- 1Graduate School of Environmental Science, Hokkaido University, Sapporo, Japan
- 2Graduate School of Medicine, University of the Ryukyus, Nishihara, Japan
- 3Graduate School of Science and Engineering, University of Toyama, Toyama, Japan
- 4Department of Biology, Keio University, Yokohama, Japan
- 5National Institute for Basic Biology Core Research Facilities, Okazaki, Japan
- 6Misaki Marine Biological Station, University of Tokyo, Miura, Japan
- 7Faculty of Science, Academic Assembly, University of Toyama, Toyama, Japan
The organization of social insect colonies requires sophisticated mechanisms to regulate caste composition according to colony demands. In termites, the soldier caste is responsible for the inhibition of soldier differentiation, but the mechanism underlying the regulation of soldier differentiation is still unclear. In this study, we performed transcriptome analyses to identify genes expressed in workers that fluctuated in the presence of soldiers in the subterranean termite Reticulitermes speratus. First, soldier differentiation was artificially induced via juvenile hormone (JH) application, and the inhibitory effects of soldier differentiation on soldier presence were evaluated. Second, transcriptomes were prepared from workers with or without soldiers under JH treatment, and expression analyses were performed to identify differentially expressed genes (DEGs) for each treatment. The expression levels of several DEGs were verified by quantitative real-time PCR. The results indicated that only a small number of DEGs were upregulated by the presence of soldiers. A homology search of DEGs and gene ontology (GO) analysis of the DEGs showed that some genes were responsible for the regulation of hormone levels, social interaction, and response to xenobiotic substances, suggesting that they could be involved in developmental arrest and pheromonal regulation in workers. Moreover, GO analysis indicated that the expression of many genes, including those involved in hormone metabolic processes, fluctuated with JH application. Suppression of soldier differentiation in the presence of soldiers could be accomplished by the expression of a large number of genes required for soldier differentiation.
Introduction
Social insects have multiple phenotypes (castes) in the same colony. The optimal composition of each caste and cooperation among individuals are needed for regular colony growth and maintenance (Wilson, 1971). Termites are major social insect groups, and their castes are normally discriminated as workers, soldiers, and reproductives (Roisin, 2000; Korb and Thorne, 2017). Termite caste differentiation is thought to be regulated by caste-specific gene expression through environmental stimuli that may trigger various hormonal conditions (Noirot, 1991; Watanabe et al., 2014). Because a distinctive sterile soldier caste is crucial for termite sociality and evolution, soldier differentiation has been extensively studied during the last two decades (Miura and Maekawa, 2020). Genes and cascades involved in specific weapon formations have been analyzed, and several important factors, including hormone-related and toolkit genes, have been clarified (e.g., Zhou et al., 2006; Toga et al., 2012; Masuoka et al., 2018; Sugime et al., 2019). The expression patterns of these factors can be affected by environmental stimuli via inter-individual (i.e., social) interactions (Watanabe et al., 2014). However, the effects of social interactions on gene expression and endocrine status have not yet been elucidated (Miura and Maekawa, 2020).
Termite soldiers are differentiated from workers via an intermediate stage called a presoldier. Presoldier and soldier formation is known to be inhibited by soldiers themselves and promoted by the reproductive caste in the same colony (Watanabe et al., 2014). Indeed, for the inhibitory mechanism by soldier existence, candidate primer pheromones [soldier inhibiting factors, SIFs (Park and Raina, 2005)], transferred from soldiers to workers, were identified in some species of Reticulitermes [γ-cadinenal and (-)-β-elemene; Tarver et al., 2009; Tarver et al., 2011; Mitaka et al., 2017]. These chemicals are terpenoids and are probably defense substances released from the frontal gland, which is developed in soldiers of phylogenetically apical termite lineages (Miura and Maekawa, 2020). Previous studies have also shown that live soldiers or whole extracts of soldiers had strong inhibitory effects on soldier differentiation (Tarver et al., 2011; Mitaka et al., 2017). Moreover, similar inhibitory effects were observed in the extracts of soldiers from more basal termite species (without frontal glands), which possess physical weapons such as enlarged mandibles and head capsule (Korb et al., 2003). Consequently, SIFs may consist of complex and lineage-specific compounds. However, because information about the internal changes of workers are lacking, it is unclear how the degree of inhibitory effects of SIFs is determined.
The percentage of soldiers is usually very small in natural colonies (Howard and Haverty, 1981), and thus soldier differentiation is not frequently observed compared to those of workers. However, soldier differentiation can be artificially induced by juvenile hormone (JH) or JH analog treatments with workers (Watanabe et al., 2014; Miura and Maekawa, 2020). In R. speratus, artificial presoldier induction rates were significantly decreased by the presence of soldiers compared to those without soldiers (Watanabe et al., 2011). Importantly, JH titers of workers with soldiers were significantly lower than those of workers without soldiers just 5 days after JH treatment. If workers are reared without soldiers, only small numbers of workers differentiate to presoldiers and soldiers (e.g., about 7% for 16 days in Coptotermes formosanus; Park and Raina, 2005). However, we never identify the soldier-destined workers before the presoldier molt normally in the mature colony. Consequently, to determine effectively whether gene expression changes are affected by the existence of soldiers (probably using SIFs), an artificial presoldier induction method is considered useful, and R. speratus workers should be investigated within 5 days after JH treatment.
Here, internal transcriptomic changes in JH-treated workers caused by coexisting soldiers were analyzed in R. speratus. The genome sequence and transcriptome of each caste/caste differentiation have been clarified for this species (Shigenobu et al., 2022; Saiki et al., submitted manuscript). Transcriptomes were prepared from worker individuals with or without soldiers within 5 days after JH treatment. Based on the differentially expressed genes (DEGs) observed and specific gene ontology (GO) terms detected, the molecular basis of physiological changes in workers with coexisting soldiers is discussed.
Materials and Methods
Termites
Mature termite colonies (total: four) were collected in Furudo, Toyama Prefecture, Japan, in 2014. The nest logs were transported to the laboratory and kept in a plastic case under constant darkness. Sixth and seventh instar workers were selected for the following experiments based on the number of antennal segments and body size (Tsunoda et al., 1986; Takematsu, 1992). All experimental insects were maintained in an incubator at 25°C under constant darkness for at least 3 days before use.
Dish Assays for Induction of Presoldier Differentiation
Dish assays were performed in accordance with the procedure described previously (Tsuchiya et al., 2008; Watanabe et al., 2011; Masuoka et al., 2013). Briefly, filter papers (55 mm diameter; Advantec, Japan) were treated with 20 and 40 μg JH III (Santa Cruz Biotechnology, Dallas, TX, United States) dissolved in 200 μL acetone. Filter papers treated with acetone alone were used as controls. After the acetone evaporated, each filter paper was moistened with approximately 450 μL of distilled water and placed in a 65 mm Petri dish. Then, 20 workers were exposed to each filter paper along with 0 or 10 soldiers. Each category was replicated four times using individuals sampled from four different colonies. All dishes were kept in an incubator at 25°C in constant darkness. The number of dead individuals and differentiated presoldiers was checked every 24 h. If a dead worker was detected, it was immediately removed from the dish. If a soldier died, a live soldier was added from a separate dish kept in the incubator. On day 16, the induction rates of newly molted presoldiers were compared between dishes with 0 or 10 soldiers. Presoldier differentiation rates (mean ± S.D. values) were calculated from dishes replicated four times (20 workers per dish) and evaluated by the generalized Wilcoxon test (80 individuals in each JH III concentration) using the statistical software Mac Statistical Analysis, version 1.5 (Esumi, Japan). Statistical significance was set at P < 0.05.
Dish Assays for RNA Extraction
Dish assays for RNA extraction were performed using the method described in Section “Dish Assays for Induction of Presoldier Differentiation.” Three days after treatment with 40 μg JH III (or acetone treatment as control), all workers in each dish were fixed with liquid nitrogen and stored at −80°C until RNA extraction.
RNA Extraction, Library Preparation, and Sequencing
Total RNA was extracted from four categories [(1) workers with 0 soldiers 3 days after acetone treatment, (2) workers with 10 soldiers 3 days after acetone treatment, (3) workers with 0 soldiers 3 days after JH treatment, (4) workers with 10 soldiers 3 days after JH treatment] using an SV Total RNA extraction kit (Promega, Madison, WI, United States). RNA extracted from 20 individuals without guts was used for each library. Four replicates derived from four different colonies (biological quadruplicates) were prepared (four libraries × four categories = 16 libraries). The amounts of total extracted RNA and DNA were quantified using a Qubit fluorometer (Life Technology, Eugene, OR, United States) and the quality was confirmed using an Agilent 2100 bioanalyzer (Agilent Technologies, Palo Alto, CA, United States). Total RNA (500 ng) was used for cDNA synthesis and purification using a low-throughput protocol with a TruSeq Stranded RNA LT Kit (Illumina, San Diego, CA, United States). A half-scale reaction of the standard protocol was used for library preparation. The quality and quantity of cDNA were validated using an Agilent 2100 bioanalyzer and a KAPA qPCR SYBR Green PCR Kit (GeneWorks, Thebarton, SA, Australia). Sixteen libraries were sequenced by single-end sequencing (101 bp) using Hiseq1500 (Illumina, San Diego, CA, United States). All reads were deposited in the DDBJ Sequence Read Archive (DRA) database under accession number DRA013774.
Identification of Differentially Expressed Genes
For each read, nucleotides with a low-quality score at the sequence ends and adapter sequences were removed using SolexaQA ver. 2.5 (Cox et al., 2010), and the cutadapt program version 1.2.1 (Martin, 2011), respectively. These reads were mapped against the R. speratus genome (Rspe OGS1.0; Shigenobu et al., 2022) using the TopHat program version 2.0.13 (Kim et al., 2013) with default settings.
The counting of reads and detection of DEGs were performed using Cufflinks pipeline version 2.2.1 (Trapnell et al., 2010). In this program, the count data were normalized and analyzed using the same algorithm implemented in DESeq (Anders and Huber, 2010). These counts were scaled using the median of the geometric means of fragment counts across all libraries. After normalization, pairwise comparisons were performed using cuffdiff command with “–frag-bias-correct” and “–multi-read-correct” options (Roberts et al., 2011; Trapnell et al., 2013). We used the following four schemes for comparison: (i) without JH and 0 soldiers vs. without JH and 10 soldiers, (ii) without JH and 0 soldiers vs. 40 μg JH and 10 soldiers, (iii) without JH and 10 soldiers vs. 40 μg JH and 10 soldiers, and (iv) 40 μg JH and 0 soldiers vs. 40 μg JH and 10 soldiers. Homology searches of DEGs obtained in (i) and (iv) (the same JH concentration and relatively small numbers of DEGs; see results) were performed by BLASTX using the NCBI non-redundant protein database (nr) (run on March 2022), and the top hit sequence was obtained for each DEG.
We also conducted a generalized linear model (GLM) analysis. Because Cufflinks pilelined did not generate raw count data, we re-mapped and counted our RNA-seq data. Briefly, we used the Bowtie program (Langmead et al., 2009) for mapping and RSEM v1.3.3 software (Li and Dewey, 2011) to estimate the relative abundance and expected read counts of all genes. The count data were normalized by the TMM (Trimmed Mean of M values) method in the edgeR software package (Robinson and Oshlack, 2010; Robinson et al., 2010; McCarthy et al., 2012). The adjusted count values were then used for the DEG analysis. Two model designs were used for DEG detection. In the first design, we considered three explanatory factors: soldier presence, JH treatment, and these interactions (Soldier presence × JH treatment). In the second design, in addition to these three factors, we used colony information as another explanatory factor. The threshold of statistical analysis is false discovery rate (FDR) cutoff of 0.05.
Annotation and Gene Ontology Enrichment Analysis
Since GO terms were only assigned to model organisms, we identified the fruit fly ortholog of each termite gene. All predicted termite amino acid sequences were searched against fruit fly (Drosophila melanogaster) amino acid sequences using BLASTP. The complete amino acid sequence datasets for the fruit flies were downloaded from Flybase version FB2012_04.1 BLASTP searches were performed using termite genes as queries, and a 10–5 e-value cutoff was used against the fruit fly dataset. The top hit proteins were defined as orthologs of the focal termite gene.
Gene ontology enrichment analysis was performed using clusterProfiler software package (version 2.4.3, R version 3.3.3; Yu et al., 2012). We performed an enrichment test for GO terms by assuming a hypergeometric distribution. To prevent high FDR due to multiple tests, we also estimated the q-values for FDR control (Storey, 2002). For these analyses, we used a list of DEGs identified by pairwise comparisons. To identify enriched GO biological processes, we conducted an enrichment analysis of these DEGs, in which p < 0.1 and q < 0.05 were used as strict cutoff values.
Quantitative Real-Time PCR
To validate the transcriptome analysis, qPCR was performed for the identified DEGs (a total of three genes, see results). Individuals were sampled from five different colonies collected in Toyama Prefecture, Japan from 2019 to 2020. The dish assay was performed following the method described above, and the presoldier induction rates were confirmed 16 days after the treatment. Total RNA was extracted from workers with 0 or 10 soldiers 3 days after treatment with 40 μg JH III using ISOGEN II (Nippon Gene, Tokyo, Japan). A total of 17–20 live workers (whole bodies) were used in each sample and homogenized using a Bead Mill 4 (Thermo Fisher, Waltham, MA, United States). Five replicates derived from five different colonies (biological quintuplicates) were prepared for each category. The amounts of RNA and DNA were quantified using a Qubit fluorometer, and the purity and quantity of the extracted RNA were determined by spectroscopic measurements at 230, 260, and 280 nm using a NanoVue spectrophotometer (GE Healthcare Bio-Sciences, Tokyo, Japan). DNase-treated RNA (2 μg per sample) was transcribed using High-Capacity cDNA Reverse Transcription Kit (Thermo Fisher). Quantitative PCR (200 nM of each primer) was performed in biological quintuplicates using PowerUP SYBR Green Master Mix (Thermo Fisher) and QuantStudio 3 Real-Time PCR System (Thermo Fisher). According to the previous study (Miyazaki et al., 2021), to determine an internal control gene, the suitability of six reference genes, EF1-alfa (accession no. AB602838), NADH-dh (AB602837), beta-actin (AB520714), GstD1 (gene ID: RS001168), EIF-1 (RS005199), and RPS18 (RS015150), were evaluated using GeNorm (Vandesompele et al., 2002) and NormFinder (Andersen et al., 2004). Specific primers were designed against each gene sequence using Primer3Plus (Untergasser et al., 2007; Supplementary Table 1). Statistical analysis, Welch’s t-test, was performed using the statistical software Mac Statistical Analysis version 3.0 (Esumi, Tokyo, Japan).
Results and Discussion
Presoldier Induction Rates
As shown in a previous study (Watanabe et al., 2011), presoldier induction rates after both 20 and 40 μg JH III treatments were significantly reduced by soldier presence (Figure 1). The JH titer (endogenous + applied JH III) levels in workers with 0 soldiers were shown to be higher than those with 10 soldiers 5 days after JH III treatment (Watanabe et al., 2011, 2014). Because we tried to effectively detect gene expression changes in workers before the reduction of JH III titer levels, total RNA was extracted from workers 3 days after 40 μg or without JH III treatments.
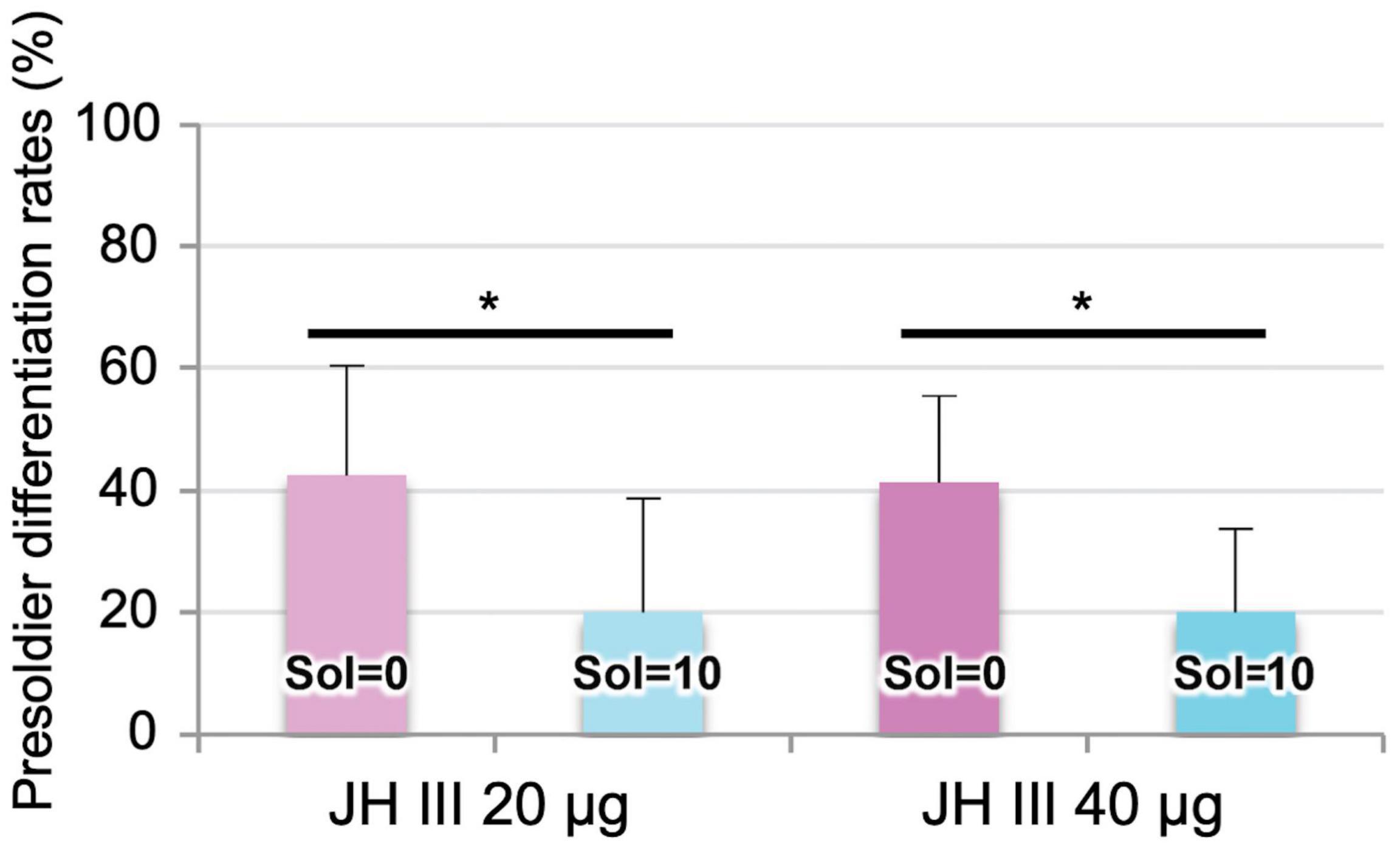
Figure 1. Presoldier induction rates (mean ± S.D., biological quadruplicates) 16 days after the 20 or 40 μg juvenile hormone (JH) III application in Reticulitermes speratus. In both applications, presoldiers were more frequently differentiated from workers with 0 soldiers (soldier absence, “Sol = 0”) than those with 10 soldiers (soldier presence, “Sol = 10”) (generalized Wilcoxon test, *P < 0.05).
Numbers of Differentially Expressed Genes
We obtained different numbers of DEGs for the four pairwise comparisons (Figure 2). The number of DEGs between 40 μg and without JH III treatments in workers with 0 soldiers was 3,089. Of these genes, 1,516 and 1,573 were upregulated in 40 μg and without JH III-treated workers, respectively (Figure 2; Supplementary Table 2). Similarly, the number of DEGs between 40 μg and without JH III treatments in workers with 10 soldiers was 3,014. Of these genes, 1,567 and 1,447 were upregulated in 40 μg and without JH III-treated workers, respectively (Figure 2; Supplementary Table 3). In contrast, there were only 44 DEGs between soldier absence (26 upregulated genes) and soldier presence (18 upregulated genes) in acetone-treated workers (Figure 2; Supplementary Table 4). The expression levels of 171 genes were significantly different between soldier absence (142 upregulated genes) and soldier presence (29 upregulated genes) in JH III-treated workers (Figure 2; Supplementary Table 5). In particular, the expression of a large number of genes (approximately 3,000) in workers was fluctuated by JH/acetone treatments. These numbers of DEGs were similar to, but slightly larger than, those between JH analog (methoprene) and acetone-treated workers in Coptotermes formosanus (2,547 unigenes) (Du et al., 2020). Reticulitermes and Coptotermes are phylogenetically closely related to each other (Bucek et al., 2019), and inferred genome sizes (800–900 Mb) and total gene numbers (approximately 13,000–15,000) of both species are very similar (Maekawa et al., 2022). Consequently, these discrepancies may be due to the differences in treated chemicals [JH homolog (this study), synthetic juvenoid (Du et al., 2020)] and/or RNA-sequencing (RNA-seq) methods [genome-based assay (this study), de novo transcriptome assembly-based assay (Du et al., 2020)]. Most of these DEGs were also identified by GLM analysis (Supplementary Tables 6, 7), suggesting that our analysis effectively listed genes which are related to soldier differentiation.
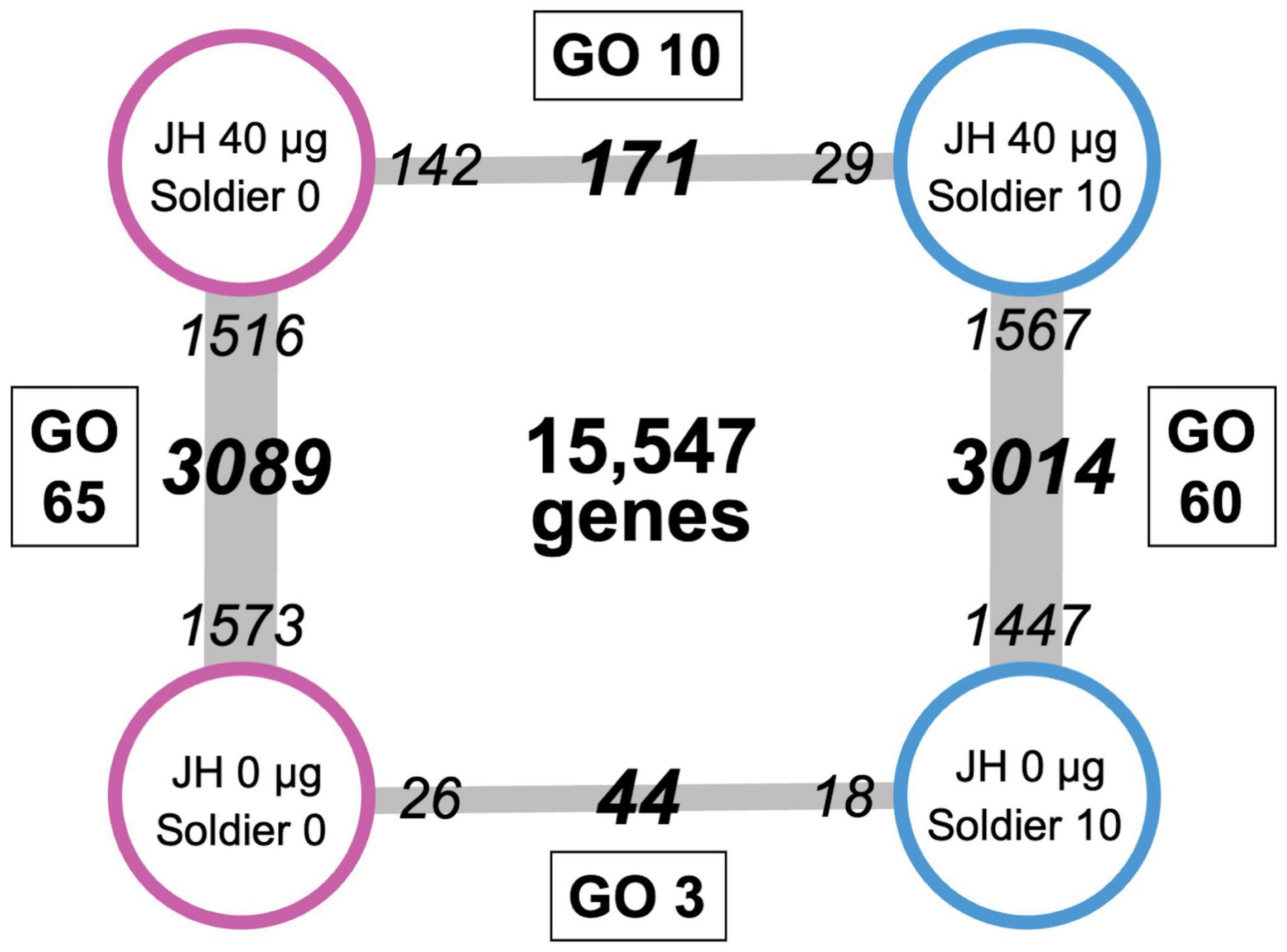
Figure 2. Numbers of differentially expressed genes (DEGs, bold italic) between each category. Small italic numerals indicate the numbers of upregulated genes in each category. Gene numbers between juvenile hormone (JH) III and acetone treatments (3,014 in soldier presence, 3,089 in soldier absence) were much larger than those between soldier presence and absence (171 in JH treatments, 44 in acetone treatments). Numbers of significant gene ontology (GO) terms of the DEGs are indicated in squares.
Quantitative Real-Time PCR
We selected three genes [cytochrome P450 (Cyp4c3; RS013835), alpha-amylase (Amy-p; RS006137), and multidrug resistance protein (Mdr49; RS002816)], which were listed as DEGs in JH III-treated workers with 0 and 10 soldiers (Supplementary Table 5). We focused on this category because it was expected that there would be large variations in gene expression levels among samples. RNA-seq analysis indicated that all these three genes were upregulated in workers with 10 soldiers. A suitable reference gene (NADH-dh) was selected for real-time qPCR analysis using GeNorm and NormFinder (Supplementary Table 8). Real-time qPCR analysis showed that high expression levels of the three genes examined were observed in JH III-treated workers with 10 soldiers (soldier presence), compared to those with 0 soldiers (soldier absence) (Figure 3). Although the statistical support of RS002816 expression levels between soldier presence and absence was weak (p = 0.106; Welch’s t-test), it should be noted that the q value of RS002816 (0.012) was higher than that of the remaining two genes (0.007; Supplementary Table 5). Overall, the qPCR analysis supported the reliability of the RNA-seq results.
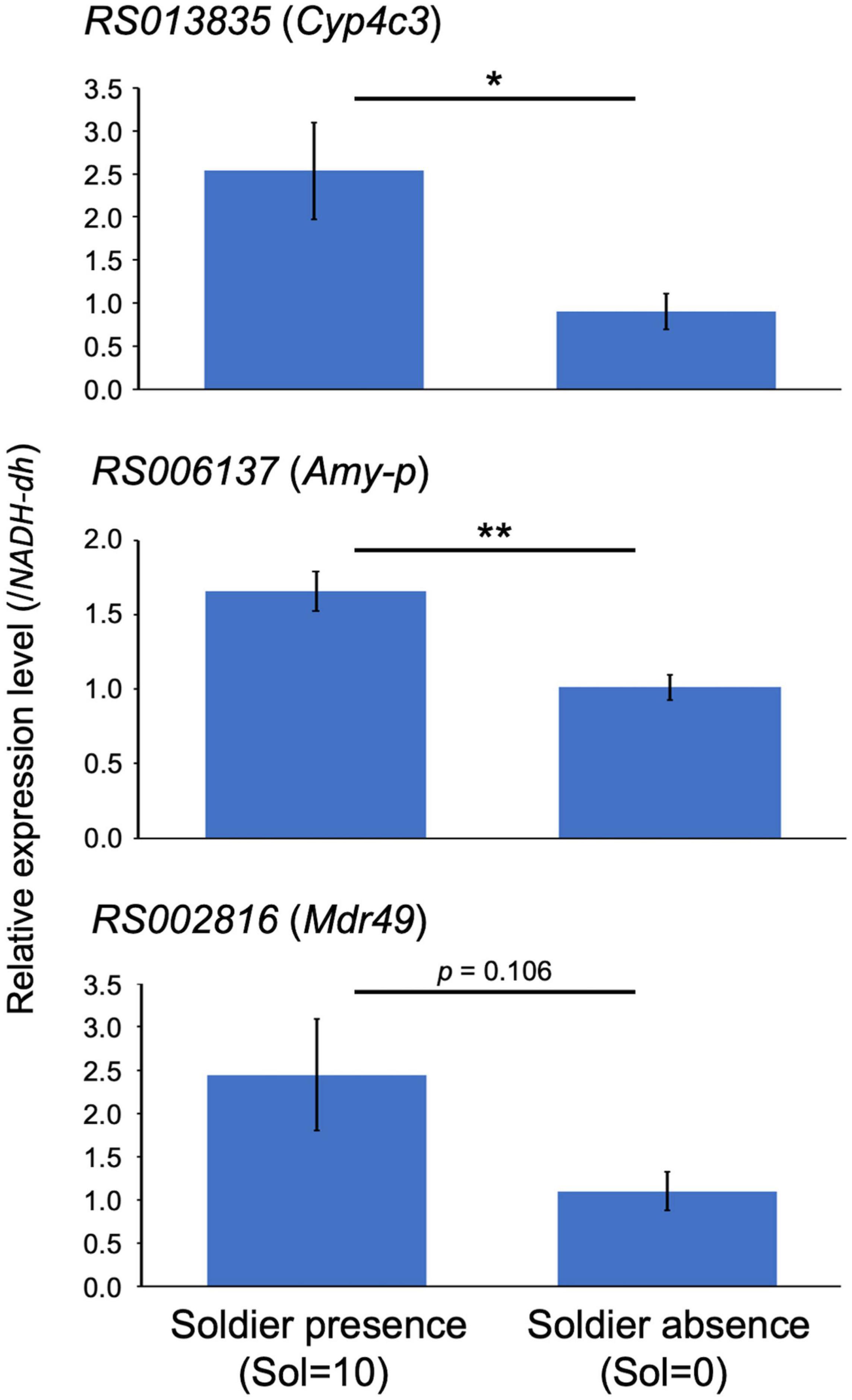
Figure 3. Quantitative real-time PCR expression levels of the three genes (mean ± S.D., biological quintuplicates) in the 40 μg juvenile hormone (JH) III-treated workers with 10 soldiers (Soldier presence, “Sol = 10”) and 0 soldiers (Soldier absence, “Sol = 0”). Each value was normalized to the expression levels of NADH-dh (Supplementary Table 8). Asterisks above the bars indicate significant differences (*P < 0.05, **P < 0.01; Welch’s t-test).
Differentially Expressed Genes Between Soldier Presence and Absence
We focused on DEGs specifically observed in workers with 0 and 10 soldiers under the same JH concentrations. Note that all DEGs discussed below were detected by both designes of GLM analysis (Supplementary Tables 6, 7), except that Cyp4c3 (RS013835) was not observed only in the first design. In the acetone treatment, three takeout protein genes (RS013762, RS014812, and RS010477) were highly expressed in workers with 0 soldiers, whereas a JH-inducible protein gene (RS007835) was highly expressed in workers with 10 soldiers (Supplementary Table 4). Takeout proteins are normally able to bind JH because they possess the JH binding protein domain conserved in insects (Noriega et al., 2006; Chamseddin et al., 2012). Although the functions of JH-inducible and takeout proteins are unanalyzed, there is a possibility that these work on the role of JH sequestration from the hemolymph of workers, such as JH binding proteins (e.g., hexamerin, Zhou et al., 2006). Alternatively, these proteins may be crusial for the change of the JH sensitivity in workers, which is similar to the case in Pheidole ants (Wheeler and Nijhout, 1984; Nijhout, 2003). In any case, these results support that JH levels in workers are affected by the three-day interaction with soldiers. Note that other JH binding proteins (including hexamerin) and JH biosynthetic/degradation genes, all of which were annotated in previous literature (Shigenobu et al., 2022), were not detected in our transcriptome analysis.
Two chemosensory protein genes (RS000584 and RS010442) were highly expressed in workers with 0 soldiers (Supplementary Table 4) and were recognized as RspeCSP1 and RspeCSP7, respectively (Shigenobu et al., 2022). Previous RNA-seq analysis in R. speratus indicated that both genes were highly expressed in the head compared to the remaining part of the body of workers (RspeCSP7) or workers and soldiers (RspeCSP1) (Shigenobu et al., 2022). Although their precise expression sites (antennae or other head parts) should be clarified, they may be involved in SIF-related social communication between soldiers and workers.
In the JH III treatment, the functions of some DEGs (total: 52) could not be identified based on the BLASTX search (hypothetical or uncharacterized proteins, no hit; Supplementary Table 5). Most DEGs shown in the acetone treatment described above (Supplementary Table 4) were not detected, but many genes involved in antimicrobial and xenobiotic responses and digestive enzymes were observed in workers with 10 soldiers [e.g., Mdr49 (RS002816), prolixicin antimicrobial protein (AttD, RS000201), laccase2 (RS004166), and Amy-p (RS006137)] and those with 0 soldiers [e.g., C-type lysozyme-3 (RS003406), toll-like receptor 6 (RS012895), and venom protease-like (RS012253)]. Interestingly, fatty acyl-CoA reductase (RS002448), a well-known soldier-specific gene (Maekawa et al., 2022), was highly expressed in workers with 10 soldiers. As this gene may be involved in the production of soldier-specific cuticular hydrocarbon (CHC) profiles (Wu et al., 2018; Maekawa et al., 2022), the presence of soldiers can induce changes in worker CHC profiles. Cyp4c3 (RS013835) was also highly expressed in workers with 10 soldiers. Because CYP4 is involved in the last step of CHC biosynthesis in D. melanogaster (Qiu et al., 2012), it may also support the above hypothesis. The soldier ratio applied in this study was quite high, and further analysis should be performed in colonies with an appropriate soldier ratio under natural conditions (about 2% in R. flavipes; Howard and Haverty, 1981) to clarify the general tendency of this hypothesis.
Finally, in JH III treatment, high expression levels of some members of the multigene family [beta-glucosidase (RS004143) and lipocalin (RS013912); Shigenobu et al., 2022] were observed in workers with 10 soldiers (Supplementary Table 5). Many paralogs of both genes have been identified in the R. speratus genome, and these paralogs showed different expression patterns among castes (Shigenobu et al., 2022). Beta-glucosidase is essential for cellulose digestion in termite workers (Watanabe and Tokuda, 2010), but RS004143 was highly expressed in the thorax and abdomen of soldiers and reproductives (Shigenobu et al., 2022). Thus, RS004143 may have a different role other than wood digestion in R. speratus. It is interesting to note that beta-glucosidase (called Neofem2) is involved in queen-recognition pheromones that probably function in the suppression of reproductive emergence in Cryptotermes secundus (Korb et al., 2009; Korb, 2016). Similarly, lipocalin is a member of the protein transporter family, but molecular phylogeny showed that RS013912 was closely related to soldier-specific protein 1 (SOL1) identified in Hodotermopsis sjostedti (Shigenobu et al., 2022). SOL1 may function as a signaling molecule for defensive social interactions among colony members (Miura et al., 1999; Miura, 2005). We suggest that both RS004143 and RS013912 are involved in chemical communication, and their expression changes in workers are affected by different social circumstances, with or without soldiers.
Gene Ontology Enrichment Analysis of Differentially Expressed Genes
We performed GO enrichment analysis of the DEGs observed in each category (Figure 2). The number of GO terms detected between 40 μg and without JH III treatments in workers with 0 soldiers was 65 (Supplementary Table 9). Similarly, the number between 40 μg and without JH III treatments in workers with 10 soldiers was 60 (Supplementary Table 10). More than half of these terms (total: 38) were common (bold italic terms in Supplementary Tables 9, 10), and four out of 38 terms (GO: 0016053, 0046394, 0072330, and 0032787; metabolic and biosynthetic processes of some molecules) were specifically observed during the worker-presoldier molt (Saiki et al., submitted manuscript). However, specific GO terms involved in hormone levels (e.g., GO: 0010817, 0042446, and 0045455) were detected only in workers with 10 soldiers (Supplementary Table 10). Moreover, the number of GO terms significantly detected between soldier presence and absence in the acetone-treated workers was only three (Supplementary Table 11); all of which were related to the regulation of hormone levels. These results clearly indicate that the JH levels in workers are affected by the presence of soldiers.
Finally, a total of 10 significant GO terms were observed between the presence and absence of soldiers in JH-treated workers (Supplementary Table 12). These terms included metabolic processes of some molecules (GO: 0006022, 1901071, and 0006040), chitin and cuticle development (GO: 0006030 and 0040003), and response to xenobiotic substances (GO: 0009617, 0042742, and 0050830). These results may be explained by the effect of the presence of soldiers on developmental arrest during JH-induced presoldier differentiation, which is generally accompanied by specific cuticle development via the tyrosine metabolic pathway (Masuoka et al., 2013; Masuoka and Maekawa, 2016).
Conclusion
Most workers treated with commercial JH III are differentiated into presoldiers, and living soldiers strongly inhibit the presoldier differentiation by rapid JH decrease soon after interaction with workers (Watanabe et al., 2011, 2014). This study aimed to understand the gene expression profiles of workers affected by coexisting soldiers. RNA-seq analysis supported that worker JH levels are affected by the presence of soldiers, probably by the functions of JH binding and inducible proteins, regulatory factors of social interaction, and response to xenobiotic substances. Further gene function analyses of candidate targets are needed to clarify this possibility.
Data Availability Statement
All sequence reads are available in the DDBJ Sequence Read Archive, accession numbers: DRA013774, DRX342915-DRX342930, and DRR357004-DRR357019 and in the BioProject Archive, accession number: PRJDB13353.
Author Contributions
MM, DW, TM, and KM designed the study. DW, KF, and KM collected the samples. MM, DW, KF, YH, and SS performed the experiments. MM, KF, SS, and KM analyzed the data. MM, YH, TM, and KM drafted the manuscript. All authors have read and approved the manuscript.
Funding
This work was partly supported by a Grant-in-Aid for Scientific Research (Nos. 25128705, JP16K07511, and JP19H03273 to KM) and by a Grant-in-Aid for JSPS Fellows (No. 12J03468 to DW) from the Ministry of Education, Culture, Sports, Science and Technology of Japan.
Conflict of Interest
The authors declare that the research was conducted in the absence of any commercial or financial relationships that could be construed as a potential conflict of interest.
Publisher’s Note
All claims expressed in this article are solely those of the authors and do not necessarily represent those of their affiliated organizations, or those of the publisher, the editors and the reviewers. Any product that may be evaluated in this article, or claim that may be made by its manufacturer, is not guaranteed or endorsed by the publisher.
Acknowledgments
We are grateful to Y. Sugime, K. Toga, R. Saiki, and Y. Masuoka for their assistance during laboratory work.
Supplementary Material
The Supplementary Material for this article can be found online at: https://www.frontiersin.org/articles/10.3389/fevo.2022.924151/full#supplementary-material
Abbreviations
JH, juvenile hormone; SIFs, soldier inhibiting factors; DEGs, differentially expressed genes; GO, gene ontology; FDR, false discovery rate; CHC, cuticular hydrocarbon; GLM, generalized linear model.
Footnotes
References
Anders, S., and Huber, W. (2010). Differential expression analysis for sequence count data. Genome Biol. 11:R106.
Andersen, C., Jensen, J., and Ørntoft, T. (2004). Normalization of real-time quantitative reverse transcription-PCR data: a model-based variance estimation approach to identify genes suited for normalization, applied to bladder and colon cancer data sets. Cancer Res. 64, 5245–5250. doi: 10.1158/0008-5472.CAN-04-0496
Bucek, A., Šobotník, J., He, S., Shi, M., McMahon, D. P., Holmes, E. C., et al. (2019). Evolution of termite symbiosis informed by transcriptome-based phylogenies. Curr. Biol. 29, 3728–3734. doi: 10.1016/j.cub.2019.08.076
Chamseddin, K. H., Khan, S. Q., Nguyen, M. L., Antosh, M., Morris, S. N. S., Kolli, S., et al. (2012). takeout-dependent longevity is associated with altered Juvenile Hormone signaling. Mech. Ageing Dev. 133, 637–646. doi: 10.1016/j.mad.2012.08.004
Cox, M. P., Peterson, D. A., and Biggs, P. J. (2010). SolexaQA: at-a-glance quality assessment of Illumina second-generation sequencing data. BMC Bioinform. 11:485. doi: 10.1186/1471-2105-11-485
Du, H., Tong, R. L., Huang, X., Liu, B., Huang, R., and Li, Z. (2020). Methoprene-induced genes in workers of Formosan subterranean termites (Coptotermes formosanus Shiraki). Insects 11:71. doi: 10.3390/insects11020071
Howard, R., and Haverty, M. I. (1981). Seasonal variation in caste proportions of field colonies of Reticulitermes flavipes (Kollar). Environ. Entomol. 10, 546–549. doi: 10.1093/ee/10.4.546
Kim, D., Pertea, G., Trapnell, C., Pimentel, H., Kelley, R., and Salzberg, S. L. (2013). TopHat2: accurate alignment of transcriptomes in the presence of insertions, deletions and gene fusions. Genome Biol. 14:R36. doi: 10.1186/gb-2013-14-4-r36
Korb, J. (2016). Genes underlying reproductive division of labor in termites, with comparisons to social Hymenoptera. Front. Ecol. Evol. 4:45. doi: 10.3389/fevo.2016.00045
Korb, J., and Thorne, B. (2017). “Sociality in termites,” in Comparative Social Evolution, eds S. R. Rubenstein and P. Abbot (New York, NY: Cambridge University Press), 124–153.
Korb, J., Roux, E. A., and Lenz, M. (2003). Proximate factors influencing soldier development in the basal termite Cryptotermes secundus (Hill). Insectes Soc. 50, 299–303. doi: 10.1007/s00040-003-0674-4
Korb, J., Weil, T., Hoffmann, K., Foster, K. R., and Rehli, M. (2009). A gene necessary for reproductive suppression in termites. Science 324, 758–758. doi: 10.1126/science.1170660
Langmead, B., Trapnell, C., Pop, M., and Salzberg, S. L. (2009). Ultrafast and memory-efficient alignment of short DNA sequences to the human genome. Genome Biol. 10:R25. doi: 10.1186/gb-2009-10-3-r25
Li, B., and Dewey, C. N. (2011). RSEM: accurate transcript quantification from RNA-Seq data with or without a reference genome. BMC Bioinformatics 12:323. doi: 10.1186/1471-2105-12-323
Maekawa, K., Hayashi, Y., and Lo, N. (2022). Termite sociogenomics: evolution and regulation of caste-specific expressed genes. Curr. Opin. Insect Sci. 50:100880. doi: 10.1016/j.cois.2022.100880
Martin, M. (2011). Cutadapt removes adapter sequences from high-throughput sequencing reads. EMBnet J. 17, 10–12. doi: 10.14806/ej.17.1.200
Masuoka, Y., and Maekawa, K. (2016). Ecdysone signaling regulates soldier-specific cuticular pigmentation in the termite Zootermopsis nevadensis. FEBS Lett. 590, 1694–1703. doi: 10.1002/1873-3468.12219
Masuoka, Y., Miyazaki, S., Saiki, R., Tsuchida, T., and Maekawa, K. (2013). High Laccase2 expression is likely involved in the formation of specific cuticular structures during soldier differentiation of the termite Reticulitermes speratus. Arthropod Struct. Dev. 42, 469–475. doi: 10.1016/j.asd.2013.08.003
Masuoka, Y., Yaguchi, H., Toga, K., Shigenobu, S., and Maekawa, K. (2018). TGFβ signaling related genes are involved in hormonal mediation during termite soldier differentiation. PLoS Genet. 14:e1007338. doi: 10.1371/journal.pgen.1007338
McCarthy, D. J., Chen, Y., and Smyth, G. K. (2012). Differential expression analysis of multifactor RNA-Seq experiments with respect to biological variation. Nucleic Acids Res. 40, 4288–4297. doi: 10.1093/nar/gks042
Mitaka, Y., Mori, N., and Matsuura, K. (2017). Multi-functional roles of a soldier-specific volatile as a worker arrestant, primer pheromone and an antimicrobial agent in a termite. Proc. R. Soc. B. 284:20171134. doi: 10.1098/rspb.2017.1134
Miura, T. (2005). Developmental regulation of caste-specific characters in social-insect polyphenism. Evol. Dev. 7, 122–129. doi: 10.1111/j.1525-142X.2005.05014.x
Miura, T., and Maekawa, K. (2020). The making of the defensive caste: physiology, development and evolution of the soldier differentiation in termites. Evol. Dev. 22:e12335. doi: 10.1111/ede.12335
Miura, T., Kamikouchi, A., Sawata, M., Takeuchi, H., Natori, S., Kubo, T., et al. (1999). Soldier caste-specific gene expression in the mandibular glands of Hodotermopsis japonica (Isoptera: Termopsidae). Proc. Natl. Acad. Sci. U S A. 96, 13874–13879. doi: 10.1073/pnas.96.24.13874
Miyazaki, S., Fujiwara, K., Kai, K., Masuoka, Y., Gotoh, H., Niimi, T., et al. (2021). Evolutionary transition of doublesex regulation from sex-specific splicing to male-specific transcription in termites. Sci. Rep. 11:15992. doi: 10.1038/s41598-021-95423-7
Nijhout, H. F. (2003). Development and evolution of adaptive polyphenisms. Evol. Dev. 5, 9–18. doi: 10.1046/j.1525-142X.2003.03003.x
Noirot, C. (1991). Caste differentiation in Isoptera: basic features, role of pheromones. Ethol. Ecol. Evol. Spec. Issue 1, 3–7. doi: 10.1080/03949370.1991.10721899
Noriega, F. G., Ribeiro, J. M. C., Koener, J. F., Valenzuela, J. G., Hernandez-Martinez, S., Pham, V. M., et al. (2006). Comparative genomics of insect juvenile hormone biosynthesis. Insect Biochem. Mol. Biol. 36, 366–374. doi: 10.1016/j.ibmb.2006.01.013
Park, Y. I., and Raina, A. K. (2005). Regulation of juvenile hormone titers by soldiers in the Formosan subterranean termite, Coptotermes formosanus. J. Insect Physiol. 51, 385–391. doi: 10.1016/j.jinsphys.2005.02.001
Qiu, Y., Tittiger, C., Wicker-Thomas, C., Le Goff, G., Young, S., Wajnberg, E., et al. (2012). An insect-specific P450 oxidative decarbonylase for cuticular hydrocarbon biosynthesis. Proc. Natl. Acad. Sci. U S A. 109, 14858–14863. doi: 10.1073/pnas.1208650109
Roberts, A., Trapnell, C., Donaghey, J., Rinn, J. L., and Pachter, L. (2011). Improving RNA-Seq expression estimates by correcting for fragment bias. Genome Biol. 12:R22. doi: 10.1186/gb-2011-12-3-r22
Robinson, M. D., and Oshlack, A. (2010). A scaling normalization method for differential expression analysis of RNA-seq data. Genome Biol. 11:R25. doi: 10.1186/gb-2010-11-3-r25
Robinson, M. D., McCarthy, D. J., and Smyth, G. K. (2010). edgeR: a Bioconductor package for differential expression analysis of digital gene expression data. Bioinformatics 26, 139–140. doi: 10.1093/bioinformatics/btp616
Roisin, Y. (2000). “Diversity and evolution of caste patterns,” in Diversity and Evolution of Caste Patterns, eds T. Abe, D. E. Bignell, and M. Higashi (Dordrecht: Kluwer Academic Publishers), 95–119.
Shigenobu, S., Hayashi, Y., Watanabe, D., Tokuda, G., Hojo, M. Y., Toga, K., et al. (2022). Genomic and transcriptomic analyses of the subterranean termite Reticulitermes speratus: gene duplication facilitates social evolution. Proc. Natl. Acad. Sci. U S A. 119:e2110361119. doi: 10.1073/pnas.2110361119
Storey, J. D. (2002). A direct approach to false discovery rates. J. R. Stat. Soc. B. 64, 479–498. doi: 10.1111/1467-9868.00346
Sugime, Y., Oguchi, K., Gotoh, H., Hayashi, Y., Matsunami, M., Shigenobu, S., et al. (2019). Termite soldier mandibles are elongated by dachshund under hormonal and Hox gene controls. Development 146:dev171942. doi: 10.1242/dev.171942
Takematsu, Y. (1992). Biometrical study on the development of the castes in Reticulitermes speratus (Isoptera, Rhinotermitidae). Jpn. J. Ent. 60, 67–76.
Tarver, M. R., Schmelz, E. A., and Scharf, M. E. (2011). Soldier caste influences on candidate primer pheromone levels and juvenile hormone-dependent caste differentiation in workers of the termite Reticulitermes flavipes. J. Insect Physiol. 57, 771–777. doi: 10.1016/j.jinsphys.2011.02.015
Tarver, M. R., Schmelz, E. A., Rocca, J. R., and Scharf, M. E. (2009). Effects of soldier-derived terpenes on soldier caste differentiation in the termite Reticulitermes flavipes. J. Chem. Ecol. 35, 256–264. doi: 10.1007/s10886-009-9594-8
Toga, K., Hojo, M., Miura, T., and Maekawa, K. (2012). Expression and function of a limb-patterning gene Distal-less in the soldier-specific morphogenesis in the nasute termite Nasutitermes takasagoensis. Evol. Dev. 14, 286–295. doi: 10.1111/j.1525-142X.2012.00545.x
Trapnell, C., Hendrickson, D. G., Sauvageau, M., Goff, L., Rinn, J. L., and Pachter, L. (2013). Differential analysis of gene regulation at transcript resolution with RNA-seq. Nat. Biotechnol. 31, 46–53. doi: 10.1038/nbt.2450
Trapnell, C., Williams, B. A., Pertea, G., Mortazavi, A., Kwan, G., van Baren, M. J., et al. (2010). Transcript assembly and quantification by RNA-Seq reveals unannotated transcripts and isoform switching during cell differentiation. Nat. Biotechnol. 28, 511–515. doi: 10.1038/nbt.1621
Tsuchiya, M., Watanabe, D., and Maekawa, K. (2008). Effect on mandibular length of juvenile hormones and regulation of soldier differentiation in the termite Reticulitermes speratus (Isoptera: Rhinotermitidae). App. Entomol. Zool. 43, 307–314. doi: 10.1303/aez.2008.307
Tsunoda, K., Doki, H., and Nishimoto, K. (1986). Effect of developmental stages of workers and nymphs of Reticulitermes speratus (Kolbe) (Isoptera: Rhinotermitidae) on caste differentiation induced by JHA treatment. Mater. Org. 21, 47–61.
Untergasser, A., Nijveen, H., Rao, X., Bisseling, T., Greurts, R., and Leunissen, J. A. M. (2007). Primer 3 Plus, an enhanced web interface to Primer 3. Nucleic Acids Res. 35, 71–74. doi: 10.1093/nar/gkm306
Vandesompele, J., De Preter, K., Pattyn, F., Poppe, B., Van Roy, N., De Paepe, A., et al. (2002). Accurate normalization of real-time quantitative RT-PCR data by geometric averaging of multiple internal control genes. Genome Biol. 3, 1–12. doi: 10.1186/gb-2002-3-7-research0034
Watanabe, D., Gotoh, H., Miura, T., and Maekawa, K. (2011). Soldier presence suppresses presoldier differentiation through a rapid decrease of JH in the termite Reticulitermes speratus. J. Insect Physiol. 57, 791–795. doi: 10.1016/j.jinsphys.2011.03.005
Watanabe, D., Gotoh, H., Miura, T., and Maekawa, K. (2014). Social interactions affecting caste development through physiological actions in termites. Front. Physiol. 5:127. doi: 10.3389/fphys.2014.00127
Watanabe, H., and Tokuda, G. (2010). Cellulolytic systems in insects. Annu. Rev. Entomol. 55, 609–632. doi: 10.1146/annurev-ento-112408-085319
Wheeler, D. E., and Nijhout, H. F. (1984). Soldier determination in Pheidole bicarinata: inhibition by adult soldiers. J. Insect Physiol. 30, 127–135.
Wu, T., Dhami, G. K., and Thompson, G. J. (2018). Soldier-biased gene expression in a subterranean termite implies functional specialization of the defensive caste. Evol. Dev. 20, 3–16. doi: 10.1111/ede.12243
Yu, G., Wang, L. G., Han, Y., and He, Q. Y. (2012). clusterProfiler: an R package for comparing biological themes among gene clusters. OMICS J. Integr. Biol. 16, 284–287. doi: 10.1089/omi.2011.0118
Keywords: caste differentiation, soldiers, workers, transcriptome, juvenile hormone, Reticulitermes
Citation: Matsunami M, Watanabe D, Fujiwara K, Hayashi Y, Shigenobu S, Miura T and Maekawa K (2022) Transcriptomics on Social Interactions in Termites: Effects of Soldier Presence. Front. Ecol. Evol. 10:924151. doi: 10.3389/fevo.2022.924151
Received: 20 April 2022; Accepted: 23 May 2022;
Published: 27 June 2022.
Edited by:
Alberto Arab, Federal University of ABC, BrazilReviewed by:
Ives Haifig, Federal University of ABC, BrazilBitao Qiu, University of Copenhagen, Denmark
Copyright © 2022 Matsunami, Watanabe, Fujiwara, Hayashi, Shigenobu, Miura and Maekawa. This is an open-access article distributed under the terms of the Creative Commons Attribution License (CC BY). The use, distribution or reproduction in other forums is permitted, provided the original author(s) and the copyright owner(s) are credited and that the original publication in this journal is cited, in accordance with accepted academic practice. No use, distribution or reproduction is permitted which does not comply with these terms.
*Correspondence: Kiyoto Maekawa, kmaekawa@sci.u-toyama.ac.jp
†These authors have contributed equally to this work and share first authorship