- 1State Key Laboratory of Freshwater Ecology and Biotechnology, Institute of Hydrobiology, Chinese Academy of Sciences, Wuhan, China
- 2College of Advanced Agricultural Sciences, University of Chinese Academy of Sciences, Beijing, China
- 3Institute of Eastern Himalaya Biodiversity Research, Dali University, Dali, China
- 4Collaborative Innovation Center for Biodiversity and Conservation in the Three Parallel Rivers Region of China, Dali, China
- 5The Provincial Innovation Team of Biodiversity Conservation and Utility of the Three Parallel Rivers Region from Dali University, Dali, China
- 6The Key Laboratory of Yunnan Education Department on Er’hai Catchment Conservation and Sustainable Development, Dali, China
The traits of organisms provide critical information for understanding changes in biodiversity and ecosystem function at large scales. In recent years, trait databases of macroinvertebrates have been developed across continents. Anyone using different databases to search for traits will encounter a series of problems that lead to uncertain results due to the inconsistency of the trait information. For example, traits for a particular macroinvertebrate taxon may be inconsistent across databases, coded in inconsistent ways, or cannot be found. However, most of the current studies do not clearly state their solutions, which seriously hinders the accuracy and comparability of global trait studies. To solve these problems, we collected representative databases from several continents, including the United States, Europe, South Africa, Bolivia, Australia, and New Zealand. By comparing the inconsistency of similar trait classifications in the nine databases, we harmonized 41 of these grouping features. We found that these databases differed widely in terms of the range and category of traits. And the method of coding traits also varies from database to database. Moreover, we showed a set of trait searching rules that integrate trait databases from different regions of the world, allowing traits to be identified more easily and uniformly using different trait databases worldwide. We also applied this method to determine the traits of 155 macroinvertebrate taxa in the Three Parallel Rivers Region (TPRR). The results showed that among a total of 155 macroinvertebrate taxa, the 41 grouping features of all genera were not fully identified, and 32 genera were not recorded (thus using family-level data). No trait information was found at all for two families, which contain two genera. This suggests that many macroinvertebrate taxa and their traits have not been fully studied, especially in those regions, including China, where macroinvertebrate trait studies are lagging. This inadequacy and unevenness have seriously hindered the study and development of macroinvertebrate trait and functional diversity worldwide. Our results complement the information on stream macroinvertebrate traits in the TPRR, a global biodiversity hotspot, and greatly promote the uniformity of global trait research and the accuracy and comparability of trait research in different regions.
Introduction
The study of functional traits has a long history. Cummins (1973) was the first to apply the functional trait approach to stream biology. Kerans and Karr (1994) proposed a benthic index of biotic integrity (B-IBI) that can be effectively applied to riverine environmental monitoring, incorporating macroinvertebrate traits. After that, many countries began to develop macroinvertebrate trait information for biological monitoring and biodiversity conservation. The earliest development was in Europe. Usseglio-Polatera et al. (2000) started to compile information on macroinvertebrate traits in 2000. Europe uses a fuzzy coding method to code traits, considering the uncertainty of macroinvertebrate traits, which indicates how strongly a taxon expresses a particular trait based on affinity (Chevenet et al., 1994; Usseglio-Polatera et al., 2000). At the same time, the United States began to synthesize the traits of its macroinvertebrate. The trait database of USGS (Vieira et al., 2006) comprehensively summarized the traits of macroinvertebrates in North America for the first time. After that, Twardochleb et al. (2021) and the US EPA (2012) released more comprehensive databases of macroinvertebrate traits by integrating relevant domestic studies. However, unlike Europe, the United States applied a binary approach in which each taxon was assigned to only one trait. These two methods have been used in Australia, New Zealand, and South Africa.
With the development of trait-based ecology, researchers have demonstrated the efficiency of analyzing functional traits and functional diversity in ecological studies (McGill et al., 2006; Alahuhta et al., 2019). A trait is a well-defined and measurable property of organisms at the individual level (McGill et al., 2006; Mouillot et al., 2013). There are several advantages of using trait data for analysis compared to species data: (1) Traits directly reflect the behavior of organisms and control their interrelationships with the environment and other organisms, so that traits can deepen the mechanistic understanding of species-environment and even interspecies relationships (Thornhill et al., 2018; Desrosiers et al., 2019); (2) Traits vary less across geographic regions and can be analyzed across a wide geographic range or even across species (Bernhardt-Roemermann et al., 2011; Heino et al., 2013); (3) Trait data have low requirements for taxonomy and are less susceptible to taxonomic ambiguities or inconsistencies in long-term data (US EPA, 2012) for that genus or family-level traits have long been used in biological assessments (Vieira et al., 2006; Schaefer et al., 2011; Rodil et al., 2013); (4) Traits can be used to calculate a range of functional diversity indices, to detect changes in the functional characteristics of communities, or as indicators to estimate ecosystem function (Carmona et al., 2016; de Bello et al., 2017; Laughlin et al., 2018). With increased human disturbance and warming, the study of biological traits will allow us to better cope with the effects of such changes on ecosystems.
Although trait-based research has developed rapidly in the past few years, trait information of organisms is still the foundation of research. However, it is often difficult to obtain the trait information of species, and the collection and collation of trait data are time-consuming and laborious (US EPA, 2012). This difficulty is especially true for the small and diverse macroinvertebrates in alpine streams. To date, descriptions of macroinvertebrate traits have been scattered among numerous studies. The development of trait databases that focused on aquatic organisms has progressed the most in Europe and North America compared to other regions. The European database contains 8586 taxa (Schmidt-Kloiber and Hering, 2015), making it the largest database. Researchers have recently collated a new database on disperse-related traits of European macroinvertebrates (Sarremejane et al., 2020). Twardochleb et al. (2021) developed a North American database of freshwater insect traits by referring to the research of Poff et al. (2006), the US Geological Survey (USGS; Vieira et al., 2006), the US EPA (2012), and other studies on North American freshwater insects. There are 3,791 macroinvertebrate taxa, which is second only to Europe in terms of data volume. In addition, similar trait databases are available for South Africa (Odume et al., 2018), Australia (Kefford et al., 2020), New Zealand (Phillips and Smith, 2018), and Bolivia (Tomanova and Usseglio-Polatera, 2007). These databases are not unique, but rather describe the same taxa from different traits, forming a complementary.
Unfortunately, there are three obvious problems when using these different databases: (1) There is no uniform way to describe traits information in these databases, and the ways used to describe traits may vary considerably. For example, US EPA (2012) classified macroinvertebrate sizes into 3 categories: small (<9 mm), medium (9–16 mm), and large (>16 mm), whereas Sarremejane et al. (2020) classified them into 7 categories, with the smallest < 0.25 mm and the largest > 8 mm. This inconsistency hampered the development of comparative studies on macroinvertebrate traits worldwide and may lead to erroneous results (Schmera et al., 2015). Kunz et al. (2021) harmonized seven grouping features from six databases in four regions, greatly facilitating the study of traits among different regions. However, many grouping features are scattered in various databases that need to be harmonized, such as rheophily and thermal preference etc., which are all important to macroinvertebrates. Moreover, recent trait-based studies have highlighted the importance of standardizing traits and trait terminology to ensure that data can be integrated more easily in the future (Schmera et al., 2015; Kunz et al., 2021). In this study, we follow the suggestion of Schmera et al. (2015). We use “grouping feature” to refer to the general properties of related traits (rather than “trait”), and “trait” refer to traits that can be measurable at the individual level (rather than “trait state,” “modality” or “trait modality”). (2) These databases contain many duplicate species, but there are inconsistent descriptions of similar traits in these same species. They may all be accurate, but this has caused great confusion for researchers in other regions to determine the macroinvertebrate traits. (3) There are still many macroinvertebrates whose traits have not been described so far. Many species are found in areas with poorly studied macroinvertebrate traits, including some biodiversity hotspots.
The Three Parallel Rivers Region (TPRR) in China has long been recognized as a global biodiversity hotspot (Myers et al., 2000; Xing and Ree, 2017), but studies on macroinvertebrate traits in this region are still scarce (Liu et al., 2021). Three international rivers, including the Yangtze, Mekong, and Salween rivers, flow parallel from north to south in a 100 km wide area and are recognized by UNESCO as a World Natural Heritage Site and a priority area for biodiversity conservation (Myers et al., 2000). In our six study regions, the northernmost Three-River Headwaters region has an average altitude of over 4,000 m and a cold and dry climate. The Dulong River, Zhubaluo River, and Gangqu River in the center are distributed parallel to each other from west to east and are located in the area where the three rivers are closest to each other. And due to the blockage of the summer monsoon by the mountains, precipitation gradually decreases from west to east (Xing and Ree, 2017). These regions and the Cangshan Mountains region have a great altitude span with a distinct vertical climate classification (Wu et al., 1988; Zeitler et al., 2015). The southernmost region of Dehong is below 1,000 m above sea level and has a much warmer climate.
By harmonizing the trait information of macroinvertebrates from different world regions, we proposed a set of trait searching rules with clear steps to help people identify macroinvertebrate traits using different trait databases. At the same time, we applied this set of rules to the TPRR and determined the macroinvertebrate traits in this region. Our aim is not only to extend the seven grouping features harmonized by Kunz et al. (2021) to more grouping features and to extrapolate this trait information to the TPRR in China, but also to try to find an accurate and comprehensive method for trait determination using different databases. This will provide a methodological basis for future research on macroinvertebrate traits in China and even the world. At the same time, it will help us improve the uniformity of research on stream macroinvertebrate traits in China and around the world and further understand the unique and rapidly changing alpine stream ecosystems.
Materials and Equipment
Study Area
The study area is located in the TPRR of Yunnan, China. The north-south-oriented Hengduan Mountains located at the southeastern rim of the Tibetan Plateau, separate Asia’s major rivers in the TPRR, including the Yangtze, Mekong, and Salween rivers. The region has high mountains and deep valleys, with snowcapped mountains over 6,700 m above sea level, most of which are in the national nature reserves and belong to global biodiversity hotspots and priority conservation areas (Myers et al., 2000; Xing and Ree, 2017). The average annual temperature in this region is only 6°C. The average annual precipitation is 624.7 mm and decreases from west to east due to the blocking effect of the Hengduan Mountains on the summer monsoon with abundant water vapor, and 73% of the precipitation is concentrated from June to September (Yu et al., 2018).
This study was conducted mainly in six regions of the TPRR, which are the Three-River Headwaters, Dulong River, Zhubaluo River, Gangqu River, Cangshan Mountain, and Dehong Prefecture region (Supplementary Figure 1 in Supplementary Material). The Three-River Headwaters region has the highest average altitude of more than 4,000 m, the lowest annual precipitation of less than 300 mm, and the lowest average annual temperature below 0°C (Shi et al., 2016). The Dehong Prefecture region has the lowest average altitude of fewer than 1,000 m and the highest average annual temperature of 20°C.1 Furthermore, the Dulong River has the highest average annual precipitation, once recording average annual precipitation of up to 4,800 mm (Li, 1996). Due to this huge difference in altitude, precipitation, and temperature, the TPRR can be a good microcosm of entire China to some extent.
Macroinvertebrate Sampling
A total of 213 sampling sites were collected from six regions, most of which were located at the headwaters of the streams and were wadable. The sites in Dehong were sampled in September 2020, Three-River Headwaters in September 2018, Dulong River in November 2018, Zhubaluo River in November 2017, Cangshan Mountain in November 2012, and Gangqu River in May 2005. All the samplings were carried out in the dry season with stable hydrological conditions. Macroinvertebrates were collected using a 500 μm mesh surber net (30 × 30 cm) within a 100 m river reach. All samples were preserved in 95% ethanol concentrations and brought back to the laboratory for identification (Morse et al., 1994; Merritt et al., 2019). In our sampling, we only focused on aquatic insects, which were identified to genus level, except Chironomidae to subfamily level.
Trait Databases
A total of 213 sampling sites were collected from six regions, most of which were located at the headwaters of the streams and were wadable. The sites in Dehong were sampled in September 2020, Three-River Headwaters in September 2018, Dulong River in November 2018, Zhubaluo River in November 2017, Cangshan Mountain in November 2012, and Gangqu River in May 2005. All the samplings were carried out in the dry season with stable hydrological conditions. Macroinvertebrates were collected using a 500 μm mesh surber net (30 × 30 cm) within a 100 m river reach. All samples were preserved in 95% ethanol concentrations and brought back to the laboratory for identification (Morse et al., 1994; Merritt et al., 2019). In our sampling, we only focused on aquatic insects, which were identified to genus level, except Chironomidae to subfamily level.
Methods
Trait Harmonization
In this study, we chose the binary approach to determine macroinvertebrate traits. The fuzzy coding method is applied in the trait database in Europe and other regions. However, we used the binary method (i.e., each taxon was assigned to only one trait) for the following reasons: (1) The development of macroinvertebrate trait research is lagging in China, and there is not much detailed trait information available. This is one of the critical reasons why Poff et al. (2006) used a binary approach to build a database of macroinvertebrate traits in the first place. (2) There are still many studies on macroinvertebrate traits in China and the world using the binary method (Krynak and Yates, 2018; McKie et al., 2018; Wang et al., 2020). (3) The advantage of this approach is that it simplifies functional and even phylogenetic analyses, thus allowing an exploration of the dominant relationships between traits and macroinvertebrate taxa (Poff et al., 2006).
The main taxonomic resolution we considered was the genus level (except for some Chironomidae where the tribe was used). There are several reasons for this: (1) Aquatic insect larvae have great taxonomic uncertainty at the species level, but it is more likely that congeneric species have similar functional traits (Gayraud et al., 2003; Poff et al., 2006). Using genus-level traits dramatically simplifies the identification work of macroinvertebrates for researchers and reduces the error rate of identification. (2) Trait assignment is inevitably more difficult at higher taxonomic resolution (e.g., species). In contrast, trait information is relatively more abundant and complete at lower taxonomic resolutions (e.g., genus or family; Kefford et al., 2020). (3) Trait information at the genus and family level has long been successfully used in biological assessments in many countries (Vieira et al., 2006; Schaefer et al., 2011; Rodil et al., 2013). Moreover, we ignored the unique grouping features in each database (including some grouping features that look similar but are challenging to harmonize. For example, in the freshwaterecology.info database, there is the grouping feature “dietary preference,” which includes specialist, moderate, and generalist. While another grouping feature “food” specifically describes whether macroinvertebrates eat detritus, animals, or plants. Although these two grouping features look similar, they are difficult to harmonize.) and only harmonized those that appear in at least two databases because these grouping features are unavailable for most of the taxa.
In the process of finding traits, we mainly have the following problems:
(1) When there are multiple sources of trait data for a particular macroinvertebrate, which one should we choose to use?
(2) How can different trait classifications from different sources be harmonized?
(3) If only species-level trait information is available, how should we determine genus-level trait information? What if congeneric species have different traits?
(4) What if a taxon fits multiple traits within a grouping feature?
(5) What if the taxon cannot be found in any of these databases?
Almost anyone searching multiple macroinvertebrate trait databases will encounter these problems. However, many studies do not provide a clear solution, especially those studies where the development of macroinvertebrate trait databases is lagging and need to refer to trait databases in other regions. These studies only provide references to databases or relevant literature and may incorporate expert advice (Addo-Bediako, 2021; Li et al., 2021; Shrestha et al., 2021). Although macroinvertebrate trait databases are described in reports and papers including their process of acquiring trait information, the use of these databases can still be confusing. For example, while the Australian database states its traits as originating from multiple published articles, the database provided simply integrates all the traits from these articles. There are both fuzzy coding data and binary data in this database, and the trait classification methods differ. For a given species, the same grouping feature also has different results. While all this information is valuable, this undoubtedly causes great confusion to database users and may lead to uncertainty in trait studies.
However, the EPA database is an exception. It integrated trait databases from 14 sources in the United States and gave specific harmonization rules. This rule can be summarized as follows: (1) Use the Poff, USGS (Vieira et al., 2006), and other databases in sequence; (2) If traits vary among species within the genera, use the most frequently recorded trait [Twardochleb et al. (2021) also used this rule]; (3) If different traits occur with the same frequency, select the more typical traits that mentioned by other sources (e.g., medium size, univoltine), or select a trait at random. However, these three rules can only solve part of the first three of the five problems mentioned above. Additional rules are needed to determine more traits using databases from multiple regions worldwide.
When we encountered the above problems, we developed a set of rules for determining taxon traits in combination with the US EPA’s method to harmonize trait classification to ensure consistency in the treatment of all traits. For question one (“When there are multiple sources of trait data for a particular macroinvertebrate, which one should we choose to use?”), we consider mainly the zoogeographic realm and the similarity of regional climate. Ecological and historical factors shape species assemblages and thus their trait composition (Southwood, 1977; Cavender-Bares et al., 2009). For example, as the oldest fauna in the world, Australia experienced a long period of isolation from the Mesozoic to Pliocene, making the creature more unique (Holt et al., 2013). And many macroinvertebrate traits may vary with altitude and climate (Magiafoglou et al., 2002; Corbet et al., 2006; Bonada and Dolédec, 2018). Thus regions with similar climates are more likely to have similar traits, especially those that are evolutionarily stable (Poff et al., 2006; Nussey et al., 2007). Based on latitudinal, climatic similarities (Beck et al., 2018) and the zoogeographic realm (Holt et al., 2013), we considered the trait databases of North America (The Nearctic Realm), Europe (The Palearctic realm), South Africa (The Ethiopian Realm), South America (The Neo-Tropical Realm), and Australia and New Zealand (The Australian Realm) in turn. In North America, traits from the latest CONUS database were given priority, followed by the Poff and EPA databases (Since EPA chose the Poff database as the first, we consider the Poff database prior to the EPA.). In Europe, traits from the latest Sarremejane database were given priority, followed by the freshwaterecology.info database. For question two (“How can different trait classifications from different sources be harmonized?”), we determined the harmonization result of many similar grouping features and traits based on the databases mentioned above (Table 1). For question three (“If only species-level trait information is available, how should we determine genus-level trait information? What if congeneric species have different traits?”), consistent with the US EPA (2012), we use the majority rule to determine genus-level traits. For question four (“What if a taxon fits multiple traits within a grouping feature?”), we have two cases: for binary data, we used the trait with the most records; for fuzzy-coded data, we used the trait with the highest affinity (Except for the secondary habit, secondary feeding style, and emerge season two, which used traits with the second-highest affinity). We do not select less special traits or randomly select a trait following the EPA method to reduce the uncertainty of the results. For question five (“What if the taxon cannot be found in any of these databases?”), we tried to replace genus-level traits with family-level traits. Many species within the same family have similar traits (Gayraud et al., 2003; Poff et al., 2006), and most databases also provide traits at the family level. Even so, there may still be undetermined traits. Therefore, like most databases, we use NA to indicate indeterminate traits.
Trait Searching Rules
The following are the specific rules we developed for determining traits within a grouping feature at the genus level (Figure 1):
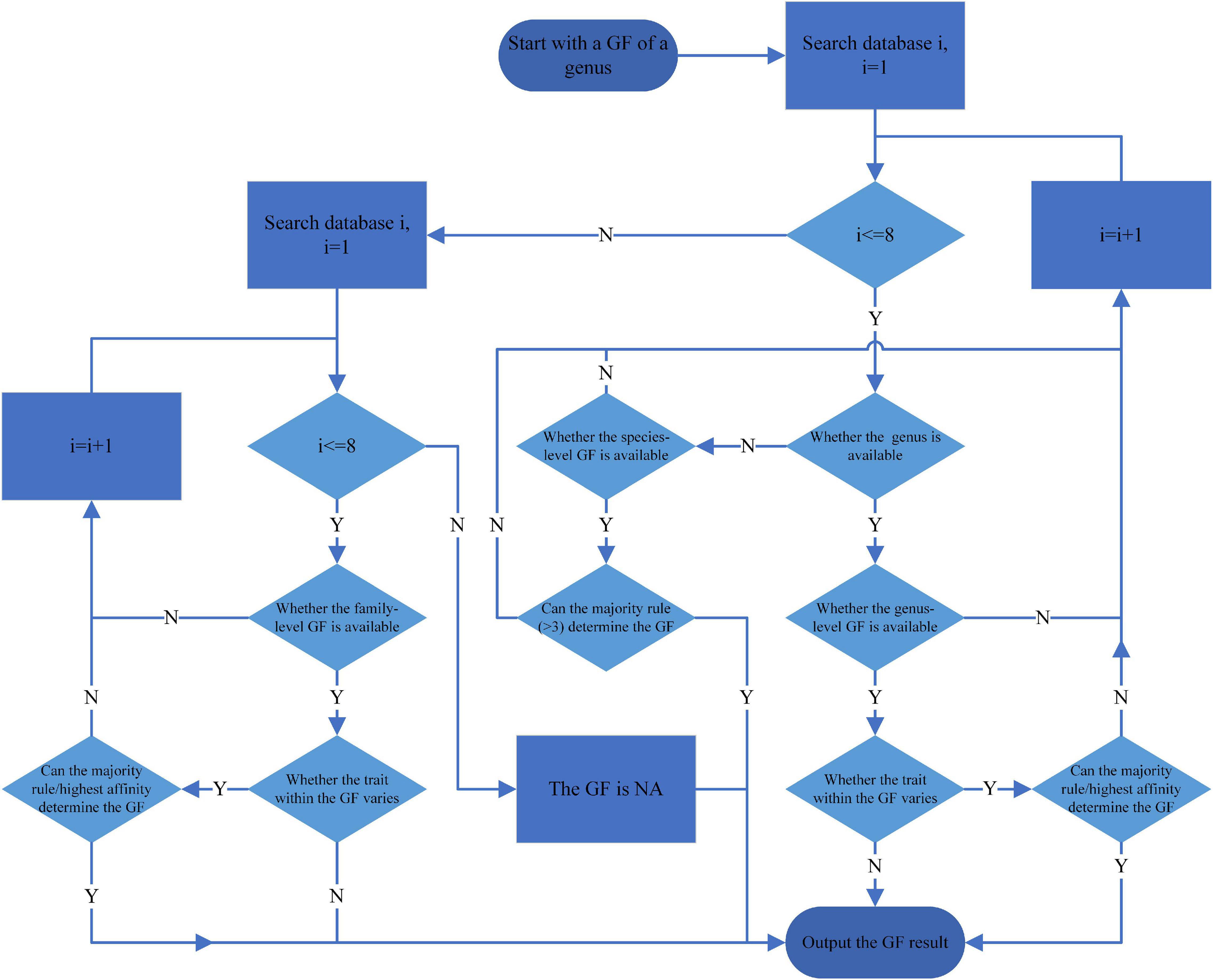
Figure 1. Flowchart for determining genus-level grouping features (GF) from the databases. The oval represents a start or end point, the rectangle represents a process, and the diamond represents a decision. According to the databases searching sequence, the databases are considered as databases one to nine, respectively.
(1) Sequentially, using the CONUS, Poff, EPA, Sarremejane, freshwaterecology.info, South African, Bolivian, Australian, and New Zealand databases. (The Australia and freshwaterecology.info databases contain many similar or identical grouping features. When we search these two databases, we combine similar grouping features and use them as one. For example, use the result when the results of multiple similar grouping features are consistent or when only one grouping feature can determine the results. Otherwise, this grouping feature is considered in an uncertain state.) It is worth noting that when the study area changes, the search order of the database should be changed according to the similarity of the area. And the local study of macroinvertebrate traits should be prioritized.
(2) When using different trait classifications from different databases, the trait classifications are determined according to the harmonization results in Table 1. The selection of standard grouping features was determined primarily by their ability to include as many trait categories as possible within all other grouping features and by the extensiveness of their use. And other similar grouping features from different databases were harmonized according to the classification of the standard grouping features.
(3) When the genus that to be found available in the database, find the trait information of the genus. If the genus fits more than one trait under a grouping feature, i.e., the trait varies within the grouping feature. Use the most frequently occurring trait (binary data) or the highest affinity trait (fuzzy coded data). For example, suppose the respiration mode is both tegument and gills. The number of tegument respiration records is three and gills is one (binary data), or the affinity of tegument respiration is three and gills is one (fuzzy coded data). In that case, the respiration mode of this genus is tegument.
(4) If the database has the genus but no information on a particular grouping feature or multiple traits has the same frequency of occurrence (binary data), or multiple traits have the same affinity (fuzzy coded data), then use the next database.
(5) If only species-level trait information is available in the database but not genus-level, use the trait that occurs most frequently among species. The number of the same trait should be at least three, and only calculate the trait with the highest affinity when encountering the fuzzy-coded data). If the rule cannot determine the trait, search the trait information at the family level of the genus. For example, if three or more of the five species within a genus have an identical trait, the genus is considered to have this trait. Otherwise, use the next database.
(6) When a grouping feature of the genus could not be determined after searching the last database, search the trait information at the family level from the first database.
(7) Use the information when a family-level grouping feature needs to be searched available in the database. If the traits within the grouping feature vary, then the most frequently occurring trait (binary data) or the one with the highest affinity (fuzzy coded data) within the grouping feature was used as in step 3 above.
(8) If the family could not be found, or different traits have the same frequency of occurrence (binary data), or multiple traits have the same affinity (fuzzy coded data), then use the next database.
(9) If the grouping feature cannot be determined after using the last database, the grouping feature is considered currently undetermined (noted as NA).
Results
In the table of harmonization results for different trait classifications we identified, 41 grouping features with 154 traits were harmonized (Table 2). Of the 41 grouping features, seven standard grouping features from Kunz et al. (2021) were selected as standard grouping features, six in the CONUS database, nine in the Poff database, nine in the EPA database, two in the Sarremejane database, one in the freshwaterecology.info database, three in the South African database, three in the Australian database, and one in the New Zealand database. All other grouping features are harmonized with the standard grouping features mentioned above. More detailed trait harmonization results are presented in Supplementary Material for convenient use by researchers.
In our process of harmonizing traits, there are roughly 3 scenarios: (1) Harmonizing grouping features with specific ranges. Examples include size, thermal preference, and adult life span. These traits are relatively easy to harmonize due to their clear ranges. (2) Harmonizing entirely categorical grouping features. It is easy to harmonize if the trait categories are consistent within the grouping features. Examples include voltinism, emergence season, and female dispersal. Conversely, if inconsistent, it is difficult to harmonize. For example, in the freshwaterecology.info database, reproduction type includes both the location of eggs (terrestrial or aquatic) and the type of eggs (free isolated. cemented isolated, fixed clutches, or free clutches). While in the South African database, the location of eggs and the type of eggs are two grouping features. (3) Harmonizing grouping features containing both categories and specific ranges. These grouping features are relatively the most difficult to harmonize, even impossible to harmonize. For example, in the EPA database, salinity tolerance is classified as fresh, brackish, and saline, while the Australian database classifies salinity tolerance according to the magnitude of electrical conductivity. Examples also include rheophily and development speed.
A total of 153 genera, 71 families, and 10 orders (Ephemeroptera, Odonata, Plecoptera, Hemiptera, Neuroptera, Megaloptera, Trichoptera, Lepidoptera, Coleoptera, and Diptera) of macroinvertebrates were found in our study (including three subfamilies of Chironomidae). When we applied the rules to determine the traits, the results showed that the grouping features of all genera were not fully determined. Furthermore, 32 genera had no records (thus using family-level traits), accounting for 20.9% of the total taxa. These genera belong to 10 orders and 22 families, which indicates that all families in this region contain genera for which no records could be found. No trait information was found for two families (Deuterophllobldae and Phryganopsychidae), which contain two genera. (See Supplementary Material for detailed results.)
Discussion
Worldwide, there are approximately 50,000 species of aquatic insects (Balian et al., 2008). However, there are fewer than 20,000 species in the United States, European, Australian, South African, New Zealand, and Bolivian databases without considering the same taxon. It is evident that the current study of macroinvertebrates is still far from adequate in terms of species numbers. This problem is particularly evident in the TPRR. Our results showed that a total of 34 genera were not recorded, accounting for approximately 22% of the total. Moreover, two families have no records. This reflects not only the inadequacy of global studies on macroinvertebrate traits but also unevenness. Many developing countries, including China, often do not have enough macroinvertebrate studies to establish a trait database. However, at the same time, numerous endemic macroinvertebrates are scattered in various regions of the globe. As a result, many studies have to exclude species without trait information (Krynak and Yates, 2018), which seriously hinders the development of comparative studies on macroinvertebrate traits worldwide and may lead to erroneous results (Schmera et al., 2015).
The trait classifications used in current research are often predefined by scientists. However, studies using such predefined and variable numbers of traits undoubtedly impact many research results. The negative impact is mainly reflected in the following points: (1) How macroinvertebrate traits are described and their numbers vary significantly from database to database. For example, the Poff database described a total of 59 traits for 20 grouping features, while the freshwaterecology.info database described nearly 40 grouping features with hundreds of traits. (2) Several similar traits of macroinvertebrates have been described in different ways. For example, dispersal is defined in the European and South African databases as aquatic active, aquatic active, aquatic passive, aerial active, and aerial passive. In contrast, the dispersal traits in the EPA and New Zealand databases are described as the level of dispersal distance before female spawning, the strength of adult flight ability, and the frequency of drift occurrence. (3) Many species have different trait descriptions in different databases. They may all be true for reasons such as differences in geographic regions and the presence of many interference factors, but the reason for this is not apparent. (4) Insufficient research on some specific species. For example, many Coleoptera adults and larvae live in water, and their morphology is entirely different, so their traits should be different. However, only the Australian and Bolivian databases classified this condition, and many other databases do not take this into account. In addition, synonymy is one of the factors leading to uncertain results. However, these problems should be left to professional taxonomists and are not the focus of this paper. (5) An unstated assumption of trait-based analyses is that characterizing traits for late instars are adequate to account for organism-environment relations, but this may not always be the case (Poff et al., 2006). Although recently Kunz et al. (2021) harmonized traits within six databases in four regions, it is undeniable that different traits provide valuable information on different aspects of trait research. Therefore, we harmonized the traits that appeared in at least two of the eight databases to promote the use of more traits.
Although we assigned most macroinvertebrate grouping features to only one trait, trait values are usually characterized by their uncertainty. The uncertainty is because macroinvertebrate traits may change at different life stages (e.g., feeding groups, respiration) and to our lack of knowledge of most taxa (Kefford et al., 2020). Some databases, including those in Europe, use the fuzzy coding method to solve this problem. However, for the reasons stated in the method section, we applied the binary method. We do not deny that the fuzzy coding method provides precious information for describing different taxon traits. This method takes into account some intraspecific variations of macroinvertebrate traits. Perhaps fuzzy coding of different traits or multi-state binary approaches should be considered in the future as research progresses. For example, Li et al. (2020) found that the voltinism of the Drunella submontane (Ephemeroptera: Ephemerellidae) varies with altitude: univoltine below 1,600 m and semivoltine above 2,100 m. Therefore, in future studies, the voltinism of the species should be determined to be univoltine or semivoltine, depending on the sampling elevation.
It is worth noting that the method we provide has its limitations. Although it is generally believed that the traits vary less across geographic regions (Bernhardt-Roemermann et al., 2011; Heino et al., 2013). And many studies have used trait information from other regions without validation. For example, studies in China referenced traits from North America and Europe (Addo-Bediako, 2021; Shrestha et al., 2021), and studies in Nepal and South Africa referenced traits from North and South America (Addo-Bediako, 2021; Shrestha et al., 2021). However, the use of trait information from other regions may not be appropriate for evolutionary labile traits, i.e., traits that are not constrained by phylogeny and thus respond to environmental change (Poff et al., 2006). Examples include traits describing body size, feeding type, and voltinism (Bonada and Dolédec, 2018; Wilkes et al., 2020). Consequently, individuals of the same species may have different traits when they inhabit habitats with different environmental conditions. In contrast, for phylogenetically constrained traits like respiration or locomotion type, it is possible to use trait information from different regions (Poff et al., 2006; Bonada and Dolédec, 2018). However, the lability of macroinvertebrate traits has not been sufficiently studied (Bonada and Dolédec, 2018; Wilkes et al., 2020). This important work will not only enable us to use trait databases more accurately but also improve our understanding and prediction of trait-environment relationships.
Despite the rapid development of trait-based ecological research, the trait-based approach has its limitations. First, traits do not evolve independently in response to single environmental stress (de Bello et al., 2017) but are related because of mechanistic relationships or phylogenetic constraints (Verberk et al., 2013; Hamilton et al., 2020), and thus appear as specific combinations of traits (Poff et al., 2006). For example, multivoltine species necessarily have rapid development and small size (Resh et al., 1988; Hamilton et al., 2020). Consequently, a causal mechanism by which a trait appears to influence a species’ persistence may be related to another correlated trait (Hamilton et al., 2020). And spurious causality may seriously confound trait-based applications (Poff et al., 2006; Pilière et al., 2016; Hamilton et al., 2020). Second, although a large number of functional diversity indices have been developed to explore the relationship between traits and the environment, the current use of functional diversity indices is confusing (Mammola et al., 2021). And the number of traits to be included in the initial trait matrix for functional diversity calculation to adequately reflect the relationship between organisms and their environment is still unresolved (Maasri, 2019). Some studies used approximately a dozen traits (Statzner et al., 2005; Wang et al., 2020), while others used more than sixty (Feld et al., 2014). This significantly hinders relevant comparative studies and meta-analyses.
A large number of macroinvertebrate trait databases have been developed worldwide, yet data usage remains challenging due to inconsistencies in trait classification. By comparing inconsistencies in trait classification in nine trait databases across six continents worldwide, we harmonized 41 common grouping features. Moreover, we try to propose a set of methods to help researchers determine traits using a unified procedure. These results link macroinvertebrate trait databases from different regions of the world, laying a solid foundation for the establishment of a globally unified trait database, and may contribute to the accuracy and comparability of trait studies in different regions in the future. However, the current macroinvertebrate trait studies are still far from adequate, especially in areas where macroinvertebrate trait studies are lagging with many macroinvertebrate taxa and their traits remain undetermined. Before reaching the goal of global harmonization, vigorous development of local macroinvertebrate trait studies in each region of the world is still needed. Therefore, this paper advocates the development of macroinvertebrate trait research in China, a region where macroinvertebrate traits are currently poorly studied and lack integration.
Data Availability Statement
The original contributions presented in the study are included in the article/Supplementary Material, further inquiries can be directed to the corresponding author.
Ethics Statement
Our research does not involve any regulated animals, including all live vertebrates and higher invertebrates, but only benthic macroinvertebrate. And we believe that this type of research does not require an ethics statement and has not previously been required for this type of research (Luo et al., 2021).
Author Contributions
SA and QC conceived the ideas and designed the methodology. SA, XL, ZT, and JH sampled the macroinvertebrate. SA, XL, and ZT identified the macroinvertebrate. SA and QC analyzed the data and led wrote the manuscript. All authors contributed critically to the drafts and gave final approval for publication.
Funding
This study was funded by the Second Tibetan Plateau Scientific Expedition and Research Program (2019QZKK0402), the National Natural Science Foundation of China (U1602262), and the State Key Laboratory of FEBL Research Grant (2019FBZ01).
Conflict of Interest
The authors declare that the research was conducted in the absence of any commercial or financial relationships that could be construed as a potential conflict of interest.
Publisher’s Note
All claims expressed in this article are solely those of the authors and do not necessarily represent those of their affiliated organizations, or those of the publisher, the editors and the reviewers. Any product that may be evaluated in this article, or claim that may be made by its manufacturer, is not guaranteed or endorsed by the publisher.
Acknowledgments
We give special thanks to Wen Xiao and Shuoran Liu from the Institute of Eastern Himalaya Biodiversity Research, Dali University, for their generous help in the field sampling.
Supplementary Material
The Supplementary Material for this article can be found online at: https://www.frontiersin.org/articles/10.3389/fevo.2022.945824/full#supplementary-material
Footnotes
References
Addo-Bediako, A. (2021). Spatial distribution patterns of benthic macroinvertebrate functional feeding groups in two rivers of the olifants river system. South Africa. J. Freshw. Ecol. 36, 97–109. doi: 10.1080/02705060.2021.1901789
Alahuhta, J., Eros, T., Karna, O., Soininen, J., Wang, J., and Heino, J. (2019). Understanding environmental change through the lens of trait-based, functional, and phylogenetic biodiversity in freshwater ecosystems. Environ. Rev. 27, 263–273. doi: 10.1139/er-2018-2071
Balian, E. V., Segers, H., Lévêque, C., and Martens, K. (2008). An introduction to the Freshwater Animal Diversity Assessment (FADA) project. Hydrobiologia 595, 3–8. doi: 10.1007/s10750-007-9235-9236
Beck, H. E., Zimmermann, N. E., McVicar, T. R., Vergopolan, N., Berg, A., and Wood, E. F. (2018). Present and future Köppen-Geiger climate classification maps at 1-km resolution. Sci. Data 5:180214. doi: 10.1038/sdata.2018.214
Bernhardt-Roemermann, M., Gray, A., Vanbergen, A. J., Berges, L., Bohner, A., Brooker, R. W., et al. (2011). Functional traits and local environment predict vegetation responses to disturbance: a pan-European multi-site experiment. J. Ecol. 99, 777–787. doi: 10.1111/j.1365-2745.2011.01794.x
Bonada, N., and Dolédec, S. (2018). Does the Tachet trait database report voltinism variability of aquatic insects between Mediterranean and Scandinavian regions? Aquat. Sci. 80:7. doi: 10.1007/s00027-017-0554-z
Carmona, C. P., de Bello, F., Mason, N. W. H., and Leps, J. (2016). Traits without borders: integrating functional diversity across scales. Trends Ecol. Evol. 31, 382–394. doi: 10.1016/j.tree.2016.02.003
Cavender-Bares, J., Kozak, K. H., Fine, P. V., and Kembel, S. W. (2009). The merging of community ecology and phylogenetic biology. Ecol. Lett. 12, 693–715. doi: 10.1111/j.1461-0248.2009.01314.x
Chevenet, F., Doledec, S., and Chessel, D. (1994). A fuzzy coding approach for the analysis of long-term ecological data. Freshw. Biol. 31, 295–309. doi: 10.1111/j.1365-2427.1994.tb01742.x
Corbet, P. S., Suhling, F., and Soendgerath, D. (2006). Voltinism of Odonata: a review. Int. J. Odonatol. 9, 1–44. doi: 10.1080/13887890.2006.9748261
Cummins, K. W. (1973). Trophic relations of aquatic insects. Annu. Rev. Entomol. 18, 183–206. doi: 10.1146/annurev.en.18.010173.001151
de Bello, F., Smilauer, P., Diniz-Filho, J. A. F., Carmona, C. P., Lososova, Z., Herben, T., et al. (2017). Decoupling phylogenetic and functional diversity to reveal hidden signals in community assembly. Methods Ecol. Evol. 8, 1200–1211. doi: 10.1111/2041-210x.12735
Desrosiers, M., Usseglio-Polatera, P., Archaimbault, V., Larras, F., Methot, G., and Pinel-Alloul, B. (2019). Assessing anthropogenic pressure in the St. Lawrence River using traits of benthic macroinvertebrates. Sci. Total Environ. 649, 233–246. doi: 10.1016/j.scitotenv.2018.08.267
Feld, C. K., de Bello, F., and Doledec, S. (2014). Biodiversity of traits and species both show weak responses to hydromorphological alteration in lowland river macroinvertebrates. Freshw. Biol. 59, 233–248. doi: 10.1111/fwb.12260
Gayraud, S., Statzner, B., Bady, P., Haybachp, A., Schöll, F., Usseglio-Polatera, P., et al. (2003). Invertebrate traits for the biomonitoring of large European rivers: an initial assessment of alternative metrics. Freshw. Biol. 48, 2045–2064. doi: 10.1046/j.1365-2427.2003.01139.x
Hamilton, A. T., Schafer, R. B., Pyne, M. I., Chessman, B., Kakouei, K., Boersma, K. S., et al. (2020). Limitations of trait-based approaches for stressor assessment: the case of freshwater invertebrates and climate drivers. Glob. Change Biol. 26, 364–379. doi: 10.1111/gcb.14846
Heino, J., Schmera, D., and Eros, T. (2013). A macroecological perspective of trait patterns in stream communities. Freshw. Biol. 58, 1539–1555. doi: 10.1111/fwb.12164
Holt, B., Lessard, J. P., Borregaard, M. K., Fritz, S. A., Araujo, M. B., Dimitrov, D., et al. (2013). An update of wallace’s zoogeographic regions of the world. Science 339, 74–78. doi: 10.1126/science.1228282
Kefford, B. J., Botwe, P. K., Brooks, A. J., Kunz, S., Marchant, R., Maxwell, S., et al. (2020). An integrated database of stream macroinvertebrate traits for Australia: concept and application. Ecol. Indic. 114:106280. doi: 10.1016/j.ecolind.2020.106280
Kerans, B. L., and Karr, J. R. (1994). A benthic index of biotic integrity (B-IBI) for rivers of the Tennessee Valley. Ecol. Appl. 4, 768–785. doi: 10.2307/1942007
Krynak, E. M., and Yates, A. G. (2018). Benthic invertebrate taxonomic and trait associations with land use in an intensively managed watershed: implications for indicator identification. Ecol. Indic. 93, 1050–1059. doi: 10.1016/j.ecolind.2018.06.002
Kunz, S., Kefford, B. J., Schmidt-Kloiber, A., Matthaei, C. D., Usseglio-Polatera, P., Graf, W., et al. (2021). Tackling inconsistencies among freshwater invertebrate trait databases: harmonising across continents and aggregating taxonomic resolution. Freshw. Biol. 67, 275–291. doi: 10.1111/fwb.13840
Laughlin, D. C., Chalmandrier, L., Joshi, C., Renton, M., Dwyer, J. M., and Funk, J. L. (2018). Generating species assemblages for restoration and experimentation: a new method that can simultaneously converge on average trait values and maximize functional diversity. Methods Ecol. Evol. 9, 1764–1771. doi: 10.1111/2041-210x.13023
Li, X. (1996). Landforms in DRung river basin (in Chinese). Yunnan Grographic Environ. Res. 8, 59–72.
Li, X., Tan, L., Du, H., Li, S., Guo, S., Mo, J., et al. (2020). Life history flexibility of Drunella submontana Brodsky, 1930 (Ephemeroptera: Ephemerellidae) along altitude gradients in Shennongjia National Park, China. Aquat. Insects 41, 55–66. doi: 10.1080/01650424.2019.1708405
Li, Z., Heino, J., Liu, Z., Meng, X., Chen, X., Ge, Y., et al. (2021). The drivers of multiple dimensions of stream macroinvertebrate beta diversity across a large montane landscape. Limnol. Oceanogr. 66, 226–236. doi: 10.1002/lno.11599
Liu, S., Li, X., Tan, L., Fornacca, D., Fang, Y., Zhu, L., et al. (2021). The ecological niche and terrestrial environment jointly influence the altitudinal pattern of aquatic biodiversity. Sci. Total Environ. 800:149404. doi: 10.1016/j.scitotenv.2021.149404
Luo, Q., Chiu, M.-C., Tan, L., and Cai, Q. (2021). An environmental flow framework for riverine macroinvertebrates during dry and wet seasons through non-linear ecological modeling. Front. Ecol. Evol. 9:734716. doi: 10.3389/fevo.2021.734716
Maasri, A. (2019). A global and unified trait database for aquatic macroinvertebrates: the missing piece in a global approach. Front. Environ. Sci. 7:65. doi: 10.3389/fenvs.2019.00065
Magiafoglou, A., Carew, M., and Hoffmann, A. (2002). Shifting clinal patterns and microsatellite variation in Drosophila serrata populations: a comparison of populations near the southern border of the species range. J. Evol. Biol. 15, 763–774. doi: 10.1046/j.1420-9101.2002.00439.x
Mammola, S., Carmona, C. P., Guillerme, T., and Cardoso, P. (2021). Concepts and applications in functional diversity. Funct. Ecol. 35, 1869–1885. doi: 10.1111/1365-2435.13882
McGill, B. J., Enquist, B. J., Weiher, E., and Westoby, M. (2006). Rebuilding community ecology from functional traits. Trends Ecol. Evol. 21, 178–185. doi: 10.1016/j.tree.2006.02.002
McKie, B. G., Sandin, L., Carlson, P. E., and Johnson, R. K. (2018). Species traits reveal effects of land use, season and habitat on the potential subsidy of stream invertebrates to terrestrial food webs. Aquat. Sci. 80:15. doi: 10.1007/s00027-018-0565-564
Merritt, R. W., Cummins, K. W., and Berg, M. B. (2019). An Introduction to the Aquatic Insects of North America, 5th Edn. Kendall Hunt: United States of America.
Morse, J. C., Yang, L., and Tian, L. (1994). Aquatic Insects of China Useful for Monitoring Water Quality. Nanjing: Hohai University Press.
Mouillot, D., Graham, N. A. J., Villeger, S., Mason, N. W. H., and Bellwood, D. R. (2013). A functional approach reveals community responses to disturbances. Trends Ecol. Evol. 28, 167–177. doi: 10.1016/j.tree.2012.10.004
Myers, N., Mittermeler, R. A., Mittermeler, C. G., Da Fonseca, G. A. B., and Kent, J. (2000). Biodiversity hotspots for conservation priorities. Nature 403, 853–858. doi: 10.1038/35002501
Nussey, D., Wilson, A., and Brommer, J. (2007). The evolutionary ecology of individual phenotypic plasticity in wild populations. J. Evol. Biol. 20, 831–844. doi: 10.1111/j.1420-9101.2007.01300.x
Odume, O., Ntloko, P., Akamagwuna, F., Dallas, H., and Barber-James, H. (2018). Development of Macroinvertebrate Trait-based Approach for Assessing and Managing Ecosystem Health in South African Rivers-incoporating a Case Study in the Tsitsa River and its Tributaries, Eastern Cape. Pretoria: Water Research Commission. WRC Project No: K1/7157.
Phillips, N., and Smith, B. (2018). New Zealand Freshwater Macroinvertebrate Trait Database. 2021. Available online at: https://niwa.co.nz/freshwater-and-estuaries/management-tools/aquatic-invertebrate-traits-database (accessed December 10, 2021).
Pilière, A. F. H., Verberk, W. C. E. P., Gräwe, M., Breure, A. M., Dyer, S. D., Posthuma, L., et al. (2016). On the importance of trait interrelationships for understanding environmental responses of stream macroinvertebrates. Freshw. Biol. 61, 181–194. doi: 10.1111/fwb.12690
Poff, N. L., Olden, J. D., Vieira, N. K. M., Finn, D. S., Simmons, M. P., and Kondratieff, B. C. (2006). Functional trait niches of North American lotic insects: traits-based ecological applications in light of phylogenetic relationships. J. N. Am. Benthol. Soc. 25, 730–755.
Resh, V. H., Brown, A. V., Covich, A. P., Gurtz, M. E., Li, H. W., Minshall, G. W., et al. (1988). The role of disturbance in stream ecology. J. N. Am. Benthol. Soc. 7, 433–455. doi: 10.2307/1467300
Rodil, I. F., Lohrer, A. M., Hewitt, J. E., Townsend, M., Thrush, S. F., and Carbines, M. (2013). Tracking environmental stress gradients using three biotic integrity indices: advantages of a locally-developed traits-based approach. Ecol. Indic. 34, 560–570. doi: 10.1016/j.ecolind.2013.06.023
Sarremejane, R., Cid, N., Stubbington, R., Datry, T., Alp, M., Cañedo-Argüelles, M., et al. (2020). DISPERSE, a trait database to assess the dispersal potential of European aquatic macroinvertebrates. Sci. Data 7:386. doi: 10.1038/s41597-020-00732-737
Schaefer, R. B., Pettigrove, V., Rose, G., Allinson, G., Wightwick, A., and von, et al. (2011). Effects of pesticides monitored with three sampling methods in 24 sites on macroinvertebrates and microorganisms. Environ. Sci. Technol. 45, 1665–1672. doi: 10.1021/es103227q
Schmera, D., Podani, J., Heino, J., Erõs, T., and Poff, N. L. (2015). A proposed unified terminology of species traits in stream ecology. Freshw. Sci. 34, 823–830. doi: 10.1086/681623
Schmidt-Kloiber, A., and Hering, D. (2015). www.freshwaterecology.info - an online tool that unifies, standardises and codifies more than 20,000 European freshwater organisms and their ecological preferences. Ecol. Indic. 53, 271–282. doi: 10.1016/j.ecolind.2015.02.007
Shi, H., Li, T., Wei, J., Fu, W., and Wang, G. (2016). Spatial and temporal characteristics of precipitation over the Three-River Headwaters region during 1961-2014. J. Hydrol. Regional Stud. 6, 52–65. doi: 10.1016/j.ejrh.2016.03.001
Shrestha, S., Tachamo-Shah, R. D., Doody, T., Cuddy, S., and Shah, D. N. (2021). Establishing the relationship between benthic macroinvertebrates and water level fluctuation in subtropical shallow wetlands. Environ. Monit. Assess. 193, 1–22. doi: 10.1007/s10661-021-09225-9225
Southwood, T. R. E. (1977). Habitat, the templet for ecological strategies? J. Anim. Ecol. 46, 337–365. doi: 10.2307/3817
Statzner, B., Bady, P., Dolédec, S., and Schöll, F. (2005). Invertebrate traits for the biomonitoring of large European rivers: an initial assessment of trait patterns in least impacted river reaches. Freshw. Biol. 50, 2136–2161. doi: 10.1111/j.1365-2427.2005.01447.x
Thornhill, I. A., Biggs, J., Hill, M. J., Briers, R., Gledhill, D., Wood, P. J., et al. (2018). The functional response and resilience in small waterbodies along land-use and environmental gradients. Glob. Change Biol. 24, 3079–3092. doi: 10.1111/gcb.14149
Tomanova, S., and Usseglio-Polatera, P. (2007). Patterns of benthic community traits in neotropical streams: relationship to mesoscale spatial variability. Fundam. Appl. Limnol. 170, 243–255. doi: 10.1127/1863-9135/2007/0170-0243
Twardochleb, L., Hiltner, E., Pyne, M., and Zarnetske, P. (2021). Freshwater insects CONUS: a database of freshwater insect occurrences and traits for the contiguous United States. Global Ecol. Biogeogr. 30, 826–841. doi: 10.1111/geb.13257
US EPA (2012). Freshwater Biological Traits Database (final report). Washington, DC: U.S. Environmental Protection Agency.
Usseglio-Polatera, P., Bournaud, M., Richoux, P., and Tachet, H. (2000). Biological and ecological traits of benthic freshwater macroinvertebrates: relationships and definition of groups with similar traits. Freshw. Biol. 43, 175–205. doi: 10.1046/j.1365-2427.2000.00535.x
Verberk, W. C. E. P., Van Noordwijk, C. G. E., and Hildrew, A. G. (2013). Delivering on a promise: integrating species traits to transform descriptive community ecology into a predictive science. Freshw. Sci. 32, 531–547. doi: 10.1899/12-092.1
Vieira, N. K. M., Poff, N. L., Carlisle, D. M., Moulton, S. R. II, Koski, M. L., and Kondratieff, B. C. (2006). A Database of Lotic Invertebrate Traits for North America. Available online at: https://pubs.usgs.gov/ds/ds187/ (accessed December 10, 2021).
Wang, J., Hu, J., Tang, T., Heino, J., Jiang, X., Li, Z., et al. (2020). Seasonal shifts in the assembly dynamics of benthic macroinvertebrate and diatom communities in a subtropical river. Ecol. Evol. 10, 692–704. doi: 10.1002/ece3.5904
Wilkes, M. A., Edwards, F., Jones, J. I., Murphy, J. F., England, J., Friberg, N., et al. (2020). Trait-based ecology at large scales: assessing functional trait correlations, phylogenetic constraints and spatial variability using open data. Glob. Change Biol. 26, 7255–7267. doi: 10.1111/gcb.15344
Wu, X. D., Lin, Z. Y., and Sun, L. (1988). A preliminary study on the climatic change of the hengduan mountains area since 1600 A.D. Adv. Atmos. Sci. 5, 437–443. doi: 10.1007/BF02656789
Xing, Y., and Ree, R. H. (2017). Uplift-driven diversification in the Hengduan Mountains, a temperate biodiversity hotspot. Proc. Natl. Acad. Sci. U S A. 114, E3444–E3451. doi: 10.1073/pnas.1616063114
Yu, J., Liu, Q., Meng, S., Zhou, G., Shah, S., and Xu, Z. (2018). Summer temperature variability inferred from tree-ring records in the central Hengduan Mountains, southeastern Tibetan Plateau. Dendrochronologia 51, 92–100. doi: 10.1016/j.dendro.2018.08.004
Keywords: macroinvertebrate, the Three Parallel Rivers Region, stream ecology, trait, trait harmonization, trait determination
Citation: Ao S, Li X, Tian Z, Hu J and Cai Q (2022) Harmonizing and Searching Macroinvertebrate Trait Information in Alpine Streams: Method and Application–A Case Study in the Three Parallel Rivers Region, China. Front. Ecol. Evol. 10:945824. doi: 10.3389/fevo.2022.945824
Received: 17 May 2022; Accepted: 06 June 2022;
Published: 23 June 2022.
Edited by:
Zhang Min, China Institute of Water Resources and Hydropower Research, ChinaReviewed by:
Kun Li, Heilongjiang University, ChinaXiaoli Tong, South China Agricultural University, China
Copyright © 2022 Ao, Li, Tian, Hu and Cai. This is an open-access article distributed under the terms of the Creative Commons Attribution License (CC BY). The use, distribution or reproduction in other forums is permitted, provided the original author(s) and the copyright owner(s) are credited and that the original publication in this journal is cited, in accordance with accepted academic practice. No use, distribution or reproduction is permitted which does not comply with these terms.
*Correspondence: Qinghua Cai, qhcai@ihb.ac.cn