- 1Key Laboratory of Genetics and Germplasm Innovation of Tropical Special Forest Trees and Ornamental Plants, School of Forestry, Hainan University, Haikou, China
- 2Institute of Zoology, Zoological Society of London, London, United Kingdom
- 3Development Research Center of People’s Government of Sanya City, Sanya, China
Due to habitat loss and hunting, the Hainan gibbon (Nomascus hainanus), the world’s rarest primate, was reduced to only two social groups and seven known individuals in 1978. Following the establishment of Bawangling National Natural Reserve (BNNR), gibbon forest habitat increased within this landscape from 56 km2 in 1980 to 300 km2, and the species had increased to five groups and 35 individuals by 2021. It is important to assess whether the large increase in habitat area was responsible for gibbon population increase, or whether gibbon recovery was associated with other factors. Here we use a 21-year longitudinal dataset of Hainan gibbon population change and habitat change, combined with vegetation survey plot data for 2021, to establish an accurate distribution baseline for natural tropical broadleaf forest across the BNNR landscape from 400 to 1300 m (the elevational range of gibbons at BNNR) and within the home range for each of the five Hainan gibbon social groups. We then utilized Landsat time-series images and analysis to compute non-linear causal relationships between forest dynamics and gibbon population growth from 2000 to 2021, both across BNNR and within each gibbon group home range. Metrics of forest dynamics include change in total forest area and forest fragmentation, and metrics of gibbon population dynamics include variation in total number of individuals for the entire population and within each social group, and variation in total number of groups. Our results demonstrate that overall gibbon population growth shows a positive relationship with improved habitat quality, with a one-year time lag of population response. However, changes in numbers of individuals within social groups do not show a similar relationship with improving habitat quality, suggesting that increasing forest cover and connectivity within the BNNR landscape are not direct determinants of Hainan gibbon recovery and that other environmental and/or anthropogenic factors are likely to be involved.
Introduction
Effective conservation of tiny threatened populations requires a robust understanding of the factors that regulate population growth and recovery (Carwardine et al., 2012; Crees et al., 2016). However, collection of large datasets for such populations can be challenging, necessitating long-term data to identify possible relationships between population dynamics and available environmental baselines. One such species in urgent need of evidence-based conservation is the Critically Endangered Hainan gibbon (Nomascus hainanus), the world’s rarest primate. It is restricted to a single population of 35 individuals within a single protected area, Bawangling National Natural Reserve (BNNR; 109°14′47.35″E, 19°5′45.17″N) in Hainan, China (Fellowes et al., 2008; Turvey et al., 2015a; Deng et al., 2017; Chan et al., 2020a; Figure 1A). Various factors have been proposed to limit growth of the Hainan gibbon population at BNNR, including human disturbance, habitat and/or resource limitation, low population density, low genetic diversity, and/or limited availability or suitability of mates (Turvey et al., 2015a). Long-term recovery of the Hainan gibbon is likely to require intensive, carefully planned conservation management, but these different proposed processes require different mitigations, making effective identification of the primary factors limiting gibbon population growth a conservation research priority.
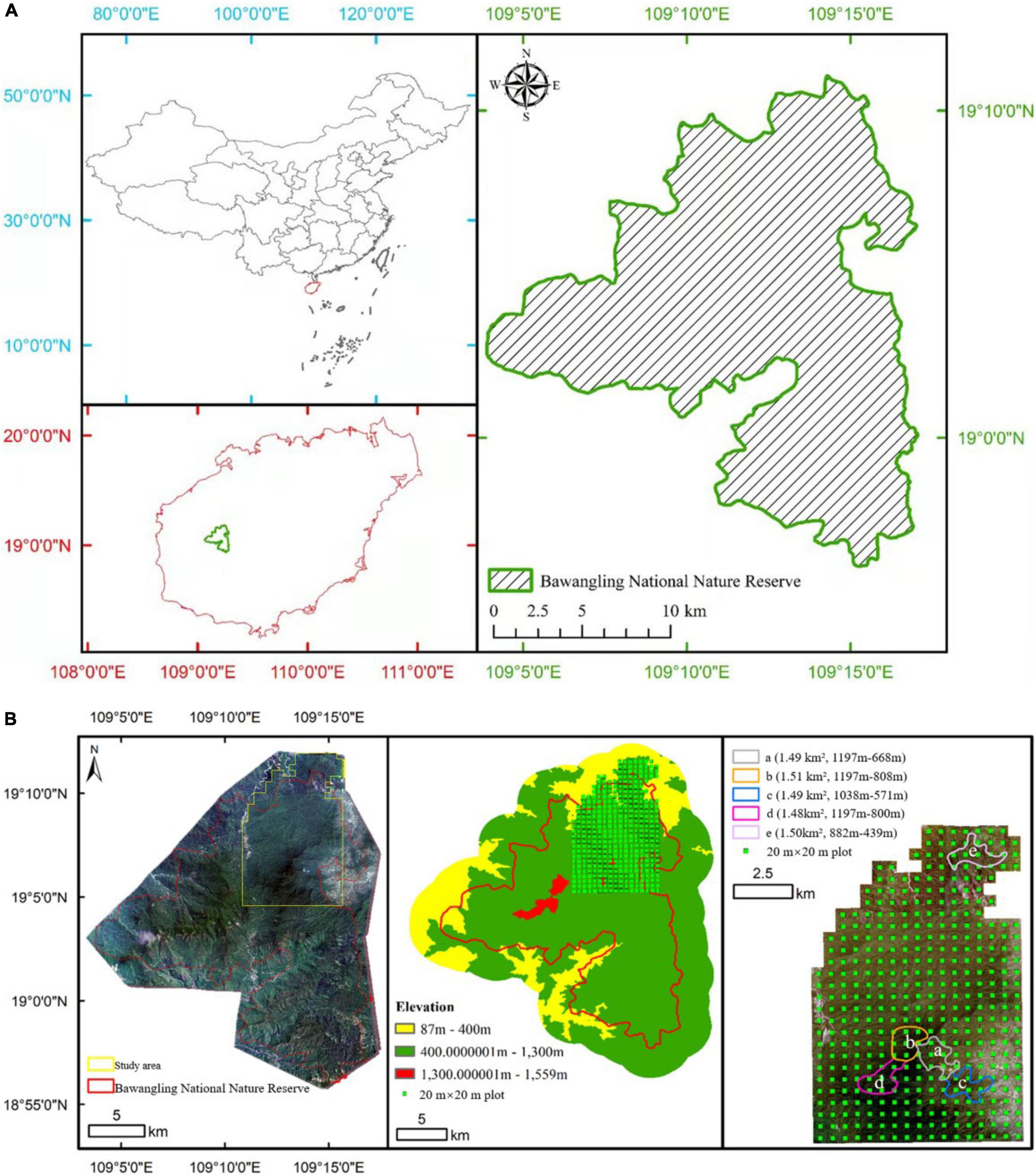
Figure 1. (A) Topographic map of Bawangling National Natural Reserve, Hainan, China; (B) location and elevation of our study area, distribution of 395 20 m × 20 m plots, and home ranges and elevations of Hainan gibbon social groups.
Hainan gibbon population decline during the twentieth century was driven by hunting and habitat loss, resulting from clearance of natural forests and monoculture plantations (Liu et al., 1984; Zhou et al., 2005). BNNR was established in 1980 in a mountainous landscape (elevation 200–1,800 m) that still retained tropical evergreen rainforest, with trees reaching a maximum of 30 m in height (Zhou et al., 2005; Zhang et al., 2020). The reserve was originally 56 km2, but was later expanded to almost 300 km2 in total area (Turvey et al., 2015a). Establishment of the reserve aimed to prevent hunting of gibbons and natural forest clearance, and to support recovery of the last remaining Hainan gibbon population. The gibbon population at BNNR consisted of only two social groups (A and B) and seven known individuals at the time of the first survey in 1978. The population has been monitored regularly since 2000 by the reserve’s management office, and has increased to five social groups and 31 known individuals in 2021: Group C formed in 2010, Group D in 2015, and Group E in 2019 (Liu et al., 1984; Fellowes et al., 2008; Bryant et al., 2016; Deng et al., 2017; Chan et al., 2020a). This multi-decadal increasing trend suggests that population recovery can be attributed directly to effective protection of gibbons and gibbon habitat within BNNR. Continued increase in natural tropical broadleaf forest habitat (increasing total forest area and decreasing fragmentation) is a primary management policy for the recently established Hainan Tropical Rainforest National Park, which has designated the Hainan gibbon as its flagship species (Chinese National Park Administration, 2019). However, the effectiveness of this policy in recovering the Hainan gibbon population has not yet been evaluated using empirical data.
Remote Sensing (RS) and Geographic Information Systems (GIS) are key tools for detecting and evaluating long-term changes in habitat cover and quality for threatened species management (Jeganathan et al., 2004; McShea et al., 2005; Wang et al., 2008; Zhang et al., 2010). Hainan gibbons are strictly dependent upon natural tropical broadleaf forest habitat (primary or secondary forest, not plantation) from 400 to 1,300 m in BNNR (Bryant et al., 2017; Fan, 2017), so it is necessary to establish a baseline of the changing distribution and quality of this habitat type over time across the BNNR landscape and within the home range of each of the five Hainan gibbon groups. It is also important to recognize that species may not respond instantaneously to environmental change, especially if they have slow life histories, and thus time lags might be expected in population responses to changing habitat conditions. Hainan gibbons require 1 year to birth one infant (Liu et al., 1984; Zhou et al., 2008; Deng et al., 2017), and thus a time lag of this magnitude might be expected in gibbon population response to improving habitat conditions. As such, time-series analyses that can find the most likely time lags in causality between variables (e.g., PCMCI algorithm; Runge et al., 2019) are a suitable methodological choice to investigate whether long-time variation in habitat change is a key determinant in Hainan gibbon population dynamics.
In this study, we first determine the distribution of natural forest habitat (primary and secondary forest) across the BNNR landscape from 400 to 1,300 m and within the home range of all five Hainan gibbon groups. We then use Landsat time-series images to determine natural forest dynamics from 2000 to 2021 across BNNR (400–1,300 m) and within each gibbon home range, and use PCMCI to quantify the relationship between natural forest dynamics and gibbon population dynamics across this 21-year period. In addition, we investigate the relationship between species richness (number of species) of known gibbon food plants and elevation, to provide further insights into environmental factors associated with gibbon dispersal and population growth. Our study provides the first assessment of whether forest dynamics have constituted a key determinant of Hainan gibbon population dynamics during the past two decades, thus identifying how landscape management at BNNR can support gibbon conservation in the future.
Materials and Methods
Field-Based Gibbon Home Range and Habitat Data
Hainan gibbon social groups have home ranges with little or no spatial overlap (Bryant et al., 2017; Deng et al., 2017), and have remained in approximately the same locations within the BNNR landscape since 1990 (BNNR Management Office, personal communication, 2021). For the purposes of comparative analysis, we investigated spatial home range locations for each group utilizing unpublished gibbon distribution data provided by the BNNR Management Office (Figure 1B).
We investigated the most accurate boundary for natural (primary and secondary forest) and non-natural forest (plantation) across the BNNR landscape from 400 to 1,300 m, by collecting vegetation and land-use data using 395 20 m × 20 m plots distributed across this elevational range over the BNNR landscape in 2021 (Figure 1B). The area of this vegetation survey represents 31.6% of the total landscape area of BNNR, and includes some areas outside the existing protected area boundary. We also randomly placed another 20 20 m × 20 m plots inside the home range of each of the five Hainan gibbon social groups in 2021, with plots spaced ≥ 50 m apart, to investigate species richness of gibbon food plants. Species were identified as potential gibbon food plants based upon previous published data and unpublished monitoring data (Supplementary Table 1).
Remote Sensing Forest Cover Data
The global distribution of all Hainan gibbon social groups in BNNR is contained within Landsat world reference system tile 124/47 (row/column number). We selected annual Landsat images (30 m resolution) for this region for the period 2000–2021 from USGS,1 selecting images closest to the peak of forest cover (defined as June–September each year) with little to no cloud cover. We performed radiometric calibration and atmospheric correction for each image (Young et al., 2017).
We used specified accurate boundaries for natural and non-natural forest across the BNNR landscape from 400 to 1,300 m, and used the support vector machine method of supervised classification to classify these two land cover types in each image (Phiri and Morgenroth, 2017). Classification requirements were met if separation test values of ROIs (Regions of Interest, selected based on field survey data and representing both land cover types) in each image were greater than 1.9. We manually corrected areas of obvious misclassification based upon field observations. We calculated a confusion matrix by combining classification results and the results of our vegetation survey, with overall classification accuracy for each year greater than 99%. The total area of natural forest across BNNR was then calculated by using the image classification results (number of pixels of each land cover type multiplied by the area of one pixel).
We used FRAGSTATS, a widely used software package for quantifying landscape structure (Ricketts, 2001; Cakir et al., 2007; McGarigal et al., 2012; Lamine et al., 2018), to calculate forest fragmentation across the entirety of BNNR and within the home range of each gibbon group. Fragmentation was calculated according to the formula F = N/A, where F is level of forest fragmentation, N is number of forest patches, and A is total forest area within an image.
Time-Series Analysis
We used the PCMCI algorithm (Runge et al., 2019) implemented in Python2 to compute non-linear causal relationships between changes in forest dynamics (change in total forest area and/or forest fragmentation) across the entirety of BNNR and within the home range of each of the five gibbon groups and Hainan gibbon population dynamics (variation in total number of individuals for the entire gibbon population and within each social group, and variation in total number of social groups) from 2000 to 2021. PCMCI is a two-stage algorithm that includes a PC algorithm and a momentary conditional independence test (MCI; Sun and Bollt, 2014); it can find the most likely time lag of causality between variables, by first running a conditional selection via the PC process to find links between variables based on different conditional independence tests, and then applying the MCI process to remove false positive controls and generate a test statistic to measure the strength of the causal relationship between variables. Model selection (data not shown) supported the use of a τmax (maximum time lag) value of 2, thus including three possible time lags (0, 1, and 2 years). The PCMCI method cannot be used for datasets where n < 7 (Runge et al., 2019). We therefore only analyzed time-series data using the PCMCI method for groups A, B, C, and D, which have existed for seven or more years across our time-series.
Environmental and Anthropogenic Determinants of Gibbon Distribution
To explore the possible effect of both elevation and human land use activities on gibbon social group distribution and formation, we used a 1.5 m-resolution RS image of the entirety of BNNR from 23 April 2019 obtained from the SPOT-7 satellite sensor. Following pre-processing, we used the maximum likelihood method of supervised classification to analyze land cover in the image (Phiri and Morgenroth, 2017). Using artificial interpretation of the image and field survey data, we divided land cover into four categories: natural forest, plantation, roads and construction, and water. We extracted the highest and lowest elevations occupied by each gibbon group using digital elevation model (DEM) data.
We investigated the relationship between elevation and gibbon food plant species richness for each gibbon social group using a generalized linear model (GLM), with a Poisson error structure because food plant richness is a discrete random variable (count data).
Results
Between 2000 and 2021, the total number of Hainan gibbon social groups and individuals increased from two groups and 10 individuals in 2000, to five groups and 35 individuals in 2021 (Figures 2A,B). Overall, Hainan gibbon habitat (natural tropical broadleaf forest) quality also improved across BNNR during this period, with total area increasing from 71.19 to 93.38 km2, and fragmentation decreasing from 0.02 to 0.004 (Figures 3A,B). Within the range of Group C, total natural forest area decreased from 1.42 to 0.95 km2, and fragmentation increased from 0.0072 to 0.042. Conversely, natural forest area and fragmentation remained largely unchanged and consistently high within the ranges of other groups (Figures 3C,D).
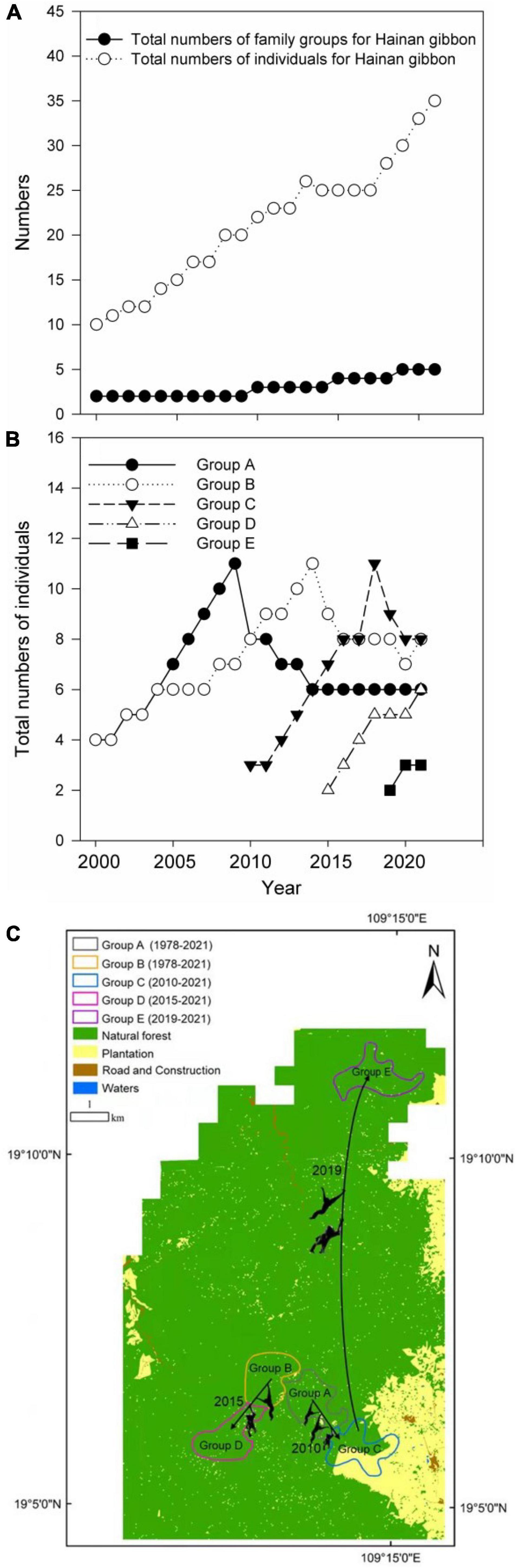
Figure 2. Population change from 2000 to 2021 in: (A) total number of Hainan gibbon individuals and social groups, (B) individuals in each social group, (C) history of formation for groups C, D, and E and boundaries of natural forest and plantation in Bawangling National Natural Reserve from 400 to 1,300 m.
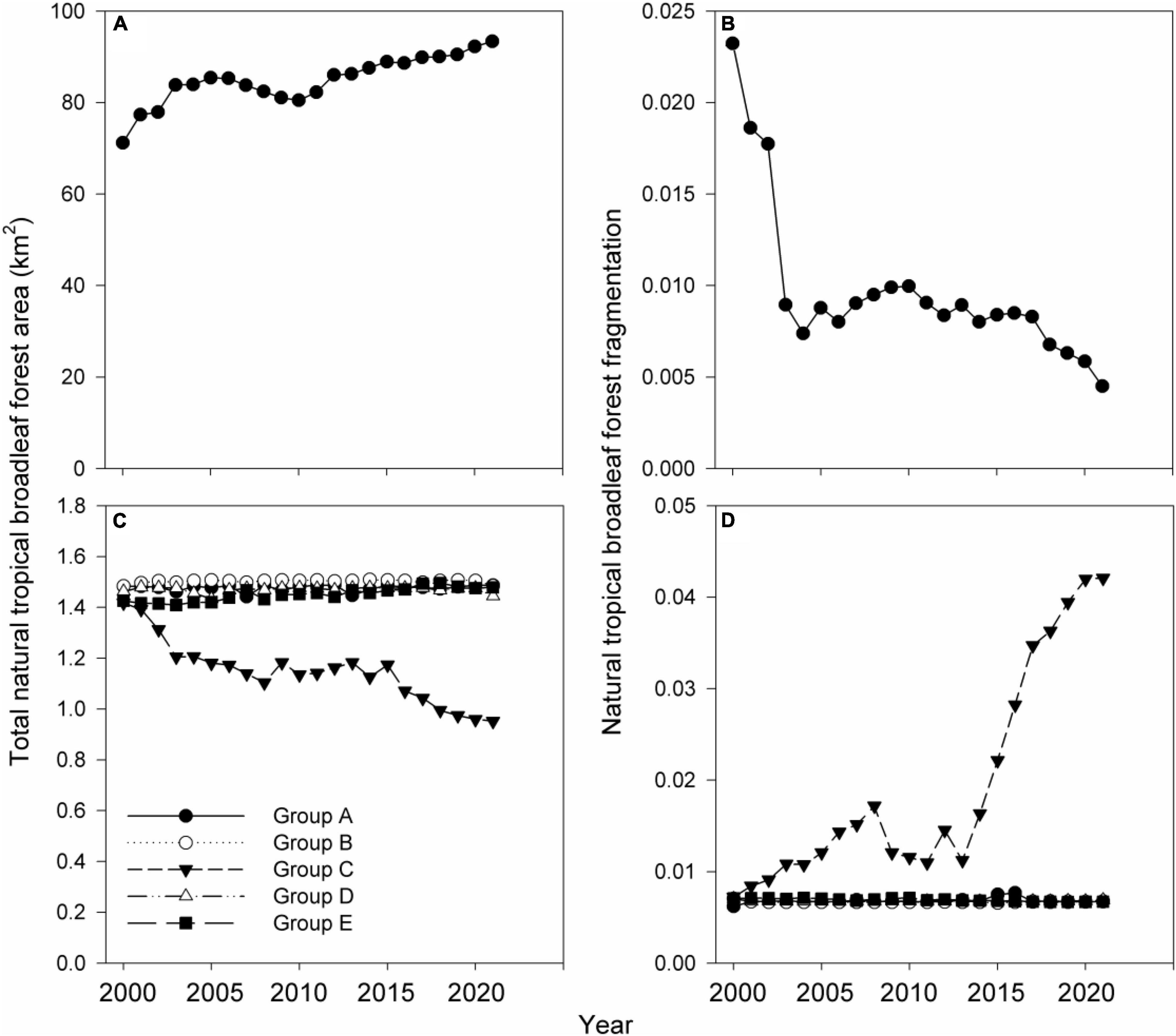
Figure 3. Change from 2000 to 2021 in (A,B) total natural forest area and fragmentation across Bawangling National Natural Reserve, (C,D) natural forest area and fragmentation within Hainan gibbon social group ranges.
PCMCI results show that variation in total number of gibbon individuals from 2000 to 2021 was determined by both natural forest area and fragmentation when the time lag was 1 year, with a positive correlation between number of individuals and natural forest area, but a negative relationship between number of individuals and forest fragmentation (p < 0.05; Figure 4A). Variation in both natural forest area and fragmentation could also determine variation in number of individuals within group C when the time lag was 1 year, with a negative correlation with natural forest area (p < 0.05; Figure 4E), and a positive correlation with fragmentation (p < 0.05; Figure 4E). However, natural forest area and fragmentation were not statistically correlated with variation in number of gibbon groups (p > 0.05; Figure 4B). Similarly neither natural forest area nor fragmentation determined variation in number of individuals within groups A, B, and D (p > 0.05; Figures 4C,D,F).
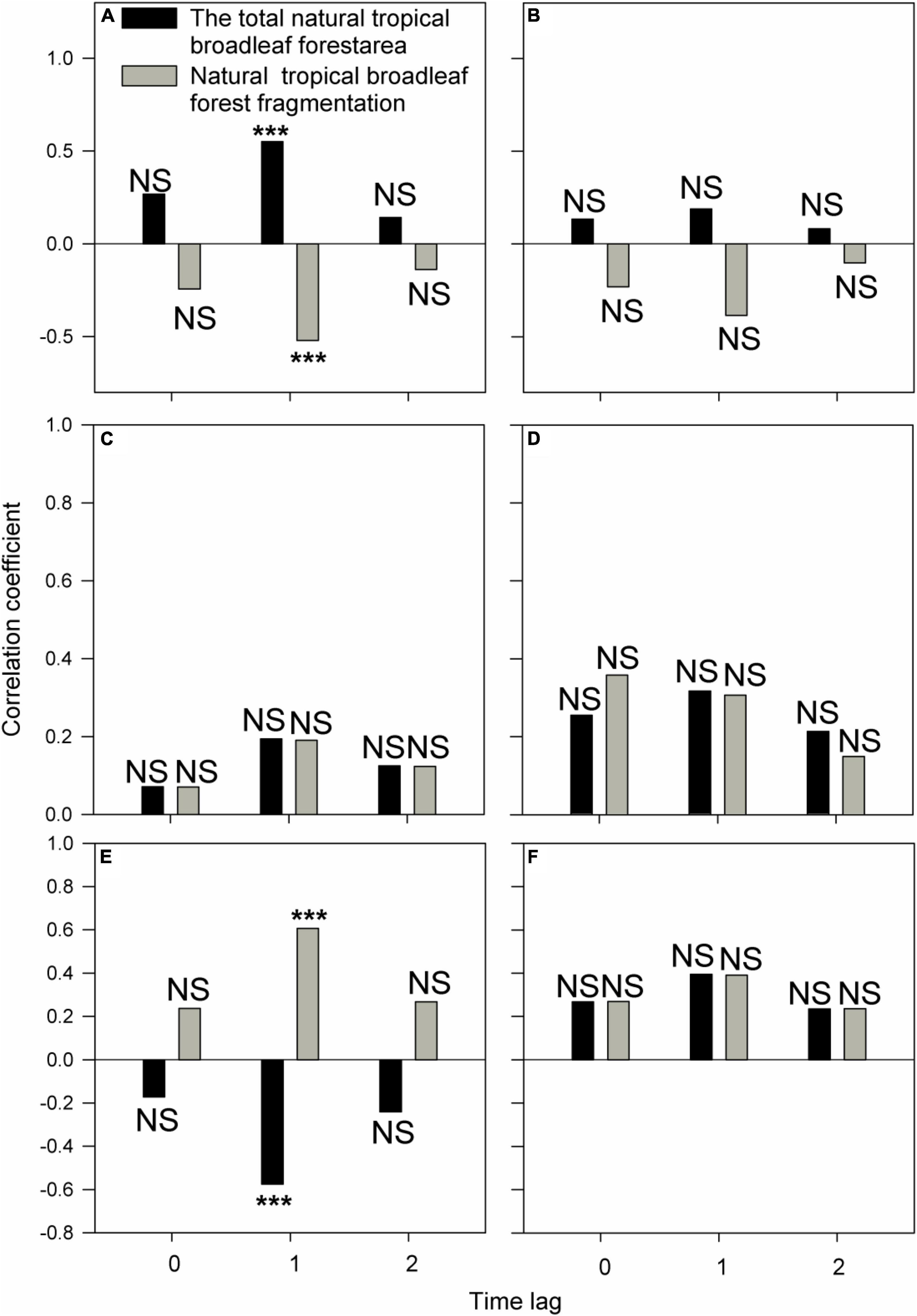
Figure 4. Relationships from 2000 to 2021 between natural forest area and fragmentation, and: (A) number of Hainan gibbon social groups, (B) total number of Hainan gibbon individuals, and number of individuals in (C) Group A, (D) Group B, (E) Group C, and (F) Group D. *** Indicates p < 0.05, NS (non-significant) indicates p > 0.05, based on PCMCI analysis. Time lag (T) varies between 0 and 2 years.
Overall, 59–115 different known gibbon food plant species were recorded across different gibbon social group ranges. There was a significant negative relationship between mean elevation and food plant species richness across the five gibbon groups (p < 0.05; Figure 5), with Group B occupying the highest elevation (mean = 1002.5 m, range = 808–1197 m) and having the lowest food plant species richness, and Group E occupying the lowest elevation (mean = 660.5 m, range = 439–832 m) and having the highest food plant species richness (Figure 5).
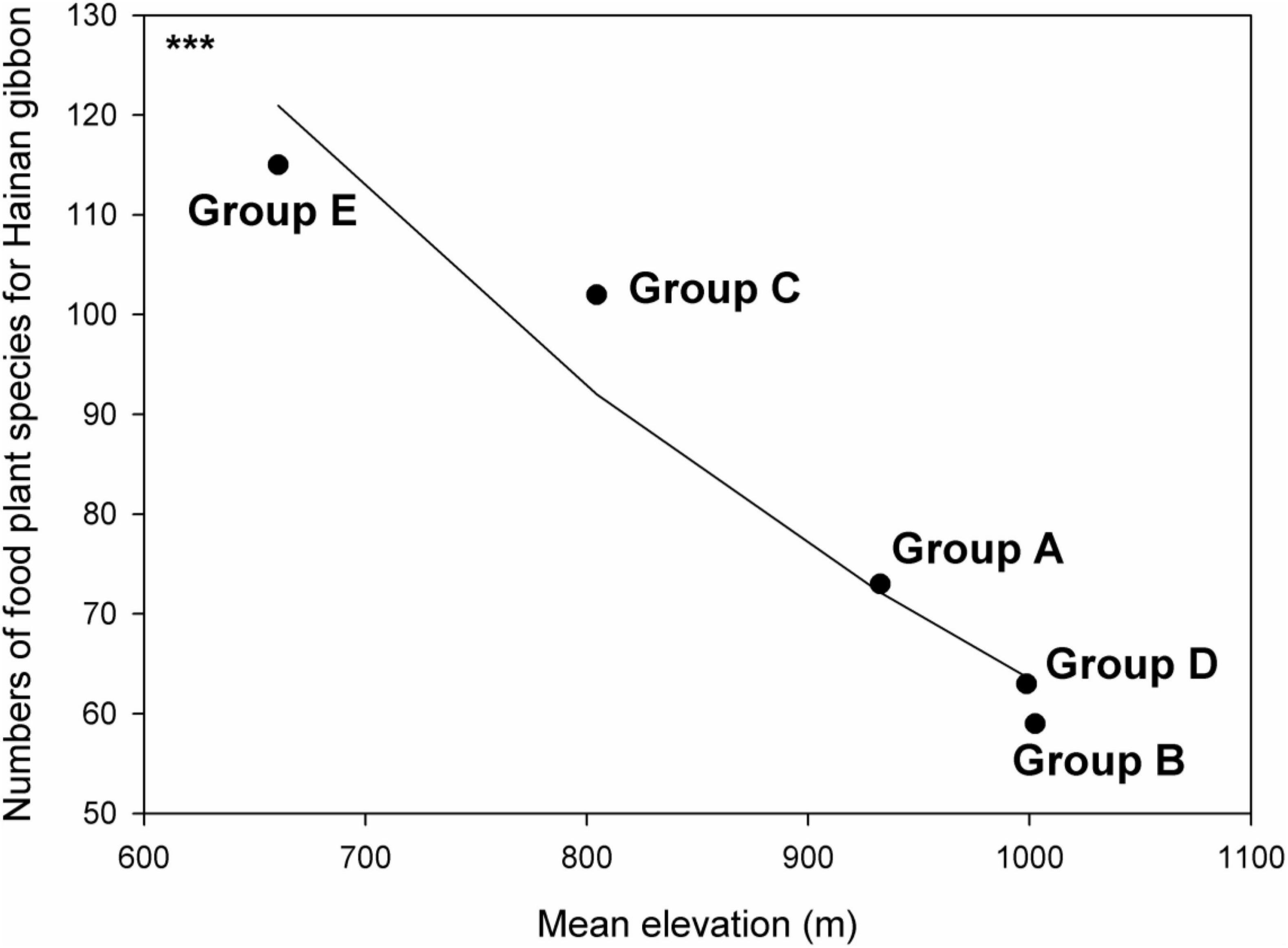
Figure 5. Relationship between Hainan gibbon food plant species richness and mean elevation (m) across five existing Hainan gibbon social groups. *** Indicates p < 0.05 based on generalized linear model with Poisson error. Fitted line generated from a generalized linear model with Poisson error structure.
Discussion
Identifying the factors that have regulated past population dynamics and influenced the rate of recovery are key research goals to inform evidence-based conservation of the world’s rarest ape (Turvey et al., 2015a; Qian et al., 2022). Here, we demonstrate that Hainan gibbon habitat quality (as measured by natural forest total area and fragmentation) has improved across the overall BNNR landscape during the past two decades. However, forest dynamics have shown differing patterns within the home ranges of different gibbon groups, with important implications for the likely influence of forest change in promoting gibbon population recovery.
Establishing an accurate understanding of the distribution and long-term change in suitable habitat for threatened species is crucial for evidence-based conservation management, and requires the use of tools such as Landsat time-series images (Oeser et al., 2020). Here we first provide an accurate assessment of the distribution of Hainan gibbon habitat (natural tropical broadleaf forest) within BNNR and within the home range of each gibbon group (Figure 2C). This baseline not only provides a key tool to understand gibbon population responses and environmental requirements, but can also be used in future studies to investigate ongoing change in Hainan gibbon habitat and population dynamics.
Our PCMCI results demonstrate that increased natural forest area and decreased forest fragmentation are highly correlated with an increase in total number of Hainan gibbon individuals with a 1-year time-lag (Figure 4). This temporal pattern is consistent with Hainan gibbon reproductive biology (Liu et al., 1984; Zhou et al., 2008; Deng et al., 2017), and a time-lag of this scale would thus be expected instead of an instant population response to improving habitat conditions. We recommend that PCMCI should be employed more widely in the future to investigate relationships between long-term dynamics of animal populations and potential determining variables, instead of commonly used linear or non-linear regressions (cf. Mougeot et al., 2003; Koenig and Liebhold, 2016; Hansen et al., 2019).
Conversely, our PCMCI analyses do not demonstrate any positive correlations over time between increased habitat quality (indicated by increased forest total area and decreased fragmentation) and increase in total number of individuals within most gibbon groups (A, B, or D) (Figure 4). One potential reason for this lack of signal is that natural forest area has been consistently high and fragmentation has been consistently low within the areas occupied by these groups across the time-series in our study. However, in contrast to the positive relationship between increased habitat quality and increased population growth at BNNR at the total gibbon population level, our PCMCI results also demonstrate an unexpected relationship between growth of Group C and deterioration of local habitat quality within the estimated area of this group’s home range. From its formation in 2010, Group C increased to a maximum of 11 individuals, whilst local natural forest area decreased by 1.47 times and local forest fragmentation increased by 5.75 times over the same period. This group is situated close to the reserve boundary adjacent to a village, and the local habitat in this region has been progressively impacted by human disturbance (e.g., encroachment and clearance of forest for conversion to agroforestry and agriculture; Turvey et al., 2015a). We do not suggest that decreasing habitat quality has been a local driver of gibbon population growth; instead, we suggest that this statistical pattern represents an incidental correlation rather than direct causation, with Group C exhibiting resilience to local habitat deterioration with no apparent adverse effect on social group growth. Population recovery of other Nomascus gibbon species has also been documented within other degraded forest landscapes (Fan et al., 2013).
These findings indicate that, whereas increased natural forest cover and connectivity is correlated with overall Hainan gibbon population increase over time across BNNR, gibbon social group dynamics are clearly not regulated by changes in these habitat quality metrics. Indeed, it is likely that observed growth of the Hainan gibbon population from 2000 onward is also associated with recovery from recent historical hunting (Fellowes et al., 2008; Deng et al., 2017) rather than solely with improving habitat quality. However, hunting restrictions and forest protection have both been simultaneously enforced by conservation management at BNNR, and their respective contributions to local gibbon recovery are thus difficult to distinguish across the same time-series.
While specific Hainan gibbon habitat requirements are still poorly understood, habitat selection by other Nomascus species is determined by local food availability (spatial distribution of food patches and seasonal variation in fruiting; Fan and Jiang, 2008b,2010; Fan et al., 2009; Ni et al., 2018) as well as local distribution of other key resources such as sleeping sites (Fan and Jiang, 2008a; Fei et al., 2012). We demonstrate a significant negative relationship between elevation and food plant species richness at BNNR (Figure 5). These further findings are thus also important for ongoing landscape management and planning for gibbon conservation, and may also help to explain the high growth rate shown by Group C, which occurs at relatively low elevation at the edge of the reserve, despite the progressive decrease in overall forest quality within its home range. Similarly, although Groups A and B both contained only four individuals in 2000, Group A increased to 11 individuals within 10 years, whereas group B, which occurs at a higher elevation within BNNR, required 15 years to increase to the same group size. These results thus provide further evidence that gibbon social group dynamics at BNNR are regulated by extrinsic or intrinsic factors other than overall changes in natural forest quality. Two of the three most recently formed gibbon groups (groups C and E) occur at lower elevations than the other groups. However, their expansion to lower-elevation habitats might merely reflect historical survival of gibbons on Hainan and elsewhere across southwestern China in more remote high-elevation refugia, rather than being associated with natural habitat characteristics of lower-elevation sites (Turvey et al., 2015b). We therefore encourage additional research to better understand specific habitat parameters important in home range site selection during new social group formation by dispersing gibbon individuals (Turvey et al., 2015a).
Hainan gibbon population recovery thus exhibits a complex relationship with increased habitat cover and connectivity at BNNR, with a positive correlation at the overall population level but no positive correlation at the social group level. In order to develop maximally effective conservation management strategies to support further Hainan gibbon population growth, we encourage further investigation into more specific metrics of natural tropical broadleaf forest quality and structure at BNNR, and their relationship to gibbon distribution and population dynamics. However, gibbons are obligate canopy-dwellers that are unlikely to cross even small canopy gaps (Chivers et al., 2013). Thus, existing patterns of forest degradation and fragmentation across the BNNR landscape are recognized to constitute a major barrier to Hainan gibbon population growth and dispersal (Zhang et al., 2010; Turvey et al., 2015a). It is therefore essential to continue efforts to restore and connect natural tropical broadleaf forest habitats across BNNR and beyond, in order to facilitate further gibbon population growth and expansion. Improving natural forest cover and quality with both short-term (e.g., canopy bridges; Chan et al., 2020b) and longer-term solutions (replanting activities, establishment of ecological corridors to other reserves) will be essential to support a resilient and increasing gibbon population on Hainan into the future. Further assessment of specific Hainan gibbon habitat requirements is a vital next step for evidence-based conservation of this Critically Endangered species, and we recommend targeted ecological research combined with continuing forest protection and reconnection (e.g., strategic reconnection of isolated major forest patches to facilitate gibbon population expansion) to constitute key components of management plans for the newly established Hainan Tropical Rainforest National Park (Liu et al., 2020).
Conclusion
Overall, two important new insights about the influence of habitat quality on Hainan gibbon population dynamics have been revealed by our study. First, due to the species’ reproductive behavior (requiring 1 year to birth one infant), the Hainan gibbon population exhibits a one-year time lag instead of an instantaneous response to improving habitat quality. Second, improving habitat quality following the establishment of BNNR is not the sole direct determinant of the observed recovery of the Hainan gibbon population within this landscape, a finding that has hugely important implications for ongoing landscape management and conservation decision-making for this species. Our study has wider implications for conservation science, by providing guidelines to identify factors that determine population recovery of the world’s rarest primate and other Critically Endangered species.
Data Availability Statement
The raw data supporting the conclusions of this article will be made available by the authors, without undue reservation.
Author Contributions
JC, HZ, and WG designed the research. YZ, ST, JC, HZ, and WG performed the research and wrote the manuscript. YZ, JC, HZ, and WG analyzed the data. All authors contributed to the article and approved the submitted version.
Funding
This research was supported by the specific research fund of the Innovation Platform for Academicians of Hainan Province, a Hainan Academician Innovation Platform Scientific Research Project (YSPTZX202017), the Scientific Research Project of Ecological Restoration of Baopoling (Sanya), a Hainan Province Postgraduate Innovative Research Project (Qhys2021-114), the Arcus Foundation, and Research England.
Conflict of Interest
The authors declare that the research was conducted in the absence of any commercial or financial relationships that could be construed as a potential conflict of interest.
Publisher’s Note
All claims expressed in this article are solely those of the authors and do not necessarily represent those of their affiliated organizations, or those of the publisher, the editors and the reviewers. Any product that may be evaluated in this article, or claim that may be made by its manufacturer, is not guaranteed or endorsed by the publisher.
Supplementary Material
The Supplementary Material for this article can be found online at: https://www.frontiersin.org/articles/10.3389/fevo.2022.953637/full#supplementary-material
Footnotes
References
Bryant, J. V., Brulé, A., Wong, M. H., Hong, X., Zhou, Z., Han, W., et al. (2016). Detection of a new Hainan gibbon (Nomascus hainanus) group using acoustic call playback. Int. J. Primatol. 37, 534–547. doi: 10.1007/s10764-016-9919-8
Bryant, J. V., Zeng, X., Hong, X., Chatterjee, H. J., and Turvey, S. T. (2017). Spatiotemporal requirements of the Hainan gibbon: does home range constrain recovery of the world’s rarest ape? Am. J. Primatol. 79:e22617. doi: 10.1002/ajp.22617
Cakir, G., Sivrikaya, F., and Keleş, S. (2007). Forest cover change and fragmentation using Landsat data in Maçka state forest enterprise in Turkey. Environ. Monit. Assess. 137, 51–66. doi: 10.1007/s10661-007-9728-9
Carwardine, J., O’Connor, T., Legge, S., Mackey, B., Possingham, H. P., and Martin, T. G. (2012). Prioritizing threat management for biodiversity conservation. Conserv. Lett. 5, 196–204. doi: 10.1111/j.1755-263X.2012.00228.x
Chan, B. P. L., Lo, Y. F. P., and Mo, Y. (2020a). New hope for the Hainan gibbon: formation of a new group outside its known range. Oryx 54, 296–296. doi: 10.1017/S0030605320000083
Chan, B. P. L., Lo, Y. F. P., Hong, X. J., Mak, C. F., and Ma, Z. (2020b). First use of artificial canopy bridge by the world’s most critically endangered primate the Hainan gibbon Nomascus hainanus. Sci. Rep. 10:15176. doi: 10.1038/s41598-020-72641-z
Chinese National Park Administration (2019). Using Ecological Corridors to Connect Fragmented Hainan Gibbon Habitats. Available online at: http://www.forestry.gov.cn/main/5497/20200819/170932364708588.html (Accessed June 23, 2022).
Chivers, D. J., Anandam, M. V., Groves, C. P., Molur, S., Rawson, B. M., Richardson, M. C., et al. (2013). “Family Hylobatidae (gibbons),” in Handbook of the Mammals of the World, Vol. 3, eds R. A. Mittermeier, A. B. Rylands, and D. E. Wilson (Barcelona: Lynx Edicions), 754–791.
Crees, J. J., Collins, A. C., Stephenson, P. J., Meredith, H. M., Young, R. P., Howe, C., et al. (2016). A comparative approach to assess drivers of success in mammalian conservation recovery programs. Conserv. Biol. 30, 694–705. doi: 10.1111/cobi.12652
Deng, H., Zhang, M., and Zhou, J. (2017). Recovery of the critically endangered Hainan gibbon Nomascus hainanus. Oryx 51, 161–165. doi: 10.1017/S0030605315000678
Fan, P. (2017). The past, present, and future of gibbons in China. Biol. Conserv. 210, 29–39. doi: 10.1159/000342696
Fan, P., and Jiang, X. (2008b). Effects of food and topography on ranging behavior of black crested gibbon (Nomascus concolor jingdongensis) in Wuliang Mountain, Yunnan, China. Am. J. Primatol. 70, 871–878. doi: 10.1002/ajp.20577
Fan, P., and Jiang, X. (2008a). Sleeping sites, sleeping trees, and sleep-related behaviors of black crested gibbons (Nomascus concolor jingdongensis) at Mt. Wuliang, Central Yunnan, China. Am. J. Primatol. 70, 153–160. doi: 10.1002/ajp.20470
Fan, P., and Jiang, X. (2010). Altitudinal ranging of black-crested gibbons at Mt. Wuliang, Yunnan: effects of food distribution, temperature and human disturbance. Folia Primatol. 81, 1–9. doi: 10.1159/000279465
Fan, P., Jiang, X., and Tian, C. (2009). The critically endangered black crested gibbon Nomascus concolor on Wuliang Mountain, Yunnan, China: the role of forest types in the species’ conservation. Oryx 43, 203–208. doi: 10.1017/S0030605308001907
Fan, P., Ren, G., Wang, W., Scott, M. B., Ma, C., Fei, H., et al. (2013). Habitat evaluation and population viability analysis of the last population of cao vit gibbon (Nomascus nasutus): implications for conservation. Biol. Conserv. 161, 39–47. doi: 10.1016/j.biocon.2013.02.014
Fei, H., Scott, M. B., Zhang, W., Ma, C., Xiang, Z., and Fan, P. (2012). Sleeping tree selection of cao vit gibbon (Nomascus nasutus) living in degraded karst forest in Bangliang, Jingxi, China. Am. J. Primatol. 74, 998–1005. doi: 10.1002/ajp.22049
Fellowes, J. R., Chan, B. P. L., Zhou, J., Chen, S., Yang, S., and Ng, S. C. (2008). Current status of the Hainan gibbon (Nomascus hainanus): progress of population monitoring and other priority actions. Asian Primates J. 1, 2–11.
Hansen, B. B., Gamelon, M., Albon, S. D., Lee, A. M., Stien, A., Irvine, R. J., et al. (2019). More frequent extreme climate events stabilize reindeer population dynamics. Nat. Commun. 10:1616. doi: 10.1038/s41467-019-09332-5
Jeganathan, P., Green, R. E., Norris, K., Vogiatzakis, I. N., Bartsch, A., Wotton, S. R., et al. (2004). Modelling habitat selection and distribution of the critically endangered Jerdon’s courser Rhinoptilus bitorquatus in scrub jungle: an application of a new tracking method. J. Appl. Ecol. 41, 224–237. doi: 10.1111/j.0021-8901.2004.00897.x
Koenig, W. D., and Liebhold, A. M. (2016). Temporally increasing spatial synchrony of North American temperature and bird populations. Nat. Clim. Change 6, 614–617. doi: 10.1038/nclimate2933
Lamine, S., Petropoulos, G. P., Singh, S. K., Szabó, S., Bachari, N. E. I., Srivastava, P. K., et al. (2018). Quantifying land use/land cover spatio-temporal landscape pattern dynamics from Hyperion using SVMs classifier and FRAGSTATS®. Geocarto Int. 33, 862–878. doi: 10.1080/10106049.2017.1307460
Liu, H., Ma, H., Cheyne, S. M., and Turvey, S. T. (2020). Recovery hopes for the world’s rarest primate. Science 368:1074. doi: 10.1126/science.abc1402
Liu, Z., Yu, S., and Yuan, X. (1984). Resource of the Hainan black crested gibbon and its present situation. Wild Animals 6, 1–4.
McGarigal, K., Cushman, S. A., and Ene, E. (2012). FRAGSTATS v4: Spatial Pattern Analysis Program for Categorical and Continuous Maps. Computer Software Program Produced by the authors at the University of Massachusetts, Amherst. Amherst, MA: University of Massachusetts.
McShea, W. J., Koy, K., Clements, T., Johnson, A., Vongkhamheng, C., and Aung, M. (2005). Finding a needle in the haystack: regional analysis of suitable Eld’s deer (Cervus eldi) forest in Southeast Asia. Biol. Conserv. 125, 101–111. doi: 10.1016/j.biocon.2005.03.013
Mougeot, F., Redpath, S. M., Leckie, F., and Hudson, P. J. (2003). The effect of aggressiveness on the population dynamics of a territorial bird. Nature 421, 737–739. doi: 10.1038/nature01395
Ni, Q., Liang, Z., Xie, M., Xu, H., Yao, Y., Zhang, M., et al. (2018). Microhabitat use of the western black-crested gibbon inhabiting an isolated forest fragment in southern Yunnan, China: implications for conservation of an endangered species. Primates 59, 45–54. doi: 10.1007/s10329-017-0634-7
Oeser, J., Heurich, M., Senf, C., Pflugmacher, D., Belotti, E., and Kuemmerle, T. (2020). Habitat metrics based on multi-temporal Landsat imagery for mapping large mammal habitat. Remote Sens. Ecol. Conserv. 6, 52–69. doi: 10.1002/rse2.122
Phiri, D., and Morgenroth, J. (2017). Developments in Landsat land cover classification methods: a review. Remote Sens. 9:967. doi: 10.3390/rs9090967
Qian, J., Mills, M., Ma, H., and Turvey, S. T. (2022). Assessing the effectiveness of public awareness-raising initiatives for the Hainan gibbon Nomascus hainanus. Oryx 56, 249–259. doi: 10.1017/S0030605320000599
Ricketts, T. H. (2001). The matrix matters: effective isolation in fragmented landscapes. Am. Nat. 158, 87–99. doi: 10.1086/320863
Runge, J., Nowack, P., Kretschmer, M., Flaxman, S., and Sejdinovic, D. (2019). Detecting and quantifying causal associations in large nonlinear time series datasets. Sci. Adv. 5:eaau4996. doi: 10.1126/sciadv.aau4996
Sun, J., and Bollt, E. M. (2014). Causation entropy identifies indirect influences, dominance of neighbors and anticipatory couplings. Physica D 267, 49–57. doi: 10.1016/j.physd.2013.07.001
Turvey, S. T., Traylor-Holzer, K., Wong, M. H. G., Bryant, J. V., Zeng, X., Hong, X., et al. (2015a). International Conservation Planning Workshop for the Hainan Gibbon: Final Report. London: Zoological Society of London.
Turvey, S. T., Crees, J. J., and Di Fonzo, M. M. (2015b). Historical data as a baseline for conservation: reconstructing long-term faunal extinction dynamics in late imperial–modern China. Proc. R. Soc. B 282:20151299. doi: 10.1098/rspb.2015.1299
Wang, W., Ren, G., He, Y., and Zhu, J. (2008). Habitat degradation and conservation status assessment of gallinaceous birds in the Trans-Himalayas, China. J. Wildl. Manage. 72, 1335–1341. doi: 10.2193/2007-077
Young, N. E., Anderson, R. S., Chignell, S. M., Vorster, A. G., Lawrence, R., and Evangelista, P. H. (2017). A survival guide to Landsat preprocessing. Ecology 98, 920–932. doi: 10.1002/ecy.1730
Zhang, H., Wang, C., Turvey, S. T., Sun, Z., Tan, Z., Yang, Q., et al. (2020). Thermal infrared imaging from drones can detect individuals and nocturnal behavior of the world’s rarest primate. Glob. Ecol. Conserv. 23:e01101. doi: 10.1016/j.gecco.2020.e01101
Zhang, M., Fellowes, J. R., Jiang, X., Wang, W., Chan, B. P., Ren, G., et al. (2010). Degradation of tropical forest in Hainan, China, 1991–2008: conservation implications for Hainan gibbon (Nomascus hainanus). Biol. Conserv. 143, 1397–1404. doi: 10.1016/j.biocon.2010.03.014
Zhou, J., Wei, F., Li, M., Chan, B. P. L., and Wang, D. (2008). Reproductive characters and mating behaviour of wild Nomascus hainanus. Int. J. Primatol. 29, 1037–1046. doi: 10.1007/s10764-008-9272-7
Keywords: habitat quality, Hainan gibbon, population dynamics, primate conservation, remote sensing, species recovery
Citation: Zou Y, Turvey ST, Cui J, Zhang H and Gong W (2022) Recent Recovery of the World’s Rarest Primate Is Not Directly Linked to Increasing Habitat Quality. Front. Ecol. Evol. 10:953637. doi: 10.3389/fevo.2022.953637
Received: 26 May 2022; Accepted: 13 June 2022;
Published: 14 July 2022.
Edited by:
Xiang Liu, Lanzhou University, ChinaCopyright © 2022 Zou, Turvey, Cui, Zhang and Gong. This is an open-access article distributed under the terms of the Creative Commons Attribution License (CC BY). The use, distribution or reproduction in other forums is permitted, provided the original author(s) and the copyright owner(s) are credited and that the original publication in this journal is cited, in accordance with accepted academic practice. No use, distribution or reproduction is permitted which does not comply with these terms.
*Correspondence: Jie Cui, 443464199@qq.com; Wenfeng Gong, gwf101@163.com; Hui Zhang, zhanghuitianxia@126.com
†These authors have contributed equally to this work