- 1School of Biological Sciences, Queen’s University Belfast, Belfast, United Kingdom
- 2Emil Racovita Institute of Speleology, Bucharest, Romania
- 3School of Geosciences, University of Aberdeen, Aberdeen, United Kingdom
- 4School of Natural and Built Environment, Queen’s University Belfast, Belfast, United Kingdom
Karst ecosystems represent up to 25% of the land surface and recent studies highlight their potential role as a sink for atmospheric methane. Despite this, there is limited knowledge of the diversity and distribution of methane-oxidizing bacteria (MOB) or methanogens in karst caves and the sub-surface environment in general. Here, we performed a survey of 14 shotgun metagenomes from cave ecosystems covering a broad set of environmental conditions, to compare the relative abundance and phylogenetic diversity of MOB and methanogens, targeting biomarker genes for methane monooxygenase (pmoA and mmoX) and methyl-coenzyme M reductase (mcrA). Taxonomic analysis of metagenomes showed 0.02–1.28% of classified reads were related to known MOB, of which Gammaproteobacterial MOB were the most abundant making up on average 70% of the surveyed caves’ MOB community. Potential for biogenic methane production in caves was also observed, with 0.008–0.39% of reads classified to methanogens and was dominated by sequences related to Methanosarcina. We have also generated a cave ecosystems protein database (CEPD) based on protein level assembly of cave metagenomes that can be used to profile genes of interest.
Introduction
Karst ecosystems represent up to 25% of the land surface and represent a varied diversity of environmental conditions but the key features that unite most caves are the absence of light, a relatively stable temperature and geographical isolation, when compared to surface ecosystems. Karst Caves represent a unique resource for studying microbial diversity and function, e.g., discovery of novel taxa and biologically active compounds, coupled biogeochemical cycles and provide easily accessible potential sub-surface analogs to the extreme conditions found on other planets (Bendia et al., 2021; Cyske et al., 2021). In the absence of photosynthesis, most cave ecosystems rely on a combination of imported surface carbon and in situ chemosynthesis. One potential source of carbon in caves is methane and methane-derived carbon has been shown to drive food-web interactions in the chemosynthetic ecosystem of Movile cave (Sarbu et al., 1996; Hutchens et al., 2004; Kumaresan et al., 2018). Karst caves have also been identified as a sink for atmospheric methane with studies showing they have a consistently lower than atmospheric levels (Mattey et al., 2013; Waring et al., 2017). Microbial methane oxidation is now widely considered to be the main cause of this phenomenon following studies showing the alternative mechanism, radiolysis by radioactivity, and was too slow to account for the rate of depletion (Lennon et al., 2017; Schimmelmann et al., 2018).
Methane can be oxidized aerobically by methane-oxidizing bacteria (MOB) belonging to phyla Proteobacteria (classes Alphaproteobacteria and Gammaproteobacteria), Verrucomicrobia and candidate division NC10. In aerobic MOB, methane is oxidized to methanol by the enzyme methane monooxygenase (MMO), this is either a membrane-bound copper-dependent particulate methane monooxygenase (pMMO) or a cytoplasmic iron-dependent soluble methane monooxygenase (sMMO) (reviewed in Karthikeyan et al., 2021). Molecular ecology studies of MOB in the environment have leveraged well-developed functional gene biomarkers for MMO, i.e., pmoA encoding the beta-subunit of the pMMO and mmoX encoding the alpha-subunit of the sMMO (Inagaki et al., 2004; McDonald et al., 2008; Knief, 2015). While most MOB require higher concentrations of methane (i.e., low-affinity MOB), a number of MOB are capable of oxidizing sub-atmospheric concentrations of methane (i.e., high-affinity MOB) referred to as atmospheric methane-oxidizing bacteria (atmMOB) (Bender and Conrad, 1992). atmMOB are phylogenetically affiliated with upland soil cluster alpha (USCα) and gamma (USCγ), these are the most abundant MOB taxa in a wide array of studied soil environments and appear to be a large contributor to terrestrial methane oxidation at atmospheric concentrations (Kolb, 2009; Deng et al., 2019; Cai et al., 2020). Methane can also be oxidized anaerobically by members of the Euryarchaeota referred to as anaerobic methane-oxidizing (ANME) archaea using alternative electron acceptors including nitrates and metal oxides (Haroon et al., 2013; Ettwig et al., 2016; Cai et al., 2018; Leu et al., 2020). ANME archaea are prevalent in marine sediments where their methane-oxidizing activity is coupled with the sulfate reducing activity of sulfate reducing bacteria (SRB) (Knittel and Boetius, 2009). ANME are not monophyletic and a number of lineages have been discovered in different genera, families, and even orders (Skennerton et al., 2017).
Methanogenic archaea produce methane in anoxic conditions and can broadly be categorized into three groups based on the substrates they use for methanogenesis; hydrogenotrophic, acetoclastic, and methylotrophic. Hydrogenotrophic methanogens oxidize hydrogen, formate, or short-chain alcohols; acetoclastic methanogens utilize acetate and methylotrophic methanogens demethylate methyl-group (C1) containing compounds including methanol and methylated amines (reviewed in Evans et al., 2019). Methanogens are a phylogenetically diverse group of archaea that can be found in anoxic sediments, such as rice paddies and peat bogs as well as extreme environments including hydrothermal vents and saline lakes (Liesack et al., 2000; Antony et al., 2013; Stewart et al., 2019; Bräuer et al., 2020). The alpha-subunit of Methyl-coenzyme M reductase A (mcrA) has been widely used as the functional biomarker gene to detect both methanogens and ANME archaea (Speth and Orphan, 2018).
A number of culture-independent studies have focused particularly on methane cycling within cave ecosystems using molecular ecology tools targeting phylogenetic and functional biomarker genes (Hutchens et al., 2004; Karwautz et al., 2017; Zhao et al., 2018; Cheng et al., 2021). atmMOB have been detected in a variety of cave environments. USCα has been found through its 16S rRNA gene sequences; to contribute up to 10% of the total microbial community of biofilms in volcanic, limestone and marble caves; USCγ has been shown through the relative abundance of pmoA gene sequences to dominate MOB communities in a number of limestone and dolomite karst caves (Pratscher et al., 2018; Zhao et al., 2018; Cheng et al., 2021).
Our knowledge of the distribution and diversity of aerobic MOB and methanogenic archaea in cave ecosystems is limited. While preliminary investigations have highlighted the dominance of the atmMOB clade USCγ in a number of karst caves’ MOB communities, it is not known if this trend extends to caves more broadly.
Here, we perform a comprehensive analysis of publicly available shotgun metagenome sequences from the cave ecosystems, with the aim to:
(i) Investigate the relative abundance and diversity of MOB and methanogenic archaea in caves using read classification of shotgun metagenomes.
(ii) Identify methane oxidation and production potential within the cave microbial communities, through the retrieval of homologs to the key functional genes, i.e., pmoA, mmoX, and mcrA.
(iii) Build a dataset on cave microbiomes in the form of the CEPD.
Materials and methods
Data acquisition
A literature search was performed on the 20th of November 2021, using the search terms, “cave metagenome” and “cave microbiome” on, PubMed, Web of Science Core Collection and Science Direct. The search was filtered to only include journal articles published from 2010 onward, containing raw sequence data from environmental samples from caves. Studies based on bat guano or cave paintings were not included in the analysis as they represent a more specific niche for microorganisms (De Leon et al., 2018; De Luca et al., 2021). In addition to sequences from caves, publically available metagenomes from a number of terrestrial and aquatic environments were used as outgroups for comparison (Bahram et al., 2018; Buck et al., 2021; Ross et al., 2022; Sánchez-Salazar et al., 2022). Raw sequence data were retrieved from the NCBI Sequence Read Archive (SRA) and EMBL-EBI European Nucleotide Archive (ENA) for downstream analysis. Where technical replicates were available these were concatenated into single metagenomes for downstream analysis.
Pre-processing
Raw FastQ files were processed using either Trimmomatic (version 0.36-6) with default parameters for Illumina generated sequences, or SolexaQA DynamicTrim (version 3.1.7.1) with the –torrent flag for Ion Torrent or 454-generated sequences (Cox et al., 2010; Bolger et al., 2014).
Taxonomic assignment
The relative abundances of taxa in metagenomes were determined by sequence read classification using Kaiju (version 1.7.4) (Menzel et al., 2016). Kaiju was run on the greedy run mode, with a minimum match length of 11, minimum match score of 75 and 5 allowed mismatches against the NCBI non-redundant (NR) protein database (version 2021-02-24). MOB and methanogens related taxa were extracted from Kaiju results at the genus level based upon a list of known MOB and methanogen genera. Figures were constructed with R, bar charts were constructed using the ggplot2 (version 3.3.5) and heatmaps were constructed using the ComplexHeatmap (version 2.10.0) package (Wickham, 2016; Zuguang et al., 2016; R Core Team, 2021).
Gene-centric analysis of metagenomes
Metagenome sequences were individually assembled by Protein-Level ASSembler (PLASS; version 4.687d7), on a protein level, using a minimum assembly length of 40 amino acids (Steinegger et al., 2018). The resulting protein sequences from cave ecosystem metagenomes were concatenated to form a unified CEPD which could be searched for proteins of interest and single-copy genes (SCGs). HMMsearch (version 3.1b2) was used to query the CEPD at an e-value threshold of 10–5 (Eddy, 2011) to retrieve homologs to functional genes. The HMM profiles for pmoA, recA, gyrB, and rpoB were acquired from Fungene and the profiles for mmoX and mcrA from KOFAM (Fish et al., 2013; Aramaki et al., 2020). The full-length sequences of HMMsearch hits were extracted from the CEPD. Only sequences greater than 80 AA residues in length were included for downstream analyses to ensure sequences were long enough for phylogenetic reconstruction. Sequences were clustered (at 95% AA identity) for phylogenetic reconstruction using CD-HIT (version 4.7), particularly in the case of the functional gene mmoX, for ease of phylogenetic curation (Fu et al., 2012).
Homologous sequences were concatenated with appropriate curated protein reference sequences and multiple sequence alignment was performed using Clustal Omega (version 1.2.4) with default parameters (Sievers and Higgins, 2018). The pmoA protein sequence reference set was constructed by combining the translations of nucleotide sequences used by Knief (2015) with pmoA homologs retrieved from the genomes of known MOB. The mmoX reference set was generated by combining mmoX sequences from the Refseq genome database with a selection of related aromatic and aliphatic soluble di-iron monooxygenases (SDIMOs) from Coleman et al. (2006). An mcrA reference set was created retrieving sequences from the Refseq genome database annotated as mcrA.
Conserved regions in these alignments were identified using trimAl (version 1.4.rev15) with the option -automated1 (Capella-Gutiérrez et al., 2009). Poorly aligned sequences were removed. Phylogenetic reconstruction of the alignments was first conducted using FastTree (version 2.1.10-0) to produce preliminary trees for removing outliers and the final trees were constructed using IQTree (version 2.0.3) with 1,000 replicates for bootstrap and 10,000 maximum iterations (Price et al., 2010; Minh et al., 2020). Phylogenetic trees were annotated and visualized using IToL (Letunic and Bork, 2021). Preliminary phylogenetic trees were used for curating hits retrieved by HMMsearch to remove false positives. Representative mmoX and mcrA sequences from metagenome hits were also used to identify closely related sequences in the NR database which were included for final phylogenetic tree construction. Curated metagenome hits were then assigned taxonomic affiliation using their clade placement in their respective phylogenetic trees. The numbers of hits for each gene were then normalized against the mean of the number of hits for the SCGs recA, gyrB, and rpoB retrieved from each metagenome as above.
Results
Data acquisition
A literature search for cave metagenome/microbiome studies resulted in 67 studies with 52 using targeted amplicon sequencing of 16S rRNA gene, 7 characterized the 18S rRNA gene, 9 characterized the ITS2 rRNA gene, and 15 used shotgun metagenomics (Supplementary Table 1). Raw shotgun metagenome sequences were available for 14 samples from six studies covering eight cave ecosystems: Frassasi caves (Italy), Acquasanta cave (Italy), Movile cave (Romania), Tiser cave (Pakistan), Kashmir cave (Pakistan), Diamantina cave (Brazil), Manao-Pee cave (Thailand), and Yumugi river cave (Indonesia). Metagenome sequences from these cave systems represented a diverse range of niches including soil, sediment, biofilms, and dry cave walls (Table 1). These were complemented by 15 metagenome samples representing six non-cave ecosystems including soils, and sediments and lake water. Metagenomes from the following ecosystems were included, where replicates were available, and three were taken. Sediment core metagenomes from oxygen stratified freshwater Alinen Mustajärvi lake in Finland (Buck et al., 2021), sediment metagenomes from the Apatlaco River, Mexico (Sánchez-Salazar et al., 2022), three soil ecosystems: dry tropical forests, moist tropical forests and Mediterranean (Bahram et al., 2018), and a lake water metagenome from the methane-rich Echo Lake (Ross et al., 2022).
Taxonomic profiling
Most of the analyzed metagenomes were dominated by sequences related to phyla Proteobacteria and Actinobacteria, which together made up on average 85 and 50% of the classified reads in cave and non-cave metagenomes, respectively (Supplementary Figure 2). The acidic snottite biofilm samples from Acquasanta and Frasassi caves showed a higher relative abundance of Proteobacterial sequences at 86–92% of classified reads whereas 82 and 93% of sequences from the dry rock and saprolite samples from Diamantina cave (Diamantina_P1b and Diamantina_P3) respectively, were classified to the phylum Actinobacteria. The metagenomes from soils samples in Tiser, Kashmir, and Manao-pee caves along with the Yumugi river cave biofilm metagenome revealed a mix of these two dominant phyla, while their surface soil counterparts had lower numbers of Actinobacterial sequences. Archaeal sequences were detected in lower abundances in all cave metagenomes at 0.1–19% of classified reads. For instance, Candidatus Thermoplasmatota related sequences contributed to 3–7% of classified reads in the snottite biofilm samples of Acquasanta and Frasassi caves. Euryarchaeota made up 19% of classified reads in the Kashmir Bot soil metagenome. Thaumarchaeota were present in the Diamantina wet saprolite metagenome (Diamantina_P7) and the Manao-pee soil metagenome at 9.5 and 3.5% of classified reads, respectively (Supplementary Figure 2A and Supplementary Tables 2A,B).
Between 0.02 and 1.29% of classified reads in cave metagenomes and between 0.11 and 1.48% in non-cave metagenomes were assigned to MOB genera. Sequences related to Gammaproteobacterial MOB represented the major proportion of cave MOB communities making up on average 70% of MOB classified reads. Gammaproteobacterial MOB were only outnumbered by Alphaproteobacterial MOB in the surface soil metagenomes. The largest cave MOB communities were detected in Movile cave metagenomes with 0.948 and 1.29% of classified reads, assigned to MOB genera, in microbial mat and sediment metagenomes, respectively (Figure 1). In comparison with metagenomes from other environments, the largest proportion of MOB reads was detected in the methane-rich Echo lake water metagenome, i.e., at 1.5% of classified sequences. Surface soils metagenomes revealed a higher relative abundance of MOB related sequences in comparison to cave soils metagenomes, with the primary difference in abundance of Alphaproteobacterial MOB. Movile cave metagenomes were enriched for nearly all MOB genera when compared to the average of the other caves (Figure 2A and Supplementary Table 2C) with the only exceptions being for the genera Methylomarinovum and Methylothermus which were not detected in the Movile cave metagenomes. However, in other cave metagenomes only a small number of reads were classified to these genera, 3 and 1, respectively. Several MOB genera showed higher relative abundance across samples, including Methylomonas, Methylobacter, and Methylocystis making up on average 13, 12, and 9% of MOB classified reads, respectively. Sequences related to the Verrucomicrobial genera Methylacidimicrobium, and Methylacidiphilum were detected in higher relative abundance in the acidic snottite biofilm samples making up on average 15% of the snottite MOB community reads compared to a mean of 2% in other metagenomes (Figure 2A and Supplementary Table 2C). The candidate division NC10 genus Candidatus Methylomirabilis had the highest relative abundance in the Diamantina wet saprolite metagenome (Diamantina_P7).
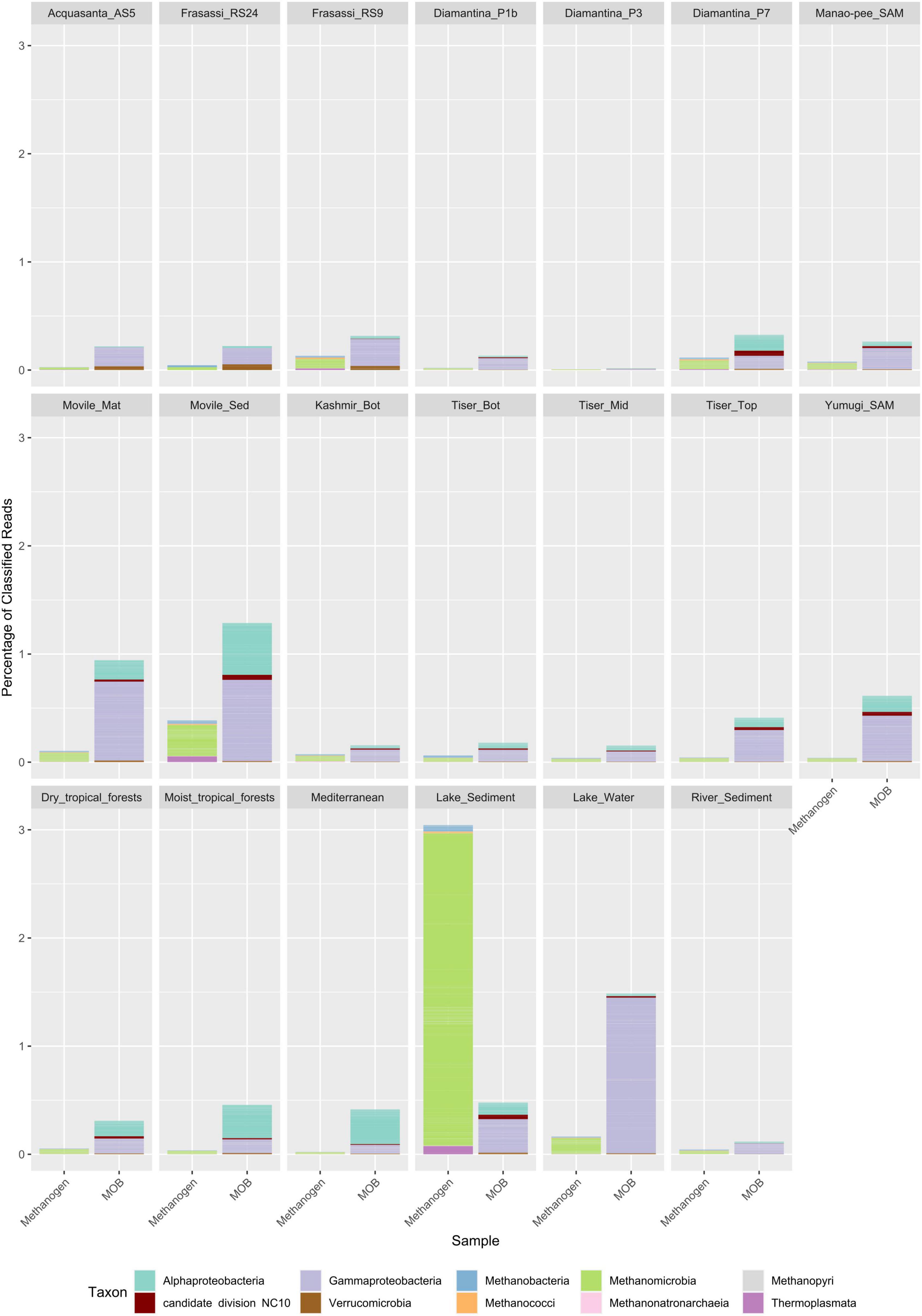
Figure 1. Taxonomic composition of methane-oxidizing bacteria (MOB) and methanogen classified reads at class. Sequencing read classification was carried out using Kaiju against the NCBI non-redundant (nr) protein database. MOB and methanogen taxa were retrieved from Kaiju output at the genus level based on a database of known MOB and methanogen genera using the NCBI taxa names. Values shown are percentage of classified sequences for the respective metagenome (Supplementary Table 2).
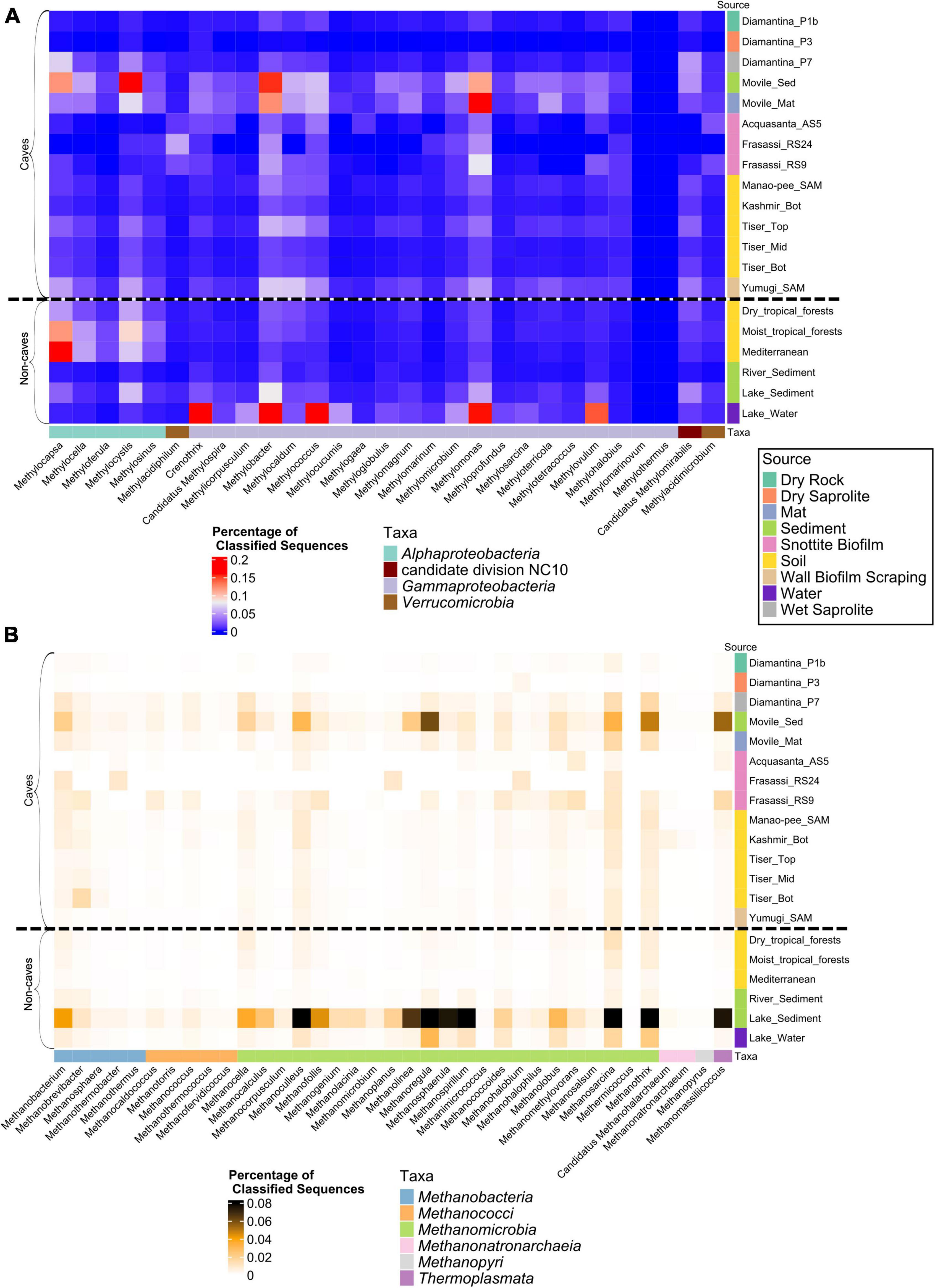
Figure 2. Heatmaps representing the relative abundance of reads classified to MOB (A) and methanogen genera (B). Sequencing read classification was carried out using Kaiju against the NCBI non-redundant (nr) protein database. MOB and methanogen taxa were retrieved from Kaiju output at the genus level based on a database of known MOB and methanogen genera using the NCBI taxa names. Values shown are percentage of classified sequences for the respective metagenome (Supplementary Table 2A).
In cave metagenomes, we observed that between 0.008 and 0.39% of classified reads were assigned to methanogenic archaea. The highest prevalence of methanogens in cave metagenomes were detected in Movile cave sediment metagenomes at 0.39% of classified reads though the lake sediment core metagenomes had a far greater contribution from methanogens at 3% of classified reads (Figure 1). Methanomicrobial sequences were the predominant methanogen accounting for on average 71% of classified reads related to methanogenic genera Sequences related to the genus Methanosarcina was the greatest contributor to the cave metagenomes’ methanogen community making up on average 13% of the metagenomes’ methanogen classified reads. Genera Methanothrix, Methanobacterium, Methanoculleus, and Methanobrevibacter were also prevalent across samples averaging 10, 8, 7, and 6% of the metagenomes’ methanogen classified reads respectively (Figure 2B and Supplementary Table 2D).
Functional gene profiles
A CEPD was generated by protein level assembly of metagenomes, with PLASS, which consists of 155,409, and 219 predicted protein sequences (Steinegger et al., 2018). One metagenome, Frasassi RS24, was too small for assembly with PLASS and was therefore not considered for functional gene profiling. The CEPD (V1) is available at https://doi.org/10.5281/zenodo.5987029.
Homolog search for the gene pmoA recovered 886 potential hits from the CEPD that included sequences from 9 of the 13 cave metagenomes; no homologs were retrieved from Acquasanta and Frassassi cave or Diamantina P1b and P3 (dry rock and dry saprolite) metagenomes. In the case of non-cave metagenomes, pmoA homologs were only retrieved from moist tropical forest soil, lake sediment, and lake water metagenomes. After phylogenetic curation the remaining sequences were assigned to their broad cluster as described by Knief (2015; Figure 3A). The largest number of homologs were retrieved from Tiser Top at 338. After normalization against SCG, Tiser Top, and Yumugi metagenomes revealed higher relative abundance of pmoA homologs, primarily made up of USCγ-related sequences. In total 260 USCγ-related sequences were detected in the CEPD with 241 of these sequences were retrieved from Tiser Top soil metagenomes. Eighteen Crenothrix related sequences were detected in Kashmir Bot. Many homologs retrieved from the CEPD were most closely aligned to evolutionarily related monooxygenases including hydrocarbon (hmoA), ethane (emoA), and ammonia monooxygenases (amoA) or clustered with functionally ambiguous clades namely: clusters 1 and 2, pxmA, TXS, and soil cluster LL F11. For instance, 251 sequences closely aligned to the hmoA sequences (genus Nocardioides) were detected in Diamantina P7 wet saprolite metagenomes and 154 sequences from different cave metagenomes were related to amoA sequences from nitrifying bacteria (Figure 3B). Sequences retrieved from non-cave metagenomes were primarily classified to Gammaproteobacterial MOB (type Ia and type Ib clades). Sequences from lake water metagenomes were related to aquatic cluster 2, deep-sea cluster 2, lake cluster 1, and pxmA and Methylobacter, sequences retrieved from lake sediment metagenomes clustered with clades aquatic cluster 6, RPC1_3-like cluster, aquatic cluster 2, lake cluster 1, and Methylocystis.
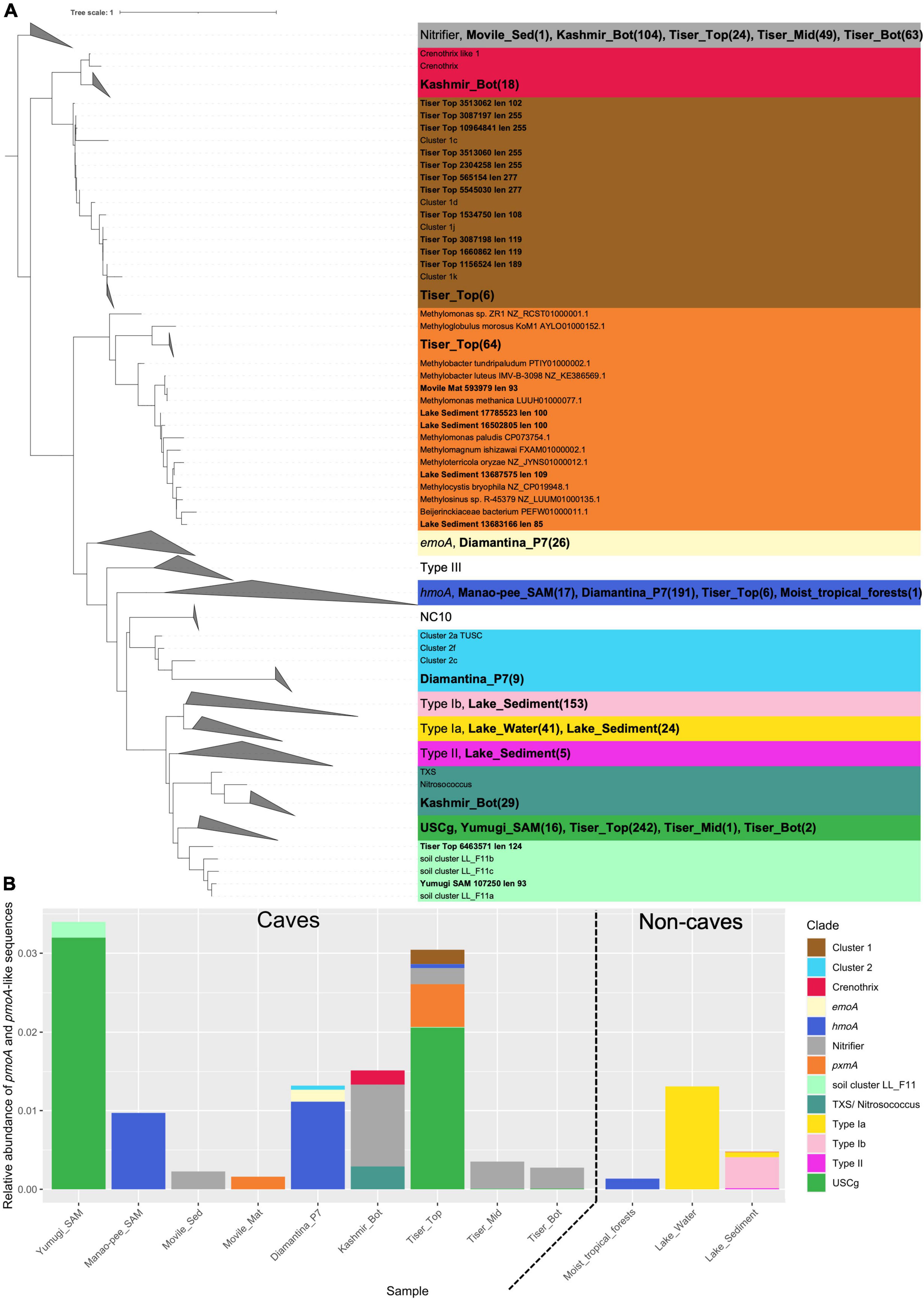
Figure 3. A maximum-likelihood phylogenetic tree of pmoA and pmoA-like protein sequences retrieved from CEPD (A) and relative abundance of different clusters in cave metagenomes (B). Protein sequences from the metagenome samples were assembled by PLASS and concatenated to form a single cave protein catalog which was searched using HMMER3. Metagenome hits were aligned to protein reference sequences from Knief (2015) using Clustal Omega. Conserved regions in these alignments were identified using trimAl followed by phylogenetic reconstruction of multiple sequence alignment using IQTree. (A) Cave sequence labels are bolded. Sequences are colored according to their assigned cluster based on clusters listed in Knief (2015). Clades containing 3 or more cave sequences and no reference sequences were collapsed, node labels show the breakdown of hits by metagenome in brackets (B) The number of sequences retrieved was normalized against the mean number of hits the single copy marker genes gyrB, recB, and rpoB in each metagenome.
Profile HMM search for mmoX homologs recovered 6,091 potential hits from the CEPD representing 10 of the 13 metagenomes; no candidate sequences were retrieved from Acquasanta and Frassassi cave or the Movile sediment metagenomes. mmoX homologs were retrieved from all non-cave metagenomes except the river sediment metagenomes. Downstream phylogenetic curation with a curated set of soluble di-iron monooxygenases (SDIMOs) AA sequences revealed that 124 sequences were aligned closely to known mmoX sequences, 22 from the Movile cave microbial mat metagenomes 98 from lake water, and 4 from lake sediment (Figure 4A). Most of the mmoX candidate sequences aligned with the group 4 SDIMOs, which consists of sequences related to alkene monooxygenases (Figure 4B).
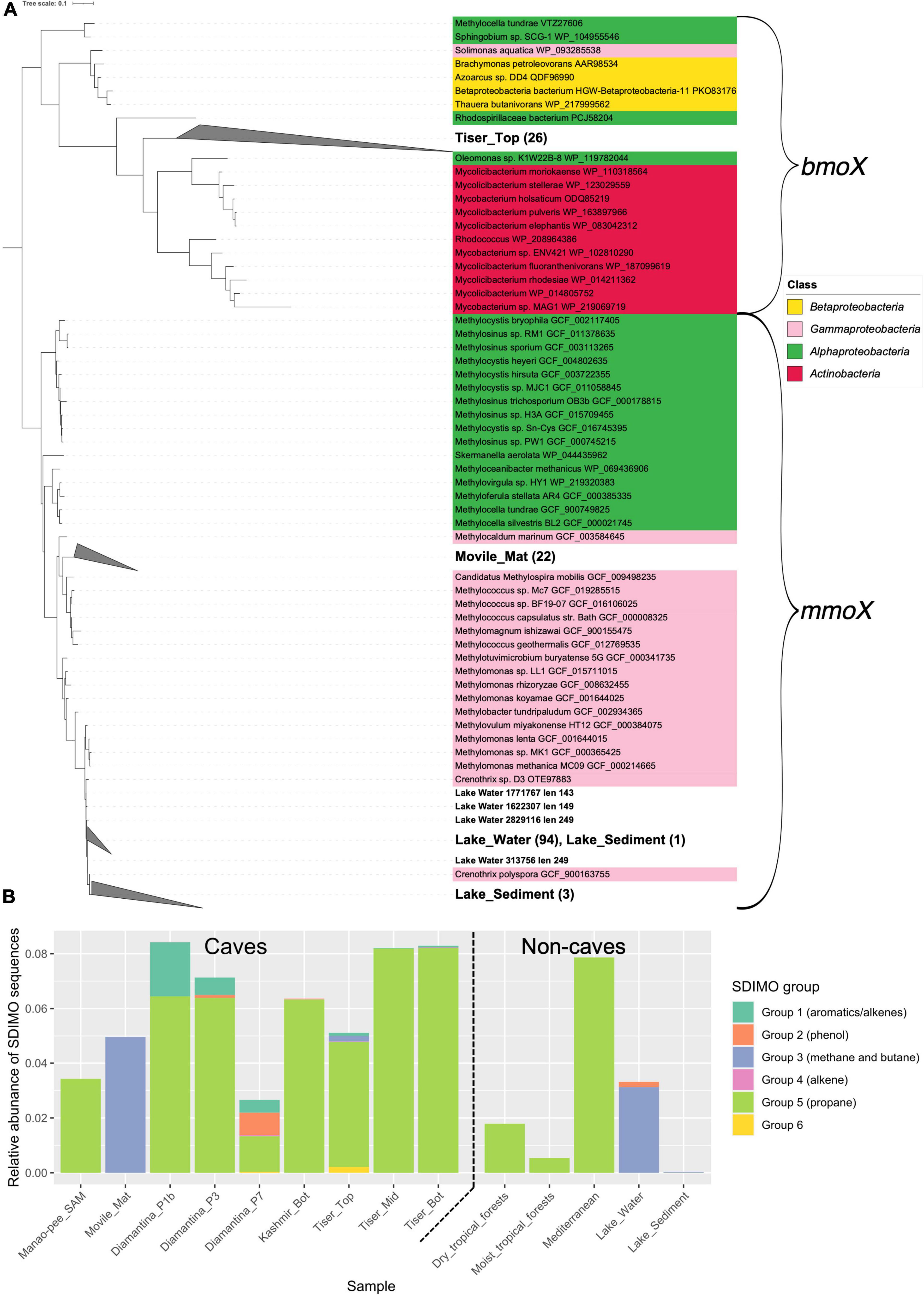
Figure 4. A maximum-likelihood phylogenetic tree of group 3 soluble di-iron monooxygenases (SDIMOs) sequences retrieved from cave metagenomes (A) relative abundance of SDIMO groups (B) retrieved from cave metagenomes. Protein sequences from the metagenome samples were assembled by PLASS and concatenated to form a single cave protein catalog which was searched using HMMER3. Hit sequences were aligned to protein reference sequences using Clustal Omega. Conserved regions in these alignments were identified using trimAl. Phylogenetic reconstruction of alignments was conducted using IQTree. (A) Sequences classified as belonging to SDIMO group 3 are shown. Sample metagenome sequence labels are bolded. Clades with more than 3 and sequences consisting only of sample metagenome sequences were collapsed, the number of sequences contained in these clades are shown in brackets. Reference sequences are colored according to their assigned NCBI taxonomy. (B) Cave SDIMO sequences were classified into the groups described in Coleman et al. (2006) where they were broadly classified into physiological roles. The number of SDIMOs retrieved from each metagenome was normalized against the mean number of hits the single copy marker genes gyrB, recB, and rpoB. The tree used to classify cave SDIMO sequences can be found in Supplementary Figure 1.
Only two mcrA sequences were recovered from the CEPD, both of which were from the Movile cave sediment metagenome. Protein similarity search against NR database revealed that one of the sequences was closely related to Methanophagales (PXF51295.1), and the other to Methanosarcinales (RLG34212.1), Candidatus Synthrophoarchaeum (MBL7117849.1), ANME-1 (NQE05873 cluster) mcrA sequences. Non-cave metagenomes returned 2,694 mcrA homologs, all retrieved from lake sediment metagenomes and primarily related to Methanomassiliicoccus, Methanothermococcus, and Methanoregulaceae.
Discussion
Previous research have focused on methane budgets and microbial role in methane cycling within specific cave ecosystems using in situ measurements, laboratory experiments and microbial surveys (Martin-Pozas et al., 2020). Here, we compare the diversity and distribution of MOB and methanogenic communities and tease out the metabolic potential within microbiomes from different cave ecosystems. Community composition assessment based on metagenome sequence profiling revealed expected patterns, i.e., the dominance of phyla Actinobacteria and Proteobacteria in cave communities, and in agreement with many 16S rRNA gene-based studies (Supplementary Figure 1; Ortiz et al., 2013; Adetutu et al., 2014; De Mandal et al., 2017; Ghosh et al., 2017). In the case of Kashmir, Tiser, Manao-pee, and Yumugi river cave community composition inferred from the 16S rRNA gene amplicon sequencing broadly agrees with the composition determined using shotgun metagenome read classification (Wiseschart et al., 2019; Turrini et al., 2020; Zada et al., 2021). Interpretation of MOB/methanogen taxa distribution and comparison of relative abundance from read classification tools (Kaiju) are limited by the database of MOB and methanogen taxa-related sequences and their inclusion in the NCBI non-redundant protein database. As Kaiju predicts the relative abundance of taxa by the classification of all reads in a metagenome rather than classifying only of a panel of SCGs, as many comparable tools do, its potential for inaccuracy must be considered (Peabody et al., 2015; Tovo et al., 2020). The advantage of this approach is however, capturing a wider diversity from low coverage metagenomes, this is particularly important when targeting low abundance functional groups like MOB. Whilst recent developments in high-throughput sequencing offer higher coverage at lower cost, most of the metagenomes used in this study have low coverage to completely profile low abundance organisms such as MOBs or generate metagenome assembled genomes.
Methanotrophs
In all cave metagenomes, MOB sequences were relatively more abundant than methanogens; this can perhaps be in part attributed to the aerobic niches being surveyed (Figure 1). While oxygen levels were not measured in these cave niches, they were all, with the exception of Movile cave sediment, retrieved from either surfaces or just below the surface, in the case of soil samples. The only surveyed metagenomes which had a higher proportion of methanogens than MOB classified reads were from the anaerobic sediment of Alinen Mustajärvi lake (Buck et al., 2021). Movile cave is isolated from surface inputs and the primary production is via chemolithoautotrophic microorganisms including MOB (Sarbu et al., 1996). The higher relative abundance of low-affinity MOB in Movile cave microbial mat metagenomes is due to the cave’s methane-enriched atmosphere fed by thermal waters (Figure 1; Sarbu, 2000). The presence of sequences related to aerobic MOBs in the Movile cave sediment raises the question of whether these sediments are indeed anoxic as first reported (Sarbu, 2000). The oxygen content of this sediment has been questioned before by Kumaresan et al. (2018) who suggested microoxic conditions may exist, owing to the presence of a high proportion of iron-oxidizing bacteria (Sideroxydans). Alternatively, the detected sequences related to aerobic MOB may have originated from the microbial mat floating in aerobic conditions at the lake water’s surface and are thus not part of the active sediment microbiome. This highlights the need for new measurements of dissolved oxygen in Movile cave lake water and sediment. Two mcrA sequences retrieved from the Movile cave sediments were most closely related to sequences from Methanophagales, Methanosarcinales, and Candidatus Synthrophoarchaeum, indicating that these mcrA sequences are most likely not methanogenic but instead methane or short-chain alkane oxidizing organisms (Laso-Pérez et al., 2016).
The snottite biofilms of Acquasanta and Frasassi caves represent another extreme niche with highly acidic pH 0–1.5 (Jones et al., 2011, 2016). Dominated by the sulfide oxidizing Acidithiobacillus this niche has been shown to harbor a low-diversity community (Macalady et al., 2007; Jones et al., 2011). These metagenomes are notably enriched in Verrucomicrobial MOB contributing on average 15% of the snottite MOB community reads compared to a mean of 2% in other samples (Figure 2A). This is likely due to Methylacidiphilum and Methylacidimicrobium’s acidic tolerance, with the growth of these genera recorded at as low as pH 0.5. Whilst the temperature range reported for Verrucomicrobial MOB is 22.5–81.6°C (van Teeseling et al., 2014; Erikstad et al., 2019), the temperature of the snottite biofilm in Frasassi is lower (13°C). Future attempts to isolate these acidic MOBs will allow researchers to compare the physiology and ecological adaptation to the specific microniches. pmoA sequences and MOB classified reads were relatively more abundant in the Tiser_Top metagenome than the Tiser_Mid or Tiser_Bot metagenomes. While no measurements of methane concentration have been taken from Tiser cave, methane gradients have previously been observed in caves, with methane concentration decreasing with distance from the cave entrance (Zhao et al., 2018; Cheng et al., 2021). Tiser cave is reported to be 12 km long and the Tiser_Top soil samples were obtained from 2 m whilst the Tiser_Mid and Tiser_Bot samples were retrieved from 300 and 700 m, respectively. In situ measurement of methane concentrations could explain the differences in relative abundance of MOB within different regions of the Tiser cave.
In comparison to the other analyzed ecosystems, it is unsurprising that the methane enriched ecosystems of Movile cave and Echo lake water have a higher proportion of MOB related sequences. The large MOB community in the lake water metagenome of Echo lake is present at a methane chemocline near the oxic-anoxic boundary of the lake’s water which is an indication of rapid methane consumption (Ross et al., 2022). Despite their similarity with high methane concentration, there was distinct differences in MOB diversity, i.e., genera such as Methylocapsa and Methylocystis are far more prevalent in Movile cave in comparison to the Echo lake. For sake of brevity, here we only focus on the comparison between different cave ecosystem communities.
The most abundant MOB clade detected through phylogenetic analysis of pmoA sequences from the metagenomes was the atmospheric MOB USCγ (Figure 3B). USCγ-related sequences were predominant in the metagenomes from Yumugi river cave and the top of Tiser cave. This abundance of USCγ is in line with previous studies which have shown USCγ dominates the MOB communities of several limestone and dolomite caves (Zhao et al., 2018; Cheng et al., 2021). Excluding hits for evolutionary related monooxygenases (i.e., amoA, hmoA, and emoA), the second most predominant pmoA cluster was the divergent homolog of pmoA, pxmA detected in Tiser_Top soil, Movile cave microbial mat, and lake sediment metagenomes. Gammaproteobacterial MOB genera such as Methylomonas, Methylomicrobium, and Methylobacter are known to harbor the divergent pXMO, i.e., pmoABC rather than the canonical pmoCAB (Tavormina et al., 2011). Whilst the substrate specificity of the pXMO remains uncertain, along with other copper-dependent monooxygenases, it will be worthwhile to follow up with process-based studies to understand their contribution to carbon cycling within cave ecosystems. Crenothrix-related pmoA sequences were retrieved from the bottom of Kashmir cave soil metagenome. Also, metagenome read classification by Kaiju revealed widespread presence of Crenothrix-related sequences across different cave metagenomes with higher relative abundances in Movile cave mat and sediment metagenomes and the methane-rich Echo lake water (Figure 2A). Crenothrix polyspora is a filamentous gammaproteobacterium, first reported as methane oxidizer by Stoecker et al. (2006), and have been shown to be major methane oxidizers in stratified lakes using stable isotope probing and single-cell imaging mass spectrometry with metabolic capacity for denitrification under oxygen limited conditions. pmoA sequences retrieved from non-cave ecosystems such as lake water and sediment metagenomes were mostly related to clades that did not contain sequences from cave ecosystems.
Methanogens
The most relatively abundant methanogenic genera detected in the cave metagenomes was Methanosarcina, averaging 13% of methanogen classified reads and being the predominant methanogenic genera in nine of the 14 cave metagenomes (Figures 2B, 3B). Methanosarcina is unique among methanogens for its ability to utilize a broad range of substrate including methylamines, methanol, hydrogen, and acetate (Hippe et al., 1979). Methanosarcina have been isolated from a wide range of environments including soils, sediments anaerobic digesters and even the microbial mat of Movile cave (Ganzert et al., 2014; Zhou et al., 2021). This metabolic flexibility could be the reason why it is prevalent in nearly all the cave metagenomes. The second most abundant genus is the strictly acetoclastic Methanothrix belonging to the order Methanosarcinales (Smith and Ingram-Smith, 2007).
In comparison to other cave metagenomes, a higher relative abundance of methanogens were detected in Movile cave sediment metagenomes, likely due to the anaerobic niches within the sediment (Figure 1). This suggests that at least some of the methane found in Movile cave could be biogenic in origin, though this needs to be confirmed with in situ measurements. The Movile cave sediment metagenome was also enriched in methylotrophic methanogen Methanomassiliicoccus, which is known to utilize methanol for methanogenesis in wetland soil (Narrowe et al., 2019). MOB release methanol extracellularly during the oxidation of methane and the role of MOB-derived methanol in methane production needs to be elucidated (Hutchens et al., 2004). Whilst the wet saprolite sample from Diamantina (Diamantina_P7) harbored the second highest number of methanogens reads (Figure 1), the lowest abundance of methanogen reads was retrieved from the dry saprolite metagenome of Diamantina cave (Diamantina_P3).
Conclusion
In summary, our analysis based on sequencing read classification and the retrieval of functional biomarkers from published cave metagenomes has shown the makeup of the methane cycling communities of a diverse cohort of subterranean ecosystems. Our observations reveal the prevalence of atmMOB USCγ pmoA in caves with access to atmospheric gas transfer and a general dominance of Gammaproteobacterial MOB communities. Also, this study shows the ubiquity of the methanogenic archaeal genus Methanosarcina in cave ecosystems. The low number of hits for methane cycling functional biomarkers in these published metagenomes highlights the need to combine shotgun sequencing with the amplicon sequencing of functional and phylogenetic biomarkers to properly capture the diversity and relative abundance of methane cycling organisms. We believe that this snapshot of MOB and methanogenic communities will lead to future research that combines in situ process measurements and linking microbial functional diversity with geochemical characterization to obtain a thorough understanding of the eco-physiology. Moreover, the CEPD created by the protein level assembly of cave shotgun metagenomes will be a useful community resource (available at https://doi.org/10.5281/zenodo.5987029) that will be regularly updated as more metagenomes are published.
Data availability statement
The datasets presented in this study can be found in online repositories. The names of the repository/repositories and accession number(s) can be found at: doi: 10.5281/zenodo.5987029 and in Table 1 of this article.
Author contributions
DK conceived the idea. AA and DK designed the study. AA, MC, and DK performed the bioinformatic analyses and interpretation of data. AA and DK wrote the first draft of the manuscript with AH-V, RD, and J-CC contributing to final version of the manuscript. All authors contributed to the article and approved the submitted version.
Funding
This study received funding from NERC DTP studentship to AA (NE/S007377/1-2429402), Department for Economy NI studentship to MC and QUB for funding to DK. RD and DK were supported by DfE US-Ireland R&D partnership project 154. AH-V was supported by CNCS-UEFISCDI project PN-IIIP4-ID-PCCF-2016-0016, PCCF 16/2018 (DARKFOOD, PI Dr. O. T. Moldovan).
Acknowledgments
The authors thank the funders of this study for their support.
Conflict of interest
The authors declare that the research was conducted in the absence of any commercial or financial relationships that could be construed as a potential conflict of interest.
Publisher’s note
All claims expressed in this article are solely those of the authors and do not necessarily represent those of their affiliated organizations, or those of the publisher, the editors and the reviewers. Any product that may be evaluated in this article, or claim that may be made by its manufacturer, is not guaranteed or endorsed by the publisher.
Supplementary material
The Supplementary Material for this article can be found online at: https://www.frontiersin.org/articles/10.3389/fevo.2022.909865/full#supplementary-material
Supplementary Figure 1 Summary of studies using high-throughput sequencing (amplicon and/or shotgun metagenomes) to study microbial diversity in caves by publication year (A) and location (B). Studies were found by a literature search, using the search terms, “cave metagenome” and “cave microbiome” on, PubMed, Web of Science Core Collection and Science Direct. Results were filtered to only include journal articles published from 2010 onward, containing original sequence data from environmental samples from caves.
Supplementary Figure 2 Taxonomic composition of classified prokaryotic metagenome sequence reads at phylum level. Sequencing read classification was carried out using Kaiju against the NCBI non-redundant (nr) protein database. All phyla below 2.5% of classified reads were collapsed to a single color (Supplementary Table 2A).
Supplementary Figure 3 A maximum-likelihood phylogenetic tree of soluble di-iron monooxygenases (SDIMOs) sequences retrieved from cave metagenomes and the NCBI non-redundant protein database.
References
Adetutu, E. M., Ball, A. S., Adetutu, E. M., and Ball, A. S. (2014). Microbial diversity and activity in caves. Microbiol. Aust. 35, 192–194. doi: 10.1071/MA14062
Antony, C. P., Kumaresan, D., Hunger, S., Drake, H. L., Murrell, J. C., and Shouche, Y. S. (2013). Microbiology of Lonar Lake and other soda lakes. ISME J. 7:468. doi: 10.1038/ISMEJ.2012.137
Aramaki, T., Blanc-Mathieu, R., Endo, H., Ohkubo, K., Kanehisa, M., Goto, S., et al. (2020). KofamKOALA: KEGG Ortholog assignment based on profile HMM and adaptive score threshold. Bioinformatics 36, 2251–2252. doi: 10.1093/BIOINFORMATICS/BTZ859
Bahram, M., Hildebrand, F., Forslund, S. K., Anderson, J. L., Soudzilovskaia, N. A., Bodegom, P. M., et al. (2018). Structure and function of the global topsoil microbiome. Nature 5607717, 233–237. doi: 10.1038/s41586-018-0386-6
Bender, M., and Conrad, R. (1992). Kinetics of CH4 oxidation in oxic soils exposed to ambient air or high CH4 mixing ratios. FEMS Microbiol. Lett. 101, 261–270. doi: 10.1111/J.1574-6968.1992.TB05783.X
Bendia, A. G., Callefo, F., Araújo, M. N., Sanchez, E., Teixeira, V. C., Vasconcelos, A., et al. (2021). Metagenome-assembled genomes from monte cristo cave (Diamantina, Brazil) reveal prokaryotic lineages as functional models for life on mars. Astrobiology 22, 293–312. doi: 10.1089/AST.2021.0016
Bolger, A. M., Lohse, M., and Usadel, B. (2014). Trimmomatic: a flexible trimmer for Illumina sequence data. Bioinformatics 30:2114. doi: 10.1093/BIOINFORMATICS/BTU170
Bräuer, S. L., Basiliko, N., Siljanen, H. M. P., and Zinder, S. H. (2020). Methanogenic archaea in peatlands. FEMS Microbiol. Lett. 367, fnaa172. doi: 10.1093/FEMSLE/FNAA172
Buck, M., Garcia, S. L., Fernandez, L., Martin, G., Martinez-Rodriguez, G. A., Saarenheimo, J., et al. (2021). Comprehensive dataset of shotgun metagenomes from oxygen stratified freshwater lakes and ponds. Sci. Data 8:131. doi: 10.1038/S41597-021-00910-1
Cai, C., Leu, A. O., Xie, G. J., Guo, J., Feng, Y., Zhao, J. X., et al. (2018). A methanotrophic archaeon couples anaerobic oxidation of methane to Fe(III) reduction. ISME J. 12, 1929–1939. doi: 10.1038/S41396-018-0109-X
Cai, Y., Zhou, X., Shi, L., and Jia, Z. (2020). Atmospheric Methane Oxidizers Are Dominated by Upland Soil Cluster Alpha in 20 Forest Soils of China. Microb. Ecol. 80, 859–871. doi: 10.1007/S00248-020-01570-1
Capella-Gutiérrez, S., Silla-Martínez, J. M., and Gabaldón, T. (2009). trimAl: A tool for automated alignment trimming in large-scale phylogenetic analyses. Bioinformatics 25, 1972–1973. doi: 10.1093/bioinformatics/btp348
Cheng, X.-Y., Liu, X.-Y., Wang, H.-M., Su, C.-T., Zhao, R., Bodelier, P. L. E., et al. (2021). USCγ Dominated Community Composition and Cooccurrence Network of Methanotrophs and Bacteria in Subterranean Karst Caves. Microbiol. Spectr. 9:e0082021. doi: 10.1128/SPECTRUM.00820-21
Coleman, N. V., Bui, N. B., and Holmes, A. J. (2006). Soluble di-iron monooxygenase gene diversity in soils, sediments and ethene enrichments. Environ. Microbiol. 8, 1228–1239. doi: 10.1111/J.1462-2920.2006.01015.X
Cox, M. P., Peterson, D. A., and Biggs, P. J. (2010). SolexaQA: At-a-glance quality assessment of Illumina second-generation sequencing data. BMC Bioinform. 11:485. doi: 10.1186/1471-2105-11-485
Cyske, Z., Jaroszewicz, W., Żabińska, M., Lorenc, P., Sochocka, M., Bielańska, P., et al. (2021). Unexplored potential: Biologically active compounds produced by microorganisms from hard-to-reach environments and their applications. Acta Biochim. Pol. 68, 565–574. doi: 10.18388/ABP.2020_5887
De Leon, M. P., Montecillo, A. D., Pinili, D. S., Siringan, M. A. T., and Park, D. S. (2018). Bacterial diversity of bat guano from Cabalyorisa Cave, Mabini, Pangasinan, Philippines: A first report on the metagenome of Philippine bat guano. PLoS One 13:e0200095. doi: 10.1371/JOURNAL.PONE.0200095
De Luca, D., Caputo, P., Perfetto, T., and Cennamo, P. (2021). Characterisation of environmental biofilms colonising wall paintings of the fornelle cave in the archaeological site of cales. Int. J. Environ. Res. Public Health 18:8048. doi: 10.3390/IJERPH18158048/S1
De Mandal, S., Chatterjee, R., and Kumar, N. S. (2017). Dominant bacterial phyla in caves and their predicted functional roles in C and N cycle. BMC Microbiol. 17:90. doi: 10.1186/S12866-017-1002-X
Deng, Y., Che, R., Wang, F., Conrad, R., Dumont, M., Yun, J., et al. (2019). Upland Soil Cluster Gamma dominates methanotrophic communities in upland grassland soils. Sci. Total Environ. 670, 826–836. doi: 10.1016/J.SCITOTENV.2019.03.299
Eddy, S. R. (2011). Accelerated profile HMM searches. PLoS Comput. Biol. 7:e1002195. doi: 10.1371/journal.pcbi.1002195
Erikstad, H. A., Ceballos, R. M., Smestad, N. B., and Birkeland, N. K. (2019). Global Biogeographic Distribution Patterns of Thermoacidophilic Verrucomicrobia Methanotrophs Suggest Allopatric Evolution. Front. Microbiol. 10:1129. doi: 10.3389/FMICB.2019.01129
Ettwig, K. F., Zhu, B., Speth, D., Keltjens, J. T., Jetten, M. S. M., and Kartal, B. (2016). Archaea catalyze iron-dependent anaerobic oxidation of methane. Proc. Natl. Acad. Sci. U.S.A. 113, 12792–12796. doi: 10.1073/PNAS.1609534113
Evans, P. N., Boyd, J. A., Leu, A. O., Woodcroft, B. J., Parks, D. H., Hugenholtz, P., et al. (2019). An evolving view of methane metabolism in the Archaea. Nat. Rev. Microbiol. 174, 219–232. doi: 10.1038/s41579-018-0136-7
Fish, J. A., Chai, B., Wang, Q., Sun, Y., Brown, C. T., Tiedje, J. M., et al. (2013). FunGene: The functional gene pipeline and repository. Front. Microbiol. 4:291. doi: 10.3389/FMICB.2013.00291/BIBTEX
Fu, L., Niu, B., Zhu, Z., Wu, S., and Li, W. (2012). CD-HIT: accelerated for clustering the next-generation sequencing data. Bioinformatics 28, 3150–3152. doi: 10.1093/BIOINFORMATICS/BTS565
Ganzert, L., Schirmack, J., Alawi, M., Mangelsdorf, K., Sand, W., Hillebrand-Voiculescu, A., et al. (2014). Methanosarcina spelaei sp. nov., a methanogenic archaeon isolated from a floating biofilm of a subsurface sulphurous lake. Int. J. Syst. Evol. Microbiol. 64, 3478–3484. doi: 10.1099/IJS.0.064956-0
Ghosh, S., Kuisiene, N., and Cheeptham, N. (2017). The cave microbiome as a source for drug discovery: Reality or pipe dream? Biochem. Pharmacol. 134, 18–34. doi: 10.1016/J.BCP.2016.11.018
Wickham, H. (2016). ggplot2: Elegant Graphics for Data Analysis. Netherland: Springer-Verlag New York.
Haroon, M. F., Hu, S., Shi, Y., Imelfort, M., Keller, J., Hugenholtz, P., et al. (2013). Anaerobic oxidation of methane coupled to nitrate reduction in a novel archaeal lineage. Nature 500, 567–570. doi: 10.1038/NATURE12375
Hippe, H., Caspari, D., Fiebig, K., and Gottschalk, G. (1979). Utilization of trimethylamine and other N-methyl compounds for growth and methane formation by Methanosarcina barkeri. Proc. Natl. Acad. Sci. U.S.A. 76:494. doi: 10.1073/PNAS.76.1.494
Hutchens, E., Radajewski, S., Dumont, M. G., McDonald, I. R., and Murrell, J. C. (2004). Analysis of methanotrophic bacteria in Movile Cave by stable isotope probing. Environ. Microbiol. 6, 111–120. doi: 10.1046/j.1462-2920.2003.00543.x
Inagaki, F., Tsunogai, U., Suzuki, M., Kosaka, A., Machiyama, H., Takai, K., et al. (2004). Characterization of C1-metabolizing prokaryotic communities in methane seep habitats at the Kuroshima Knoll, southern Ryukyu Arc, by analyzing pmoA, mmoX, mxaF, mcrA, and 16S rRNA genes. Appl. Environ. Microbiol. 70, 7445–7455. doi: 10.1128/AEM.70.12.7445-7455.2004
Jones, D. S., Albrecht, H. L., Dawson, K. S., Schaperdoth, I., Freeman, K. H., Pi, Y., et al. (2011). Community genomic analysis of an extremely acidophilic sulfur-oxidizing biofilm. ISME J. 61, 158–170. doi: 10.1038/ismej.2011.75
Jones, D. S., Schaperdoth, I., and Macalady, J. L. (2016). Biogeography of sulfur-oxidizing Acidithiobacillus populations in extremely acidic cave biofilms. ISME J. 1012, 2879–2891. doi: 10.1038/ismej.2016.74
Karthikeyan, O. P., Smith, T. J., Dandare, S. U., Parwin, K. S., Singh, H., Loh, H. X., et al. (2021). Metal(loid) speciation and transformation by aerobic methanotrophs. Microbiome 91:156. doi: 10.1186/S40168-021-01112-Y
Karwautz, C., Kus, G., Stöckl, M., Neu, T. R., and Lueders, T. (2017). Microbial megacities fueled by methane oxidation in a mineral spring cave. ISME J. 121, 87–100. doi: 10.1038/ismej.2017.146
Knief, C. (2015). Diversity and Habitat Preferences of Cultivated and Uncultivated Aerobic Methanotrophic Bacteria Evaluated Based on pmoA as Molecular Marker. Front. Microbiol. 6:1346. doi: 10.3389/FMICB.2015.01346
Knittel, K., and Boetius, A. (2009). Anaerobic oxidation of methane: progress with an unknown process. Annu. Rev. Microbiol. 63, 311–334. doi: 10.1146/ANNUREV.MICRO.61.080706.093130
Kolb, S. (2009). The quest for atmospheric methane oxidizers in forest soils. Environ. Microbiol. Rep. 1, 336–346. doi: 10.1111/J.1758-2229.2009.00047.X
Kumaresan, D., Stephenson, J., Doxey, A. C., Bandukwala, H., Brooks, E., Hillebrand-Voiculescu, A., et al. (2018). Aerobic proteobacterial methylotrophs in Movile Cave: genomic and metagenomic analyses. Microbiome 6:1. doi: 10.1186/s40168-017-0383-2
Laso-Pérez, R., Wegener, G., Knittel, K., Widdel, F., Harding, K. J., Krukenberg, V., et al. (2016). Thermophilic archaea activate butane via alkyl-coenzyme M formation. Nature 539, 396–401. doi: 10.1038/NATURE20152
Lennon, J. T., Nguyễn-Thùy, D., Phạm, T. M., Drobniak, A., Tạ, P. H., Phạm, N., et al. (2017). Microbial contributions to subterranean methane sinks. Geobiology 15, 254–258. doi: 10.1111/GBI.12214
Letunic, I., and Bork, P. (2021). Interactive Tree Of Life (iTOL) v5: an online tool for phylogenetic tree display and annotation. Nucleic Acids Res. 49, W293–W296. doi: 10.1093/NAR/GKAB301
Leu, A. O., Cai, C., McIlroy, S. J., Southam, G., Orphan, V. J., Yuan, Z., et al. (2020). Anaerobic methane oxidation coupled to manganese reduction by members of the Methanoperedenaceae. ISME J. 14, 1030–1041. doi: 10.1038/S41396-020-0590-X
Liesack, W., Schnell, S., and Revsbech, N. P. (2000). Microbiology of flooded rice paddies. FEMS Microbiol. Rev. 24, 625–645. doi: 10.1111/J.1574-6976.2000.TB00563.X
Macalady, J. L., Jones, D. S., and Lyon, E. H. (2007). Extremely acidic, pendulous cave wall biofilms from the Frasassi cave system, Italy. Environ. Microbiol. 9, 1402–1414. doi: 10.1111/J.1462-2920.2007.01256.X
Martin-Pozas, T., Gonzalez-Pimentel, J. L., Jurado, V., Cuezva, S., Dominguez-Moñino, I., Fernandez-Cortes, A., et al. (2020). Microbial Activity in Subterranean Ecosystems: Recent Advances. Appl. Sci. 10:8130. doi: 10.3390/APP10228130
Mattey, D. P., Fisher, R., Atkinson, T. C., Latin, J. P., Durrell, R., Ainsworth, M., et al. (2013). Methane in underground air in Gibraltar karst. Earth Planet. Sci. Lett. 374, 71–80. doi: 10.1016/J.EPSL.2013.05.011
McDonald, I. R., Bodrossy, L., Chen, Y., and Murrell, J. C. (2008). Molecular Ecology Techniques for the Study of Aerobic Methanotrophs. Appl. Environ. Microbiol. 74:1305. doi: 10.1128/AEM.02233-07
Menzel, P., Ng, K. L., and Krogh, A. (2016). Fast and sensitive taxonomic classification for metagenomics with Kaiju. Nat. Commun. 71:1257. doi: 10.1038/ncomms11257
Minh, B. Q., Schmidt, H. A., Chernomor, O., Schrempf, D., Woodhams, M. D., Von Haeseler, A., et al. (2020). IQ-TREE 2: new models and efficient methods for phylogenetic inference in the genomic era. Mol. Biol. Evol. 37, 1530–1534. doi: 10.1093/MOLBEV/MSAA015
Narrowe, A. B., Borton, M. A., Hoyt, D. W., Smith, G. J., Daly, R. A., Angle, J. C., et al. (2019). Uncovering the diversity and activity of methylotrophic methanogens in freshwater wetland soils. mSystems 4:e00320–19. doi: 10.1128/MSYSTEMS.00320-19
Ortiz, M., Neilson, J. W., Nelson, W. M., Legatzki, A., Byrne, A., Yu, Y., et al. (2013). Profiling bacterial diversity and taxonomic composition on speleothem surfaces in kartchner caverns, AZ. Microb. Ecol. 65, 371–383. doi: 10.1007/S00248-012-0143-6/FIGURES/4
Peabody, M. A., Van Rossum, T., Lo, R., and Brinkman, F. S. L. (2015). Evaluation of shotgun metagenomics sequence classification methods using in silico and in vitro simulated communities. BMC Bioinform. 16:362. doi: 10.1186/S12859-015-0788-5
Pratscher, J., Vollmers, J., Wiegand, S., Dumont, M. G., and Kaster, A. K. (2018). Unravelling the identity, metabolic potential and global biogeography of the atmospheric methane-oxidizing upland soil cluster α. Environ. Microbiol. 20, 1016–1029. doi: 10.1111/1462-2920.14036
Price, M. N., Dehal, P. S., and Arkin, A. P. (2010). FastTree 2 - Approximately maximum-likelihood trees for large alignments. PLoS One. 5:e9490. doi: 10.1371/journal.pone.0009490
R Core Team (2021). R: A Language and Environment for Statistical Computing. Vienna: R Foundation for Statistical Computing.
Ross, A. M., Peoples, L. M., Bilbrey, E. M., and Church, M. J. (2022). Draft metagenome-assembled genomes from methane-rich echo lake, montana. Microbiol. Resour. Announc. 11:e0111221. doi: 10.1128/MRA.01112-21
Sánchez-Salazar, E. A., Hernández-Jaimes, L., Breton-Deval, L., and Sánchez-Reyes, A. (2022). Draft genome sequence of methanobacterium paludis ibt-c12, recovered from sediments of the Apatlaco River, Mexico. Microbiol. Resour. Announc. 11, 906–927. doi: 10.1128/MRA.00906-21
Sarbu, S. (2000). “A chemoautotrophically based groundwater ecosystem,” in Subterranean Ecosystems, eds H. Wilkens, D. Culver, and W. Humphreys (Amsterdam?: Elsevier), 319–343.
Sarbu, S. M., Kane, T. C., and Kinkle, B. K. (1996). A chemoautotrophically based cave ecosystem. Science 272, 1953–1954. doi: 10.1126/science.272.5270.1953
Schimmelmann, A., Fernandez-Cortes, A., Cuezva, S., Streil, T., and Lennon, J. T. (2018). Radiolysis via radioactivity is not responsible for rapid methane oxidation in subterranean air. PLoS One 13:e0206506. doi: 10.1371/JOURNAL.PONE.0206506
Sievers, F., and Higgins, D. G. (2018). Clustal Omega for making accurate alignments of many protein sequences. Protein Sci. 27:135. doi: 10.1002/PRO.3290
Skennerton, C. T., Chourey, K., Iyer, R., Hettich, R. L., Tyson, G. W., and Orphan, V. J. (2017). Methane-fueled syntrophy through extracellular electron transfer: uncovering the genomic traits conserved within diverse bacterial partners of anaerobic methanotrophic archaea. MBio 8:e530–e517. doi: 10.1128/MBIO.00530-17
Smith, K. S., and Ingram-Smith, C. (2007). Methanosaeta, the forgotten methanogen? Trends Microbiol. 15, 150–155. doi: 10.1016/J.TIM.2007.02.002
Speth, D. R., and Orphan, V. J. (2018). Metabolic marker gene mining provides insight in global mcrA diversity and, coupled with targeted genome reconstruction, sheds further light on metabolic potential of the Methanomassiliicoccales. PeerJ 6:e5614. doi: 10.7717/PEERJ.5614/SUPP-9
Steinegger, M., Mirdita, M., and Söding, J. (2018). Protein-level assembly increases protein sequence recovery from metagenomic samples manyfold. bioRxiv, 16, 603–606. doi: 10.1101/386110
Stewart, L. C., Algar, C. K., Fortunato, C. S., Larson, B. I., Vallino, J. J., Huber, J. A., et al. (2019). Fluid geochemistry, local hydrology, and metabolic activity define methanogen community size and composition in deep-sea hydrothermal vents. ISME J. 13, 1711–1721. doi: 10.1038/S41396-019-0382-3
Stoecker, K., Bendinger, B., Schöning, B., Nielsen, P. H., Nielsen, J. L., Baranyi, C., et al. (2006). Cohn’s Crenothrix is a filamentous methane oxidizer with an unusual methane monooxygenase. Proc. Natl. Acad. Sci.U.S.A. 103, 2363–2367. doi: 10.1073/PNAS.0506361103
Tavormina, P. L., Orphan, V. J., Kalyuzhnaya, M. G., Jetten, M. S. M., and Klotz, M. G. (2011). A novel family of functional operons encoding methane/ammonia monooxygenase-related proteins in gammaproteobacterial methanotrophs. Environ. Microbiol. Rep. 3, 91–100. doi: 10.1111/J.1758-2229.2010.00192.X
Tovo, A., Menzel, P., Krogh, A., Cosentino Lagomarsino, M., and Suweis, S. (2020). Taxonomic classification method for metagenomics based on core protein families with Core-Kaiju. Nucleic Acids Res. 48:E93. doi: 10.1093/NAR/GKAA568
Turrini, P., Tescari, M., Visaggio, D., Pirolo, M., Lugli, G. A., Ventura, M., et al. (2020). The microbial community of a biofilm lining the wall of a pristine cave in Western New Guinea. Microbiol. Res. 241:126584. doi: 10.1016/J.MICRES.2020.126584
van Teeseling, M. C. F., Pol, A., Harhangi, H. R., van der Zwart, S., Jetten, M. S. M., Op den Camp, H. J. M., et al. (2014). Expanding the verrucomicrobial methanotrophic world: description of three novel species of Methylacidimicrobium gen. Appl. Environ. Microbiol. 80, 6782–6791. doi: 10.1128/AEM.01838-14
Waring, C. L., Hankin, S. I., Griffith, D. W. T., Kertesz, M. A., Kobylski, V., Wilson, N. L., et al. (2017). Seasonal total methane depletion in limestone caves. Sci. Rep. 7:8314. doi: 10.1038/S41598-017-07769-6
Wiseschart, A., Mhuantong, W., Tangphatsornruang, S., Chantasingh, D., and Pootanakit, K. (2019). Shotgun metagenomic sequencing from Manao-Pee cave, Thailand, reveals insight into the microbial community structure and its metabolic potential. BMC Microbiol. 19:144. doi: 10.1186/S12866-019-1521-8
Zada, S., Xie, J., Yang, M., Yang, X., Sajjad, W., Rafiq, M., et al. (2021). Composition and functional profiles of microbial communities in two geochemically and mineralogically different caves. Appl. Microbiol. Biotechnol. 105, 8921–8936. doi: 10.1007/S00253-021-11658-4
Zhao, R., Wang, H., Cheng, X., Yun, Y., and Qiu, X. (2018). Upland soil cluster γ dominates the methanotroph communities in the karst Heshang Cave. FEMS Microbiol. Ecol. 94:192. doi: 10.1093/FEMSEC/FIY192
Zhou, J., Holmes, D. E., Tang, H. Y., and Lovley, D. R. (2021). Correlation of key physiological properties of methanosarcina isolates with environment of origin. Appl. Environ. Microbiol. 87, 1–15. doi: 10.1128/AEM.00731-21
Keywords: methanotrophs, methanogens, methane, karst caves, biogeochemical cycles
Citation: Allenby A, Cunningham MR, Hillebrand-Voiculescu A, Comte J-C, Doherty R and Kumaresan D (2022) Occurrence of methane-oxidizing bacteria and methanogenic archaea in earth’s cave systems—A metagenomic analysis. Front. Ecol. Evol. 10:909865. doi: 10.3389/fevo.2022.909865
Received: 31 March 2022; Accepted: 21 July 2022;
Published: 15 August 2022.
Edited by:
Tiziana Di Lorenzo, Research Institute on Terrestrial Ecosystems, Department of Earth System Sciences and Technologies for the Environment (CNR), ItalyReviewed by:
Hongmei Wang, China University of Geosciences Wuhan, ChinaStefano Amalfitano, Water Research Institute, Department of Earth System Sciences and Technologies for the Environment (CNR), Italy
Clemens Karwautz, University of Vienna, Austria
Copyright © 2022 Allenby, Cunningham, Hillebrand-Voiculescu, Comte, Doherty and Kumaresan. This is an open-access article distributed under the terms of the Creative Commons Attribution License (CC BY). The use, distribution or reproduction in other forums is permitted, provided the original author(s) and the copyright owner(s) are credited and that the original publication in this journal is cited, in accordance with accepted academic practice. No use, distribution or reproduction is permitted which does not comply with these terms.
*Correspondence: Alexander Allenby, YWFsbGVuYnkwMkBxdWIuYWMudWs=; Deepak Kumaresan, ZC5rdW1hcmVzYW5AcXViLmFjLnVr