering their location. Flight-initiation-distance has been used extensively as a metric to investigate behavioral decision-making in response to a perceived predation threat. It is typically defined as the distance an organism is from a perceived threat when it chooses to flee (Ydenberg and Dill, 1986; Stankowich and Blumstein, 2005). The factors affecting flight-initiation-distance may be divided into cues that indicate the probability and severity of the threat posed by the predator and those that reflect the cost of departure. The degree of threat can be indicated by a predator’s presence (Kats and Dill, 1998; Bytheway et al., 2013; Smolka et al., 2013) and/or attention, predator velocity, prey distance from cover (Bateman and Fleming, 2015), body condition (Seltmann et al., 2012), or crypsis (Heatwole, 1968) can affect flight-initiation-distance. A prey animal’s experience with predators (Bateman and Fleming, 2013; Ramasamy et al., 2015) and habituation or sensitization to stimuli (Blumstein, 2016) are also relevant in determining the likelihood of capture. Cost of departure may be reflected by a variety of habitat-related factors, including size and density of food (Ydenberg and Dill, 1986; Møller et al., 2015), territoriality (Stankowich and Blumstein, 2005) and competition (Barnard, 1980). Flight-initiation-distance can vary seasonally (Uchida et al., 2016) and with change in climate (Møller, 2014).
What is particularly interesting about flight-initiation-distance, in contrast with the literature on “stress,” is the clarity of interpretation that becomes possible when the outcome is a decision with straightforward implications rather than a murky and self-referential continuum like “stress” is often treated. That leads to the question, is ELHS activation a decision in the same sense that flight-initiation is a decision? The fundamental similarities in these different trade-offs are remarkable. Instead of deciding if or when to flee from a predator and risking the costs of errors therein incurred, an organism engaging in decision-making about activating the ELHS must navigate risks associated with remaining in its normal seasonal life-history stage to face an energetic crisis unprepared, or by reducing the duration over which such a crisis can be survived. Rather than the cost of surrendering a physical location the cost includes surrendering fitness-related investments associated with the normal life-history stage and also potentially increasing the risk of damage associated with Type II/allostatic overload. Furthermore, ELHS activation is functionally comparable to a behavioral decision. Despite tissue-specific mechanisms for regulating hormone levels, the primary activation of glucocorticoid signaling is generally agreed to commence at the hypothalamus, with the neuroendocrine secretion of CRF (e.g., Sapolsky et al., 2000; Romero and Wingfield, 2016; Blas and Fairhurst, 2022). Neural integration is required to synthesize the diverse range of established internal and external cues that are known to influence hypothalamic responsiveness (Angelier and Wingfield, 2013; Wingfield, 2015; Romero and Gormally, 2019). In addition to direct physiological metrics, perception and higher cognitive functions are directly and intimately involved. This could be an exciting and productive area of research. However, a functional parallel is not a prerequisite for useful incorporation of decision-making research into this theoretical framework. While physiology can introduce unique opportunities and constraints in response mechanisms, the optimization of response to threat in a context of variably reliable information is a truly universal challenge.
Error management and bias in the glucocorticoid response decision
Decision-making is a common subject of interest across disciplines. While there is no single way to understand all decisions, the same themes evident in flight-initiation decisions recur in the activation of the ELHS, i.e., error and risk, probability and severity, and cost and benefit. Johnson et al. (2013) performed a cross-disciplinary synthesis that focused on the origins of cognitive bias and the importance of error in an explanatory framework. They called this framework error management theory (Johnson et al., 2013). It predicts the presence and direction of bias in decision-making based on the costs involved in different types of errors (Johnson et al., 2013). Errors may be classified as false positive, where an organism responds to a threat that doesn’t materialize; or false negative, where they fail to respond to a genuine threat (Johnson et al., 2013). Relevant decisions should favor the least costly error. Even where cognition should be adequate to execute a nuanced decision, as expected in human psychology, innate biases can save time and maximize the probability of survival. “A behavior that seems ‘biased,”’ the authors note, “would not seem biased if we had complete information about the consequences of those actions for survival and reproduction” (Johnson et al., 2013).
This approach to bias in decision-making can be applied to glucocorticoid responsiveness leading to ELHS activation. There are circumstances under which there is no uncertainty about the need to respond– the immediacy of a threat is clear when PRP = 0 and energy reserves are exhausted. However, for an animal relying on anticipatory or indirect cues to estimate PRP, uncertainty is inherent in the decision to respond. The choice to respond (or not) to an expected cue may be interpreted as a source of information about the direction of bias. In the framework of error management theory, this direction provides information about the relative historical costs of different response errors in ELHS activation. To some extent, this is done routinely: unexpected results are often accompanied by identification of a particularly likely hazard, or speculation as to why some stimuli are perceived as “stressful.” For example, many birds become less responsive to capture-handling stress (as measured by glucocorticoid elevation) during molt, and this has been interpreted to indicate that the inhibitory effect of glucocorticoid hormones on feather development are excessively costly (Romero and Remage-Healey, 2000; Cyr et al., 2008). This is true (Lattin et al., 2011) but the fact that birds do elevate glucocorticoids while molting suggests that the story is incomplete. Specifically, birds may trade-off the risk of glucocorticoid interference in feather growth with the risk of failing to respond, resulting in differing bias in the response decision. The literature is rife with anecdotal discussions of risk, and, while they are extremely useful, until we can design a model that considers a larger suite of risks, we can expect that our model will fail to generalize. No matter how valid one component of risk is a single advantage or disadvantage is almost never the sole basis for a decision.
Rather than concluding with anecdotal explanations, what if our conceptual framework allowed aberrant data to be fed into a broader evaluation of risk thereby allowing the inclusion of situational, experiential, and evolutionarily relevant indicators of declining PRP? What if these data could improve the power of the model to infer meaning and predict not only responsiveness to stimuli but developmental plasticity, or inter- and intra-individual variability? What if such a framework could be applied consistently across species, populations, sex, life-history stages, and even individuals? Existing models are far from achieving this capacity, but it may be possible. Before such a model can be conceived, its underlying principles must be established. If we accept error management theory as an applicable tool and energetic crisis/low PRP as the critical risk of a false negative error (non-response to a real threat), the next step toward a model that utilizes existing data requires that we explore the interpretation of such data through this lens.
Error and the emergency life-history stage: Interpreting responsiveness to diverse cues
When interpretation is constrained to managing errors in a decision to engage the ELHS, stimuli previously described as classical “stressors” may be reclassified as cues that an animal uses to indicate the probability and/or severity of a threat to the PRP. Table 2 groups these diverse stimuli into general categories, identifies their relevance to the PRP, describes situations in which they may be most useful with respect to error management, and identifies scenarios where the use of these cues may result in increased error. In favoring the risk of a false negative error, some animals may rely on prolonged energy imbalance to trigger the ELHS.
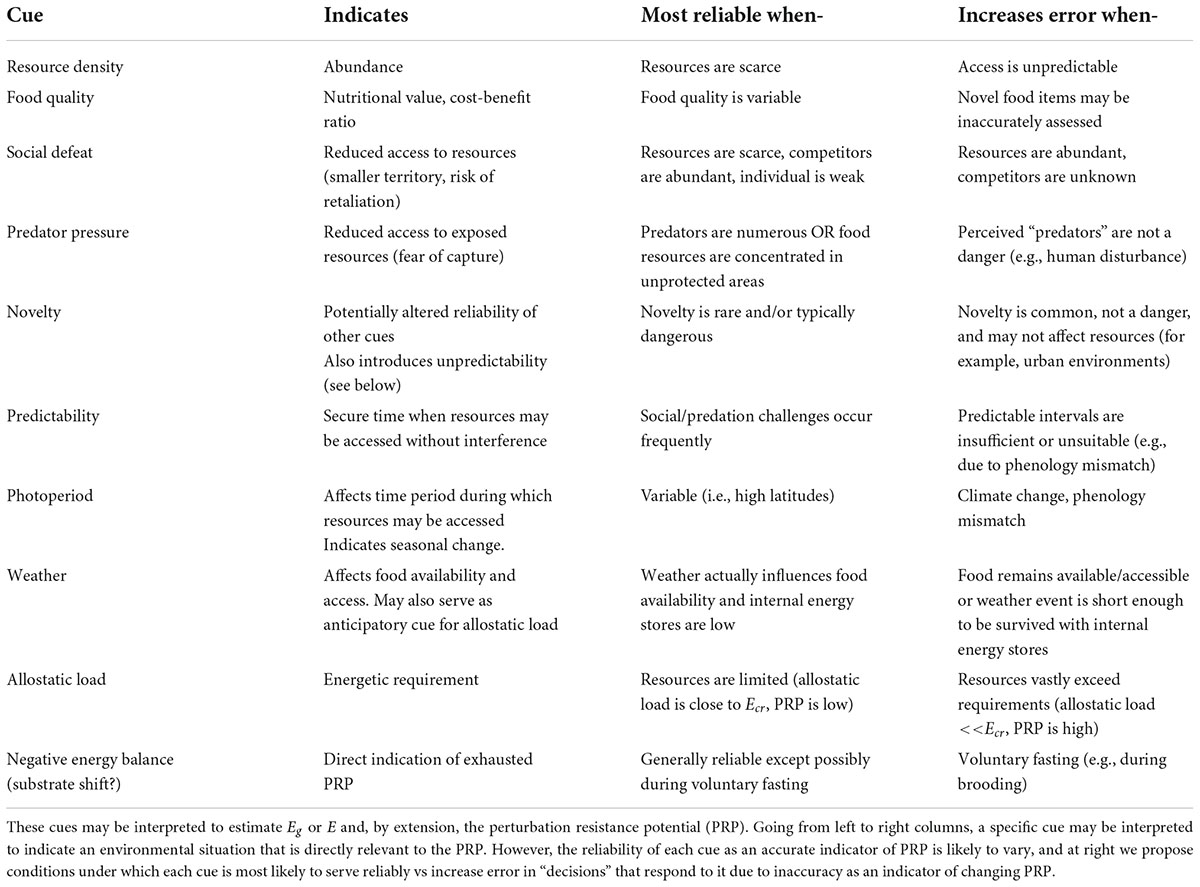
Table 2. Cues that may be integrated to decide whether to respond by elevating glucocorticoid levels.
There are many types of questions that can be asked to understand how an animal uses cues to decide when to elevate glucocorticoids. Qualitatively which cues does the animal respond to, and which does it ignore? Do the types of cues change seasonally? Quantitatively, inquiry may be cue-specific (how sensitive is an animal to a particular cue?) or general (how responsive is the animal to the most relevant cues?). Variability is also of interest because phenotypic variation and plasticity are determined through evolution, not just by proximal mechanisms. In addition to its utility in interpreting data, variability also has specific implications for the individual’s ability to respond to environmental change. Each of these will be considered, in turn, in the following sections.
Interpretation of qualitative information and cue-specific responsiveness
The cues to which an animal responds by elevating glucocorticoids should reliably reflect or predict an impending energetic crisis. However, there are many different types of cues that may be indicative, and their reliability is likely to vary between environments and even with some types of individual variation (Table 2). Actual resource density, when it is low, is almost always expected to be a reliable indicator of critically low PRP, with the exception of animals that hibernate, aestivate, or routinely fast through times of scarcity. But even where resource density is high not all individuals have equal access to resources (Table 2). For instance, social defeat, predation pressure, and novel changes in the environment can potentially have reliable and negative ramifications for resource accessibility under the right circumstances. Predictability of “stressor” delivery is known to mute the glucocorticoid response to other cues, and positively affects resource availability by establishing intervals when foraging may be performed safely (Cyr and Romero, 2009).
Within the categories identified in Table 2 each species must rely on a unique suite of cues to identify predators, conspecifics, variation in weather, desirable and undesirable food sources, and so on. However, by considering types of cues, we may use categorical responsiveness based on an error management model to make broad inferences about the conditions historically faced by that species. Because the most relevant cues are likely to differ by season, life history stage, geographic location, social structure and other factors (Table 2), the variability in responsiveness over time and across populations further adds to the specificity of that inference.
Through the lens of error management, non-responsiveness to cues of a potential stressor also has specific implications that are often overlooked in standard capture-stress protocols. In viewing responsiveness as another form of information about the utility of cues, the first interpretation of non-responsiveness is that the stimulus is not a historically relevant predictive cue for the species in question. While the failure to respond to a standard capture-stress protocol may reflect generalized non-responsiveness (a characteristic discussed below), there are circumstances under which it simply may be an insufficiently reliable cue for that species or in that context. For example, lack of response may occur in areas where predation is minimal, or when an animal has become habituated to human contact (i.e., has learned that human contact is not a reliable predictor of low PRP, or lack of prior experience with humans as predators in general as seen in some isolated species). The interpretation of non-responsiveness to restraint-stress as generalized suppression should, in this framework, be secondary, and ideally supported by additional evidence of non-response to other common and predictable cues of low PRP.
Interpretation of variation in general responsiveness
General responsiveness is also expected to vary. The question of when and where responsiveness is broadly adaptive has been extensively considered by Wingfield et al. (2011). Here, the authors assert that ELHS activation should be unfavorable/unlikely in environments characterized by extremely high predictability but also extremely low predictability over the course of evolution (Wingfield et al., 2011). Because perturbations are rare in highly predictable environments, few cues would be reliable predictors of a critical perturbation; thus, a lack of response would almost never be an error. As such, an animal may not respond to many possible cues yet an irrefutable cue such as prolonged negative energy balance would still be expected to initiate an ELHS. In the case of extremely low predictability (high environmental stochasticity), cues indicating perturbations may be unreliable, not because perturbations are rare but because they are frequent and occur unpredictably. Responding frequently to unreliable cues would result in numerous false-positive errors, which may be maladaptive by inhibiting reproduction and other fitness-enhancing strategies in otherwise survivable circumstances. In these cases, an error management perspective would also predict that an organism would reduce its responsiveness overall, again with an exception for hard physiological evidence of ongoing crisis (Table 2). Both predictable and unpredictable environments stand in contrast with an environment that has unknown or unpredictable cue reliability. There is an important difference between knowing that many or most cues are unreliable, as occurs in species that evolved in highly stochastic environments, and not knowing which cues might be reliable, as occurs in a habitat – or climate – to which a species is new or not adapted to.
General responsiveness may also be considered analogous to bias in the context of error management theory (Johnson et al., 2013). When the probability of an outcome is uncertain, overall decision bias is expected to occur in the direction of the least costly error. Thus, a high level of generalized responsiveness, would constitute a bias toward over-responding. A smoke detector is a common example of the utility of an over-response bias (Nesse, 2005) because the consequences of failing to respond are extreme compared with the relatively minimal costs of false activation. So, too, is responsiveness to cues of an impending energetic crisis. However, the balance of costs is expected to change seasonally, and this, in turn, should predict the magnitude and direction of bias in overall responsiveness. If a bias toward under-response is observed, an error management model would assume that the fitness cost of a false positive exceeds that of a false negative as in an environment where perturbations are often comparatively short or mild. Responsiveness might also be minimal and limited to cues most proximal to an actual crisis, like starvation, in cases where perturbations can quickly and easily be escaped such as via altitudinal migration.
Intra-individual variation: Seasonality
The relevance of different types of cues change across seasons, but it does so more when seasonal or life-history changes are large, as in long-distance migrants. Hence, cue use (Table 2) should change qualitatively throughout the year. Because the costs that influence response bias change seasonally, general responsiveness is also expected to change across seasons. During the breeding season, the consequences of a false positive response are much more severe, because it may result in current reproduction being abandoned when a threat is not imminent. This false positive is particularly consequential for animals with a short breeding season, a fast pace of life or those with low potential for future reproduction (Bókony et al., 2009). When the consequences of false positive errors become sufficiently dire, selection may favor an increase in tolerance of false negative responses to reduce the probability of incorrectly activating the ELHS and inhibiting reproduction. Thus, an animal should become less likely to respond to all but the most reliable cues. This may explain why animals with short breeding seasons, such as arctic-breeding songbirds, often display muted or no elevation in glucocorticoid levels in response to a stressor when nesting (e.g., Wingfield and Romero, 2001; Krause et al., 2018).
Conversely, when not faced with a trade-off between survival and reproduction, the consequences of a false positive response are minimal and an animal is expected to be heavily biased toward over-responsiveness (i.e., to increase survival). This lack in consequences to over-response when fitness trade-offs are not as prevalent is never entirely true due to the damaging nature of chronic glucocorticoid exposure (Wada, 2019). However, when coupled with habituation or behavioral responses that minimize over-stimulation by common cues, elevated general responsiveness may be expected when fitness investments related to the typical functioning of life-history stages are low. This may explain why the same arctic-breeding songbirds have a highly responsive HPA axis to environmental extremes when they have recently arrived on the breeding ground but have not yet committed to nesting (e.g., Wingfield and Romero, 2001; Krause et al., 2018).
Intra-individual variation: Plasticity
It is in the face of changing environments that most types of variability are expected to occur. Developmental plasticity, habituation, and sensitization are all mechanisms by which an organism can learn to optimize its choice of cues and its general and cue-specific responsiveness to its current environment. Plasticity may occur within or across generations (Snell-Rood and Snell-Rood, 2020). In a changing environment, plasticity is an important alternative to reliance on instinctive responses shaped through evolutionary history (Van Donselaar et al., 2018). The extent to which such plasticity can occur is, itself, likely to be shaped through evolution in environments subject to change.
Novel environments should initially cause generalized sensitization because they reflect unknown cue reliability (which, again, is different from known low reliability). However, the ability of animals to habituate to “stressors” reflects the ability to learn about cue reliability and reduce responsiveness to specific stimuli accordingly. It is interesting to note that habituation to novel stimuli is often accompanied by potentiation of the response to other novelties and reflects both a generalized response to novelty – i.e., sensitization – coupled with a protective response to prevent repeated false-positive responses to a specific unreliable stimulus.
Inter-individual variation
Inter-individual variation may occur as a consequence of the very different environments experienced by individuals. It may also reflect phenotypic diversity in hereditary responsiveness arising through shifting selection pressures, as occurs with other phenotypes under variable selection. Differing hereditary behavioral phenotypes with distinctive profiles of stress responsiveness and aggression have been identified as “Hawks” and “Doves,” with each having its own suite of advantages and disadvantages resulting in the maintenance of both types over the course of natural selection (Korte et al., 2005). The effect of social structure on genetically heritable phenotypes may be complex due to epistatic effects between individuals (Sinervo et al., 2008). However, to some extent such phenotypes may be established by epigenetic or other mechanisms during development (Weaver et al., 2004). The extent to which such developmental programming occurs or endures is likely to vary considerably between species (Crino et al., 2014).
Risk of false positive errors: Variation in outcomes of emergency life-history stage activation
The ELHS consists of a highly conserved suite of physiological and behavioral responses that are characteristic of glucocorticoid action across species, foremost of which is cessation of the normal life-history stage, with particular emphasis on reproductive inhibition. However, the conserved nature of this core response does not prohibit variable association with diverse behavioral outcomes. Once neural integration has led to an endocrine decision – elevated secretion of glucocorticoid hormones – the positive and negative impacts of ELHS activation can be influenced by the behavioral and other flexible consequences of glucocorticoid stimulation. A key example of this is the common but contradictory effects of glucocorticoid action on locomotor activity (Word et al., 2022). The role of glucocorticoids in the “take it or leave it” decision in response to perturbations has long been cited (e.g., Wingfield et al., 1998), but the fact that in some species, individuals, and (most interestingly) circumstances and hormone exposure may lead variably to one or the other reflects the importance of neural integration in determining outcomes (e.g., Angelier and Wingfield, 2013). In this case, the glucocorticoid signal itself is but one of many cues used by the animal to determine an appropriate behavioral outcome (Table 3). Integration may then affect, not only behavioral outcomes, but in some cases may even act via other physiological pathways to influence target tissue sensitivity, altering or even reshaping the physiology of the ELHS itself.
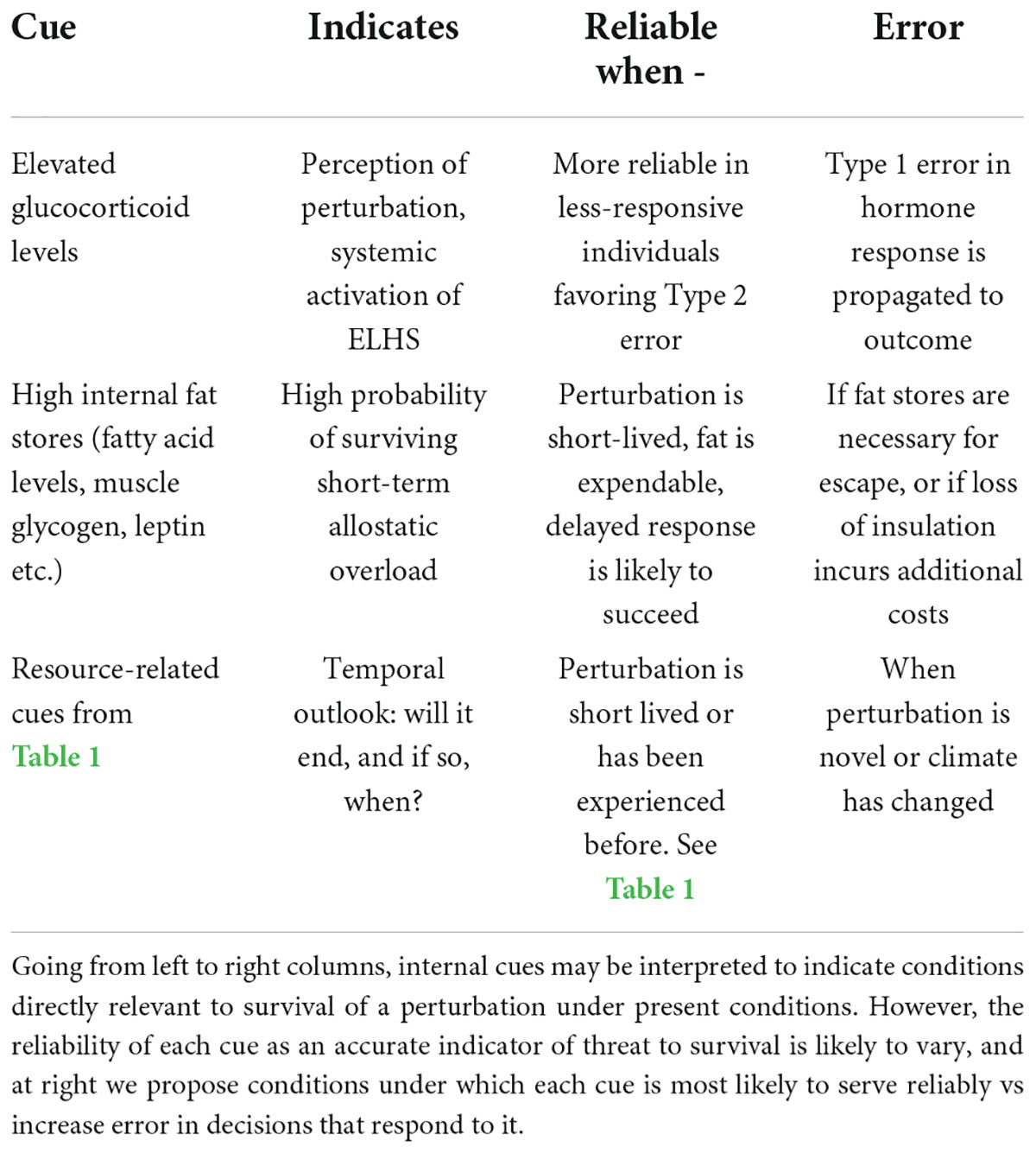
Table 3. Internal cues that may be integrated in a decision to respond via behavioral and other variable responses to elevated glucocorticoid levels.
Future directions: Informing the model
In creating a model that can be informed by known variability, it may be useful to summarize the existing features of the model as they might be perceived and calculated by an animal. Figures 7 and 8 organize the relevant cues from Table 2 into an equation consistent with the existing allostasis model. Based on the specifications of the model, allostatic overload Type I, glucocorticoid excess, and ELHS activation all occur when the animals’ integrated perception yields a perceived PRP ≤ 0.
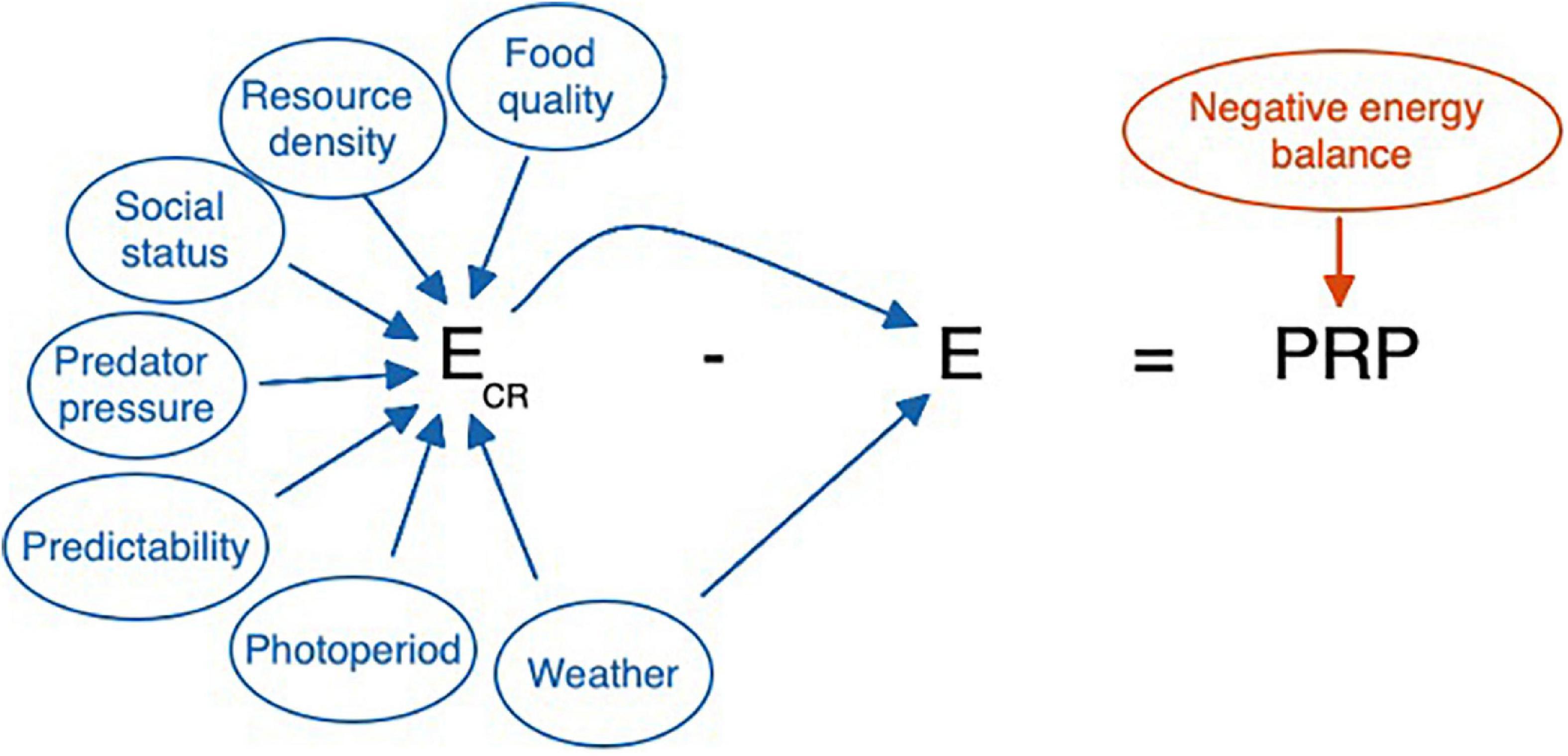
Figure 7. Cues that may be used by a highly responsive individual, such as a non-reproductive animal, in determining whether a perturbation merits activation of the HPA axis. Ecr, cumulative resource availability, may be higher or lower for an individual based on the status of any of the parameters used as cues. Ecr is itself a cue for allostatic load, E, because reduced resource availability often increases the workload necessary to meet energetic requirements. Weather can both influence food availability (e.g., covering food, making insects or predators less active) and offer predictive information about allostatic load (if it’s cold, it is going to be an expensive day). In the absence of predictive cues, prolonged negative energy balance will provide the direct and corrective cue indicating that a false negative error has occurred, and the perturbation resistance potential (PRP) is less than zero. However, for some individuals a response at this point may be too late. Note that here E = Ee + Ei. Variation in Ee and Ei can directly affect E and thus PRP. When Eo occurs as well, this would increase E further and reduce PRP. Ee, Ei, and Eo can all vary independently affecting E, and thus PRP, in different ways. Here we focus on E = Ee + Ei.
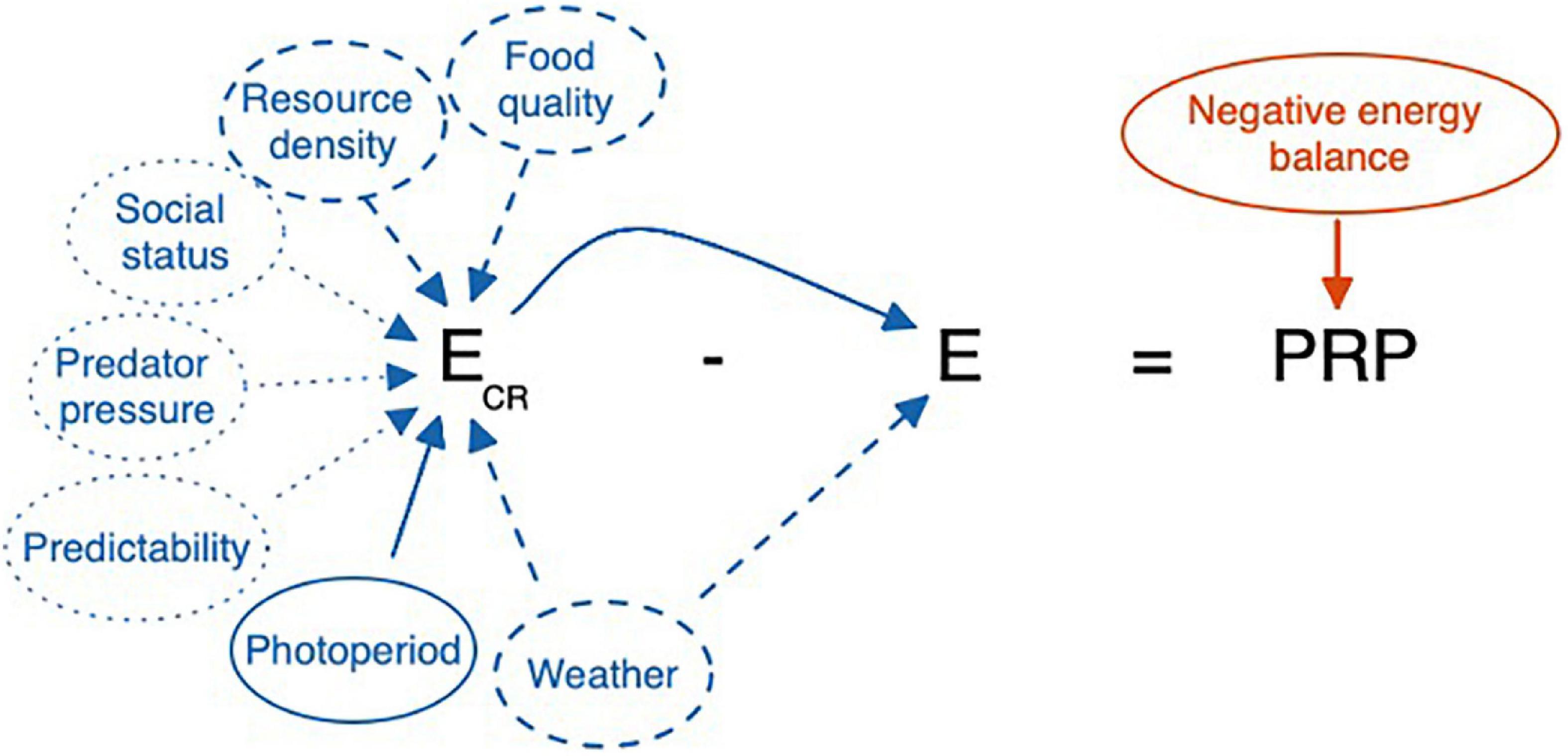
Figure 8. Cues that may be used by a highly unresponsive individual, such as a short-season breeder in a highly unpredictable environment. Photoperiod being fixed in a natural environment, the animal may utilize extreme conditions of resource availability or weather as cues. However, in favoring the risk of a false negative error, some animals may rely on prolonged energy imbalance to trigger the emergency life history stage. Here photoperiod is a major reliable cue. Food quality and resource density are less reliable whereas social status, predator pressure, and overall predictability are least reliable as indicators of Ecr. Note that here E = Ee + Ei. Variation in Ee and Ei can directly affect E and thus PRP. When Eo occurs as well, this would increase E further and reduce PRP. Ee, Ei, and Eo can all vary independently affecting E, and thus PRP, in different ways. Here we focus on E = Ee + Ei.
If perception of PRP is the fundamental driver of glucocorticoid elevation with respect to allostatic load, then this leads directly to revision of several points in the original model. The core points – revised, annotated, and re-prioritized – are presented here:
1. The PRP describes the gap between allostatic load and resource availability (Ecr – Eload). This quantity, or perception thereof, is proposed to correlate with glucocorticoid levels – not allostatic load per se.
2. Allostatic load is an energetic quantity (Eload). The importance of this term is reduced when emphasis is changed to PRP because it is not always a primary determinant of PRP.
3. Resource availability is designated Eg or, cumulatively, Ecr. Ecr improves alignment with known responses to negative energy balance and accounts for individual variation in body reserves and resource access. Resource availability assumes more importance as perception of this quantity is critical to estimating PRP.
4. The ELHS is induced by elevated secretion of glucocorticoid hormones.
5. Allostatic overload Type 1 occurs when an animal is in negative energy balance. Prolonged negative energy balance is the ultimate, undisputed signal of a threat to PRP and should always be responded to.
6. Allostatic overload Type 2 occurs when allostatic load is chronically high and an animal is not in negative energy balance. If an animal relies on cues that indicate a threat to PRP and responds with anticipatory elevation in glucocorticoid levels, this could lead to ELHS activation and/or homeostatic overload as described in the Reactive Scope model and increases the probability that future cues/perturbations will do so. This response is adaptive only when the cues in use accurately predict onset of critically low PRP.
The distinction between Type I and Type II overload, then, becomes one of responding directly to physiological starvation (actual PRP = 0) versus an alternative predictor of impending negative energy balance that may or may not accurately reflect low PRP. Where cues accurately predict threats to energy balance, Type II overload could be functionally indistinguishable from Type I overload, with the exception that ELHS activation might precede the onset of negative energy balance. Only where responses are consistently erroneous – a false positive error – is Type II overload likely to occur because if responses are both frequent and accurate then Type I overload occurs, and if they are infrequent then no overload should result. This is consistent with the observation that Type II overload is most likely to occur in humans and captive animals, both of which live in an environment where resource availability is disconnected from virtually all historically relevant cues. It also suggests that as climate change causes shifts in habitats across the globe, highly responsive individuals and groups may become more vulnerable to Type II allostatic overload.
Future directions should also address more the preparative actions of glucocorticoids in expression of the EHLS when environmental conditions continue to worsen (Sapolsky et al., 2000; Vera et al., 2017). To what extent do these preparative actions allow activation of the EHLS while some components of Ecr are available to fuel the transition to coping and abandonment of the current life history stage before complete exhaustion. Recent evidence suggests that a heat-shock-related protein, FKBP5 interacts with GR to reduce its activity and could also act as an ultra-short loop feedback signal. This could enhance the flexibility of the HPA axis in relation to environmental change (Zimmer et al., 2020). Furthermore, Wada (2019) points out that more integration is needed of glucocorticoid effects and potential damage and repair that will contribute to fitness. These are essential components of coping with environmental change. Relationships of glucocorticoid responses to environment, their repeatability and fitness is just beginning to be explored (Taff et al., 2018; Schoenle et al., 2021).
Author contributions
KW conducted the research and drafted the manuscript. JW was involved in the design of the investigations and provided funding. All authors contributed to the writing and revising the manuscript.
Funding
The investigations presented here were funded by the University of California, Davis Endowment in Physiology and NSF grant numbers ARC – 0909133, BIO-1066241, and IOS-1558049 to JW.
Conflict of interest
The authors declare that the research was conducted in the absence of any commercial or financial relationships that could be construed as a potential conflict of interest.
Publisher’s note
All claims expressed in this article are solely those of the authors and do not necessarily represent those of their affiliated organizations, or those of the publisher, the editors and the reviewers. Any product that may be evaluated in this article, or claim that may be made by its manufacturer, is not guaranteed or endorsed by the publisher.
References
Angelier, F., and Wingfield, J. C. (2013). Importance of the glucocorticoid stress response in a changing world: Theory, hypotheses and perspectives. Gen. Comp. Endocrinol. 190, 118–128. doi: 10.1016/j.ygcen.2013.05.022
Baker, M. E., Funder, J. W., and Kattoula, S. R. (2013). Evolution of hormone selectivity in glucocorticoid and mineralocorticoid receptors. J. Steroid Biochem. Mol. Biol. 137, 57–70. doi: 10.1016/j.jsbmb.2013.07.009
Baker, M. E., Nelson, D. R., and Studer, R. A. (2015). Origin of the response to adrenal and sex steroids: Roles of promiscuity and co-evolution of enzymes and steroid receptors. J. Steroid Biochem. Mol. Biol. 151, 12–24. doi: 10.1016/j.jsbmb.2014.10.020
Barnard, C. J. (1980). Equilibrium flock size and factors affecting arrival and departure in feeding house sparrows. Anim. Behav. 28, 503–511. doi: 10.1016/S0003-3472(80)80058-3
Bateman, P. W., and Fleming, P. A. (2013). Switching to Plan B: Changes in the escape tactics of two grasshopper species (Acrididae: Orthoptera) in response to repeated predatory approaches. Behav. Ecol. Sociobiol. 68, 457–465. doi: 10.1007/s00265-013-1660-0
Bateman, P. W., and Fleming, P. A. (2015). Escape behaviour in shore crabs: Constraints of body size and available shelter. J. Zool. 297, 265–269. doi: 10.1111/jzo.12276
Blas, J., and Fairhurst, G. D. (2022). “Stress ecophysiology,” in Sturkie’s Avian Physiology, 7th Edn, eds C. G. Scanes and S. Dridi (London: Academic Press), 1029–1078.
Blumstein, D. T. (2016). Habituation and sensitization: New thoughts about old ideas. Anim. Behav. 120, 255–262. doi: 10.1016/j.anbehav.2016.05.012
Bókony, V., Lendvai, ÁZ., Liker, A., Angelier, F., Wingfield, J. C., Chastel, O., et al. (2009). Stress response and the value of reproduction: Are birds prudent parents? Am. Nat. 173, 589–598. doi: 10.1086/597610
Breuner, C. W., and Orchinik, M. (2009). Pharmacological characterization of intracellular, membrane, and plasma binding sites for corticosterone in house sparrows. Gen. Comp. Endocrinol. 163, 214–224. doi: 10.1016/j.ygcen.2009.01.027
Busch, D. S., Sperry, T. S., Peterson, E., Do, C.-T., Wingfield, J. C., and Boyd, E. H. (2008a). Impacts of frequent, acute pulses of corticosterone on condition and behavior of Gambel’s white-crowned sparrow (Zonotrichia leucophrys gambelii). Gen. Comp. Endocrinol. 158, 224–233. doi: 10.1016/j.ygcen.2008.07.010
Busch, D. S., Sperry, T. S., Wingfield, J. C., and Boyd, E. H. (2008b). Effects of repeated, short-term, corticosterone administration on the hypothalamo-pituitary-adrenal axis of the white-crowned sparrow (Zonotrichia leucophrys gambelii). Gen. Comp. Endocrinol. 158, 211–223. doi: 10.1016/j.ygcen.2008.06.004
Bytheway, J. P., Carthey, A. J. R., and Banks, P. B. (2013). Risk vs. reward: How predators and prey respond to aging olfactory cues. Behav. Ecol. Sociobiol. 67, 715–725. doi: 10.1007/s00265-013-1494-9
Carroll, S. M., Bridgham, J. T., and Thornton, J. W. (2008). Evolution of Hormone Signaling in Elasmobranchs by Exploitation of Promiscuous Receptors. Mol. Biol. Evol. 25, 2643–2652. doi: 10.1093/molbev/msn204
Carroll, S. M., Ortlund, E. A., and Thornton, J. W. (2011). Mechanisms for the Evolution of a Derived Function in the Ancestral Glucocorticoid Receptor. PLoS Genet. 7:e1002117. doi: 10.1371/journal.pgen.1002117
Cherel, Y., Robin, J. P., Walch, O., Karmann, H., Netchitailo, P., and Le Maho, Y. L. (1988). Fasting in king penguin. I. Hormonal and metabolic changes during breeding. Am. J. Physiol. Regul. Integr. Comp. Physiol. 254:R170–R177.
Close, D. A., Yun, S.-S., McCormick, S. D., Wildbill, A. J., and Li, W. (2010). 11-Deoxycortisol is a corticosteroid hormone in the lamprey. Proc. Natl. Acad. Sci. U.S.A. 107, 13942–13947. doi: 10.1073/pnas.0914026107
Costantini, D., Marasco, V., and Møller, A. P. (2011). A meta-analysis of glucocorticoids as modulators of oxidative stress in vertebrates. J. Comp. Physiol. B 181, 447–456. doi: 10.1007/s00360-011-0566-2
Crino, O. L., Driscoll, S. C., and Breuner, C. W. (2014). Corticosterone exposure during development has sustained but not lifelong effects on body size and total and free corticosterone responses in the zebra finch. Gen. Comp. Endocrinol. 196, 123–129. doi: 10.1016/j.ygcen.2013.10.006
Cyr, N. E., and Romero, L. M. (2009). Identifying hormonal habituation in field studies of stress. Gen. Comp. Endocrinol. 161, 295–303. doi: 10.1016/j.ygcen.2009.02.001
Cyr, N. E., Wikelski, M., and Romero, L. M. (2008). Increased energy expenditure but decreased stress responsiveness during molt. Physiol. Biochem. Zool. 81, 452–462. doi: 10.1086/589547
Dallman, M. F. (1993). Stress update: Adaptation of the hypothalamic-pituitary-adrenal axis to chronic stress. Trends Endocrinol. Metab. 4, 62–69. doi: 10.1016/S1043-2760(05)80017-7
Goodman, M. N., Larsen, P. R., Kaplan, M. M., Aoki, T. T., Young, V. R., and Ruderman, N. B. (1980). Starvation in the rat. II. Effect of age and obesity on protein sparing and fuel metabolism. Am. J. Physiol. 239:E277–E286. doi: 10.1152/ajpendo.1980.239.4.E277
Goymann, W., and Wingfield, J. C. (2004). Allostatic load, social status, and stress hormones – the costs of social status matter. Anim. Behav. 67, 591–602.
Gwinner, E. (1996). Circannual clocks in avian reproduction and migration. Ibis 138, 47–63. doi: 10.1111/j.1474-919X.1996.tb04312.x
Hatchwell, B. J., Shar, S. P., Simeon, M., and McGowan, A. (2009). Factors influencing overnight loss of body mass in the communal roosts of a social bird. Funct. Ecol. 23, 367–372.
Hau, M., Perfito, N., and Moore, I. T. (2008). Timing of breeding in tropical birds: Mechanisms and evolutionary implications. Ornithol. Neotropical 19, 39–59.
Heatwole, H. (1968). Relationship of escape behavior and camouflage in Anoline lizards. Copeia 1968, 109–113. doi: 10.2307/1441557
Jimeno, B., Hau, M., and Verhulst, S. (2018). Corticosterone levels reflect variation in metabolic rate, independent of “stress”. Sci. Rep. 8:13020. doi: 10.1038/s41598-018-31258-z
Johnson, D. D. P., Blumstein, D. T., Fowler, J. H., and Haselton, M. G. (2013). The evolution of error: Error management, cognitive constraints, and adaptive decision-making biases. Trends Ecol. Evol. 28, 474–481. doi: 10.1016/j.tree.2013.05.014
Juster, R.-P., and McEwen, B. S. (2015). Sleep and chronic stress: New directions for allostatic load research. Sleep Med. 16, 7–8. doi: 10.1016/j.sleep.2014.07.029
Kats, L. B., and Dill, L. M. (1998). The scent of death: Chemosensory assessment of predation risk by prey animals. Écoscience 5, 361–394. doi: 10.1080/11956860.1998.11682468
Koob, G. F., and Le Moal, M. (2001). Drug addiction, dysregulation of reward, and allostasis. Neuropsychopharmacology 24, 97–129. doi: 10.1016/S0893-133X(00)00195-0
Korte, S. M., Koolhaas, J. M., Wingfield, J. C., and McEwen, B. S. (2005). The Darwinian concept of stress: Benefits of allostasis and costs of allostatic load and the trade-offs in health and disease. Neurosci. Biobehav. Rev. 29, 3–38. doi: 10.1016/j.neubiorev.2004.08.009
Krause, J. S., Pérez, J. H., Chmura, H. E., Meddle, S. L., Hunt, K. E., Gough, L., et al. (2018). Weathering the storm: Do arctic blizzards cause repeatable changes in stress physiology and body condition in breeding songbirds? Gen. Comp. Endocrinol. 267, 183–192. doi: 10.1016/j.ygcen.2018.07.004
Landys, M. M., Ramenofsky, M., and Wingfield, J. C. (2006). Actions of glucocorticoids at a seasonal baseline as compared to stress-related levels in the regulation of periodic life processes. Gen. Comp. Endocrinol. 148, 132–149. doi: 10.1016/j.ygcen.2006.02.013
Lattin, C. R., Reed, J. M., DesRochers, D. W., and Romero, L. M. (2011). Elevated corticosterone in feathers correlates with corticosterone-induced decreased feather quality: A validation study. J. Avian Biol. 42, 247–252. doi: 10.1111/j.1600-048X.2010.05310.x
Lattin, C., and Romero, L. (2014). Chronic stress alters concentrations of corticosterone receptors in a tissue-specific manner in wild house sparrows (Passer domesticus). J. Exp. Biol. 217, 2601–2608. doi: 10.1242/jeb.103788
McEwen, B. S. (1998). Stress, adaptation, and disease: Allostasis and allostatic load. Ann. N. Y. Acad. Sci. 840, 33–44. doi: 10.1111/j.1749-6632.1998.tb09546.x
McEwen, B. S. (2004). “Protective and Damaging Effects of the Mediators of Stress and Adaptation: Allostasis and Allostatic Load,” in Allostasis, Homeostasis, and the Costs of Physiological Adaptation, ed. J. Shulkin (New York, NY: Cambridge University Press), 65–98.
McEwen, B. S., and Stellar, E. (1993). Stress and the individual: Mechanisms leading to disease. Arch. Intern. Med. 153, 2093–2101. doi: 10.1001/archinte.1993.00410180039004
McEwen, B. S., and Wingfield, J. C. (2003). The concept of allostasis in biology and biomedicine. Horm. Behav. 43, 2–15. doi: 10.1016/S0018-506X(02)00024-7
McEwen, B. S., and Wingfield, J. C. (2010). What is in a name? Integrating homeostasis, allostasis and stress. Horm. Behav. 57, 105–111. doi: 10.1016/j.yhbeh.2009.09.011
McMaster, A., Jangani, M., Sommer, P., Han, N., Brass, A., Beesley, S., et al. (2011). Ultradian cortisol pulsatility encodes a distinct, biologically important signal. PLoS One 6:e15766. doi: 10.1371/journal.pone.0015766
Møller, A. P. (2014). Life history, predation and flight initiation distance in a migratory bird. J. Evol. Biol. 27, 1105–1113. doi: 10.1111/jeb.12399
Møller, A. P., Tryjanowski, P., Díaz, M., Kwieciński, Z., Indykiewicz, P., Mitrus, C., et al. (2015). Urban habitats and feeders both contribute to flight initiation distance reduction in birds. Behav. Ecol. 26, 861–865. doi: 10.1093/beheco/arv024
Monaghan, P., and Spencer, K. A. (2014). Stress and life history. Curr. Biol. 24:R408–R412. doi: 10.1016/j.cub.2014.04.017
Morton, M. L. (1967). Diurnal feeding patterns in white-crowned sparrows, Zonotrichia leucophrys gambelii. Condor 69, 491–512. doi: 10.2307/1366149
Nesse, R. M. (2005). Natural selection and the regulation of defenses: A signal detection analysis of the smoke detector principle. Evol. Hum. Behav. 26, 88–105. doi: 10.1016/j.evolhumbehav.2004.08.002
Ortlund, E. A., Bridgham, J. T., Redinbo, M. R., and Thornton, J. W. (2007). Crystal Structure of an Ancient Protein: Evolution by Conformational Epistasis. Science 317, 1544–1548. doi: 10.1126/science.1142819
Pearson, O. P. (1954). The daily energy requirements of a wild Anna hummingbird. Condor 56, 317–322. doi: 10.2307/1365017
Picard, M., Juster, R.-P., and McEwen, B. S. (2014). Mitochondrial allostatic load puts the “gluc” back in glucocorticoids. Nat. Rev. Endocrinol. 10, 303–310. doi: 10.1038/nrendo.2014.22
Prager, E. M., Brielmaier, J., Bergstrom, H. C., McGuire, J., and Johnson, L. R. (2010). Localization of mineralocorticoid receptors at mammalian synapses. PLoS One 5:e14344. doi: 10.1371/journal.pone.0014344
Ramasamy, R. A., Allan, B. J. M., and McCormick, M. I. (2015). Plasticity of escape responses: Prior predator experience enhances escape performance in a coral reef fish. PLoS One 10:e0132790. doi: 10.1371/journal.pone.0132790
Romero, L. M., and Gormally, B. M. G. (2019). How truly conserved is the “well conserved” vertebrate stress response? Integ. Comp. Biol. 59, 273–281. doi: 10.1093/icb/icz011
Romero, L. M., and Remage-Healey, L. (2000). Daily and seasonal variation in response to stress in captive starlings (Sturnus vulgaris): Corticosterone. Gen. Comp. Endocrinol. 119, 52–59. doi: 10.1006/gcen.2000.7491
Romero, L. M., and Wingfield, J. C. (2016). Tempests, Poxes, Predators, And People: Stress In Wild Animals And How They Cope. New York, NY: Oxford University Press.
Romero, L. M., Dickens, M. J., and Cyr, N. E. (2009). The reactive scope model – A new model integrating homeostasis, allostasis, and stress. Horm. Behav. 55, 375–389. doi: 10.1016/j.yhbeh.2008.12.009
Sapolsky, R. M., Romero, L. M., and Munck, A. U. (2000). How do glucocorticoids influence stress responses? Integrating permissive, suppressive, stimulatory, and preparative actions. Endocr. Rev. 21, 55–89. doi: 10.1210/er.21.1.55
Schoenle, L. A., Zimmer, C., Miller, E. T., and Vitousek, M. N. (2021). Does variation in glucocorticoid concentrations prepdict fitness? A phylogenetic meta-analysis. Gen. Comp. Endcrinol. 300:113611. doi: 10.1016/j.ygcen.2020.113611
Seltmann, M. W., Öst, M., Jaatinen, K., Atkinson, S., Mashburn, K., and Hollmén, T. (2012). Stress responsiveness, age and body condition interactively affect flight initiation distance in breeding female eiders. Anim. Behav. 84, 889–896. doi: 10.1016/j.anbehav.2012.07.012
Sinervo, B., Clobert, J., Miles, D. B., McAdam, A., and Lancaster, L. T. (2008). The role of pleiotropy vs signaller–receiver gene epistasis in life history trade-offs: Dissecting the genomic architecture of organismal design in social systems. Heredity 101, 197–211. doi: 10.1038/hdy.2008.64
Smolka, J., Raderschall, C. A., and Hemmi, J. M. (2013). Flicker is part of a multi-cue response criterion in fiddler crab predator avoidance. J. Exp. Biol. 216, 1219–1224. doi: 10.1242/jeb.076133
Snell-Rood, E., and Snell-Rood, C. (2020). The developmental support hypothesis: Adaptive plasticity in neural development in response to cues of social support. Phil. Trans. R. Soc. B 375:20190491. doi: 10.1098/rstb.2019.0491
Stankowich, T., and Blumstein, D. T. (2005). Fear in animals: A meta-analysis and review of risk assessment. Proc. R. Soc. Lond. B 272, 2627–2634. doi: 10.1098/rspb.2005.3251
Sterling, P. (2004). “Principles of allostasis: optimal design, predictive regulation, pathophysiology and rational therapeutics,” in Allostasis, homeostasis and the costs of adaptation, ed. J. Schulkin (Cambridge: Cambridge Univ. Press), 1–36.
Sterling, P. (2020). What is Health? Allsotasis and the evolution of human design. Cambridge MA: MIT Press.
Sterling, P., and Eyer, J. (1988). “Allostasis: a new paradigm to explain arousal pathology,” in Handbook of Life Stress, Cognition and Health, eds S. Fisher and J. Reason (New York, NY: Wiley Press), 629–649.
Taff, C. C., Schoenle, L. A., and Vitousek, M. N. (2018). The repeatability of glucocorticoids: A review and meta-analysis. Gen. Comp. Endocrinol. 260, 136–145. doi: 10.1016/j.ygcen.2018.01.011
Uchida, K., Suzuki, K., Shimamoto, T., Yanagawa, H., and Koizumi, I. (2016). Seasonal variation of flight initiation distance in Eurasian red squirrels in urban versus rural habitat. J. Zool. 298, 225–231. doi: 10.1111/jzo.12306
Van Donselaar, J. L., Atma, J. L., Kruyf, Z. A., and LaCroix, D. (2018). Urbanization alters fear behavior in blck-capped chickdees. Urban. Ecosyst. 21, 1043–1051. doi: 10.1007/s11252-018-0783-5
Vera, F., Zenuto, R., and Antenucci, C. D. (2017). Expanding the actions of cortisol and corticosterone in wild vertebrtaes: A necessary step to overcome the emerging challenges. Gen. Comp. Endocrinol. 246, 337–353. doi: 10.1016/j.ygcen.2017.01.010
Wada, H. (2019). Damage-fitness model: The missing piece in integrative stress models. Stress 22, 548–562. doi: 10.1080/10253890.2019.1614556
Weaver, I. C. G., Cervoni, N., Champagne, F. A., D’Alessio, A. C., Sharma, S., Seckl, J. R., et al. (2004). Epigenetic programming by maternal behavior. Nat. Neurosci. 7, 847–854. doi: 10.1038/nn1276
Wingfield, J. C. (2004). “Allostatic load and life cycles: Implications for neuroendocrine control mechanisms,” in Allostasis Homeostasis and the Costs of Adaptation, ed. J. Sculkin (Cambridge: Cambridge Univ. Press), 302–342.
Wingfield, J. C. (2005). The concept of allostasis: Coping with a capricious environment. J. Mammal. 86, 248–254.
Wingfield, J. C. (2015). Coping with change: A framework for environmental signals and how neuroendocrine pathways might respond. Front. Neuroendocrinol. 37:89–96. doi: 10.1016/j.ymfe.2014.11.005
Wingfield, J. C., and Romero, L. M. (2001). “Adrenocortical Responses to Stress and Their Modulation in Free-Living Vertebrates,” in Handbook of Physiology, Section 7: The Endocrine System, Volume 4: Coping With The Environment: Neural and Endocrine Mechanisms, ed. B. S. McEwen (Oxford: Oxford University Press), 211–236.
Wingfield, J. C., Kelley, J. P., and Angelier, F. (2011). What are extreme environmental conditions and how do organisms cope with them. Curr. Zool. 57, 363–374.
Wingfield, J. C., Krause, J. S., Perez, J. H., Chmura, H. E., Németh, Z., Word, K. R., et al. (2015). A mechanistic approach to understanding range shifts in a changing world: What makes a pioneer? Gen. Comp. Endocrinol. 222, 44–53. doi: 10.1016/j.ygcen.2015.08.022
Wingfield, J. C., Maney, D. L., Breuner, C. W., Jacobs, J. D., Lynn, S., Ramenofsky, M., et al. (1998). Ecological Bases of Hormone-Behavior Interactions: The “Emergency Life History Stage.”. Am. Zool 38, 191–206.
Wingfield, J. C., Pérez, J. H., Krause, J. S., Word, K. R., González-Gómez, P. L., Lisovski, S., et al. (2017). How birds cope physiologically and behaviorally with extreme climatic events. Phil. Trans. R. Soc. B 372:20160140.
Word, K. R., Austin, S. H., and Wingfield, J. C. (2022). Allostatic load in Gambel’s White Crowned Sparrow, Zonotrichia leucophrys gambelii: Relationships with glucocorticoids. Front. Ecol. Evol. 10:855152. doi: 10.3389/fevo.2022.855152
Ydenberg, R. C., and Dill, L. M. (1986). The economics of fleeing from predators. Adv. Study Behav. 16, 229–249.
Keywords: allostasis, allostatic load, perturbation resistance potential, glucocorticoid, environment
Citation: Word KR, Austin SH and Wingfield JC (2022) Allostasis revisited: A perception, variation, and risk framework. Front. Ecol. Evol. 10:954708. doi: 10.3389/fevo.2022.954708
Received: 27 May 2022; Accepted: 05 September 2022;
Published: 29 September 2022.
Edited by:
Tasmin Rymer, James Cook University, AustraliaReviewed by:
Pedro Dias, Universidad Veracruzana, MexicoDouglas E. Crews, The Ohio State University, United States
Copyright © 2022 Word, Austin and Wingfield. This is an open-access article distributed under the terms of the Creative Commons Attribution License (CC BY). The use, distribution or reproduction in other forums is permitted, provided the original author(s) and the copyright owner(s) are credited and that the original publication in this journal is cited, in accordance with accepted academic practice. No use, distribution or reproduction is permitted which does not comply with these terms.
*Correspondence: John C. Wingfield, amN3aW5nZmllbGRAdWNkYXZpcy5lZHU=