- 1Division of Ecology and Evolution, Research School of Biology, The Australian National University, Acton, ACT, Australia
- 2Sciences Department, Museums Victoria, Melbourne, VIC, Australia
- 3Australian Museum Research Institute, Australian Museum, Sydney, NSW, Australia
- 4Department of Life Sciences, Natural History Museum, London, United Kingdom
The application of high-throughput, short-read sequencing to degraded DNA has greatly increased the feasibility of generating genomic data from historical museum specimens. While many published studies report successful sequencing results from historical specimens; in reality, success and quality of sequence data can be highly variable. To examine predictors of sequencing quality, and methodological approaches to improving data accuracy, we generated and analyzed genomic sequence data from 115 historically collected museum specimens up to 180 years old. Data span both population genomic and phylogenomic scales, including historically collected specimens from 34 specimens of four species of Australian rock-wallabies (genus Petrogale) and 92 samples from 79 specimens of Australo-Papuan murine rodents (subfamily Murinae). For historical rodent specimens, where the focus was sampling for phylogenomics, we found that regardless of specimen age, DNA sequence libraries prepared from toe pad or bone subsamples performed significantly better than those taken from the skin (in terms of proportion of reads on target, number of loci captured, and data accuracy). In total, 93% of DNA libraries from toe pad or bone subsamples resulted in reliable data for phylogenetic inference, compared to 63% of skin subsamples. For skin subsamples, proportion of reads on target weakly correlated with collection year. Then using population genomic data from rock-wallaby skins as a test case, we found substantial improvement in final data quality by mapping to a high-quality “closest sister” de novo assembly from fresh tissues, compared to mapping to a sample-specific historical de novo assembly. Choice of mapping approach also affected final estimates of the number of segregating sites and Watterson's θ, both important parameters for population genomic inference. The incorporation of accurate and reliable sequence data from historical specimens has important outcomes for evolutionary studies at both population and phylogenomic scales. By assessing the outcomes of different approaches to specimen subsampling, library preparation and bioinformatic processing, our results provide a framework for increasing sequencing success for irreplaceable historical specimens.
Introduction
The application of high-throughput, short-read sequencing to historical museum specimens has accelerated the pace of collections-based genomics. Historical museum specimens that were not sampled with the intention to preserve genetic material (e.g., skins, skeletons and fluid-preserved specimens) are now the only viable source of genomic data for many rare, elusive or extinct species, or extirpated populations. Such specimens have proven critical in reconstructing evolutionary history across the Tree of Life (Mason et al., 2011; Guschanski et al., 2013; Besnard et al., 2016; McCormack et al., 2016; Roycroft et al., 2021b), and in understanding genetic responses of species to recent environmental change and anthropogenic impact (Bi et al., 2013, 2019; Roycroft et al., 2021b). Genomic data from museum specimens can fill crucial sample gaps for studies of evolutionary processes across scales of divergence, from population-level to macroevolutionary analyses.
For studies at the population level, historical museum specimens can provide more comprehensive geographic sampling, especially where species are threatened or extirpated (e.g., Ewart et al., 2019; Roycroft et al., 2021b), reducing the effects of sample-bias on population genomic inference (e.g., Battey et al., 2020). Sampling that spans the entire historical range of species allows more accurate estimation of population structure and divergence vs. isolation-by-distance, thereby enabling robust delimitation of species boundaries (Perez et al., 2018). The inclusion of historical specimens may also decrease the impact of “ghost” populations on inference, where failure to sample a population can misrepresent estimates of gene flow and our understanding of introgression (Beerli, 2004; Slatkin, 2005; Hey et al., 2018; Linck et al., 2019). Further, historical genomic data from across space and time increases scope for studies of adaptive evolution and selection (Alves et al., 2019), responses to environmental change (Bi et al., 2013, 2019; Schmitt et al., 2019) and genomic erosion during population decline (Hung et al., 2014; Irestedt et al., 2019; van der Valk et al., 2019; Gauthier et al., 2020; Roycroft et al., 2021b).
Historical museum specimens are also the only source of genetic data for type specimens, and for most rare, elusive or extirpated taxa that are otherwise missing from studies at a phylogenomic or macroevolutionary scale (Ruane and Austin, 2017; McGuire et al., 2018; Wood et al., 2018; Lyra et al., 2020). The inclusion of these specimens mitigates the impact of missing taxa on phylogenetic inference (Streicher et al., 2016), the estimation of speciation and extinction rates (Höhna et al., 2011; Höhna, 2014; Craig et al., 2022) and molecular dating (Linder et al., 2005). Recent studies have also demonstrated how genomic data from extinct taxa can provide unprecedented capacity to resolve long-standing taxonomic uncertainty and reconstruct recent population decline (Grewe et al., 2021; Roycroft et al., 2021b; Pyron et al., 2022). The ability to place extinct or elusive taxa in a phylogenetic and genomic context provides an opportunity to obtain a high-resolution evolutionary reconstruction of all recently extant species, with important implications for conservation biology of persisting species.
While many published studies report successful sequencing results from historical specimens across evolutionary scales, sequencing attempts that result in poor quality or unusable data are typically not reported in scientific literature. Predictors of sequencing success from museum specimens are therefore difficult to assess. Previous studies have suggested that DNA is preserved longer in certain tissue types, e.g., hard tissue like teeth and bone (Adler et al., 2011; Rowe et al., 2011; Burrell et al., 2015; Damgaard et al., 2015; Dabney and Meyer, 2019) and avian toe pads (Tsai et al., 2020) compared to soft tissues like skin. As well as specimen tissue type, decisions during library preparation and bioinformatic processing may also impact final data quality from historical specimens. The consequences of sequencing quality and accuracy on evolutionary inference depend on the research question, and differ between population and phylogenetic studies. For example, erroneous read mapping, variant calling, or missing data may have the most significant impact on the estimation of positive selection in studies of molecular evolution (e.g., Roycroft et al., 2021a), or on fine-scale population genomic parameters. In these cases, studies may focus on ensuring only high-quality and gap-free data are included. In contrast, phylogenomic or macroevolutionary studies may substantially benefit by the inclusion of rare or enigmatic taxa, while tolerating higher levels of missing data. In the latter case, there may be greater emphasis placed on minimizing specimen damage but optimizing sequencing success.
To optimize sequence success and quality at different evolutionary scales, we assessed (1) a phylogenomic dataset of 92 samples from 79 historical museum specimens of Australo-Papuan rodents (family Muridae, tribes Hydromyini and Rattini), and (2) a population genomic dataset from 34 historical skins of four species in the Australian rock-wallaby genus Petrogale (Macropodidae: Marsupialia). Using the rodent data, we assess the effect of tissue subsample type, specimen age and library indexing strategy on sequencing success. Using the rock-wallaby data, we test the impact of bioinformatic processing on data accuracy and estimation of population genomic parameters. Specimen collection years range from 1841 to 1997 and were sourced from six different museums spanning three continents. By integrating results across population and phylogenomic datasets, we highlight how steps from specimen subsampling, library preparation, to post-sequencing bioinformatics can be optimized to increase the usability and accuracy of genome sequence data obtained from historical museum specimens.
Materials and Methods
Sampling
Rodents
We sequenced 92 samples from 79 specimens (63 species) of Australo-Papuan endemic rodents from the subfamily Murinae, including samples from the tribes Hydromyini and Rattini. Most of these species are known only from museum specimens, including seven extinct species, emphasizing the need to use historical museum specimens to ensure comprehensive sampling. Samples were obtained from museum collections in Australia (Museums Victoria, Australian Museum, Western Australian Museum, Australian National Wildlife Collection), Europe (Natural History Museum in London), and America (American Museum of Natural History; Supplementary Table 1). Specimens were collected between 1841 and 1997 and preserved as dry preparations (also known as “study skins”). We sampled either skin (n = 49), toe pad (n = 34) or bone (n = 9) from each specimen. For nine specimens, we collected multiple samples comprising different tissue types. For skins, we sampled ~25 mm2 (5 × 5 mm) from the exposed area of the underbelly, where the preparatory incision had previously been made. For toe pads, we removed ~1 mm2 from a single digit. This subsample size difference was intended to maximize the amount of respective DNA obtained from each sample, as preliminary results indicated toe pad yielded more DNA than skin. The DNA quantity for each sample was later normalized during library preparation. Bone was sampled opportunistically, where the specimen had experienced previous damage resulting in broken/exposed bone that could be sampled without additional consequence to the specimen.
Rock-Wallabies
We sampled 56 museum skins from four species of rock-wallaby from the brachyotis group of the genus Petrogale, and eight reference samples from modern tissues (one from each known lineage; Potter et al., 2014). Historical specimens sampled included P. brachyotis (n = 18); P. burbidgei (n = 3); P. concinna (n = 16) and P. wilkinsi (n = 19). Samples were obtained from Australian museum collections (Australian National Wildlife Collection, Museums Victoria and the Western Australian Museum, Supplementary Table 2). Specimen collection years ranged from 1912 to 1977, and specimens were all preserved as dry study skins. To minimize invasive sampling, we took ~ 5 mm x 5 mm pieces of skin from the ear, or dried skin still attached to skulls.
DNA Extraction
For rodent samples, DNA was extracted following a modified version of a standard phenol-chloroform-isoamyl DNA extraction protocol (Roycroft et al., 2021b, and provided in the Supplementary Material), in the Museums Victoria Ancient DNA facility. For rock-wallaby samples, DNA was extracted using the DNeasy Blood and Tissue Kit (Qiagen GmbH, Hilden, Germany) using aerosol barrier pipette tips, with working surfaces and equipment wiped down with Lookout DNA Erase (Sigma-Aldrich) before each use. Extractions were undertaken in a dedicated trace DNA laboratory at the Australian National University.
Library Preparation, Hybridisation, and Sequencing
Both the rodent and rock-wallaby datasets were obtained through exon capture target enrichment. All sample libraries were prepared using (Meyer and Kircher, 2010) protocol, including modifications made by Bi et al. (2013). For rodent samples, we used a murine-specific custom exon capture design (SeqCap EZ Developer Library; Roche NimbleGen), targeting 1.27 Mb of genomic DNA (1417 exons, see Roycroft et al., 2020). Rodent samples were either indexed with a single unique barcode, or with a dual-indexing approach, and pooled across multiple captures with up to 92 samples at equimolar ratios (1.2 μg total). Dual-indexed samples were barcoded with a combination of one of 96 unique p5 index sequences, and one of 24 unique p7 index sequences. For rock-wallaby samples, we used a Petrogale-specific custom exon capture approach (SeqCap EZ Developer Library; Roche NimbleGen), which targets 1.83 Mb of genomic DNA (3960 exons), designed using transcriptome data from a yellow-footed rock-wallaby (Petrogale xanthopus) (see Bragg et al., 2016; Potter et al., 2017, 2022). Rock-wallaby samples were indexed with a single unique barcode, and all 56 samples were pooled at equimolar ratios (1.2 μg total).
For both datasets, pooled libraries were then hybridized for ~72 h, with 5 μg of mouse Cot-1 DNA (Life Technologies Corporation), barcode specific blocking oligos (1000 pmol) and target probes following the SeqCap EZ Developer Library protocol. Post incubation, the hybridization reaction was amplified in two independent enrichment PCRs and then cleaned up using the QIAquick PCR purification kit (Qiagen). Quality control checks were made using the DyNAmo Flash SYBR green qPCR kit (Thermo Fisher Scientific Inc.; see Bi et al., 2012) to assess global enrichment of the target exons by comparing pre-capture pooled genomic libraries to the post-capture cleaned hybridization reaction and specifically designed to hit targets of the hybridization probes. After passing these quality control checks, the enriched hybridization samples were run on a BioAnalyzer (2100; Agilent Technologies, Inc.) to check the quality and quantity of the libraries prior to sequencing. Each pooled library was then sequenced on a single lane of an Illumina HiSeq 2500 (100 bp paired-end run) at the ACRF Biomolecular Resource Facility.
Sample Processing and Bioinformatics
We processed raw sequencing data from all specimens using Exon Capture Pipeline for Phylogenetics (ECPP, https://github.com/Victaphanta/ECPP), following the protocol described in Roycroft et al. (2020). For a subset of rodent samples, we ran mapDamage2 (Jónsson et al., 2013) to assess the extent of DNA misincorporation. For rock-wallaby samples, reflecting population genetic sampling, we compared the effect of mapping to a sample-specific reference versus mapping to the highest-quality assembly from the closest non-historical sister sample. Initially, we implemented the sample-specific reference approach which creates a de novo assembly for each historical sample (the “historical de novo” dataset). This is the default approach in ECPP, and in other commonly used target capture assembly pipelines (e.g., Bragg et al., 2015; Faircloth, 2016; Singhal et al., 2017). These historical de novo assemblies were used to create sample-specific references, and raw reads were then mapped back to each reference using BBmap (version 35.82, sourceforge.net/projects/bbmap/) with a minid threshold of 0.95. As a comparison, we also used a high-quality “closest sister” reference approach to map reads (see Roycroft et al., 2021b). To do this, we generated a reference set of high-quality de novo assemblies from fresh tissue samples of various Petrogale sub-species. Using the same mapping approach as above, we mapped the raw reads from historical samples to the closest sister sample (i.e., lowest evolutionary distance from each historical population) with a high-quality assembly (the “high-quality de novo” dataset). The sample with the lowest evolution distance was determined based on divergence between the sample and the reference in substitutions per site, calculated in IQ-TREE 1.6.9 (Nguyen et al., 2015). In all cases, reads from historical samples were mapped to a high-quality de novo assembly of the same sub-species. For all rodent specimens, we only applied this “high-quality de novo” mapping approach, as preliminary results showed this had superior performance over the default method. Final alignments for all data were filtered at a threshold of 3% heterozygosity per locus, and processed with BMGE (Criscuolo and Gribaldo, 2010) to remove poorly represented regions.
Summary Statistics and Branch Length Estimation
To estimate population genomic summary statistics, we filtered the rock-wallaby dataset to 3742 loci that were >90% sample-complete and split samples into seven populations (Petrogale brachyotis brachyotis; BB, Petrogale brachyotis victoriae; BV, Petrogale concinna canescens; CC, Petrogale concinna monastria; CM, Petrogale wilkinsi core population; W, Petrogale wilkinsi Gulf of Carpentaria population; wGU, and Petrogale wilkinsi Groote Eylandt population; wGR). A total of 22 originally sequenced rock-wallaby samples were excluded due to insufficient coverage and poor data quality (see Supplementary Table 2). For each population, we calculated the number of segregating sites and proportion of segregating sites to valid sites (to account for missing data) in PopGenome in R (Pfeifer et al., 2014). We also estimated Watterson's theta (θ, Watterson, 1975) and Tajima's D (Tajima, 1989) in PopGenome, as these are common metrics used to assess genetic diversity and population dynamics. We repeated all calculations for both the “historical de novo” and “high-quality de novo” datasets, across all loci. We also performed all calculations using only exons which matched between the two Petrogale datasets, to directly compare the effect of mapping strategy on parameter estimation. We tested for significant differences across datasets using Welch's two-sample t-test. As a further comparison, we used IQ-TREE 1.6.9 (Nguyen et al., 2015) with codon partitions to infer terminal branch lengths (in substitutions per site) for both the “historical de novo” and “high-quality de novo” datasets. Accurate estimation of tip branch lengths are important, as they are increasingly used as metrics for speciation rates (e.g., ClaDS, Maliet et al., 2019) and in analyses of variation in rates of molecular evolution (e.g., Ivan et al., 2022).
Using the final processed rodent phylogenomic data, we calculated the proportion of reads on target (i.e., the total proportion of deduplicated sequenced reads that mapped to the target region) and the proportion of total target loci successfully captured (>40% of target region) for all specimens. For each sample, we also calculated the average heterozygosity across all loci as a measure of sequence quality and accuracy, where outliers with high values are assumed to contain a higher rate of error. We then compared these metrics across sampled tissue type (toe pad or bone vs. skin) and library indexing strategy (single vs. dual-indexed). Toe pad and bone samples were grouped, due to the comparatively high success rate among these two tissue types and the low overall number of samples from bone. Using the stats package in R, we applied generalized linear models (GLM) to model two categorical predictors (indexing strategy and tissue type) and a continuous predictor (specimen age) on four continuous response variables; proportion of reads on target, loci captured, average coverage and heterozygosity. We also used a two-sample t-test to test each of these variables for significant differences in response to indexing strategy and tissue type.
Results
Predictors of Capture Efficacy and Sequence Quality in Phylogenomic Data
Across all rodent genomic libraries sequenced, 91% (31 out of 34) toe pad and 89% (8 out of 9) bone subsamples resulted in useable sequence data, compared to 63% (31 out of 49) of skin samples (Supplementary Table 1). Unusable samples were those that either returned no sequence data after processing in ECPP, or where data was returned, were primarily sequencing contaminants. We took a conservative approach to screening for contaminant samples, by excluding all samples that showed a terminal branch length at least ~20% greater than close relatives sequenced using high-quality DNA. Results from mapDamage2 suggested that the effect of DNA damage was relatively minor, but was more evident in subsamples taken from the skin, compared to bone or toe pad of the sample specimen (see Supplementary Figure 1 for an example).
The proportion of reads on target (Figure 1A) was significantly lower for single-indexed samples than for dual-indexed samples, while the difference between the number of loci captured (Figure 1B) or average coverage (Figure 1C) was not significant (Table 1). Generalized linear models (GLM) found that collection year was a significant predictor (p < 0.05) for the proportion of reads on target (Supplementary Table 3). Proportion of reads on target tended to be higher for samples that were collected more recently, especially for skin subsamples (Figure 1E). Our GLMs also indicated indexing approach was a significant predictor (p < 0.01) of heterozygosity (Supplementary Table 3), with average heterozygosity across loci (Figure 1D) significantly higher for single-indexed samples than for dual-indexed samples (Table 1). Interactions between collection year, tissue type, and indexing strategy also had significant effect on heterozygosity (Supplementary Table 3).
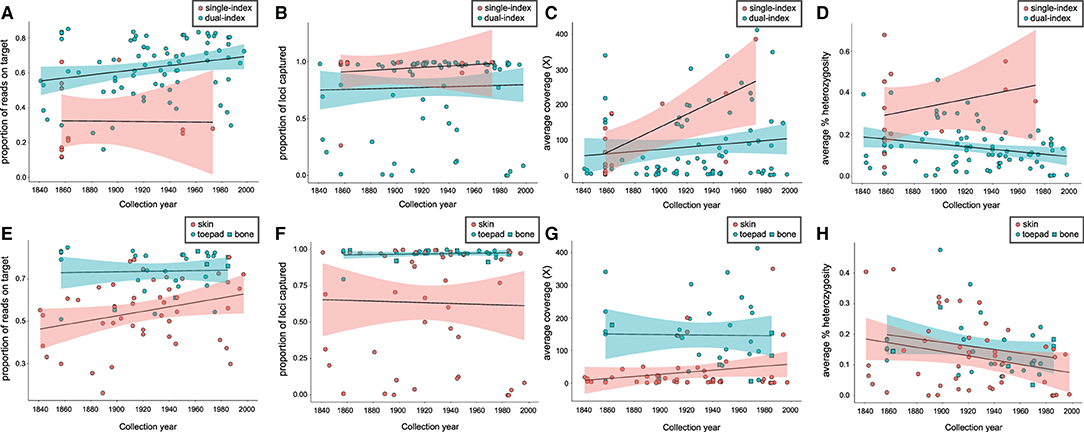
Figure 1. Relationship between year of specimen collection and indexing strategy for (A) proportion of reads on target, (B) proportion of targeted loci captured, (C) average coverage, (D) average percent heterozygosity, and for dual-indexed samples only; between year of specimen collection and tissue type for (E) proportion of reads on target, (F) proportion of targeted loci captured, (G) average coverage, (H) average percent heterozygosity.
When dual-indexed samples were grouped by source tissue type, the average coverage, reads on target and loci captured were all significantly higher in toe pad/bone subsamples compared to skin subsamples (Table 2). There was no significant difference in average heterozygosity when comparing tissue types. There was a weak relationship between specimen age and reads on target (Figure 1E) for skin subsamples (r = 0.29, p < 0.05), and no relationship for toe pad/bone subsamples (r = 0.037, p = 0.84). There was no relationship between specimen age and the proportion of loci captured (Figure 1F) for either skin or toe pad/bone subsamples.
The Effect of Mapping Strategy on Population Genomic Parameters
To assess the potential impact of mapping strategy on downstream population genomic inference, we compared inferred population parameters between the “historical de novo” and “high-quality de novo” datasets for Petrogale rock-wallabies. A total of 34 out of 56 rock-wallaby skins (61%) yielded sufficient data for use in population genomic analyses. The proportion of segregating sites to valid sites was always higher for the historical de novo approach, suggesting a higher error rate (Table 3). In five out of seven populations, the “historical de novo” mapping approach resulted in a greater absolute number of apparent variable sites (up to 10% more per population) than the “high-quality de novo” approach. In both cases where the “high-quality de novo” dataset had a greater absolute number of variable sites, this was explained by a 38% (CM) and 77% (wGU) increase, respectively, in total number of recovered sites when using the “high-quality de novo” mapping approach compared to the “historical de novo” approach. In five out of seven populations, the “high-quality de novo” mapping approach also resulted in fewer missing sites than the “historical de novo” approach.
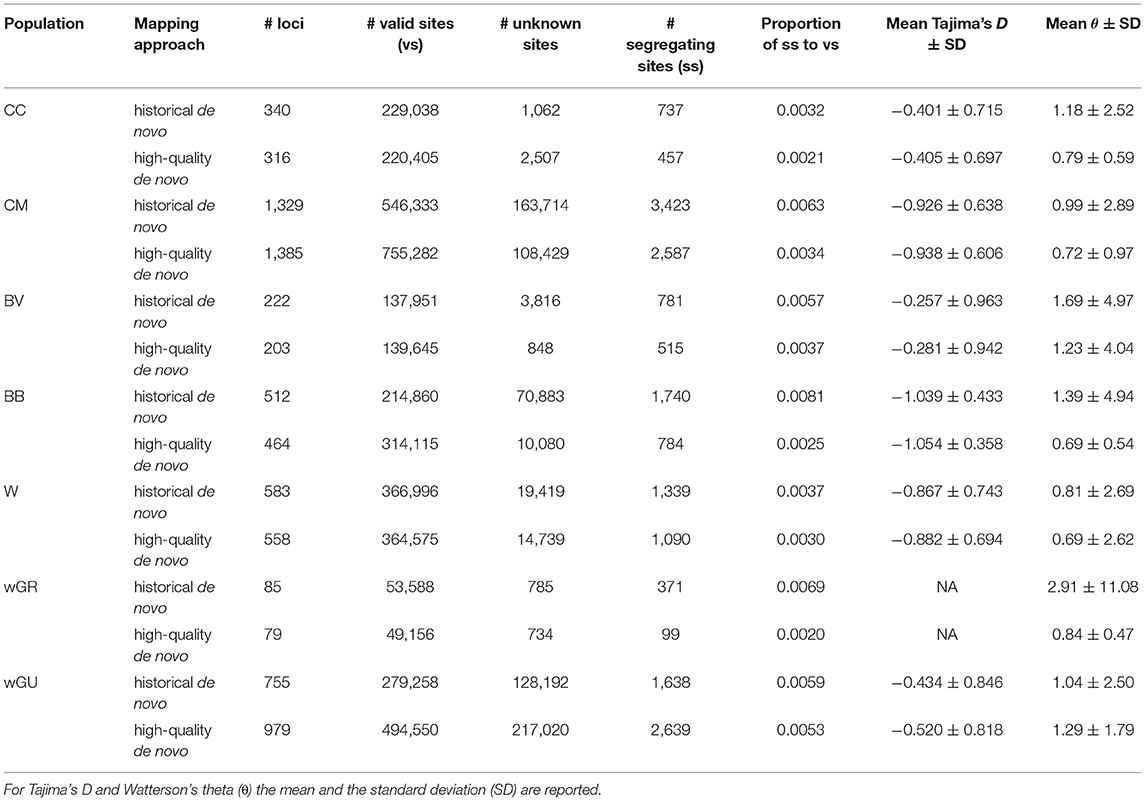
Table 3. Population genomic summary statistics across rock-wallaby populations for both “historical de novo” and “high-quality de novo” mapping approaches.
In all cases except for the wGU population, the inferred number of segregating sites and the estimated θ values were consistently higher in the “historical de novo” dataset compared to the “high-quality de novo” dataset (Figure 2A and Table 3). The impact of mapping strategy resulted in a significant difference (p < 0.05) in the number of segregating sites for the CC, CM, BB and wGU populations using a two-sample t-test (Figure 2A). However, the differences were not significant when only overlapping loci were considered (Supplementary Table 4). We also recovered a significant difference in θ estimates for CC, CM, BB and wGU (Figure 2B and Table 3), and for CC, CM and BB in overlapping loci (Supplementary Table 4). The proportion of segregating sites to valid sites was smaller for the “high-quality de novo” dataset compared to the “historical de novo” dataset across populations, with a significant difference in the CC, CM, BB, WGR populations (Table 3), and only for CM in overlapping loci (Supplementary Table 4). While estimated mean Tajima's D values were consistently higher for the “high-quality de novo” dataset compared to the “historical de novo” dataset, the difference was only significant in the wGU population (Table 3).
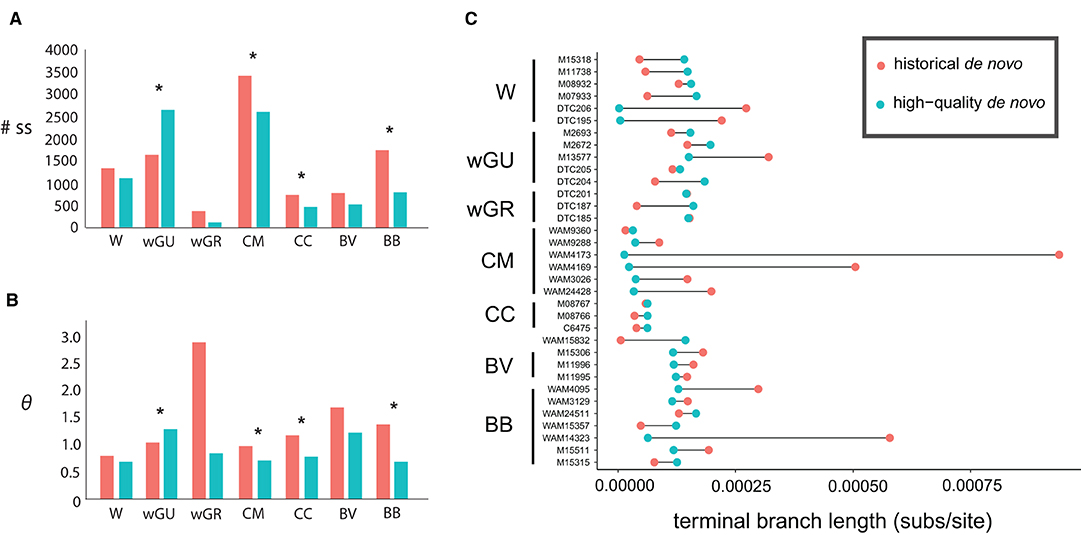
Figure 2. Comparison between the “historical de novo” (pink) and “high-quality de novo” (blue) datasets for (A) total number of segregating sites, and (B) average Watterson's theta (θ) estimates, and (C) terminal branch length (substitutions per site). Samples are identified by the voucher number and grouped by population; W, wilkinsi; wGU, wilkinsi Gulf of Carpentaria; wGR, wilkinsi Groote Eylandt; CM, concinna monastria; CC, concinna canescens; BV, brachyotis victoriae; BB, brachyotis brachyotis. Significant differences between the “historical de novo” and “high-quality de novo” datasets for each population are designated by * in (A,B).
The difference in terminal branch length for Petrogale samples in the “historical de novo” and “high-quality de novo” datasets was variable, but with all large differences having over-inflated branch length in the “historical de novo” dataset. This was especially evident for individuals within the CM and BB populations (Figure 2C). The impact of these individuals is also reflected in population-level significant differences in summary statistics (Table 3 and Figure 2). Overall, samples with comparatively lower quality (i.e., lower coverage, higher heterozygosity in historical de novo dataset) tended to show the most reduction in terminal branch length when using the “high-quality de novo” reference compared to the “historical de novo” reference. Higher quality (i.e., higher coverage, fewer errors in historical de novo dataset) samples tend to show slightly longer terminal branch length using the “high-quality de novo” reference compared to the “historical de novo” reference.
Discussion
Using population and phylogenomic data generated from historical museum specimens, we demonstrate that choices prior to DNA extraction (i.e., type of tissue subsampled), during library preparation (i.e., indexing) and post-sequencing bioinformatic processing (i.e., mapping) have significant impacts on the success, usability, and quality of genomic sequence data and inference. Further, we show how the use of a high-quality reference assembly for mapping reads from historical specimens can result in significant differences in the amount of final data recovered, inferred population genomic summary statistics and phylogenetic tip lengths compared to a de novo sample-specific approach. This demonstrates the importance of the availability of high-quality reference assemblies from closely-related taxa, especially for studies including sequence data from historical specimens where maximum data recovery and accurate variant calling are crucial.
In synthesizing our results, we provide a framework for optimizing pre- and post-sequencing protocols for irreplaceable historical dried mammal specimens at both population and phylogenomic scales.
Pre-sequencing Predictors of Data Quality From Historical Specimens
Across rodent specimens, DNA extracted from subsamples of toe pad or bone consistently performed better than skins in terms of sequencing success, capture specificity (proportion of sequence reads on target), data quality and accuracy (heterozygosity), and completeness (final number of loci captured). This is consistent with previous studies of avian toe pads and bone compared to skin (Tsai et al., 2020), and from ancient DNA studies that have found harder tissues like bones and teeth to preserve DNA for longer than soft tissues (Adler et al., 2011; Burrell et al., 2015; Damgaard et al., 2015; Dabney and Meyer, 2019). Notably however, we found no effect of specimen age on the proportion of reads on target or number of loci captured for toe pad or bone subsamples, indicating a high level of protection from post-mortem DNA damage and degradation in these tissue types. This is in contrast with results from McCormack et al. (2016), who found a decrease in total assembled sequence data for avian specimens with age, but appears to be consistent with results from Sawyer et al. (2012), who found minimal effect of DNA fragmentation across time in specimens up to 60,000 years. In our data, rodent toe pads and bone also yield high-quality endogenous DNA with no observable relationship to specimen age, demonstrating the feasibility of obtaining reliably high-quality genomic sequence data from specimens spanning the last three centuries.
In contrast, DNA sequence libraries prepared from skin subsamples had a significantly lower rate of sequencing success (63% for skins, compared to 93% in toe pad/bone), and a weak but significant relationship (r = 0.29, p < 0.05) between specimen age and sequenced reads on target (Figure 1E). Where DNA is more fragmented and has a greater degree of post-mortem damage (e.g., Supplementary Figure 1), overall capture efficiency is likely to be lower. This would explain the difference in loci captured for skin subsamples compared to toe pad and bone (Figure 1F). If capture efficiency is lower in these samples, then the relative amplification of off-target DNA in the post-capture PCR is likely to be greater. In turn, this may explain the effect of tissue type and specimen age we observed for skin subsamples for proportion of reads on target (Figure 1E). Previous studies (Pääbo et al., 2004) have suggested a relationship between specimen age and DNA quality, as well as a decrease in endogenous DNA via degradation and an increase in exogenous DNA via contamination over time. However, recent studies suggest that the specimen preservation and storage may be crucial factors for collections-age material (McCormack et al., 2016; McDonough et al., 2018). In our data, DNA degradation with specimen age was only evident for skin subsamples, and not for toe pad and bone. As the skins of prepared museum specimens are thinner and more exposed to the environment than toe pad or bone, DNA content and quality in these tissues is likely dependent on the conditions of specimen storage, superficial treatment of the skin with chemicals (e.g., arsenic), and handling of the specimen.
We also found a significant difference between proportion of reads on target and heterozygosity in single- vs. dual-indexed samples, with reads on target being lower and heterozygosity higher in single-indexed samples. Higher average heterozygosity in single-indexed samples may be explained by a combination of cross-index contamination during genomic library preparation, and background cross-indexing during sequencing. Both types of contamination can be reduced by using unique (or partially unique) dual indexes (Kircher et al., 2012). Interestingly, we also report overall fewer reads on target for single-indexed samples. This may be explained by contaminant libraries using the same index from the laboratory environment, resulting in an apparent lower overall capture efficiency. In this case, dual-indexing also reduces the likelihood that any cross-library contamination contains a matching pair of indexes, especially where effort is made to alternate between combinations of indexes across experiments. However, we note that the overall number of single-indexed libraries in our data was low compared to the number dual-indexed libraries, and so it is possible that the patterns we observe are an artifact of sample library variability.
Practical Guidelines for Specimen Selection and Subsampling
While historically preserved DNA has the potential to be highly valuable, this is in addition to the existing intrinsic taxonomic, morphological and historical significance of specimens. Destructive sampling may interfere with potential diagnostic characters, which are often taxonomic group specific (e.g., ear length and shape, nose leaf morphology, toe pad morphology and number, etc.). Further, museum specimens are finite and irreplaceable sources of genomic material, especially for specimens of rare or extinct taxa. As such, it is critical to follow minimally invasive procedures when subsampling material from historical specimens, as well as ensuring optimal genomic library preparation and bioinformatic post-processing decisions to maximize data accuracy and utility. For dry museum skins of small mammals like rodents, subsamples of skin from around the preparatory incision may be the least invasive, however our results suggest that DNA quality and sequencing success from such subsamples is variable, and as such there is an increased chance that DNA extraction and sequencing from these subsamples will fail. Sampling from harder tissue types like toe pad or bone is therefore more likely to result in high-quality genomic data. Where practical considerations warrant subsampling from skin in the first instance, our results show that library preparation using a dual-indexing, rather than single-indexing, may minimize contamination and maximize the chance of obtaining useable data. Recent advances in sequencing genomic DNA from formalin-fixed specimens (e.g., Hykin et al., 2015; Ruane and Austin, 2017), and historical ethanol-preserved specimens (e.g., Derkarabetian et al., 2019) may also present viable options for sampling as an alternative to skins, although with variable success.
Post-sequencing Optimisation of Historical Sequence Data
Using population genomic data from Petrogale rock-wallabies, we demonstrate that increased reference quality can have substantial impact on population genomic parameters and terminal branch length estimation. Previous studies have also demonstrated the impact of reference choice, for example Shafer et al. (2017) found that a reference-based approach recovered lower inbreeding coefficient (FIS) values than a de novo approach for RAD-seq data. In our case, we hypothesize that for historical samples, mapping to a sample-specific de novo assembly can reinforce error that is present at low levels in the historical sequence data (e.g., due to DNA damage or sequencing error). Our results show that the use of a high-quality de novo reference can both reduce error and increase data completeness.
At an individual level, we found that samples with the overall lowest quality tended to show the most significant reduction in terminal branch length when using the “high-quality de novo” reference compared to the “historical de novo” reference. For cases where historical samples were comparatively high quality from the outset, terminal branch length tended to be slightly longer using the “high-quality de novo” reference compared to the “historical de novo” reference. This was due to an increase in legitimate variable sites when using a more complete and contiguous reference for mapping historical reads. While the “high-quality de novo” mapping approach is likely to have the most impact on samples of lower initial sequence quality, total population sample size may also be a contributing factor. For example, although the overall inferred terminal branch length differences were relatively small for samples within the CC population (Figure 2C), the alternative mapping approaches resulted in significant differences in population genomic summary statistics (Table 3). For populations with lower sample sizes, small changes in allele frequencies may have greater relative effect on estimated summary statistics (e.g., Fumagalli, 2013).
When summary statistics were inferred at a population-level, we saw a significant impact on the inferred number of segregating sites and Watterson's θ estimates in four of the seven populations. This was despite most populations containing individuals with higher-quality sequence data (see Figure 2C), which may be expected to mask the impact of low-frequency errors. In the CM and BB populations, pronounced differences in terminal branch length of individuals correspond to significant differences in inferred summary statistics. However, populations with significant differences in inferred summary statistics at a population level did not always show obvious differences in terminal branch length (e.g., the CC population). In cases where sequence quality is reduced pervasively across individuals in a population, errors introduced by DNA damage, sequencing error or bioinformatic processing are likely to have greater consequences. This may then impact the accuracy of downstream inference of genetic diversity, population structure, population size and demographic processes.
The Importance of Data Accuracy for Population Genomic Inference From Historical Specimens
The inclusion of historical museum specimens in population genomics provides the opportunity to sample extirpated populations, potentially contributing to the delimitation of species boundaries and conservation units, assessment of extinction risk and studies of population decline (e.g., Mondol et al., 2013; Nakahama and Isagi, 2018; Nakahama, 2021). Optimizing sequence quality from historical specimens is crucial in empirical systems like Petrogale, where complex patterns of mito-nuclear discordance (Potter et al., 2012, 2014), introgression (Potter et al., 2015, 2017, 2022), and incomplete lineage sorting across the landscape can only be resolved with comprehensive geographic sampling. In addition, data quality and completeness are especially important in studies using targeted exon capture approaches for population genomics (e.g., Bi et al., 2012; Belkadi et al., 2016; Potter et al., 2016), where there are often limited segregating sites within exonic loci. In such cases, a decrease in data completeness can reduce power to detect genuine population level variation, but equally, the impact of erroneous variant calling can be more severe.
It has long been recognized that variation in data quality, accuracy and completeness can have considerable impact on inference and conclusions in population genomic studies. The allele frequency spectrum, a summary of the distribution of derived allele frequencies, is commonly used in population genomic inference. Estimated allele frequencies can be highly sensitive to bioinformatic approaches, potentially impacting estimates of demographic expansion and isolation-with-migration models (Shafer et al., 2017). Many analytical approaches use allele frequency estimates to determine population structure (e.g., STRUCTURE, Pritchard et al., 2000), gene flow (e.g., DILS, Fraïsse et al., 2021; ABBA-BABA tests, Durand et al., 2011; TreeMix, Pickrell and Pritchard, 2012), and demographic history (e.g., δaδi, Gutenkunst et al., 2009; range expansion tests, Peter and Slatkin, 2013, 2015). Inflation of the number of variable sites, as reported in our results, could have profound influence if skewed to increase the number of minor alleles in a population, influencing patterns of demographic expansion, and evaluation of selection and adaptation, common population genomic analyses where museum specimens have been incorporated (e.g., Bi et al., 2013; Ewart et al., 2019; Dussex et al., 2021). Low frequency variants, or minor alleles, can significantly influence population structure (Linck and Battey, 2019) and estimates of demographic history (e.g., Shafer et al., 2017).
Our results showing the effect of de novo assembly quality on population genomic summary statistics demonstrate the importance of maximizing the quality and contiguity of the mapping reference and highlight the complexities in bioinformatic processing and analyzing data from historical museum specimens. This is especially true in contexts where accuracy is crucial. While sample-specific de novo assemblies have been routinely used in many target capture bioinformatic pipelines (e.g., Bragg et al., 2015; Faircloth, 2016; Singhal et al., 2017) to mitigate against reference bias (Sousa and Hey, 2013), we caution against a true “sample-specific” approach for historical specimens. While some historical specimens can provide high-quality de novo assemblies, these are typically not as contiguous as de novo assembly obtained from fresh tissue. Where fresh tissues are available from the same or closely related species, studies should endeavor to generate “high-quality de novo” assemblies from close relatives as a reference prior to sampling historical specimens. For population level studies, bias may be further reduced by selecting loci at random from multiple fresh specimens per lineage (e.g., Potter et al., 2016), or data recovery increased by mapping individuals to a common and highly complete reference for each population (e.g., Potter et al., 2018). The application of iterative mapping approaches (e.g., “pseudoreferencing,” Sarver et al., 2017) may also serve to further reduce bias where raw data is mapped to a divergent reference. It is likely that the consequence of “reference bias,” even at moderate evolutionary divergences (e.g., above population level to 10 million years), is less than the consequence of potential error and loss of data introduced using a de novo assembly generated from historical sequencing reads, however further studies are needed to quantify the impact of evolutionary divergence. Reference genomes for diverse taxa are also now being generated by the research community at a rapid rate, providing an additional source for mapping reads from historical specimens in future work.
Data Availability Statement
The data presented in this study are deposited in the NCBI sequence read archive (SRA) at BioProject Accession PRJNA846960.
Author Contributions
ER, SP, and CM conceived and designed the study. ER, SP, ME, RPM, and KR contributed to specimen sampling. ER, SP, KR, and MP performed laboratory work. AM provided bioinformatic tools. ER and SP analyzed the data and wrote the manuscript. All authors edited and approved the final manuscript.
Funding
This project was supported by funding from Bioplatforms Australia through the Australian Government National Collaborative Research Infrastructure Strategy, as part of the Oz Mammals Genomics Initiative. This project was also supported by funding (to ER) from the Holsworth Wildlife Research Endowment (Equity Trustees Charitable Foundation and the Ecological Society of Australia), Dame Margaret Blackwood Soroptimist Scholarship, the Museums Victoria 1854 Scholarship, and to SP and MP from a Center for Biodiversity Analysis Ignition Grant. MP was a recipient of a Discovery Early Career Research Award from the Australian Research Council (DE130100777).
Conflict of Interest
The authors declare that the research was conducted in the absence of any commercial or financial relationships that could be construed as a potential conflict of interest.
Publisher's Note
All claims expressed in this article are solely those of the authors and do not necessarily represent those of their affiliated organizations, or those of the publisher, the editors and the reviewers. Any product that may be evaluated in this article, or claim that may be made by its manufacturer, is not guaranteed or endorsed by the publisher.
Acknowledgments
The authors wish to acknowledge the Traditional Owners and Custodians of the lands on which we work. We recognize the past atrocities against First Peoples of Australia, who have never ceded sovereignty. We acknowledge the contribution of the Oz Mammals Genomics Initiative consortium in the generation of data used in this publication (https://ozmammalsgenomics.com/consortium/). We thank Niccy Aitken and Anna MacDonald for advice with laboratory protocols. We are also indebted to collections staff and to many individuals who contributed material at the Australian Museum, Museums Victoria, Western Australian Museum, Australian National Wildlife Collection (https://ror.org/059mabc80), American Museum of Natural History and Natural History Museum in London. We thank Pierre-Henri Fabre for assistance in sampling specimens from London.
Supplementary Material
The Supplementary Material for this article can be found online at: https://www.frontiersin.org/articles/10.3389/fevo.2022.931644/full#supplementary-material
References
Adler, C. J., Haak, W., Donlon, D., and Cooper, A. (2011). Survival and recovery of DNA from ancient teeth and bones. J. Archaeol. Sci. 38, 956–964. doi: 10.1016/j.jas.2010.11.010
Alves, J. M., Carneiro, M., Cheng, J. Y., de Matos, A. L., Rahman, M. M., Loog, L., et al. (2019). Parallel adaptation of rabbit populations to myxoma virus. Science 363, 1319–1326. doi: 10.1126/science.aau7285
Battey, C. J., Ralph, P. L., and Kern, A. D. (2020). Space is the place: Effects of continuous spatial structure on analysis of population genetic data. Genetics 215, 193–214. doi: 10.1534/genetics.120.303143
Beerli, P. (2004). Effect of unsampled populations on the estimation of population sizes and migration rates between sampled populations. Mol. Ecol. 13, 827–836. doi: 10.1111/j.1365-294X.2004.02101.x
Belkadi, A., Pedergnana, V., Cobat, A., Itan, Y., Vincent, Q. B., Abhyankar, A., et al. (2016). Whole-exome sequencing to analyze population structure, parental inbreeding, and familial linkage. Proc. Natl. Acad. Sci. U.S.A. 113, 6713–6718. doi: 10.1073/pnas.1606460113
Besnard, G., Bertrand, J. A. M., Delahaie, B., Bourgeois, Y. X. C., Lhuillier, E., and Thébaud, C. (2016). Valuing museum specimens: High-throughput DNA sequencing on historical collections of New Guinea crowned pigeons (Goura). Biol. J. Linn. Soc. 117, 71–82. doi: 10.1111/bij.12494
Bi, K., Linderoth, T., Singhal, S., Vanderpool, D., Patton, J. L., Nielsen, R., et al. (2019). Temporal genomic contrasts reveal heterogeneous evolutionary responses within and among montane chipmunk species during recent climate change. PLoS Genet. 15, e1008119. doi: 10.1371/journal.pgen.1008119
Bi, K., Linderoth, T., Vanderpool, D., Good, J. M., Nielsen, R., and Moritz, C. (2013). Unlocking the vault: Next-generation museum population genomics. Mol. Ecol. 22, 6018–6032. doi: 10.1111/mec.12516
Bi, K., Vanderpool, D., Singhal, S., Linderoth, T., Moritz, C., and Good, J. M. (2012). Transcriptome-based exon capture enables highly cost-effective comparative genomic data collection at moderate evolutionary scales. BMC Genomics 13:403. doi: 10.1186/1471-2164-13-403
Bragg, J. G., Potter, S., Bi, K., Catullo, R., Donnellan, S. C., Eldridge, M. D. B., et al. (2016). Resources for phylogenomic analyses of Australian terrestrial vertebrates. Mol. Ecol. Resour. 17, 869–876. doi: 10.1111/1755-0998.12633
Bragg, J. G., Potter, S., Bi, K., and Moritz, C. (2015). Exon capture phylogenomics: efficacy across scales of divergence. Mol. Ecol. Resour. 16, 1059–1068. doi: 10.1111/1755-0998.12449
Burrell, A. S., Disotell, T. R., and Bergey, C. M. (2015). The use of museum specimens with high-throughput DNA sequencers. J. Hum. Evol. 79, 35–44. doi: 10.1016/j.jhevol.2014.10.015
Craig, J. M., Kumar, S., and Hedges, S. B. (2022). Limitations of phylogenomic data can drive inferred speciation rate shifts. Mol. Biol. Evol. 39, 1–11. doi: 10.1093/molbev/msac038
Criscuolo, A., and Gribaldo, S. (2010). BMGE (Block Mapping and Gathering with Entropy): A new software for selection of phylogenetic informative regions from multiple sequence alignments. BMC Evol. Biol. 10:210. doi: 10.1186/1471-2148-10-210
Dabney, J., and Meyer, M. (2019). Extraction of highly degraded DNA from ancient bones and teeth. Methods Mol. Biol. 1963, 25–29. doi: 10.1007/978-1-4939-9176-1_4
Damgaard, P. B., Margaryan, A., Schroeder, H., Orlando, L., Willerslev, E., and Allentoft, M. E. (2015). Improving access to endogenous DNA in ancient bones and teeth. Sci. Rep. 5, 1–12. doi: 10.1038/srep11184
Derkarabetian, S., Benavides, L. R., and Giribet, G. (2019). Sequence capture phylogenomics of historical ethanol-preserved museum specimens: Unlocking the rest of the vault. Mol. Ecol. Resour. 19, 1531–1544. doi: 10.1111/1755-0998.13072
Durand, E. Y., Patterson, N., Reich, D., and Slatkin, M. (2011). Testing for ancient admixture between closely related populations. Mol. Biol. Evol. 28, 2239–2252. doi: 10.1093/molbev/msr048
Dussex, N., van der Valk, T., Morales, H. E., Wheat, C. W., Díez-del-Molino, D., von Seth, J., et al. (2021). Population genomics of the critically endangered kākāpo. Cell Genomics 1:100002. doi: 10.1016/j.xgen.2021.100002
Ewart, K. M., Johnson, R. N., Ogden, R., Joseph, L., Frankham, G. J., and Lo, N. (2019). Museum specimens provide reliable SNP data for population genomic analysis of a widely distributed but threatened cockatoo species. Mol. Ecol. Resour. 19, 1578–1592. doi: 10.1111/1755-0998.13082
Faircloth, B. C. (2016). PHYLUCE is a software package for the analysis of conserved genomic loci. Bioinformatics 32, 786–788. doi: 10.1093/bioinformatics/btv646
Fraïsse, C., Popovic, I., Mazoyer, C., Spataro, B., Delmotte, S., Romiguier, J., et al. (2021). DILS: Demographic inferences with linked selection by using ABC. Mol. Ecol. Resour. 21, 2629–2644. doi: 10.1111/1755-0998.13323
Fumagalli, M. (2013). Assessing the effect of sequencing depth and sample size in population genetics inferences. PLoS ONE 8, 14–17. doi: 10.1371/journal.pone.0079667
Gauthier, J., Pajkovic, M., Neuenschwander, S., Kaila, L., Schmid, S., Orlando, L., et al. (2020). Museomics identifies genetic erosion in two butterfly species across the 20th century in Finland. Mol. Ecol. Resour. 20, 1191–1205. doi: 10.1111/1755-0998.13167
Grewe, F., Kronforst, M. R., Pierce, N. E., and Moreau, C. S. (2021). Museum genomics reveals the Xerces blue butterfly (Glaucopsyche xerces) was a distinct species driven to extinction. Biol. Lett. 17:e0123. doi: 10.1098/rsbl.2021.0123
Guschanski, K., Krause, J., Sawyer, S., Valente, L. M., Bailey, S., Finstermeier, K., et al. (2013). Next-generation museomics disentangles one of the largest primate radiations. Syst. Biol. 62, 539–554. doi: 10.1093/sysbio/syt018
Gutenkunst, R. N., Hernandez, R. D., Williamson, S. H., and Bustamante, C. D. (2009). Inferring the joint demographic history of multiple populations from multidimensional SNP frequency data. PLoS Genet. 5, e100695. doi: 10.1371/journal.pgen.1000695
Hey, J., Chung, Y., Sethuraman, A., Lachance, J., Tishkoff, S., Sousa, V. C., et al. (2018). Phylogeny estimation by integration over isolation with migration models. Mol. Biol. Evol. 35, 2805–2818. doi: 10.1093/molbev/msy162
Höhna, S. (2014). Likelihood inference of non-constant diversification rates with incomplete taxon sampling. PLoS ONE 9, e84184. doi: 10.1371/journal.pone.0084184
Höhna, S., Stadler, T., Ronquist, F., and Britton, T. (2011). Inferring speciation and extinction rates under different sampling schemes. Mol. Biol. Evol. 28, 2577–2589. doi: 10.1093/molbev/msr095
Hung, C. M., Shaner, P. J. L., Zink, R. M., Liu, W. C., Chu, T. C., Huang, W. S., et al. (2014). Drastic population fluctuations explain the rapid extinction of the passenger pigeon. Proc. Natl. Acad. Sci. U.S.A. 111, 10636–10641. doi: 10.1073/pnas.1401526111
Hykin, S. M., Bi, K., and McGuire, J. A. (2015). Fixing formalin: A method to recover genomic-scale DNA sequence data from formalin-fixed museum specimens using high-throughput sequencing. PLoS ONE 10, e141579. doi: 10.1371/journal.pone.0141579
Irestedt, M., Ericson, P. G. P., Johansson, U. S., Oliver, P., Joseph, L., and Blom, M. P. K. (2019). No signs of genetic erosion in a 19th century genome of the extinct Paradise Parrot (Psephotellus pulcherrimus). Diversity 11:40058. doi: 10.3390/d11040058
Ivan, J., Moritz, C., Potter, S., Bragg, J., Turakulov, R., and Hua, X. (2022). Temperature predicts the rate of molecular evolution in Australian Eugongylinae skinks. Evolution 76, 252–261. doi: 10.1111/evo.14342
Jónsson, H., Ginolhac, A., Schubert, M., Johnson, P. L. F., and Orlando, L. (2013). MapDamage2.0: Fast approximate Bayesian estimates of ancient DNA damage parameters. Bioinformatics 29, 1682–1684. doi: 10.1093/bioinformatics/btt193
Kircher, M., Sawyer, S., and Meyer, M. (2012). Double indexing overcomes inaccuracies in multiplex sequencing on the Illumina platform. Nucleic Acids Res. 40, 1–8. doi: 10.1093/nar/gkr771
Linck, E., and Battey, C. J. (2019). Minor allele frequency thresholds strongly affect population structure inference with genomic data sets. Mol. Ecol. Resour. 19, 639–647. doi: 10.1111/1755-0998.12995
Linck, E., Epperly, K., Van Els, P., Spellman, G. M., Bryson, R. W., McCormack, J. E., et al. (2019). Dense geographic and genomic sampling reveals paraphyly and a cryptic lineage in a classic sibling species complex. Syst. Biol. 68, 956–966. doi: 10.1093/sysbio/syz027
Linder, H. P., Hardy, C. R., and Rutschmann, F. (2005). Taxon sampling effects in molecular clock dating: An example from the African Restionaceae. Mol. Phylogenet. Evol. 35, 569–582. doi: 10.1016/j.ympev.2004.12.006
Lyra, M. L., Lourenço, A. C. C., Pinheiro, P. D. P., Pezzuti, T. L., Baêta, D., Barlow, A., et al. (2020). High-throughput DNA sequencing of museum specimens sheds light on the long-missing species of the Bokermannohyla claresignata group (Anura: Hylidae: Cophomantini). Zool. J. Linn. Soc. 190, 1235–1255. doi: 10.1093/zoolinnean/zlaa033
Maliet, O., Hartig, F., and Morlon, H. (2019). A model with many small shifts for estimating species-specific diversification rates. Nat. Ecol. Evol. 3, 1086–1092. doi: 10.1038/s41559-019-0908-0
Mason, V. C., Li, G., Helgen, K. M., and Murphy, W. J. (2011). Efficient cross-species capture hybridization and next-generation sequencing of mitochondrial genomes from noninvasively sampled museum specimens. Genome Res. 21, 1695–1704. doi: 10.1101/gr.120196.111
McCormack, J. E., Tsai, W. L. E., and Faircloth, B. C. (2016). Sequence capture of ultraconserved elements from bird museum specimens. Mol. Ecol. Resour. 16, 1189–1203. doi: 10.1111/1755-0998.12466
McDonough, M. M., Parker, L. D., McInerney, N. R., Campana, M. G., and Maldonado, J. E. (2018). Performance of commonly requested destructive museum samples for mammalian genomic studies. J. Mammal. 99, 789–802. doi: 10.1093/jmammal/gyy080
McGuire, J. A., Cotoras, D. D., O'Connell, B., Lawalata, S. Z. S., Wang-Claypool, C. Y., Stubbs, A., et al. (2018). Squeezing water from a stone: High- throughput sequencing from a 145-year old holotype resolves (barely) a cryptic species problem in flying lizards. PeerJ 2018, 1–16. doi: 10.7717/peerj.4470
Meyer, M., and Kircher, M. (2010). Illumina sequencing library preparation for highly multiplexed target capture and sequencing. Cold Spring Harb. Protoc. 2010, pdb.prot5448. doi: 10.1101/pdb.prot5448
Mondol, S., Bruford, M. W., and Ramakrishnan, U. (2013). Demographic loss, genetic structure and the conservation implications for indian tigers. Proc. R. Soc. B Biol. Sci. 280:e0496. doi: 10.1098/rspb.2013.0496
Nakahama, N. (2021). Museum specimens: An overlooked and valuable material for conservation genetics. Ecol. Res. 36, 13–23. doi: 10.1111/1440-1703.12181
Nakahama, N., and Isagi, Y. (2018). Recent transitions in genetic diversity and structure in the endangered semi-natural grassland butterfly, Melitaea protomedia, in Japan. Insect Conserv. Divers. 11, 330–340. doi: 10.1111/icad.12280
Nguyen, L. T., Schmidt, H. A., Von Haeseler, A., and Minh, B. Q. (2015). IQ-TREE: A fast and effective stochastic algorithm for estimating maximum-likelihood phylogenies. Mol. Biol. Evol. 32, 268–274. doi: 10.1093/molbev/msu300
Pääbo, S., Poinar, H., Serre, D., Jaenicke-Després, V., Hebler, J., Rohland, N., et al. (2004). Genetic analyses from ancient DNA. Annu. Rev. Genet. 38, 645–679. doi: 10.1146/annurev.genet.37.110801.143214
Perez, M. F., Franco, F. F., Bombonato, J. R., Bonatelli, I. A. S., Khan, G., Romeiro-Brito, M., et al. (2018). Assessing population structure in the face of isolation by distance: Are we neglecting the problem? Divers. Distrib. 24, 1883–1889. doi: 10.1111/ddi.12816
Peter, B. M., and Slatkin, M. (2013). Detecting range expansions from genetic data. Evolution 67, 3274–3289. doi: 10.1111/evo.12202
Peter, B. M., and Slatkin, M. (2015). The effective founder effect in a spatially expanding population. Evolution 69, 721–734. doi: 10.1111/evo.12609
Pfeifer, B., Wittelsbürger, U., Ramos-Onsins, S. E., and Lercher, M. J. (2014). PopGenome: An efficient swiss army knife for population genomic analyses in R. Mol. Biol. Evol. 31, 1929–1936. doi: 10.1093/molbev/msu136
Pickrell, J. K., and Pritchard, J. K. (2012). Inference of population splits and mixtures from genome-wide allele frequency data. PLoS Genet. 8, e1002967. doi: 10.1371/journal.pgen.1002967
Potter, S., Bragg, J. G., Blom, M. P., Deakin, J. E., Kirkpatrick, M., Eldridge, M. D., et al. (2017). Chromosomal speciation in the genomics era: disentangling phylogenetic evolution of rock-wallabies. Front. Genet. 8, e00010. doi: 10.3389/fgene.2017.00010
Potter, S., Bragg, J. G., Peter, B. M., Bi, K., and Moritz, C. (2016). Phylogenomics at the tips: inferring lineages and their demographic history in a tropical lizard, Carlia amax. Mol. Ecol. 25, 1367–1380. doi: 10.1111/mec.13546
Potter, S., Bragg, J. G., Turakulov, R., Eldridge, M. D. B., Deakin, J., Kirkpatrick, M., et al. (2022). Limited Introgression between Rock-Wallabies with Extensive Chromosomal Rearrangements. Mol. Biol. Evol. 39:msab333. doi: 10.1093/molbev/msab333
Potter, S., Close, R. L., Taggart, D. A., Cooper, S. J. B., and Eldridge, M. D. B. (2014). Taxonomy of rock-wallabies, Petrogale (Marsupialia: Macropodidae). IV. Multifaceted study of the brachyotis group identifies additional taxa. Aust. J. Zool. 62, 401–414. doi: 10.1071/ZO13095
Potter, S., Eldridge, M. D. B., Taggart, D. A., and Cooper, S. J. B. (2012). Multiple biogeographical barriers identified across the monsoon tropics of northern Australia: phylogeographic analysis of the brachyotis group of rock-wallabies. Mole. Ecol. 21, 2254–2269. doi: 10.1111/j.1365-294X.2012.05523.x
Potter, S., Moritz, C., and Eldridge, M. D. B. (2015). Gene flow despite complex Robertsonian fusions among rock-wallaby (Petrogale) species. Biol. Lett. 11, 20150731. doi: 10.1098/rsbl.2015.0731
Potter, S., Xue, A. T., Bragg, J. G., Rosauer, D. F., Roycroft, E. J., and Craig, M. (2018). Pleistocene climatic changes drive diversification across a tropical savanna. Mol. Ecol. 27, 520–532. doi: 10.1111/mec.14441
Pritchard, J. K., Stephens, M., and Donnelly, P. (2000). Inference of population structure using multilocus genotype data. Genetics 155, 945–959. doi: 10.1093/genetics/155.2.945
Pyron, R. A., Beamer, D. A., Holzheuser, C. R., Lemmon, E. M., Lemmon, A. R., Wynn, A. H., et al. (2022). Contextualizing enigmatic extinctions using genomic DNA from fluid-preserved museum specimens of Desmognathus salamanders. Conserv. Genet. 23, 375–386. doi: 10.1007/s10592-021-01424-4
Rowe, K. C., Singhal, S., Macmanes, M. D., Ayroles, J. F., Morelli, T. L., Rubidge, E. M., et al. (2011). Museum genomics: Low-cost and high-accuracy genetic data from historical specimens. Mol. Ecol. Resour. 11, 1082–1092. doi: 10.1111/j.1755-0998.2011.03052.x
Roycroft, E., Achmadi, A., Callahan, C. M., Esselstyn, J. A., Good, J. M., Moussalli, A., et al. (2021a). Molecular evolution of ecological specialisation: genomic insights from the diversification of murine rodents. Genome Biol. Evol. 13, 1–16. doi: 10.1093/gbe/evab103
Roycroft, E., MacDonald, A. J., Moritz, C., Moussalli, A., Miguez, R. P., and Rowe, K. C. (2021b). Museum genomics reveals the rapid decline and extinction of Australian rodents since European settlement. Proc. Natl. Acad. Sci. U.S.A. 118:e2021390118. doi: 10.1073/pnas.2021390118
Roycroft, E. J., Moussalli, A., and Rowe, K. C. (2020). Phylogenomics uncovers confidence and conflict in the rapid radiation of australo-papuan rodents. Syst. Biol. 69, 431–444. doi: 10.1093/sysbio/syz044
Ruane, S., and Austin, C. C. (2017). Phylogenomics using formalin-fixed and 100+ year-old intractable natural history specimens. Mol. Ecol. Resour. 17, 1003–1008. doi: 10.1111/1755-0998.12655
Sarver, B., Keeble, S., Cosart, T., Tucker, P., Dean, M., and Good, J. (2017). Phylogenomic insights into mouse evolution using a pseudoreference approach. Genome Biol. Evol. 9, 726–739. doi: 10.1093/gbe/evx034
Sawyer, S., Krause, J., Guschanski, K., Savolainen, V., and Pääbo, S. (2012). Temporal patterns of nucleotide misincorporations and DNA fragmentation in ancient DNA. PLoS ONE 7, e0034131. doi: 10.1371/journal.pone.0034131
Schmitt, C. J., Cook, J. A., Zamudio, K. R., and Edwards, S. V. (2019). Museum specimens of terrestrial vertebrates are sensitive indicators of environmental change in the Anthropocene. Philos. Trans. R. Soc. B Biol. Sci. 374:387. doi: 10.1098/rstb.2017.0387
Shafer, A. B. A., Peart, C. R., Tusso, S., Maayan, I., Brelsford, A., Wheat, C. W., et al. (2017). Bioinformatic processing of RAD-seq data dramatically impacts downstream population genetic inference. Methods Ecol. Evol. 8, 907–917. doi: 10.1111/2041-210X.12700
Singhal, S., Grundler, M., Colli, G., and Rabosky, D. L. (2017). Squamate Conserved Loci (SqCL): A unified set of conserved loci for phylogenomics and population genetics of squamate reptiles. Mol. Ecol. Resour. 17, e12–e24. doi: 10.1111/1755-0998.12681
Slatkin, M. (2005). Seeing ghosts: The effect of unsampled populations on migration rates estimated for sampled populations. Mol. Ecol. 14, 67–73. doi: 10.1111/j.1365-294X.2004.02393.x
Sousa, V., and Hey, J. (2013). Understanding the origin of species with genome-scale data: modelling gene flow. Nat. Rev. Genet. 14, 404–414. doi: 10.1038/nrg3446
Streicher, J. W., Schulte, J. A., and Wiens, J. J. (2016). How should genes and taxa be sampled for phylogenomic analyses with missing data? An Empirical Study in Iguanian Lizards. Syst. Biol. 65:58. doi: 10.1093/sysbio/syv058
Tajima, F. (1989). Statistical method for testing the neutral mutation hypothesis by DNA polymorphism. Genetics 123, 585–595. doi: 10.1093/genetics/123.3.585
Tsai, W. L. E., Schedl, M. E., Maley, J. M., and McCormack, J. E. (2020). More than skin and bones: Comparing extraction methods and alternative sources of DNA from avian museum specimens. Mol. Ecol. Resour. 20, 1220–1227. doi: 10.1111/1755-0998.13077
van der Valk, T., Díez-del-Molino, D., Marques-Bonet, T., Guschanski, K., and Dalén, L. (2019). Historical genomes reveal the genomic consequences of recent population decline in eastern gorillas. Curr. Biol. 29, 165–170.e6. doi: 10.1016/j.cub.2018.11.055
Watterson, G. A. (1975). On the number of segregating sites in genetical models without recombination. Theor. Popul. Biol. 7, 256–276. doi: 10.1016/0040-5809(75)90020-9
Keywords: bioinformatics, collections, exon capture, genomics, historical DNA, Petrogale, phylogenomics, Murinae
Citation: Roycroft E, Moritz C, Rowe KC, Moussalli A, Eldridge MDB, Portela Miguez R, Piggott MP and Potter S (2022) Sequence Capture From Historical Museum Specimens: Maximizing Value for Population and Phylogenomic Studies. Front. Ecol. Evol. 10:931644. doi: 10.3389/fevo.2022.931644
Received: 29 April 2022; Accepted: 24 June 2022;
Published: 22 July 2022.
Edited by:
Jonathan J. Fong, Lingnan University, ChinaReviewed by:
Nicolás Mongiardino Koch, University of California, San Diego, United StatesNiklas Wahlberg, Lund University, Sweden
Copyright © 2022 Roycroft, Moritz, Rowe, Moussalli, Eldridge, Portela Miguez, Piggott and Potter. This is an open-access article distributed under the terms of the Creative Commons Attribution License (CC BY). The use, distribution or reproduction in other forums is permitted, provided the original author(s) and the copyright owner(s) are credited and that the original publication in this journal is cited, in accordance with accepted academic practice. No use, distribution or reproduction is permitted which does not comply with these terms.
*Correspondence: Emily Roycroft, ZW1pbHkucm95Y3JvZnRAYW51LmVkdS5hdQ==