- 1K. Lisa Yang Center for Conservation Bioacoustics, Cornell Lab of Ornithology, Cornell University, Ithaca, NY, United States
- 2Faculty of Agriculture, Sam Ratulangi University, Manado, Indonesia
- 3Cornell Statistical Consulting Unit, Cornell University, Ithaca, NY, United States
Acoustic communication serves a crucial role in the social interactions of vocal animals. Duetting—the coordinated singing among pairs of animals—has evolved independently multiple times across diverse taxonomic groups including insects, frogs, birds, and mammals. A crucial first step for understanding how information is encoded and transferred in duets is through quantifying the acoustic repertoire, which can reveal differences and similarities on multiple levels of analysis and provides the groundwork necessary for further studies of the vocal communication patterns of the focal species. Investigating acoustic tradeoffs, such as the tradeoff between the rate of syllable repetition and note bandwidth, can also provide important insights into the evolution of duets, as these tradeoffs may represent the physical and mechanical limits on signal design. In addition, identifying which sex initiates the duet can provide insights into the function of the duets. We have three main goals in the current study: (1) provide a descriptive, fine-scale analysis of Gursky’s spectral tarsier (Tarsius spectrumgurskyae) duets; (2) use unsupervised approaches to investigate sex-specific note repertoires; and (3) test for evidence of acoustic tradeoffs in the rate of note repetition and bandwidth of tarsier duet contributions. We found that both sexes were equally likely to initiate the duets and that pairs differed substantially in the duration of their duets. Our unsupervised clustering analyses indicate that both sexes have highly graded note repertoires. We also found evidence for acoustic tradeoffs in both male and female duet contributions, but the relationship in females was much more pronounced. The prevalence of this tradeoff across diverse taxonomic groups including birds, bats, and primates indicates the constraints that limit the production of rapidly repeating broadband notes may be one of the few ‘universals’ in vocal communication. Future carefully designed playback studies that investigate the behavioral response, and therefore potential information transmitted in duets to conspecifics, will be highly informative.
Introduction
Animal vocal communication serves several social functions, including mate attraction, species recognition, territory and mate defense, and threat notification (Wilkins et al., 2013; Price et al., 2015). Natural selection, sexual selection, and neutral evolution are the three primary processes by which observed patterns of differentiation in acoustic signals form across populations. These processes can ultimately lead to the formation of new species, a process known as speciation (Jones, 1997; Wilkins et al., 2013; Blute, 2019; Shuker and Kvarnemo, 2021). The study of animal vocalization systems sets the groundwork for isolating and defining common patterns of phenotypic variation that link species across vast evolutionary distances, providing us with a better understanding of common ancestral constraints that have guided evolution (Derryberry et al., 2012).
The various evolutionary processes mentioned above can drive differentiation across populations, but the selection for certain traits is not without limits. Eventually a trait, such as the loudness of a call or the frequency bandwidth of a note, can no longer be shaped by natural or sexual selection. These limits may be imposed by neural or biomechanical constraints, or a combination of the two (Wilkins et al., 2013). Acoustic neural constraints are limits imposed on vocal production by the capacity of the neural pathways in the brain to produce acoustic signals (Römer, 1993; DeVoogd, 2004; Fitch et al., 2016), while biomechanical constraints are imposed by the physical and morphological composition of the vocal production structure(s), including lung capacity (Fedurek et al., 2017), mouth or beak size and shape (Derryberry et al., 2012), and laryngeal configuration and motor control (Lieberman et al., 1969; Podos, 1996; Fedurek et al., 2017). Such constraints limit the physical abilities of the individual in such a way that reaching these constraints presumably conveys information about the individual’s fitness. It follows that if these constraints are honest indicators of caller quality, individuals who are capable of nearing or reaching the evolutionary limit of a certain trait will be more attractive to potential mates, as successfully exhibiting costly traits can be an indicator of a high-quality individual (Clutton-Brock and Albon, 1979; Reby and McComb, 2003; Terleph et al., 2016; Sun et al., 2021).
One constraint of interest in acoustic signals is the tradeoff between the rate of trill notes and the bandwidth of those notes. In order to produce high frequency trills with wide bandwidths, individuals must make rapid and comprehensive vocal modifications, which may be physically demanding (Podos, 1996; Ballentine et al., 2004). The presumed energetic and/or morphological constraints on modifications of the vocal tract result in high frequency trills with relatively narrower bandwidths. This results in a triangular distribution on a graph, where at low trill frequencies, both wide and narrow bandwidths are possible, while at higher trill frequencies, only narrower bandwidths are exhibited (Derryberry et al., 2012; Wilson et al., 2014). Thus, it appears there are limits to the properties (such as frequency, bandwidth, or amplitude) of acoustic communication. While the limits themselves may vary based on species and vocalization type, the presence of some kind of acoustic tradeoff is thought to be near universal (Podos, 1997).
Acoustic tradeoffs such as this one have been studied in birds, mice, bats, and primates (Podos, 1996; Ballentine et al., 2004; Pasch et al., 2011; Derryberry et al., 2012; Wilson et al., 2014; Clink et al., 2018; Sun et al., 2021). One study examined the ability of a species of sparrow to learn a song containing high frequency trills with artificially broad bandwidths (Podos, 1996). While numerous studies have shown that songbirds are able to learn species-specific songs with high accuracy (Thorpe, 1961; Marler, 1970; Brainard and Doupe, 2002), the individuals exposed to the artificial song were unable to learn it with high fidelity, indicating that morphological acoustic tradeoffs are likely responsible for this inability to replicate an artificial song (Podos, 1996). In Himalayan leaf-nosed bats (Hipposideros armiger), the tradeoff between trill note frequency and individual note bandwidth was found to reflect the quality of the caller; higher quality callers (as indicated using body mass as a proxy) were able to produce higher frequency trills with broader bandwidths (Sun et al., 2021). One of the few studies examining this tradeoff in primates found that female Northern gray gibbons (Hylobates funereus) exhibited vocal patterns consistent with this constraint: an increase in trill rate was correlated with a decrease in note bandwidth (Clink et al., 2018). More studies on a wide variety of taxa are needed to determine the extent to which this acoustic tradeoff is present in animal vocalizations, and if this tradeoff represents one of the few documented universals in vertebrate vocal communication.
A note repertoire is an itemization of different note types produced by a species, while a vocal repertoire is an expansion of a note repertoire by the addition of combinations of the individual note types (Clarke et al., 2006). Repertoire descriptions are often made more robust by the addition of descriptions of the various contexts in which each vocalization type is made, but a crucial first step is analyzing the repertoire across individuals in the same context (Clarke et al., 2006; Blue, 2020). The compilation and thorough definition of a species’ repertoire is a straight-forward yet powerful mode of communication system analysis. While interesting in their own right, vocal and note repertoires can reveal differences and similarities on multiple levels of analysis, including species, sex, and individual, and can reveal how species transmit and receive information about external states such as the presence and type of predator (Clarke et al., 2006; Price et al., 2015; Segbroeck et al., 2017; Sainburg et al., 2020). Large repertoires are presumed to be costly to develop, and in some cases repertoire size reflects aspects of caller quality including age, condition, and parasites (Balsby and Hansen, 2010). Additionally, the establishment of note and vocal repertoires provides the groundwork necessary for further studies and analyses of the vocal communication patterns of the focal species (Blue, 2020; Sainburg et al., 2020). The accumulation of comprehensive vocal repertoires for many taxa is vital to our complete understanding of the vocal communication patterns, functions, and contexts of those taxa, and will be invaluable in informing future studies, especially in vocal but otherwise cryptic or elusive species.
Comprehensive note and vocal repertoires have been compiled for many taxa, including many species of birds (Ficken et al., 1978) and non-human primates (Winter et al., 1966; Gros-Louis et al., 2008; Blue, 2020). Commonly, vocalizations are classified into groups based on physical characteristics observable in a spectrogram, including duration, volume, note frequency, and note shape. While the vocal repertoire size (number of vocalization categories) is often used as an indicator of vocal complexity, repertoire size alone does not provide any information about the functions of the call types or associated contexts (Blue, 2020). Studies that rely solely on acoustic data provide valuable analyses of note types and classifications, but the addition of behavioral observations can allow for the inference of vocalization function by providing social and environmental context (Winter et al., 1966; Ficken et al., 1978; Gros-Louis et al., 2008). Important information can also be gained by understanding the distribution of call types based on individual maturity level, social affiliation, and sex (Clarke et al., 2006; Nousek et al., 2006; Clink et al., 2017; Andrieu et al., 2020).
Gibbons (Hylobatidae), indris (Indriidae), titi monkeys (Callicebinae), and some tarsier species (Tarsiidae) are pair-living primates that produce species- and sex-specific coordinated vocalizations between mated pairs (Haimoff, 1986; Geissmann, 2002; De Gregorio et al., 2022). Reproductive pairs of Lepilemur edwardsi also show coordinated vocal exchanges, but these are not considered proper songs (Méndez-Cárdenas and Zimmermann, 2009). The precise functions of duetting in these various species are, for the most part, yet unknown, although there are a number of hypothesized duet functions, most notably the advertisement and strengthening of the pairbond (Smith, 1994; Geissmann, 1999; Geissmann and Orgeldinger, 2000), territorial communications with extrapair individuals (Clink et al., 2020), and reunion of the mated pair after a period of separation, such as occurs with individual foraging (Méndez-Cárdenas and Zimmermann, 2009). In a review on 59 duetting avian bird species, it was shown that duets used solely for extra-pair communication were more likely to consist of sex-specific notes. The authors noted that the sample size for sex-specific number of notes was too small for statistical analysis, but the median number of notes for males and females in those species with available data was similar (Dahlin and Benedict, 2014). It is unclear whether sex-specificity (or lack thereof) in primate duets is related to differences in function of the duets, as most gibbon species (Geissmann, 2002), tarsiers (MacKinnon and MacKinnon, 1980), and indris (Giacoma et al., 2010) exhibit varying degrees of sex-specificity, whereas titi monkey duets do not (Clink et al., 2022).
In this study, we have three specific aims: (1) provide a descriptive, fine-scale analysis of tarsier duets, including information on which sex initiates the duet, duration of the duets, and total number of notes; (2) use unsupervised approaches to investigate sex-specific note repertoires; and (3) test for evidence of acoustic tradeoffs on the rate of note repetition and bandwidth of tarsier duet contributions. The purpose of aim (1) is purely descriptive, so we do not have any associated hypotheses or predictions. For aim (2), we hypothesize that due to the sex-specificity in duet contributions and the presumed extra-pair communication function of tarsier duets, the number of notes in the note repertoires will be sex-specific, following the trends seen in the other duetting primate species, such as gibbons and indris (Geissmann, 2002; Giacoma et al., 2010). For aim (3), we hypothesize that there are constraints in vocal production that make it difficult to produce broadband notes at a relatively fast rate, and therefore predict that in light of the evidence for acoustic tradeoffs in multiple taxa, including a species of non-human primate, tarsiers will also conform to these vocal patterns.
Materials and methods
Gursky’s spectral tarsier
Gursky’s spectral tarsier (Tarsius spectrumgurskyae; hereafter tarsiers) is a species of small, nocturnal primate endemic to the northern part of the island of Sulawesi in Indonesia (MacKinnon and MacKinnon, 1980; Gursky, 2000, 2002). They are the only faunivorous primate and survive on a diet of insects (Gursky, 2002). Tarsiers live in social groups generally consisting of one adult mated pair and two to four of their juvenile offspring (MacKinnon and MacKinnon, 1980). They are known to be highly territorial and occupy semi-overlapping home ranges (MacKinnon and MacKinnon, 1980). After a night of hunting, tarsiers will return to the same sleep tree or trees each morning, and the mated pair will perform a series of duets around sunrise, roughly between the hours of 0500 and 0600 local time (MacKinnon and MacKinnon, 1980; Gursky, 2000, 2002). Occasionally, juveniles will join in these coordinated vocal displays, in which cases the duets become choruses (Voigt et al., 2006; De Gregorio et al., 2022).
Study system and data collection
We collected focal and autonomous acoustic recordings of tarsiers during July and August of 2018 in Tangkoko National Park on the northeastern tip of the island of Sulawesi, Indonesia (Figure 1). We did not tag or label individual animals for identification in any way, so their reliable territoriality, fidelity to sleep trees, and minimally overlapping ranges allowed specific pairs and individuals to be distinguished. Tarsiers’ general lack of fear of humans means that habituation was unnecessary and that alterations in behavior due to observer presence were minimal (MacKinnon and MacKinnon, 1980).
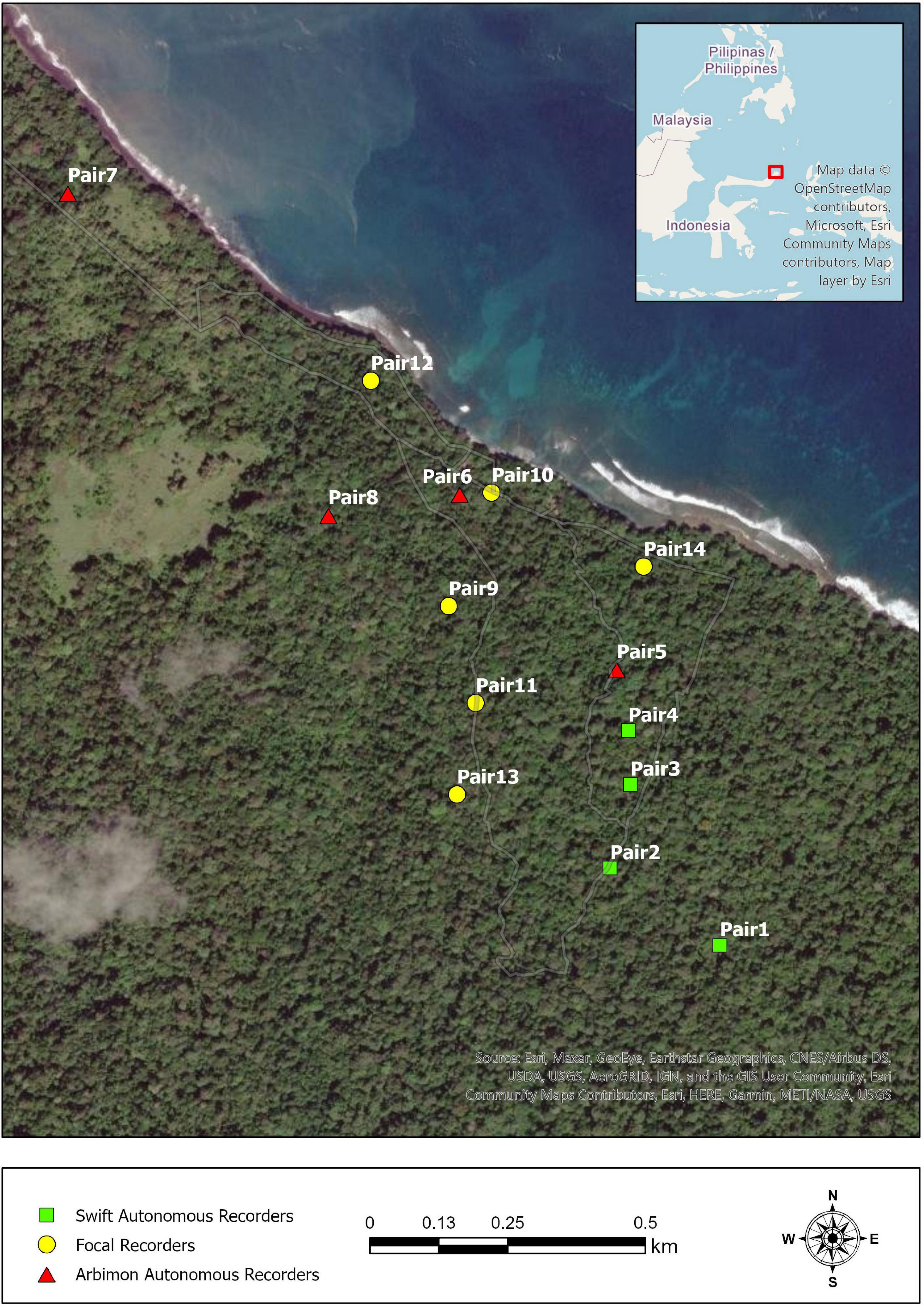
Figure 1. Map of recording locations of tarsier pairs in Tangkoko National Park, North Sulawesi, Indonesia. Each point denotes the recording location of a tarsier pair, and the shape of the points reflects the type of recorder used (see section “Materials and methods” for details).
We used a RØDE NT-USB Condenser Microphone (Røde Microphones, Sydney, Australia) in conjunction with a 32 GB Apple iPad Air (Apple Inc., Cupertino, CA, United States) and the Voice Record Pro application (sampling rate of 44.1 kHz and 16 bits) for focal recordings. DC and a research assistant opportunistically took focal recordings in the early mornings. We took autonomous recordings via either an ARBIMON (Aide et al., 2013) portable recorder (44.1 kHz and 16 bits) or a Swift recorder (Koch et al., 2016) (48 kHz and 16 bits). ARBIMON units recorded daily from 1800 to 0600, while Swift units recorded 24 h continuously. The ARBIMON units had substantially reduced storage capabilities compared to the Swift units, which is why we recorded using different settings and recording schedules. Since tarsiers limit their duetting to a time window of approximately 1 h per day, the variable recording schedules had limited impact on our data collection capabilities. Different autonomous recording units may have different detection ranges due to variation in microphone sensitivities; we aimed to minimize these potential differences by only using high signal-to-noise ratio (SNR) recordings for analyses. Indeed distance from the animal to the recording device can influence the spectral feature estimates, so we limited our analyses to high-quality calls (>12 dB SNR), using high SNR as a proxy for recording distance (Zollinger et al., 2012). Each autonomous unit recorded from 2 to 7 days’ worth of data. Differences in recording durations were due to battery and/or unit malfunction. It has been reported that tarsier duets can be heard up to 500 m by a human observer, but early field tests indicated that the detection range of the recording units for high-quality recordings was much less than that, and generally restricted to animals calling <50 meters from the recording unit (Gursky, 2015; Clink et al., 2019). We saved all recordings as Waveform Audio Files. ARBIMON recorders saved 1-h long files at a size of 317.5 MB, Swift recorders saved 40-min files at a size of 230.4 MB, and focal recordings files were of variable duration and size. We downsampled the 48 kHz to 44.1 kHz sound files using the open-source program Audacity (version 3.1.3) before further analysis. Full details of acoustic data collection methods can be found in Clink et al. (2019). We used only duets (as opposed to choruses or solos) and only those that showed a completed song progression in a spectrogram.
Acoustic analysis
We imported each sound file into Raven Pro v. 1.6 (K. Lisa Yang Center for Conservation Bioacoustics, Cornell Lab of Ornithology, Ithaca, NY, United States) and converted them into spectrograms using the following settings: a 1,600-sample Hann window, 3 dB filter bandwidth of 39.6 Hz, with a 2048-point discrete Fourier transform and 50% overlap, resulting in a time grid hop size of 18.1 ms and a frequency grid spacing of 21.5 Hz. IAC annotated all duets by hand using the selection table functionality in Raven Pro. For each note within the duet, we documented the begin time, end time, minimum frequency, maximum frequency, and sex of the individual. We were able to easily distinguish between male and female duet contributions given the sex-specific differences (MacKinnon and MacKinnon, 1980). Although generally the robust features in Raven are preferable as they reduce variability in intra-observer reliability in terms of how the annotation boxes are selected, they calculate the features based on the energy within the selection and are not appropriate when there is a substantial amount of overlap between signals from different individuals (Charif et al., 2010). Therefore, we calculated bandwidth based on the minimum and maximum frequency bounds of the annotation boxes. This required us to maintain the same brightness, contrast, and focus settings (brightness: 50; contrast: 50) to minimize variation in how annotation boxes were drawn. To calculate the rate of note output, we counted the number of notes emitted per 3-s. Previous analyses calculated note rate using 1-s (Clink et al., 2018), but we found that the rate of note output for tarsiers was relatively slow compared to previous studies (∼1 note per 1-s). Therefore, using a longer duration time bin allowed us to capture more variation in the rate of note output for tarsier duets. In order to allow our results to be compared to other results in this field, we have also standardized these rates into 1-s rates; so, although we used a longer time interval (3-s) to measure the rate, we divided these values by 3 so that our reported values could be used for cross-taxa comparisons. See Figure 2 for representative spectrograms of tarsier duets and phrases and Figure 3 indicating male and female contributions to the duet and a schematic of how we estimated note features for the present analysis.
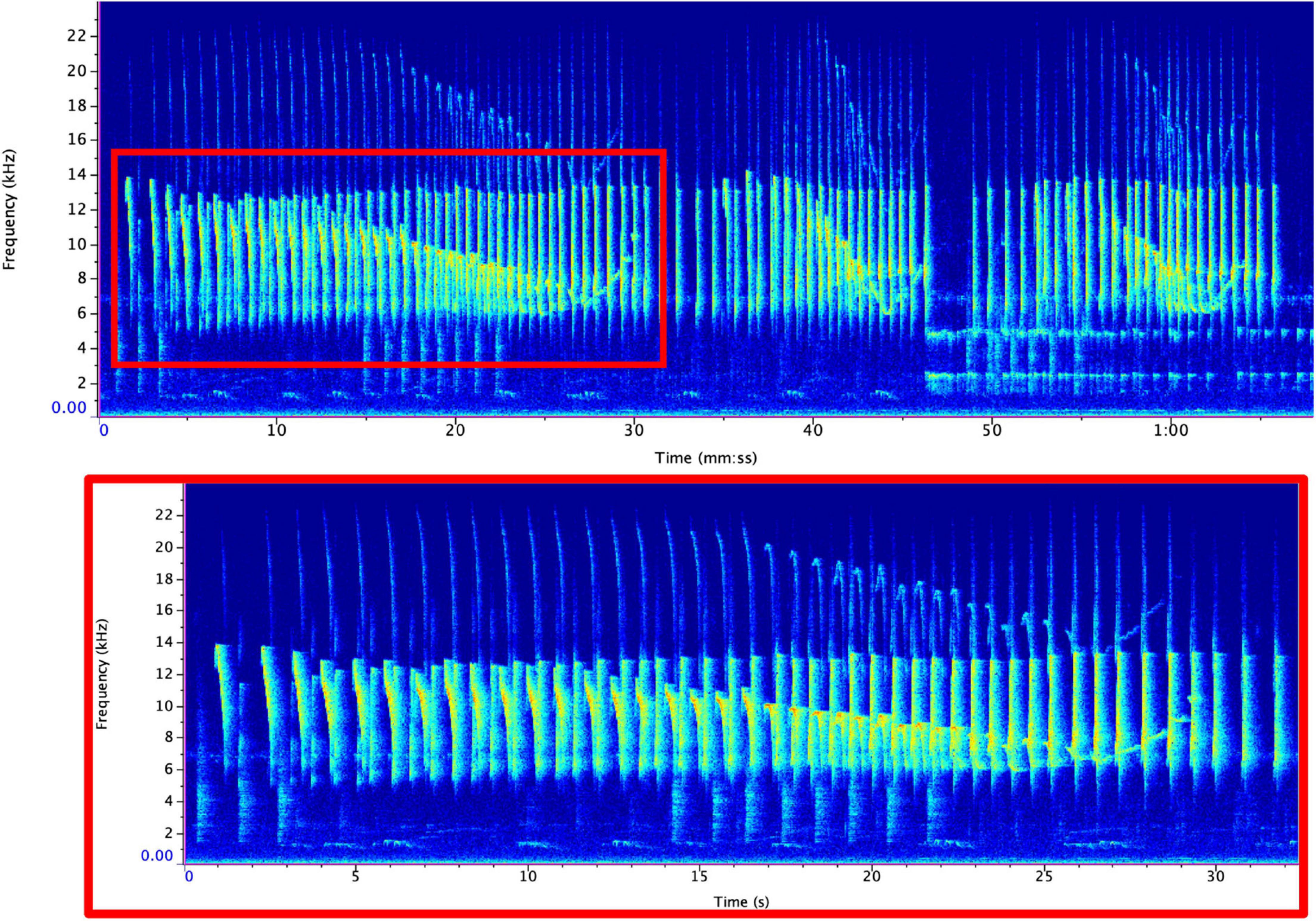
Figure 2. Representative spectrogram of a tarsier duet (top) and phrase (bottom). A single duet (top) can be comprised of many phrases (bottom). Phrases can vary in length but generally follow the structure shown above. Spectrograms were created using RavenPro with the same settings that were used for analysis (see text for details).
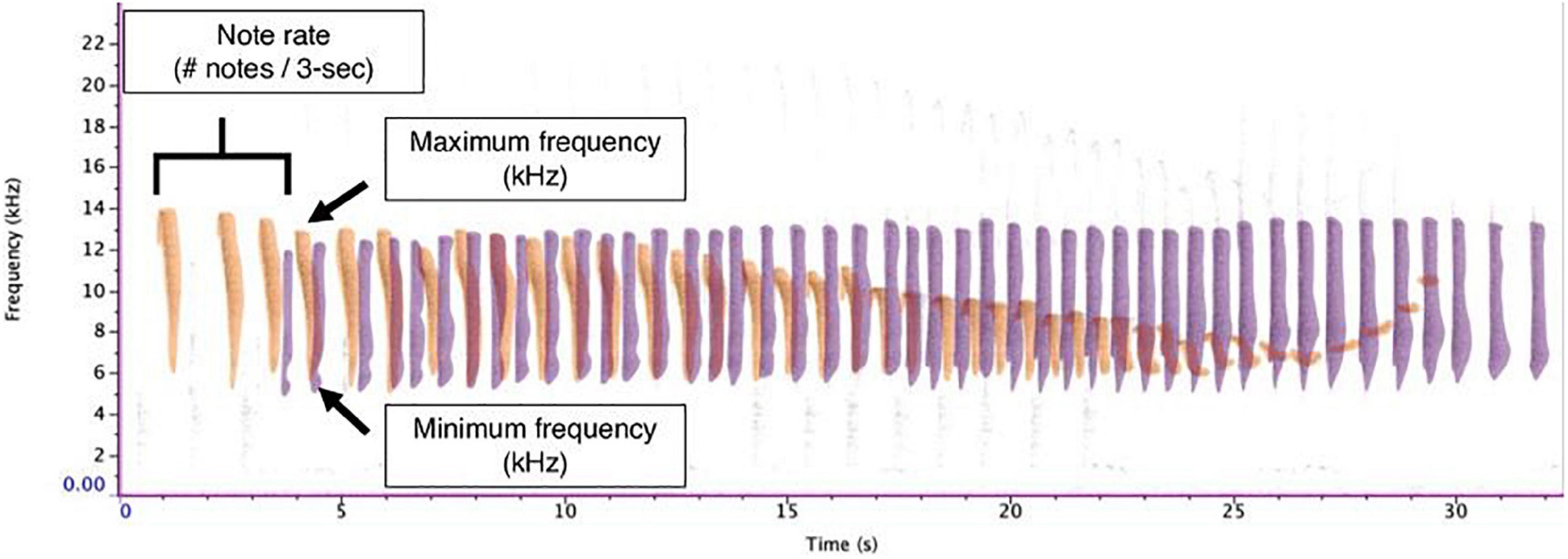
Figure 3. Exemplar of male and female duet contributions and analyzed features. The male duet contribution is shown in purple, and the female duet contribution is shown in orange. Note rate was calculated as the number of notes per 3-s interval. Note bandwidth was determined by subtracting the minimum frequency from the maximum frequency.
Unsupervised analysis of note types
We aimed to identify the number of unique clusters or note types in the male and female tarsier duet contributions. To identify the number of unique clusters we used an unsupervised random forests framework that can be used to identify patterns in an unlabeled dataset (Breiman, 2001). We analyzed male and female notes separately from each other due to structural differences in their respective duet contributions. We used the R programming environment to implement the random forest network using the “randomForest” package (Liaw and Wiener, 2002); we specified the number of trees equal to 10,000 and otherwise used the default settings. As input for the “randomForest” algorithm, we used four features computed from each note–minimum frequency (Hz), maximum frequency (Hz), bandwidth (Hz), and duration (s). This algorithm returns a dissimilarity metric for each observation which can be used to identify groupings within the data. To identify the optimal number of clusters in our dataset we applied k-medoids clustering (Kaufman and Rousseeuw, 2009) to the distance matrix output of the random forest algorithm using the “pam” function in the “cluster” R package (Maechler et al., 2012). K-medoids is more robust version of K-means (Madhulatha, 2011). K-medoids requires the input of the number of clusters (k) so we ran the algorithm iteratively for values of k from 2 to 10 and then calculated a silhouette coefficient for each cluster solution. Silhouette coefficients range from −1 to 1 and provide a measure of how similar an object is relative to the established clusters; a higher silhouette coefficient indicates a more appropriate cluster solution (Rousseeuw, 1987). To identify the optimum number of clusters in our dataset we chose the cluster number with the highest silhouette coefficient. We used a uniform manifold learning technique (McInnes et al., 2018) for visualization of the results using the R package “umap” (Konopka, 2020). UMAP is a dimensionality reduction technique that has been used to effectively visualize differences in acoustic signals of multiple bird taxa (Parra-Hernández et al., 2020), forest soundscapes (Sethi et al., 2020) and female gibbon vocalizations (Clink and Klinck, 2021). We input a feature vector consisting of the four features estimated for each note into the UMAP algorithm, which returned two coordinates or two-dimensional embeddings that can be used to visualize clustering of note types within our dataset. In our study, we define gradation as the degree to which clusters are separated from each other–low gradation means high cluster separation (Wadewitz et al., 2015).
Acoustic tradeoffs statistical analysis
To investigate the relationship between note bandwidth and note rate we used a series of Bayesian multilevel models implemented using the R package “brms” (Bürkner, 2017a,b). The “brms” package provides an interface to the probabilistic programming language STAN (Carpenter et al., 2017). Due to the structural differences in male and female duet contributions we analyzed males and females separately. For both males and females, we created a series of three models. The first model, which we considered the null model, included note bandwidth as the outcome and a random effect for pair identity. The second model, which we used to test for evidence of acoustic tradeoffs, included note bandwidth as an outcome, note rate as a predictor, and pair identity as a random effect. The third model contained a random intercept and slope, with note rate as a predictor. The third model allowed correlation between the random intercepts and slopes.
We used a model selection approach to compare model fit between two models fit to the same data using leave-one-out cross-validation (LOO) (Vehtari et al., 2017) implemented in the “brms” package. The “loo_compare” function returns the difference between the expected log-predictive density (ELPD) of all models relative to the model with the highest ELPD (elpd_diff). The function also returns an estimate of the uncertainty (se_diff), which can be used to assess if the differences among models are reliable (Bürkner, 2017a). We simulated a total of 8,000 samples for inference from four chains, with each chain utilizing 2,000 samples for warmup. We specified weakly informative normal priors for the slope and intercept parameters, and weakly informative half-t priors for the variance components. To further assess fit of the top models we used the posterior predictive check function in “bayesplot” that simulates data from the posterior predictive distribution; if the model is a good fit, then data simulated from the posterior predicted distribution should be similar to the observed data (Gabry et al., 2019). To ensure proper mixing and convergence we inspected trace plots.
Results
Descriptive analysis
We report the results of 6,681 notes from 28 tarsier individuals (14 males and 14 females). We initially analyzed 50 duets, but we omitted one highly irregular male contribution from our final analyses, bringing our total to 50 female duet contributions and 49 male duet contributions. On average, female notes had lower maximum and higher minimum frequencies covering a narrower bandwidth than male notes. Female notes also had a longer average duration than male notes. Duets ranged in duration from 12.88 to 203.96 s, and the median duration of the duets was 64.80 s ± 44.29 standard deviation (Figure 4). Males initiated 25 of the duets and females initiated 25 of the duets. See Table 1 for a summary of sample size along with mean, standard deviation and range of features included in the present analysis.
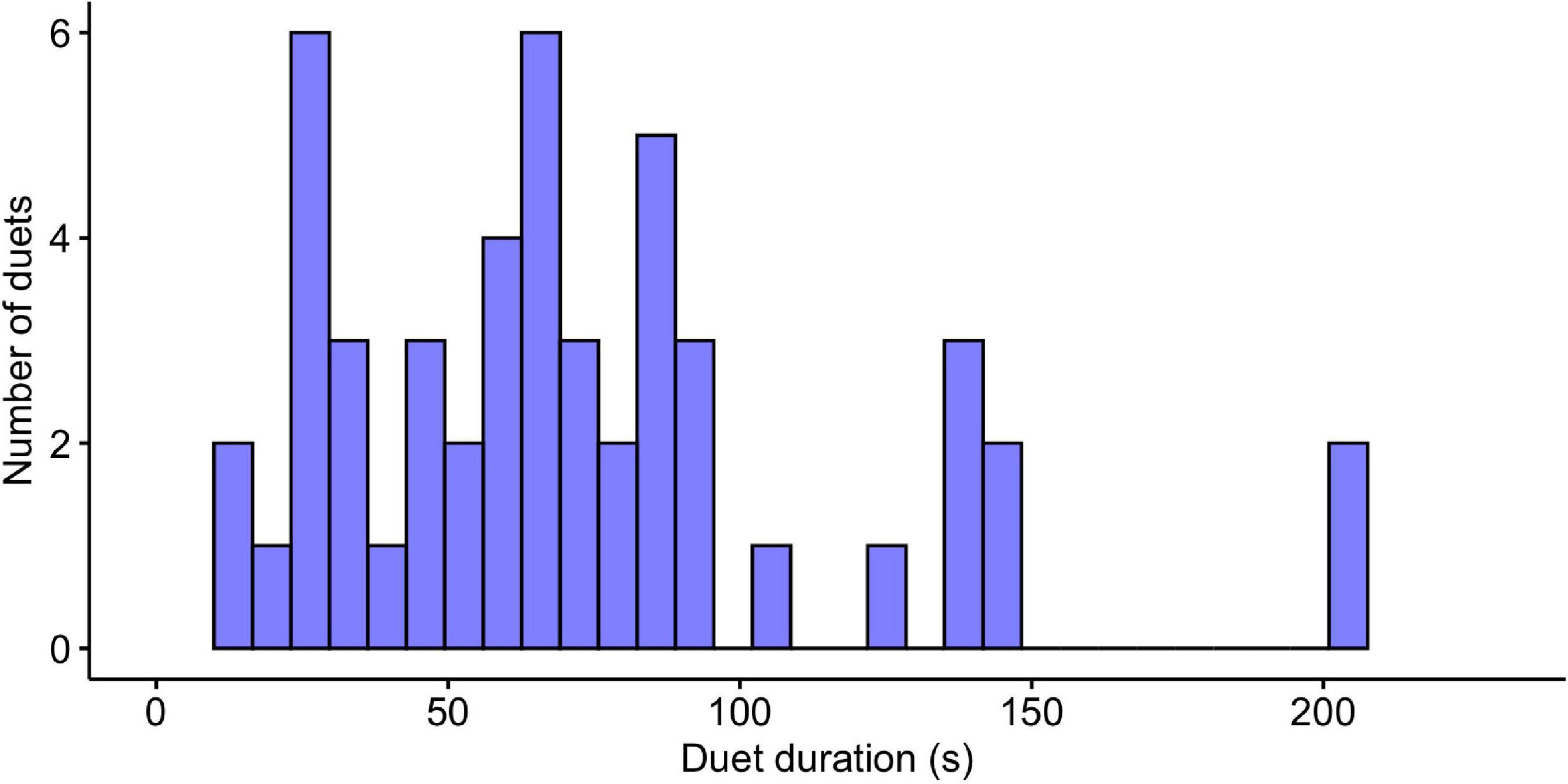
Figure 4. Histogram indicating the durations of tarsier duets (N = 50). Duets ranged in duration from 12.88 to 203.96 s and show a distribution with a slight right skew.
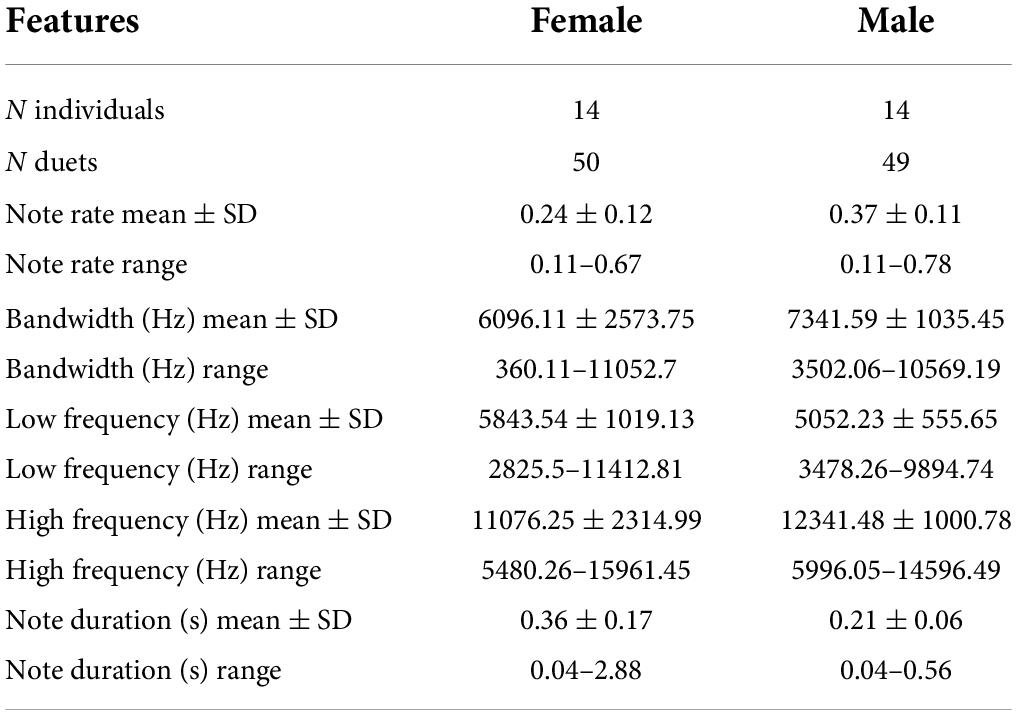
Table 1. Sample size along with mean, standard deviation and range of features included in the present analysis.
Unsupervised analysis of note types
Using the unsupervised random forest analysis, we found evidence for two clusters in male note types and three clusters in female note types. Visual inspection of the UMAP biplots does not show a strong tendency to cluster in the dataset, although female notes do show stronger clustering than males (Figure 5). The lack of many discrete clusters in both the unsupervised analysis and UMAP biplots is indicative of strong gradation in male and female tarsier note types.
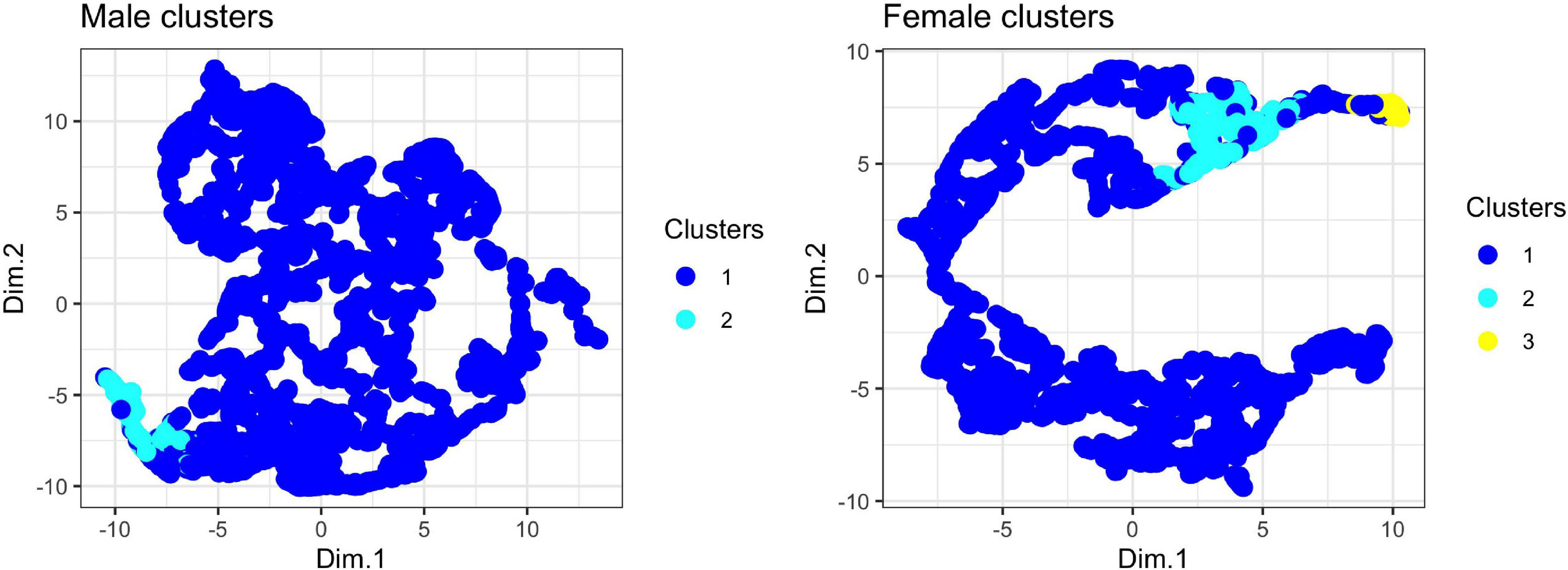
Figure 5. UMAP projections for male and female tarsier duet contributions. For each note in the tarsier duet, we input a feature vector consisting of the four features estimated into the UMAP algorithm, which returned two-dimensional embeddings (Dim. 1 and Dim. 2) used to visualize clustering of note types. Each point represents a single note from the tarsier duet. The color indicates cluster assignment by the random forest algorithm (see text for details).
Acoustic tradeoffs statistical analysis
For both females and males, we found that the model with note rate as a predictor was ranked higher than the null model, providing evidence for acoustic tradeoffs in these two variables (Figure 6). The estimate for the influence of note rate on note bandwidth for the female model was substantially lower (estimate = −13312.17, 95% CI = −15451.09 to −11323.88]) than the estimate for the male note rate (estimate = −679.78, 95% CI = [−1836.83 to 508.25]; Figure 7 and Table 2). Although the male estimates were negative the 95% confidence interval did overlap zero. The top model for females included a random intercept, and slope for pair and performed substantially better than the null model (elp_diff = −163.4; se_diff = 19.4). The top model for males also performed substantially better than the null model (elp_diff = −36.5; se_diff = 11.7) and included a random intercept and slope.
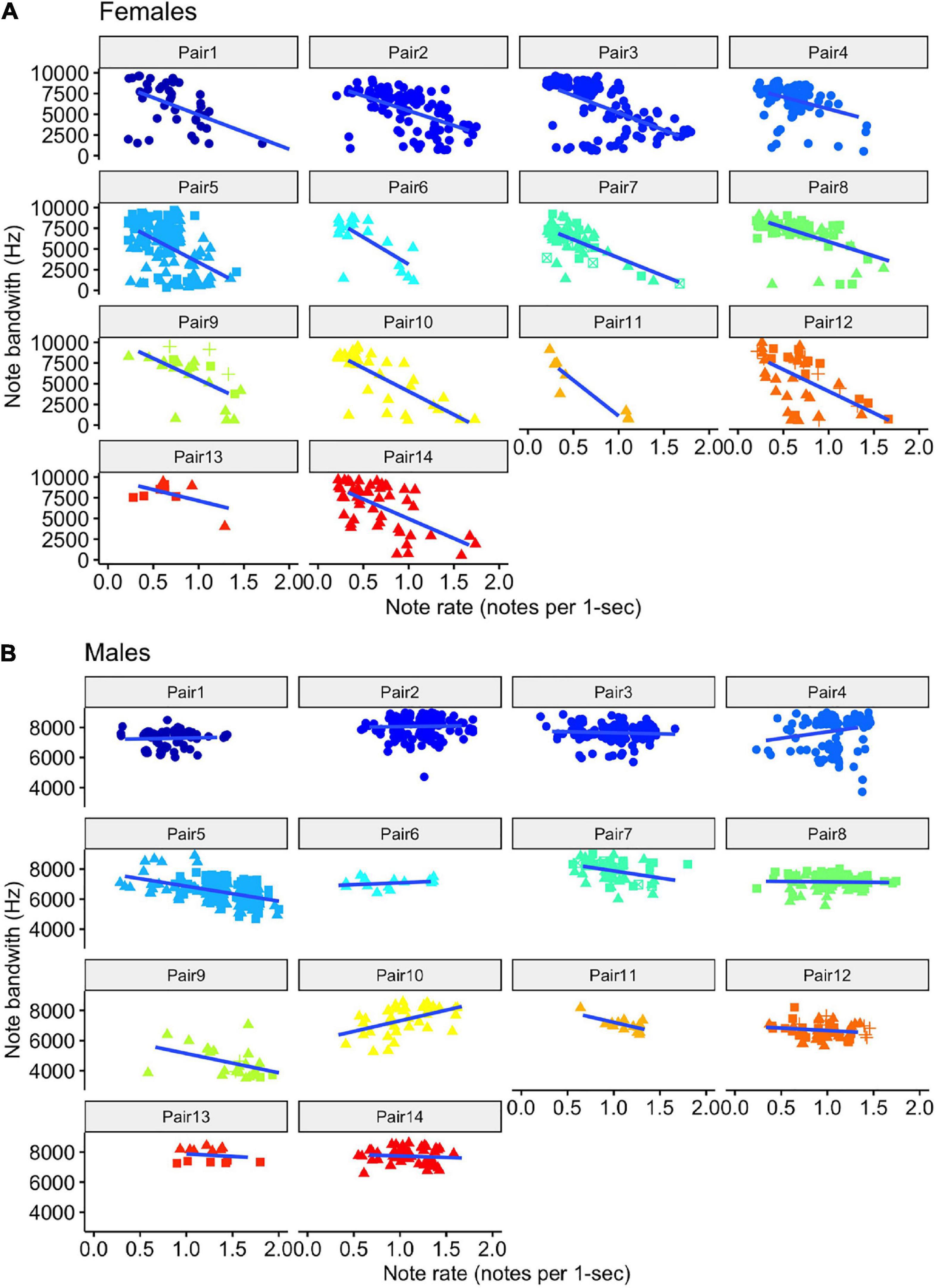
Figure 6. Scatter plots of note bandwidth as a function of the rate of note output (number of notes per 1-s) for female (A) and male (B) tarsier duets. Note that the female (A) scatterplots use a broader bandwidth scale than the male (B) scatterplots, reflecting the generally wider note bandwidth exhibited by the females. The female scatterplots also show much stronger negative slopes than the male scatterplots. The shape of the points indicates which duet the notes came from. Trend lines were added using the “geom_smooth” function in “ggplot2” to visualize differences across pairs (Wickham, 2016).
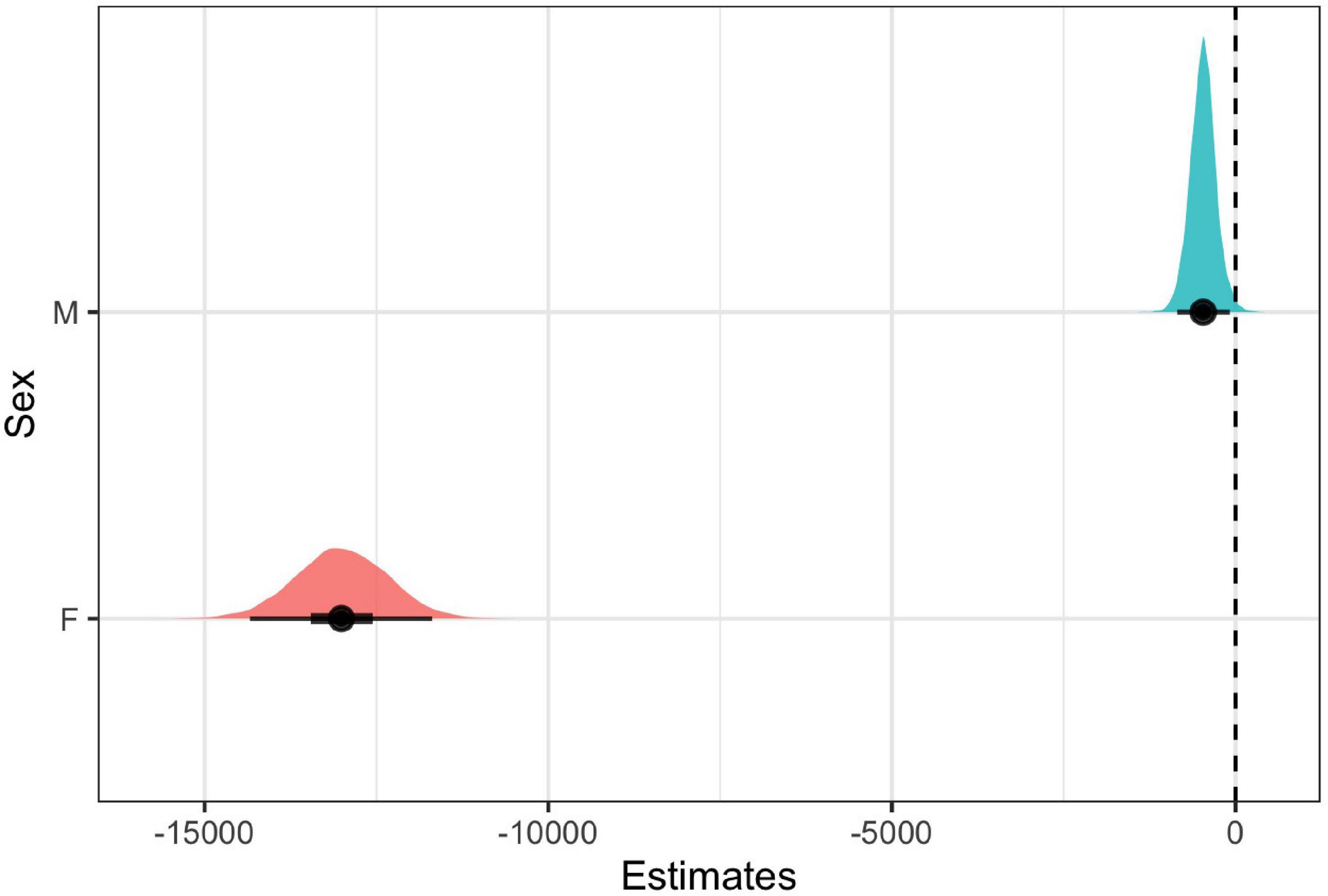
Figure 7. Coefficient estimates ± 95% credible intervals for male and female models with bandwidth as an outcome and note rate as a predictor. We considered the predictors reliable if the confidence intervals (indicated in black) did not overlap zero. Each point indicates the median posterior density credible interval value, the inner black bars represent the 50% credible interval, and the outer black bars represent the 95% credible intervals. The colored distribution plots indicate the associated uncertainty in the point estimates.
Discussion
Summary of results
Our results show that both males and females were equally likely to begin a duet bout. Both male and female note repertoires show highly graded notes rather than discrete note categories, with male notes having a higher degree of gradation. Additionally, our results provide evidence for an acoustic tradeoff between the rate of note repetition and the frequency bandwidth of those notes for both male and female tarsier duet contributions, with a much stronger effect for female contributions.
The degree of note gradation can indicate different functions for various notes. For instance, discrete vocalizations are associated with predator notification and identification in meerkats (Suricata suricatta), Japanese great tits (Parus major minor), and vervet monkeys (Cercopithecus aethiops) (Seyfarth et al., 1980; Suzuki, 2014; Rauber and Manser, 2017), while in black-capped chickadees (Parus atricapillus), discrete notes were associated with courtship behaviors and graded note types were associated with escape and conflict behaviors (Ficken et al., 1978). Given that both tarsier sexes had highly graded note types and our acoustic data is not accompanied by contextual data, we are unable to draw any definite conclusions about the various purposes of each note type; however, future studies that consider the complete note repertoire of the species along with contextual observations and/or playback studies may be able to discern functionality differences in notes based on degree of gradation.
Given the limitations of the data used for our analyses and considering we did not have information regarding animal age, pair-length or other demographic parameters, we can only speculate as to why we observed a stronger pattern of this acoustic tradeoff in female duet contributions compared to male duet contributions. It is possible that this difference is due to the inherent differences in the sex-specific contributions of the tarsier duets, as there is greater variability in female duet contribution note bandwidth. Our results also lead to bigger questions about the function of the male and female contributions and why the female contribution is more complex than the male. In duetting birds, it has been proposed that sex-specificity in duets is due to an extra-pair communication function (Dahlin and Benedict, 2014), and it seems likely that this is also the case with tarsier duets. In addition, males also had a larger range of note rates and in some cases individual males showed patterns opposite that predicted by the acoustic tradeoff we examined. Therefore, it appears that other pressures apart from those consistent with the acoustic tradeoff shaped male tarsier duet contributions.
Previous research on the acoustic tradeoff between rate of note repetition and note bandwidth showed the existence of this tradeoff in a multitude of taxa, including birds, mice, bats, and non-human primates (Podos, 1996; Ballentine et al., 2004; Pasch et al., 2011; Derryberry et al., 2012; Wilson et al., 2014; Clink et al., 2018; Sun et al., 2021). Our findings add another species to this growing list, contributing to the literature that suggests that the existence of this acoustic tradeoff may be effectively universal. This is significant, as universals are relatively rare in animal behavior research (Ferrer-i-Cancho et al., 2013), and can serve to guide our understanding of how certain traits and behaviors evolved over time. However, the evolutionary causes of this acoustic tradeoff are not yet fully understood and may vary between species. Our study does not definitively rule out either morphological or neurological causes of this acoustic performance constraint but adds more literature to the discourse on the ubiquity of this acoustic tradeoff.
Potential limitations
Our study had a few limitations which warrant discussion. First, we examined only notes included in the duets of this tarsier species. This excludes all other vocalizations, including those emitted during hunting, mating, aggressive, parent-offspring, and feeding contexts. Inclusion of these vocalizations may result in different outcomes relating to repertoire size. In addition, different call types may have different constraints, so it is unclear if the acoustic tradeoff between the rate of note repetition and the bandwidth of those notes is prevalent across call types. Additionally, we did not collect nor present data on the non-acoustic behaviors of the individuals at the time of recording (i.e., height in a tree, proximity to conspecifics, maturity, age, reproductive status, presence of predators). It is possible that, like gibbons, tarsier duets vary across different contexts (Clarke et al., 2006; Andrieu et al., 2020). Future studies that compare duets emitted under different contexts (e.g., territorial encounters vs. reuniting at the sleep tree) will be informative. Due to significant temporal and spectral overlap between male and female duet contributions, we were unable to use the robust features in Raven for our unsupervised analysis. These features are calculated based on the energy in the selection and given the substantial overlap in male and female notes the values would have been skewed. This means we were restricted to the four aforementioned features of note duration, minimum frequency, maximum frequency, and bandwidth. If we were able to use the robust features and include a larger number of features that described the notes, then our unsupervised clustering results may have been different.
Future directions
The evidence for the acoustic tradeoff between note rate and bandwidth may be due to either morphological or neurological constraints, or a combination of the two. More research is needed to examine the extent of the existing morphological limitations on the vocal production system, as well as the existence of neurological constraints. Future studies compiling a more exhaustive vocal repertoire of the tarsier, as well as the contexts in which each call type is produced, would be extremely valuable and inform many subsequent studies in tarsier acoustics. Additionally, future studies that take into account variables such as age, weight, time since pairing, and number of offspring will likely make significant contributions toward determining if these tradeoffs are honest reflections of caller quality.
Data availability statement
All R code and data needed to recreate the analyses are available on GitHub: https://github.com/DenaJGibbon/Tarsier-acoustic-tradeoffs.
Ethics statement
The animal study was reviewed and approved by the Institutional approval was provided by the Cornell University (IACUC 2017-0098).
Author contributions
DC and JT performed field work and recorded acoustic data. IC annotated all spectrograms. DC ran analyses in the R programming environment. IC and DC collaborated to develop, revise, and prepare the manuscript for publication. LJ provided input on statistical methods. All authors reviewed and approved of the final version.
Funding
Funding for the field work portion of this project was provided to DC by the Fulbright ASEAN U.S. Scholar Grant (no award number given).
Acknowledgments
We are highly appreciative of early and informative conversations with Russ Charif regarding acoustic tradeoffs. We thank the Fulbright US Scholar Program for providing funding for the data collection for this project. We are incredibly grateful to Vandem Tundu for his assistance with data collection. We also acknowledge the staff at the American Indonesian Exchange Foundation for their help obtaining the necessary permits. We also thank Rob Raguso for his indispensable mentorship, advice, and encouragement.
Conflict of interest
The authors declare that the research was conducted in the absence of any commercial or financial relationships that could be construed as a potential conflict of interest.
Publisher’s note
All claims expressed in this article are solely those of the authors and do not necessarily represent those of their affiliated organizations, or those of the publisher, the editors and the reviewers. Any product that may be evaluated in this article, or claim that may be made by its manufacturer, is not guaranteed or endorsed by the publisher.
References
Aide, T. M., Corrada-Bravo, C., Campos-Cerqueira, M., Milan, C., and Vega, G. (2013). Real-time bioacoustics monitoring and automated species identification. PeerJ 1:e103.
Andrieu, J., Penny, S. G., Bouchet, H., Malaivijitnond, S., Reichard, U. H., and Zuberbühler, K. (2020). White-handed gibbons discriminate context-specific song compositions. PeerJ 8:e9477. doi: 10.7717/peerj.9477
Ballentine, B., Hyman, J., and Nowicki, S. (2004). Vocal performance influences female response to male bird song: an experimental test. Behav. Ecol. 15, 163–168. doi: 10.1093/beheco/arg090
Balsby, T. J. S., and Hansen, P. (2010). Element repertoire: change and development with age in whitethroat sylvia communis song. J. Ornithol. 151, 469–476. doi: 10.1007/s10336-009-0481-4
Blue, S. K. (2020). “The vocal repertoire of tibetan macaques (Macaca thibetana) and congeneric comparisons,” in The Behavioral Ecology of the Tibetan Macaque, ed J. H. Li, L. Sun, and P. M. Kappeler (Cham: Springer), 119–139. doi: 10.1007/978-3-030-27920-2_7
Blute, M. (2019). A new, new definition of evolution by natural selection. Biol. Theory 14, 280–281. doi: 10.1007/s13752-019-00328-4
Brainard, M. S., and Doupe, A. J. (2002). What songbirds teach us about learning. Nature 417, 351–358. doi: 10.1038/417351a
Bürkner, P.-C. (2017a). Advanced Bayesian multilevel modeling with the R package brms. arXiv [Preprint]. 1705.11123.,
Bürkner, P.-C. (2017b). Brms: an R package for Bayesian multilevel models using Stan. J. Statist. Softw. 80, 1–28. doi: 10.18637/jss.v080.i01
Carpenter, B., Gelman, A., Hoffman, M. D., Lee, D., Goodrich, B., Betancourt, M., et al. (2017). Stan: a probabilistic programming language. J. Statist. Softw. 76, 1–32. doi: 10.18637/jss.v076.i01
Charif, R. A., Waack, A. M., and Strickman, L. M. (2010). Raven Pro 1.4 User’s Manual. Ithaca, NY: Cornell Lab of Ornithology, 25506974.
Clarke, E., Reichard, U. H., and Zuberbühler, K. (2006). The syntax and meaning of wild gibbon songs. PLoS One 1:e73. doi: 10.1371/journal.pone.0000073
Clink, D. J., and Klinck, H. (2021). Unsupervised acoustic classification of individual gibbon females and the implications for passive acoustic monitoring. Methods Ecol. Evol. 12, 328–341. doi: 10.1111/2041-210X.13520
Clink, D. J., Bernard, H., Crofoot, M. C., and Marshall, A. J. (2017). Investigating individual vocal signatures and small-scale patterns of geographic variation in female Bornean gibbon (Hylobates muelleri) great calls. Int. J. Primatol. 38, 656–671. doi: 10.1007/s10764-017-9972-y
Clink, D. J., Charif, R. A., Crofoot, M. C., and Marshall, A. J. (2018). Evidence for vocal performance constraints in a female nonhuman primate. Anim. Behav. 141, 85–94. doi: 10.1016/j.anbehav.2018.05.002
Clink, D. J., Lau, A. R., Kanthaswamy, S., Johnson, L. M., and Bales, K. L. (2022). Moderate evidence for heritability in the duet contributions of a South American primate. J. Evol. Biol. 35, 51–63. doi: 10.1111/jeb.13962
Clink, D. J., Tasirin, J. S., and Klinck, H. (2019). Vocal individuality and rhythm in male and female duet contributions of Gursky’s spectral tarsier. Curr. Zool. 66, 173–186. doi: 10.1093/cz/zoz035
Clink, D. J., Tasirin, J. S., and Klinck, H. (2020). Vocal individuality and rhythm in male and female duet contributions of a nonhuman primate. Curr. Zool. 66, 173–186.
Clutton-Brock, T. H., and Albon, S. D. (1979). The roaring of red deer and the evolution of honest advertisement. Behaviour 69, 145–170. doi: 10.1163/156853979X00449
Dahlin, C. R., and Benedict, L. (2014). Angry birds need not apply: a perspective on the flexible form and multifunctionality of avian vocal duets. Ethology 120, 1–10. doi: 10.1111/eth.12182
De Gregorio, C., Carugati, F., Valente, D., Raimondi, T., Torti, V., Miaretsoa, L., et al. (2022). Notes on a tree: reframing the relevance of primate choruses, duets, and solo songs. Ethol. Ecol. Evol. 34, 205–219. doi: 10.1080/03949370.2021.2015451
Derryberry, E. P., Seddon, N., Claramunt, S., Tobias, J. A., Baker, A., Aleixo, A., et al. (2012). Correlated evolution of beak morphology and song in the neotropical woodcreeper radiation. Evolution 66, 2784–2797. doi: 10.1111/j.1558-5646.2012.01642.x
DeVoogd, T. J. (2004). Neural constraints on the complexity of avian song. Brain Behav. Evol. 63, 221–232. doi: 10.1159/000076783
Fedurek, P., Zuberbühler, K., and Semple, S. (2017). Trade-offs in the production of animal vocal sequences: insights from the structure of wild chimpanzee pant hoots. Front. Zool. 14, 1–10. doi: 10.1186/s12983-017-0235-8
Ferrer-i-Cancho, R., Hernández-Fernández, A., Lusseau, D., Agoramoorthy, G., Hsu, M. J., and Semple, S. (2013). Compression as a universal principle of animal behavior. Cogn. Sci. 37, 1565–1578. doi: 10.1111/cogs.12061
Ficken, M. S., Ficken, R. W., and Witkin, S. R. (1978). Vocal repertoire of the black-capped chickadee. Auk 95, 34–48. doi: 10.2307/4085493
Fitch, W. T., De Boer, B., Mathur, N., and Ghazanfar, A. A. (2016). Monkey vocal tracts are speech-ready. Sci. Adv. 2:e1600723. doi: 10.1126/sciadv.1600723
Gabry, J., Simpson, D., Vehtari, A., Betancourt, M., and Gelman, A. (2019). Visualization in Bayesian workflow. J. R. Statist. Soc. 182, 389–402. doi: 10.1111/rssa.12378
Geissmann, T. (1999). Duet songs of the siamang, Hylobates syndactylus: II. Testing the pair-bonding hypothesis during a partner exchange. Behaviour 136, 1005–1039. doi: 10.1163/156853999501694
Geissmann, T. (2002). Duet-splitting and the evolution of gibbon songs. Biol. Rev. 77, 57–76. doi: 10.1017/S1464793101005826
Geissmann, T., and Orgeldinger, M. (2000). The relationship between duet songs and pair bonds in siamangs, Hylobates syndactylus. Anim. Behav. 60, 805–809. doi: 10.1006/anbe.2000.1540
Giacoma, C., Sorrentino, V., Rabarivola, C., and Gamba, M. (2010). Sex differences in the song of Indri indri. Int. J. Primatol. 31, 539–551. doi: 10.1007/s10764-010-9412-8
Gros-Louis, J. J., Perry, S. E., Fichtel, C., Wikberg, E., Gilkenson, H., Wofsy, S., et al. (2008). Vocal repertoire of Cebus capucinus: acoustic structure, context, and usage. Int. J. Primatol. 29, 641–670. doi: 10.1007/s10764-008-9263-8
Gursky, S. (2000). Sociality in the spectral tarsier, Tarsius spectrum. Am. J. Primatol. 51, 89–101. doi: 10.1002/(SICI)1098-2345(200005)51:1<89::AID-AJP7>3.0.CO;2-7
Gursky, S. (2002). Determinants of gregariousness in the spectral tarsier (Prosimian: Tarsius spectrum). J. Zool. 256, 401–410. doi: 10.1017/S0952836902000444
Gursky, S. (2015). Ultrasonic vocalizations by the spectral tarsier, Tarsius spectrum. Folia Primatol. 86, 153–163. doi: 10.1159/000371885
Haimoff, E. H. (1986). Convergence in the duetting of monogamous Old World primates. J. Hum. Evol. 15, 51–59. doi: 10.1016/S0047-2484(86)80065-3
Jones, G. (1997). Acoustic signals and speciation: the roles of natural and sexual selection in the evolution of cryptic species. Adv. Study Behav. 26, 317–354. doi: 10.1016/S0065-3454(08)60383-6
Kaufman, L., and Rousseeuw, P. J. (2009). Finding Groups in Data: An Introduction to Cluster Analysis. Hoboken, NJ: John Wiley & Sons.
Koch, R., Raymond, M., Wrege, P., and Klinck, H. (2016). SWIFT: a small, low-cost acoustic recorder for terrestrial wildlife monitoring applications. in North American Ornithological Conference. Washington, DC.
Konopka, T. (2020). UMAP: Uniform Manifold Approximation and Projection. R Package Version 0.2. 5.0.
Lieberman, P. H., Klatt, D. H., and Wilson, W. H. (1969). Vocal tract limitations on the vowel repertoires of rhesus monkey and other nonhuman primates. Science 164, 1185–1187. doi: 10.1126/science.164.3884.1185
MacKinnon, J., and MacKinnon, K. (1980). The behavior of wild spectral tarsiers. Int. J. Primatol. 1, 361–379. doi: 10.1007/BF02692280
Madhulatha, T. S. (2011). “Comparison between k-means and k-medoids clustering algorithms,” in International Conference on Advances in Computing and Information Technology, eds D. C. Wyld, M. Wozniak, N. Chaki, N. Meghanathan, and D. Nagamalai (Berlin: Springer), 472–481. doi: 10.1007/978-3-642-22555-0_48
Maechler, M., Rousseeuw, P., Struyf, A., Hubert, M., and Hornik, K. (2012). Cluster: Cluster Analysis Basics and Extensions. R Package Version 1.56.
Marler, P. (1970). A comparative approach to vocal learning: song development in white-crowned sparrows. J. Comp. Physiol. Psychol. 71:1. doi: 10.1037/h0029144
McInnes, L., Healy, J., and Melville, J. (2018). Umap: uniform manifold approximation and projection for dimension reduction. arXiv [Preprint]. 1802.03426 doi: 10.21105/joss.00861
Méndez-Cárdenas, M. G., and Zimmermann, E. (2009). Duetting-A mechanism to strengthen pair bonds in a dispersed pair-living primate (Lepilemur edwardsi)? Am. J. Phys. Anthropol. 139, 523–532. doi: 10.1002/ajpa.21017
Nousek, A. E., Slater, P. J. B., Wang, C., and Miller, P. J. O. (2006). The influence of social affiliation on individual vocal signatures of northern resident killer whales (Orcinus orca). Biol. Lett. 2, 481–484. doi: 10.1098/rsbl.2006.0517
Parra-Hernández, R. M., Posada-Quintero, J. I., Acevedo-Charry, O., and Posada-Quintero, H. F. (2020). Uniform manifold approximation and projection for clustering taxa through vocalizations in a Neotropical passerine (rough-legged tyrannulet. Phyllomyias burmeisteri). Animals 10:1406. doi: 10.3390/ani10081406
Pasch, B., George, A. S., Campbell, P., and Phelps, S. M. (2011). Androgen-dependent male vocal performance influences female preference in Neotropical singing mice. Anim. Behav. 82, 177–183. doi: 10.1016/j.anbehav.2011.04.018
Podos, J. (1996). Motor constraints on vocal development in a songbird. Anim. Behav. 51, 1061–1070. doi: 10.1006/anbe.1996.0107
Podos, J. (1997). A performance constraint on the evolution of trilled vocalizations in a songbird family (Passeriformes: Emberizidae). Evolution 51, 537–551. doi: 10.1111/j.1558-5646.1997.tb02441.x
Price, T., Wadewitz, P., Cheney, D., Seyfarth, R., Hammerschmidt, K., and Fischer, J. (2015). Vervets revisited: a quantitative analysis of alarm call structure and context specificity. Sci. Rep. 5:13220. doi: 10.1038/srep13220
Rauber, R., and Manser, M. B. (2017). Discrete call types referring to predation risk enhance the efficiency of the meerkat sentinel system. Sci. Rep. 7:44436. doi: 10.1038/srep44436
Reby, D., and McComb, K. (2003). Anatomical constraints generate honesty: acoustic cues to age and weight in the roars of red deer stags. Anim. Behav. 65, 519–530. doi: 10.1006/anbe.2003.2078
Römer, H. (1993). Environmental and biological constraints for the evolution of long-range signalling and hearing in acoustic insects. Philos. Trans. R. Soc. Lond. Biol. Sci. 340, 179–185. doi: 10.1098/rstb.1993.0056
Rousseeuw, P. J. (1987). Silhouettes: a graphical aid to the interpretation and validation of cluster analysis. J. Comput. Appl. Math. 20, 53–65. doi: 10.1016/0377-0427(87)90125-7
Sainburg, T., Thielk, M., and Gentner, T. Q. (2020). Finding, visualizing, and quantifying latent structure across diverse animal vocal repertoires. PLoS Comput. Biol. 16:e1008228. doi: 10.1371/journal.pcbi.1008228
Segbroeck, M. V., Knoll, A. T., Levitt, P., and Narayanan, S. (2017). MUPET-mouse ultrasonic profile extraction: a signal processing tool for rapid and unsupervised analysis of ultrasonic vocalizations. Neuron 94, 465.e5–485.e5. doi: 10.1016/j.neuron.2017.04.005
Sethi, S. S., Jones, N. S., Fulcher, B. D., Picinali, L., Clink, D. J., Klinck, H., et al. (2020). Characterizing soundscapes across diverse ecosystems using a universal acoustic feature set. Proc. Natl. Acad. Sci. U.S.A. 117, 17049–17055. doi: 10.1073/pnas.2004702117
Seyfarth, R. M., Cheney, D. L., and Marler, P. (1980). Vervet monkey alarm calls: semantic communication in a free-ranging primate. Anim. Behav. 28, 1070–1094. doi: 10.1016/S0003-3472(80)80097-2
Shuker, D. M., and Kvarnemo, C. (2021). The definition of sexual selection. Behav. Ecol. 32, 781–794. doi: 10.1093/beheco/arab055
Smith, W. J. (1994). Animal duets: forcing a mate to be attentive. J. Theor. Biol. 166, 221–223. doi: 10.1006/jtbi.1994.1019
Sun, C., Zhang, C., Lucas, J. R., Gu, H., Feng, J., and Jiang, T. (2021). Vocal performance reflects individual quality in male Great Himalayan leaf-nosed bats (Hipposideros armiger). Integr. Zool. 1–10. doi: 10.1111/1749-4877.12545
Suzuki, T. N. (2014). Communication about predator type by a bird using discrete, graded and combinatorial variation in alarm calls. Anim. Behav. 87, 59–65. doi: 10.1016/j.anbehav.2013.10.009
Terleph, T. A., Malaivijitnond, S., and Reichard, U. H. (2016). Age related decline in female lar gibbon great call performance suggests that call features correlate with physical condition. BMC Evol. Biol. 16:4. doi: 10.1186/s12862-015-0578-8
Thorpe, W. H. (1961). Bird-Song: The Biology of Vocal Communication and Expression in Birds. New York, NY: Cambridge University Press.
Vehtari, A., Gelman, A., and Gabry, J. (2017). Practical Bayesian model evaluation using leave-one-out cross-validation and WAIC. Statist. Comput. 27, 1413–1432. doi: 10.1007/s11222-016-9696-4
Voigt, C., Leitner, S., and Gahr, M. (2006). Repertoire and structure of duet and solo songs in cooperatively breeding white-browed sparrow weavers. Behaviour 159–182. doi: 10.1163/156853906775900739
Wadewitz, P., Hammerschmidt, K., Battaglia, D., Witt, A., Wolf, F., and Fischer, J. (2015). Characterizing vocal repertoires-Hard vs. Soft classification approaches. PLoS One 10:e0125785. doi: 10.1371/journal.pone.0125785
Wickham, H. (2016). Ggplot2: Elegant Graphics For Data Analysis. Berlin: Springer. doi: 10.1007/978-3-319-24277-4
Wilkins, M. R., Seddon, N., and Safran, R. J. (2013). Evolutionary divergence in acoustic signals: causes and consequences. Trends Ecol. Evol. 28, 156–166. doi: 10.1016/j.tree.2012.10.002
Wilson, D. R., Bitton, P.-P., Podos, J., and Mennill, D. J. (2014). Uneven sampling and the analysis of vocal performance constraints. Am. Natural. 183, 214–228. doi: 10.1086/674379
Winter, P., Ploog, D., and Latta, J. (1966). Vocal repertoire of the squirrel monkey (Saimiri sciureus), its analysis and significance. Exp. Brain Res. 1, 359–384. doi: 10.1007/BF00237707
Keywords: primate vocalization, duetting, acoustic tradeoff, Gursky’s spectral tarsier, universals
Citation: Comella I, Tasirin JS, Klinck H, Johnson LM and Clink DJ (2022) Investigating note repertoires and acoustic tradeoffs in the duet contributions of a basal haplorrhine primate. Front. Ecol. Evol. 10:910121. doi: 10.3389/fevo.2022.910121
Received: 31 March 2022; Accepted: 27 June 2022;
Published: 02 August 2022.
Edited by:
Mingbo Yin, Fudan University, ChinaReviewed by:
Marco Gamba, University of Turin, ItalyOliver M. Beckers, Murray State University, United States
Copyright © 2022 Comella, Tasirin, Klinck, Johnson and Clink. This is an open-access article distributed under the terms of the Creative Commons Attribution License (CC BY). The use, distribution or reproduction in other forums is permitted, provided the original author(s) and the copyright owner(s) are credited and that the original publication in this journal is cited, in accordance with accepted academic practice. No use, distribution or reproduction is permitted which does not comply with these terms.
*Correspondence: Isabel Comella, ic265@cornell.edu