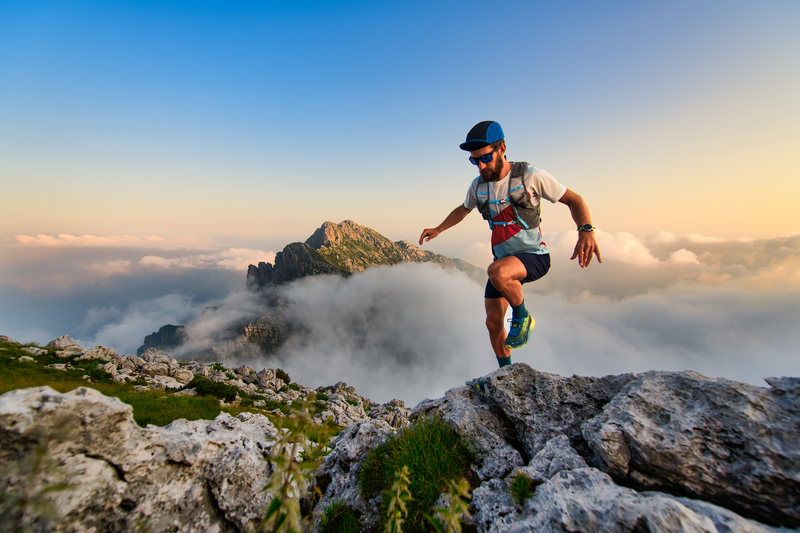
95% of researchers rate our articles as excellent or good
Learn more about the work of our research integrity team to safeguard the quality of each article we publish.
Find out more
ORIGINAL RESEARCH article
Front. Ecol. Evol. , 13 June 2022
Sec. Evolutionary and Population Genetics
Volume 10 - 2022 | https://doi.org/10.3389/fevo.2022.907414
Plant populations at the leading edge of the species’ native range often exhibit genetic structure as a result of genetic drift and adaptation to harsh environmental conditions. Hence, they are likely to harbour rare genetic adaptations to local environmental conditions and therefore are of particular interest to understand climate adaptation. We examined genetic structure of nine northern marginal mainland, peninsular and isolated island natural populations of northern red oak (Quercus rubraL.), a valuable long-lived North American hardwood tree species, covering a wide climatic range, using 17 nuclear microsatellites. We found pronounced genetic differentiation of a disjunct isolated island population from all mainland and peninsular populations. Furthermore, we observed remarkably strong fine-scale spatial genetic structure (SGS) in all investigated populations. Such high SGS values are uncommon and were previously solely observed in extreme range-edge marginal oak populations in one other study. We found a significant correlation between major climate parameters and SGS formation in northern range-edge red oak populations, with more pronounced SGS in colder and drier regions. Most likely, the harsh environment in leading edge populations influences the density of reproducing trees within the populations and therefore leads to restricted overlapping of seed shadows when compared to more central populations. Accordingly, SGS was negatively correlated with effective population size and increased with latitude of the population locations. The significant positive association between genetic distances and precipitation differences between populations may be indicative of isolation by adaptation in the observed range-edge populations. However, this association was not confirmed by a multiple regression analysis including geographic distances and precipitation distances, simultaneously. Our study provides new insights in the genetic structure of long-lived tree species at their leading distribution edge.
Long-lived trees are especially challenged by the changing environmental conditions in the face of climate change. Largely outcrossing tree species with effective means of pollen and seed dispersal harbour considerable amounts of genetic variation, to cope with new environmental conditions during their lifetimes (Hamrick et al., 1979; Rajora et al., 2000; Khodwekar and Gailing, 2017; Rajora and Zinck, 2021). However, it is unclear whether adaptive genetic variation and phenotypic plasticity are sufficient for them to adapt to rapidly changing conditions (Aitken et al., 2008; Depardieu et al., 2020). Range margin populations are expected to play significant roles in species’ range expansion anticipated under climate change. Therefore, genetic variation in marginal tree populations under extreme conditions is of high interest for contemporary research to predict species’ adaptability to persist in forest systems (Hampe and Petit, 2005; Pandey and Rajora, 2012a,b; Chhatre and Rajora, 2014). Since marginal populations of sessile plant species are confronted with extreme abiotic conditions, they are often exposed to stronger natural selection (Chhatre and Rajora, 2014). Hence, range-edge populations are especially challenged by the risk of extinction, and often exhibit low levels of allelic diversity as compared to central populations (e.g., Arnaud-Haond et al., 2006; Pandey and Rajora, 2012a; Chhatre and Rajora, 2014). On the other hand, high selective pressure makes them likely to develop highly adapted genotypes in theory (Willi et al., 2007), which might be important to maintain or expand their distribution range (Rehm et al., 2015). Even though experimental support for this assumption remains absent, range-edge populations represent locations of extremes and are therefore of high interest to study local adaptation to harsh environmental conditions (Gibson et al., 2009).
The Isolation by distance (IBD) theory (Wright, 1943) predicts differences between distant subpopulations as a result of random genetic drift and geographically limited gene dispersal. If different subpopulations face restricted gene flow over long time periods, random loss of alleles in small subpopulations can leave signatures in the genome (Willi et al., 2007). This applies especially to range-edge plant populations, due to the restricted pollen dispersal and founder events (Pohjanmies et al., 2016). As physical barriers have a severe impact on the gene flow between different subpopulations, the stepping stone model (Kimura and Weiss, 1964) was proposed as an expansion of the IBD theory. Apart from IBD, additional factors can impact the genetic composition of separated subpopulations. Abiotic factors like temperature or precipitation can act selectively on adaptive alleles (RamÍrez-Valiente et al., 2009). Genetic differentiation can arise from divergent environmental selection (Wang and Bradburd, 2014) resulting in isolation by adaptation (IBA) (Nosil et al., 2008) and various studies reported signs of environmental impact on genetic differentiation (Sexton et al., 2014). Since marginal populations are assumed to persist under borderline conditions in a harsh environment, occurrence of IBA is more likely in range-edge populations. The presence of IBA and IBD can be detected by comparing a genetic distance matrix with abiotic and geographic parameter matrices, respectively. For IBA, a significant correlation between genetic and environmental parameter distances is expected, while a significant correlation between geographic and genetic distances would indicate IBD (Funk et al., 2011; Dewoody et al., 2015).
Seed accumulation near mother trees due to gravity effects on heavy seeds (Sagnard et al., 2011), and animal seed caching (Peakall and Beattie, 1995) contribute to the formation of fine-scale spatial genetic structures (SGS) in oaks. It has been shown, that inbreeding has a strong effect on the formation of fine-scale SGS (Epperson, 2007). Contrarily, outcrossing and self-incompatible species generally exhibit lower degrees of SGS (Vekemans and Hardy, 2004). Range-edge conditions, such as low effective population density and isolation from conspecific populations, can occur in fragmented or isolated populations, and are positively correlated with the formation of fine-scale SGS (Vekemans and Hardy, 2004), as it was observed for example in maritime pine, Pinus pinaster Aiton (De-Lucas et al., 2009), eastern white cedar, Thuja occidentalis L. (Pandey and Rajora, 2012b), and Sitka spruce, Picea sitchensis (Bong.) Carrière (Gapare and Aitken, 2005). Therefore, in marginal populations with low adult tree densities, comparatively high SGS can be expected. Fine-scale SGS analyses are suitable to provide insights into basic evolutionary processes, such as gene dispersal, genetic drift, and natural selection (Vekemans and Hardy, 2004; Cavers et al., 2005). Knowledge of fine-scale SGS can also provide a basis for the development of representative sampling schemes for seed harvest and scientific research (Vornam et al., 2004).
Northern red oak (Quercus rubra L., section Lobatae) is a wind pollinated hardwood tree species, widely distributed in North America, where it grows from Quebec, Ontario, New Brunswick, and Nova Scotia in southern Canada southwards to south-western Georgia and Alabama, United States (Godfrey, 1988). During the last glacial maximum, the northern edge of the contemporary distribution range was covered with ice. Northern red oak re-colonized the area after the decline of the glaciers probably via diverse migration routes (Schlarbaum et al., 1982). Recent colonization and steep environmental gradients make Q. rubra a good model to study the effects of neutral and adaptive processes on genetic variation in range-edge populations. However, studies focusing on Q. rubra range-edge populations are rather limited. A comprehensive single nucleotide polymorphism study based on 73 evenly distributed northern red oak populations from the native range revealed a clinal trend of genetic variation along latitude and longitude (Merceron et al., 2017). Furthermore, several studies reported genetic diversity parameters based on nuclear microsatellites from the centre of the native range (Aldrich et al., 2003), as well as for populations from the northern edge of the natural distribution area (Lind and Gailing, 2013; Sullivan et al., 2013; Lind-Riehl et al., 2014; Borkowski et al., 2017). Borkowski et al. (2017) reported a loss of allelic richness toward the north of the native range of northern red oak due to postglacial migration. Range-edge populations have been investigated in Q. rubra and the closely related Quercus ellipsoidalis (Sullivan et al., 2013; Lind-Riehl et al., 2014; Lind-Riehl and Gailing, 2015) but only from a small part of the species’ range in the Great Lakes Region. Therefore, information on genetic structure of northern red oak populations from other parts of its marginal range, especially from the unstudied eastern range, is crucial.
Potentially as a result of low density of reproducing trees under suboptimal environmental conditions, pronounced fine-scale SGS was observed in northern range-edge populations of some oak and conifer species (Gapare and Aitken, 2005; Pandey and Rajora, 2012b; Lind-Riehl and Gailing, 2015). In northern red oak, however, knowledge of the degree of fine-scale SGS remains limited. To our knowledge, reports on fine-scale SGS in Q. rubra have solely been published on juvenile northern red oak trees, colonizing an aspen (Populus tremuloides Michx.)- white pine (Pinus strobus L.) forest, which may undergo pronounced changes in SGS while maturing (Jones et al., 2006), and to compare SGS between managed and unmanaged populations (Lind-Riehl and Gailing, 2015). We are not aware of additional reports of SGS in marginal populations of northern red oak.
In the present study, we aim to evaluate the genetic composition, as well as the spatial genetic structure of northern red oak populations from various locations (mainland, peninsula and isolated island) at the northern margin of the natural distribution area. We hypothesize that (1) there is strong genetic differentiation at a small geographic scale between populations from the northern edge of the native range as a result of founder effects and selection during postglacial remigration, (2) strong within-population fine-scale spatial genetic structures exist due to the comparatively low gene flow in range-edge populations and low effective population density, (3) signatures of isolation by adaptation may be detected as a result of steep environmental gradients and adaptation to harsh range-edge conditions, and (4) the recent re-colonization of the northern range-edge resulted in weak patterns of isolation by distance.
We sampled a total of eight natural Q. rubra populations from the northern margins of the species range in four provinces in Canada (Figure 1 and Table 1). Three of these populations were from mainland Ontario and New Brunswick, four from Nova Scotia peninsula, and one from Prince Edward Island (PEI). The Phelps and McConnell Lake Road populations sampled from Ontario are preserved as growth and yield research plots and are believed to be of post-fire origin. The Crafts Cove population from New Brunswick is located in a private forest near the land margin on one side and surrounded by farms on others in a fragmented landscape. Four populations sampled on the peninsula of Nova Scotia, which is only linked to the mainland by the sparsely forested Chignecto isthmus, are believed to be of post-fire origin. These populations are relatively close to each other within a distance of 60 km. The Crafts Cove population from New Brunswick is separated by the Bay of Fundy from the Nova Scotia populations. The isolated PEI red oak population is located in the East Royalty area at the northeast limit of Charlottetown city. With the commencement of European settlement, much of the Prince Edward Island forest was cleared in eighteenth and early nineteenth centuries for settlements and agriculture. The sampled Charlottetown northern red oak population in the current study represents one of the last remnants of the red oak tree community on the island. This population is located in the protected natural forest “Royalty Oaks Natural Area” of Charlottetown, which had been in private property from 1819 to the 1980s and exhibited the healthiest and strongest phenotypes in terms of trunk height and form. We aimed to sample 50 individuals per population. However, in two populations only 31 and 40 individuals could be sampled (Table 1). Trees were randomly selected within each population, maintaining a distance of at least 10 m between the sampled trees. All sampled populations are of natural origin and have previously been investigated using chloroplast microsatellite markers (Götz et al., 2020). Additionally, we included data for a population, which was sampled previously from the Upper Peninsula of Michigan (Sullivan et al., 2013). This particular population (Brockway Mountain, BM in this study; BR-1 in Sullivan et al., 2013) is located at the range-edge of the species. Even though there were many northern red oak trees in this region, the sampled population in the Brockway Mountains consisted of mostly shrubby trees (Lind and Gailing, 2013). The habitus of the sampled trees supports the assumption of range-edge conditions, as the shrubby growth is most likely a result of the heavy winds and snow loads during the winter months and points to a transition to the conifer dominated forests, which are common in more northern regions. The distribution of the sampled individuals within populations is presented in Supplementary Figure 1. Fresh and clean leaves from each of the 427 trees sampled from eight Canadian red oak populations were collected and placed, stored and dried in paper bags with silica gel until DNA extraction. All plant material (leaf samples, and extracted DNA) of the current study is deposited at the Department of Forest Genetics and Forest Tree Breeding, University of Göttingen and is available upon request. Research permits were not required because all populations except the Crafts Cove population were located on public land without any conservation issue or designation. The owner of the farm on which Crafts Cove population is located gave permission and helped us for sample collection as outlined in Acknowledgements.
Figure 1. Location and sample size of the northern red oak populations within their natural distribution area (highlighted). BM, Brockway Mountain; MR, McConnell Lake Road; PH, Phelps; CC, Crafts Cove; OL, Oak Lake; MB, Maitland Bridge; NM, Nine Mile Woods; WL, Wentzells Lake; CT, Charlottetown.
DNA was extracted from about one cm2 of dried leaves, using the DNeasy™ 96 Plant Kit (Qiagen; Hilden, Germany) following the manufacturer’s manual. All samples were genotyped at 20 microsatellite markers, of which three (quru-GA-1F07, FIR024, FIR104) had to be removed due to the difficulty in delimiting different alleles (Supplementary Table 1). The actual marker set included seven nuclear genomic simple sequence repeat (nSSR) markers [1p10, 2p24, 3A05, 3D15 (Sullivan et al., 2013), quru-GA-0C11, quru-GA-1C06, quru-GA-0E09 (Aldrich et al., 2003)] and ten expressed sequence tag simple sequence repeat (EST-SSR) markers (FIR013, FIR028, FIR031, FIR035, GOT021, GOT040, PIE040, PIE125, VIT023, VIT107), developed for Q. robur (Durand et al., 2010) and adapted to Q. rubra (Sullivan et al., 2013). Five different multiplex PCR reactions were prepared for each sample, by multiplexing three to seven primer pairs in PCR reactions (Supplementary Table 1). A touchdown PCR protocol was used for each multiplex with the following parameters: 15 min of denaturation at 95°C, followed by ten cycles of 60 s denaturation at 94°C, 60 s annealing at 60°C decreased by 1°C each cycle, and 60 s of elongation at 72°C. Afterwards, 25 cycles of denaturation (94°C/60 s), annealing (50°C/60 s) and elongation (72°C/60 s) were run. Finally, 20 min of elongation was used. The plates were cooled down to 16°C before electrophoretic separation. Forward primers were labelled with different fluorescent dyes as displayed in Supplementary Table 1.
The size of amplified DNA fragments and genotypes of individual sampled trees were determined with Genemapper software v3.7 (Applied Biosystems, Foster City, CA, United States) after electrophoretic separation of diluted PCR products on an ABI Genetic Analyzer 3130xl (Applied Biosystems, Foster City, CA, United States).
We used the statistical programming language R (R core team, 2018), with R studio (RStudio Team, 2020) to calculate the following genetic parameters for each population: Number of alleles per locus (A), observed heterozygosity (Ho), expected heterozygosity (He), allelic richness (AR), and fixation index (FIS). The methods were implemented in the DiveRsity package (Keenan et al., 2013). A rarefaction approach to the lowest sample size was applied to calculate allelic richness for comparability. Ninety five percent confidence intervals of the Fixation index (FIS) were based on 1,000 bootstraps. GenAlEx 6.5 software (Peakall and Smouse, 2006) was used to estimate the number and frequency of private alleles (AP). Bottleneck analysis was carried out using the BOTTLENECK 1.2.02 software (Cornuet and Luikart, 1996), based on a stepwise mutational model, 1000 iterations, and the Wilcoxon test for heterozygosity excess. Tests for linkage disequilibrium (pairwise locus comparisons, Markov chain approach, 10,000 dememorization, 100 batches, 5,000 iterations), deviation from Hardy-Weinberg equilibrium (exact test for each locus and population, Markov chain approach with 10,000 dememorization, 20 batches, and 5,000 interactions per batch), and estimation of null alleles (for all loci and populations, maximum likelihood estimation, EM algorithm) were performed using the GENEPOP 4.7.5 software (Rousset, 2008).
Contemporary effective population size (Ne) was estimated using the NeEstimator 2.1 software (Do et al., 2014) employing the Linkage Disequilibrium method described by Waples (2006) and a critical allele frequency value of 0.02, which was suggested for sample sizes >25 (Waples and Do, 2010) and used by Rajora and Zinck (2021) for eastern white pine, Pinus strobus.
Interpopulation genetic differentiation and genetic structure of nine red oak populations were examined using FST, genetic distance, principal coordinate, and Bayesian STRUCTURE analyses.
Bayesian cluster analysis was done using the STRUCTURE 2.3.4 software (Pritchard et al., 2000). Data were analysed using an admixture model with 105 burn-ins, 106 MCMC repetitions and 20 repetitions for each K value, ranging from 1 to 10. Allele frequencies were assumed to be correlated. We used both the default and LOCPRIOR models separately for the STRUCTURE analysis. The LOCPRIOR model considers sampling locations for genetic assignment/clustering (Hubisz et al., 2009). The most likely K-value was determined using ΔK of the method of Evanno et al. (2005) in STRUCTURE HARVESTER (Earl and VonHoldt, 2012).1 The high-performance computing cluster of the association of scientific data processing of University of Göttingen was used to perform resource intensive data analyses. The python program StrAuto (Chhatre and Emerson, 2017) enabled us to use parallel computing processing units.
FST values between each population and its complement (population-specific FST values) were calculated using GESTE 2.0 (Foll and Gaggiotti, 2006). This software uses a hierarchical Bayesian approach to estimate FST values and adds an option to normalize data for several factors to check whether they are associated with FST formation. Parameters were set to a sample size of 20,000, thinning interval of 25, 100 pilot runs with 5,000 iterations, and 50,000 additional burn-ins. Pairwise FST values, as well as overall F-statistics were calculated using Weir and Cockerham’s FST estimator Θ (Weir and Cockerham, 1984), as implemented in the FSTAT 2.9.4 software (Goudet, 2003).
Isolation by distance was tested by performing Mantel tests between genetic and geographic distances using 10,000 permutations. Isolation by adaptation was tested by performing Mantel tests between the genetic and climate distance matrices. A multiple regression model in the R package MMRR (Wang, 2013) was used to test simultaneously for an effect of environmental factors (precipitation, temperature, and solar radiation) and geographical distances on the genetic distance matrix. Pairwise differences in environmental parameters were calculated using the Euclidian distance between mean annual population location values. The genetic distance matrix was calculated in GenAlEx using the Euclidian distance (Smouse and Peakall, 1999). Furthermore, principal coordinates analysis (PCoA) was performed based on a standardized covariance method of the genotypic genetic distance matrix between populations using GenAlEx. The corresponding scatterplot was examined in a three dimensional space using the ParaView software (Ahrens et al., 2005) to examine the most representative two dimensional view for presentation.
FST outlier analysis was carried out using the Bayescan 2.1 software (Foll and Gaggiotti, 2006), performing multiple runs based on a sample size of 5,000, a thinning interval of 10, 25 pilot runs, and 50,000 additional burn ins, each. Prior odds were set to 10, 100, 1,000, and 10,000, respectively.
Presence of SGS was inferred using the kinship coefficient Fij (Loiselle et al., 1995). For this purpose, we used the SPAGeDi 1.5 software (Hardy and Vekemans, 2002). Ten thousand permutations were run to validate the results statistically. Distance class size was determined by SPAGeDi 1.5 to provide similar distance classes within the single runs. The best number of classes was found for each population using a trial-and-error approach. We aimed for at least 100 comparisons per class, and a low oscillating graph. Four to eight distance classes, depending on the population, were considered optimal. To quantify the extent of spatial genetic structure, Sp statistics (Vekemans and Hardy, 2004) were calculated using the formula:
where bF is the regression slope of the kinship coefficient F on the natural logarithm of spatial distance, and F1 is the mean kinship coefficient between individuals belonging to the first distance class. Sp statistics provide a more robust way to compare SGS than the sole observation of significant distance classes, or regression slopes do. To examine the SGS extent, we performed an autocorrelation analysis of all populations combined, with the GenAlEx 6.5 software (Peakall and Smouse, 2006). This large sample size enabled us to investigate SGS at a finer scale, using fixed distance classes of 5 meters, while keeping a high number (>100) of comparisons. Significance of SGS extent was tested using a one-sided test, applying a level of significance of 0.05.
Correlation analysis of Sp values with different climate data was done using standard functions of R (R core team, 2018). Normal distribution was tested for all parameters (Shapiro-Wilk test). Significance of the correlation coefficients was tested using Pearson’s product moment correlation. Additionally, Mantel tests (10,000 permutations) were performed between a distance matrix of Sp values and environmental distance matrices. The climate data used for the correlation analyses were taken from the public climate data base WorldClim (Fick and Hijmans, 2017), represented by a 30 s raster (about 1 km2). To provide representative data for long living species, we used the mean values of a 30 years’ time frame, ranging from 1970 CE to 2000 CE. The open source program QGIS (QGIS Development Team, 2009) was used to extract climate data from the provided raster files. Data management was done using Microsoft Office (Redmond, WA, United States). Graphical adjustment for visualization of different figures was done using the vector graphics editor Inkscape (Inkscape Project, 2020).
Genetic diversity parameters, FIS and Ne for the studied red oak populations are provided in Table 2. Overall, Brockway Mountain range-edge population had the highest genetic diversity, and the isolated island Charlottetown population showed the lowest genetic diversity with the exception of observed heterozygosity. Despite its relatively large sample size, the Charlottetown population exhibited the lowest average number of alleles per locus, as well as allelic richness, which was adjusted for unequal sample sizes. Furthermore, we did not observe any private alleles within the Charlottetown population. The number of private alleles for the remaining populations ranged from one to nine. Expected and observed heterozygosity were similar in all sampled populations, with mean values of 0.67 and 0.64, respectively. Again, Charlottetown population deviated from the pattern, as it was the only population, which exhibited a higher HO than HE value, resulting in a significant negative fixation index. Significantly positive fixation indices, on the other hand, were observed in six populations (BM, MB, WL, OL, PH, MR). Nine Mile Woods and Crafts Cove populations exhibited FIS values which were not significantly different from zero.
Table 2. Hardy-Weinberg equilibrium test (HWE, Fisher’s exact test), p-values mean values of linkage disequilibrium (LD) test between all pairwise loci comparisons, mean estimates of null allele frequencies (Null), population-specific FST value, contemporary (Ne) effective population size and its 95% confidence intervals, average number of alleles per locus (A), allelic richness (AR), number of private alleles (AP), expected (HE) and observed (HO) heterozygosity (standard error in brackets), and fixation index (FIS–95% confidence intervals in brackets) of nine northern red oak populations.
The contemporary effective population size was, overall, highest for the four populations from Nova Scotia (OL, MB, NM, WL) with estimates ranging from 189 (95% CI = 124–367) to 432 (95% CI = 221–4,517). The lowest estimated contemporary effective population size was found for the isolated Charlottetown population (22, 95% CI = 19–26) (Table 2).
Population-specific FST values ranged between 0.0120 and 0.0624 (Table 2), with the exception of the Charlottetown population, which showed a much higher population-specific FST value of 0.1500. Additional calculations with the exclusion of the Charlottetown outlier population resulted in similar values for the mainland populations (Supplementary Table 2). Pairwise FST comparisons (Supplementary Table 3) revealed predominantly low FST values, ranging from 0.004 to 0.076. The pairwise FST values for the isolated Charlottetown population were higher than for the remaining comparisons, ranging from 0.046 to 0.076, while the remaining comparisons never exceeded 0.036. Individual locus statistics are reported in Supplementary Table 4.
We observed the most prominent peak at K = 4 (Supplementary Figure 2) after performing Evanno et al. (2005) adjustments in STRUCTURE HARVESTER (Earl and VonHoldt, 2012). All populations showed genetic admixture to varying degrees. However, the Bayesian cluster analysis model resulted in clear differentiation between Charlottetown and the remaining populations for all K values (Figure 2). At K = 3, Craft Cove population was differentiated from other populations. For K = 4, the McConnell Lake Road population was further differentiated from other populations. Thus, at K = 4, each of the Charlottetown, McConnell Lake Road, and Crafts Cove populations belonged to individual genetic clusters and exhibited differentiation from the four populations from Nova Scotia (OL, MB, NM, WL), and one population from Brockway Mountain, all of which belonged to the same genetic cluster (Figure 2 and Supplementary Figure 3). This was also the case for K = 5 and K = 6. The four populations from Nova Scotia revealed a similar genetic structure; even for K > 6 subclusters (Figure 2 and Supplementary Figure 3). The Brockway Mountain population exhibited a slightly different pattern, when compared with the Nova Scotia populations, but could not be assigned to a separate subcluster. Single samples within the Brockway Mountain and Phelps populations were assigned to a shared subcluster with high probabilities. Interestingly, the Crafts Cove population showed genetic differentiation from Nova Scotia populations. Including the Locprior option in the model revealed additional differences between the populations Phelps and Brockway Mountain (Supplementary Figure 3). Differentiation increased with the assumption of additional subclusters, up to six (Supplementary Figure 3). For higher values of K, no further differentiation was observed (Supplementary Figure 3).
Figure 2. Summary bar plots of estimated membership coefficient (Q) of the studied individuals from nine red oak populations for K 2-6 from STRUCTURE analysis using the default model where population locations were not considered (no LOCPRIOR). BM, Brockway Mountain; MR, McConnell Lake Road; PH, Phelps; CC, Crafts Cove; OL, Oak Lake; MB, Maitland bridge; NM, Nine Mile Woods; WL, Wentzells Lake; CT, Charlottetown.
We ran an analysis with a modified dataset, excluding the Charlottetown outlier population. The patterns of the remaining populations were not different from the ones in the previous analysis (data not shown).
The principal coordinates analysis (PCoA) of the nine populations revealed a similar phylogeographic pattern (Supplementary Figure 4) and supported the results of the Bayesian Structure analysis (Figure 2). By far the highest differentiation was observed between the Charlottetown population and the remaining populations, of which the four populations from Nova Scotia clustered most closely. The Crafts Cove and McConnell Lake Road populations exhibited a slightly higher differentiation from the Nova Scotia populations, when compared to the Brockway Mountain and Phelps populations. These two populations were also assigned to somewhat unique genetic clusters in the Bayesian Structure analysis at K = 3 (Supplementary Figure 3). The scatterplot has been viewed interactively in a three-dimensional virtual model to verify the two-dimensional Supplementary Figure 4. The first three axes of the PCoA explained 70.3% (X = 40.6%, Y = 16.0%, Z = 13.7%) of the total variation.
The bottleneck analysis resulted in a normally L shaped distribution of allele frequencies in all populations (Supplementary Table 5). Furthermore, we found no signs of significant heterozygosity excess in any of the populations. Contrarily, there was significant heterozygosity deficiency in most of the tested populations, with the exception of the two populations Charlottetown and Crafts Cove.
The outlier analysis revealed four significant FST outliers (quru-GA-0E09, 1C06, OC11, FIR028, Supplementary Figure 5) with lower FST values than expected under neutrality at prior odds of 10 and a False Discovery Rate (FDR) of 0.05 (Supplementary Figure 5). With more conservative settings, at the prior odds of 100 or a FDR of 0.01, only quru-GA-0E09 remained significant, and at prior odds >100 or combination of prior odds of 100 and FDR < 0.05, none of the markers was detected as an outlier locus.
All investigated northern red oak populations exhibited significant kinship coefficients within the first distance class (Figure 3). Sp values ranged from 0.0067 to 0.0213 with an arithmetic mean of 0.0155. The extent of SGS within populations ranged between 33 and 137 m (Table 3). A spatial autocorrelation analysis with fixed distance classes, and combined data across all populations resulted in significant SGS (Figure 4 and Supplementary Table 6) within 45 m (0.001 < p < 0.046, one-sided) with continuously decreasing autocorrelation coefficients between 0.090 (10 m) and 0.013 (45 m). We observed no significant SGS for distance classes above 45 m (Figure 4).
Figure 3. Kinship coefficient (Fij), 95% confidence intervals (grey lines), and Sp values, representing the spatial genetic structure within nine northern red oak populations.
Table 3. Kinship coefficients of the first distance class (F1), their regression slope on natural log distance (bF), resulting Sp value, and the extent of SGS within northern red oak populations.
Figure 4. Correlogram showing autocorrelation at 10–100 m distances for all nine northern red oak populations combined. Broken lines represent 95% confidence intervals.
Sp values showed a significant and negative correlation (Supplementary Figure 6) with mean annual temperature (R2 = 0.533, p = 0.0255), annual precipitation (R2 = 0.633, p = 0.0103), and Ne (R2 = 0.562, p = 0.020) of the sampled population locations. However, we did not find a significant correlation (p = 0.312) between Sp values and the mean daily solar radiation of the sampled population locations. The correlation of Sp values with latitude were marginally significant (p = 0.077). Similar to the observed correlation between Sp values and environmental parameters described above, we observed significant correlations between differences in Sp values and temperature (p = 0.023), as well as precipitation differences (p = 0.044, Table 4).
Table 4. Matrix of the p-values and coefficient of determination (R2) of pairwise Mantel tests between distance matrices of different parameters.
Mantel tests of various pairwise parameter combinations (Table 4) showed a marginally significant correlation (R2=0.2729, p = 0.047) of the genetic distance with the geographic distance matrix. Due to the uneven distribution of distances between the populations, the frequency distribution of the original data slightly deviated from the normal distribution. However, natural log transformation of the geographic distances resulted in p and R2 values (R2 = 0.1624, p = 0.039) comparable to non-transformed data.
Mantel tests, as well as linear regression with individual variables revealed a significant correlation between matrices of genetic distance and precipitation differences (R2 = 0.1618, p = 0.015). The observed significant Mantel tests indicate isolation by adaptation (IBA) (Nosil et al., 2008) for our dataset. However, simultaneous examination of all environmental and geographic distance matrices on genetic distances revealed non-significance of the model (p = 0.161) from the multiple regression analysis. When only precipitation and geographic distances were included as explanatory variables, the model was marginally significant (p = 0.058). According to this model, none of the individual factors was significant.
Our results show that the isolated island Charlottetown population has lowest allelic and gene diversity of all populations and the lowest effective population size. The Charlottetown red oak population is completely isolated from the mainland of New Brunswick and peninsular Nova Scotia restricting gene exchange severely (see below). Besides severely restricted gene flow, genetic drift and founder events may have also contributed to low genetic diversity in this population. The second lowest allelic diversity was observed for the Crafts Cove population, which is consistent with its location in a small forest patch on a dairy farm with one side almost on the edge of Washademoak Lake front and a road going through this small population. Thus, lower genetic diversity in the Crafts Cove population may be due to fragmentation, restricted gene flow, bottleneck effects, and genetic drift. Lower genetic diversity could be expected in peninsular populations due to potential founder effects and reduced gene flow from the mainland. However, comparatively high genetic diversity was observed in peninsular populations from Nova Scotia and the range-edge Brockway Mountain population from the Keweenaw peninsula of Northern Michigan. These populations are located in regions with continuous forest cover suggesting that marginal peninsular conditions do not necessarily result in reduced genetic diversity if such populations are well-connected with other populations of the species. Because we did not have comparable real central populations in this study, we could not compare genetic diversity patterns between central vs. marginal populations of northern red oak. Borkowski et al. (2017) reported genetic diversity of non-range-edge populations with allelic richness of 10.13–12.14 (mean = 11.00 for populations of latitude < 44), and one isolated outlier island population (Isle Royale) with allelic richness as low as 5.50. We observed very similar allelic richness in the isolated PEI population Charlottetown (5.51), but slightly lower values in mainland populations as compared to values reported in Borkowski et al. (2017), ranging between 6.61 and 9.01 (mean = 8.11), which could be a result of their closer proximity to the species’ range-edge. Lower genetic diversity in range-edge populations when compared with central populations has previously been reported in long-lived trees (e.g., Pandey and Rajora, 2012a).
We found comparatively high genetic differentiation among northern red oak populations in our study. FST values in the investigated populations were lower, when the isolated outlier population from Charlottetown was excluded. A previous study reported lower FST values for more central northern red oak populations (Borkowski et al., 2017). This is consistent with the central-marginal populations theory (Eckert et al., 2008) and empirical data (e.g., Pandey and Rajora, 2012a; Chhatre and Rajora, 2014). Our results from the STRUCTURE, PCoA and FST analyses clearly demonstrate that the isolated Charlottetown population from PEI is most genetically differentiated from the other eight red oak populations studied. This population showed the highest population-specific FST (0.150) as well as pairwise FST (≥0.046) estimates. Contrarily, the mean population-specific FST value of the other populations was 0.028, and pairwise FST estimates ranged from 0.004 to 0.036. The pronounced genetic differentiation of the Charlottetown red oak population is most likely due to its geographic isolation. About 10,000 years ago, PEI was linked to the mainland via lowland, but became an island due to the rising ocean level from deglaciation approximately 5,000 years ago. Therefore, the Charlottetown red oak population is not only marginal, but also geographically isolated from external gene flow. Island formation can lead to complex genetic differentiation patterns (Gómez et al., 2003), and differentiation from mainland populations (Dias et al., 2016). Our results are consistent with Borkowski et al. (2017), who also observed high population-specific FST (0.058–0.197, mean = 0.105) values for three isolated Q. rubra populations on Isle Royale in Lake Superior at the northern range in a range-wide study based on the same methods and an overlapping set of markers. Red oak populations from previously ice sheet covered regions in the North exhibited much higher population-specific FST values (0.014–0.197; mean = 0.097, n = 15), when compared to more southern populations (0.010–0.040; mean = 0.023, n = 8) (Borkowski et al., 2017). In our present study, population-specific FST values were lower (0.012–0.150, mean = 0.041) than values observed in Borkowski et al. (2017) for northern populations. Populations-specific FST values of northern red oak populations after excluding the isolated island populations (PEI and Isle Royale) were only slightly lower in the present study as compared to Borkowski et al. (2017) (0.012–0.061, mean = 0.028 vs. 0.011–0.071, mean = 0.031) and the estimates were similar to population-specific FST values reported earlier for southern populations (0.010–0.040; mean = 0.023) (Borkowski et al., 2017). Thus, high population-specific FST values in our study and in Borkowski et al. (2017) are most likely due to genetic differentiation of geographically isolated red oak populations from the remaining populations as a result of limited gene exchange, genetic drift, and or founder effects.
Although all red oak populations we studied showed genetic admixture to a certain degree, our results suggest generally four genetic groups among these nine red oak populations. Each of the Charlottetown, Craft Cove and McConnell Lake Road populations appear to belong to their own genetic cluster and four populations from Nova Scotia apparently belong to the same genetic group along with the Brockway Mountain population. This grouping was consistent between the Structure and PCoA analyses (Figure 2 and Supplementary Figures 3, 4). Since the Nova Scotia red oak populations are located in the same region less than 60 km apart on the peninsula, high genetic similarities among these populations are expected because of potentially high inter-population gene flow and their origin from a common ancestral gene pool. Indeed, we observed FST values lower than average (FSTMean = 0.0295) among the Nova Scotia red oak populations (ranging from 0.0045 to 0.0197). Nova Scotia is a Peninsula connected to the mainland only by the Chignecto isthmus, which is barely forested. Therefore, restricted gene flow from the mainland red oak populations is quite likely, which may facilitate genetic similarities among the peninsular populations from the same region. Although the Crafts Cove population was from the mainland, it was the second most differentiated red oak population (Table 2, Figure 2, and Supplementary Figures 3, 4). This is likely due to restricted gene flow to this population because it is a small population located in a fragmented landscape, where it is surrounded by cattle farms on three sides and a lake and a road on one side. Contrary to our expectation, the McConnell Lake Road population was found to be genetically differentiated from the Phelps population (Figure 2, Supplementary Figures 3, 4, and Supplementary Table 3), both populations from Ontario and located about 50 km apart. Interestingly, chloroplast haplotype composition in the two populations from Ontario also displayed strong differences (Supplementary Figure 7). The McConnell Lake Road red oak population is located very close (about 10 km) to the extreme northern edge of the species natural range, whereas, the Phelps population is about 50–60 km south of the extreme northern edge. Thus, restricted gene flow and genetic drift may have caused this genetic differentiation.
The bottleneck analysis did not result in statistical support of a recent genetic bottleneck in any of the populations. However, the low allelic richness and the absence of private alleles within the island population Charlottetown represent strong indications for a past prolonged bottleneck on Prince Edwards Island. Although, effective population size estimates mostly revealed wide confidence intervals, the Charlottetown population exhibited a very low contemporary effective population size (Ne = 22; 95% CI = 19–26), which supports our assumption of a prolonged bottleneck. Since the beginning of European settlement on the island in the early 1700s, large parts of the forest have been cleared to create space for buildings, agriculture, and roads. This reduction in population size has most likely created a severe bottleneck effect in the northern red oak populations on the island.
The significant heterozygote deficiency in the majority of mainland populations is usually interpreted as an indication of inbreeding. Accordingly, we observed significant positive FIS values in six populations from the mainland. The majority of markers exhibited positive values in most of the populations, reaching up to 15 out of 17 markers. Also, fine-scale SGS as observed in the current study and previous investigations of range-edge northern red oak populations (Lind-Riehl and Gailing, 2015) can promote inbreeding due to the geographic proximity of related mature trees. Interestingly, the Charlottetown population showed a significant negative FIS value with only two loci exhibiting positive FIS values. Small effective population size can contribute to heterozygote excess in predominantly outcrossing species (Balloux, 2004). Thus, potentially balancing selection might be an additional factor for the observed negative FIS value in the Charlottetown population (Ziehe and Roberds, 1989). Similar observations were previously reported for American chestnut [Castanea dentata (Marshall) Borkh.] (Gailing and Nelson, 2017). Furthermore, it has been suggested, that leading range-edge populations restore genetic diversity quickly due to long-distance pollen flow and inbreeding depression (Hampe et al., 2013). Hence, the negative FIS value might be a sign of recovery from a past bottleneck effect.
Isolation by distance (IBD) is expected as a result of geographically limited gene dispersal (Wright, 1943). Borkowski et al. (2017) reported significant range-wide IBD for northern red oak populations, while they found no significant relation between geographic distance and FST values from a geographically restricted region in Michigan and Wisconsin. Mantel tests and multiple regression analysis detected only a marginally significant correlation between geographic and genetic distances of the different populations in our current study. A likely explanation for the low IBD observed in northern red oak populations is their origin from different source refugia and the convergence of postglacial migration routes after the retreat of the Wisconsin glacier. The changing environment, including formation and modification of several topographic barriers of North America during the Wisconsin glacier decline has probably opened multiple possible migration routes of northern red oak remnants from different refugia (Schlarbaum et al., 1982). Multiple factors like genetic drift, selection, and gene flow in this area with diverse environmental conditions, might have affected intraspecific genetic variation of northern red oak populations in the Great Lakes region (Schlarbaum et al., 1982). Thus, convergence of postglacial remigration routes may have resulted in contemporary patches of genetically differentiated northern red oak populations at small geographic distances, concealing a correlation between genetic and geographic distance matrices. Genetic differentiation between populations from various locations without the indication of a gradient might not be influenced by the geographical distance alone, but also by topographic and environmental characteristics. IBD can lead to an overestimation of genetic groups from STRUCTURE (e.g., Perez et al., 2018). However, we detected only weak signals of IBD, and the results of the Bayesian structure analysis are supported by the PCoA and pairwise FST values.
Our study suggests highly pronounced spatial genetic structure in nearly all populations. Each population exhibited significant kinship coefficients within the first distance class, ranging from 0.0067 to 0.0254. The highest values were observed for the populations Brockway Mountain and Phelps, both of which exhibited single samples of a clearly distinct sub-cluster for K = 5 and 6. This internal structuring might partially explain the pronounced SGS. Sp statistics (SpMean = 0.0155) were about three times as high, when compared to Lind-Riehl and Gailing (2015) (Spredoak = 0.005), which ranged between 0.002 and 0.009 in northern red oak populations from northern Michigan and northern Wisconsin. Vekemans and Hardy (2004) reported Sp statistics and kinship coefficients of re-analysed published data for different species, including sessile oak (Quercus petraea) and pedunculate oak (Q. robur), which are very similar to Q. rubra in terms of mating system, gene flow, and seed dispersal (Streiff et al., 1998). Those species were also investigated using microsatellite markers and exhibited comparatively low kinship coefficients, within the first distance class, of 0.031 (Q. petraea), and 0.011 (Q. robur) and Sp values of 0.0083 (Q. petraea), and 0.003 (Q. robur) (Vekemans and Hardy, 2004). Similar to our observations, unexpected high Sp values at the northern range-edge were recently observed in Q. robur populations (Vakkari et al., 2020). These populations from Finland, at the margin of pedunculate oaks’ native range, exhibited high Sp values in a comparable range as reported here, namely between 0.0193 and 0.0283. The sampled populations in Vakkari et al. (2020) mostly consisted of scarce adult trees with population sizes between 60 and 300 reproductive trees (Vakkari et al., 2020) suggesting an effect of population size and the number of reproducing trees on SGS formation. Lind-Riehl and Gailing (2015) observed Sp values between 0.005 and 0.017 with a mean of 0.0105 for four northern pin oak (Q. ellipsoidalis) populations at the uttermost edge of the species’ natural distribution range. Likewise, pronounced SGS was observed in other tree species (Gapare and Aitken, 2005; Pandey and Rajora, 2012b) in isolated leading edge populations. SGS seems to increase toward more northern populations, which may be related to the harsher climatic conditions of range-edge populations, their population size and density of reproducing trees. Concordantly, a negative correlation between effective population size and Sp values was observed in the present study. To analyse the effect of geographic isolation and environmental conditions in range-edge populations on SGS comprehensively, more reference samples from populations of the actual centre of the native range and the northern edge of the native distribution area and data on population history would be desirable. Especially, management (Lind-Riehl and Gailing, 2015), and disturbance history (Gonzales et al., 2010; Budde et al., 2017) also have an effect on SGS and therefore may influence the results.
Differences in the abiotic factors temperature and precipitation were not correlated with population-specific FST values (Supplementary Figure 8). However, Mantel tests revealed a significant correlation between matrices of genetic distance and precipitation differences (R2 = 0.1618, p = 0.015). Higher genetic differences related to higher precipitation differences, may indicate an impact of climatic conditions on the genetic composition of the studied range-edge populations. Our dataset represents various populations from the northern edge of the species’ native range and thus the edge of their biological niche. In such a borderline environment, high selection can be expected, shaping the community by limiting the number of reproductive individuals within different populations which may lead to IBA or stronger fine-scale SGS (see above). However, since precipitation differences are highly significantly correlated with geographic distances (R2 = 0.63, p < 0.001), the correlation between genetic and precipitation differences could also be the result of neutral processes. Consequently, a multiple regression analysis model including precipitation and geographic distances revealed only a marginally significant effect on genetic distances (p = 0.058). However, geographic distances were strongly correlated with differences in environmental parameters (Table 4), and precipitation differences with differences in the remaining environmental parameters (Table 4). The method, as currently implemented in the software is limited in the interpretation of strongly related variables (Wang, 2013). Hence, the results might be biased and the actual role of IBA remains unclear. Further investigations of a larger number of range-edge and central populations along steep environmental gradients with an increased marker set, especially SNPs from expressed genes would be desirable to detect loci under selection and significant IBA. Such markers could provide valuable insights in the role of single alleles in evolutionary adaptation and contribute to the selection of highly adapted genotypes for marginal climate conditions.
Both, precipitation and temperature showed a significant and negative linear correlation with Sp values with correlation coefficients of -0.796 (p = 0.0103) and -0.730 (p = 0.0255), respectively. Likewise, a significant correlation between differences in precipitation/temperature and Sp values (p = 0.020) was found. Thus, our data suggest a relation between major climate parameters, especially precipitation and SGS formation. Nevertheless, there might be other related factors impacting the SGS formation, and our observation does not necessarily provide evidence for causation. The most likely cause for more pronounced SGS in colder and drier regions is a lower number of reproducing trees in marginal populations during population regeneration. Low numbers of reproducing trees within a population lead to less overlapping seed shadows, and thus, more pronounced SGS. There is evidence for significant effects of the population density on SGS (Vekemans and Hardy, 2004). Indeed, Sp values were significantly negatively (p = 0.020) correlated with Ne, supporting the assumption that the number and density of reproducing trees have significant effects on SGS. Also, wildfire events have been shown to promote the formation of SGS in Pinus halepensis Mill. (Budde et al., 2017). In fact, some of the investigated populations were impacted by fire events. However, the post-fire originated Nova Scotia populations exhibited comparatively low levels of SGS. Hence, our results did not indicate a major role of fire events in SGS formation in our sample set. Temperature and precipitation were correlated with fine-scale SGS in alpine conifer species, and can impact the microevolution in populations of particular species (Mosca et al., 2018). Similar to our results in northern red oak, a significant negative effect of spring precipitation on fine-scale SGS and a higher impact of precipitation as compared to temperature was reported for most of the tested conifer species (Mosca et al., 2018). Our observations in northern red oak therefore provide further evidence for a possible direct or indirect effect of harsh climatic conditions on SGS formation as suggested in Mosca et al. (2018). Specifically, Mosca et al. (2018) suggested that environmental conditions have a selective impact on the reproducing trees and the frequency of mast years, as well as the maturing offspring. Accordingly, an unbalanced representation of genotypes in the natural regeneration could directly impact SGS (Mosca et al., 2018). While, none of the discussed studies provided direct evidence for actual selection impacting SGS, it may have contributed to the repeatedly observed high SGS in range-edge populations (Gapare and Aitken, 2005; Pandey and Rajora, 2012b; Vakkari et al., 2020).
We found high levels of genetic differentiation between the island population Charlottetown and the remaining mainland and peninsular populations. This particular population has most likely undergone a genetic bottleneck due to human impact and geographic isolation. Comparatively low genetic differentiation was observed between several mainland and peninsular populations from different locations of the northern range-edge. Using a Bayesian cluster analysis, we were able to detect subtle genetic differences between different northern red oak populations from the northern edge of the native range. Moreover, we observed remarkably high SGS and an indication of inbreeding in the sampled populations, which was most likely linked to the typical characteristics of the species’ range-edge populations (low population density, lower number of reproducing trees). SGS formation was significantly linked to major climate parameters, such as temperature and precipitation. Our results highlight the importance of investigations of marginal tree populations in the face of climate change and for sustainable forest management. To provide a more exhaustive data set and to verify our findings, further examinations with additional populations from the leading range-edge, and more central parts of the native range would be desirable. Also, large marker sets, especially a large number of SNPs in expressed genes, to screen for outlier loci and signs of selection are needed to disentangle adaptive from neutral processes.
The raw data supporting the conclusions of this article are provided in a Supplementary Material and will be made available by the authors, without undue reservation.
OG and OR conceived, designed, and directed the study. OR selected and sampled the Canadian red oak populations and contributed to the data analysis, interpreting results, and writing, reviewing, and revising the manuscript. JG performed the lab work and data analysis, prepared the first draft of the manuscript, and contributed to the revision and results interpretation. OG contributed to the interpretation of the results and writing, reviewing, and editing the manuscript. All authors contributed to the article and approved the submitted version.
We acknowledge support by the Open Access Publication Funds of the Georg-August University of Göttingen. This research was funded by the German Federal Ministry of Food and Agriculture, grant number 22023314 to OG and partly from the Natural Sciences and Engineering Research Council of Canada Discovery Grant (RGPIN 2017-04589) to OR. OR received the research stay for University Academics and Scientists Scholarship (57552334) from German Exchange Service (DAAD).
The authors declare that the research was conducted in the absence of any commercial or financial relationships that could be construed as a potential conflict of interest.
All claims expressed in this article are solely those of the authors and do not necessarily represent those of their affiliated organizations, or those of the publisher, the editors and the reviewers. Any product that may be evaluated in this article, or claim that may be made by its manufacturer, is not guaranteed or endorsed by the publisher.
We thank Alexandra Dolynska for technical support and Mary Jane Rodger, General Manager and Jennica Hunsinger, Research Intern of Medway Community Forest Co-op, Andree Morneault of Nipissing Forest Resource Management Inc., Steve Chenier, Gary Schneider of MacPhail Woods, and Chandler Colpit of Mcrea Farms for their help in locating and field sampling of northern red oak populations in Canada. Finally, we also thank reviewers for providing valuable suggestions for improvement.
The Supplementary Material for this article can be found online at: https://www.frontiersin.org/articles/10.3389/fevo.2022.907414/full#supplementary-material
Ahrens, J., Geveci, B., and Law, C. (2005). ParaView: an end-user tool for large data visualization paraviewweb view project. Vis. Handb. 836, 717–742.
Aitken, S. N., Yeaman, S., Holliday, J. A., Wang, T., and Curtis-McLane, S. (2008). Adaptation, migration or extirpation: climate change outcomes for tree populations. Evolut. Appl. 1, 95–111. doi: 10.1111/j.1752-4571.2007.00013.x
Aldrich, P. R., Parker, G. R., Michler, C. H., and Romero-Severson, J. (2003). Whole-tree silvic identifications and the microsatellite genetic structure of a red oak species complex in an Indiana old-growth forest. Can. J. For. Res. 33, 2228–2237. doi: 10.1139/x03-160
Arnaud-Haond, S., Teixeira, S., Massa, S. I., Billot, C., Saenger, P., Coupland, G., et al. (2006). Genetic structure at range edge: low diversity and high inbreeding in Southeast Asian mangrove (Avicennia marina) populations. Mol. Ecol. 15, 3515–3525. doi: 10.1111/j.1365-294X.2006.02997.x
Balloux, F. (2004). Heterozygote excess in small populations and the heterozygote-excess effective population size. Evolution 58, 1891–1900. doi: 10.1111/j.0014-3820.2004.tb00477.x
Borkowski, D. S., Hoban, S. M., Chatwin, W., and Romero-Severson, J. (2017). Rangewide population differentiation and population substructure in Quercus rubra L. Tree Genet. Genomes 13:67. doi: 10.1007/s11295-017-1148-6
Budde, K. B., González-Martínez, S. C., Navascués, M., Burgarella, C., Mosca, E., Lorenzo, Z., et al. (2017). Increased fire frequency promotes stronger spatial genetic structure and natural selection at regional and local scales in Pinus halepensis Mill. Ann. Bot. 119, 1061–1072. doi: 10.1093/aob/mcw286
Cavers, S., Degen, B., Caron, H., Lemes, M. R., Margis, R., Salgueiro, F., et al. (2005). Optimal sampling strategy for estimation of spatial genetic structure in tree populations. Heredity 95, 281–289. doi: 10.1038/sj.hdy.6800709
Chhatre, V. E., and Emerson, K. J. (2017). StrAuto: automation and parallelization of STRUCTURE analysis. BMC Bioinformat. 18:197. doi: 10.1186/s12859-017-1593-0
Chhatre, V. E., and Rajora, O. P. (2014). Genetic divergence and signatures of natural selection in marginal populations of a keystone, long-lived conifer, eastern white pine (Pinus strobus) from Northern Ontario. PLoS One 9:e97291. doi: 10.1371/journal.pone.0097291
Cornuet, J. M., and Luikart, G. (1996). Description and power analysis of two tests for detecting recent population bottlenecks from allele frequency data. Genetics 144, 2001–2014. doi: 10.1093/genetics/144.4.2001
De-Lucas, A. I., GonzÁlez-MartÍnez, S. C., Vendramin, G. G., Hidalgo, E., and Heuertz, M. (2009). Spatial genetic structure in continuous and fragmented populations of Pinus pinaster Aiton. Mol. Ecol. 18, 4564–4576. doi: 10.1111/j.1365-294X.2009.04372.x
Depardieu, C., Girardin, M. P., Nadeau, S., Lenz, P., Bousquet, J., and Isabel, N. (2020). Adaptive genetic variation to drought in a widely distributed conifer suggests a potential for increasing forest resilience in a drying climate. New Phytol. 227, 427–439. doi: 10.1111/nph.16551
Dewoody, J., Trewin, H., and Taylor, G. (2015). Genetic and morphological differentiation in Populus nigra L.: isolation by colonization or isolation by adaptation? Mol. Ecol. 24, 2641–2655. doi: 10.1111/mec.13192
Dias, E. F., Moura, M., Schaefer, H., and Silva, L. (2016). Geographical distance and barriers explain population genetic patterns in an endangered island perennial. AoB Plants 8:plw072. doi: 10.1093/aobpla/plw072
Do, C., Waples, R. S., Peel, D., Macbeth, G. M., Tillett, B. J., and Ovenden, J. R. (2014). Ne Estimator v2: re-implementation of software for the estimation of contemporary effective population size (Ne) from genetic data. Mol. Ecol. Resour. 14, 209–214.
Durand, J., Bodénès, C., Chancerel, E., Frigerio, J. M., Vendramin, G., Sebastiani, F., et al. (2010). A fast and cost-effective approach to develop and map EST-SSR markers: oak as a case study. BMC Genomics 11:570. doi: 10.1186/1471-2164-11-570
Earl, D. A., and VonHoldt, B. M. (2012). STRUCTURE HARVESTER: a website and program for visualizing STRUCTURE output and implementing the Evanno method. Conserv. Genet. Resour. 4, 359–361. doi: 10.1007/s12686-011-9548-7
Eckert, C. G., Samis, K. E., and Lougheed, S. C. (2008). Genetic variation across species’ geographical ranges: the central–marginal hypothesis and beyond. Mol. Ecol. 17, 1170–1188. doi: 10.1111/j.1365-294X.2007.03659.x
Epperson, B. K. (2007). Plant dispersal, neighbourhood size and isolation by distance. Mol. Ecol. 16, 3854–3865. doi: 10.1111/j.1365-294X.2007.03434.x
Evanno, G., Regnaut, S., and Goudet, J. (2005). Detecting the number of clusters of individuals using the software STRUCTURE: a simulation study. Mol. Ecol. 14, 2611–2620. doi: 10.1111/j.1365-294X.2005.02553.x
Fick, S. E., and Hijmans, R. J. (2017). WorldClim 2: new 1-km spatial resolution climate surfaces for global land areas. Int. J. Climatol. 37, 4302–4315. doi: 10.1002/joc.5086
Foll, M., and Gaggiotti, O. (2006). Identifying the environmental factors that determine the genetic structure of populations. Genetics 174, 875–891. doi: 10.1534/genetics.106.059451
Funk, D. J., Egan, S. P., and Nosil, P. (2011). Isolation by adaptation in Neochlamisus leaf beetles: host-related selection promotes neutral genomic divergence. Mol. Ecol. 20, 4671–4682. doi: 10.1111/j.1365-294X.2011.05311.x
Gailing, O., and Nelson, C. D. (2017). Genetic variation patterns of american chestnut populations at EST-SSRs. Botany 95, 799–807. doi: 10.1139/cjb-2016-0323
Gapare, W. J., and Aitken, S. N. (2005). Strong spatial genetic structure in peripheral but not core populations of Sitka spruce [Picea sitchensis (Bong.) Carr.]. Molecular Ecology 14, 2659–2667. doi: 10.1111/j.1365-294X.2005.02633.x
Godfrey, R. K. (1988). Trees, Shrubs, and Woody Vines of Northern Florida and Adjacent Georgia and Alabama. Athens, GA: University of Georgia Press, 341.
Gibson, S. Y., Van Der Marel, R. C., and Starzomski, B. M. (2009). Climate change and conservation of leading-edge peripheral populations. Conser. Biol. 23, 1369–1373. doi: 10.1111/j.1523-1739.2009.01375.x
Gómez, A., González-Martínez, S. C., Collada, C., Climent, J., and Gil, L. (2003). Complex population genetic structure in the endemic Canary Island pine revealed using chloroplast microsatellite markers. Theor. Appl. Genet. 107, 1123–1131. doi: 10.1007/s00122-003-1320-2
Gonzales, E., Hamrick, J. L., Smouse, P. E., Trapnell, D. W., and Peakall, R. (2010). The impact of landscape disturbance on spatial genetic structure in the guanacaste tree, enterolobium cyclocarpum (fabaceae). J. Heredity 101, 133–143. doi: 10.1093/jhered/esp101
Goudet, J. (2003). Fstat (ver. 2.9. 4), a Program to Estimate and Test Population Genetics Parameters. Lausanne: Department of Ecology & Evolution, Biology Building, UNIL.
Götz, J., Krutovsky, K. V., Leinemann, L., Müller, M., Rajora, O. P., and Gailing, O. (2020). Chloroplast haplotypes of northern red oak (Quercus rubra L.) stands in Germany suggest their origin from Northeastern Canada. Forests 11, 1–15. doi: 10.3390/f11091025
Hampe, A., Pemonge, M. H., and Petit, R. J. (2013). Efficient mitigation of founder effects during the establishment of a leading-edge oak population. Proc. R. Soc. B 280:20131070. doi: 10.1098/rspb.2013.1070
Hampe, A., and Petit, R. J. (2005). Conserving biodiversity under climate change: the rear edge matters. Ecol. Lett. 8, 461–467. doi: 10.1111/j.1461-0248.2005.00739.x
Hamrick, J. L., Linhart, Y. B., and Mitton, J. B. (1979). Relationships between life history characteristics and electrophoretically detectable genetic variation in plants. Annu. Rev. Ecol. Syst. 10, 173–200.
Hardy, O. J., and Vekemans, X. (2002). spagedi: a versatile computer program to analyse spatial genetic structure at the individual or population levels. Mol. Ecol. Notes 2, 618–620. doi: 10.1046/j.1471-8278
Hubisz, M. J., Falush, D., Stephens, M., and Pritchard, J. K. (2009). Inferring weak population structure with the assistance of sample group information. Mol. Ecol. Resour. 9, 1322–1332. doi: 10.1111/j.1755-0998.2009.02591.x
Inkscape Project (2020). Inkscape. Available online at: https://inkscape.org
Jones, F. A., Hamrick, J. L., Peterson, C. J., and Squiers, E. R. (2006). Inferring colonization history from analyses of spatial genetic structure within populations of Pinus strobus and Quercus rubra. Mol. Ecol. 15, 851–861. doi: 10.1111/j.1365-294X.2005.02830.x
Keenan, K., Mcginnity, P., Cross, T. F., Crozier, W. W., and Prodöhl, P. A. (2013). DiveRsity: an R package for the estimation and exploration of population genetics parameters and their associated errors. Methods Ecol. Evol. 4, 782–788. doi: 10.1111/2041-210X.12067
Khodwekar, S., and Gailing, O. (2017). Evidence for environment-dependent introgression of adaptive genes between two red oak species with different drought adaptations. Am. J. Bot. 104, 1088–1098. doi: 10.3732/ajb.1700060
Kimura, M., and Weiss, G. H. (1964). The stepping stone model of population structure and the decrease of genetic correlation with distance. Genetics 49, 561–576. doi: 10.1093/oxfordjournals.molbev.a025590
Lind, J. F., and Gailing, O. (2013). Genetic structure of Quercus rubra L. and Quercus ellipsoidalis E. J. Hill populations at gene-based EST-SSR and nuclear SSR markers. Tree Genet. Genomes 9, 707–722. doi: 10.1007/s11295-012-0586-4
Lind-Riehl, J., and Gailing, O. (2015). Fine-scale spatial genetic structure of two red oak species, Quercus rubra and Quercus ellipsoidalis. Plant Syst. Evol. 301, 1601–1612. doi: 10.1007/s00606-014-1173-y
Lind-Riehl, J. F., Sullivan, A. R., and Gailing, O. (2014). Evidence for selection on a CONSTANS-like gene between two red oak species. Ann. Bot. 113, 967–975. doi: 10.1093/aob/mcu019
Loiselle, B. A., Sork, V. L., Nason, J., and Graham, C. (1995). Spatial genetic structure of a tropical understory shrub, Psychotria officinalis (Rubiaceae). Am. J. Bot. 82, 1420–1425.
Merceron, N. R., Leroy, T., Chancerel, E., Romero-Severson, J., Borkowski, D. S., Ducousso, A., et al. (2017). Back to America: tracking the origin of European introduced populations of Quercus rubra L. Genome 60, 778–790. doi: 10.1139/gen-2016-0187
Mosca, E., Di Pierro, E. A., Budde, K. B., Neale, D. B., and González-Martínez, S. C. (2018). Environmental effects on fine-scale spatial genetic structure in four Alpine keystone forest tree species. Mol. Ecol. 27, 647–658. doi: 10.1111/mec.14469
Nosil, P., Egan, S. P., and Funk, D. J. (2008). Heterogeneous genomic differentiation between walking-stick ecotypes: “Isolation by adaptation” and multiple roles for divergent selection. Evolution 62, 316–336. doi: 10.1111/j.1558-5646.2007.00299.x
Pandey, M., and Rajora, O. P. (2012a). Genetic diversity and differentiation of core versus peripheral populations of eastern white cedar, Thuja occidentalis L. (Cupressaceae). Am. J. Bot. 99, 690–699. doi: 10.3732/ajb.1100116
Pandey, M., and Rajora, O. P. (2012b). Higher fine-scale genetic structure in peripheral than in core populations of a long-lived and mixed-mating conifer - Eastern white cedar (Thuja occidentalis L.). BMC Evol. Biol. 12:48. doi: 10.1186/1471-2148-12-48
Peakall, R., and Smouse, P. E. (2006). GENALEX 6: genetic analysis in Excel. Population genetic software for teaching and research. Mol. Ecol. Notes 6, 288–295. doi: 10.1111/j.1471-8286.2005.01155.x
Peakall, R. O. D., and Beattie, A. J. (1995). Does ant dispersal of seeds in Sclerolaena diacantha (Chenopodiaceae) generate local spatial genetic structure? Heredity 75, 351–361.
Perez, M. F., Franco, F. F., Bombonato, J. R., Bonatelli, I. A., Khan, G., Romeiro-Brito, M., et al. (2018). Assessing population structure in the face of isolation by distance: Are we neglecting the problem? Divers. Distrib. 24, 1883–1889. doi: 10.1111/ddi.12816
Pohjanmies, T., Elshibli, S., Pulkkinen, P., Rusanen, M., Vakkari, P., Korpelainen, H., et al. (2016). Fragmentation-related patterns of genetic differentiation in pedunculate oak (Quercus robur) at two hierarchical scales. Silva Fennica 50, 1–15. doi: 10.14214/sf.1510
Pritchard, J. K., Stephens, M., and Donnelly, P. (2000). Inference of population structure using multilocus genotype data. Genetics 155, 945–959.
QGIS Development Team (2009). QGIS Geographic Information System. Beaverton, OR: Open Source Geospatial Foundation.
R core team (2018). An introduction to dplR. Indust. Commer. Train. 10, 11–18. doi: 10.1108/eb003648
Rajora, O. P., Rahman, M. H., Buchert, G. P., and Dancik, B. P. (2000). Microsatellite DNA analysis of genetic effects of harvesting in old-growth eastern white pine (Pinus strobus) in Ontario, Canada. Mol. Ecol. 9, 339–348. doi: 10.1046/j.1365-294X.2000.00886.x
Rajora, O. P., and Zinck, J. W. R. (2021). Genetic diversity, structure and effective population size of old-growth vs. second-growth populations of keystone and long-lived conifer, eastern white pine (Pinus strobus): conservation value and climate adaptation potential. Front. Genet. 12:650299. doi: 10.3389/fgene.2021.650299
RamÍrez-Valiente, J. A., Lorenzo, Z., Soto, A., Valladares, F., Gil, L., and Aranda, I. (2009). Elucidating the role of genetic drift and natural selection in cork oak differentiation regarding drought tolerance. Mol. Ecol. 18, 3803–3815. doi: 10.1111/j.1365-294X.2009.04317.x
Rehm, E. M., Olivas, P., Stroud, J., and Feeley, K. J. (2015). Losing your edge: climate change and the conservation value of range-edge populations. Ecol. Evol. 5, 4315–4326. doi: 10.1002/ece3.1645
Rousset, F. (2008). Genepop’007: a complete re-implementation of the genepop software for Windows and Linux. Mol. Ecol. Resour. 8, 103–106. doi: 10.1111/j.1471-8286.2007.01931.x
Sagnard, F., Oddou-Muratorio, S., Pichot, C., Vendramin, G. G., and Fady, B. (2011). Effects of seed dispersal, adult tree and seedling density on the spatial genetic structure of regeneration at fine temporal and spatial scales. Tree Genet. Genomes 7, 37–48. doi: 10.1007/s11295-010-0313-y
Schlarbaum, S. E., Adams, R. P., Bagley, W. T., and Wayne, W. J. (1982). Postglacial migration pathways of Quercus rubra L., northern red oak, as indicated by regional genetic variation patterns. Silvae Genet. 31, 150–158.
Sexton, J. P., Hangartner, S. B., and Hoffmann, A. A. (2014). Genetic isolation by environment or distance: Which patterns of gene flow is most common? Evolution 68, 1–15. doi: 10.1111/evo.12258
Smouse, P. E., and Peakall, R. (1999). Spatial autocorrelation analysis of individual multiallele and multilocus genetic structure. Heredity 82, 561–573. doi: 10.1038/sj.hdy.6885180
Streiff, R., Labbe, T., Bacilieri, R., Steinkellner, H., Glössl, J., and Kremer, A. (1998). Within-population genetic structure in Quercus robur L. and Quercus petraea (Matt.) Liebl. assessed with isozymes and microsatellites. Mol. Ecol. 7, 317–328. doi: 10.1046/j.1365-294x.1998.00360.x
Sullivan, A. R., Lind, J. F., McCleary, T. S., Romero-Severson, J., and Gailing, O. (2013). Development and characterization of genomic and gene-based microsatellite markers in North American red oak species. Plant Mol. Biol. Rep. 31, 231–239. doi: 10.1007/s11105-012-0495-6
Vakkari, P., Rusanen, M., Heikkinen, J., Huotari, T., and Kärkkäinen, K. (2020). Patterns of genetic variation in leading-edge populations of Quercus robur: genetic patchiness due to family clusters. Tree Genet. Genomes 16:73. doi: 10.1007/s11295-020-01465-9
Vekemans, X., and Hardy, O. J. (2004). New insights from fine-scale spatial genetic structure analyses in plant populations. Mol. Ecol. 13, 921–935. doi: 10.1046/j.1365-294X.2004.02076.x
Vornam, B., Decarli, N., and Gailing, O. (2004). Spatial distribution of genetic variation in a natural beech stand (Fagus sylvatica L.) based on microsatellite markers. Conserv. Genet. 5, 561–570. doi: 10.1023/B:COGE.0000041025.82917.ac
Wang, I. J. (2013). Examining the full effects of landscape heterogeneity on spatial genetic variation: a multiple matrix regression approach for quantifying geographic and ecological isolation. Evolution 67, 3403–3411. doi: 10.1111/evo.12134
Wang, I. J., and Bradburd, G. S. (2014). Isolation by environment. Mol. Ecol. 23, 5649–5662. doi: 10.1111/mec.12938
Waples, R. S. (2006). A bias correction for estimates of effective population size based on linkage disequilibrium at unlinked gene loci. Conserv. Genet. 7, 167–184.
Waples, R. S., and Do, C. H. I. (2010). Linkage disequilibrium estimates of contemporary Ne using highly variable genetic markers: a largely untapped resource for applied conservation and evolution. Evol. Appl. 3, 244–262.
Weir, B. S., and Cockerham, C. C. (1984). Estimating F-Statistics for the Analysis of Population Structure. Evolution 38, 1358–1370.
Willi, Y., Van Buskirk, J., Schmid, B., and Fischer, M. (2007). Genetic isolation of fragmented populations is exacerbated by drift and selection. J. Evol. Biol. 20, 534–542. doi: 10.1111/j.1420-9101.2006.01263.x
Keywords: spatial genetic structure (SGS), northern red oak, microsatellites, island population, range-edge populations, genetic differentiation, genetic diversity, isolation by adaptation
Citation: Götz J, Rajora OP and Gailing O (2022) Genetic Structure of Natural Northern Range-Margin Mainland, Peninsular, and Island Populations of Northern Red Oak (Quercus rubra L.). Front. Ecol. Evol. 10:907414. doi: 10.3389/fevo.2022.907414
Received: 29 March 2022; Accepted: 09 May 2022;
Published: 13 June 2022.
Edited by:
Alison G. Nazareno, Federal University of Minas Gerais, BrazilReviewed by:
Suzana Alcantara, Federal University of Santa Catarina, BrazilCopyright © 2022 Götz, Rajora and Gailing. This is an open-access article distributed under the terms of the Creative Commons Attribution License (CC BY). The use, distribution or reproduction in other forums is permitted, provided the original author(s) and the copyright owner(s) are credited and that the original publication in this journal is cited, in accordance with accepted academic practice. No use, distribution or reproduction is permitted which does not comply with these terms.
*Correspondence: Oliver Gailing, b2dhaWxpbkBnd2RnLmRl; Om P. Rajora, b20ucmFqb3JhQHVuYi5jYQ==
Disclaimer: All claims expressed in this article are solely those of the authors and do not necessarily represent those of their affiliated organizations, or those of the publisher, the editors and the reviewers. Any product that may be evaluated in this article or claim that may be made by its manufacturer is not guaranteed or endorsed by the publisher.
Research integrity at Frontiers
Learn more about the work of our research integrity team to safeguard the quality of each article we publish.