- 1Liziping Giant Panda’s Ecology and Conservation Observation and Research Station of Sichuan Province (Science and Technology Department of Sichuan Province), China West Normal University, Nanchong, China
- 2Key Laboratory of Southwest China Wildlife Resources Conservation (Ministry of Education), China West Normal University, Nanchong, China
- 3Chongqing Zoo, Chongqing, China
- 4Science and Technology Division, China West Normal University, Nanchong, China
The intestinal microflora of animals plays a key role in metabolism, immunity, and development. Birds distributed across multiple ecological habitats. However, little is known about the differences in the intestinal microflora of birds among different ecological types. In this study, bird feces from different ecological types and orders were collected in Chongqing Zoo, China. In this study, high throughput sequencing of the 16S ribosomal RNA (rRNA) gene (amplicon sequencing) and metagenomics were used to analyze the composition and function differences of gut microbiota communities among different ecological types/orders. Firmicutes and Proteobacteria were the dominant bacteria phyla for all samples but there were significant differences in the α-diversity, community structure and microbial interactions between birds of different ecological types. The function differences involve most aspects of the body functions, especially for environmental information processing, organismal systems, human diseases, genetic information processing, and metabolism. These results suggest that diet and habitat are potential drivers of avian gut microbial aggregation. This preliminary study is of great significance for further research on the intestinal microflora of different ecological types of birds.
Introduction
The gastrointestinal tract of an animal is colonized by a complex and abundant ensemble of microbes (Ley et al., 2008). The study of microbiotas associated with different environments, including animal hosts, has become one of the most fruitful areas of biological research during the last decade (Thompson et al., 2017; Parfrey et al., 2018; Moran et al., 2019). The crucial functions performed by these microbes on behalf of their host also have been revealed, showing that gut microbiota may perform important roles in metabolism regulation (Flint et al., 2012), immune defense (Nicholson et al., 2012), normal host development (Browne et al., 2017), brain physiology and behavior (Sherwin et al., 2019), and nutrient absorption (Clemente et al., 2012). The microbiota may even influence mate selection and induce hybrid inviability (Sharon et al., 2010; Brucker and Bordenstein, 2013). In vertebrates, diet (Candela et al., 2012) and lifestyle (Nicholson et al., 2012) may affect the structure of the host’s gut microbiota. Overall, studies have shown that the gut microbiota of avian hosts is largely determined by the host species (Yang et al., 2016). However, environmental factors are also important drivers and can play a vital role in shaping the characteristics of gut microbes (Chen et al., 2017; Grond et al., 2019).
Birds are a distinct species of evolutionary life because they have unique life history characteristics and development strategies compared to other vertebrates. Birds harbor diverse microbes within their guts just like other vertebrates, with the dominant phyla of Firmicutes, Proteobacteria, Actinobacteria, and Bacteroidetes (Kohl, 2012; Waite and Taylor, 2014). However, the gut microbial community structures of birds differ significantly from those of other vertebrates (Waite and Taylor, 2015). These differences may reflect the distinctive morphological characteristics and high-energy requirements involved in supporting the power of flight in birds (Kohl, 2012). For instance, avian species are generally characterized by short gastrointestinal tracts, high body temperature and short food retention time (Barnes, 1972). Birds have great value, including scientific research value, economic value, ornamental value, etc. However, the research on bird gut microbiota is lagging behind that for many other vertebrates; most studies on microbiomes have focused on human and husbandry hosts (Rosenberg and Gophna, 2011), commercially important species such as turkeys (Danzeisen et al., 2015; Kursa et al., 2021), chickens (Ngunjiri et al., 2019; Juricova et al., 2021), geese (Hermier et al., 1994; Qin et al., 2012), and endemic wildlife species such as penguins (Dewar et al., 2013), scavenging vultures (Roggenbuck et al., 2014) and the critically endangered kakapo (Waite et al., 2012).
Birds are mobile organisms, which—depending on the species—require a wide variety of habitats on different spatial scales (Angelstam et al., 2004) and often in groups of mixed species, resulting in overlapping foraging sites (Grond et al., 2014; Ryu et al., 2014). Studies have shown that different hosts can spread gut microbes in a shared environment through physical contact, air, water, soil, food, and other media (Grond et al., 2014; Alm et al., 2018; Li et al., 2021). Therefore, gut microbes can spread from one host to another, instead of vertically spreading in the host (Groussin et al., 2017; Garud et al., 2019). This is particularly relevant for mixed populations with overlapping ecological niches, which can enhance the connection between microbial communities and bacterial cross-species levels due to the sharing of habitats and food resources (van Veelen et al., 2017; Perofsky et al., 2019). This can create similarities in gut bacterial diversity. In particular, migratory birds increase the chance of different pathogens being carried from different regions while also increasing the diversity of intestinal microbes (Huang et al., 2014). However, the influence of different modes of exposure of birds on their intestinal microbial structure is still relatively unknown. This was the focus of the present study due to its great significance to bird protection and infectious disease prevention.
In this study, taking Chongqing Zoo as the research area, differences in the intestinal microbes of birds of different ecological types in this area were investigated through high-throughput amplicon and metagenomics sequencing. The specific goals were as follows: (i) to compare the intestinal microbial composition and community diversity of birds of different ecological types; (ii) to compare the intestinal microbial composition and community diversity of birds of different orders; and (iii) to reveal the functional differences of intestinal microorganisms in birds of different ecological types and orders. Determining the gut microbiota associated with the lifestyles of different birds could provide an important indicator of bird lifestyle and health, as well as insights into the characteristics of the intestinal microbiota of different birds. This study will enhance basic research on birds and has implications for the protection of endangered species, providing a reference for avian disease prevention and control management.
Materials and methods
Study area
Chongqing Zoo is located at No. 1 Xijiao Village, Jiulongpo District, Chongqing, China and covers 43.5 hectares. Chongqing is in southwestern China and at the upper reaches of the Yangtze River. It straddles the transition zone between the Qinghai-Tibet Plateau and the plains of the middle and lower reaches of the Yangtze River between 105°11′–110°11′ east and 28°10′–32°13′ north. Chongqing has a humid subtropical monsoon climate with an average annual temperature of 16–18°C, an average temperature of 26–29°C in the hottest month and an average temperature of 4–8°C in the coldest month. The average annual rainfall is relatively abundant, with 1,000–1,350 mm in most areas. Most of the precipitation is concentrated from May to September, accounting for about 70% of the total annual rainfall. According to the classification of animals, the park is divided into eight functional areas: children’s play area, bird area, central recreation area, primate area, raptor area, herbivore area, popular science area, and back mountain recreation area. There are 11 raptor venues.
Chongqing zoo has more than 4,000 wild animals from more than 260 species on display, with rare and protected animals accounting for more than 80%. Including pisces, amphibia, reptile, aves, and mammalia. The present study focused on nine avian orders: Galliformes, Ciconiiformes, Gruiformes, Anseriformes, Pelecaniformes, Phoenicopteriformes, Passeriformes, Bucerotiformes, and Psittaciformes. According to their habitat and diet at the zoo, these species were divided into three ecological types: waterfowl, terrestrial, and arboreal (Supplementary Table 1).
Sample collection and DNA extraction
Sampling was conducted from July to August 2020. Bird feces were collected in 2.0 ml (smaller birds) and 5.0 ml (larger birds) centrifuge tubes. Each sample was labeled with the bird species and the date of collection, and then the day’s samples were packed in a sealed bag. The samples were transported to the laboratory within 2 h and stored at −80°C.
DNA extraction was performed using the E.Z.N.A. Stool DNA Kit (Omega Bio-Tek, United States) according to the manufacturer’s instructions with slight modifications. Glass beads (200 mg) and SLX Mlus buffer (540 μl) were added to the sample and the cells were lysed at 65 Hz for 90 s. The stool samples were then disposed of immediately according to the instructions in the kit manual. The total DNA was eluted from the column with 50 μl elution buffer twice in succession. Finally, the purity and concentration of DNA were determined by spectrophotometer (ND-2000C).
16S rRNA gene V3-V4 amplification, quantification and sequencing
The universal bacterial primers 338F (5′-ACTCCTACGGAGGGCAG-3′) and 806R (5′-GGACATCHVGGTWTTCTAAT-3′) were used to target the V3-V4 region of 16s rRNA gene for the establishment of bacterial library (Caporaso et al., 2011). The PCR program conditions were as follows: initial denaturation at 95°C for 3 min; 35 cycles of denaturing at 95°C for 30 s, 30 s at 55°C, and 45 s at 72°C; and a final extension at 72°C for 10 min. Samples for each specific error correction 12-bp barcode primers used to allow the multiplexing of the sample. The PCR products of all samples were quantified by PicoGreen dsDNA assay and pooled together in equimolar concentrations. Each amplification product was submitted to Mega Biotech on the Illumina MiSeq PE300 platform. The raw reads were deposited in the NCBI Sequence Read Archive (SRA) database under the registration numbers PRJNA784649 and PRJNA861449.
Sequence data analysis
The raw 16S rRNA gene sequencing reads were demultiplexed, quality-filtered by fastp version 0.20.0 (Chen et al., 2018) and merged by FLASH version 1.2.7 (Magoè and Salzberg, 2011) with the following criteria: (i) truncate 300 bp reads at any site with an average quality score of <20 within a 50 bp sliding window, and discard truncated reads shorter than 50 bp. Reads containing ambiguous characters were also discarded; (ii) only overlapping sequences exceeding 10 bp were assembled according to their overlapped sequence, the maximum mismatch ratio of the overlap region was 0.2. Reads that could not be assembled were discarded; and (iii) distinguish samples based on barcode and primers. Sequence direction was adjusted using exact barcode matching with two nucleotide mismatches in primer matching.
Based on a 97% similarity cut-off (Stackebrandt and Goebel, 1994; Edgar, 2013), operational taxonomic units (OTUs) were clustered with UPARSE version 7.1, and chimeric sequences were identified and removed. The classification of each OTU representative sequence was analyzed by RDP Classifier version 2.2 (Wang et al., 2007) against the 16S rRNA database (e.g., Silva v138) using a confidence threshold of 0.7. QIIME also removed non-target sequences, including mitochondrial and chloroplast sequences, from the final OTU dataset. To better convey the biological information in these samples, the average relative abundance of bacterial communities was visualized by bar graphs at the phylum and genus levels.
Statistical analysis
First, Kruskal–Wallis rank-sum tests were used to evaluate the differences in dominant bacteria abundance at both phylum/genus levels between different ecological types/different orders. Second, the Sobs and Shannon indices of each sample were calculated using mothur version 1.30.1 and the differences in the abundance and diversity among different ecological types/orders were determined using Student’s t-test. Third, PCoA (principal coordinate analysis) based on the Bray–Curtis distance matrix was used to evaluate the differences in intestinal microbial community structure among different types/orders of birds. Finally, the PICRUSt prediction was used to predict the functional composition of all bird gut microbial communities in the different ecological types. The greengene id corresponding to each OTU, the Clusters of Orthologous Groups (COG), and Kyoto Encyclopedia of Genes and Genomes (KEGG) functions of the OTU were annotated to obtain the function level of COG and KEGG, and the abundance information of each function in different samples.
Metagenome sequencing and analysis
On the free online platform of the Majorbio cloud plat form,1 fastp2 (Chen et al., 2018) (version 0.20.0) were used to generate clean reads by removing adapter sequences, modifying and removing low-quality reads (reads with N bases, with a minimum length threshold of 50 bp and a minimum quality threshold of 20), and leveraging the original reads of metagenome sequencing. In order to identify and remove the host-originated reads, BWA3 (Li and Durbin, 2009) (version 0.7.9a) was used to map the clean reads into the host reference genome. MEGAHIT (Li et al., 2015) (parameters: kmer_min = 47, kmer_max = 97, step = 10)4 (version 1.1.2) were used to assemble these high-quality reads into contigs, which employed a concise de Bruijn graphs. The final assembly result selected contigs with the length greater than or equal to 300 bp. Open reading frames (ORFs) in contigs were identified by using MetaGene (Noguchi et al., 2006). A predictive ORF of length equal to or greater than 100 bp of amino acid sequence was translated to amino acid sequence.
A non-redundant gene catalog was constructed by using CD-HIT5 (Fu et al., 2012) (version 4.6.1) with the sequence identity of 90% and the coverage of 90%. By using SOA Paligner (Li et al., 2008) (version 2.21), the quality-controlled Reads were mapped into the non-redundant gene catalog with 95% identity and the genetic abundance of each sample was evaluated. Representative sequences of non-redundant gene catalog were annotated on the NCBI NR database and the blastp implemented by DIAMOND v0.9.19 was annotated, where the e-value intercept of le-5 was annotated by Diamnd6 (Buchfink et al., 2015) (version 0.8.35). For functional analyses, KEGG annotation was conducted by using Diamond (Buchfink et al., 2015) (version 0.8.35) to annotate the Kyoto Encyclopedia of Genes and Genomes database7 (version 94.2) with an e-value cutoff of 1e-5. Carbohydrate-active enzymes annotation was conducted by leveraging hmmscan to annotate CAZy database8 with an e-value cutoff of 1e-5. Utilizing linear discriminant to analyze (LDA) effect quantity (LEfSe), the different characteristics of KEGG Level 2 and CAZyme were determined with two as the threshold of logarithmic LDA score.
Results
Samples, sequences and operational taxonomic units between different ecological types/orders
After removing the mitochondrial and chloroplast sequences, a total of 3,018,312 effective target 16S rRNA reads were obtained (Supplementary Table 2). The length of the sequence obtained from the sample is mainly distributed in 401–440 bp, with an average length of 419 bp.
According to 97% similarity, non-repetitive sequences were clustered by OTU, and a total of 4,444 OTUs were obtained. There are 1,857 OTUs in waterfowl birds, 1,778 OTUs existing in terrestrial birds, and 3,261 OTUs in arboreal birds. Venn diagrams showed that there were 668 OTUs shared among waterfowl, terrestrial, and arboreal birds (Figure 1A) and 13 OTUs shared among 9 orders (Figure 1B). In the orders Ciconiiformes, Gruiformes, Galliformes, Passeriformes, Bucerotiformes, Anseriformes, Psittaciformes, Pelecaniformes, and Phoenicopteriformes, the number of OTUs were 67, 119, 2295, 614, 253, 182, 216, 1,015, and 17, respectively.
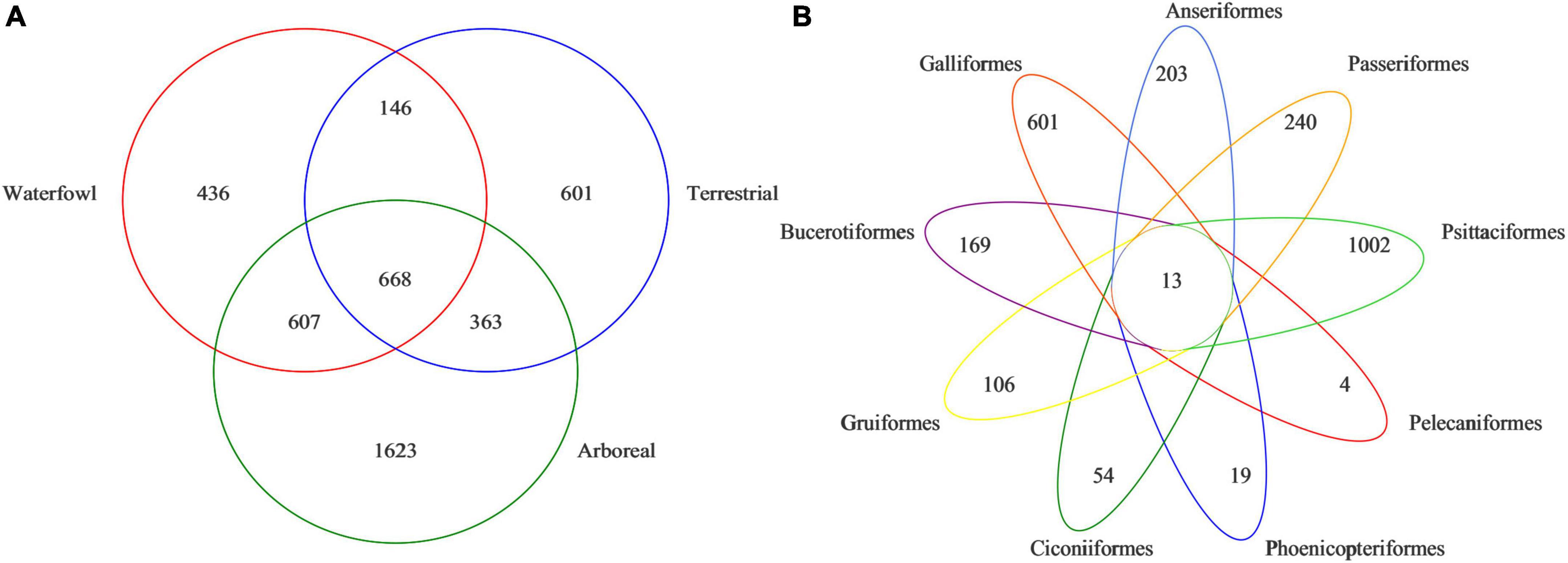
Figure 1. Venn diagram showing overlap/non-overlap of operational taxonomic units (OTUs) between the (A) three ecological types and (B) nine orders.
Intestinal microbial composition among different ecological types/orders
The two main phyla were Firmicutes (50.58%) and Proteobacteria (29.95%) (Figure 2). The remaining phyla with a relative abundance of greater than 1% were Bacteroidota (7.82%), Fusobacteria (4.22%), Actinobacteria (4.01%) and Campilobacterota (1.15%). Bacteria with a relative abundance of less than 1% and no annotation results were classified as “others.” There were significant differences between the three ecological types (Figure 2B). For example, the average relative abundance of Proteobacteria in terrestrial birds (16.94%) was significantly lower than that in waterfowl birds (37.06%) and arboreal birds (33.55%), and the average relative abundance of Fusobacteriota and Campilobacterota in waterfowl birds (10.03 and 2.75%, respectively) was significantly higher than that in terrestrial birds (0.11 and 0.02%, respectively) and arboreal birds (0.28 and 0.05%, respectively).
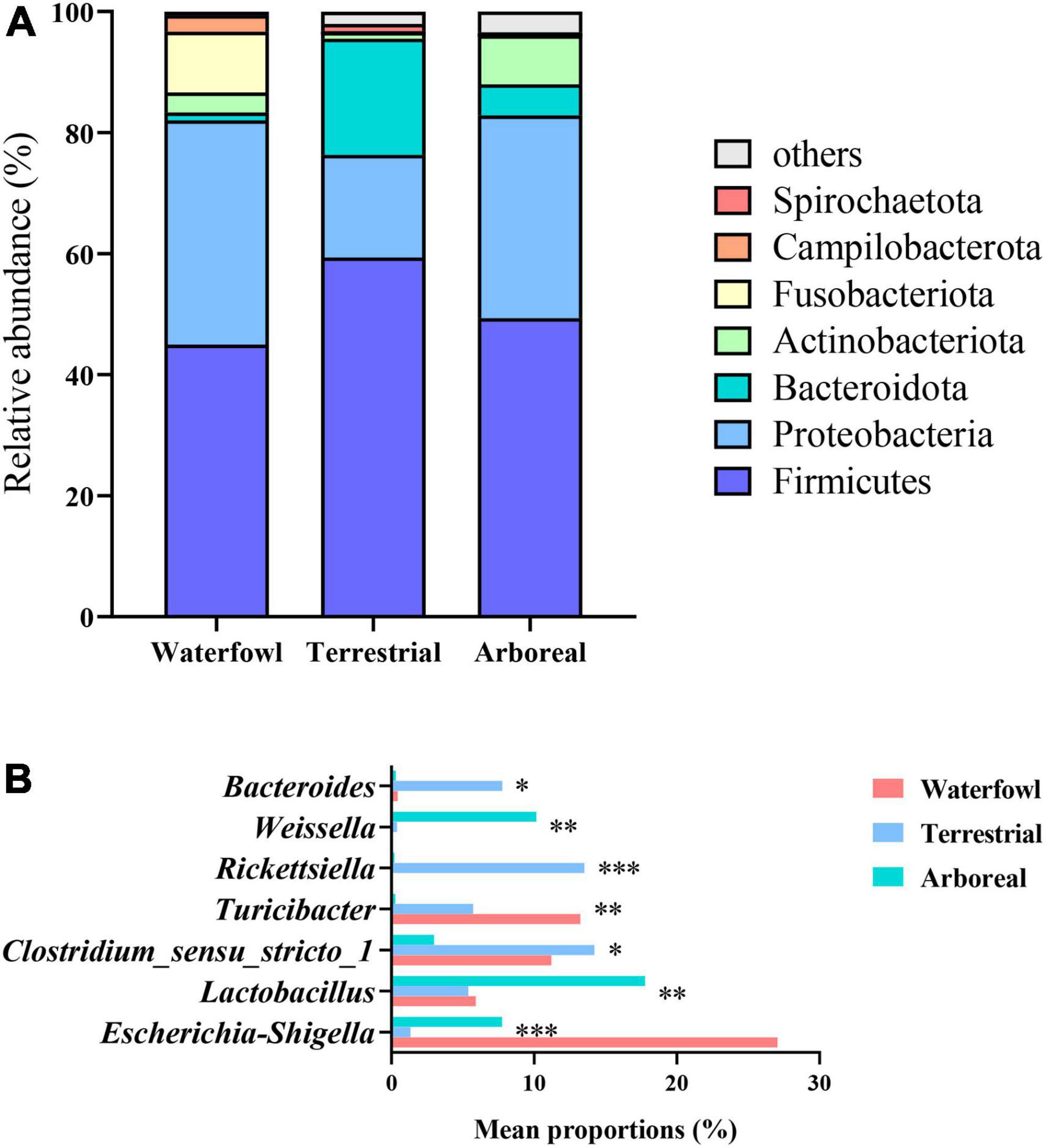
Figure 2. Relative abundance of microbiota composition in the bird gut at the phylum level between the different ecological types of birds. (A) Microbiota composition for different ecological types; (B) differences in microbiota composition among different ecological types. (*0.01 < P < 0.05, **0.001 < P < 0.01, ***P < 0.001).
There were also significant differences in the relative abundance of bacterial phylum between different orders of birds (Supplementary Figure 1). For example, the average relative abundance of Firmicutes (4.10%) and Proteobacteria (10.94%) in Phoenicopteriformes was significantly lower than that in other orders. The average relative abundance of Fusobacteriota in Phoenicopteriformes (83.03%) was significantly higher than that in other orders and the average relative abundance of Actinobacteriota (16.99%) in Bucerotiformes was also significantly higher than that in other orders. The average relative abundance of Campilobacterota in Anseriformes was significantly higher than that in other orders.
A total of 1,229 genera were identified in the samples. Bacteria with a relative abundance of less than 1% and without annotation results at this level were attributed to “others,” which accounted for 31.73% of all genera. The dominant bacterial genera were Escherichia–Shigella (13.71%), Clostridium_sensu_stricto_1 (9.75%), Lactobacillus (9.17%), and Turicibacter (7.23%). There were differences between the three ecological types (Supplementary Figure 2). For example, the relative abundance of Escherichia–Shigella was highest in waterfowl birds and lowest in terrestrial birds. The relative abundance of Lactobacillus was highest in arboreal birds and lowest in terrestrial birds. The relative abundance of Clostridium_sensu_stricto_1 was highest in terrestrial birds and lowest in arboreal birds. The relative abundance of Turicibacter was highest in waterfowl birds and lowest in arboreal birds.
There were also significant differences in the relative abundance of bacterial genera between different orders of birds (Supplementary Figure 3). For example, the relative abundance of Escherichia–Shigella was highest in Ciconiiformes and lowest in Bucerotiformes. The relative abundance of Cetobacterium was highest in Phoenicopteriformes. The relative abundance of Lactobacillus was highest in Psittaciformes and lowest in Phoenicopteriformes. The relative abundance of Clostridium_sensu_stricto_1 was highest in Ciconiiformes and lowest in Phoenicopteriformes. The relative abundance of Turicibacter was highest in Anseriformes and lowest in Phoenicopteriformes.
Diversity among different ecological types/orders
There was no significant difference in the Sobs index among different ecological types (Figure 3A). However, the Shannon index of waterfowl was significantly lower than that of the other two ecological types (Figure 3B). For different orders, Psittaciformes had the highest Sobs index and Pelecaniformes had the lowest (Figure 3C), while Bucerotiformes had the highest Shannon index and Phoenicopteriformes had the lowest (Figure 3D).
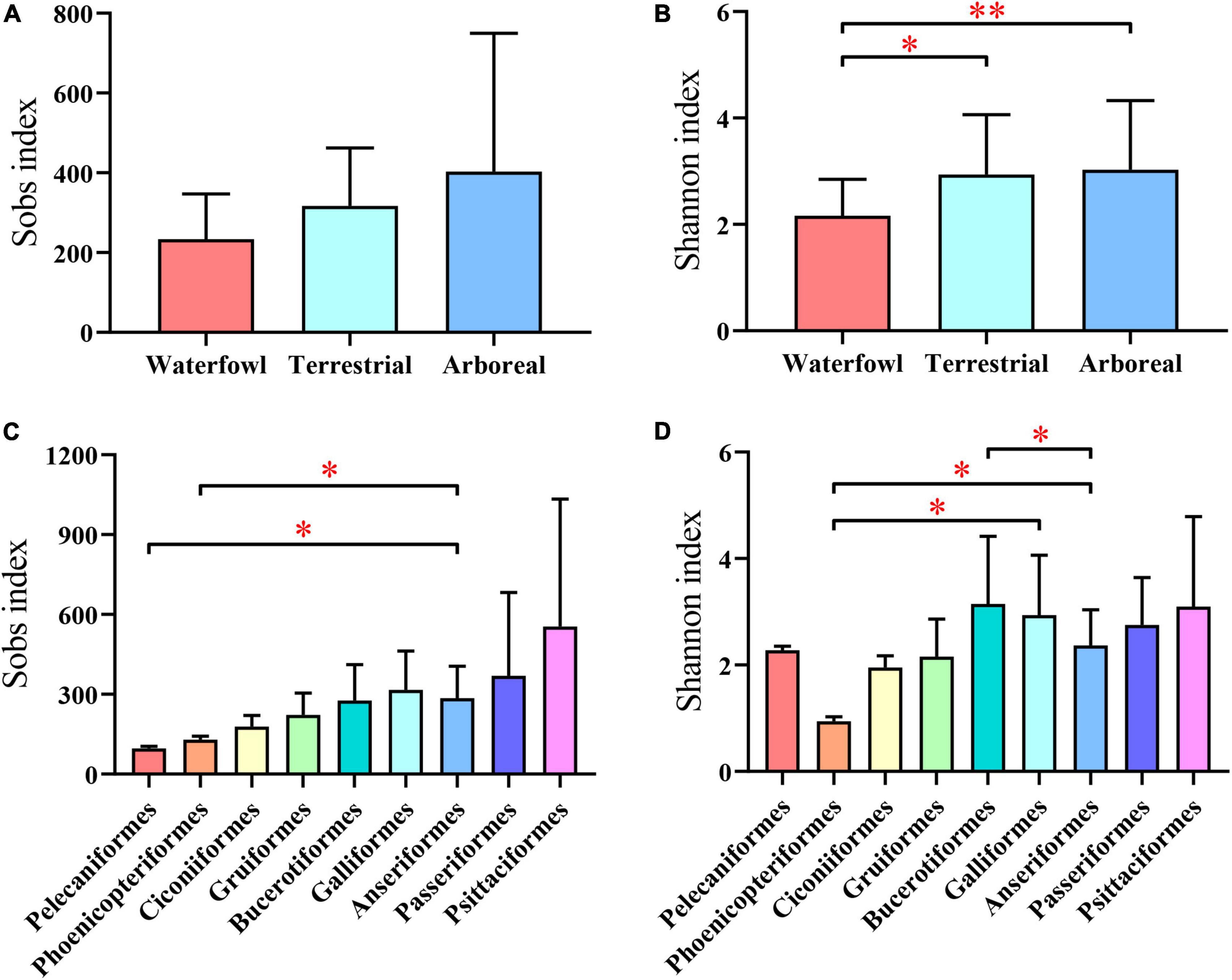
Figure 3. The observed species index (Sobs index) and Shannon index at the OTU level of intestinal communities for (A,B) different ecological types and (C,D) different orders. Student’s t-test was used to test the significance. (*0.01 < P < 0.05, **0.001 < P < 0.01).
The contribution rates of PC1 and PC2 in the principal coordinate analysis based on Bray–Curtis dissimilarity were 16.11 and 13.1%, respectively (Figure 4). There was a significant separation of the three ecological types (Figure 4A). The PERMANOVA analysis revealed significant differences in the intestinal bacterial communities of the three ecological types of birds based on Bray–Curtis distance matrixes (Table 1). From the perspective of birds of different orders, Galliformes and Anseriformes were significantly separated from other orders (Figure 4B).
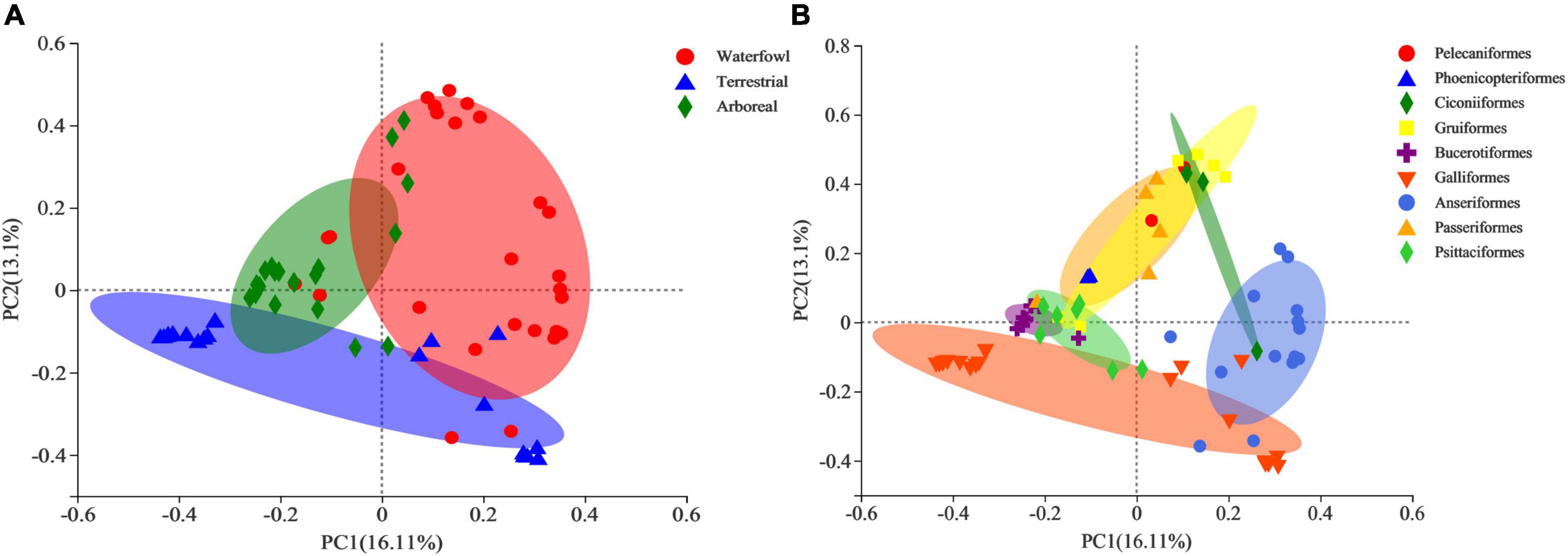
Figure 4. Principal coordinate analysis (PCoA) diagrams based on the Bray–Curtis distance matrix at the operational taxonomic unit (OTU) level for (A) different ecological types and (B) different orders.

Table 1. PERMANOVAs exploring the effects of ecological types on birds intestinal bacteria community based on Bray–Curtis distance matrixes.
Function estimation between different ecological types/orders
By the PICRUSt prediction, similar COG functional classification model was found in three ecology types and nine order bird fecal microbial communities (Supplementary Figure 4). There were higher relative abundance sequences related to amino acid transport and metabolism, translation, ribosomal structure and biogenesis, carbohydrate transport and metabolism, transcription, cell wall/membrane/envelope biogenesis. Prediction software PICRUSt enriched 17 categorizable dominant pathways (relative abundance >1%) at the level 3 KEGG pathway, such as metabolic pathways, biosynthesis of secondary metabolites, microbial metabolism in diverse environments. Among them, 11 pathways had significant differences between different ecological types (P < 0.05) (Figure 5 and Supplementary Table 3). It is worth noting that there were significant differences in the relative abundance of the microbial metabolism in diverse environments, ABC transporters, two-component system, and quorum sensing at KEGG pathway level 3 and the relative abundance of these functions for terrestrial was significantly lower than that of the other two ecological types. Biosynthesis of secondary metabolites, Biosynthesis of amino acids, Carbon metabolism, Pyrimidine metabolism, Aminoacyl-tRNA biosynthesis, and Ribosome at KEGG pathway level 3 was also different among the three ecological types and the relative abundance of these functions for terrestrial was significantly higher than that of the other two ecological types (Figure 5 and Supplementary Table 3).
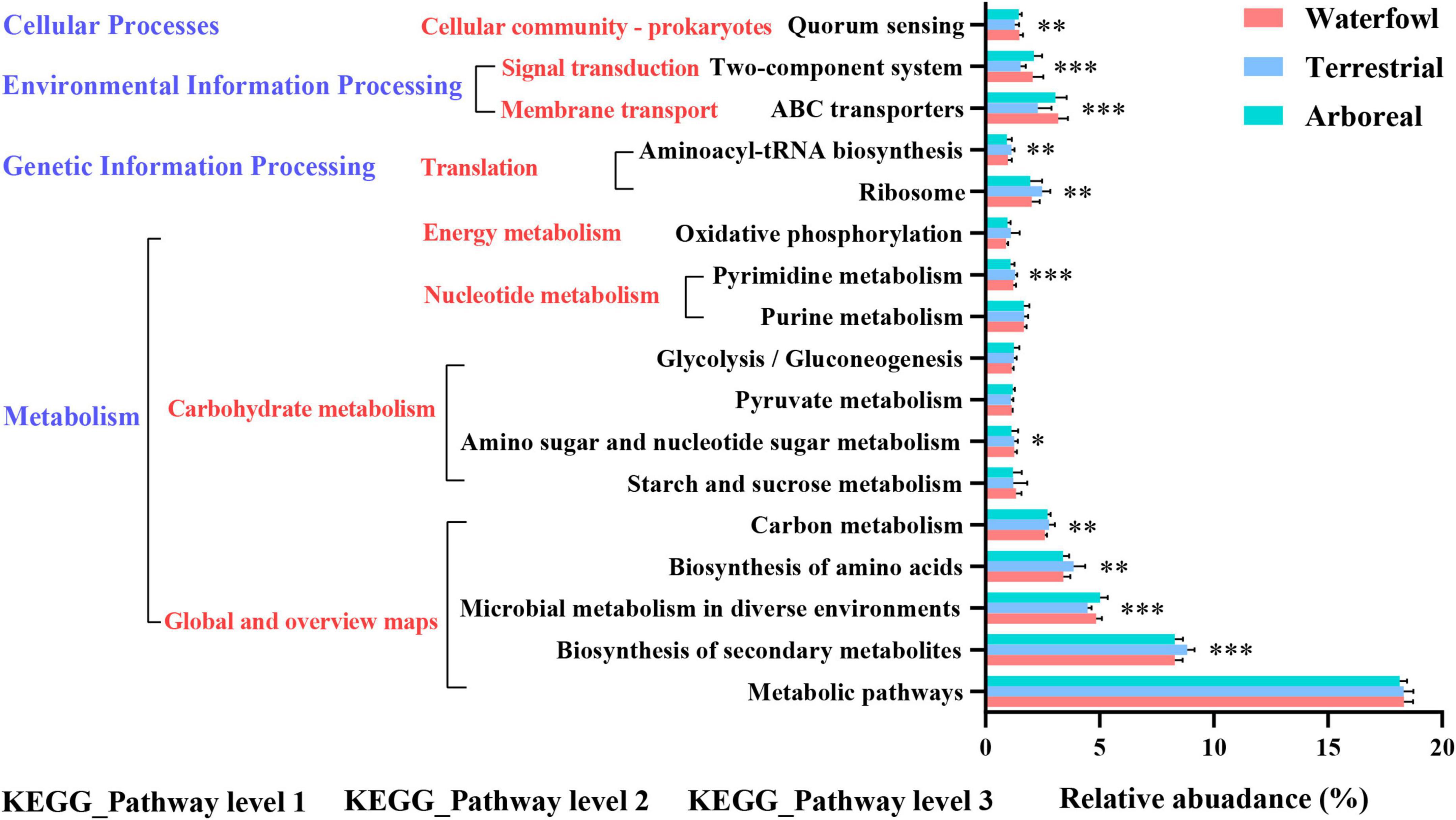
Figure 5. Functional predictions for intestinal microflora with significantly different KEGG pathways (P < 0.05) for the different ecological types of birds. KEGG pathways at Level 1, Level 2, and Level 3 are represented. (*0.01 < P < 0.05, **0.001 < P < 0.01, ***P < 0.001).
In order to explore the potential function of intestinal bacteria in different ecological types of birds, a total of 12 bird fecal samples (waterfowl, four samples; terrestrial, four samples; arboreal, four samples) were used to perform metagenomic sequencing. In total, 3.14 million contigs and 2,642 million bp of assembly sequence were obtained with an average contig N50 of 15,563 bp (Supplementary Table 4). The representative sequences of the non-redundant gene catalog were annotated to obtain five domains, and the bacterial domain was further studied in this paper. The metagenomic analysis confirmed 3,653 KOs. Waterfowl displayed higher abundances in KEGG Level 2 categories of carbohydrate metabolism, cellular community-prokaryotes, xenobiotics biodegradation and metabolism, infectious disease: bacterial, development and regeneration, and excretory system (Figure 6A); Arboreal displayed higher abundances in KEGG Level 2 categories of membrane transport, metabolism of other amino acids, endocrine system, cancer: overview, infectious disease: viral, and infectious disease: parasitic (Figure 6A); Whereas replication and repair, translation, glycan biosynthesis and metabolism, metabolism of cofactors and vitamins, folding, sorting and degradation, biosynthesis of other secondary metabolites, cell growth and death, drug resistance: antimicrobial, environmental adaptation, aging, transport and catabolism, metabolism of terpenoids and polyketides, transcription, endocrine and metabolic disease, immune system, circulatory system, immune disease, and cardiovascular disease exhibited higher abundance in terrestrial groups (Figure 6A). According to the LEfse results of the CAZyme, three families had significantly higher relative abundance in waterfowl than other two ecological types, including glycoside hydrolases (GH1, GH4, GH8, GH15, GH31, GH37, GH13_18, GH13_27, and GH13_29), carbohydrate esterases (CE1 and CE9), and glycosyl transferases (GT8 and GT99); Four families had significantly higher relative abundance in terrestrial, including glycosyl transferases (GT2_Glycos_transf_2, GT4, GT28, GT30, GT41, and GT87), glycoside hydrolases (GH3, GH51, GH78, GH95, GH97, GH129, and GH43_10), carbohydrate esterases (CE11), and auxiliary activities (AA6); Three families had significantly higher relative abundance in arboreal, including glycoside hydrolases (GH25, GH32, GH65, GH73, GH103 and GH13_31), carbohydrate esterases (CE7 and CE10), and auxiliary activities (AA3 and AA4) (Figure 6B).
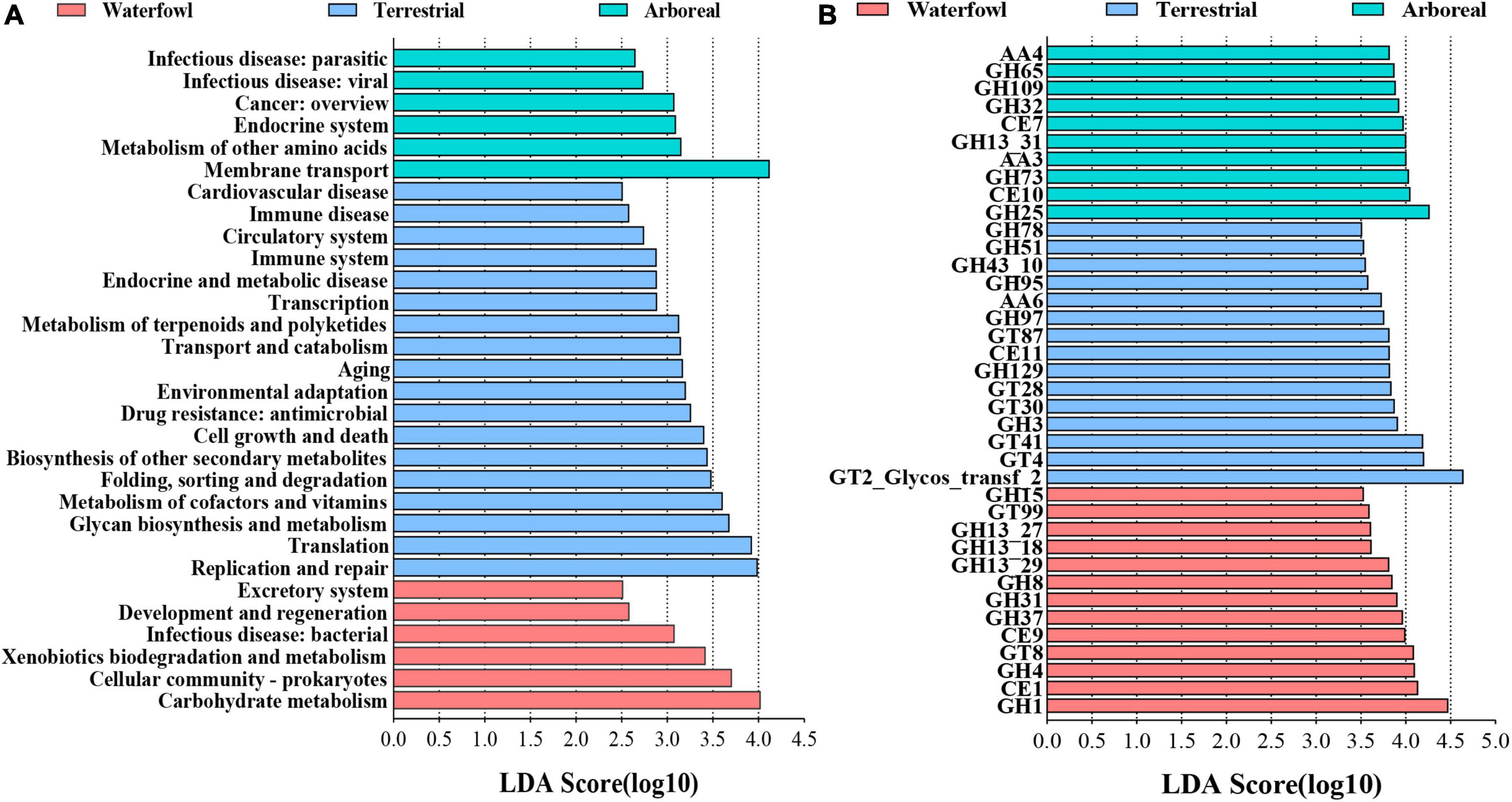
Figure 6. Linear discriminant analysis (LDA) effect size (LEfSe) analysis of KEGG level 2 (A) and CAZy (B) between the different ecological types of birds. GT, glycosyl transferases; CE, carbohydrate esterases; GH, glycoside hydrolases; AA, auxiliary activities. LDA >2.
Discussion
Birds are mobile organisms and different species require a wide variety of habitats at different spatial scales (Angelstam et al., 2004). To date, there has been little attention to the differences in intestinal microbiota among different ecological types and orders of birds. In this study, the composition and structure of intestinal microbial communities in birds of different ecological types and orders were investigated. Significant differences in the gut microbes of three different ecological types were observed. However, functional analysis showed that diverse gut microbiota performed similar functions despite differences in taxonomic composition.
The intestinal microbiota of waterfowl and terrestrial of birds was mainly Firmicutes and Proteobacteria, which were consistent with the findings of previous bird studies (Waite and Taylor, 2014). However, the gut microbes of terrestrial birds are mainly Firmicutes and Bacteroidota. The most populous phylum was Firmicutes, which included species that catabolize complex carbohydrates, polysaccharides, sugars, and fatty acids to provide an energy source for the host (Flint et al., 2008; Tap et al., 2009). Proteobacteria, Fusobacteriota, Campilobacterota, and Spirochaetota phylum were significantly different among the three types of birds (Figure 2B). Proteobacteria are a bacterial classification that is considered to have highly complex functions (Kersters et al., 2006). The relative abundance of Fusobacteriota and Campilobacterota in waterfowl was significantly higher than that of other groups. Cetobacterium is the most abundant in Fusobacteriota, which can improve the utilization rate of carbohydrates by fish (Wang et al., 2021). Studies have also shown that Fusobacteriota species can produce butyrate to promote the accumulation of body fat and increases immunity (Panda et al., 2009). The phylum Fusobacteria has also been found in other birds, such as Pygoscelis adeliae (Dewar et al., 2013), Dromaius novaehollandiae (Bennett et al., 2013), and Aegypius monachus (Roggenbuck et al., 2014). Among different orders of birds, Fusobacteriota and Proteobacteria are the main orders of Phoenicopteriformes; Firmicutes and Bacteroidota are the main orders of Galliformes; Firmicutes and Proteobacteria are the main orders of other orders of birds. The high abundance of Fusobacteriota in Phoenicopteriformes may be in response to the complex diet of this order of birds. Based on this, it is speculated that the relatively high content of Fusobacteriota in Phoenicopteriformes may help to enhance fat accumulation, thereby increasing the survival rate.
At the genus level, the relative abundance of Escherichia–shigella was significantly different (Supplementary Figure 3) in the different ecological types, with a relatively higher abundance in waterfowl. This genus is also present in the gastrointestinal tract of herbivorous carnivores animals, such as red panda (Zeng et al., 2018). Lactobacillus is one of the groups with abundant differences, and the relative abundance in arboreal birds is relatively high. This genus, which is a beneficial commensal for humans and animals, has been studied and used in medicine and the food industry for years. Previous studies have suggested that some species of Lactobacillus are associated with weight gain in human and animal infants (Angelakis and Raoult, 2010; Million et al., 2012). However, some scholars believe that certain types of Lactobacillus are related to weight gain in infancy, but not in adults and animals (Lahtinen et al., 2012). Although the effects of the enrichment of Lactobacillus might be different in infancy and adulthood, it could be inferred that Lactobacillus enrichment could generally improve the gastrointestinal tract and thus protect the gut from pathogens and promote efficient nutrient and energy extraction in the host. In addition, Clostridium_sensu_stricto_1, which was present in high relative abundance, can decompose cellulose and is used as the dominant bacteria in the study of giant panda intestinal microbes. This is related to the fact that giant pandas use bamboo with high cellulose as their main diet (Zhu et al., 2011). At the same time, Cellulosilyticum can also convert cellulose into metabolites. These findings may be related to the complex feeding habits of terrestrial and waterfowl birds.
Although the bird’s intestine contains abundant probiotics, many studies have found that the intestinal microflora of birds also contains potential pathogens. The identification and research of avian enteric pathogens is of great value for understanding bird health and protection of endangered species. For example, by studying the composition of the intestinal microbes of the Strigops habroptila, it was possible to detect potential pathogenic bacteria in the intestine of the bird before its number affects the health of the bird (Waite et al., 2012). In addition, many potential human pathogens have been found in the study of bird intestinal microbes, such as Larus melanocephalus (Camarda et al., 2006), Himantopus himantopus and other waterbirds (Santos et al., 2012; Grond et al., 2014). For example, the Corynebacterium detected in the sample included several species that can cause diseases in mammals and birds (Potti et al., 2002; Hoelzle et al., 2013). Clostridium, Staphylococcus, Enterococcus, Streptococcus, and Paracoccus may cause bird disease and death. Furthermore, we have detected some zoonotic disease pathogens. Campylobacter may cause bacterial gastritis in humans (Whelan et al., 1988), Escherichia–Shigella is a potential human pathogen causing diarrhea (Hermes et al., 2009), and Pseudomonas is an opportunistic human pathogen (Chan et al., 2015). These pathogenic bacteria were present in most birds, but there are significant differences between different types of birds, which indicates that the environment may affect the structure of bird intestinal microbes.
The significant differences in bird gut microbial structure were also reflected in α-diversity, Bray–Curtis clustering and microbial function. There were significant differences in intestinal community structure among the three types of birds. These differences may be reflected in the different sources of food resources and habitat environment of different types of birds (Supplementary Table 1). In the principal coordinate analysis based on Bray–Curtis differences, the separation between different orders of birds was not obvious. Different birds have different eating habits to adapt to different ecological habitats. The results showed that diet can be used as an important predictor of the intestinal microflora of birds, which was inconsistent with previous studies showing that vertical transmission plays an important role in shaping the structure of the host’s intestinal microbe (Abdul Rahman et al., 2015). Yu et al. (2022) demonstrated that there were two gut microbial community types in wild plateau pikas (Ochotona curzoniae), which had different microbial diversity, interactions, and functions. Fan et al. (2022) also showed the composition and functional profiles of gut microbiota in plateau pikas varied among seasons, and environmental factors (especially diet) had profound effects on it. Yan et al. (2022) found that free-living wild camels (Camelus ferus) higher energy utilization efficiency compared to that of captive-living counterparts, therefore enable the host to adjust to a relatively barren field environment. These studies provide the evidence of how external factors affect the intestinal environment of animals. Diet has been identified as an important driver of the gut microbial community structure, such as the unique aggregation for carnivores, herbivores and omnivores (Ley et al., 2008; Li et al., 2021).
The various microbes in the bird gut may perform many functions that are critical to the life of birds. In this study, PICRUSt was used to infer potential 16S rRNA sequenced genetic profiles. The results demonstrated that the most abundant functional categories were associated with amino acid transport and metabolism, translation, ribosomal structure and biogenesis, carbohydrate transport and metabolism, transcription, cell wall/membrane/envelope biogenesis. The importance of carbohydrate metabolism and amino acid metabolism were previously predicted in a meta-analysis of avian microbiota using PICRUSt (Waite and Taylor, 2014); however, the interpretation of the predictive results should be cautious due to the inherent limitations of PICRUSt. For example, the prediction is dependent on reference genomes that are phylogenetically similar to those presented in a community (Langille et al., 2013). As the reference genome sequencing of bird gut microbiota was not as extensive as that for other communities (such as the human microbiota), the prediction accuracy of PICRUSt in birds needs further validation. Furthermore, PICRUSt can only handle OTUs that match the available database, thereby overlooking novel, unstudied OTUs (Langille et al., 2013). Even though some functions could be inferred using predictive methods such as PICRUSt, many of the actual functions of the gut microbiota remain to be uncovered with the help of multiple “omics” approaches (Pérez-Cobas et al., 2013).
Different microbial compositions, diversity, and community structure imply different functional characteristics. Further metagenomic analysis uncovered the significant differences in gut microbial functions (KEGG and Carbohydrate-Active enzymes functions) among birds of different ecological types (Figure 6). Significant differences in pathways among different ecological types of birds can be divided into six categories (environmental information processing, cellular processes, organismal systems, human diseases, Genetic Information Processing, and metabolism). These pathways were significantly enriched in the gut microbiota of terrestrial birds, possibly due to their richer food sources and more diverse environments. A large part of these functions was related to human diseases that have essentially influenced by environmental factors (Kanehisa et al., 2010) or organism systems and played an important role in ensuring the normal function of the host and maintaining a stable state (Xing et al., 2019). In the arboreal, the pathways of membrane transport being more abundant. Higher levels of membrane transport function also indicate higher metabolic levels in arboreal (Kleinzeller, 1981). LEfSE analysis based on the CAZy databases showed that the largest proportion differences among the three types of birds was GH families. GHs had a crucial role in breakdown complex carbohydrates (Lee et al., 2014) and played an indispensable role in processing various exogenous and endogenous glycoconjugate in human gut microbiota (Pellock et al., 2018).
This study confirmed the potential impact of diet on the composition of the microbial community of birds. However, non-invasive fecal sampling poses the risk that samples may not be representative of age, gender or health, and these factors may also affect the composition of animals’ gut microbiota. Therefore, more efforts are needed to fully understand the gut microbiota of different ecological types of birds. Additionally, whether and how these microorganisms directly interact with birds remains unknown. Nevertheless, this study provides a useful reference for further in-depth study of intestinal microflora of different ecological types of birds using multiple methods.
Conclusion
This study showed significant differences in the composition and diversity of the gut microbial community of birds between different ecological types, indicating that diet and habitat are potential drivers of bird gut microbial aggregation. However, despite these differences, the functions of the intestinal flora of different birds were similar and the abundance of genes potentially involved in nutrient metabolism was high. This preliminary study is of great significance to inform further research on the intestinal microflora of birds of different ecological types.
Data availability statement
The datasets presented in this study can be found in online repositories. The names of the repository/repositories and accession number(s) can be found below: https://www.ncbi.nlm.nih.gov/, PRJNA784649; https://www.ncbi.nlm.nih.gov/, PRJNA861449.
Ethics statement
Ethical review and approval was not required for the animal study because this study complies with current Chinese law. Non-invasive sample collection does not involve killing laboratory animals.
Author contributions
MH and JW conceived and designed the study. JW and JL collected data and samples in the field. MH, JW, and JL processed samples in the lab and analyzed the data. JX, MH, JW, and YY wrote the manuscript. All authors contributed to the article and approved the submitted version.
Funding
This work was financially supported by the National Natural Science Foundation of China (31900337), the Second Qinghai-Tibet Plateau Comprehensive Scientific Survey (2019QZKK05010502), the Innovation Team Funds of China West Normal University (KCXTD2022-7), and the Fundamental Research Funds of China West Normal University (20B006).
Acknowledgments
Thanks to all the students who assisted with data collection and the experiments.
Conflict of interest
The authors declare that the research was conducted in the absence of any commercial or financial relationships that could be construed as a potential conflict of interest.
Publisher’s note
All claims expressed in this article are solely those of the authors and do not necessarily represent those of their affiliated organizations, or those of the publisher, the editors and the reviewers. Any product that may be evaluated in this article, or claim that may be made by its manufacturer, is not guaranteed or endorsed by the publisher.
Supplementary material
The Supplementary Material for this article can be found online at: https://www.frontiersin.org/articles/10.3389/fevo.2022.920869/full#supplementary-material
Supplementary Figure 1 | Relative abundance differences of microbiota composition among different birds orders in birds’ gut at phylum level. (A) Microbiota composition among different bird orders; (B) Differences of microbiota composition among different bird orders. (*0.01 < P < 0.05, **0.001 < P < 0.01, ***P < 0.001).
Supplementary Figure 2 | Relative abundance differences of microbiota composition among different ecological types in birds’ gut at genus level. (A) Microbiota composition among different ecological types; (B) Differences of microbiota composition among different ecological types. (*0.01 < P < 0.05, **0.001 < P < 0.01, ***P < 0.001).
Supplementary Figure 3 | Relative abundance differences of the birds’ gut microbiota composition among different orders at genus level. (A) Microbiota composition among different orders; (B) Differences of microbiota composition among different orders. (*0.01 < P < 0.05, **0.001 < P < 0.01, ***P < 0.001).
Supplementary Figure 4 | Histogram of COG function classification of intestinal microorganisms among different ecological types (A) and orders (B).
Supplementary Table 1 | Samples number and diet of each bird orders in three ecological types.
Supplementary Table 2 | The number of reads pre- post- filter Sequences.
Supplementary Table 3 | Functional predictions for intestinal microflora with significantly different KEGG pathways for birds among different ecological types.
Supplementary Table 4 | Metagenomic sequences of ecological types on birds.
Footnotes
- ^ https://cloud.majorbio.com/
- ^ https://github.com/OpenGene/fastp
- ^ http://bio-bwa.sourceforge.net
- ^ https://github.com/voutcn/megahit
- ^ http://www.bioinformatics.org/cd-hit/
- ^ https://github.com/bbuchfink/diamond
- ^ http://www.genome.jp/kegg/
- ^ http://www.cazy.org/
References
Abdul Rahman, N., Parks, D. H., Willner, D. L., Engelbrekson, A. L., Goffredi, S. K., Warnecke, F., et al. (2015). A molecular survey of Australian and North American termite genera indicates that vertical inheritance is the primary force shaping termite gut microbiomes. Microbiome 3:5. doi: 10.1186/s40168-015-0067-8
Alm, E. W., Daniels-Witt, Q. R., Learman, D. R., Ryu, H., Jordan, D. W., Gehring, T. M., et al. (2018). Potential for gulls to transport bacteria from human waste sites to beaches. Sci. Total Environ. 615, 123–130. doi: 10.1016/j.scitotenv.2017.09.232
Angelakis, E., and Raoult, D. (2010). The increase of Lactobacillus species in the gut flora of newborn broiler chicks and ducks is associated with weight gain. PLoS One 5:e10463. doi: 10.1371/journal.pone.0010463
Angelstam, P., Roberge, J. M., Lõhmus, A., Bergmanis, M., Brazaitis, G., Dönz-Breuss, M., et al. (2004). Habitat modelling as a tool for landscape-scale conservation: A review of parameters for focal forest birds. Ecol. Bull. 51, 427–453. doi: 10.2307/20113327
Barnes, E. M. (1972). The avian intestinal flora with particular reference to the possible ecological significance of the cecal anaerobic bacteria. Am. J. Clin. Nutr. 25, 1475–1479. doi: 10.1093/ajcn/25.12.1475
Bennett, D. C., Tun, H. M., Kim, J. E., Leung, F. C., and Cheng, K. M. (2013). Characterization of cecal microbiota of the emu (Dromaius novaehollandiae). Vet. Microbiol. 166, 304–310. doi: 10.1016/j.vetmic.2013.05.018
Browne, H. P., Neville, B. A., Forster, S. C., and Lawley, T. D. (2017). Transmission of the gut microbiota: Spreading of health. Nat. Rev. Microbiol. 15, 531–543. doi: 10.1038/nrmicro.2017.50
Brucker, R. M., and Bordenstein, S. R. (2013). The hologenomic basis of speciation: Gut bacteria cause hybrid lethality in the genus Nasonia. Science 341, 667–669. doi: 10.1126/science.1240659
Buchfink, B., Xie, C., and Huson, D. H. (2015). Fast and sensitive protein alignment using DIAMOND. Nat. Methods 12, 59–60. doi: 10.1038/nmeth.3176
Camarda, A., Circella, E., Pennelli, D., Madio, A., Bruni, G., Lagrasta, V., et al. (2006). Wild Birds as biological indicators of environmental pollution: Biotyping and antimicrobial resistance patterns of Escherichia coli isolated from Audouin’s gulls (Larus Audouinii) living in the Bay of Gallipoli (Italy). Ital. J. Anim. Sci. 5, 287–290. doi: 10.4081/ijas.2006.287
Candela, M., Biagi, E., Maccaferri, S., Turroni, S., and Brigidi, P. (2012). Intestinal microbiota is a plastic factor responding to environmental changes. Trends Microbiol. 20, 385–391. doi: 10.1016/j.tim.2012.05.003
Caporaso, J. G., Lauber, C. L., Walters, W. A., Berg-Lyons, D., Lozupone, C. A., and Turnbaugh, P. J. (2011). Global patterns of 16S rRNA diversity at a depth of millions of sequences per sample. Proc. Natl. Acad. Sci. U.S.A. 108, 4516–4522. doi: 10.1073/pnas.1000080107
Chan, K. G., Liu, Y. C., and Chang, C. Y. (2015). Inhibiting N-acyl-homoserine lactone synthesis and quenching Pseudomonas quinolone quorum sensing to attenuate virulence. Front. Microbiol. 6:1173. doi: 10.3389/fmicb.2015.01173
Chen, C. Y., Chen, P. C., Weng, F. C. H., Shaw, G. T. W., and Wang, D. (2017). Habitat and indigenous gut microbes contribute to the plasticity of gut microbiome in oriental river prawn during rapid environmental change. PLoS One 12:e0181427. doi: 10.1371/journal.pone.0181427
Chen, S., Zhou, Y., Chen, Y., and Gu, J. (2018). fastp: An ultra-fast all-in-one FASTQ preprocessor. Bioinformatics 34, i884–i890. doi: 10.1093/bioinformatics/bty560
Clemente, J., Ursell, L., Parfrey, L., and Knight, R. (2012). The impact of the gut microbiota on human health: An integrative view. Cell 148, 1258–1270. doi: 10.1016/j.cell.2012.01.035
Danzeisen, J. L., Clayton, J. B., Hu, H., Dan, K., Brian, M. C., Hayer, S. S., et al. (2015). Temporal relationships exist between cecum, ileum, and litter bacterial microbiomes in a commercial turkey flock, and subtherapeutic penicillin treatment impacts ileum bacterial community establishment. Front. Vet. Sci. 2:56. doi: 10.3389/fvets.2015.00056
Dewar, M. L., Arnould, J. P. Y., Dann, P., Trathan, P., Groscolas, R., and Smith, S. (2013). Interspecific variations in the gastrointestinal microbiota in penguins. Microbiologyopen 2, 195–204. doi: 10.1002/mbo3.66
Edgar, R. C. (2013). UPARSE: Highly accurate OTU sequences from microbial amplicon reads. Nat. Methods 10, 996–998. doi: 10.1038/NMETH.2604
Fan, C., Zhang, L., Jia, S., Tang, X., Fu, H., Li, W., et al. (2022). Seasonal variations in the composition and functional profiles of gut microbiota reflect dietary changes in plateau pikas. Integr. Zool. 17, 379–395. doi: 10.1111/1749-4877.12630
Flint, H. J., Bayer, E. A., Rincon, M. T., Lamed, R., and White, B. A. (2008). Polysaccharide utilization by gut bacteria: Potential for new insights from genomic analysis. Nat. Rev. Microbiol. 6, 121–131. doi: 10.1038/nrmicro1817
Flint, H. J., Scott, K. P., Louis, P., and Duncan, S. H. (2012). The role of the gut microbiota in nutrition and health. Nat. Rev. Gastroenterol. Hepatol. 9, 577–589. doi: 10.1038/nrgastro.2012.156
Fu, L., Niu, B., Zhu, Z., Wu, S., and Li, W. (2012). CD-HIT: Accelerated for clustering the next-generation sequencing data. Bioinformatics 28, 3150–3152. doi: 10.1093/bioinformatics/bts565
Garud, N. R., Good, B. H., Hallatschek, O., and Pollard, K. S. (2019). Evolutionary dynamics of bacteria in the gut microbiome within and across hosts. PLoS Biol. 17:e3000102. doi: 10.1371/journal.pbio.3000102
Grond, K., Ryu, H., Baker, A. J., Domingo, J. S., and Buehler, D. M. (2014). Gastro-intestinal microbiota of two migratory shorebird species during spring migration staging in Delaware Bay, USA. J. Ornithol. 155, 969–977. doi: 10.1007/s10336-014-1083-3
Grond, K., Santo Domingo, J. W., Lanctot, R. B., Jumpponen, A., Bentzen, R. L., Boldenow, M. L., et al. (2019). Composition and drivers of gut microbial communities in arctic-breeding shorebirds. Front. Microbiol. 10:2258. doi: 10.3389/fmicb.2019.02258
Groussin, M., Mazel, F., Sanders, J. G., Smillie, C. S., Lavergne, S., Thuiller, W., et al. (2017). Unraveling the processes shaping mammalian gut microbiomes over evolutionary time. Nat. Commun. 8:14319. doi: 10.1038/ncomms14319
Hermes, R. G., Molist, F., Ywazaki, M., Nofrarías, M., Gomez de Segura, A., Gasa, J., et al. (2009). Effect of dietary level of protein and fiber on the productive performance and health status of piglets. J. Anim. Sci. 87, 3569–3577. doi: 10.2527/jas.2008-1241
Hermier, D., Rousselot-Pailley, D., Peresson, R., and Sellier, N. (1994). Influence of orotic acid and estrogen on hepatic lipid storage and secretion in the goose susceptible to liver steatosis. Biochim. Biophys. Acta. 1211, 97–106. doi: 10.1016/0005-2760(94)90143-0
Hoelzle, L. E., Scherrer, T., Muntwyler, J., Wittenbrink, M. M., Philipp, W., and Hoelzle, K. (2013). Differences in the antigen structures of Corynebacterium pseudotuberculosis and the induced humoral immune response in sheep and goats. Vet. Microbiol. 164, 359–365. doi: 10.1016/j.vetmic.2013.02.031
Huang, W., Zhou, L., and Zhao, N. (2014). Temporal-spatial patterns of intestinal parasites of the Hooded Crane (Grus monacha) wintering in lakes of the middle and lower Yangtze River floodplain. Avian Res. 5:6. doi: 10.1186/s40657-014-0006-6
Juricova, H., Matiasovicova, J., Kubasova, T., Cejkova, D., and Rychlik, I. (2021). The distribution of antibiotic resistance genes in chicken gut microbiota commensals. Sci. Rep. 11:3290. doi: 10.1038/s41598-021-82640-3
Kanehisa, M., Goto, S., Furumichi, M., Tanabe, M., and Hirakawa, M. (2010). KEGG for representation and analysis of molecular networks involving diseases and drugs. Nucleic Acids Res. 38, D355–D360. doi: 10.1093/nar/gkp896
Kersters, K., De Vos, P., Gillis, M., Swings, J., Vandamme, P., and Stackebrandt, E. (2006). Introduction to the Proteobacteria. Springer 5, 3–37. doi: 10.1007/0-387-30745-1_1
Kleinzeller, A. (1981). “Relationship between membrane transport and metabolism concluding remarks,” in Proceedings of the 28th International Congress of Physiological Sciences: Physiology of Non-excitable Cells, Budapest, 229–230. doi: 10.1016/B978-0-08-026815-6.50030-3
Kohl, K. D. (2012). Diversity and function of the avian gut microbiota. J. Comp. Physiol. B. 182, 591–602. doi: 10.1007/s00360-012-0645-z
Kursa, O., Tomczyk, G., Sawicka-Durkalec, A., Giza, A., and Somiany-Szwarc, M. (2021). Bacterial communities of the upper respiratory tract of turkeys. Sci. Rep. 11:2544. doi: 10.1038/s41598-021-81984-0
Lahtinen, S. J., Davis, E., and Ouwehand, A. C. (2012). Lactobacillus species causing obesity in humans: Where is the evidence? Benef. Microbes 3, 171–174. doi: 10.3920/BM2012.0041
Langille, M. G. I., Zaneveld, J., Caporaso, J. G., McDonald, D., Knights, D., Reyes, J. A., et al. (2013). Predictive functional profiling of microbial communities using 16S rRNA marker gene sequences. Nat. Biotechnol. 31, 814–821. doi: 10.1038/nbt.2676
Lee, S., Cantarel, B., Henrissat, B., Gevers, D., Birren, B. W., Huttenhower, C., et al. (2014). Gene-targeted metagenomic analysis of glucan-branching enzyme gene profiles among human and animal fecal microbiota. ISME J. 8, 493–503. doi: 10.1038/ismej.2013.167
Ley, R. E., Hamady, M., Lozupone, C., Turnbaugh, P. J., Ramey, R. R., Bircher, J. S., et al. (2008). Evolution of mammals and their gut microbes. Science 320, 1647–1651. doi: 10.1126/science.1155725
Li, D., Liu, C.-M., Luo, R., Sadakane, K., and Lam, T.-W. (2015). MEGAHIT: An ultra-fast single-node solution for large and complex metagenomics assembly via succinct de Bruijn graph. Bioinformatics 31, 1674–1676. doi: 10.1093/bioinformatics/btv033
Li, H., and Durbin, R. (2009). Fast and accurate short read alignment with Burrows–Wheeler transform. Bioinformatics 25, 1754–1760. doi: 10.1093/bioinformatics/btp324
Li, R., Li, Y., Kristiansen, K., and Wang, J. (2008). SOAP: Short oligonucleotide alignment program. Bioinformatics 24, 713–714. doi: 10.1093/bioinformatics/btn025
Li, Y., Zhang, T., Shi, M., Zhang, B., Hu, X., Xu, S., et al. (2021). Characterization of intestinal microbiota and fecal cortisol, T3, and IgA in forest musk deer (Moschus berezovskii) from birth to weaning. Integr. Zool. 16, 300–312. doi: 10.1111/1749-4877.12522
Magoè, T., and Salzberg, S. L. (2011). FLASH: Fast length adjustment of short reads to improve genome assemblies. Bioinformatics 27, 2957–2963. doi: 10.1093/bioinformatics/btr507
Million, M., Maraninchi, M., Henry, M., Armougom, F., Richet, H., Carrieri, P., et al. (2012). Obesity-associated gut microbiota is enriched in Lactobacillus reuteri and depleted in Bifidobacterium animalis and Methanobrevibacter smithii. Int. J. Obesity 36, 817–825. doi: 10.1038/ijo.2011.153
Moran, N. A., Ochman, H., and Hammer, T. J. (2019). Evolutionary and ecological consequences of gut microbial communities. Annu. Rev. Ecol. Evol. Syst. 50, 451–475. doi: 10.1146/annurev-ecolsys-110617-062453
Ngunjiri, J. M., Taylor, K. J. M., Abundo, M. C., Jang, H., Elaish, M., Kc, M., et al. (2019). Farm stage, bird age, and body site dominantly affect the quantity, taxonomic composition, and dynamics of respiratory and gut microbiota of commercial layer chickens. Appl. Environ. Microbiol. 85:e03137-18. doi: 10.1128/AEM.03137-18
Nicholson, J. K., Holmes, E., Kinross, J., Burcelin, R., Gibson, G., Jia, W., et al. (2012). Host-gut microbiota metabolic interactions. Science 336, 1262–1267. doi: 10.1126/science.1223813
Noguchi, H., Park, J., and Takagi, T. (2006). MetaGene: Prokaryotic gene finding from environmental genome shotgun sequences. Nucleic Acids Res. 34, 5623–5630. doi: 10.1093/nar/gkl723
Panda, A. K., Rao, S. V., Raju, M. V. L. N., and Sunder, G. S. (2009). Effect of butyric acid on performance, gastrointestinal tract health and carcass characteristics in broiler chickens. Asian Aust. J. Anim. Sci. 22, 1026–1031. doi: 10.5713/ajas.2009.80298
Parfrey, L. W., Moreau, C. S., and Russell, J. A. (2018). Introduction: The host-associated microbiome: Pattern, process and function. Mol. Ecol. 27, 1749–1765. doi: 10.1111/mec.14706
Pellock, S. J., Walton, W. G., Biernat, K. A., Torres-Rivera, D., Creekmore, B. C., Xu, Y., et al. (2018). Three structurally and functionally distinct β-glucuronidases from the human gut microbe Bacteroides uniformis. J. Biol. Chem. 293, 18559–18573. doi: 10.1074/jbc.RA118.005414
Pérez-Cobas, A. E., Gosalbes, M. J., Friedrichs, A., Knecht, H., Artacho, A., Eismann, K., et al. (2013). Gut microbiota disturbance during antibiotic therapy: A multi-omic approach. Gut 62, 1591–1601. doi: 10.1136/gutjnl-2012-303184
Perofsky, A. C., Lewis, R. J., and Meyers, L. A. (2019). Terrestriality and bacterial transfer: A comparative study of gut microbiomes in sympatric Malagasy mammals. ISME J. 13, 50–63. doi: 10.1038/s41396-018-0251-5
Potti, J., Moreno, J., Yorio, P., Briones, V., García-Borboroglu, P., Villar, S., et al. (2002). Bacteria divert resources from growth for magellanic penguin chicks. Ecol. Lett. 5, 709–714. doi: 10.1046/j.1461-0248.2002.00375.x
Qin, J., Li, Y., Cai, Z., Li, S., Zhu, J., Zhang, F., et al. (2012). A metagenome-wide association study of gut microbiota in type 2 diabetes. Nature 490, 55–60. doi: 10.1038/nature11450
Roggenbuck, M., Bærholm Schnell, I., Blom, N., Bælum, J., Bertelsen, M. F., Sicheritz-Pontén, T., et al. (2014). The microbiome of New World vultures. Nat. Commun. 5:5498. doi: 10.1038/ncomms6498
Rosenberg, E., and Gophna, U. (2011). Beneficial microorganisms in multicellular life forms. Berlin: Springer, doi: 10.1007/978-3-642-21680-0
Ryu, H., Grond, K., Verheijen, B., Elk, M., Buehler, D. M., and Santo Domingo, J. W. (2014). Intestinal microbiota and species diversity of Campylobacter and Helicobacter spp. in migrating shorebirds in Delaware Bay. Appl. Environ. Microbiol. 80, 1838–1847. doi: 10.1128/AEM.03793-13
Santos, S. S., Pardal, S., Proença, D. N., Lopes, R. J., Ramos, J. A., Mendes, L., et al. (2012). Diversity of cloacal microbial community in migratory shorebirds that use the Tagus estuary as stopover habitat and their potential to harbor and disperse pathogenic microorganisms. FEMS Microbiol. Ecol. 82, 63–74. doi: 10.1111/j.1574-6941.2012.01407.x
Sharon, G., Segal, D., Ringo, J. M., Hefetz, A., Zilber-Rosenberg, L., and Rosenberg, E. (2010). Commensal bacteria play a role in mating preference of Drosophila melanogaster. Proc. Natl. Acad. Sci. U.S.A. 107, 20051–20056. doi: 10.1073/pnas.1009906107
Sherwin, E., Bordenstein, S. R., Quinn, J. L., Dinan, T. G., and Cryan, J. F. (2019). Microbiota and the social brain. Science 366:eaar2016. doi: 10.1126/science.aar2016
Stackebrandt, E., and Goebel, B. M. (1994). Taxonomic note: A Place for DNA-DNA reassociation and 16S rRNA sequence analysis in the present species definition in bacteriology. Int. J. Syst. Evol. Microbiol. Sci. 44, 846–849. doi: 10.1099/00207713-44-4-846
Tap, J., Mondot, S., Levenez, F., Pelletier, E., Caron, C., Furet, J. P., et al. (2009). Towards the human intestinal microbiota phylogenetic core. Environ. Microbiol. 11, 2574–2584. doi: 10.1111/j.1462-2920.2009.01982.x
Thompson, L. R., Sanders, J. G., McDonald, D., Amir, A., Ladau, J., Locey, K. J., et al. (2017). A communal catalogue reveals Earth’s multiscale microbial diversity. Nature 551, 457–463. doi: 10.1038/nature24621
van Veelen, H. P. J., Falcao Salles, J., and Tieleman, B. I. (2017). Multi-level comparisons of cloacal, skin, feather and nest-associated microbiota suggest considerable influence of horizontal acquisition on the microbiota assembly of sympatric woodlarks and skylarks. Microbiome 5:156. doi: 10.1186/s40168-017-0371-6
Waite, D. W., and Taylor, M. W. (2014). Characterizing the avian gut microbiota: Membership, driving influences, and potential function. Front. Microbiol. 5:223. doi: 10.3389/fmicb.2014.00223
Waite, D. W., and Taylor, W. (2015). Exploring the avian gut microbiota: Current trends and future directions. Front. Microbiol. 6:673. doi: 10.3389/fmicb.2015.00673
Waite, D. W., Deines, P., and Taylor, M. W. (2012). Gut Microbiome of the critically endangered New Zealand Parrot, the Kakapo (Strigops habroptilus). PLoS One 7:e35803. doi: 10.1371/journal.pone.0035803
Wang, A., Zhang, Z., Ding, Q., Yang, Y., Bindelle, J., Ran, C., et al. (2021). Intestinal Cetobacterium and acetate modify glucose homeostasis via parasympathetic activation in zebrafish. Gut Microbes 13, 1–15. doi: 10.1080/19490976.2021.1900996
Wang, Q., Garrity, G. M., Tiedje, J. M., and Cole, J. R. (2007). Naiïve Bayesian classifier for rapid assignment of rRNA sequences into the new bacterial taxonomy. Appl. Environ. Microbiol. 73, 5261–5367. doi: 10.1128/AEM.00062-07
Whelan, C. D., Monaghan, P., Girdwood, R. W. A., and Fricker, C. R. (1988). The significance of wild birds (Larus sp.) in the epidemiology of Campylobacter infections in humans. Epidemiol. Infect. 101, 259–267. doi: 10.2307/3863431
Xing, H., Chen, J., Peng, M., Wang, Z., Liu, F., Li, S., et al. (2019). Identification of signal pathways for immunotoxicity in the spleen of common carp exposed to chlorpyrifos. Ecotoxicol. Environ. Saf. 182:109464. doi: 10.1016/j.ecoenv.2019.109464
Yan, L., Tang, L., Zhou, Z., Lu, W., Wang, B., Sun, Z., et al. (2022). Metagenomics reveals contrasting energy utilization efficiencies of captive and wild camels (Camelus ferus). Integr. Zool. 17, 333–345. doi: 10.1111/1749-4877.12585
Yang, Y., Deng, Y., and Cao, L. (2016). Characterising the interspecific variations and convergence of gut microbiota in Anseriformes herbivores at wintering areas. Sci. Rep. 6:32655. doi: 10.1038/srep32655
Yu, Q., Li, G., and Li, H. (2022). Two community types occur in gut microbiota of large-sample wild plateau pikas (Ochotona curzoniae). Integr. Zool. 17, 366–378. doi: 10.1111/1749-4877.12575
Zeng, Y., Zeng, D., Zhon, Y., Niu, L., Deng, J., Li, Y., et al. (2018). Microbial biogeography along the gastrointestinal tract of a red panda. Front. Microbiol. 9:1411. doi: 10.3389/fmicb.2018.01411
Keywords: 16S rRNA gene, metagenomics, gut microbiome, high-throughput sequencing, ecological types, function prediction
Citation: Wang J, Hong M, Long J, Yin Y and Xie J (2022) Differences in intestinal microflora of birds among different ecological types. Front. Ecol. Evol. 10:920869. doi: 10.3389/fevo.2022.920869
Received: 15 April 2022; Accepted: 25 July 2022;
Published: 10 August 2022.
Edited by:
Cui Wang, University of Helsinki, FinlandReviewed by:
Huan Li, Lanzhou University, ChinaAlison Gould, California Academy of Sciences, United States
Copyright © 2022 Wang, Hong, Long, Yin and Xie. This is an open-access article distributed under the terms of the Creative Commons Attribution License (CC BY). The use, distribution or reproduction in other forums is permitted, provided the original author(s) and the copyright owner(s) are credited and that the original publication in this journal is cited, in accordance with accepted academic practice. No use, distribution or reproduction is permitted which does not comply with these terms.
*Correspondence: Jianmei Xie, xiejm280114@cwnu.edu.cn; Yanqiang Yin, yinyanqiang123@163.com