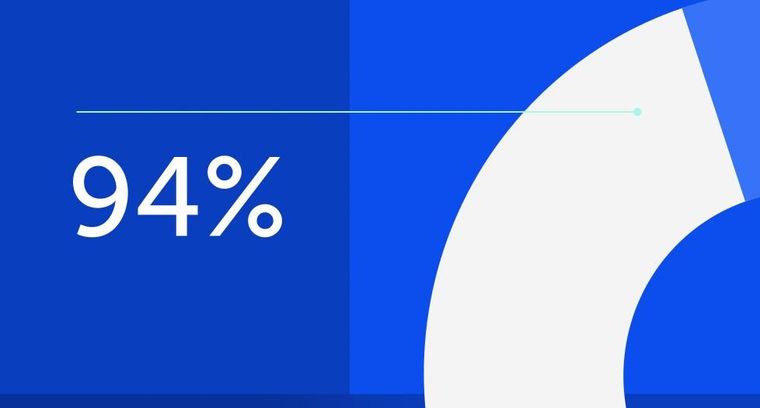
94% of researchers rate our articles as excellent or good
Learn more about the work of our research integrity team to safeguard the quality of each article we publish.
Find out more
ORIGINAL RESEARCH article
Front. Ecol. Evol., 06 May 2022
Sec. Conservation and Restoration Ecology
Volume 10 - 2022 | https://doi.org/10.3389/fevo.2022.901107
This article is part of the Research TopicBiodiversity Conservation and Ecological Function Restoration in Freshwater EcosystemsView all 16 articles
Thermokarst lakes are formed following ice-rich permafrost thaw and widely distribute in the cold regions with high latitude and elevation. However, the micro-eukaryotic communities (MECs) in thermokarst lakes are not well studied. Employing 18S rRNA gene sequencing, we assessed the biogeography of MECs and their driving factors in sediments of thermokarst lakes across the Qinghai-Tibet Plateau (QTP). Results showed that Diatom, Gastrotricha, Nematozoa, Ciliophora, and Cercozoa were dominant lineages in relative abundance and OTU richness. MECs varied substantially across the lakes in terms of diversity and composition. Structural equation modeling and mantel test showed that both OTU richness and community structure of MECs had close relationships with spatial factors, climatic factors, and sediment properties, particularly with latitude, mean annual precipitation, pH, as well as nutrient concentrations and stoichiometric ratios. Moreover, different groups of microbial eukaryotes (taxonomic groups and co-occurrence modules) responded differentially to the measured environmental variables. The results suggested that the biogeography of sediment MECs of thermokarst lakes on the QTP were jointly controlled by spatial and climatic factors as well as sediment properties. This study provides the first view of the composition, diversity, and underlying drivers of MECs dynamic in surface sediments of thermokarst lakes across the QTP.
Formed as a result of ice-rich permafrost thaw, thermokarst lakes and ponds are the most important aquatic ecosystems in the Arctic and sub-Arctic regions (Kokelj and Jorgenson, 2013; Farquharson et al., 2016; In’T Zandt et al., 2020a; Veremeeva et al., 2021) and pervasively distributed on the Qinghai-Tibet Plateau (QTP; Zou et al., 2017) In cold regions with high elevation and altitude, thermokarst lakes have been recognized as biogeochemical hotspots, particularly due to their important roles in organic carbon (OC) stock and greenhouse gases emission (Walter et al., 2006; Du Toit, 2018; In’T Zandt et al., 2020a; Jongejans et al., 2021). In circumpolar thermokarst lakes, the storage of OC is estimated to 102 Pg C (Olefeldt et al., 2016) and the methane (CH4) emission is estimated to be 4.1 ± 2.2 Tg CH4 per year (Wik et al., 2016). Over the past decades, global permafrost temperature increased by 0.3°C per decade (Biskaborn et al., 2019), leading to tremendous permafrost thaw and consequential changes of thermokarst lakes in size and abundance (Karlsson et al., 2012; Luo et al., 2015; Pastick et al., 2019), as well as serious influences on regional and global biogeochemistry (Shirokova et al., 2013; In’T Zandt et al., 2020a; Le Moigne et al., 2020). Given their crucial roles in biogeochemical processes, microbial diversity and community have been attracting increasing research interests, while most of the studies focused on bacteria and archaea, particularly on those mediating carbon dioxide (CO2) and CH4 metabolism (He et al., 2012; Matheus Carnevali et al., 2018; Vigneron et al., 2019; In’T Zandt et al., 2020b). However, the micro-eukaryotic communities in thermokarst lakes are not well known.
In lake ecosystems, microeukaryotes are a versatile group, encompassing an enormous diversity and playing fundamental roles in ecosystem structure and function (Schaechter, 2012; Keck et al., 2020). Microeukaryotes (algae, fungi, protozoa, metazoan, etc.) act as primary producers, consumers, decomposers, parasites, and saprotrophs, structuring aquatic food webs and driving biogeochemical cycles (Schaechter, 2012; Rodríguez-Martínez et al., 2020). Hence, microeukaryotes are crucial in maintaining ecosystem functions and stability. Moreover, microeukaryotes have sensitive responses to local and global environmental changes by shifting their abundance and diversity, and thus being recognized as important ecological indicators of lacustrine ecosystems (Payne, 2013; Capo et al., 2016; Keck et al., 2020; Pearman et al., 2020). Surface sediments also host a huge number and diversity of microorganisms (Lozupone and Knight, 2007; Roeske et al., 2012). Sediment microeukaryotes drive important biogeochemical processes. For example, Bacillariophyta (diatoms) contribute to primary production, and osmotrophic-saprotrophic protists are important for detrital decomposition (Rodríguez-Martínez et al., 2020). Many previous studies focused on the paleolimnology to reveal the long-term dynamics of lacustrine microeukaryotes and their reflected environmental changes by studying sediment cores (Smol, 1992; Capo et al., 2016; Keck et al., 2020). In thermokarst lakes, sediments are originated from thawing permafrost and continuously supplemented by collapse of surrounding permafrost (West and Plug, 2008). These organic-rich sediments represent an important stock of C (Strauss et al., 2013; Anthony et al., 2014) and are an active area of C metabolism (Heslop et al., 2015; Winkel et al., 2019). Aside from the studies associated with C cycling, we lack of knowledge about how micro-eukaryotic communities change across a large spatial scale and how environmental variables drive these communities.
In this study, we investigated the micro-eukaryotic communities (MECs) in sediments of thermokarst lakes across the QTP using 18S rRNA gene sequencing. Our aim was to document the diversity and structural properties of the MECs, and to address the question: how do MECs in thermokarst lake sediments respond to spatial and climatic factors as well as sediment physicochemical properties across the QTP?
This work was conducted on the QTP in July 2021. In total, 44 thermokarst lakes (TL01–TL44) were investigated spanning an extensive area with longitude (LON) from 90.6 E to 98.6 E, latitude (LAT) from 30.2 N to 35.0 N, and elevation from 3569 to 4959 m above the sea level (Figure 1). The average elevation of the study sites is 4426 m. The map of permafrost distribution (Figure 1) on the QTP (Zou et al., 2017) was downloaded from the National Tibetan Plateau Data Center. The spatial dataset of climate on the Tibetan Plateau (Zhou, 2018) was also downloaded from the National Tibetan Plateau Data Center1 and used to extract the mean annual temperature (MAT) and mean annual precipitation (MAP) of our study regions. For sediment sampling of each lake, the top 15 cm of the sediment was collected from 3 points and mixed together for the following analyses. The microbial samples were collected in a 45 mL sterile centrifuge tube and frozen in liquid nitrogen immediately in the field. In the laboratory, the sediment samples were air-dried to determine sediment organic carbon (SOC), total nitrogen (TN), and total phosphorus (TP). SOC was measured by the potassium dichromate oxidation spectrophotometric method (Chinese standard method HJ615-2011) (Wang et al., 2020; Luo et al., 2021). TN was measured using the modified Kjeldahl Method (Chinese standard method HJ717-2014) (Qian et al., 2019; Wang et al., 2020). TP was measured using the ascorbic acid colorimetric method after microwave extraction with nitric acid (Dancer et al., 1998).
Figure 1. Map of the 44 study thermokarst lakes across the Qinghai-Tibet Plateau. The distribution of the permafrost was cited from Zou et al. (2017).
DNA was extracted using the Magen Hipure Soil DNA Kit (Magen, Guangzhou, China) following the manufacture instruction. DNA extracts were quantified using a Qubit 3.0 Fluorometer (Life Technologies, Guangzhou, China). A total of 20 ng DNA was as the template for the polymerase chain reaction (PCR) to generate amplicons. The 18S rDNA hypervariable regions of V9 were amplified using forward primer 1380F (CCCTGCCHTTTGTACACAC) and reverse primer 1510R (CCTTCYGCAGGTTCACCTAC). The 25 μl PCR mixture was prepared with 2.5 μl of TransStart buffer, 2 μl of dNTPs, 1 μl of each primer, 0.5 μl of TransStart Taq DNA polymerase, and 20 ng template DNA. The PCR reaction was conducted on a thermal cycler (ABI GeneAmp® 9700, Guangzhou, China). DNA libraries were verified on 1.5% agarose gels (Biowest Agarose, Guangzhou, China) and quantified using a Qubit 3.0 Fluorometer (Life Technologies, Guangzhou, China). DNA libraries were multiplexed and loaded on an Illumina NovaSeq PE250 platform according to manufacture instructions (Illumina, San Diego, CA, United States). Raw sequence data were analyzed using QIIME 1.9.1 (Caporaso et al., 2010). Sequences were quality filtered and clustered to generate operational taxonomic units (OTUs) at a threshold of 97% similarity against the SILVA 138 database (Quast et al., 2013) using QIIME. Raw sequence data can be accessed at the China National Center for Bioinformation (PRJCA005279).
Spearman correlations were used to assess the relationships between OTU richness of the overall micro-eukaryotic communities (MECs) and major phylum (phylum with relative abundance >1%) versus environmental variables, as well as between the relative abundance of the major phylum versus environmental variables. The first structural equation modeling (SEM) analysis was conducted to depicting the relationships between environmental factors (spatial factors, climatic factors, pH, nutrient, and nutrient ratios) and community structure and alpha diversity of MECs. The second SEM was conducted to depict the relationships between environmental factors and the major modules (contain more than 10% of the nodes) of the co-occurrence network of the MECs. In SEMs, the environmental factors, MECs, and major modules were reduced in dimensions by non-metric multidimensional scaling (NMDS) using the R package vegan 2.5-7 (Oksanen et al., 2007) and the first axis of NMDS was used. SEMs were conducted using the R package lavaan 0.6-10 (Rosseel, 2012). Phylogenetic tree of microbial eukaryotes was constructed in the R package ggtree 3.2.1 (Yu et al., 2017) using OTUs which presented at least 10 lakes and had an average relative abundance >0.01%. A heatmap was built in the phylogenetic tree to show the relationships (Spearman correlation) between these OTUs and environmental factors. Mantel test was used to assess the relationships between environmental variables and the variations of MECs in terms of overall MECs, major phylum, and major network modules.
The co-occurrence network of the MECs was constructed based on the OTUs used in the phylogenetic tree using the R package igraph 1.2.11 (Csardi, 2013) and visualized using Gephi 0.9.2 (Bastian et al., 2009). The spearman correlation between all pairs of OTUs was calculated, and the P-values were adjusted using the FDR method for false discovery (Benjamini and Hochberg, 1995). Only strong and significant correlations (R > 0.5, or R < −0.5, P < 0.05) were used in constructing the network. Topological parameters were calculated, such as clustering coefficient (a measure of local connections), average degree (average number of neighbors for all nodes), and modularity (the tendency to contain subclusters of nodes). Module structures were established and the major modules were identified as those containing more than 10% of the nodes. The topological role of each node (OUT) was identified according to the within-module connectivity (Zi) and among-module connectivity (Pi). Module hubs are the highly linked nodes within the modules (Zi ≥ 2.5 and Pi < 0.62). Connectors are the linking nodes between different modules (Pi ≥ 0.62 and Zi < 2.5). Network hubs are those with Zi ≥ 2.5 and Pi ≥ 0.62. Other nodes were determined as peripherals (Zi < 2.5 and Pi < 0.62). All the analyses were carried out in R 4.1.2 (R Core Team, 2017).
Across these studied thermokarst lakes, a total of 11,499 OTUs were identified from the quality filtered sequences. The OTU richness ranged from 650 to 1734 (Figure 2) with an average value of 1130 (Figure 3A), and was positively correlated (p < 0.05) with MAP, SOC, TN, C:N, C:P, and N:P, while negatively correlated (p < 0.05) with latitude and pH (Figure 3B). The SEM result further showed that nutrient stoichiometric ratios had significantly direct effects on alpha diversity of the micro-eukaryotic communities (Figure 4). However, pH and climatic factors had significantly indirect effects on alpha diversity through the influences on nutrient concentrations and stoichiometric ratios (Figure 4).
Figure 2. Alpha diversity (OTU richness) of the microbial eukaryotes in sediments of thermokarst lakes across the Qinghai-Tibet Plateau.
Figure 3. (A) OTU richness of the overall micro-eukaryotic communities and major phylum. (B) Spearman correlations show the relationships between alpha diversity and environmental factors. The color represents the correlation coefficient, which is shown in number when the result is significant (p < 0.05).
Figure 4. Structural equation modeling analysis depicting the relationships between spatial factors (including latitude, longitude, and elevation), climatic factors (including mean annual temperature and mean annual precipitation), pH, nutrients (including sediment organic carbon, total nitrogen, and total phosphorus), nutrient ratios (including C:N, C:P, and N:P ratios), micro-eukaryotic community, and alpha diversity. Solid and dashed arrows represent the significant and non-significant relationships, respectively. Red and green arrows represent negative and positive relationships, respectively. The significant path coefficients were shown adjacent to the path with *, **, and *** denote the significant level of p < 0.05, p < 0.01, and p < 0.001, respectively.
In terms of each major phylum, Ciliophora had the highest OTU richness of 161 on average, followed by Diatom (148 OTUs on average), Cercozoa (122 OTUs on average), and others (Figure 3A). The OTU richness of Gastrotricha, Nematozoa, Annelida, Ascomycota, and Apicomplexa was significantly correlated (p < 0.05) with spatial factors (longitude, latitude, and/or elevation) (Figure 3B). The OTU richness of Diatom, Gastrotricha, Annelida, Chlorophyta, and Euglenozoa were significantly correlated (p < 0.05) with a climatic factor (MAP). In addition, the OTU richness of the most phylum was significantly correlated (p < 0.05) with sediment properties, particularly with sediment pH, SOC, TN, C:P, and N:P (Figure 3B).
The overall micro-eukaryotic communities were dominated by Diatom (16.9%), followed by Gastrotricha (11.3%), Nematozoa (11.0%), Arthropoda (9.2%), Ciliophora (8.8%), Annelida (8.2%), and Cercozoa (5.5%) (Figure 5A). The taxonomic composition was largely different between any pair of lakes, supported by the high community dissimilarities (Bray-Curtis distance), which ranged from 0.534 to 0.986. However, the relative abundance of the major phylum was rarely associated with environmental factors (Figure 5B). On the contrary, in terms of individual OTUs (OTUs with the average relative abundance >0.01% and appeared in at least 10 lakes), most of them had significant relationships with one or more environmental variables, particularly with latitude, MAP, pH, SOC, TN, C:P, and N:P (Figure 6).
Figure 5. (A) Community composition of sediment microbial eukaryotes at the phylum level. Only phyla with a relative abundance >1% are shown. “Overall” represents the whole dataset including all samples. (B) Spearman correlations show the relationships between the relative abundance of the major phylum and environmental factors. The color represents the correlation coefficient, which is shown in number when the result is significant (p < 0.05).
Figure 6. Phylogenetic tree of microbial eukaryotes was constructed by OTUs with relative abundance >0.01%. Tree tips are colored by major phylum. The red and black arrows in the inner ring represent module hubs and connectors of the co-occurrence network. The heatmap indicates the relationships between OTUs and environmental variables (the color represents Spearman’s correlation coefficient and only significant results are shown). The bars in the outer ring represent the relative abundance of the OTUs and are colored by phylum.
Mantel tests showed that the variation of micro-eukaryotic communities across the lakes was significantly associated with all the measured spatial factors (except longitude), climatic factors, and sediment properties (Table 1). The variation of the subcommunities of Diatom, Gastrotricha, Arthropoda, Ciliophora, Cercozoa, Ochrophyta, Chlorophyta, Euglenozoa, and Amoebozoa was also strongly associated with most of the environmental variables (Table 1). The fitted SEM model explained 53.5% of the variation in micro-eukaryotic communities, further suggesting that climatic factors and pH had positive and negative direct effects on MECs, respectively (Figure 4).
Table 1. Mantel tests between environmental factors and micro-eukaryotic communities (overall, dominant phyla, and network modules).
The resulting eukaryotic co-occurrence network consisted of 690 nodes and 2,909 edges (Figure 7). Only 4.8% of the edges were negative. Some topological properties were calculated to describe the complex co-occurrence relationships between OTUs. The average path length (the average shortest path lengths between all pairs of nodes) was 4.15 edges with a diameter (the maximum length) of 11 edges. The clustering coefficient was 0.33, the average degree was 8.43, and the modularity was 0.57 (>0.4 suggests modular structure of the network). The results suggested clustered topology and modular structure of the eukaryotic co-occurrence network. In this network, OTUs were clustered into four major modules containing more than 10% of the nodes (Figure 7). These modules consisted of OTUs from different taxonomic groups and responded differently to environmental variables (Figure 7). Module-A was dominated by Ciliophora, Cercozoa, Ochrophyta, and Euglenozoa in terms of OTU richness. Module-B was dominated by Diatom, Chlorophyta, and Ciliophora. Module-C was dominated by Ciliophora, Chlorophyta, and Cercozoa. Module-D was dominated by Ciliophora, Ascomycota, Ochrophyta, and Gastrotricha. Mantel tests showed that all the modules were significantly associated with latitude, MAP, and pH (Table 1). In addition, these modules were also associated differently with elevation, conductivity, SOC, TP, C:N, and C:P (Table 1). The SEM result further suggested that module-A was directly affected by nutrient stoichiometric ratios, module-B was directly affected by spatial factors and climatic factors, and module-C was directly affected by nutrient concentration (Figure 8). In the network, keystone taxa were identified according to the within-module and among-module connectivity (Zi and Pi, respectively) of the nodes. There were nine module hubs (Pi < 0.62 and Zi ≥ 2.5) and 38 connectors (Pi ≥ 0.62 and Zi < 2.5) identified in this network (Figure 7). Latitude, MAP, pH, SOC, TN, C:P, and N:P had strong relationships with some of the keystone taxa (Figure 6).
Figure 7. Co-occurrence network and module structure of micro-eukaryotic communities in sediments of the thermokarst lakes. Nodes represent OTUs and are colored by (A) taxonomic groups and (B) major modules (modules contain more than 10% of the nodes). Edges represent strong and significant Spearman’s correlations (R > 0.5, or R < –0.5, P < 0.05). P-values were adjusted using the FDR method for false discovery. (C) Topological roles of the nodes in the network. Zi represents within-module connectivity. Pi represents among-module connectivity. Each dot represents an OTU colored by phylum. (D) The taxonomic composition of the major modules shown in number of OTUs.
Figure 8. Structural equation modeling analysis depicting the relationships between spatial factors (including latitude, longitude, and elevation), climatic factors (including mean annual temperature and mean annual precipitation), pH, nutrient (including sediment organic carbon, total nitrogen, and total phosphorus), nutrient ratios (including C:N, C:P, and N:P ratios), and the major modules (ModA, ModB, ModC, and ModD). Solid and dashed arrows represent the significant and non-significant relationships, respectively. Red and green arrows represent negative and positive relationships, respectively. The significant path coefficients were shown adjacent to the path with *, **, and *** denote the significant level of p < 0.05, p < 0.01, and p < 0.001, respectively.
In our study, diverse microbial eukaryotes (a total of 11,499 OTUs) were identified from the sediments of the studied thermokarst lakes with an average OTU richness of 1,130 per lake (Figure 3). In general, Diatom, Gastrotricha, Nematozoa, Arthropoda, Ciliophora, Annelida, and Cercozoa had a high relative abundance of the sequences (>5% of relative abundance on average). In fact, the relative proportion of microbial eukaryotes in a sample inferred from 18S rRNA gene sequencing can be either greater or lower than their actual abundance, because eukaryotic genomes can encode for multiple copies of this gene (Gong et al., 2013). Changes observed in gene reads proportion have to be considered with caution. However, our data also showed that these microbial eukaryotes had a high diversity. For example, Ciliophora, Diatom, and Cercozoa had more than 100 OTUs on average, and Euglenozoa, Chlorophyta, Ochrophyta, and Nematozoa had more than 50 OTUs (Figure 3). These microbial eukaryotes are also observed in many other lacustrine environments with high abundance and diverse (Kammerlander et al., 2015; Filker et al., 2016; Capo et al., 2017; Debroas et al., 2017). MECs are crucial components of aquatic environments, contributing to ecosystem structure and function, such as biodiversity, biogeochemical cycling, and climate regulation (Schaechter, 2012; Simon et al., 2015; Rodríguez-Martínez et al., 2020). However, MECs in aquatic ecosystems vary drastically along time and across space, leading to consequent variations in ecosystem structure and function (Allison and Martiny, 2008; Capo et al., 2016; Keck et al., 2020; Pearman et al., 2020).
Based on accumulating evidence proposed by previous studies, the dynamics of MECs are thought to be influenced by various abiotic and biotic factors (Simon et al., 2015; Wang et al., 2015). For example, the distribution patterns of MECs are proposed to be influenced by spatial factors (Olsen et al., 2013; Wang et al., 2016), local precipitation and irradiation (Williamson et al., 2010; Jones et al., 2013), pH (Liu et al., 2014; Shen et al., 2014; Gong et al., 2015), temperature (Salonen et al., 2018; Liu et al., 2021), and nutrients (Salonen et al., 2018; Liu et al., 2021). Understanding how environmental variables shape community structures of MECs in thermokarst lakes is of importance for comprehensive biogeography studies as well as for predictions of the lake ecosystems in future climate change. In our studied thermokarst lakes, MECs varied substantially across these lakes in terms of diversity, composition, and structure (Figures 3, 4). Multiple analyses showed that both OTU richness and community structure of MECs had close relationships with spatial factors, climatic factors, and sediment properties, particularly with latitude, MAP, pH, as well as nutrient concentrations and stoichiometric ratios. Moreover, different groups of microbial eukaryotes (taxonomic groups and co-occurrence modules) responded differentially to the measured environmental variables.
It has been well demonstrated that pH is an overriding important factor in controlling diversity and composition of both bacterial communities (Fierer and Jackson, 2006; Lauber et al., 2009; Griffiths et al., 2011) and MECs (Mulder et al., 2005; Tsyganov et al., 2013; Shen et al., 2014) in soil from local to global scales. In our study of lake sediments, pH had direct effects on MECs (Figure 4) and had negative relationships with the OTU richness of MECs as well as major phylum, including Gastrotricha, Annelida, Cercozoa, Ochrophyta, Chlorophyta, and Rotifera (Figure 3). The divergence of pH also had significant relationships with the variation of most of the major taxonomic groups and all the major modules (Table 1). These results suggest that pH is an important factor in shaping MECs in sediments of thermokarst lakes. In addition, climatic conditions, particularly warming, have been suggested to influence MECs in terrestrial (Bates et al., 2013) and aquatic (Domis et al., 2014; Rigosi et al., 2014; Capo et al., 2017) ecosystems. In our study, however, MAP had significant relationships with diversity and composition of MECs. Moreover, our study also supported the important role of nutrient concentration and stoichiometric ratios. Nutrients are undoubtedly other major drivers in ecosystem structure and function. Even moderate changes in nutrient concentration and relative balance (stoichiometric ratio) can lead to significant modifications of MECs (Wu et al., 2011; Domis et al., 2014; Rigosi et al., 2014; Capo et al., 2017).
Our data identified a large number of OTUs and a high relative abundance of sequences as Diatom, which predominantly function as phototrophic organisms. In addition, other phototrophic groups, Chlorophyta and Ochrophyta were also high in OTU richness (Figure 3) and sequence proportion (Figure 5). The shallow depth and low suspended sediment concentrations of the thermokarst lakes allow greater light penetration to lake sediments. Moreover, sediments had plenty of nutrients that were further supplemented from the terrestrial input. Thus, phototrophic organisms are presented in sediments with high abundance and diversity. In the studies of limnology and paleolimnology (Davidson et al., 2013; Soares et al., 2013; Capo et al., 2017), Chlorophyta and diatoms are sensitive to nutrient changes and have high abundance in eutrophic conditions. Ciliophora was commonly found to have high abundance in polar sediment and permafrost soil with high organic matter content (Mackelprang et al., 2011; Jansson and Tas, 2014; Geisen et al., 2015; Hindshaw et al., 2017). Ciliophora is predominantly heterotrophic, and often bacterivorous and algae grazers (Sherr and Sherr, 2002), exhibiting a very clear response to nutrient changes (Capo et al., 2017). High abundance of their preys following nutrient enrichment can lead to the predominance of Ciliophora taxa (Capo et al., 2017). Paleolimnological studies have observed a positive relationship between P concentration and relative abundance of Ciliates and suggested that Ciliophora could be ecological indictors of eutrophication levels in aquatic environments (Xu et al., 2014; Pawlowski et al., 2016). In our study, we found that the variation of the Ciliophora had significant relationships with nutrient concentrations and stoichiometric ratios (Table 1). In aquatic ecosystems, Cercozoans are also bacterivorous and algae grazers. Previous studies showed that temperature fluctuations have strong influence on richness and relative abundance of Cercozoa (Tveit et al., 2015; Capo et al., 2016). In our study, however, the richness and relative abundance of Cercozoa were significantly associated with pH and C:N (Figures 3, 5), and the variation of Cercozoa had close relationships with most of the measured spatial, climatic, and physicochemical variables (Table 1).
In our case, the results showed strong relationships between environmental factors and MECs, as well as differential relationships between some microbial eukaryotic groups and measured environmental variables, which are consistent with many previous studies as discussed above. However, a number of gaps still exist in our understanding of the dynamic of MECs. For example, our measured environmental variables only explained half of the variation in MECs, suggesting potential influences of other abiotic variables, but most likely, abiotic variables (such as predation and mutualistic/parasitic interactions) (Zhao et al., 2019; Shi et al., 2020) on MECs. Moreover, in thermokarst lakes, the sediments are initially originated from permafrost soil and continuously supplemented by collapse of surrounding permafrost (Zhao et al., 2019; Shi et al., 2020). The sinking of plankton eukaryotes also contributes significantly to the sequences of MECs from sediment samples (Capo et al., 2017; Broman et al., 2019). Thus, the sediments have a considerable proportion of terrestrial and plankton microbial eukaryotes. The limited abiotic and biotic variables as well as the complex composition of the MECs in sediment samples may impede our comprehensive understanding of the biogeography of MECs in thermokarst lakes and require further studies.
Thermokarst lakes are widely distributed on the Qinghai-Tibet Plateau. In this study, we investigated the micro-eukaryotic communities in sediment of the thermokarst lakes across the QTP. We found that Diatom, Ciliophora, Nematozoa, and Cercozoa were dominant in terms of both OTU richness and relative abundance. OTU richness of the overall communities and major phylum were significantly correlated with multiple environmental variables. SEM and mantel test suggested that micro-eukaryotic communities in sediment of thermokarst lakes were jointly controlled by spatial, climatic, and physicochemical factors. Within these factors, latitude, MAP, pH, nutrient concentrations, and stoichiometric ratios were the most relevant variables. Different micro-eukaryotic groups, such as different phyla and network modules, responded differently to these variables. Due to the fact that sediment MECs in thermokarst lakes also contain the microbial eukaryotes from water column and surrounding terrestrial soil, a microbial source tracking will deepen further our understanding of the environmental responses of sediment MECs.
The datasets presented in this study can be found in online repositories. The names of the repository/repositories and accession number(s) can be found below: ngdc.cncb.ac.cn (Accession: PRJCA005279).
ZR designed the study, did the analyses, performed the field and laboratory work, and prepared the manuscript. YZ did the visualization. XL did the analyses and performed the laboratory work. CZ performed the laboratory work and prepared the manuscript. All authors read and reviewed the manuscript.
This study was supported by the start-up funding for the new introduced talents of the Beijing Normal University (28707-111032105).
The authors declare that the research was conducted in the absence of any commercial or financial relationships that could be construed as a potential conflict of interest.
All claims expressed in this article are solely those of the authors and do not necessarily represent those of their affiliated organizations, or those of the publisher, the editors and the reviewers. Any product that may be evaluated in this article, or claim that may be made by its manufacturer, is not guaranteed or endorsed by the publisher.
We are grateful to Yongming Deng and Xuan Jia for their assistance in the field work.
Allison, S. D., and Martiny, J. B. H. (2008). Resistance, resilience, and redundancy in microbial communities. Proc. Natl. Acad. Sci. U.S.A. 1051, 11512–11519. doi: 10.1073/pnas.0801925105
Anthony, K. M. W., Zimov, S. A., Grosse, G., Jones, M. C., Anthony, P. M., Iii, F. S. C., et al. (2014). A shift of thermokarst lakes from carbon sources to sinks during the Holocene epoch. Nature 511, 452–456. doi: 10.1038/nature13560
Bastian, M., Heymann, S., and Jacomy, M. (2009). “Gephi: an open source software for exploring and manipulating networks,” in Proceedings of the International AAAI Conference on Web and Social Media, Washington, DC.
Bates, S. T., Clemente, J. C., Flores, G. E., Walters, W. A., Parfrey, L. W., Knight, R., et al. (2013). Global biogeography of highly diverse protistan communities in soil. ISME J. 7, 652–659. doi: 10.1038/ismej.2012.147
Benjamini, Y., and Hochberg, Y. (1995). Controlling the false discovery rate: a practical and powerful approach to multiple testing. J. R. Stat. Soc. Ser. B 57, 289–300. doi: 10.2307/2346101
Biskaborn, B. K., Smith, S. L., Noetzli, J., Matthes, H., Vieira, G., Streletskiy, D. A., et al. (2019). Permafrost is warming at a global scale. Nat. Commun. 10:264. doi: 10.1038/s41467-018-08240-4
Broman, E., Li, L., Fridlund, J., Svensson, F., Legrand, C., and Dopson, M. (2019). Spring and late summer phytoplankton biomass impact on the coastal sediment microbial community structure. Microb. Ecol. 77, 288–303. doi: 10.1007/s00248-018-1229-6
Capo, E., Debroas, D., Arnaud, F., Guillemot, T., Bichet, V., Millet, L., et al. (2016). Long-term dynamics in microbial eukaryotes communities: a palaeolimnological view based on sedimentary DNA. Mol. Ecol. 25, 5925–5943. doi: 10.1111/mec.13893
Capo, E., Debroas, D., Arnaud, F., Perga, M., Chardon, C., and Domaizon, I. (2017). Tracking a century of changes in microbial eukaryotic diversity in lakes driven by nutrient enrichment and climate warming. Environ. Microbiol. 19, 2873–2892. doi: 10.1111/1462-2920.13815
Caporaso, J. G., Kuczynski, J., Stombaugh, J., Bittinger, K., Bushman, F. D., Costello, E. K., et al. (2010). QIIME allows analysis of high-throughput community sequencing data. Nat. Methods 7, 335–336. doi: 10.1038/nmeth.f.303
Csardi, M. G. (2013). Package ‘igraph’. Available online at: https://cran.r-project.org/web/packages/igraph/index.html (accessed August 1, 2021).
Dancer, W. S., Eliason, R., and Lekhakul, S. (1998). Microwave assisted soil and waste dissolution for estimation of total phosphorus. Commun. Soil Sci. Plan. 29, 1997–2006. doi: 10.1080/00103629809370089
Davidson, T. A., Jeppesen, E., Holmes, J., Jones, V., Mackay, A. W., and Rose, N. (2013). The role of palaeolimnology in assessing eutrophication and its impact on lakes. J. Paleolimnol. 49, 391–410. doi: 10.1007/s10933-012-9651-0
Debroas, D., Domaizon, I., Humbert, J., Jardillier, L., Lepère, C., Oudart, A., et al. (2017). Overview of freshwater microbial eukaryotes diversity: a first analysis of publicly available metabarcoding data. FEMS Microbiol. Ecol. 93, 1–14. doi: 10.1093/femsec/fix023
Domis, L. N. D. S., Van de Waal, D. B., Helmsing, N. R., Van Donk, E., and Mooij, W. M. (2014). Community stoichiometry in a changing world: combined effects of warming and eutrophication on phytoplankton dynamics. Ecology 95, 1485–1495. doi: 10.1890/13-1251.1
Du Toit, A. (2018). Permafrost thawing and carbon metabolism. Nat. Rev. Microbiol. 16:519. doi: 10.1038/s41579-018-0066-4
Farquharson, L. M., Mann, D. H., Grosse, G., Jones, B. M., and Romanovsky, V. E. (2016). Spatial distribution of thermokarst terrain in Arctic Alaska. Geomorphology 273, 116–133. doi: 10.1016/j.geomorph.2016.08.007
Fierer, N., and Jackson, R. B. (2006). The diversity and biogeography of soil bacterial communities. Proc. Natl. Acad. Sci. U.S.A. 103, 626–631. doi: 10.1073/pnas.0507535103
Filker, S., Sommaruga, R., Vila, I., and Stoeck, T. (2016). Microbial eukaryote plankton communities of high-mountain lakes from three continents exhibit strong biogeographic patterns. Mol. Ecol. 25, 2286–2301. doi: 10.1111/mec.13633
Geisen, S., Tveit, A. T., Clark, I. M., Richter, A., Svenning, M. M., Bonkowski, M., et al. (2015). Metatranscriptomic census of active protists in soils. ISME J. 9, 2178–2190. doi: 10.1038/ismej.2015.30
Gong, J., Dong, J., Liu, X., and Massana, R. (2013). Extremely high copy numbers and polymorphisms of the rDNA operon estimated from single cell analysis of oligotrich and peritrich ciliates. Protist 164, 369–379. doi: 10.1016/j.protis.2012.11.006
Gong, J., Shi, F., Ma, B., Dong, J., Pachiadaki, M., Zhang, X., et al. (2015). Depth shapes α- and β-diversities of microbial eukaryotes in surficial sediments of coastal ecosystems. Environ. Microbiol. 17, 3722–3737. doi: 10.1111/1462-2920.12763
Griffiths, R. I., Thomson, B. C., James, P., Bell, T., Bailey, M., and Whiteley, A. S. (2011). The bacterial biogeography of British soils. Environ. Microbiol. 13, 1642–1654. doi: 10.1111/j.1462-2920.2011.02480.x
He, R., Wooller, M. J., Pohlman, J. W., Catranis, C., Quensen, J., Tiedje, J. M., et al. (2012). Identification of functionally active aerobic methanotrophs in sediments from an arctic lake using stable isotope probing. Environ. Microbiol. 14, 1403–1419. doi: 10.1111/j.1462-2920.2012.02725.x
Heslop, J. K., Walter Anthony, K. M., Sepulveda-Jauregui, A., Martinez-Cruz, K., Bondurant, A., Grosse, G., et al. (2015). Thermokarst lake methanogenesis along a complete talik profile. Biogeosciences 12, 4317–4331. doi: 10.5194/bg-12-4317-2015
Hindshaw, R. S., Lindsay, M. R., and Boyd, E. S. (2017). Diversity and abundance of microbial eukaryotes in stream sediments from Svalbard. Polar Biol. 40, 1835–1843. doi: 10.1007/s00300-017-2106-3
In’T Zandt, M. H., Liebner, S., and Welte, C. U. (2020a). Roles of thermokarst lakes in a warming world. Trends Microbiol. 28, 769–779.
In’T Zandt, M. H., Frank, J., Yilmaz, P., Cremers, G., Jetten, M. S. M., and Welte, C. U. (2020b). Long-term enriched methanogenic communities from thermokarst lake sediments show species-specific responses to warming. FEMS Microbes 1:xtaa008. doi: 10.1093/femsmc/xtaa008
Jansson, J. K., and Tas, N. (2014). The microbial ecology of permafrost. Nat. Rev. Microbiol. 12, 414–425. doi: 10.1038/nrmicro3262
Jones, A. C., Liao, T. V., Najar, F. Z., Roe, B. A., Hambright, K. D., and Caron, D. A. (2013). Seasonality and disturbance: annual pattern and response of the bacterial and microbial eukaryotic assemblages in a freshwater ecosystem. Environ. Microbiol. 15, 2557–2572. doi: 10.1111/1462-2920.12151
Jongejans, L. L., Liebner, S., Knoblauch, C., Mangelsdorf, K., Ulrich, M., Grosse, G., et al. (2021). Greenhouse gas production and lipid biomarker distribution in Yedoma and Alas thermokarst lake sediments in Eastern Siberia. Glob. Change Biol. 27, 2822–2839. doi: 10.1111/gcb.15566
Kammerlander, B., Breiner, H. W., Filker, S., Sommaruga, R., Sonntag, B., and Stoeck, T. (2015). High diversity of protistan plankton communities in remote high mountain lakes in the European Alps and the Himalayan mountains. FEMS Microbiol. Ecol. 91:fiv010. doi: 10.1093/femsec/fiv010
Karlsson, J. M., Lyon, S. W., and Destouni, G. (2012). Thermokarst lake, hydrological flow and water balance indicators of permafrost change in Western Siberia. J. Hydrol. 464-465, 459–466. doi: 10.1016/j.jhydrol.2012.07.037
Keck, F., Millet, L., Debroas, D., Etienne, D., Galop, D., Rius, D., et al. (2020). Assessing the response of micro-eukaryotic diversity to the Great Acceleration using lake sedimentary DNA. Nat. Commun. 11:3831. doi: 10.1038/s41467-020-17682-8
Kokelj, S. V., and Jorgenson, M. T. (2013). Advances in thermokarst research. Permafrost. Periglac. 24, 108–119. doi: 10.1002/ppp.1779
Lauber, C. L., Hamady, M., Knight, R., and Fierer, N. (2009). Pyrosequencing-based assessment of soil pH as a predictor of soil bacterial community structure at the continental scale. Appl. Environ. Microb. 75, 5111–5120. doi: 10.1128/AEM.00335-09
Le Moigne, A., Bartosiewicz, M., Schaepman Strub, G., Abiven, S., and Pernthaler, J. (2020). The biogeochemical variability of Arctic thermokarst ponds is reflected by stochastic and niche-driven microbial community assembly processes. Environ. Microbiol. 22, 4847–4862. doi: 10.1111/1462-2920.15260
Liu, K., Liu, Y., Hu, A., Wang, F., Liang, J., Zhang, Z., et al. (2021). Temporal variability of microbial communities during the past 600 years in a Tibetan lake sediment core. Palaeogeogr. Palaeoclimatol. Palaeoecol. 584:110678. doi: 10.1016/j.palaeo.2021.110678
Liu, L., Yang, J., Lv, H., and Yu, Z. (2014). Synchronous dynamics and correlations between bacteria and phytoplankton in a subtropical drinking water reservoir. FEMS Microbiol. Ecol. 90, 126–138. doi: 10.1111/1574-6941.12378
Lozupone, C. A., and Knight, R. (2007). Global patterns in bacterial diversity. Proc. Natl. Acad. Sci. U.S.A. 104, 11436–11440. doi: 10.1073/pnas.0611525104
Luo, J., Niu, F., Lin, Z., Liu, M., and Yin, G. (2015). Thermokarst lake changes between 1969 and 2010 in the Beilu River Basin, Qinghai-Tibet Plateau, China. Sci. Bull. 60, 556–564. doi: 10.1007/s11434-015-0730-2
Luo, X., Liu, K., Shen, Y., Yao, G., Yang, W., Mortimer, P. E., et al. (2021). Fungal community composition and diversity vary with soil horizons in a subtropical forest. Front. Microbiol. 12:650440. doi: 10.3389/fmicb.2021.650440
Mackelprang, R., Waldrop, M. P., DeAngelis, K. M., David, M. M., Chavarria, K. L., Blazewicz, S. J., et al. (2011). Metagenomic analysis of a permafrost microbial community reveals a rapid response to thaw. Nature 480, 368–371. doi: 10.1038/nature10576
Matheus Carnevali, P. B., Herbold, C. W., Hand, K. P., Priscu, J. C., and Murray, A. E. (2018). Distinct microbial assemblage structure and archaeal diversity in sediments of Arctic thermokarst lakes differing in methane sources. Front. Microbiol. 9:1192. doi: 10.3389/fmicb.2018.01192
Mulder, C., Harm, J. V. W., and Annemarie, P. V. W. (2005). Numerical abundance and biodiversity of below-ground taxocenes along a pH gradient across the Netherlands. J. Biogeogr. 32, 1775–1790. doi: 10.1111/j.1365-2699.2005.01321.x
Oksanen, J., Kindt, R., Legendre, P. O., Hara, B., Stevens, M. H. H., Oksanen, M. J., et al. (2007). The vegan package. Commun. Ecol. Package 10, 631–637.
Olefeldt, D., Goswami, S., Grosse, G., Hayes, D., Hugelius, G., Kuhry, P., et al. (2016). Circumpolar distribution and carbon storage of thermokarst landscapes. Nat. Commun. 7:13043. doi: 10.1038/ncomms13043
Olsen, L. M., Van Ardelan, M., Hewes, C. D., Holm-Hansen, O., Reiss, C., Bizsel, N., et al. (2013). Microbial biogeography during austral summer 2007 in the surface waters around the South Shetland Islands. Aquat. Microb. Ecol. 70, 131–140. doi: 10.3354/ame01650
Pastick, N. J., Jorgenson, M. T., Goetz, S. J., Jones, B. M., Wylie, B. K., Minsley, B. J., et al. (2019). Spatiotemporal remote sensing of ecosystem change and causation across Alaska. Glob. Change Biol. 25, 1171–1189. doi: 10.1111/gcb.14279
Pawlowski, J., Lejzerowicz, F., Apotheloz-Perret-Gentil, L., Visco, J., and Esling, P. (2016). Protist metabarcoding and environmental biomonitoring: time for change. Eur. J. Protistol. 55, 12–25. doi: 10.1016/j.ejop.2016.02.003
Payne, R. J. (2013). Seven reasons why protists make useful bioindicators. Acta Protozool. 52:105. doi: 10.4467/16890027AP.13.0011.1108
Pearman, J. K., Biessy, L., Thomson-Laing, G., Waters, S., Vandergoes, M. J., Howarth, J. D., et al. (2020). Local factors drive bacterial and microeukaryotic community composition in lake surface sediment collected across an altitudinal gradient. FEMS Microbiol. Ecol. 96:fiaa070. doi: 10.1093/femsec/fiaa070
Qian, Y., Cheng, C., Drouillard, K., Zhu, Q., Feng, H., He, S., et al. (2019). Bioaccumulation and growth characteristics of Vallisneria natans (Lour.) Hara after chronic exposure to metal-contaminated sediments. Environ. Sci. Pollut. R. 26, 20510–20519. doi: 10.1007/s11356-019-05347-z
Quast, C., Pruesse, E., Yilmaz, P., Gerken, J., Schweer, T., Yarza, P., et al. (2013). The SILVA ribosomal RNA gene database project: improved data processing and web-based tools. Nucleic Acids Res. 41, 590–596. doi: 10.1093/nar/gks1219
R Core Team (2017). R: A Language and Environment for Statistical Computing. Vienna: R Foundation for Statistical Computing.
Rigosi, A., Carey, C. C., Ibelings, B. W., and Brookes, J. D. (2014). The interaction between climate warming and eutrophication to promote cyanobacteria is dependent on trophic state and varies among taxa. Limnol. Oceanogr. 59, 99–114. doi: 10.4319/lo.2014.59.1.0099
Rodríguez-Martínez, R., Leonard, G., Milner, D. S., Sudek, S., Conway, M., Moore, K., et al. (2020). Controlled sampling of ribosomally active protistan diversity in sediment-surface layers identifies putative players in the marine carbon sink. ISME J. 14, 984–998. doi: 10.1038/s41396-019-0581-y
Roeske, K., Sachse, R., Scheerer, C., and Roeske, I. (2012). Microbial diversity and composition of the sediment in the drinking water reservoir Saidenbach (Saxonia, Germany). Syst. Appl. Microbiol. 35, 35–44. doi: 10.1016/j.syapm.2011.09.002
Rosseel, Y. (2012). lavaan: an R package for structural equation modeling. J. Stat. Softw. 48, 1–36. doi: 10.3389/fpsyg.2014.01521
Salonen, I. S., Chronopoulou, P., Leskinen, E., and Koho, K. A. (2018). Metabarcoding successfully tracks temporal changes in eukaryotic communities in coastal sediments. FEMS Microbiol. Ecol. 95:fiy226. doi: 10.1093/femsec/fiy226
Shen, C., Liang, W., Shi, Y., Lin, X., Zhang, H., Wu, X., et al. (2014). Contrasting elevational diversity patterns between eukaryotic soil microbes and plants. Ecology 95, 3190–3202. doi: 10.1890/14-0310.1
Sherr, E. B., and Sherr, B. F. (2002). Significance of predation by protists in aquatic microbial food webs. Antonie Van Leeuwenhoek 81, 293–308. doi: 10.1023/a:1020591307260
Shi, T., Li, M., Wei, G., Liu, J., and Gao, Z. (2020). Distribution patterns of microeukaryotic community between sediment and water of the Yellow River Estuary. Curr. Microbiol. 77, 1496–1505. doi: 10.1007/s00284-020-01958-9
Shirokova, L. S., Pokrovsky, O. S., Kirpotin, S. N., Desmukh, C., Pokrovsky, B. G., Audry, S., et al. (2013). Biogeochemistry of organic carbon, CO2, CH4, and trace elements in thermokarst water bodies in discontinuous permafrost zones of Western Siberia. Biogeochemistry 113, 573–593. doi: 10.1007/s10533-012-9790-4
Simon, M., Lopez-Garcia, P., Deschamps, P., Moreira, D., Restoux, G., Bertolino, P., et al. (2015). Marked seasonality and high spatial variability of protist communities in shallow freshwater systems. ISME J. 9, 1941–1953. doi: 10.1038/ismej.2015.6
Smol, J. P. (1992). Paleolimnology: an important tool for effective ecosystem management. J. Aquat. Ecosyst. Health 1, 49–58. doi: 10.1007/BF00044408
Soares, E. M., Figueredo, C. C., Gücker, B., and Boëchat, I. G. (2013). Effects of growth condition on succession patterns in tropical phytoplankton assemblages subjected to experimental eutrophication. J. Plankton Res. 35, 1141–1153. doi: 10.1093/plankt/fbt061
Strauss, J., Schirrmeister, L., Grosse, G., Wetterich, S., Ulrich, M., Herzschuh, U., et al. (2013). The deep permafrost carbon pool of the Yedoma region in Siberia and Alaska. Geophys. Res. Lett. 40, 6165–6170. doi: 10.1002/2013GL058088
Tsyganov, A. N., Milbau, A., Beyens, L., Institutionen För Ekologi, M. O. G., Umeå, U., and Teknisk-naturvetenskapliga, F. (2013). Environmental factors influencing soil testate amoebae in herbaceous and shrubby vegetation along an altitudinal gradient in subarctic tundra (Abisko, Sweden). Eur. J. Protistol. 49, 238–248. doi: 10.1016/j.ejop.2012.08.004
Tveit, A. T., Urich, T., Frenzel, P., and Svenning, M. M. (2015). Metabolic and trophic interactions modulate methane production by Arctic peat microbiota in response to warming. Proc. Natl. Acad. Sci. U.S.A. 112, E2507–E2516. doi: 10.1073/pnas.1420797112
Veremeeva, A., Nitze, I., Günther, F., Grosse, G., and Rivkina, E. (2021). Geomorphological and climatic drivers of thermokarst lake area increase trend (1999-2018) in the Kolyma Lowland Yedoma Region, North-Eastern Siberia. Remote Sens. Basel 13:178. doi: 10.3390/rs13020178
Vigneron, A., Cruaud, P., Bhiry, N., Lovejoy, C., and Vincent, W. F. (2019). Microbial community structure and methane cycling potential along a thermokarst pond-peatland continuum. Microorganisms 7:486. doi: 10.3390/microorganisms7110486
Walter, K. M., Zimov, S. A., Chanton, J. P., Verbyla, D., and Chapin, F. S. (2006). Methane bubbling from Siberian thaw lakes as a positive feedback to climate warming. Nature 443, 71–75. doi: 10.1038/nature05040
Wang, K., Ye, X., Zhang, H., Chen, H., Zhang, D., and Liu, L. (2016). Regional variations in the diversity and predicted metabolic potential of benthic prokaryotes in coastal northern Zhejiang, East China Sea. Sci. Rep. 6:38709. doi: 10.1038/srep38709
Wang, Y., Liu, L., Chen, H., and Yang, J. (2015). Spatiotemporal dynamics and determinants of planktonic bacterial and microeukaryotic communities in a Chinese subtropical river. Appl. Microbiol. Biot. 99, 9255–9266. doi: 10.1007/s00253-015-6773-0
Wang, Y., Ren, Z., Ma, P. P., Wang, Z. M., Niu, D. C., Fu, H., et al. (2020). Effects of grassland degradation on ecological stoichiometry of soil ecosystems on the Qinghai-Tibet Plateau. Sci. Total Environ. 722:e137910. doi: 10.1016/j.scitotenv.2020.137910
West, J. J., and Plug, L. J. (2008). Time-dependent morphology of thaw lakes and taliks in deep and shallow ground ice. J. Geophys. Res. 113:1009. doi: 10.1029/2006JF000696
Wik, M., Varner, R. K., Anthony, K. W., MacIntyre, S., and Bastviken, D. (2016). Climate-sensitive northern lakes and ponds are critical components of methane release. Nat. Geosci. 9, 99–105. doi: 10.1038/ngeo2578
Williamson, C. E., Salm, C., Cooke, S., and Saros, J. (2010). How do UV radiation, temperature, and zooplankton influence the dynamics of alpine phytoplankton communities? Hydrobiologia 648, 73–81. doi: 10.1007/s10750-010-0147-5
Winkel, M., Sepulveda-Jauregui, A., Martinez-Cruz, K., Heslop, J. K., Rijkers, R., Horn, F., et al. (2019). First evidence for cold-adapted anaerobic oxidation of methane in deep sediments of thermokarst lakes. Environ. Res. Commun. 1:21002. doi: 10.1088/2515-7620/ab1042
Wu, T., Ayres, E., Bardgett, R. D., Wall, D. H., and Garey, J. R. (2011). Molecular study of worldwide distribution and diversity of soil animals. Proc. Natl. Acad. Sci. U.S.A. 108, 17720–17725. doi: 10.1073/pnas.1103824108
Xu, Y., Vick-Majors, T., Morgan-Kiss, R., Priscu, J. C., and Amaral-Zettler, L. (2014). Ciliate diversity, community structure, and novel taxa in lakes of the McMurdo Dry Valleys, Antarctica. Biol. Bull. 227, 175–190. doi: 10.1086/BBLv227n2p175
Yu, G., Smith, D. K., Zhu, H., Guan, Y., and Lam, T. T. Y. (2017). ggtree: an R package for visualization and annotation of phylogenetic trees with their covariates and other associated data. Methods Ecol. Evol. 8, 28–36. doi: 10.1111/2041-210x.12628
Zhao, Z., He, J., Geisen, S., Han, L., Wang, J., Shen, J., et al. (2019). Protist communities are more sensitive to nitrogen fertilization than other microorganisms in diverse agricultural soils. Microbiome 7:33. doi: 10.1186/s40168-019-0647-0
Zhou, C. P. (2018). The Spatial Dataset of Climate on the Tibetan Plateau (1961-2020). Beijing: National Tibetan Plateau Data Center.
Keywords: thermokarst lakes, sediment, microbial eukaryotes, latitude, climate, nutrient
Citation: Ren Z, Zhang Y, Li X and Zhang C (2022) Biogeography of Micro-Eukaryotic Communities in Sediment of Thermokarst Lakes Are Jointly Controlled by Spatial, Climatic, and Physicochemical Factors Across the Qinghai-Tibet Plateau. Front. Ecol. Evol. 10:901107. doi: 10.3389/fevo.2022.901107
Received: 21 March 2022; Accepted: 29 March 2022;
Published: 06 May 2022.
Edited by:
Zhang Min, China Institute of Water Resources and Hydropower Research, ChinaReviewed by:
Jianjun Wang, Nanjing Institute of Geography and Limnology (CAS), ChinaCopyright © 2022 Ren, Zhang, Li and Zhang. This is an open-access article distributed under the terms of the Creative Commons Attribution License (CC BY). The use, distribution or reproduction in other forums is permitted, provided the original author(s) and the copyright owner(s) are credited and that the original publication in this journal is cited, in accordance with accepted academic practice. No use, distribution or reproduction is permitted which does not comply with these terms.
*Correspondence: Ze Ren, cmVuemVkeWtAZ21haWwuY29t; Cheng Zhang, emhhbmdjaGVuZ0BibnUuZWR1LmNu
Disclaimer: All claims expressed in this article are solely those of the authors and do not necessarily represent those of their affiliated organizations, or those of the publisher, the editors and the reviewers. Any product that may be evaluated in this article or claim that may be made by its manufacturer is not guaranteed or endorsed by the publisher.
Research integrity at Frontiers
Learn more about the work of our research integrity team to safeguard the quality of each article we publish.