- 1Plant Ecology and Nature Conservation, Institute of Biochemistry and Biology, University of Potsdam, Potsdam, Germany
- 2Biodiversity Research Centre, Agriculture and Natural Resources Sciences, Namibia University of Science and Technology, Windhoek, Namibia
- 3School of Veterinary Medicine, University of Namibia, Windhoek, Namibia
- 4Geological Remote Sensing, Institute of Geosciences, University of Potsdam, Potsdam, Germany
- 5Department of Ecological Dynamics, Leibniz-Institute for Zoo and Wildlife Research, Berlin, Germany
In semi-arid environments characterized by erratic rainfall and scattered primary production, migratory movements are a key survival strategy of large herbivores to track resources over vast areas. Veterinary Cordon Fences (VCFs), intended to reduce wildlife-livestock disease transmission, fragment large parts of southern Africa and have limited the movements of large wild mammals for over 60 years. Consequently, wildlife-fence interactions are frequent and often result in perforations of the fence, mainly caused by elephants. Yet, we lack knowledge about at which times fences act as barriers, how fences directly alter the energy expenditure of native herbivores, and what the consequences of impermeability are. We studied 2-year ungulate movements in three common antelopes (springbok, kudu, eland) across a perforated part of Namibia's VCF separating a wildlife reserve and Etosha National Park using GPS telemetry, accelerometer measurements, and satellite imagery. We identified 2905 fence interaction events which we used to evaluate critical times of encounters and direct fence effects on energy expenditure. Using vegetation type-specific greenness dynamics, we quantified what animals gained in terms of high quality food resources from crossing the VCF. Our results show that the perforation of the VCF sustains herbivore-vegetation interactions in the savanna with its scattered resources. Fence permeability led to peaks in crossing numbers during the first flush of woody plants before the rain started. Kudu and eland often showed increased energy expenditure when crossing the fence. Energy expenditure was lowered during the frequent interactions of ungulates standing at the fence. We found no alteration of energy expenditure when springbok immediately found and crossed fence breaches. Our results indicate that constantly open gaps did not affect energy expenditure, while gaps with obstacles increased motion. Closing gaps may have confused ungulates and modified their intended movements. While browsing, sedentary kudu's use of space was less affected by the VCF; migratory, mixed-feeding springbok, and eland benefited from gaps by gaining forage quality and quantity after crossing. This highlights the importance of access to vast areas to allow ungulates to track vital vegetation patches.
Introduction
Fences are the most prominent anthropogenic structure traversing the globe's landscapes (Jakes et al., 2018; McInturff et al., 2020). They fragment habitats, shape environments (e.g., Pirie et al., 2017), cause injuries and mortality to wildlife (e.g., Harrington and Conover, 2006; Mbaiwa and Mbaiwa, 2006; Aquino and Nkomo, 2021; McKay et al., 2021) and impede animal movements across various temporal and spatial scales. Short-term foraging (Vanak et al., 2010) and seasonal migratory movements (Sawyer et al., 2013; Nandintsetseg et al., 2019; Seigle-Ferrand et al., 2021) are constrained, redirected to less suitable habitats, or even eliminated by fences. This holds especially true for wild ungulates, as many species track resources across vast areas by following landscape-wide wave-like greening patterns (e.g., Bischof et al., 2012). Through this they increase forage quality by tracking plant maturation stages of high nutritional value (Fryxell, 1991; Esmaeili et al., 2021) or the duration of the experienced green season (Aikens et al., 2020). At the same time, when larger herbivores can reach and leave places at the right times (Holdo et al., 2007) and in critical densities, grazing stimulates the growth of many grassland plant species. This can increase and maintain both the productivity of grasslands (Hempson et al., 2015b; Geremia et al., 2019) and landscape-wide plant diversity (van Klink et al., 2015; Bakker et al., 2016). Notably, in order to survive it is crucial for native ungulates to be capable of balancing movement- and reproduction-related energy expenditure, reduction of predation risk, and energy intake (Bischof et al., 2012). In this sense, fences are an important element of contemporary ecology.
The recent review on fence ecology by McInturff et al. (2020) provides frameworks for better understanding the ecological effects of fences and guidance for future research in this field. In general, the authors point out that the extent of direct and indirect effects of fencing (e.g., changes in grazing pressure and connected changes in plant communities) result from the purpose and the type of fences (e.g., conservation vs. political boundary fences or livestock vs. wildlife fences). In particular, they show that the literature on physiological consequences is especially limited in terms of energy expenditure. The few existing studies on this topic rather focus on counts of certain behaviors such as jumping (e.g., McKillop and Sibly, 1988; Harrington and Conover, 2006; Laskin et al., 2020) than on direct measurements of energetic consequences. The question of what direct energetic consequences arise from fence interactions remains unanswered. Likewise, tracking-based studies that include multiple species and last among multiple seasons concentrate on only some effects of permeable fences.
Resource-tracking ungulates have been studied frequently in temperate regions (e.g., Bischof et al., 2012; Geremia et al., 2019; Aikens et al., 2020; Esmaeili et al., 2021), and an important framework which unites optimal foraging theory and landscape ecology is available (Abrahms et al., 2021). However, very high resolution, vegetation-type specific and fence-oriented analyses are scarce, especially in semi-arid environments with scattered distributed resources. This scarcity is of concern, since arid and semi-arid savannas cover about 26% of the terrestrial surface (Safriel et al., 2005) and their rather patchy distribution of greening; initiated by spatio-temporal erratic rainfall events; likely causes the movement patterns of resource-tracking ungulates to differ from those in temperate regions with wave-like greening (Abrahms et al., 2021).
Effects of fences on resource-tracking ungulates are increasingly studied and this substantially improves the knowledge within the emerging discipline of fence ecology. For instance, studies show that even permeable fences affect ungulate home range formation (e.g., Sawyer et al., 2013; Seigle-Ferrand et al., 2021; Robb et al., 2022) and that ungulate-fence interactions peak during specific times (e.g., green season: Xu et al., 2021, day time: Wilkinson et al., 2021). Still, our ability to predict peak times of animal-fence encounters, and our understanding of energetic gains caused by permeable fence lines during resource-tracking are limited.
Strikingly, a contrast between political actions and intentions becomes apparent in the light of ungulate movements and fencing. While more and more impermeable, political fences are being built (e.g., Linnell et al., 2016; Safner et al., 2021) and increasingly impede wildlife movement and migration, most countries are parties to global conservation conventions such as the Convention on the Conservation of Migratory Species of Wild Animals (CMS). Clearly, in order to achieve the aims of such conventions it is imperative for the parties to thoroughly understand animal-fence interactions to minimize the negative effects of fences by implementing appropriate measures. This is important, since additionally the IUCN World Commission on Protected Areas Connectivity Conservation Specialist Group, which i.a. guides stakeholders to increase ecological connectivity, highlights the importance of the unimpeded movement for the creation of ecological networks for animal conservation (Hilty et al., 2020). In this context, the necessity to improve our understanding of animal-fence interactions is further underlined by a rapidly growing knowledge of large-scale animal movements (Tucker et al., 2018) and discoveries of new ungulate migrations globally (Kauffman et al., 2021b).
An extreme case of ungulate migration barriers are Veterinary Cordon Fences (VCFs), mainly located in Africa, the continent with the highest diversity of native large mammal species (Malhi et al., 2016; Owen-Smith et al., 2020). Set up to prevent wildlife-livestock disease transmission (e.g., foot-and-mouth disease, Caron et al., 2013) VCFs are constructed in a wildlife-proof manner. For over 60 years now (Gadd, 2012) these fences have blocked off the movements of native mammals. Height and wire density of VCFs eliminate the success of crossing strategies such as jumping over or slipping under the fence. Still, wildlife, which are compelled by various needs, attempt to cross such boundaries, which manifests in injuries and death numbers (Mbaiwa and Mbaiwa, 2006). Elephants regularly break these fences (Ferguson et al., 2012; Mogotsi et al., 2016) and, thereby, open these barriers for other species' movement.
Using a natural experimental setup along 70 km of Namibia's VCF regularly damaged by elephants, we investigate fence interactions, associated energetic consequences, and habitat sensing and use related to the fence of three common African ungulate species (Tragelaphus oryx—common eland, Tragelaphus strepsiceros—greater kudu, Antidorcas marsupialis—springbok) in a semi-arid savanna system. We tracked individuals for over 2 years with high resolution GPS localizations (5 to 15 min intervals) and three-dimensional accelerometer data (measured in bursts every 2.5–5 min).
We selected the three antelope species for the following reasons. First, as common species in the study region they are formative drivers within the ecosystem. Second, they are a substantial part of Namibia's wildlife-based land use (Lindsey, 2011), so an improvement of the knowledge on their fence interaction is of high concern. Third, they differ in terms of body mass, animal functional and movement type and hence, in their energetic and habitat requirements. Thus, we are able to compare the effects of the fence with regard to these differences.
Springbok is a medium-sized, non-water reliant mixed feeder (Hempson et al., 2015a) living in smaller groups in dry seasons and aggregating in large groups on fresh green areas during the green season (Skinner and Chimimba, 2005). If unimpeded they may be migratory, and are well-known for historical large treks (Roche, 2008). Springboks primarily crawl under fences and, consequently, are already impeded by jackal-proof cattle fences with closely spaced strands or wire mesh (Bigalke, 1972). The eland is the largest African antelope, classified as a non-water reliant mixed feeder (Hempson et al., 2015a) and the group sizes vary as do those of springbok. Eland are mainly nomadic, but sedentarism and migratory movements have been observed as well (Hillman, 1988). They jump over fences up to a height of 2 m (Owen-Smith, 1985) and more. The sedentary greater kudu (Tragelaphus strepsiceros) is a large, low water dependent browser living in small, relatively constant groups of less than 14 females (Skinner and Chimimba, 2005). The kudu is able to jump over fences up to 2 m as well (Owen-Smith, 1985).
Focusing on two major aspects, we are particularly interested in quantifying the energetic consequences of antelope-fence interactions and the underlying drivers and effects of such interactions. We expect that the animals interact with the fence since they track scattered resources on a large scale. Moreover, we assume that interacting with a fence causes a detectable increase in energy expenditure, which we define as an energy loss from an animal's energy budget. We expect this loss to be more pronounced in migratory species. The energy loss should result from entanglements, an increased effort to cross sections with remaining obstacles, or predator avoidance strategies, such as increased movement speeds, since some predators include fences into their hunting strategies (e.g., van Dyk and Slotow, 2003). While the larger area requirements of migratory species should induce rather frequent fence interactions, we expect sedentary ungulates to generally interact less with fences. When crossing a fence, we expect jumping species to spend more energy as compared to species which crawl under fences. Fence interactions should predominantly occur during seasonal turning points, i.e., when green or dry seasons start, since ungulates respond to the spatio-temporal dynamics of plant phenology (Esmaeili et al., 2021). According to the forage maturation hypothesis (Fryxell, 1991), species of high body mass should gain higher quantities of forage, whereas lighter species should gain forage of higher quality when the fence is crossed.
We discuss our key results in the light of strategic fence management within a landscape of in space and time scattered resources. Since there is an ongoing debate on the effectiveness of veterinary fences when only wildlife-wildlife interactions are likely (Sutmoller, 2002; Gadd, 2012; Ferguson et al., 2013; Weaver et al., 2013; Durant et al., 2015), strategic opening and closing of fence sections might satisfy two contrary claims: the ability to control disease outbreaks and un- or less hindered animal movements to maintain ecosystem functioning at a multi-use landscape scale.
Methods
Study area
We studied ungulate-fence interaction along the boundary of the regions Kunene, Omusati, and Oshana in the north of Namibia, 80 km south-west of the Etosha pan (15.2235°E, 19.2576°S). We focused on a section of Namibia's VCF (Figure 1), separating the private nature reserve “Etosha Heights” (EH) and Etosha National Park (ENP). Both are fence-less wildlife conservation areas of 460 and 22,941 km2, respectively.
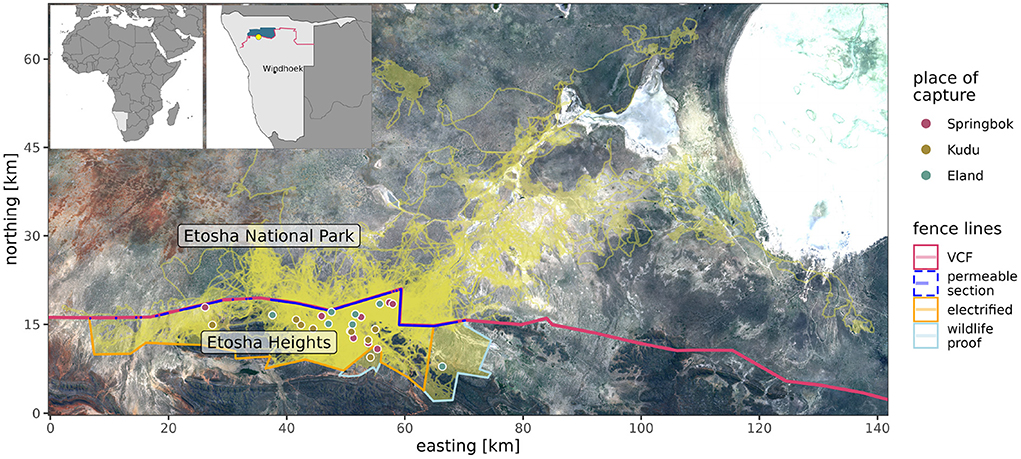
Figure 1. Study area with focal fence lines, identified parts where crossings occurred (permeable section) and locations of animal capturing (see legend) and movement trajectories (in light yellow). Background Sentinel 2 (Bands 3,4,5) image, March 2020 (Contains modified Copernicus Sentinel data [2020]). Upper left maps show location in Africa and Namibia. Namibia map shows veterinary cordon fence (VCF, red line) and focal wildlife conservation areas (blue).
The VCF, also known as the red-line, consists of two fence-lines separated by a 10 m buffer area. The northern fence-line, maintained by Etosha National Park, is a 2.8 m, 21-strand, wildlife-proof fence of which the lower 1.5 m is covered with jackal-proof wire mesh. The southern fence-line, which is maintained by the Directorate of Veterinary Services of the Ministry of Agriculture, Water and Land Reform, is a standard 1.5 m, 7-strand, stock-proof fence. The private reserve's southern, western and eastern fence-line, which is not part of the VCF, is a standard 2.4 m, 18-strand, wildlife-proof and electrified fence.
The VCF is frequently damaged by elephants and other wildlife since i.a. water supply is restricted to artificial water points during drier times. These water sources are numerous and well-maintained on EH, but they are scarce in the southern parts of ENP. Fence maintenance is limited by economic, or personnel capacities and breaches frequently remained for extended periods. Maintained sections were often broken again within a few days. The characteristics of fence breaches varied from partly broken strands to sections where strands and wire mesh were trampled down completely. The latter was the case along sections that were intensively used by many species.
Climate in the study region is semi-arid. Rainfall is highly variable but mainly occurs from September to April, when temperatures are high (mean temperature: 26°C). A colder dry season lasts from May to August (mean temperature: 18°C). Mean annual precipitation in the study area is 250 to 350 mm (averaged from 1981 to 2017 with CHIRPS data, Funk et al., 2015) with a gradient of increasing rainfall from south-west to north-east. In 2019, annual rainfall was 191 mm. In 2020 a severe, 2-year drought ended and annual rainfall was 333 mm as measured by a local weather station (WS-GP1 Delta-T Devices Ltd, UK).
Research was based at the private reserve which encompasses diverse landscape features ranging from rocky dolomite mountains over to plains with petric calcisols and correlated vegetation forms such as mountainous mixed woodlands (dominated by Terminalia prunioides, Commiphora glandulosa, and Combretum apiculatum), Colophospermum mopane wood- and shrublands, Vachellia nebrownii, and Catophractes alexandrii shrublands as well as grasslands dominated by Enneapogon desvauxii, Aristida adscensionsis, and Eragrostis nindensis. Most of the native mammals of the region occur in the study area and it is particularly high in abundance of carnivores and megaherbivores.
Study species
We selected Antidorcas marsupialis (springbok), Tragelaphus oryx (eland), and T. strepsiceros (kudu) females since they form larger herds and, thus, have the higher ecological impact per area. The females are the reproductive units of the populations and are likely to not fight and get injured because of the tracking device. The species' movement types range from migratory to sedentary. All three species are classified as non- or low-water reliant (Hayward and Hayward, 2012; Hempson et al., 2015a), but use water holes frequently in dry seasons, if available. The species differ in body mass (average weight of females: springbok—37 kg, kudu—152 kg, eland—305 kg, Skinner and Chimimba, 2005) and consequently in energy demand. We chose browsing and sedentary kudu as controls for the other species, since sedentarism should lead to few fence interactions, if the smaller and constant home ranges are free of fence lines. Mixed feeding and mixed migrating (as defined by Kauffman et al., 2021a) springbok are known to be selective feeders (Skinner and Louw, 1996) and should interact frequently with fences while moving to high quality feeding grounds. Mixed feeding and mainly nomadic eland are known to prefer grass and forbs during green season (e.g., Hillman, 1988) and should interact frequently with fences while moving to highly productive feeding grounds.
The three species are common in southern Africa's semi-arid savanna. While eland have largely been re-established on private reserves, kudu and springbok mostly occur naturally on game, mixed, and cattle farms. On Etosha Heights only eland were relocated from the Waterberg Plateau Park (250 km south-east of the study area) in the early 2000s.
Species collaring, tracking, and activity data
Springbok, kudu and eland were equipped with e-Obs GPS collars (e-Obs GmbH, Germany; springbok: Collar 1D with 320 g, eland and kudu Collar Big 3D with 840 g or Collar Big 4D with 960 g). Each individual was chosen from a different dry season group to avoid spatio-temporal overlap of the tracking data and cover the movement of many individuals per tracking device.
Animals were chemically restrained using the Pneudart system with either P-Type or C-Type remote delivery devices (RDDs) ranging in size from 0.5 cc to 2.0 cc, with needles from 0.5 to 2 inch in length and equipped with retaining barbs. RDDs were projected by either the Pneudart X-Caliber or Model 389 remote projectors.
Immobilizing drug cocktails were tailored by choice of veterinary medicine and dose according to species; springbok—thiafentanyl, ketamine and azaperone, eland—thiafentanyl and etorphine and medetomidine, kudu—thiafentanyl and azaperone and/or medetomidine. Hyaluronidase was added to eland and kudu cocktails. Reversal was carried out with naltrexone (opioid antagonist) and atipamizole or yohimbine (alpha 2 agonist antagonists) as appropriate. Animal handling permits were approved by the respective animal welfare licensing committee Namibian National Commission On Research Science & Technology, certificate number RCIV00032018 (authorization numbers: 20190602, AN20190808).
GPS localizations resulted from short recording sequences of 3 s with GPS fixes taken every second. For the analysis these three single fixes were averaged per recording sequence. The recording sequences were carried out by the collars every 15, 7.5, or 5 min depending on the battery size (Supplementary Table 1).
Three-dimensional acceleration was measured during recording sequences every five or two and a half minutes depending on battery size (Supplementary Table 1). One recording sequence lasted 3.3 s with a sampling frequency of 33.3 Hz per axis (X, Y, Z), resulting in 110 (k) measurements per axis (i as each single measurement). For the analysis the acceleration data was used to calculate the mean overall dynamic body acceleration (ODBA) per recording sequence (Equation 1) as an established proxy of energy expenditure (e.g., Qasem et al., 2012; Bryce et al., 2022).
For the analysis we used the data of nine springbok, seven kudu and seven eland individuals. During dry seasons we observed individual group sizes ranging from 12 to 45 per collared springbok, from three to six per collared kudu, and from seven to 14 per collared eland. Individual tracking days ranged from 147 to 939. Details are provided in Supplementary Table 1.
Identification and definition of fence interaction
All analyses were performed with R (version 4.1.2, R Core Team, 2021). Fence interactions were identified with geo-data packages terra, sp and sf (Pebesma, 2021; Pebesma and Bivand, 2021; Hijmans, 2022) and packages for data handling tidyr, dplyr and data.table (Dowle and Srinivasan, 2021; Wickham, 2021; Wickham et al., 2021).
We buffered the fence-lines with 50 m to yield areas with a width of 100 m. In the case of the VCF, we focused on the wildlife-proof fence-line for this. We chose a 50 m buffer to account for the inaccuracy of the geo-data (fence positions and GPS localizations) and, simultaneously, to avoid misclassification of fence interactions due to a too broad buffer. Further, the 50 m buffer assured comparability to other studies using a similar approach (Xu et al., 2021; Laguna et al., 2022). We calculated the distance to the actual fence-line for each localization. Polygons of the areas were seamlessly separated by the fence -line.
We identified tracks of fence interaction by selecting subsequent localizations, which fell into the buffered fence area. In order to not overestimate the total number of interaction events, we allowed short excursions from the buffered area within a fence interaction event and, thus, reduced the total amount of events. For this, we used a time threshold, which was identified with a sensitivity analysis and defined as the value at which the total number of fence interaction events did not change more than 2.5% (Supplementary Figure 1). Following this, we allowed subsequent localizations with a time threshold of 60 min to be outside of that buffered area if the former and next were inside and assigned them to the same fence interaction event. Events with less than two localizations were classified as quick and all others as “long” (example see Figure 2).
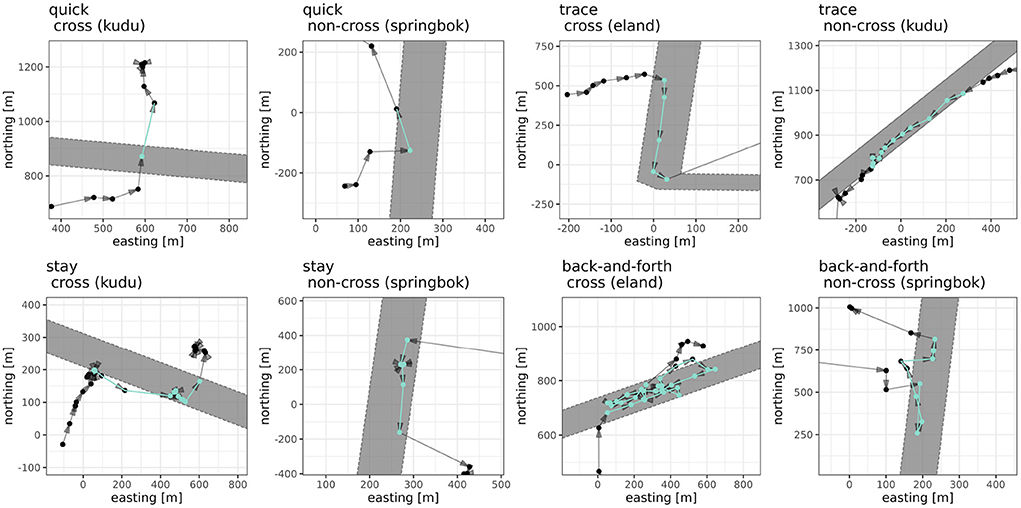
Figure 2. Examples of classified fence interactions. Shown are examples for each of the classified types (quick, trace, stay, back-and-forth) and subtypes (cross, non-cross), see panel titles. Gray area depicts the fence area (fence line with a 50 m buffer).
We adapted the fence interaction types introduced in Xu et al. (2021), and distinguished basic interactions into crossing events, i.e., movement from EH to ENP or opposite (cross), and fence visits (non-cross). Depending on movement behavior and time spent at a fence, basic interactions were classified into four interaction types: (i) quick, as immediate cross or short visit, (ii) trace (no changes in direction), (iii) back-and-forth (changes in direction, at least one relative angle larger than 120°), and (iv) stay (at least one event of subsequent speeds below 0.5 m/s lasting longer than 15 min regardless of changes in direction). Fence interactions events of the type quick consisted either out of the two subsequent localizations with the fence area between (if no localization was at the fence area) or out of the localization at the fence area. In order to complete the list of identifiable fence interaction types the type stay was added to the original types introduced in Xu et al. (2021).
Seasonal dynamics of wildlife-fence interactions
To asses the seasonal dynamics of ungulate-fence interactions, we first extracted the general temporal trend of detected cross and non-cross events and, second, analyzed the relationship between time to seasonal turning point and fence interaction events. Thereto we calculated the averaged number of cross and non-cross (per fence type) events per day and tracked individuals, thereby accounting for differing numbers of tracked animals along the full observation time. On this data we performed a fast Fourier transformation (function fft in stats-package, R Core Team, 2021) to identify meaningful frequencies for time series creation. As we found a local peak in magnitude at 29 days (roughly the lunar cycle), which corresponded to prior analysis of general activity patterns (Gordigiani et al., 2022), we used this period for the time series creation. Then, we extracted the trend by decomposing the time series (function decompose in stats-package, R Core Team, 2021) and analyzed it graphically.
Since we expected fence interaction to peak at seasonal turning points, i.e., starting of the green season initiated by first rainfall, we graphically checked for this in the temporal trend of fence interaction events. Based on this, we tested for a relation between fence interaction per se and the onset of the green season by fitting generalized linear mixed-effects models (GLMM, binomial error structure and logit link function, lme4-package, Bates et al., 2021) with binary data on daily crossing or non-crossing events as response variables, respectively, and number of observed individuals and days to first rain as predictors and the respective year as a random factor to account for intra-annual differences. We included the fixed effect number of observed individuals per day as a controlling factor to account for the variety of observation periods for the different individuals and the resulting higher probability to detect a fence interaction if more individuals were observed. Since the predictor variable days to first rain is only meaningful for times after the green season, we limited our analysis to the interval between 45 days after the last rainfall (recorded by our local weather station, maximum: 180 days), or the main drop in vegetation greenness (visually verified from NDVI time series), and the first rainfall (recorded by our local weather station, minimum: 0 days) of the respective year. We compared the models to null-models using a chi-square test to assess if they are not random if appropriate. Null-models contained only the random factor. We used partR2-function with 500 iterations to calculate R2 and 95%–confidence intervals for full models and semi-partial R2 for predictors (Stoffel et al., 2020).
Direct effects of wildlife-fence interactions on energy expenditure
We compared mean ODBAs (as proxy for energy expenditure) 45 min before the actual fence interaction, during the interaction and 45 min after for each combination of fence interaction type and species. We chose a time window of 45 min because we aimed to average on at least nine consecutive 3D-acceleration measurements and, simultaneously, to restrict the number of covered movement phases. Thus, the time window resulted from the maximum acceleration recording rate of 5 min. We used heteroscedastic one-way repeated measures ANOVAs for trimmed means (Mair and Wilcox, 2020) using WRS2-package for the comparisons. Trim level for the means was 0.2. For the identified general significant differences between average ODBA before, during and after an interaction, we used post-hoc tests with adjusted p-values (Hochberg's approach) for assessing actual differences. Single fence interactions were treated as repeated measures per species. Furthermore we were interested in differences between fence interaction events of the same type (quick, trace, stay, back-and-forth) but different subtype, i.e., cross or non-cross. We used robust two-way between-within subjects ANOVAs on trimmed means (Mair and Wilcox, 2020) to test for such and used the bootstrap-based approach for modified one-step estimators (500 iterations) to compute the main effect (for further details of these methods, see Mair and Wilcox, 2020).
We define a fence related energy loss to occur, if the energy expenditure during a fence interaction event increased as compared to the expenditure before and after the event. In this regard, we assume that the fence interaction mainly contributed to the increase in energy expenditure, and that this results in a loss within the animal's energy budget.
Indirect effects of fence gaps on energetics
In order to quantify what the animals gained from an opened fence we calculated Normalized Difference Vegetation Index (NDVI) and Modified Soil Adjusted Vegetation Index (MSAVI, see Qi et al., 1994) time series from both the Sentinel-2 and Landsat-8 missions. We used these data to first define vegetation types and, second, quantify differences in regional productivity per vegetation type.
Preparation of forage indices
Highly seasonal vegetation in the study region makes traditional vegetation mapping approaches difficult; dry and green season vegetation indices of the same species can vary wildly based on water availability (Ibrahim et al., 2021). As vegetation type changes over the study period (2019–2021) were minimal, we adopted a time-aware means of vegetation classification, which takes advantage of multi-year vegetation data. We first collected 190 well-known data points covering the 13 dominant vegetation types in the study area (Supplementary Figure 2). Vegetation typing was based on le Roux et al. (1988). Using the NDVI and MSAVI time series derived from these well-known points, we derived the “distance” (e.g., correlation coefficient) to any other location's time series; this approach has been used extensively to cluster locations into self-similar groups (e.g., Murtagh and Contreras, 2012; Rheinwalt et al., 2015; Smith et al., 2017). For each 30 m pixel in the study region, we then derived a correlation distance to each of the 13 dominant vegetation types; vegetation type was assigned based on the maximum correlation among vegetation types (Supplementary Figure 2). We validated our map during subsequent in-situ field assessments and found vegetation classes to be reliable at the scale of our analysis.
Calculation of loss and gain in forage indices
We calculated an available area for each individual using Minimal Convex Polygons (MCP derived with adehabitatHR-package, Calenge, 2006) covering all GPS locations. Subsequently we derived the mean MSAVI and its time series slope per MCP, vegetation type and day as follows. First, mean MSAVI (as index of greenness and proxy for forage quantity; see Borowik et al., 2013; Esmaeili et al., 2021) per vegetation type, Sentinel-2 scene and MCP were calculated (global function from terra-package, Hijmans, 2022). Second, the irregular Sentinel-2 time series were regularized using Z's ordered observation function (zoo-package, Zeileis and Grothendieck, 2005) and extrapolated to daily values via local polynomial regression fitting (degree of smoothing: span = 0.15). Third, we used an exponential smoothing state space model (ets-function from forecast-package, Hyndman and Khandakar, 2008 with multiplicative error, additive trend and non-season type) to derive the slope in MSAVI (as index of greening and proxy for forage quality; see Bischof et al., 2012; Esmaeili et al., 2021) for each day, relative to the former day (daily rate of green-up). We proceeded for each GPS-location in a similar way (getting regular, daily MSAVI time series and slopes), so that we retrieved an interpolated MSAVI- and slope value time series for each position.
As to derive metrics of time-, vegetation-type- and individual-specific gain on each fence site, we calculated the difference between daily mean of the MSAVI (value and slope) of all GPS positions from that day (within or beyond the fence line) and the daily mean MSAVI (value and slope) of the full MCP. Positive values indicated a gain while negative ones indicated a loss as compared to the average within the MCP. Note that GPS locations (32,269 from 921,661) were excluded from the analysis either because individuals did not visit both fence sites intensively enough (minimum 200 records) or because reliable vegetation type classification was not possible due to remoteness of locations.
We averaged (mean ± SE) daily MSAVI values (proxy of forage quantity) and slopes (proxy of forage quality) for each combination of species, season and vegetation type. For this we only used daily individual values with more than one observation per vegetation type. Furthermore, we only focused on the seasons with substantial green vegetation (early, mid and late green and early and mid dry season), the nine vegetation types mostly used (grasslands on lime or sand; open small shrublands: C. alexandri, V. nebrownii or small C. mopane; shrub- and woodlands: medium sized or tall C. mopane, V. reficiens, mixed stands on plain outcorps; see Supplementary Figure 2 for further details) and those species which regularly crossed the fence (eland, springbok). Furthermore we transformed the gain values to interpretable scales. The quantitative gain (difference in MSAVI) was transformed to the percentage of the mean MSAVI of the MCP per vegetation type and day and the sign was kept. We proceeded in the same way for the slope (difference in MSAVI change).
Results
Seasonal dynamics of wildlife-fence interactions
Based on our high-frequency movement data of 24 GPS-collared individuals with a total of 1.7 million GPS localizations, we identified 2905 fence interaction events (springbok: 932 events, kudu: 875 events, eland: 1098 events) between June 2019 and November 2021. Number and intensity of wildlife-fence interactions differed across seasons (Figure 3). Crossing events were relatively infrequent from the beginning of the rainy seasons toward the middle of the dry seasons (November to July) and peaked just before the onset of the rainy seasons (2019-10-19, 2020-09-26, 2021-09-21). Non-cross events at the permeable VCF did not show a clear pattern along the observation time, while non-cross events at the electrified fence (EF) tended to also peak around the onset of the rainy season (Supplementary Figure 4). Visually comparing crossing and vegetation greenness dynamics, we found crossings to peak when vegetation greenness was lowest and vice versa crossings to be lowest during peak vegetation greenness.
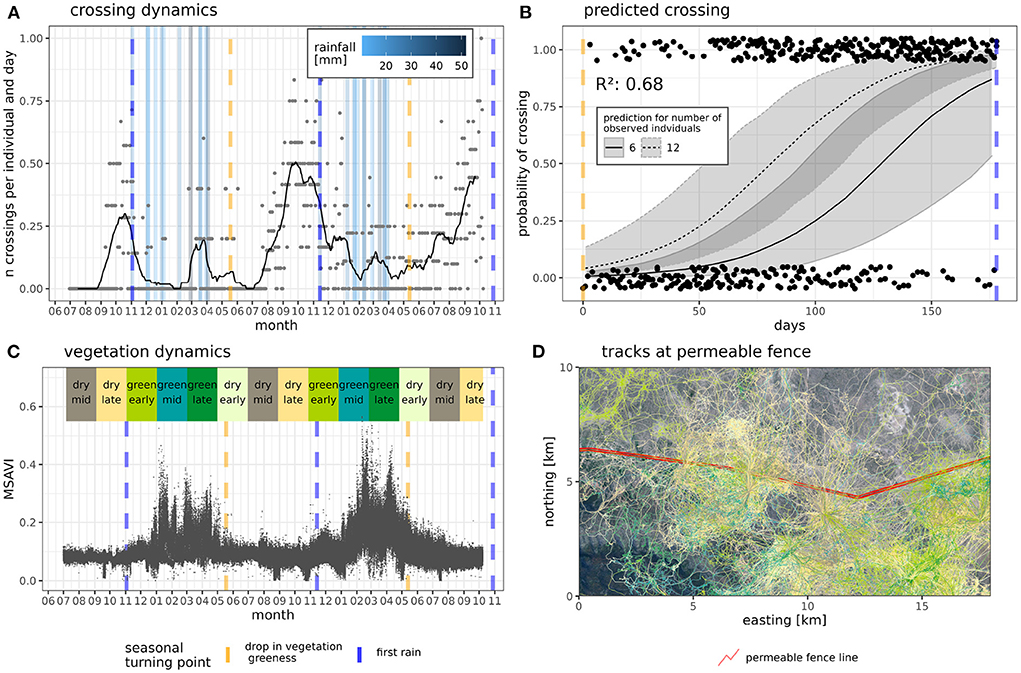
Figure 3. Fence interaction dynamics and predictions. (A) Number of crossings averaged by number of observed individuals between June 2019 and Nov 2021 (points), trend from time series (line) and rainfall events (vertical bars, see legend). (B) Observed fence interactions (binomial, jittered points) and probability of fence crossing (binomial regression line) between dry season and first rain event. Orange dashed line indicates drop in vegetation greenness in dry season, vertical blue dashed line marks first rain event of the respective year. Prediction of crossing probability (line) with 95% confidence intervals of binomial regression based on differing numbers of observed individuals (see linetype in legend). (C) MSAVI values at each GPS location (points) and range of season (colored rectangles on top) for clarification of seasonal dynamics. (D) Map of example extent with movement tracks and permeable fence line (South of line—private wildlife reserve, North of line—Etosha National Park). Tracks are color coded by season [see season colors in (C)].
The GLMM to predict fence crossing at the VCF explained 64.3% of the variation in observed crossing (marginal R2; 95% CI: 0.55–0.76). The number of days to first rain already explained 28.1% (semi-partial R2, 95% CI: 0.08–0.50) of the variation (Supplementary Table 2). The model was significantly different from the null model. The controlling factor number of observed individuals had a positive effect (0.30 ± 0.07 SE) and the predictor variable days to first rain had a negative effect (−0.04 ± 0.01 SE), indicating an increase in crossing probability the closer the first rain is to come. The GLMMs to predict non-cross interaction at the VCF and the electrified fence were not significantly different from the respective model without days to first rain as a predictor variable (see Supplementary Table 3). Based on the parsimony criterion we discarded the models of non-crossing events.
The seasonal dynamics of fence interactions differed between species. While trends of springbok and eland showed pronounced peaks in crossing the VCF just before the first rainfalls, kudu showed less pronounced peaks. Trends of non-cross interactions (Supplementary Figure 4) showed no clear patterns at the VCF. Trends of non-cross interactions at the electrified fence showed local peaks during the green season 2020/21 for eland and a local peak for springbok only just before the onset of the green season 2019/20 but not for the following year.
Direct effects of wildlife-fence interactions on energy expenditure
The most common fence interaction types with effects on energy expenditure were quick cross (springbok: 321 events, kudu: 98 events, eland: 353 events), stay non-cross (springbok: 142, kudu: 325, eland: 265) and trace non-cross (springbok: 127, kudu: 157, eland: 129). Energy expenditure (ODBA) differed during the fence interaction in comparison to before and after the interaction (a selection of results at Figure 4; full overview at Supplementary Figures 5, 6) for ten out of the 24 combinations of fence interaction type and species (springbok: quick non-cross, trace non-cross, stay cross; kudu: quick cross, trace non-cross, stay non-cross; eland: quick cross, quick non-cross, stay cross, stay non-cross;). Therein we found an increase in energy expenditure during the fence interaction for quick cross, quick non-cross and trace non-cross and a decrease for stay fence interactions.
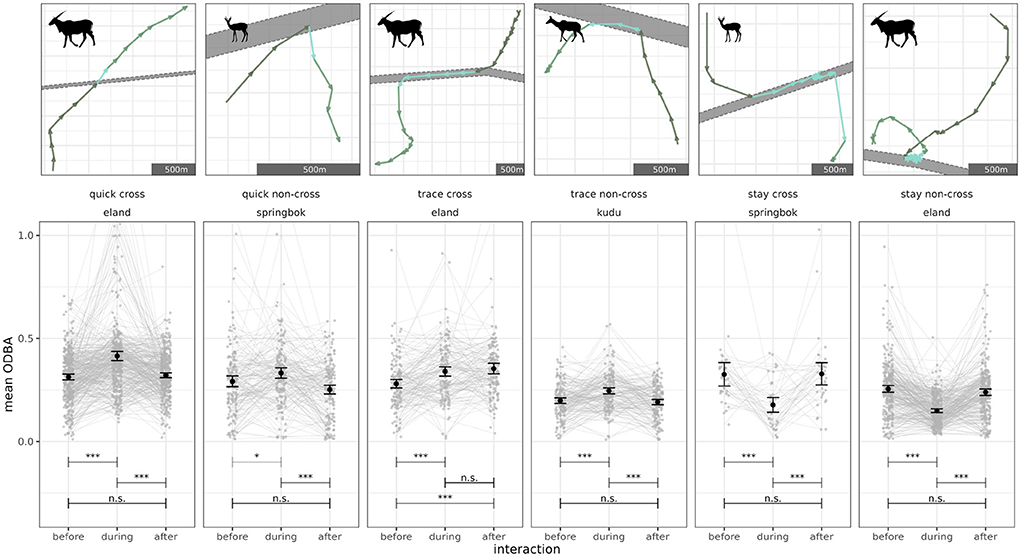
Figure 4. Impacts of fence interaction on ODBA (overall dynamic body acceleration) as proxy for energy expenditure (selection of results). First row: single example tracks for quick, trace and stay fence interaction types each with cross and non-cross sub-types. Dark green indicates the track before the animal meets the fence, blue green indicates the interaction (during) and light green indicates after the interaction. Movement direction is indicated by arrows. Axes are scaled according to the data (see scale bars). Second row: average ODBA (gray points) of all measurements 45 min before or after the respective fence interaction and during the interaction with each event connected by a line. Overall mean (black points) and standard error (black error bars) shown per before, during or after the interaction sample group. Panels refer to fence interaction type, if the fence was crossed (cross) or not crossed (non-cross) and the selected species column-wise (see panel titles). y-axis is limited to 1.0 for presentation purposes, plotted data is not. Test significance indicated as ratio between critical p-value of post-hoc test and p-value of post-hoc test with: <1: n.s. (non-significant); > 1: *; > 10: **; > 100: ***.
We compared equal fence interaction types with different subtypes (cross vs. non-cross) species-wise and found no differences for back-and-forth and trace interactions of springboks. In all other cases, differences were significant and the estimated effect was positive, indicating a higher mean ODBA when animals of the respective species crossed as compared to the same fence interaction type but without crossing (Supplementary Table 5).
Indirect effects of fence gaps on energetics
During the growing season, when animals were at ENP, forage quantity (MSAVI value) and quality (MSAVI slope) of sites used by individuals on a day were often higher than the average of forage within the individuals' available area (MCP) on that day. This gain in both forage quality and quantity occurred in 35% of all combinations of day, individual and vegetation type (total cases: eland—2,435, springbok—3,014). Eland gained forage quantity on 64% and forage quality on 35% of all days they were outside the fenced area (as compared to the mean of the available area). Springbok gained forage quantity on 57% and forage quality on 66% of all days they were outside the fenced area. Only one kudu crossed the VCF in a notable manner (sufficient number of observations per time unit), consequently kudu were not considered for this analysis.
For eland and springbok, we found on average (species-wise mean) a gain in forage quantity (Figure 5) at ENP (as compared to the mean of the available area, which includes both sides of fence) in 76% of the combinations of season, species and vegetation type (eland: 76%, springbok: 76%) and a gain in forage quality in 56% of these combinations (eland: 60%, springbok: 52%). A loss in both forage quantity and quality was only identified in 13% of the combinations (eland: 6%, springbok: 19%).
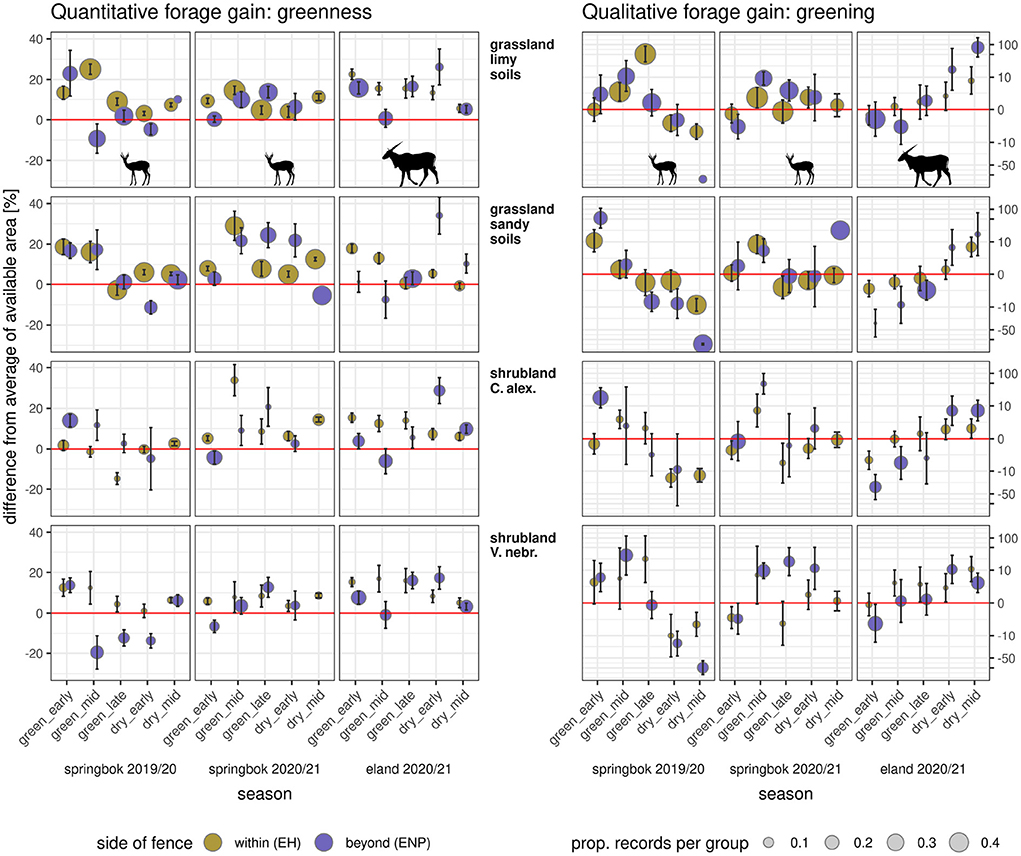
Figure 5. Gain or loss in forage quality and quantity in relation to the fence side. Species- and season-wise (columns of panels, see column titles on bottom) and vegetation type specific (rows of panels, see row titles; C. alex., Catophractes alexandri; V. nebr.. Vachellia nebrownii; details in Supplementary Figure 2) gains calculated from time series of Sentinel-2 satellite images. Left: gain in MSAVI (or forage quantity) as the difference between mean daily (interpolated) MSAVI at positions and mean daily MSAVI of the available area (individual-wise MCP). Right: gain in MSAVI slope (or forage quality) as the difference between mean daily (interpolated) MSAVI slope at positions and mean daily MSAVI slope of available area (individual-wise MCP). Differences are scaled as percentages of the available area's mean with algebraic signs kept. Points depict the mean of records per season (x-axis) with standard error (error bars). Positive values indicate a gain and negative values indicate a loss. Point colors refer to location (see legend). Point size is relative to the proportion of observations falling into the respective vegetation type per species and season (see legend).
The vegetation types eland visited the most (> 50% of all 85,651 single observations beyond the VCF during the focal seasons from six individuals) were Catophractes alexandri shrubland (CA, 12.4 %), open grassland (OP, 13.3%), Vachellia (Acacia) nebrownii shrubland (AN, 15.6%) and grassland on lime (SP, 18.2%). For all of these vegetation types, average MSAVI was always higher on visited patches at ENP (beyond the VCF) as compared to the mean of the available area during late green, early and late dry season (e.g., SP late green season: 17.3 % higher [3664 observations], CA mid early dry season: 27.7% higher [1643 observations], OP late green season: 2.7% higher [9991 observations]). Average values of gain in MSAVI slope (forage quality) differed largely among vegetation types and seasons. We recorded the highest gain in forage quality on SP during mid green and the largest loss on AN during early green season.
Springbok mostly visited (50% of all 75,838 single observations beyond the fence during the focal seasons from six individuals) the vegetation types Catophractes alexandri shrubland (CA, 10.3%), Vachellia (Acacia) nebrownii shrubland (AN, 12.1%), open grassland (OP, 20.6%), and grassland on lime (SP, 30.2%). For all of these vegetation types we identified a higher MSAVI on average during early green season 2019/20 and mid green season 2020/21 (e.g., SP early green season 2019/20: 23.9% higher [1293 observations], OP mid green season 2020/21: 20.6% higher [1368 observations], CA early green season 2019/20: 14.0% higher [5918 observations], AN mid green season 2020/21: 3.6% higher [2764 observations]). On almost all of these vegetation types, the average gain in forage quality was higher beyond the fence than the mean of the available area during the mid green season (except CA 2019/2020). The highest values of gain in forage quality were recorded for grassland (OP) during the early green season in 2019/20 and for shrubland (AN) in mid green season 2019/20. The largest losses in forage quality were recorded for grassland (OP) during the late green season and shrubland (CA) during the early green season in 2019/20 and for grassland (OP) during early dry season as well as shrubland (AN) during the early green season 2020/21 (Figure 5).
Discussion
The movement tracks of three large African antelope species highlight the importance of the Veterinary Cordon Fences' (VCF) permeability to avoid a direct energy loss at the fence and to access sufficient forage under temporally and spatially erratic plant production. Our results further demonstrate that animal-fence interactions are very common if fences are located within individual home ranges. On 68% of all of our tracking days we recorded interactions of the tracked individuals with the VCF. The majority of these interactions (88%) was observed for migratory springbok and eland. Both species often traveled long distances along the VCF [on average springbok: 28.9 ± 11.2 (SD) km/year, eland: 64.5 ± 52.2 (SD) km/year].
The assigned energetic signatures showed that depending on the type of animal-fence interaction, antelopes either spend less, the same amount, or in many cases more energy when they interact with a fence than immediately before or after encountering the fence. Interestingly, when we compared fence interactions of the same type but with differing crossing success, we found an increased energy expenditure when crossing was successful. Reviewing the 21 studies identified to consider fence related energy expenditure in McInturff et al. (2020), we did not find a single one to include direct measurements of ODBA at fences. Rather, the studies examined behavior through observations and focused on the effectiveness of fencing. In this sense our findings fundamentally contribute to the emerging discipline of fence ecology by providing first insights to the energetic impacts of fences for African ungulates.
The individuals which successfully crossed the VCF were capable of following patchy distributed feeding-resources over a large spatial scale [average area [95% utilization distribution] used at ENP or beyond the VCF: springbok: 246 ± 493 (SD) km2, eland: 193 ± 217 (SD) km2] and benefited from a clear gain in forage quality and quantity. We found temporal peaks in fence crossing shortly before the onset of the growing season and, connected to that, a significant increase in crossing probability shortly before the onset of the first seasonal rain. The ungulates visited greener patches (11.9% greener on average) and also expanded the duration of their experienced green season as they reached some patches with early occurring first flushes and others with delayed dry out. This is an immanent behavior of non-sedentary ungulates (e.g., Esmaeili et al., 2021) but demonstrating this in relation to a VCF and for native antelopes of southern Africa has to our knowledge never been done before and provides fundamental knowledge for strategic management planning.
Seasonal dynamics of wildlife-fence interactions
Our findings support evidence that seasonal patterns of plant-phenology trigger large scale movement of non-sedentary ungulates globally [e.g., Owen-Smith et al. (2020) (Africa), Peters et al. (2019) (Europe), Aikens et al. (2020) (North America), Schroeder et al. (2014) (South America), Nandintsetseg et al. (2019) (Eastern Asia)]. Our simple model to predict crossing events in relation to time toward the seasonal turning point appears plausible. However, the fact that crossings peaked weeks before we measured the first significant rainfall was unexpected. We see a combination of mechanisms driving this finding.
In contrast to temperate climates where greening occurs along temperature gradients in a wave-like pattern (Aikens et al., 2020), the greening in most semi-arid landscapes strongly depends on effective rainfall (Archibald and Scholes, 2007; Ibrahim et al., 2021). In combination with an uneven spatial distribution of such rainfall events, this creates an erratic mosaic of greening patches along ephemeral rivers, in depressions and at the rainfall locations themselves at different times. While herbaceous plants respond directly to rainwater in the top soil layer, woody plants can store water and many trees and shrubs flower and foliate weeks before the rain season starts (Archibald and Scholes, 2007; Ibrahim et al., 2021). These first flushes are of good nutritional value (Makhado et al., 2016; Marius et al., 2021) and are eaten by many ungulates, irrespective of the feeding type (Skinner and Chimimba, 2005). Consequently, ungulates will track patches of sufficient flushed biomass in semi-arid landscapes. Notably, they must be capable of reaching such greened places rapidly, since growth periods of arid-adapted vegetation are often short (Noy-Meir, 1973). On the one hand, large depressions such as the Makgadikgadi pans in Botswana or the Etosha pan in Namibia form reliable grazing sources as water from vast and wetter areas drains into them, which causes periodical ungulate migrations like the Makgadikgadi zebra migration (Bartlam-Brooks et al., 2011) or the historic West–East Etosha migration of oryx and elephant (Tinley, 1971). On the other hand, patches, which green-up due to local rainfall events, drive the movements of ungulates at a smaller scale. For instance, all of the tracked springbok groups moved northwards across the VCF to a drainage depression immediately after the first rainfalls in 2019 when a severe drought ended, but did not move there the following two green seasons. In this sense, small scale rainfall events might have triggered some antelope movements across the fence before we measured the first rainfall of the respective year.
The detected peak in fence crossings toward the end of the dry season is likely to be caused by local green-ups across the fence where the ungulates moved to. Simultaneously, as natural surface water was not available and the overall green biomass was still low, the animals regularly moved back to the artificial water holes within the private reserve and, thereby, crossed the fence very often. Interestingly, once the rain seasons started, some individuals went long distances and times outside the private reserve (up to 80 km and more than 6 months away), others did shorter excursions outside and yet others did not leave the private reserve at all. When vegetation greenness dropped again, the tagged individuals of all ungulate species returned to the private reserve and visited the artificial waterholes on almost a daily basis. These dynamics show that fence interactions occur all year long, but depending on movement types seasonal peaks appear. At least for the VCF, where gaps were created by elephants, we found a drop in fence encounters during the green season caused by the ability of many individuals to occupy sufficient feeding areas regardless of the fence side.
Our results confirm recent findings of Wilkinson et al. (2021), who found ungulates to mostly cross fences during the day but did not find the seasons to influence this behavior. Focusing on crossing per se, we found clear seasonal dynamics in interactions with the VCF, demonstrating that only during peak dry seasons the ungulates' movements were less affected by this fence, while an intact VCF would have blocked them during the rest of the year. Conclusively, a permanent non-permeable fence would have tremendous fitness consequences for antelopes, especially when the green season is about to start.
Direct effects of wildlife-fence interactions on energy expenditure
We found major forms of animal-fence interactions to be either quick, trace-like or including a stay event. This contrasts with the findings of Xu et al. (2021) who found Odocoileus hemionus (mule deer) and Antilocapra americana (pronghorn) to dominantly interact quickly with fences. On the one hand, these differences could result from behavioral traits, e.g., Found and St. Clair (2019) found that bolder elk (Cervus canadensis) are rather tolerant to anthropogenic infrastructures, or predator avoidance strategies, e.g., van Dyk and Slotow (2003) found African wild dog to particularly hunt along fence-lines, leading to rather short ungulate-fence interactions. On the other hand, such differences likely result from the lower temporal GPS resolution (Xu et al., 2021) used (hourly vs. 5–15 min in our study), possibly leading to fewer identifications of other than quick interactions. The high temporal resolution of our localization data enabled us to identify hundreds of events during which the antelopes paused at fences for considerable time. This finding adds to the types of animal-fence interactions identified by Xu et al. (2021) (based on movement data) and is in line with the frequently observed “vigilance” behavior of Wilkinson et al. (2021) at Lake Nakuru National Park (Kenya) (based on camera trap data). Of our 24 tracked individuals, 18 crossed the VCF more than 1,400 times in total within the 2 years of observation. We recorded crossings for all three species, with eland and springbok regularly trespassing the VCF. Permeable fences are often crossed by ungulates and other wildlife (e.g., Kesch et al., 2014, 2015; Dupuis-Desormeaux et al., 2018; Jones et al., 2019; Seigle-Ferrand et al., 2021), although their movement patterns are affected (e.g., McKillop and Sibly, 1988; Harrington and Conover, 2006; Sawyer et al., 2013; Laskin et al., 2020; Wilkinson et al., 2021). Permeable fences may not act as a general barrier but as an obstacle which leads to altered behavior and, consequently, energy expenditure. The latter we explicitly analyzed through measured changes in ODBA of the animal and found direct effects of the fences on the antelopes' motion patterns.
We identified plenty of events with eland or kudu spending dozens of minutes to a few hours at the same location while encountering a fence (stay non-cross). By definition these events were marked by sequences of non-locomotion and the comparably low ODBA proposes stationary halts or vigilance behavior during such events. This may indicate confusion of the antelopes when a fence blocked their intended movement, followed by a new movement decision-making process. Such fence interactions lasted on average more than 2 h and are frequently observed in ungulates (Park et al., 2021; Wilkinson et al., 2021). Although spending less energy directly, ungulates likely lose a lot of time during such events. For instance, we found an eland to spend a total of 4 days out of the 442 observation days during such stay non-cross fence interactions (32 single events).
Most fence interactions resulted in increased ODBA (most frequent consequence of interaction in eland, second most in springbok and kudu). This highlights the severe direct impact fences can have on energy expenditure of wildlife. In cases of crossing at the VCF (quick cross of eland), the high ODBA values likely result from the necessity to jump over half broken fence sections, entanglement in wires or similar behavior as observed for many ungulate species globally (e.g., Harrington and Conover, 2006; Laskin et al., 2020; Wilkinson et al., 2021). In cases of non-cross (quick non-cross of springbok, trace non-cross of springbok and kudu), the increased activity at the fences is likely caused by attempts to cross or urgent behavior during the search for a gap. Ungulates will cross (semi-) permeable fences and will frequently try to cross impermeable fences which traverse their habitats. For instance, Xu et al. (2021) estimate 250 fence encounters per year for a pronghorn migrating through the rangelands of western Wyoming, USA; we found 44 encounters for a springbok only at the VCF (maximum: springbok 6770 with 134 encounters in 2020). Bearing this in mind, single short-term events of increased energy expenditure will sum up over time and, thus, negatively influence individual long-term energy balances.
Further, we identified many situations without any significant change of ODBA at the fence. Despite non-cross interactions of trace-like walks along the fence line, which might represent only slight changes in movement direction due to the barrier or periods of foraging events, we found specific types of cross events in all species to not clearly affect ODBA. Likely these events occurred on locations with more or less clear, easily identifiable gaps and persistent gaps allowing successful crossing at known locations and thus becoming a habituated behavior, which has been documented earlier (e.g., Dupuis-Desormeaux et al., 2018; Wilkinson et al., 2021).
These first results on energetic consequences of fences for ungulates demonstrate that, first, impermeable fence lines will lead to an increase in motion and thus in energy expenditure. Second, they show that inconsistent and vague permeable fence lines will lead to high energy expenditure during the crossing. Third, persistent and obvious gaps will not directly affect energy expenditure during crossing. Therefore, our findings form a solid base for further investigations on such short-term effects, e.g., the temporal dynamics of energy expenditure along each interaction event or the long-term dynamics at specific locations as to study learning effects.
Indirect effects of fence gaps on energetics
Individuals of all three antelope species which traveled beyond the VCF fed on up to 40% greener vegetation as compared to the average found in their available area. They were also able to move to areas with fresher biomass and, thus, optimized energy intake. This emphasizes the importance of permeable fences for ungulates that enable them to track a sufficient amount of resources.
Our results align well with recent findings on the forage maturation hypothesis (Esmaeili et al., 2021) and demonstrate the dynamics in animal habitat formations (Aikens et al., 2020). Esmaeili et al. (2021) extended Fryxell (1991) and others' work where highest forage quality occurs at intermediate maturation stages of plants. They adjusted the maturation stage—energy uptake optima according to body sizes, digestive system and water dependency of larger herbivores and tested for this using a global set of animal movement and plant phenology data. Although they generally show that the smaller the herbivores are the more they select for high quality forage, they particularly did not find springbok to select for high quality forage and slightly avoiding higher quantities. Already in the postulation of the forage maturation hypothesis, Fryxell (1991) points out that forage maturation effects should be limited under semi-arid conditions, with short and unpredictable rainfall, and in smaller, selective ruminants. However, Esmaeili et al. (2021) worked on relatively coarse temporal and spatial scales which might have concealed species-specific, dynamic selectivity for forage quantity and quality. As claimed by Nghiyalwa et al. (2021), remote sensing based analysis of phenology dynamics in savannas requires high resolution satellite data.
By using such high resolution satellite data and focussing on vegetation types our results show dynamic patterns of forage ground selection. For instance, we found springbok during the beginning of the green season to be on patches of higher quantity (as compared to the average of all patches of a vegetation type within each individuals available area) but mostly not of higher quality, while selected patches during peak and end of the green season showed rather higher quality than quantity, pointing at the selectivity of these rather small ungulates to high-quality forage. We found the large eland to constantly be on patches of above-average quantity with fluctuating quality, demonstrating their selectivity for patches of high quantities of forage.
Aikens et al. (2020) demonstrate that habitat formation in ungulates is a dynamic process following the fluxes of resource availability. They focus on temperate landscapes with wave-like green-up patterns. However, the principle that ungulates move to areas where at a specific point in time high-quality forage is available must hold true for other climates too. Indeed, it has been known for decades that many southern African ungulates switch food sources during the course of the year (Skinner and Chimimba, 2005). Our analysis shows that the tracked species move to specific vegetation patches of better phenological quality than others within their available area. With this, we provide a basis for further studies in order to expand the green-wave surfing concept to dynamic habitat formation (Aikens et al., 2020) and show in detail how remarkably these animals track the scattered resources of such semi-arid landscapes, a fact that has been known to indigenous peoples since antiquity (Roche, 2005).
Management implications and future perspective
We see gaps in the VCF, which were mostly created by elephants, to be of significant benefit for our study antelopes. Especially the mixed-feeding springbok and eland were able to track scattered, high-quality resources on a large scale and thus likely improved individual health. Furthermore, these gaps mediated the grazing pressure between the pastures on both sides of the fence and, thereby, likely reduced the risk of ecosystem degradation. Also, as ungulates were able to reach resources and mates on both fence sides, these gaps likely play a key role in improving genetic diversity and reproductive success. In the future, the larger herbivores will have an exacerbated need to reach resource patches across vast areas since the degree of aridity in southwestern Africa will increase as a result of climate change (Iturbide et al., 2020) and suitable habitats are likely to move beyond the boundaries of current protected areas, increasing the need for larger unfragmented areas (Turpie et al., 2010).
Although this study focuses on interactions between wild herbivores and the VCF, we recognize that the VCF plays a major role in Namibia's beef economy due to international requirements for certified disease-free meat exports, which include the presence of an effective fence. However, our findings could add to the ongoing debate regarding social, economic and ecological benefits and impacts of this fence (e.g., Sutmoller, 2002; Scoones et al., 2010; Weaver et al., 2013; Mogotsi et al., 2016). Within this debate, the benefits and the long-term maintenance of the VCF are increasingly being questioned. While negative effects on wildlife ecology are observed (Martin, 2005), the trade regulations set by the World Organization for Animal Health (OIE) induce the government to maintain the fence, which is frequently damaged by elephants and other wildlife.
A key problem with gaps created by elephants is that animals which use these gaps, such as our study ungulates and other species, are exposed to a severe risk of entanglement. Temporary repairs may only increase this risk since animals are habituated to use such sections and will likely intensively try to cross at these locations. Consequently, effective measures are needed to eliminate such injury risks in the future.
A first step could be the implementation of managed gaps in the VCF on locations where complimentary land-uses on both sides of the fence minimize the risk of wildlife-livestock disease transfer and anti-poaching measures are effectively implemented (Hayward, 2012). Many studies have shown that there are a variety of effective options for such. Gaps can be installed to be selective for certain species (e.g., exclusion of rhinos by rock walls: Dupuis-Désormeaux et al., 2016; exclusion of livestock by tubular metal grills: Gates et al., 2012; selective fence designs: Laskin et al., 2020; Segar and Keane, 2020) or simply open for all species. Animals will incorporate them into their movement corridors over time (Dupuis-Desormeaux et al., 2018). Since our study recognized clear temporal peaks in cross-fence movements, in the event of permanent corridors not being an option, a gated system at pressure points in the VCF could be opened at peak movement periods.
Important opportunities would arise from the implementation of gaps as a management tool. Since technical solutions for an automated surveillance of wildlife exist [e.g., semi-automated species identification of camera trap data: Janzen et al., 2017], and even individuals of certain species can be identified (e.g., zebras: Gosling et al., 2019), populations could be monitored at the gaps in fences. Simultaneously, the health status of individuals could be checked and, in times of disease outbreaks, gaps could be closed to restore an impermeable barrier.
A major challenge thereby is the placement of gaps. Clearly, the better they are located on natural movement corridors, the less animals will be restricted. Our data shows that gaps created by elephants were intensively used, especially on either ephemeral rivers or where the fence cuts off larger patches of plant communities. This implies that elephants break fences on important locations naturally; we see these gaps as ‘animal-informed movement corridors’ which likely form a solid baseline for placement decisions.
Conclusion
High resolution tracking, accelerometer and satellite imagery data enabled us to uncover new insights into ungulate-fence interactions and connected consequences. Along the 70 km section of the VCF between ENP and EH, we documented 1,471 crossings of springbok, kudu and eland (17 individuals in total) during the 27 month period. These were of a total of 2905 fence interactions (at the VCF and other fences) mostly resulting in augmented behavior by the study animals, which was often related to an increase in energy expenditure when encountering a fence. Particularly, non-sedentary eland and springbok were mainly affected by the VCF, while sedentary kudu were rarely affected. Fence interactions showed seasonal dynamics, peaking right before first seasonal rains. Gaps within the VCF allowed individuals to cross in order to track scattered resources on vast areas, leading to foraging benefits. This would have been impeded if the fence was an impermeable barrier.
Antelopes inhabiting semi-arid environments face a patchy distribution of resources in both time and space. Depending on foraging and movement type, they conquer this sparsity by traveling through vast areas in an adaptive, resource-tracking manner. Wildlife-proof fences stop such movements and, thereby, tremendously interfere with connected ecological processes. The latter can only be restored or sustained if fences are either permeable, e.g., by containing gaps, or are removed completely.
Our findings provide a solid base for management decisions and future research. We emphasize that research focussing on resource-tracking of ungulates in semi-arid ecosystems must use high spatial resolution satellite data of plant phenology, ideally vegetation-type specific, to account for the patchy distribution of resources and to identify small-scale reactions. Regarding the VCF's future, we see managed gaps to be a good first step toward a sustainable development of these unique, native ecosystems.
Data availability statement
The datasets presented in this study can be found in online repositories. The names of the repository/repositories and accession number(s) can be found below: https://www.movebank.org/cms/webapp?gwt_fragment=page=studies,path=study904829042. Further data for this study can be requested.
Ethics statement
The animal study was reviewed and approved by Namibia, National Commission on Research, Science and Technology.
Author contributions
RH, MH, SK-S, and NB contributed to conception and design of the study. RH and JS organized the database. RH, JS, and TS performed the statistical analysis. RH wrote the first draft of the manuscript. JS, MJ, MH, NB, and TS wrote sections of the manuscript. All authors contributed to manuscript revision, read, and approved the submitted version.
Funding
This work was part of the ORYCS-project within the SPACES II program, supported by the German Federal Ministry of Education and Research (Grant Number FKZ 01LL1804A). The publication of this work was funded by the Deutsche Forschungsgemeinschaft (DFG, German Research Foundation)—project number 491466077.
Acknowledgments
We thank André Nel and the team of Etosha Heights Private Reserve for full support during field research. We acknowledge the support of the Ministry of Environment, Tourism and Forestry, Namibia and the Namibian National Commission On Research Science & Technology, who permitted this research (certificate number RCIV00032018 with authorization numbers: 20190602, 20190808). Further, we thank the reviewers of this publication for their valuable work, which helped to improve the overall quality and Dr. McInturff who provided us with further information on his fence ecology paper.
Conflict of interest
The authors declare that the research was conducted in the absence of any commercial or financial relationships that could be construed as a potential conflict of interest.
Publisher's note
All claims expressed in this article are solely those of the authors and do not necessarily represent those of their affiliated organizations, or those of the publisher, the editors and the reviewers. Any product that may be evaluated in this article, or claim that may be made by its manufacturer, is not guaranteed or endorsed by the publisher.
Supplementary material
The Supplementary Material for this article can be found online at: https://www.frontiersin.org/articles/10.3389/fevo.2022.907079/full#supplementary-material
References
Abrahms, B., Aikens, E. O., Armstrong, J. B., Deacy, W. W., Kauffman, M. J., and Merkle, J. A. (2021). Emerging perspectives on resource tracking and animal movement ecology. Trends Ecol. Evol. 36, 308–320. doi: 10.1016/j.tree.2020.10.018
Aikens, E. O., Mysterud, A., Merkle, J. A., Cagnacci, F., Rivrud, I. M., Hebblewhite, M., et al. (2020). Wave-like patterns of plant phenology determine ungulate movement tactics. Curr. Biol. 30, 3444.e4–3449.e4. doi: 10.1016/j.cub.2020.06.032
Aquino, A. G. H. E. O., and Nkomo, S. L. (2021). Spatio-temporal patterns and consequences of road kills: a review. Animals 11, 799. doi: 10.3390/ani11030799
Archibald, S., and Scholes, R. (2007). Leaf green-up in a semi-arid African savanna -separating tree and grass responses to environmental cues. J. Veget. Sci. 18, 583–594. doi: 10.1111/j.1654-1103.2007.tb02572.x
Bakker, E. S., Gill, J. L., Johnson, C. N., Vera, F. W. M., Sandom, C. J., Asner, G. P., et al. (2016). Combining paleo-data and modern exclosure experiments to assess the impact of megafauna extinctions on woody vegetation. Proc. Natl. Acad. Sci. U.S.A. 113, 847–855. doi: 10.1073/pnas.1502545112
Bartlam-Brooks, H. L., Bonyongo, M. C., and Harris, S. (2011). Will reconnecting ecosystems allow long-distance mammal migrations to resume? A case study of a zebra Equus burchelli migration in Botswana. ORYX 45, 210–216. doi: 10.1017/S0030605310000414
Bates, D., Maechler, M., Bolker, B., and Walker, S. (2021). lme4: Linear Mixed-Effects Models using Eigen and S4. R package version 1.1-27.1.
Bigalke, R. (1972). Observations on the behaviour and feeding habits of the springbok, Antidorcas marsupialis. Zool. Afr. 7, 333–359. doi: 10.1080/00445096.1972.11447448
Bischof, R., Loe, L. E., Meisingset, E. L., Zimmermann, B., van Moorter, B., and Mysterud, A. (2012). A migratory northern ungulate in the pursuit of spring: jumping or surfing the green wave? Am. Nat. 180, 407–424. doi: 10.1086/667590
Borowik, T., Pettorelli, N., Sönnichsen, L., and Jȩdrzejewska, B. (2013). Normalized difference vegetation index (NDVI) as a predictor of forage availability for ungulates in forest and field habitats. Eur. J. Wildlife Res. 59, 675–682. doi: 10.1007/s10344-013-0720-0
Bryce, C. M., Dunford, C. E., Pagano, A. M., Wang, Y., Borg, B. L., Arthur, S. M., et al. (2022). Environmental correlates of activity and energetics in a wide-ranging social carnivore. Anim. Biotelemetry 10, 1. doi: 10.1186/s40317-021-00272-w
Calenge, C. (2006). The package adehabitat for the R software: tool for the analysis of space and habitat use by animals. Ecol. Modell. 197, 1035. doi: 10.1016/j.ecolmodel.2006.03.017
Caron, A., Miguel, E., Gomo, C., Makaya, P., Pfukenyi, D. M., Foggin, C., et al. (2013). Relationship between burden of infection in ungulate populations and wildlife/livestock interfaces. Epidemiol. Infect. 141, 1522–1535. doi: 10.1017/S0950268813000204
Dowle, M., and Srinivasan, A. (2021). data.table: Extension of 'data.frame'. R package version 1.14.10.
Dupuis-Désormeaux, M., Davidson, Z., Mwololo, M., Kisio, E., and MacDonald, S. E. (2016). Usage of specialized fence-gaps in a black rhinoceros conservancy in Kenya. Afr. J. Wildlife Res. 46, 22–32. doi: 10.3957/056.046.0022
Dupuis-Desormeaux, M., Kaaria, T. N., Mwololo, M., Davidson, Z., and MacDonald, S. E. (2018). A ghost fence-gap: Surprising wildlife usage of an obsolete fence crossing. PeerJ 2018, e5950. doi: 10.7717/peerj.5950
Durant, S. M., Becker, M. S., Creel, S., Bashir, S., Dickman, A. J., Beudels-Jamar, R. C., et al. (2015). Developing fencing policies for dryland ecosystems. J. Appl. Ecol. 52, 544–551. doi: 10.1111/1365-2664.12415
Esmaeili, S., Jesmer, B. R., Albeke, S. E., Aikens, E. O., Schoenecker, K. A., King, S. R. B., et al. (2021). Body size and digestive system shape resource selection by ungulates: a cross-taxa test of the forage maturation hypothesis. Ecol. Lett. 24, 2178–2191. doi: 10.1111/ele.13848
Ferguson, K., Adam, L., and Jori, F. (2012). “An adaptive monitoring programme for studying impacts along the Western Boundary Fence of Kruger National Park, South Africa,” in Fencing for Conservation: Restriction of Evolutionary Potential Or a Riposte to Threatening Processes?, 105–123. doi: 10.1007/978-1-4614-0902-1_7
Ferguson, K. J., Cleaveland, S., Haydon, D. T., Caron, A., Kock, R. A., Lembo, T., et al. (2013). Evaluating the potential for the environmentally sustainable control of foot and mouth disease in sub-saharan Africa. EcoHealth 10, 314–322. doi: 10.1007/s10393-013-0850-6
Found, R., and St. Clair, C. C. (2019). Influences of personality on ungulate migration and management. Front. Ecol. Evol. 7, 438. doi: 10.3389/fevo.2019.00438
Fryxell, J. M. (1991). Forage quality and aggregation by large herbivores. Am. Nat. 138, 478–498. doi: 10.1086/285227
Funk, C., Peterson, P., Landsfeld, M., Pedreros, D., Verdin, J., Shukla, S., et al. (2015). The climate hazards infrared precipitation with stations - a new environmental record for monitoring extremes. Sci. Data 2, 1–21. doi: 10.1038/sdata.2015.66
Gadd, M. E. (2012). “Barriers, the beef industry and unnatural selection: a review of the impact of veterinary fencing on mammals in Southern Africa,” in Fencing for Conservation: Restriction of Evolutionary Potential Or a Riposte to Threatening Processes? (New York, NY: Springer), 153–186. doi: 10.1007/978-1-4614-0902-1_9
Gates, C. C., Jones, P., Suitor, M., Jakes, A., Boyce, M. S., Kunkel, K., et al. (2012). “The influence of land use and fences on habitat effectiveness, movements and distribution of pronghorn in the grasslands of North America,” in Fencing for Conservation: Restriction of Evolutionary Potential Or a Riposte to Threatening Processes? (New York, NY: Springer), 277–294. doi: 10.1007/978-1-4614-0902-1_15
Geremia, C., Merkle, J. A., Eacker, D. R., Wallen, R. L., White, P. J., Hebblewhite, M., et al. (2019). Migrating bison engineer the green wave. Proc. Natl. Acad. Sci. U.S.A. 116, 25707–25713. doi: 10.1073/pnas.1913783116
Gordigiani, L., Viviano, A., Brivio, F., Grignolio, S., Lazzeri, L., Marcon, A., et al. (2022). Carried away by a moonlight shadow: activity of wild boar in relation to nocturnal light intensity. Mammal Res. 67, 39–49. doi: 10.1007/s13364-021-00610-6
Gosling, L. M., Muntifering, J., Kolberg, H., and Uiseb, K. (2019). Equus zebra ssp. hartmannae. IUCN Red List of Threatened Species. e.T7958A45171819.
Harrington, J. L., and Conover, M. R. (2006). Characteristics of ungulate behavior and mortality associated with wire fences. Wildlife Soc. Bull. 34, 1295–1305. doi: 10.2193/0091-7648(2006)34[1295:COUBAM]2.0.CO;2
Hayward, M. W. (2012). Perspectives on Fencing for Conservation Based on Four Case Studies: Marsupial Conservation in Australian Forests; Bushmeat Hunting in South Africa; Large Predator Reintroduction in South Africa; and Large Mammal Conservation in Poland, pages 7-20. Springer New York, New York, NY. doi: 10.1007/978-1-4614-0902-1_2
Hayward, M. W., and Hayward, M. D. (2012). Waterhole use by African fauna. African Journal of Wildlife Research 42, 117–127. doi: 10.3957/056.042.0209
Hempson, G. P., Archibald, S., and Bond, W. J. (2015a). A continent-wide assessment of the form and intensity of large mammal herbivory in Africa. Science 350, 1056–1061. doi: 10.1126/science.aac7978
Hempson, G. P., Archibald, S., Bond, W. J., Ellis, R. P., Grant, C. C., Kruger, F. J., et al. (2015b). Ecology of grazing lawns in Africa. Biol. Rev. 90, 979–994. doi: 10.1111/brv.12145
Hillman, J. (1988). Home range and movement of the common eland (Taurotragus oryx Pallas 1766) in Kenya. Afr. J. Ecol. 26, 135–148. doi: 10.1111/j.1365-2028.1988.tb00964.x
Hilty, J., Worboys, G. L., Keeley, A., Woodley, S., Lausche, B. J., Locke, H., et al. (2020). Guidelines for Conserving Connectivity Through Ecological Networks and Corridors. Gland: IUCN doi: 10.2305/IUCN.CH.2020.PAG.30.en
Holdo, R. M., Holt, R. D., Coughenour, M. B., and Ritchie, M. E. (2007). Plant productivity and soil nitrogen as a function of grazing, migration and fire in an African savanna. J. Ecol. 95, 115–128. doi: 10.1111/j.1365-2745.2006.01192.x
Hyndman, R. J., and Khandakar, Y. (2008). Automatic time series forecasting: the forecast package for R. J. Stat. Softw. 26, 1–22. doi: 10.18637/jss.v027.i03
Ibrahim, S., Kaduk, J., Tansey, K., Balzter, H., and Lawal, U. M. (2021). Detecting phenological changes in plant functional types over West African savannah dominated landscape. Int. J. Remote Sens. 42, 567–594. doi: 10.1080/01431161.2020.1811914
Iturbide, M., Gutiérrez, J. M., Alves, L. M., Bedia, J., Cerezo-Mota, R., Cimadevilla, E., et al. (2020). An update of IPCC climate reference regions for subcontinental analysis of climate model data: definition and aggregated datasets. Earth Syst. Sci. Data 12, 2959–2970. doi: 10.5194/essd-12-2959-2020
Jakes, A. F., Jones, P. F., Paige, L. C., Seidler, R. G., and Huijser, M. P. (2018). A fence runs through it: a call for greater attention to the influence of fences on wildlife and ecosystems. Biol. Conserv. 227, 310–318. doi: 10.1016/j.biocon.2018.09.026
Janzen, M., Visser, K., Visscher, D., MacLeod, I., Vujnovic, D., and Vujnovic, K. (2017). Semi-automated camera trap image processing for the detection of ungulate fence crossing events. Environ. Monitor. Assess. 189, 1–13. doi: 10.1007/s10661-017-6206-x
Jones, P. F., Jakes, A. F., Telander, A. C., Sawyer, H., Martin, B. H., and Hebblewhite, M. (2019). Fences reduce habitat for a partially migratory ungulate in the northern sagebrush steppe. Ecosphere 10, e02782. doi: 10.1002/ecs2.2782
Kauffman, M. J., Aikens, E. O., Esmaeili, S., Kaczensky, P., Middleton, A., Monteith, K. L., et al. (2021a). Causes, consequences, and conservation of ungulate migration. Annu. Rev. Ecol. Evol. Syst. 52, 453–478. doi: 10.1146/annurev-ecolsys-012021-011516
Kauffman, M. J., Cagnacci, F., Chamaillé-Jammes, S., Hebblewhite, M., Hopcraft, J. G. C., Merkle, J. A., et al. (2021b). Mapping out a future for ungulate migrations. Science 372, 566–569. doi: 10.1126/science.abf0998
Kesch, K. M., Bauer, D. T., and Loveridge, A. J. (2014). Undermining game fences: who is digging holes in Kalahari sands? Afr. J. Ecol. 52, 144–150. doi: 10.1111/aje.12096
Kesch, M. K., Bauer, D. T., and Loveridge, A. J. (2015). Break on through to the other side: the effectiveness of game fencing to mitigate human-wildlife conflict. Afr. J. Wildlife Res. 45, 76–87. doi: 10.3957/056.045.0109
Laguna, E., Barasona, J. A., Carpio, A. J., Vicente, J., and Acevedo, P. (2022). Permeability of artificial barriers (fences) for wild boar (Sus scrofa) in Mediterranean mixed landscapes. Pest Manage. Sci. 78, 2277–2286. doi: 10.1002/ps.6853
Laskin, D. N., Watt, D., Whittington, J., and Heuer, K. (2020). Designing a fence that enables free passage of wildlife while containing reintroduced bison: a multispecies evaluation. Wildlife Biol. 2020, 1–14. doi: 10.2981/wlb.00751
le Roux, C., Grunow, J., Bredenkamp, G., Morris, J., and Scheepers, J. (1988). A classification of the vegetation of the Etosha National Park. South Afr. J. Bot. 54, 1–10. doi: 10.1016/S0254-6299(16)31355-2
Lindsey, P. A. (2011). An Analysis of Game Meat Production in Namibia: Linkages with Food Security. Harare: TRAFFIC East/Southern Africa.
Linnell, J. D., Trouwborst, A., Boitani, L., Kaczensky, P., Huber, D., Reljic, S., et al. (2016). Border security fencing and wildlife: the end of the transboundary paradigm in Eurasia? PLoS Biol. 14, e1002483. doi: 10.1371/journal.pbio.1002483
Mair, P., and Wilcox, R. (2020). Robust statistical methods in R using the WRS2 package. Behav. Res. Methods 52, 464–488. doi: 10.3758/s13428-019-01246-w
Makhado, R. A., Potgieter, M. J., and Luus-Powell, W. J. (2016). Nutritional value of colophospermum mopane as source of browse and its chemical defences against browsers: a review. J. Anim. Plant Sci. 26, 569–576. Available online at: https://ssrn.com/abstract=2793169
Malhi, Y., Doughty, C. E., Galetti, M., Smith, F. A., Svenning, J. C., and Terborgh, J. W. (2016). Megafauna and ecosystem function from the Pleistocene to the Anthropocene. Proc. Natl. Acad. Sci. U.S.A. 113, 838–846. doi: 10.1073/pnas.1502540113
Marius, L. N., Shipandeni, M. N., Rodríguez-Campos, L. A., Osafo, E. L., Mpofu, I. D., Ansah, T., et al. (2021). Seasonal variation in chemical composition and in-vitro gas production of woody plant species of semi-arid condition of Namibia. Agroforest. Syst. 95, 1191–1204. doi: 10.1007/s10457-021-00643-5
Martin, R. B. (2005). “The influence of veterinary control fences on certain wild large mammal species in the Caprivi, Namibia,” in Occasional Papers of the IUCN Species Survival Commission (Gland; Cambridge).
Mbaiwa, B. Y. J. E., and Mbaiwa, O. I. (2006). The effects of veterinary fences on wildlife populations in Okavango Delta, Botswana. Int. J. Wilderness 12, 17–24.
McInturff, A., Xu, W., Wilkinson, C. E., Dejid, N., and Brashares, J. S. (2020). Fence ecology: frameworks for understanding the ecological effects of fences. Bioscience 70, 971–985. doi: 10.1093/biosci/biaa103
McKay, T. L., Pigeon, K. E., Larsen, T. A., and Finnegan, L. A. (2021). Close encounters of the fatal kind: landscape features associated with central mountain caribou mortalities. Ecol. Evol. 11, 2234–2248. doi: 10.1002/ece3.7190
McKillop, I. G., and Sibly, R. M. (1988). Animal behaviour at electric fences and the implications for management. Mammal Rev. 18, 91–103. doi: 10.1111/j.1365-2907.1988.tb00078.x
Mogotsi, K., Kgosikoma, O. E., and Lubinda, K. F. (2016). Wildlife-livestock interface, veterinary cordon fence damage, lack of protection zones, livestock theft and owner apathy: Complex socio-ecological dynamics in Foot and Mouth disease control in southern Africa. Pastoralism 6, 21. doi: 10.1186/s13570-016-0068-7
Murtagh, F., and Contreras, P. (2012). Algorithms for hierarchical clustering: an overview. Wiley Interdiscipl. Rev. Data Mining Know. Discov. 2, 86–97. doi: 10.1002/widm.53
Nandintsetseg, D., Bracis, C., Olson, K. A., Böhning-Gaese, K., Calabrese, J. M., Chimeddorj, B., et al. (2019). Challenges in the conservation of wide-ranging nomadic species. J. Appl. Ecol. 56, 1916–1926. doi: 10.1111/1365-2664.13380
Nghiyalwa, H. S., Urban, M., Baade, J., Smit, I. P., Ramoelo, A., Mogonong, B., et al. (2021). Spatio-temporal mixed pixel analysis of savanna ecosystems: a review. Remote Sens. 13, 3870. doi: 10.3390/rs13193870
Noy-Meir, I. (1973). Desert ecosystems: environment and producers. Annu. Rev. Ecol. Syst. 4, 25–51. doi: 10.1146/annurev.es.04.110173.000325
Owen-Smith, N. (1985). The ecological potential of the kudu for commercial production in Savanna regions. J. Grassland Soc. Southern Afr. 2, 7–10. doi: 10.1080/02566702.1985.9648005
Owen-Smith, N., Hopcraft, G., Morrison, T., Chamaillé-Jammes, S., Hetem, R., Bennitt, E., et al. (2020). Movement ecology of large herbivores in African savannas: current knowledge and gaps. Mammal Rev. 50, 252–266. doi: 10.1111/mam.12193
Park, H. B., Woo, D., Choi, T. Y., and Hong, S. (2021). Assessment of the behavioural response of Korean water deer (Hydropotes inermis argyropus) to different fence heights. Animals 11, 938. doi: 10.3390/ani11040938
Pebesma, E., and Bivand, R. (2021). sp: Classes and Methods for Spatial Data. R package version 1.4–5.
Peters, W., Hebblewhite, M., Mysterud, A., Eacker, D., Hewison, A. J. M., Linnell, J. D. C., et al. (2019). Large herbivore migration plasticity along environmental gradients in Europe: life-history traits modulate forage effects. Oikos 128, 416–429. doi: 10.1111/oik.05588
Pirie, T. J., Thomas, R. L., and Fellowes, M. D. (2017). Game fence presence and permeability influences the local movement and distribution of south African mammals. Afr. Zool. 52, 217–227. doi: 10.1080/15627020.2017.1410074
Qasem, L., Cardew, A., Wilson, A., Griffiths, I., Halsey, L. G., Shepard, E. L., et al. (2012). Tri-axial dynamic acceleration as a proxy for animal energy expenditure; should we be summing values or calculating the vector? PLoS ONE 7, e31187. doi: 10.1371/journal.pone.0031187
Qi, J., Chehbouni, A., Huete, A. R., Kerr, Y. H., and Sorooshian, S. (1994). A modified soil adjusted vegetation index. Remote Sens. Environ. 48, 119–126. doi: 10.1016/0034-4257(94)90134-1
R Core Team (2021). R: A Language and Environment for Statistical Computing. R Foundation for Statistical Computing, Vienna.
Rheinwalt, A., Goswami, B., Boers, N., Heitzig, J., Marwan, N, Krishnan, R., et al. (2015). “Teleconnections in climate networks: a network-of-networks approach to investigate the influence of sea surface temperature variability on monsoon systems,” in Machine Learning and Data Mining Approaches to Climate Science, eds V. Lakshmanan, E. Gilleland, A. McGovern, and M. Tingley (Cham: Springer), 23–33. doi: 10.1007/978-3-319-17220-0_3
Robb, B. S., Merkle, J. A., Sawyer, H., Beck, J. L., and Kauffman, M. J. (2022). Nowhere to run: semi-permeable barriers affect pronghorn space use. J. Wildlife Manage. 86, e22212. doi: 10.1002/jwmg.22212
Roche, C. (2005). Springbok… drink the rain's blood': indigenous knowledge and its use in environmental history-the case of the /Xam and an understanding of springbok treks *. South Afr. Hist. J. 53, 1–22. doi: 10.1080/02582470509464887
Roche, C. (2008). ‘The fertile brain and inventive power of man’: anthropogenic factors in the cessation of springbok treks and the disruption of the Karoo ecosystem, 1865–1908. Africa 78, 157–188. doi: 10.3366/E0001972008000120
Safner, T., Gracanin, A., Gligora, I., Pokorny, B., Flajšman, K., Apollonio, M., et al. (2021). State border fences as a threat to habitat connectivity: a case study from south-eastern Europe. Sumarski List 145, 269–278. doi: 10.31298/sl.145.5-6.6
Safriel, U., Adeel, Z., Niemeijer, D., Puigdefabregas, J., White, R., Lal, R., et al. (2005). “Dryland systems,” in Ecosystems and Human Well-being: Current State and Trends.: Findings of the Condition and Trends Working Group, eds R. Hassan, R. Scholes, and N. Ash (Washington, DC: Island Press), 623–662.
Sawyer, H., Kauffman, M. J., Middleton, A. D., Morrison, T. A., Nielson, R. M., and Wyckoff, T. B. (2013). A framework for understanding semi-permeable barrier effects on migratory ungulates. J. Appl. Ecol. 50, 68–78. doi: 10.1111/1365-2664.12013
Schroeder, N. M., Matteucci, S. D., Moreno, P. G., Gregorio, P., Ovejero, R., Taraborelli, P., et al. (2014). Spatial and seasonal dynamic of abundance and distribution of guanaco and livestock: insights from using density surface and null models. PLoS ONE 9, e85960. doi: 10.1371/journal.pone.0085960
Scoones, I., Bishi, A., Mapitse, N., Moerane, R., Penrith, M. L., Sibanda, R., et al. (2010). Foot-and-mouth disease and market access: challenges for the beef industry in southern Africa. Pastoralism 1, 135–164. doi: 10.3362/2041-7136.2010.010
Segar, J., and Keane, A. (2020). Species and demographic responses to wildlife-friendly fencing on ungulate crossing success and behavior. Conserv. Sci. Pract. 2, e285. doi: 10.1111/csp2.285
Seigle-Ferrand, J., Marchand, P., Morellet, N., Gaillard, J., Hewison, A., Saïd, S., et al. (2021). On this side of the fence: functional responses to linear landscape features shape the home range of large herbivores. J. Anim. Ecol. 91, 443–457. doi: 10.1111/1365-2656.13633
Skinner, G., and Louw, J. (1996). The springbok (Antidorcas marsupialis)—ecology and physiology. Transvaal Musuem Monogr. 10, 19–35.
Skinner, J. D., and Chimimba, C. T. (2005). The Mammals of the Southern African Sub-Region. Cambridge: Cambridge University Press. doi: 10.1017/CBO9781107340992
Smith, T., Bookhagen, B., and Rheinwalt, A. (2017). Spatiotemporal patterns of High Mountain Asia's snowmelt season identified with an automated snowmelt detection algorithm, 1987-2016. Cryosphere 11, 2329–2343. doi: 10.5194/tc-11-2329-2017
Stoffel, M. A., Nakagawa, S., and Schielzeth, H. (2020). partr2: Partitioning r2 in generalized linear mixed models. bioRxiv. doi: 10.1101/2020.07.26.221168
Sutmoller, P. (2002). The fencing issue relative to the control of foot-and-mouth disease. Ann. N. Y. Acad. Sci. 969, 191–200. doi: 10.1111/j.1749-6632.2002.tb04377.x
Tinley, K. L. (1971). The case for saving Etosha. Afr. Wild Life 25, 1–16. doi: 10.1111/j.1468-0483.1971.tb00784.x
Tucker, M. A., Böhning-Gaese, K., Fagan, W. F., Fryxell, J. M., Moorter, B. V., Alberts, S. C., et al. (2018). Moving in the Anthropocene: global reductions in terrestrial mammalian movements. Science 359, 466–469. doi: 10.1126/science.aam9712
Turpie, A. J., Midgley, G., Brown, C., Barnes, J., Pallett, J., Desmet, P., et al. (2010). Climate Change Vulnerability and Adaptation Assessment for Namibia ' s Biodiversity and Protected. Ministry of Environment and Tourism, Directorate of Parks & Wildlife Management.
van Dyk, G., and Slotow, R. (2003). The effects of fences and lions on the ecology of African wild dogs reintroduced to Pilanesberg National Park, South Africa. Afr. Zool. 38, 79–94. doi: 10.1080/15627020.2003.11657196
van Klink, R., van der Plas, F., van Noordwijk, C. G., Wallisdevries, M. F., and Olff, H. (2015). Effects of large herbivores on grassland arthropod diversity. Biol. Rev. 90, 347–366. doi: 10.1111/brv.12113
Vanak, A. T., Thaker, M., and Slotow, R. (2010). Do fences create an edge-effect on the movement patterns of a highly mobile mega-herbivore? Biol. Conserv. 143, 2631–2637. doi: 10.1016/j.biocon.2010.07.005
Weaver, G. V., Domenech, J., Thiermann, A. R., and Karesh, W. B. (2013). Foot and mouth disease: a look from the wild side. J. Wildlife Dis. 49, 759–785. doi: 10.7589/2012-11-276
Wickham, H., Francois, R., Henry, L., and Muller, K. (2021). dplyr: A Grammar of Data Manipulation. R package version 1.0.7.
Wilkinson, C. E., McInturff, A., Kelly, M., and Brashares, J. S. (2021). Quantifying wildlife responses to conservation fencing in East Africa. Biol. Conserv. 256, 109071. doi: 10.1016/j.biocon.2021.109071
Xu, W., Dejid, N., Herrmann, V., Sawyer, H., and Middleton, A. D. (2021). Barrier Behaviour Analysis (BaBA) reveals extensive effects of fencing on wide-ranging ungulates. J. Appl. Ecol. 58, 690–698. doi: 10.1111/1365-2664.13806
Keywords: veterinary cordon fence, ungulate, fence ecology, resource-tracking, energy expenditure, accelerometer, GPS, wildlife and habitat management
Citation: Hering R, Hauptfleisch M, Jago M, Smith T, Kramer-Schadt S, Stiegler J and Blaum N (2022) Don't stop me now: Managed fence gaps could allow migratory ungulates to track dynamic resources and reduce fence related energy loss. Front. Ecol. Evol. 10:907079. doi: 10.3389/fevo.2022.907079
Received: 29 March 2022; Accepted: 28 June 2022;
Published: 18 July 2022.
Edited by:
Perry S. Barboza, Texas A&M University, United StatesReviewed by:
Christine Wilkinson, University of California, Berkeley, United StatesAlex Mcınturff, University of Washington, United States
Copyright © 2022 Hering, Hauptfleisch, Jago, Smith, Kramer-Schadt, Stiegler and Blaum. This is an open-access article distributed under the terms of the Creative Commons Attribution License (CC BY). The use, distribution or reproduction in other forums is permitted, provided the original author(s) and the copyright owner(s) are credited and that the original publication in this journal is cited, in accordance with accepted academic practice. No use, distribution or reproduction is permitted which does not comply with these terms.
*Correspondence: Robert Hering, cm9iZXJ0LmhlcmluZ0B1bmktcG90c2RhbS5kZQ==