- 1Institute of Geographic Sciences and Natural Resources Research, Chinese Academy of Sciences, Beijing, China
- 2Key Laboratory of Land Surface Pattern and Simulation, Chinese Academy of Sciences, Beijing, China
- 3University of Chinese Academy of Sciences, Beijing, China
- 4School of Soil and Water Conservation, Beijing Forestry University, Beijing, China
Water scarcity is a core issue that constraints the high-quality development of arid areas in northwestern China. Zhangye is an oasis city located in the Heihe River Basin in northwestern China. It is populated with an agriculture-dominated economy and faces more and more serious water crises. Virtual water is an indicator that can measure the embodied water in the traded products, which has been widely applied for making rational policies for water resources management. In addition, clarifying water requirements in agricultural sectors under future climate change scenarios is essential to develop more appropriate adaptation strategies. From this perspective, this study aims to evaluate and compare virtual water flows among various sectors in Zhangye for the years 2012 and 2017 with a single regional input-output model and to further clarify the future water requirement tendency in agriculture during 2020–2050 under different shared socioeconomic pathways and representative concentration pathways (SSP-RCP) scenarios. The results showed that the planting sector directly contributed most of the total water consumption with the highest direct coefficient of 3307.5 m3/yuan in 2012, whereas the manufacture of food products and tobacco processing sector had the largest proportion of indirect water consumption (99%) mainly from intermediate inputs of agricultural products. Water consumption intensity of all sectors on average decreased by 22% during 2012–2017, indicating an increasing water utilization efficiency in economic industries. Household consumption also can improve water utilization efficiency as the major pathway for final consumption (86.4% in 2017). Water scarcity in Zhangye was becoming increasingly prominent since virtual water net exports were higher than local consumption, especially in the agriculture, manufacturing, and energy supply industries. Moreover, under climate change scenarios, we found the highest level of water requirement per unit area occurred in 2000, but it still had an incremental potential by 2050, especially in SSP585. The high requirement intensity and large-scale maize planting caused a rising tendency of total crop water requirement with an annual increasing rate of 8.4% from 1980 to 2050. This makes it possible to adapt to climate change through scientific management measures and technical means. We further made policy implications for adaptive management of water resources in Zhangye.
Introduction
Water scarcity is a severe challenge to sustainable development worldwide. Water supply is becoming increasingly limited, especially in developing countries, due to the uncertainty of global climate change, the continuous rise in population and food demand, and a shift in consumption patterns toward more water-intensive products (Qasemipour and Abbasi, 2019; Arunrat et al., 2022). In northwestern China, which is mostly dominated by arid areas, water scarcity has been seriously exacerbated by the imbalance of water availability and water demand during rapid industrialization and urbanization (Zhang and Anadon, 2014; Zheng et al., 2020). High-quality development in arid areas largely depends on the water availability of inland rivers and effective management measures (Deng and Zhao, 2015; Jin et al., 2018; Wang and Wei, 2019; Wang H. et al., 2022). Arid areas are particularly sensitive to climate change, which causes ecological deterioration, frequent drought events, and water yield changes (Gain and Wada, 2014; Wu et al., 2017). Illustrating the dual impacts of climate change and socioeconomic development on water supply demand patterns is important for realizing adaptive management of water resources. Virtual water theory is widely applied in the research of water resources management by revealing the occupancy of production and domestic water in the socioeconomic system (Shi and Zhan, 2015; Zhang et al., 2019). However, characterizing the current water consumption and its future tendency under global climate change from the perspective of virtual water remains a major challenge. It plays a significant role in promoting sustainable water resources management and regional high-quality development.
The conception of virtual water was first proposed by Allan (1998), which refers to the amount of water resources during the production of products and services. Virtual water is different from physical water and exists “invisibly.” In the early twentieth century, virtual water was applied to conduct a virtual water strategy in northwest arid areas of China. Water resources are the material basis for survival. Physical water is distributed unevenly and is difficult to transport. Therefore, transferring water resources within industrial sectors and trade among regions in the form of virtual water are the fundamental patterns of the water cycle in socioeconomic systems (Liu et al., 2020). Research applying virtual water theory has gradually extended from food circulation and food security strategy in international trade to sectoral connection and regional trade (Dong et al., 2014; Zhang et al., 2017; Zhang et al., 2019). Virtual water theory has provided a promising solution to alleviate the uneven distribution of water resources by sectoral transfer and regional trade of water resources.
Quantifying virtual water mainly includes two methods, namely, (1) classifying products and (2) then calculating the consumption of virtual water based on the production tree (Chapagain et al., 2006). As it is difficult to quantify water consumption of industrial products in a complex process and its intermediate consumption and final consumption cannot be distinguished (Zhang et al., 2019), this method is merely applied to virtual water measurement of primary agricultural products (such as crops and livestock) at the micro level. Another method is an input-output (IO) analysis. An IO table can describe both the input and output relationship of industrial sectors and the distribution of final products in detail. An IO table with water accounts embedded helps to clarify virtual water flows among various sectors, products, and services from the entire national economic system (Qasemipour et al., 2020; Xu and Li, 2020). For example, Zhao et al. (2010) analyzed the virtual water trade in Heihe River Basin (HRB) based on the IO analysis. Feng et al. (2014) specifically clarified the water footprint of major industries in the upper, middle, and lower reaches of the Yellow River Basin with a multiregional input-output model (MRIO). Du et al. (2021) combined the MRIO model and complex network theory to identify the structure of provincial virtual water trade. Generally, the IO analysis is applied to the research on industrial transformation and resources allocation at the macroeconomic level. However, it cannot describe production details due to data limits. Recently, an integrated method has been popularized by adding satellite accounts with detailed sectoral information to the IO model (Wu et al., 2017; Zhang et al., 2017), which is significant for improving the data availability and promoting research at a finer scale.
Previous studies have extended virtual water theory based on calculating virtual water and analyzing trade flows. Some studies focused on the evolution trends of water utilization and the patterns of regional virtual water trade (Dong et al., 2014; Zhang et al., 2016). Some studies also investigated driving factors of virtual water flows and their resource and environment effects, then analyzed tradeoffs of development in the socioeconomic system and environmental system by connecting with land use and carbon footprint (Zheng et al., 2019; Wang et al., 2021). Furthermore, sustainable management schemes of water resources were discussed in the field of water scarcity, utilization efficiency, and regional industrial advantages (Li et al., 2019; Wang and Wei, 2019; Islam et al., 2021). Previous studies have found that there is a significant correlation between virtual water trade and regional water scarcity. Virtual water flows increasingly from water-scarce developing regions to water-rich developed regions (Deng et al., 2021; Zhai et al., 2021). This phenomenon is detrimental to the decoupling development of economic growth and resource utilization. Sandström et al. (2018) considered that reducing virtual water imports by replacing imported crops with local crop production contributes to balancing water resources in water-scarce countries and constructing global sustainable food systems. However, few studies have investigated water scarcity based on the comprehensive perspective of sectoral water allocation and virtual water trade to clarify management strategies of water-intensive industries in water-scarce regions.
In addition, clarifying how climate change will affect crop water requirements during agricultural production is important to help alleviate water scarcity. Crop water requirement was mostly analyzed and used for estimation of water footprint and virtual water content through the CROPWAT model (Deng et al., 2020; Qasemipour et al., 2020; Long et al., 2021). Nevertheless, with the continuous warming of the global climate, increasing variability in climatic factors, such as changes in temperature and precipitation, will significantly affect water consumption in the food production system and bring more challenges to water management (Bocchiola et al., 2013; Arunrat et al., 2020). Especially, understanding crop water requirement changes under future climate change at the local scale is essential to adaptive water management strategies in the region where agricultural production depends on both rainfall (green water) and irrigation water (blue water) (Rosenzweig et al., 2014; Arunrat et al., 2022). Currently, a number of studies have been conducted to analyze water footprints and virtual water content of crops under the combined shared socioeconomic pathways (SSPs) and representative concentration pathways (RCPs) scenarios (Wada and Bierkens, 2014; Arunrat et al., 2022; Li et al., 2022). However, regarding the variations in water requirement of crop production in climate change scenarios, the results differ or contradict each other (Zhuo et al., 2016). Therefore, evaluating crop water requirements under local climate change conditions is becoming necessary. Zhangye City in the HRB is a typical water-scarce region in northwestern China. Scholars have fully been discussing the supply and demand of water resources and their utilization strategies in the HRB (Wu et al., 2017; Zhang et al., 2017; Wang and Wei, 2019). However, it is still blank to identify water consumption and its response mechanism under the complex systematic changes caused by future climate. This is vitally beneficial for predicting future water utilization and flexibly adjusting management schemes of water resources.
Taking adaptive management of water resources to alleviate water supply demand pressure is essential in water-scarce regions. In this study, Zhangye City in the HRB was selected as a case area. The objectives were to analyze water consumption and virtual water flows among regional trade and sectoral transfer in Zhangye using an IO model and further specifically clarify crop water requirement in agricultural sectors using the CROPWAT model and its changing tendency in the future under the SSP-RCP scenarios. Finally, we proposed policy implications for adaptive management of water resources in Zhangye City from the perspectives of industrial transformation, efficiency improvement, and virtual water trade.
Materials and Methods
Study Area
The HRB is the second largest inland river basin in China (Figure 1A). The water supply of the HRB mainly comes from atmospheric precipitation and meltwater in Qilian Mountain. Zhangye City is located in the northeast of Gansu Province. It is a typical large city located in the upper and middle reaches of the HRB (Figure 1B). It covers an area of 42,000 km2, between latitude 37°28’N to 39°57’N and longitude 97°20’E to 102°12’E. Zhangye administratively covers Ganzhou, Linze, Minle, Shandan, Gaotai, and Su’nan counties (Figure 1C). The topography of Zhangye slopes from the northeast to the southwest, with the elevation ranging from 1,144 to 5,583 m (Figure 1C). Qilian Mountain stands along the northwest-southeast boundary of Zhangye with an average elevation of 3,200 m. In the middle reaches, Hexi Corridor plain is the biggest oasis in the HRB, covering 38% of the total area in Zhangye. Desert grassland and Gobi Desert cover the north of the city. Therefore, Zhangye has enjoyed the unique landform of deserts and oasis. It has an arid and semiarid climate. The high temperature and more than 80% of the precipitation are mainly concentrated from May to September. With the elevation decreasing, the annual average precipitation gradually decreases from 450 to 100 mm, whereas the annual average temperature increases from –16.3 to 8.9°C from southeast to northwest (Figure 1B). The plain in the middle usually has the highest temperature and the most abundant precipitation.
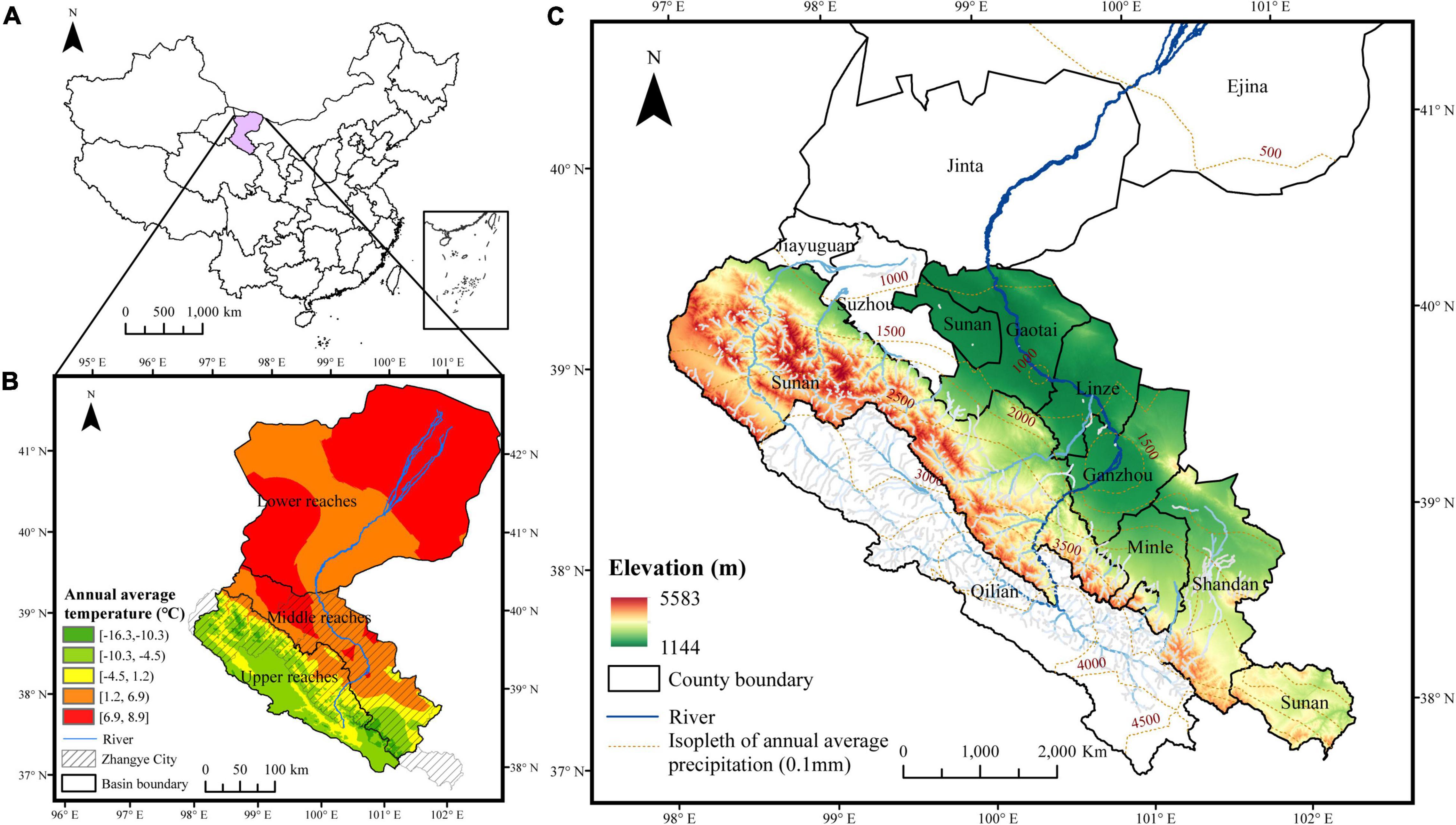
Figure 1. Geographical location of Zhangye in the HRB, northwestern China. (A) The map of China. (B) The location of the Heihe River Basin. (C) The location of Zhangye city.
In the middle reaches, the vast Hexi Corridor plain is a major agricultural area in the HRB, benefiting from flat terrain, rich light and heat resources, and the large range of temperatures between day and night. Agricultural irrigation accounts for 88% of the total water consumption in Zhangye. Water scarcity is the key factor restricting agricultural production. However, unreasonable industrial structure and increasing domestic demand have further exacerbated the contradiction of water supply and demand, as well as a series of ecological and environmental problems such as desertification and salinization. Thus, alleviating the contradiction of water supply and demand in the view of virtual water under climate change can benefit regional high-quality development.
Data
Economic Data to Construct the Input-Output Table
The 2012 IO table of Zhangye was collected from the data prepared by Wu et al. (2017), based on which the 2017 IO table was further compiled through a non-survey approach. It consists of 39 sectors, including agriculture, mining, manufacturing, construction, and service industries, and their subordinate sectors. The classification of sectors depends on National Economical Industry Classification (GB/T 4754-2017) and data availability. There are two types of data required for implementing the IO model, including the economic production and consumption data and the water consumption statistics. Data on total output, added value, and final consumption at county-level sectors were collected and calculated from statistical yearbooks. Water consumption data in Zhangye were derived from the water resources bulletin. Among each sector, data for the agriculture and construction industry were collected directly, whereas data for other industries were calculated based on water consumption per unit of GDP, industrial output value, and water consumption amounts at the national level and city level. Then, we corrected data through the total water consumption of industries and tested the data using sectoral water quota and product outputs in Zhangye. An intermediate input matrix was obtained using the RAS technique to adjust the bilateral proportion of intermediate inputs and intermediate outputs, as well as to calculate several iterations with the constraints of row and column sums. We finally obtained the IO tables with water accounts embedded in Zhangye in 2012 and 2017.
Climate Data
Historical climate data (1980–2020) were obtained from the National Meteorological Information Center.1 It provides a daily dataset of surface climate data (V3.0), including maximum temperature, minimum temperature, average relative humidity, average wind speed, and sunshine hours. Future climate data were from the Coupled Model Intercomparison Project (CMIP6) multimode climate scenario data.2 The Sixth Assessment Report of the Intergovernmental Panel on Climate Change has adopted a new set of emission scenarios developed using SSPs (Pedersen et al., 2021). SSPs include five scenarios (SSP1: sustainability; SSP2: middle of the road; SSP3: regional rivalry; SSP4: inequality; and SSP5: fossil fuel development), which represent different combinations of mitigation and adaptation challenges and a set of qualitative storylines and quantitative development indicators, including population, economy, education, city, and technology (O’Neill et al., 2017; Huang et al., 2019). The CMIP has conducted remarkable work in designing climate model standards and developing shared climate simulation data to support new scientific issues of climate change. The new phase CMIP6 proposed a new projection scenario, which combines different SSPs and RCPs, which makes future scenarios more reasonable (Eyring et al., 2016; Simpkins, 2017). Climate models are key tools to simulate climate change scenarios. The CMIP6 has provided various outputs of multi-series climate models, where ESM and Earth models have been confirmed to have significant improvement in simulation performance for extreme temperature and precipitation in arid and semiarid areas (Karim et al., 2020; Lim Kam Sian et al., 2021; Wang L. et al., 2022). In this study, we selected data for the period 2020–2050 from four core scenarios integrated by MPI-ESM1-2-HR and EC-Earth3-Veg models (SSP126: the green road with low radiation forcing; SSP245: the middle road with moderate radiation forcing; SSP370: the rocky road with moderate radiation forcing; and SSP585: the highway with high radiation forcing). By using climate output data from these scenarios to adjust the parameters of the Penman-Monteith combination equation, we predicted the trend of agricultural crop water requirement in Zhangye under different scenarios in the future.
Methods
The Input-Output Model of Water Consumption
The IO model forms a matrix to describe the quantitative relationship of input and output in various economic sectors across time. Recently, the IO model with water accounts embedded (Figure 2) has been widely applied in research on water resources management because of its advantages in structured data and unique correlation analysis. Calculating the sectoral water consumption relies on several key coefficients. The direct water consumption coefficient represents the amount of physical water consumed by sectoral per unit output value during production. Apart from the direct water consumption, intermediate products and services provided by other sectors also consume water invisibly. It is regarded as indirect water and reflected by the indirect water consumption coefficient. The sum of the direct water consumption coefficient and indirect water consumption coefficient is equal to the total water input coefficient. It refers to the total amount of water consumption to provide a final product of sectoral per unit output value.
In Eq. 1, dj is the direct water consumption coefficient of sector j, Wj is the direct water consumption of sector j, Xj is the total output value of sector j, and n represents the number of sectors. In Eq. 2, direct water consumption coefficients of all sectors constitute the row vector d, and tandk represent the row vectors of total water input coefficients and indirect water consumption coefficients, respectively. In Eqs 3 and 4, I is an identity matrix of order n. aij is the technical coefficient, referring to the product’s value provided by sector i when producing per unit output value in sector j. xij is the product’s value in sector j provided by sector i. Technical coefficients of all sectors constitute the direct IO coefficients matrix A. (I−A)−1 is known as the Leontief inverse matrix. It links direct water consumption coefficients d and total water input coefficients t, reflecting the relationship between the direct and total input when considering the intermediate inputs from other sectors. In Eq. 5, the row vector t of total water input coefficients was multiplied by the column vector Y of final consumption in sectoral products. On the right side of Eq. 5, the total water consumption W in the macro national economic system includes water consumption from the household, government, capital, import, and export. In Eqs 6 and 7, we obtained the total water consumption matrix VW by multiplying the row vector t and the intermediate IO matrix among sectors. We further established the sectoral transfer matrix TVW of virtual water by subtracting matrix VW from its transpose matrix.
CROPWAT Model for Crop Water Requirement Calculation
CROPWAT model is an approach to calculate crop evapotranspiration using crop coefficients and meteorological and soil data to obtain crop water requirements. Agriculture is the largest sector of water consumption in Zhangye. Thus, we assumed non-agricultural sectors are not sensitive to climate change and only investigated the impact of climate change on the water consumption in agricultural sectors to simplify our calculation. As the water content of crops contains only 0.1--1% of evapotranspiration, the accumulative evapotranspiration during the growth period is approximately equal to the crop water requirement. We used CROPWAT 8.0 developed by Joss Swennenhuis for the Water Resources Development and Management Service of FAO3 to calculate the crop water requirement of two typical crops (wheat and maize) in Zhangye. The calculations were as follows:
In Eq. 8, we defined the crop water requirement as ETc. It is the accumulation of daily crop water requirements, namely, ETc,d, during the entire growth period from the initial stage to the end of the late-season stage. In Eq. 9, ETc,d was calculated by the reference evapotranspiration ET0,d and the crop coefficient Kc. The reference evapotranspiration ET0,d, also named the reference crop water requirement, was calculated by the Penman-Monteith combination method recommended by FAO. The equation of the Penman-Monteith combination is shown in Eq. 10, where Rn is the net radiation at the crop surface (MJ⋅m–2⋅day–1), G is the soil heat flux density (MJ⋅m–2⋅day–1), T is daily air temperature (°C), u2 is the daily wind speed at 2 m height (m/s), esandea represent saturation vapor pressure and actual vapor pressure (kPa), respectively, △ is the slope curve of vapor pressure (kPa/°C), and γ is the psychrometric constant (kPa/°C).
The crop coefficient Kc incorporates the effects of crop characteristics and soil evaporation to reflect the difference in crop water requirement influenced by crop types, planting areas, and soil and climate conditions. Kc coefficients differ in different growth stages, including the initial stage (Kcini), the mid-season stage (Kcmid), and the end of the late-season stage (Kcend). FAO has provided stages length of various planting periods and reference Kc coefficients of various crops globally under non-stressed, well-managed, and subhumid climates (RHmin45%,u22m/s), as well as adjustment methods of crop coefficients in different growth stages.
In Eqs 11 and 12, Kcmid,Kcend were adjusted by reference coefficients Kcmid(Tab),Kcend(Tab). No adjustment was required when Kcend(Tab)0.45. Kcini was determined comprehensively by the magnitude and time interval of wetting events, soil texture, and reference evapotranspiration. RHmin is daily minimum relative humidity (%), u2 is the daily wind speed at 2 m height (m/s), and h is plant height during the growth stage (m). In Eq. 13, e0(Tdew)ande0(Tmax) represent the saturation vapor pressure under mean dewpoint temperature and mean daily maximum air temperature, respectively. In arid and semiarid climates, Tdew can be replaced by subtracting 2°C from the average value of Tmin.
Based on this, we got Kc coefficients of wheat and maize in Zhangye in different growth stages. We showed growth stages length and Kc coefficients curves of wheat and maize in Zhangye (Table 1 and Figure 3). We also obtained the accumulative crop water requirement per unit area during the entire growth period, ETc(m3/hm2). In Eq. 14, we further calculated the total crop water requirement, namely, the virtual water content of the crop, VWCc(m3) by combining with yield per unit area Yc and crop yield Ac.
Results
Water Consumption of Different Industrial Sectors
By analyzing the composition of water consumption coefficients of each industrial sector calculated by the IO model, we found the structure and intensity of water consumption when providing sectoral final products (Table 2). Planting was the largest water consumption sector, whose direct consumption accounted for the most. The direct water consumption coefficient (d) of the planting sector was 3,307.47 m3/yuan in 2012 and 2,311.78 m3/yuan in 2017, more than 100 times higher than that of other sectors. Furthermore, the direct consumption coefficients (d) were relatively higher in sectors, including non-metallic minerals mining and dressing, chemical industry, and electricity and heat production and supply, which were regarded as direct water consumption sectors along with agriculture. In contrast, coal mining and dressing sector sectors in the manufacturing industry (other than the chemical industry and petroleum and coking processing sector), and all sectors in construction and service industries were all indirect water consumption-dominated sectors. Specifically, the service industry rarely consumed water directly. The direct consumption coefficients (d) were less than 1 m3/yuan in 2012 in service sectors, including finance, real estate, telecommunication, computer and software services, and education, health, cultural, and sporting services. The manufacture of food products and tobacco processing sector was the second-largest water consumption sector after agriculture. It had the highest indirect water consumption coefficient (k) among all sectors with 527.31 m3/yuan in 2012 and 472.25 m3/yuan in 2017, accounting for approximately 99% of its total water input coefficient. Besides, the indirect consumption coefficients (k) were generally higher than the direct consumption coefficients (d) in the construction and service sectors, and coal mining and dressing sectors, accounting for 93.5, 80, and 86% of their total water input coefficients (t), respectively. These sectors consumed water mainly from intermediate inputs of products and services and were regarded as indirect water consumption sectors.
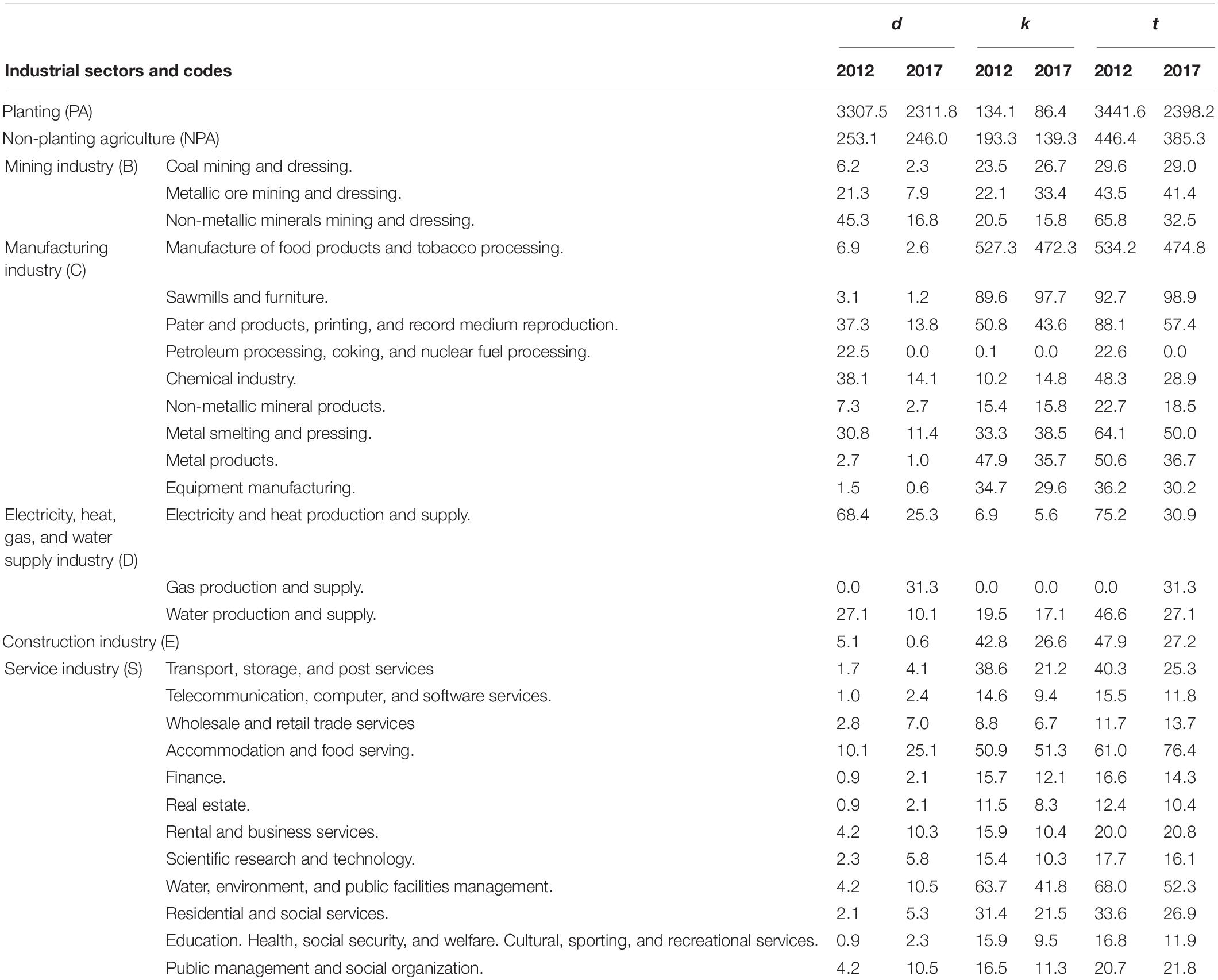
Table 2. Water consumption coefficients of Zhangye industrial sectors in 2012 and 2017 (unit: m3/yuan).
Water consumption intensity in all sectors averagely decreased by 22% in 2017 compared with 2012 (Table 2). Specifically, the total water input coefficient (t) of the electricity and heat production and supply sector decreased the most by 58.9%, followed by the non-metallic minerals mining and dressing sector by 50.5%. As the largest water consumption sector, the total water input coefficient (t) of planting decreased by 30.3%. Conversely, the direct consumption coefficients (d) of the service industry increased significantly by 1.5 times, and water consumption intensity in wholesale and retail trade services and accommodation and food-serving services increased by 17.6 and 25.2%, respectively. Although total water consumption intensity decreased, some indirect water consumption coefficients (k) still increased in sectors, including the chemical industry, coal mining and dressing, metallic ore mining and dressing, and metal smelting and pressing. These sectors alleviated their own water stress by increasing intermediate inputs from other sectors. Our results showed that water utilization efficiency in most of the sectors in Zhangye has increased. However, direct water consumption intensity in the service industry was increasing with its gradual increasing proportion in economic industries, which has restricted the water utilization efficiency in the service industry.
Analysis of water resources consumption from the viewpoint of the consumer side showed that household consumption was the major pathway for final consumption in Zhangye (Figure 4). Households accounted for 80.6 and 86.4% of total final consumption in 2012 and 2017, respectively, and consumed the total water from planting, mining, and electricity, heat, gas, and water supply industries. The proportion of urban and rural household consumption in final consumption changed from 5:3 in 2012 to 4:5 in 2017 with the rapid urbanization. Therefore, the structure of water consumption from households converted from rural dominant to urban dominant. Water consumption from the capital was dominant in the construction industry, accounting for 98% of sectoral output. Additionally, water consumption from the capital was also distributed in non-planting agriculture, manufacturing (such as general and special-purpose machinery), and service (such as telecommunication, computer, and software services) industries. The government consumed water (59%) mostly in the non-planting agricultural sector. It was also the major pathway for final consumption in public utility sectors, including public management and social organization, transport, storage and post services, and education, health, cultural, and sporting services. However, the proportion of water consumption by the government decreased from 5% in 2012 to 2% in 2017, which was occupied to the increasing household consumption.
Virtual Water Trade and Sectoral Transfer
Regional trade of virtual water in Zhangye was generally in the direction of net exports (Figure 5). We calculated the proportion of net flows to local water consumption to represent the intensity of the virtual water trade. Virtual water net-export sectors accounted for most of the economic industries of Zhangye. Specifically, the intensity of virtual water net exports was greater than 1 in agriculture, manufacturing, and electricity, heat, gas, and water supply industries. Virtual water net exports were higher than local consumption in these industries due to a large amount of water consumption and commodity exports, especially in the manufacture of food products and the tobacco processing sector. However, virtual water trade in coal mining and dressing, and most manufacturing sectors like non-metallic mineral products sector was in the direction of virtual water net imports with high external dependence on water utilization. From 2012 to 2017, the virtual water net import intensity in the mining industry (especially coal mining and dressing sector) has increased by 4.9 times with a higher external dependence. Moreover, the intensity of virtual water net exports in most sectors has declined except for service and electricity, heat, gas, and water supply industries. The direction of virtual water trade has even changed from net imports to net exports in service sectors, including finance, accommodation, and food-serving services, scientific research and technical services, and education, health, cultural, and sporting services. This indicates the increasing output value and export advantages in the service industry. Although the tendency of virtual water net exports in Zhangye has been alleviated over the past 5 years, it was still much higher than local consumption. Virtual water outflows hidden in products and services will continue to exacerbate water scarcity in Zhangye.
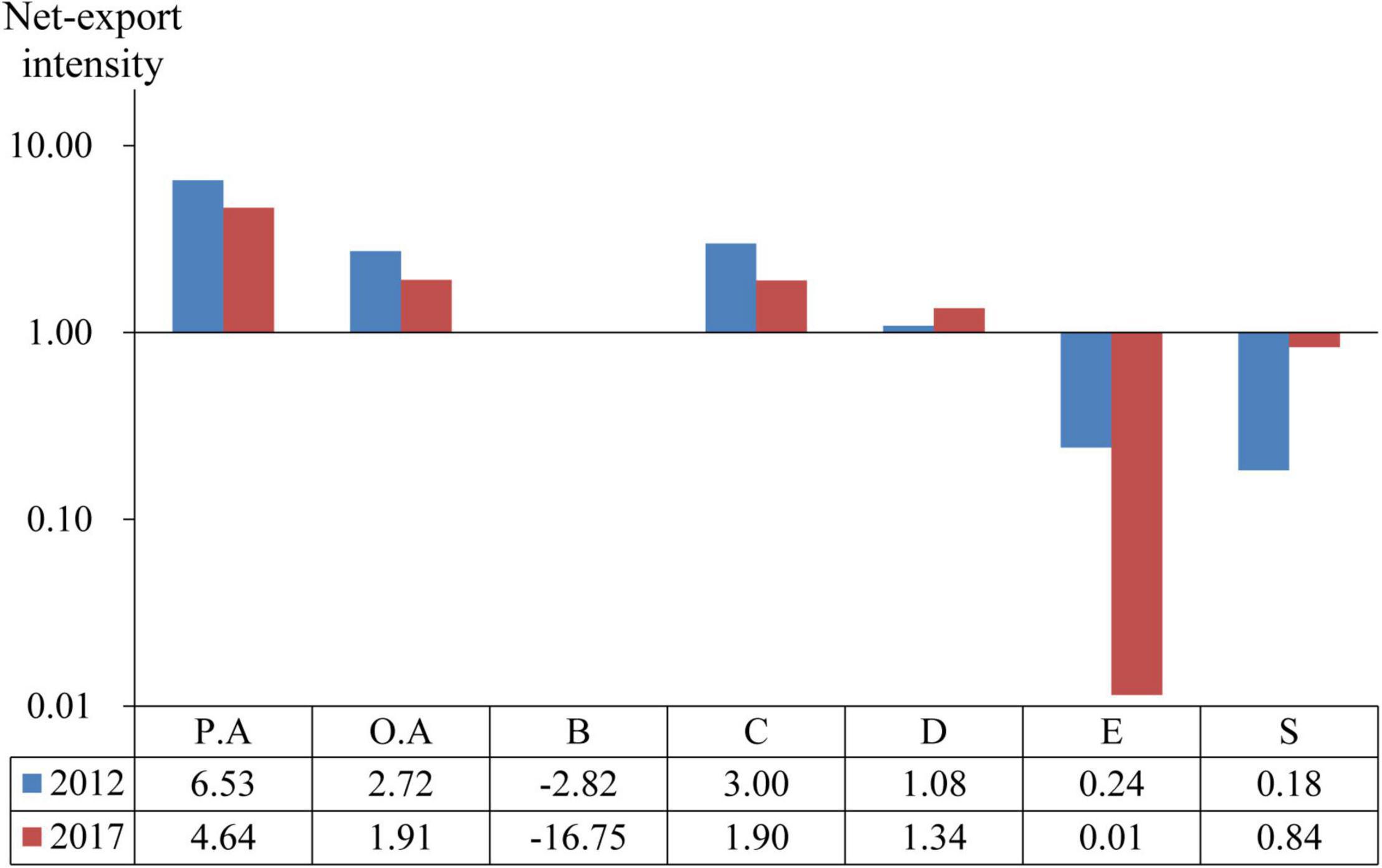
Figure 5. Net export intensity of virtual water of different sectors in Zhangye in 2012 and 2017. Positive value represents net exports, while negative value represents net imports.
Some basic sectors have provided virtual water through intermediate inputs of products and services to support economic industries. We analyzed virtual water transfer among different industrial sectors in Zhangye based on the sectoral transfer matrix (Table 3). Virtual water net output sectors were commonly basic for social development, especially in agriculture, mining, and electricity, heat, gas, and water supply industries, whereas others were net input sectors. Virtual water transfer between agriculture and manufacturing industries was the largest (5 × 108 m3) in 2017, which was 91.8% of the total net transfer due to plenty of intermediate inputs from agriculture to the manufacture of food products and the tobacco processing sector. Another major source of virtual water transfer was the electricity, heat, gas, and water supply industry, where 50.2% of water output was provided to the service industry in 2017. Additionally, the mining industry has exported virtual water to manufacturing and construction industries and received it from the service industry through infrastructure services and financing and technical support. However, since construction and service were indirect water consumption industries, they received virtual water from various sectors and depended on more intermediate products and services of manufacturing (62.9%) and agriculture (60.0%) industries, respectively.
The Trend of Crop Water Requirement Under SSP-RCP Scenarios
Crop water requirement per unit area (ETc) of wheat and maize had an incremental potential under the foreseeable climate change scenarios (Figure 6). In the historical years, ETwheat and ETmaize increased from 1980 to 2000, then decreased from 2000 to 2020. The peak of ETwheat and ETmaize were 5.3 and 3.6% higher than that in 1980, respectively. ETmaize was approximately 1.5 times that of ETwheat with a widening gap. In the following three decades, compared with the historical average level in recent 40 years, ETwheat and ETmaize would decrease by nearly 5% with the impacts of climate change under SSP-RCP scenarios. However, ETwheat and ETmaize would still show an increasing trend from 2020 to 2050. According to four SSP-RCP scenarios, crop water requirement per unit area varied from high to low and appeared in the scenarios of SSP585 > SSP245 > SSP126 > SSP370. This showed that crop water requirement per unit area would be higher under higher radiation forcing and rapid socioeconomic scenarios. Specifically, ETwheat and ETmaize were expected to reach the highest and increase rapidly under the SSP585 scenario. The results indicated that the water requirement intensity of crops would increase after 2020 as the extreme climate events occurred frequently and temperature changed substantially under high fossil-fueled development and radiation forcing level.
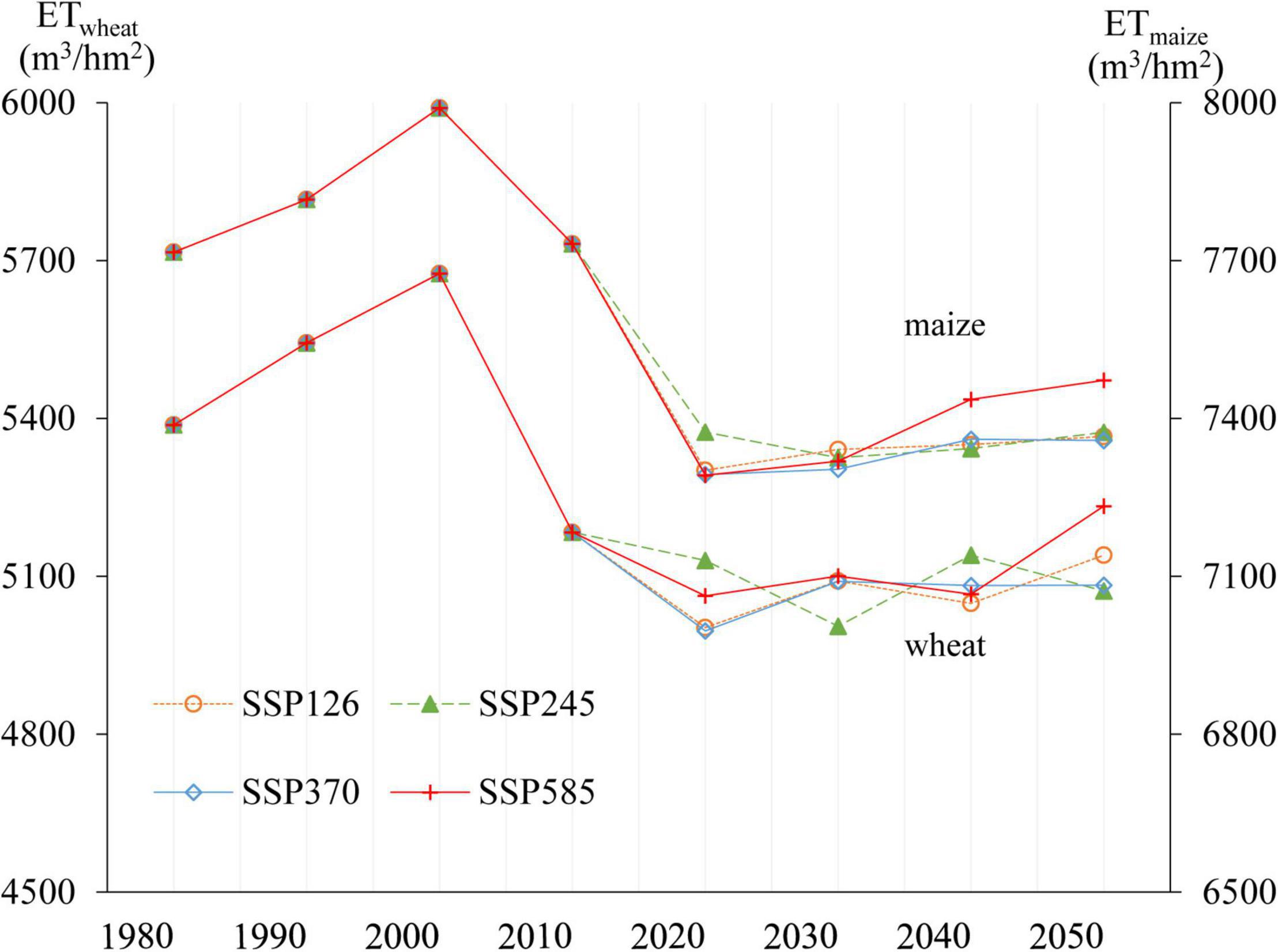
Figure 6. Crop water requirement per unit area (ETc) of wheat and maize in Zhangye under different SSP-RCP scenarios during 1980–2050.
The total crop water requirement or virtual water of crops (VWCc) under the SSP-RCP scenarios was further calculated by time-series forecasted crop yields and water requirement per unit area. It showed that VWCc was increasing with an annual rate of 8.4% from 1980 to 2050 dominated by maize water consumption (Figure 7). The trend of virtual water content varied similarly under four scenarios. Taking SSP126 scenario as an example, though the crop water requirement per unit area was highest in 2000, the total virtual water content of maize and wheat was the lowest at the same time, then gradually increased since 2000. Specifically, VWCwheat and VWCmaize in Zhangye increased by nearly 70% during 1980–2020. As the planting proportion of wheat and maize changed from 117:27 to 61:156 during the past four decades, VWCwheat decreased while VWCmaize increased with the adjusted planting areas and yield changes. VWCmaize in 2020 had reached 4.3 times that in 1980. During 2020–2050, VWCwheat would continue to decrease by 0.61%, whereas VWCwheat would increase by 0.96% annually. Under predictable climate change in the future, total virtual water content of crops would constantly rise dominated by maize water consumption with an average annual rate of 6.3%, which varies slower than the historical period, but 99.7% higher than that in the year 1980.
Our results showed that crop water requirement in agricultural sectors represented by maize and wheat had been increasing over the past four decades because of cultivation structure adjustment and high intensity of water requirement of maize. According to Zhang et al. (2017), water consumption for maize and wheat planting sectors accounted for more than 60% of total agricultural water consumption of Zhangye in 2012. Therefore, the large-scale maize planting has caused large-scale irrigation water consumption. This kind of agricultural planting pattern with large water consumption made water scarcity of Zhangye increasingly prominent. Although crop water requirement per unit area was predicted lower than historical level, total virtual water content was higher, indicating that long-term climate change makes it possible to increase agricultural irrigation water consumption. If the current agricultural planting pattern with large water consumption continues for long, climate change and large-scale maize planting will exacerbate the risk of water utilization in the future.
Conclusion and Discussion
Global warming has exacerbated the uncertainty of frequent extreme climate events and seriously threatened sustainable water utilization. The irrational water utilization structure of the socioeconomic system in Zhangye made local water scarcity prominent. In this study, we applied the IO model to analyze water consumption and virtual water linkages among different economic sectors and used CROPWAT model to estimate future crop water requirement under different SSP-RCP scenarios for an agriculture-dominated region Zhangye. Based on the IO analysis, we found that planting sector was the largest water consumption sector dominated by direct consumption in all economic industries of Zhangye. Others consumed water mainly from intermediate products and services, especially in sectors including manufacture of food products and tobacco processing, coal mining and dressing, construction, and service. Water consumption intensity in most sectors decreased from 2012 to 2017 except service industry restricted by its higher direct water consumption. On the side of final consumption, changing household behavior of water consumption was essential to improve water utilization efficiency. Economic industries of Zhangye obviously relied on sectors with higher outputs of virtual water, including agriculture, mining, and electricity, heat, gas, and water supply. Nevertheless, the amount of virtual water net exports was much higher than that of local consumption. By using CROPWAT model combined with future climate data, we found that water requirement per unit area of maize and wheat reached the highest in 2000 and would increase after 2020, especially in SSP585 scenario. Under long-term climate change scenarios, the high intensity of water requirement and large-scale planting of maize would increase crop water requirement in agricultural sectors.
Our study extends the previous study that water intensity and final consumption are major factors of virtual water transfer in China (Wang et al., 2021). Specifically, the result of sectoral water consumption intensity decreasing during 2012–2017 indicates the increasing water utilization efficiency in economic industries. This was mainly driven by the effective water-saving measures in agriculture, electricity production and supply, and non-metallic minerals mining and dressing sector. Recently, it can be inferred that the total amount control of water resources and the constraints from industrial water quota of Zhangye have played an important role in improving sectoral water management. As for final consumption, household consumption is the major pathway for final consumption; thus, household behavior of saving water can effectively guide production and business activities. We also consider that both high intensity of virtual water net exports and the increasing tendency of agricultural water consumption will exacerbate water scarcity of Zhangye currently and even in the future, and are detrimental to tackle with climate risks. This finding is consistent with the existing view that water resources distribute unevenly with virtual water outflows more in water-scarce region (Deng et al., 2021; Zhai et al., 2021). Such water consumption structure is abnormal and needs to be improved through regional trade. Additionally, we calculated virtual water content of maize and wheat based on CROPWAT model, which was 32.5% lower than that of the total water consumption in maize and wheat planting sectors by using MRIO model (Zhang et al., 2017). This confirms that the result of virtual water content is reasonable to reflect water consumption of the two agricultural subsectors to a certain extent. Crop water requirement per unit area depends on regional climatic conditions and crop varieties; moreover, virtual water content of crops is also affected by agricultural material inputs, irrigation intensity, planting arrangement, and cropland management measures. Our analysis showed that under SSP-RCP scenarios crop water requirement per unit area would be lower than historical level, but virtual water content of crops would be higher. This indicates that scientific management measures and technical means are expected to reduce virtual water content effectively and benefit for climate change adaptation in agricultural sectors.
We further propose several implications for water resources adaptive management of Zhangye. Our findings revealed different characteristics of water consumption in various industries, which is helpful to make differentiated strategies for industrial structure transformation. Zhangye is an agriculture-dominated city; thus, agriculture has great water-saving potential and should take more responsibility. This can reduce both the highest amount of direct water consumption in agriculture and indirect water consumption in manufacture of food products and tobacco processing sector. Specifically, the planting proportion of maize and other high-water-consumptive crops should be appropriately reduced through cultivation structure adjustment. Agricultural irrigation efficiency can be promoted by improving irrigation and water conservancy and applying water-saving technologies. In 2017, water-saving irrigation area of Zhangye was 1,873 km2, reaching the highest level in Gansu Province. Micro-irrigation and low-pressure pipe irrigation have been used to increase water-saving irrigation area by 59.5%, but spray irrigation, drip irrigation, and canal anti-seepage have not been widely applied, which are expected to reduce per unit irrigation area by nearly 30% referring to the existing water-saving irrigation demonstration. Then, water resources saved in agriculture can be reasonably used in the secondary and tertiary industries through water rights trading. For example, saved water in agricultural irrigation can be replaced for industrial production. Additionally, an extended producer responsibility guides producers to select upstream raw materials with lower water consumption and environmental pollution as intermediate inputs. Then, excessive water consumption of intermediate products can be avoided that was provided by basic industries like agriculture, and electricity, heat, gas, and water supply. As for the development in mining and most manufacturing sectors, it will not exacerbate local water consumption directly due to their net import direction of virtual water trade. In spite of this, they should focus more on reducing water environment pollution and discharge during production activities. Construction and service industries have lower water consumption but smaller scale in economic industries of Zhangye. Our analysis showed an incremental developing tendency in service industry that was reflected by the rising intensity of water consumption and virtual water net exports. Service industry should further develop toward the structure of resource saving and technical innovation by improving production technology and management modes.
Zhangye has met unprecedented opportunities and developing moments from the belt and road to make better use of both domestic and international markets and resources. Our analysis of virtual water trade indicated that adopting virtual water strategy rationally is important to transform the unbalanced situation of increasing exports of virtual water flow and local water scarcity. Therefore, taking measures to build a sustainable virtual water trade pattern will help alleviate the contradiction of local water supply and demand. Specifically, in the economic industries of Zhangye, most sectors export more virtual water than they import, especially in agriculture, manufacturing, and electricity, heat, gas, and water supply industries. It is obvious that most of the products produced in these sectors are water intensive. In the view of regional trade, on the one hand, the pattern of virtual water trade can be adjusted by reducing exports and increasing imports of water-intensive products like crops, processed food, and energy supply. On the other hand, while optimizing industrial structure to develop the scale of secondary and tertiary industries, international market should also be broadened to expand the exported competitive advantage of service industry with high economic added value. Moreover, considering that most economic industries consumed water indirectly, the industrial demand for water-intensive products and services can be transferred outside the region by promoting imports of intermediate products and services, including crops and energy supply. As the first pilot of water-saving cities in China, Zhangye has conducted active exploration in water resources allocation, water rights trading, and water saving and intensive utilization. However, facing the increasingly local water scarcity and serious threat from climate change, adaptive water resources management is necessary to change the focus of water management from resources themselves to adaptability improvement of social and economic systems (Jin et al., 2020). Our implications are expected to promote optimal allocation of water resources and contribute to the high-quality development of the water-saving city Zhangye. Although our analysis improved the understanding of virtual water consumption and transfer among sectors and future crop water requirement changes in Zhangye, there are several limitations that require future research. One of the limitations in our study is that specific direction of virtual water trade in Zhangye has not been described due to the lack of universal methods and comprehensive data for MRIO tables at city level. Using gravity models combined with field survey data to reveal internal trade relationships of virtual water may help improve our research (Zheng et al., 2021). Another limitation is that for future virtual water estimation under different climate change scenarios we only consider the agricultural sectors. However, climate change is a complex system that affects more than just water utilization for agriculture. In spite of this, our findings of agricultural crop water requirement can nevertheless be extended to water consumption tendency in the socioeconomic system as agriculture is the largest water consumption sector. Consequently, our findings recommend that effective measures can help alleviate the increasing water scarcity and realize adaptive management of water resources in Zhangye. It further motivates research on response mechanism of water utilization and management to future climate change. Our research provides significant reference for sustainable utilization of water resources and high-quality development of Zhangye under the background of climate change mitigation and adaptation.
Data Availability Statement
The raw data supporting the conclusions of this article will be made available by the authors, without undue reservation.
Author Contributions
ZL and YW contributed to conception and design of the study. YW organized the database and wrote the first draft of the manuscript. ZL and HW performed the analysis and wrote sections of the manuscript. All authors contributed to manuscript revision, read, and approved the submitted version.
Funding
This research was financially supported by the Young Scientists Fund of the National Natural Science Foundation of China (Grant No. 71804175), the NSFC-Yunnan Joint Fund Key Support Project (Grant No. U2102208), and the Major Program of the National Natural Science Foundation of China (Grant No. 41890824).
Conflict of Interest
The authors declare that the research was conducted in the absence of any commercial or financial relationships that could be construed as a potential conflict of interest.
Publisher’s Note
All claims expressed in this article are solely those of the authors and do not necessarily represent those of their affiliated organizations, or those of the publisher, the editors and the reviewers. Any product that may be evaluated in this article, or claim that may be made by its manufacturer, is not guaranteed or endorsed by the publisher.
Footnotes
- ^ http://data.cma.cn/
- ^ https://esgf-node.llnl.gov/projects/cmip6/
- ^ https://www.fao.org/land-water/databases-and-software/cropwat/
References
Allan, J. A. (1998). Virtual water: a strategic resource global solutions to regional deficits. Groundwater 36, 545–546. doi: 10.1111/j.1745-6584.1998.tb02825.x
Arunrat, N., Pumijumnong, N., Sereenonchai, S., Chareonwong, U., and Wang, C. (2020). Assessment of climate change impact on rice yield and water footprint of large-scale and individual farming in Thailand. Sci. Total Environ. 726:137864. doi: 10.1016/j.scitotenv.2020.137864
Arunrat, N., Sereenonchai, S., Chaowiwat, W., and Wang, C. (2022). Climate change impact on major crop yield and water footprint under CMIP6 climate projections in repeated drought and flood areas in Thailand. Sci. Total Environ. 807:150741. doi: 10.1016/j.scitotenv.2021.150741
Bocchiola, D., Nana, E., and Soncini, A. (2013). Impact of climate change scenarios on crop yield and water footprint of maize in the Po valley of Italy. Agric. Water Manage. 116, 50–61. doi: 10.1016/j.agwat.2012.10.009
Chapagain, A. K., Hoekstra, A. Y., and Savenije, H. H. (2006). Water saving through international trade of agricultural products. Hydrol. Earth Syst. Sci. 10, 455–468. doi: 10.5194/hess-10-455-2006
Deng, C., Zhang, G., Li, Z., and Li, K. (2020). Interprovincial food trade and water resources conservation in China. Sci. Total Environ. 737:139651. doi: 10.1016/j.scitotenv.2020.139651
Deng, J., Li, C., Wang, L., Yu, S., Zhang, X., and Wang, Z. (2021). The impact of water scarcity on Chinese inter-provincial virtual water trade. Sustain. Prod. Consum. 28, 1699–1707. doi: 10.1016/j.spc.2021.09.006
Deng, X., and Zhao, C. (2015). Identification of water scarcity and providing solutions for adapting to climate changes in the Heihe River Basin of China. Adv. Meteorol. 2015:279173. doi: 10.1155/2015/279173
Dong, H., Geng, Y., Fujita, T., Fujii, M., Hao, D., and Yu, X. (2014). Uncovering regional disparity of China’s water footprint and inter-provincial virtual water flows. Sci. Total Environ. 500, 120–130. doi: 10.1016/j.scitotenv.2014.08.094
Du, R., Zheng, X., Tian, L., Liu, K., Qian, L., Wu, Q., et al. (2021). A study on drivers of water consumption in China from a complex network perspective. Front. Phys. 9:769420. doi: 10.3389/fphy.2021.769420
Eyring, V., Bony, S., Meehl, G. A., Senior, C. A., Stevens, B., Stouffer, R. J., et al. (2016). Overview of the Coupled Model Intercomparison Project Phase 6 (CMIP6) experimental design and organization. Geosci. Model Dev. 9, 1937–1958. doi: 10.5194/gmd-9-1937-2016
Feng, K., Hubacek, K., Pfister, S., Yu, Y., and Sun, L. (2014). Virtual scarce water in China. Environ. Sci. Technol. 48, 7704–7713. doi: 10.1021/es500502q
Gain, A. K., and Wada, Y. (2014). Assessment of future water scarcity at different spatial and temporal scales of the Brahmaputra River Basin. Water Resour. Manage. 28, 999–1012. doi: 10.1007/s11269-014-0530-5
Huang, J., Qin, D., Jiang, T., Wang, Y., Feng, Z., Zhai, J., et al. (2019). Effect of fertility policy changes on the population structure and economy of China: from the perspective of the shared socioeconomic pathways. Earth Future 7, 250–265. doi: 10.1029/2018EF000964
Islam, K. N., Kenway, S. J., Renouf, M. A., Wiedmann, T., and Lam, K. L. (2021). A multi-regional input-output analysis of direct and virtual urban water flows to reduce city water footprints in Australia. Sust. Cities Soc. 75:103236. doi: 10.1016/j.scs.2021.103236
Jin, G., Deng, X., Zhao, X., Guo, B., and Yang, J. (2018). Spatiotemporal patterns in urbanization efficiency within the Yangtze River Economic Belt between 2005 and 2014. J. Geogr. Sci. 28, 1113–1126. doi: 10.1007/s11442-018-1545-2
Jin, G., Shi, X., Zhang, L., and Hu, S. (2020). Measuring the SCCs of different Chinese regions under future scenarios. Renew. Sustain. Energy Rev. 130:109949. doi: 10.1016/j.rser.2020.109949
Karim, R., Tan, G., Ayugi, B., Babaousmail, H., and Liu, F. (2020). Evaluation of historical CMIP6 model simulations of seasonal mean temperature over Pakistan during 1970–2014. Atmosphere 11:1005. doi: 10.3390/atmos11091005
Li, M., Cao, X., Liu, D., Fu, Q., Li, T., and Shang, R. (2022). Sustainable management of agricultural water and land resources under changing climate and socio-economic conditions: a multi-dimensional optimization approach. Agric. Water Manage. 259:107235. doi: 10.1016/j.agwat.2021.107235
Li, Y., Zhang, Z., and Shi, M. (2019). What should be the future industrial structure of the Beijing-Tianjin-Hebei city region under water resource constraint? An inter-city input-output analysis. J. Clean Prod. 239:118117. doi: 10.1016/j.jclepro.2019.118117
Lim Kam Sian, K. T. C., Wang, J., Ayugi, B. O., Nooni, I. K., and Ongoma, V. (2021). Multi-decadal variability and future changes in precipitation over southern africa. Atmosphere 12:742. doi: 10.3390/atmos12060742
Liu, X., Shi, L., Engel, B. A., Sun, S., Zhao, X., Wu, P., et al. (2020). New challenges of food security in Northwest China: water footprint and virtual water perspective. J. Clean Prod. 245:118939. doi: 10.1016/j.jclepro.2019.118939
Long, A., Yu, J., He, X., Deng, X., Su, S., Zhang, J., et al. (2021). Linking local water consumption in inland arid regions with imported virtual water: approaches, application and actuators. Adv. Water Resour. 151:103906. doi: 10.1016/j.advwatres.2021.103906
O’Neill, B. C., Oppenheimer, M., Warren, R., Hallegatte, S., Kopp, R. E., Pörtner, H. O., et al. (2017). IPCC reasons for concern regarding climate change risks. Nat. Clim. Change 7, 28–37. doi: 10.1038/nclimate3179
Pedersen, J. S. T., Santos, F. D., van Vuuren, D., Gupta, J., Coelho, R. E., Aparício, B. A., et al. (2021). An assessment of the performance of scenarios against historical global emissions for IPCC reports. Glob. Environ. Change 66:102199. doi: 10.1016/j.gloenvcha.2020.102199
Qasemipour, E., Abbasi, A., and Tarahomi, F. (2020). Water-saving scenarios based on input–output analysis and virtual water concept: a case in Iran. Sustainability 12:818. doi: 10.3390/su12030818
Qasemipour, E., and Abbasi, A. (2019). Virtual water flow and water footprint assessment of an arid region: a case study of South Khorasan province. Iran. Water 11:1755. doi: 10.3390/w11091755
Rosenzweig, C., Elliott, J., Deryng, D., Ruane, A. C., Müller, C., Arneth, A., et al. (2014). Assessing agricultural risks of climate change in the 21st century in a global gridded crop model intercomparison. Proc. Natl. Acad. Sci. U. S. A. 111, 3268–3273. doi: 10.1073/pnas.1222463110
Sandström, V., Lehikoinen, E., and Peltonen-Sainio, P. (2018). Replacing imports of crop based commodities by domestic production in Finland: potential to reduce virtual water imports. Front. Sustain. Food Syst. 12:67. doi: 10.3389/fsufs.2018.00067
Shi, C., and Zhan, J. (2015). An input–output table based analysis on the virtual water by sectors with the five northwest provinces in China. Phys. Chem. Earth. Parts A/B/C 79, 47–53. doi: 10.1016/j.pce.2015.03.004
Simpkins, G. (2017). Progress in climate modelling. Nat. Clim. Change 7, 684–685. doi: 10.1038/nclimate3398
Wada, Y., and Bierkens, M. F. (2014). Sustainability of global water use: past reconstruction and future projections. Environ. Res. Lett. 9:104003. doi: 10.1088/1748-9326/9/10/104003
Wang, F., Cai, B., Hu, X., Liu, Y., and Zhang, W. (2021). Exploring solutions to alleviate the regional water stress from virtual water flows in China. Sci. Total Environ. 796:148971. doi: 10.1016/j.scitotenv.2021.148971
Wang, H., Wang, W. J., Wang, L., Ma, S., Liu, Z., Zhang, W., et al. (2022). Impacts of future climate and land use/cover changes on water-related ecosystem services in Changbai mountains, Northeast China. Front. Ecol. Evol. 10:854497. doi: 10.3389/fevo.2022.854497
Wang, L., Zhang, J., Shu, Z., Wang, Y., Bao, Z., Liu, C., et al. (2022). Evaluation of the ability of CMIP6 global climate models to simulate precipitation in the Yellow River Basin China. Front. Earth Sci. 9:751974. doi: 10.3389/feart.2021.751974
Wang, S., and Wei, Y. (2019). Water resource system risk and adaptive management of the Chinese Heihe River Basin in Asian arid areas. Mitig. Adapt. Strateg. Glob Change 24, 1271–1292. doi: 10.1007/s11027-019-9839-y
Wu, F., Bai, Y., Zhang, Y., and Li, Z. (2017). Balancing water demand for the Heihe River basin in Northwest China. Phys. Chem. Earth. Parts A/B/C 101, 178–184. doi: 10.1016/j.pce.2017.07.002
Xu, M., and Li, C. (2020). Application of the Water Footprint: Water Stress Analysis and Allocation. Singapore: Springer.
Zhai, Y., Bai, Y., Shen, X., Ji, C., Zhang, T., and Hong, J. (2021). Can grain virtual water flow reduce environmental impacts? Evidence from China. J. Clean Prod. 314:127970. doi: 10.1016/j.jclepro.2021.127970
Zhang, C., and Anadon, L. D. (2014). A multi-regional input–output analysis of domestic virtual water trade and provincial water footprint in China. Ecol. Econ. 100, 159–172. doi: 10.1016/j.ecolecon.2014.02.006
Zhang, X., Liu, J., Zhao, X., Yang, H., Deng, X., Jiang, X., et al. (2019). Linking physical water consumption with virtual water consumption: methodology, application and implications. J. Clean Prod. 228, 1206–1217. doi: 10.1016/j.jclepro.2019.04.297
Zhang, Y., Zhou, Q., and Wu, F. (2017). Virtual water flows at the county level in the Heihe River Basin. China. Water 9:687. doi: 10.3390/w9090687
Zhang, Z., Yang, H., and Shi, M. (2016). Spatial and sectoral characteristics of China’s international and interregional virtual water flows–based on multi-regional input–output model. Econ. Syst. Res. 28, 362–382. doi: 10.1080/09535314.2016.1165651
Zhao, X., Yang, H., Yang, Z., Chen, B., and Qin, Y. (2010). Applying the input-output method to account for water footprint and virtual water trade in the Haihe River basin in China. Environ. Sci. Technol. 44, 9150–9156. doi: 10.1021/es100886r
Zheng, H., Többen, J., Dietzenbacher, E., Moran, D., Meng, J., Wang, D., et al. (2021). Entropy-based Chinese city-level MRIO table framework. Econ. Syst. Res. 1–26. doi: 10.1080/09535314.2021.1932764
Zheng, H., Zhang, Z., Zhang, Z., Li, X., Shan, Y., Song, M., et al. (2019). Mapping carbon and water networks in the north China urban agglomeration. One Earth 1, 126–137. doi: 10.1016/j.oneear.2019.08.015
Zheng, X., Huang, G., Liu, L., Zheng, B., and Zhang, X. (2020). A multi-source virtual water metabolism model for urban systems. J. Clean Prod. 275:124107. doi: 10.1016/j.jclepro.2020.124107
Keywords: virtual water, input-output analysis, crop water requirement, CMIP6, scenario analysis, Zhangye
Citation: Wang Y, Wu H and Li Z (2022) Assessment of Sectoral Virtual Water Flows and Future Water Requirement in Agriculture Under SSP-RCP Scenarios: Reflections for Water Resources Management in Zhangye City. Front. Ecol. Evol. 10:901873. doi: 10.3389/fevo.2022.901873
Received: 22 March 2022; Accepted: 11 April 2022;
Published: 18 May 2022.
Edited by:
Jinyan Zhan, Beijing Normal University, ChinaReviewed by:
Qing Zhou, Huazhong Agricultural University, ChinaGui Jin, China University of Geosciences Wuhan, China
Copyright © 2022 Wang, Wu and Li. This is an open-access article distributed under the terms of the Creative Commons Attribution License (CC BY). The use, distribution or reproduction in other forums is permitted, provided the original author(s) and the copyright owner(s) are credited and that the original publication in this journal is cited, in accordance with accepted academic practice. No use, distribution or reproduction is permitted which does not comply with these terms.
*Correspondence: Zhihui Li, bGl6aGlodWlAaWdzbnJyLmFjLmNu