- 1College of Tourism and Urban Rural Planning, Chengdu University of Technology, Chengdu, China
- 2College of Architecture and Environment, Sichuan University, Chengdu, China
China is a country that experiences severe natural hazards. In comparison to urban residents, farmers in rural areas of China are more susceptible to these natural hazards, whose impact is multidimensional; however, existing research has mainly focused on the household level. Based on China Household Finance Survey (CHFS) data in 2019, a total of 17,900 farmer households have been chosen to discuss the influences of natural hazards on the rural income and subjective well-being from the individual perspective and the family perspective; further, regional differences within the sphere of influence have been analyzed. Empirical results demonstrate that (1) the farmer household income is a factor that affects subjective well-being, but does not play a decisive role. (2) From the perspective of spatial differences and laws, subjective well-being and the income of farmers vary significantly. The subjective well-being in North China (NC) is the highest, while the subjective well-being in the Central South (CS) is the lowest. The distribution trend of rural income is high in Southeast China (SE) and low in Northwest China (NW). (3) Natural hazards can lower the subjective well-being [Mean ATT (average treatment effect) = –0.1040] and income (Mean ATT = –0.1715) of farmers significantly. Moreover, the influences of natural hazards on subjective well-being are lower than that on income. Therefore, it is imperative to ascertain the impact of natural hazards on farmers’ subjective well-being and household income. Further, the government should consider regional differences and the different affected groups, and also strengthen the farmers’ ability to cope with hazards and their post-hazard recovery ability during the implementation of hazard rescue.
Introduction
Natural hazards and disasters have significant influences on human society, leading to casualties, economic losses, and environmental damages (Chen et al., 2020). With increases in the frequency and severity of global natural hazards, they have become one of the most concerning global problems. According to Mori et al. (2021), the annual global deaths caused by natural hazards are about 60,000, which accounts for 0.1% of the total global annual deaths. This leads to annual economic losses of about 8.66–43.3 billion dollars.
Owing to global climatic changes and its unique geographical environment, China is influenced by significant natural hazards. Recently, frequent natural hazards have influenced production, life, and social development to a large extent. China’s Ministry of Emergency Management has found that natural hazards have claimed 107 million victims, 162,000 house collapses, 11,739,000 ha of affected crop area, and 334.02 billion yuan of direct economic losses in 2021. It is important to note that, in comparison to urban areas, rural areas are faced with greater threats of natural hazards due to the poor living and geographic conditions, along with the weak ability of residents to defend themselves against hazards.
Natural hazards refer to abnormal phenomena that occur in nature. In this study, natural hazards include various meteorological hazards and geological hazards, such as earthquakes, floods, landslides, debris flow, and typhoons. Existing studies on natural hazards mainly focus on a single type of hazard (Kind et al., 2017). The research content mainly includes causes and spatial-temporal evolutions of natural hazards, risk assessment and avoidance, influences, and response analysis (Lohmann et al., 2019; Palanca-Tan, 2020). Researchers have carried out relatively in-depth and systematic studies on the influences of natural hazards, primarily from multiple aspects like the economy, agriculture, industry, and income gap. However, there are few empirical studies on the influences of natural hazards on individuals and households of farmers in China. In particular, studies on subjective well-being are still very few (Wang et al., 2000). The income of rural residents is an important and intuitive index to measure the influences of hazards from the economic perspective. Nevertheless, subjective well-being can reflect the influences of natural hazards on individuals more comprehensively (Berlemann and Eurich, 2021). This is because subjective well-being is a subjective evaluation of the life satisfaction of individuals affected by hazards (Diener et al., 2013), and is affected by a variety of factors (Helliwell, 2003). Therefore, it is also necessary to discuss the subjective well-being of farmers, in addition to the relationship between rural household income and natural hazards.
In summary, it is imperative to understand the influences of natural hazards in China, which witnesses the frequent occurrence of natural hazards. For this reason, this study focuses on the national macroscopic scale. Based on the China Household Finance Survey (CHFS) data of 17,900 farmer households in 29 provinces in China, regional differences in the subjective well-being and income of Chinese farmers were analyzed. Next, the influences of natural hazards on the subjective well-being and income of peasants were quantified by propensity score matching (PSM) based on the idea of quasi-natural experimentation. This study not only empirically analyzes natural hazards in rural areas, but also provides a valuable reference for the implementation of hazard rescue and decision-making on post-hazard development.
Literature Review and Hypotheses
Income and Natural Hazards
Income has always been an important economic concern, and is studied as an important multidisciplinary individual/family characteristic. Through a literature review, it is found that studies on income mainly focus on income inequality (the income gap) (Butler et al., 2020), income distribution (Kind et al., 2017), and factors influencing income (Kan et al., 2006; Westmore, 2018). Specifically, the major factors influencing income include human capital, social capital, resource endowment, policy institutions, and natural hazards (Ward and Shively, 2015; Kumar, 2019). Additionally, rural residents’ income is often associated with the urban–rural gap, livelihood capital, livelihood risk, and poverty (Wu et al., 2015; Liu et al., 2020).
At present, academia has focused its efforts on the relationships between hazards and the affected subject: human society. Some studies on natural hazards and income issues have originated in the west, and after Kunreuther (1997) proposed the concept of “hazard economics”, related studies have received extensive research attention. Existing studies mainly analyze the influences of hazards on economics from the macroscopic economy scale and microscopic individual scale. In terms of the economy, scholars focus on the influences of natural hazards and their influences on national economic growth. For example, based on the hurricane damage index built using panel data in the United States, Strobl (2011) found that hurricanes decreased the annual economic growth rate of a county by 0.45%, on average. Nevertheless, state-scale calculations have demonstrated that hurricanes may not affect national economic growth. However, Murlidharan and Shah (2022) pointed out that hazards have significantly negative influences on national economic growth in the short-term, but such influences weaken gradually with the passage of time. In terms of individuals, researchers mainly focus on the economic impact of natural hazards on people (including individuals and families) on the micro-level. Palanca-Tan (2020) carried out a case study in a fishing village in the Philippines, determining that extreme climate first affected the fishing industry, influencing the household income of fishermen and finally intensifying the vulnerability of families. Coffman and Noy (2012) pointed out, through an empirical study of the DID model, that natural hazards have significant long-term influences on income, and the practical per capita income differs by about 112–267 dollars from those in neighboring counties. Some researchers believe that natural hazards lower the income in the short-term, but not the long-term. It is worth noting that Belasen and Polachek (2008) investigated employment and income variations between hurricane-affected counties and unaffected counties. They found that the income of directly affected counties increased by 4.35%, while that of neighboring counties decreased by 4.5%, on average.
In addition to the abovementioned hazards, studies on the relationship between natural hazards and income have been carried out for common hazard types, such as earthquakes (Wei et al., 2017), landslides (Mertens et al., 2016), floods (Mottaleb et al., 2013), and droughts (Arouri et al., 2015). Generally speaking, the influences of natural hazards on the income of rural residents are generally consistent. That is, in the short-term, natural hazards decrease the income significantly, but the influence weakens as time goes on. Further, the income level might exceed the level before the hazard after a period of time. However, the exposure degree to natural hazards leads to differences in the natural environment of affected regions. Further, different hazard prevention abilities of individuals lead to varying influences of these hazards on individuals and families (Clark et al., 1998). Based on the above theoretical analysis, a hypothesis is proposed:
H1: Natural hazards have significantly negative influences on farmer household income.
Subjective Well-Being and Natural Hazards
With continued social development, subjective well-being is attracting the attention of more and more scholars and policymakers. It has become a multidisciplinary research topic, involving the economy, psychology, and sociology. Nevertheless, the concept of subjective well-being is quite controversial, with no consensus being reached yet. Most people believe that subjective well-being is a positive and intrinsic psychological state with strong subjective emotions (Tsou and Liu, 2001). Diener et al. (1999) believed that subjective well-being is an extensive category, including the general judgment of people’s emotional responses and life satisfaction. Some studies often use the terms subjective well-being and life satisfaction interchangeably, although some believe that they are not completely consistent (Berlemann, 2016). For the purposes of this study, the two terms are synonyms, referring to a residents’ cognition and evaluation regarding their actual quality of life based on subjective emotion.
Studies on subjective well-being focus on the influencing factors. Existing studies have proven that factors influencing subjective well-being mainly comprise personal characteristics, including age, gender, educational background, health conditions, marriage status, and income (Clark, 2018; Frey and Stutzer, 2002); family characteristics, such as fixed assets, housing conditions, and family expenditure (Zhang et al., 2018; Lohmann et al., 2019); social development, including the unemployment rate, social fairness, and income inequality (Oishi et al., 2011; Dell’Anno and Amendola, 2015); the natural environment, including climatic changes and environmental pollution (Cuñado and de Gracia, 2013; Zhang et al., 2017); and policy institutions, such as policy beliefs, public services, political freedoms, and democratic rights (Frey and Stutzer, 2002; Helliwell, 2006). In addition to the abovementioned factors, social relations, material welfare, neighborhood location, etc., also affect subjective well-being (Rafael et al., 2003; Stevenson and Wolfers, 2008). With continued research in this field, the scale has shifted from microscopic individuals to macroscopic regions, especially focusing on the spatial differences and causes of residents’ happiness in different regions and geographical backgrounds. Based on Gallup data in the United States, Rentfrow et al. (2009) carried out a correlation analysis on the relationships between the economy, education, and careers with subjective well-being at the state-scale. Based on 28 provinces (cities and municipalities) in China, Zhang et al. (2018) found that housing conditions have positive influences on the general satisfaction of residents. Eren and Aşıcı (2017) concluded—from urban-level analysis—that there are significant differences in subjective well-being in different cities.
It is important to note that, due to the comprehensive and systematic effects of hazards, some scholars have introduced the concept of subjective well-being into their studies. The influences of natural hazards on human society can be divided into two types: direct and indirect (Berlemann, 2016). Direct influences refer to damage to houses, raw materials, and resources caused by natural hazards. Indirect influences refer to secondary influences after damage to physical infrastructure (Cavallo and Noy, 2011). However, the effects of natural hazards on happiness are mainly indirect. Based on questionnaire data on influences of the Nepal earthquake in 2015, Sapkota (2018) found that subjective well-being has a significantly negative correlation with the degree of experienced damage. However, a cross-sectional survey in Germany in 2012 found that flood experiences may not influence subjective well-being (Osberghaus and Kühling, 2016). Based on survey data on survivals from the Philippines typhoon, Hamama-Raz et al. (2017) found that individual resources could facilitate a feeling of subjective well-being after natural hazards. Additionally, researchers have investigated some natural hazards, such as hurricanes (Berlemann, 2016), droughts (Carroll et al., 2009), forest fires (Ambrey et al., 2017), etc., mainly focusing on America, Japan, Germany, and some countries in South and Southeast Asia (Goebel et al., 2013; Lohmann et al., 2019). Few studies have been conducted in China (Wang et al., 2000). To the best of our knowledge, this study is the first demonstration of the subjective well-being of Chinese farmers under the influences of various natural hazards.
H2: Natural hazards can lower the subjective well-being of farmers.
Data and Methods
Data Source
All data in this study was obtained from the CHFS, issued by the Research Center of Southwestern University of Finance and Economics1. This data includes the general demographic features, financial status, assets, income, consumption, employment, insurance, and other family information. In 2019, CHFS data samples covered 29 provinces (except Xinjiang, Tibet, Hong Kong, Macao, and Taiwan). It collected the information of 107,008 family members from 34,643 households. Herein, based on the research objective, the CHFS data were processed as follows: firstly, heads of household samples in the individual variable databases were screened out and correspond to the family variable database one by one (both individual variable database and family variable database contain ID numbers, so samples are matched by ID numbers here). Secondly, eliminate all non-rural households in the sample of head of household screened out in the previous step; Then, to make the empirical results more accurate, all rural samples with missing dependent variables and explanatory variables were deleted. Thirdly, missing samples, in terms of the dependent variables and explanatory variables, were deleted. Finally, the study obtained a valid sample of 17,900 households nationwide, including those affected and unaffected by natural hazards.
Variable Definition and Descriptive Statistics
Dependent Variables
In this study, the dependent variables include subjective well-being and income. The subjective well-being index is assessed based on the responses of heads of households to the question: “generally speaking, do you feel happy now?” Subjective well-being used the five-point Likert scale, where values of 1–5 reflect very unhappy to very happy states. The levels account for 1.23, 4.21, 26.70, 41.52, and 26.34% of the population, respectively. The mean subjective well-being of respondents was 3.875, which was between Moderate and Subjective well-being. Household income refers to the total household income of respondents in the last year, comprising income from wages, income from agricultural production and management, income from industrial business, income from properties, and transfer income. The annual average income of all respondent households was 52,170.57 yuan.
Explanatory Variables
According to the abovementioned theoretical analysis and research hypothesis, the core explanatory variable is whether respondents and their families have been affected by natural hazards from 2014 to 2019. In the questionnaire, interviewers determined whether respondents and their families have been affected by natural hazards through the following question: “Did your family have been affected by natural hazards since 2014?” If respondents answered “Yes,” the value was recorded as 1; otherwise, it was recorded as 0.
Control Variables
Existing empirical studies have demonstrated that subjective well-being and the income of farmers are influenced by multiple factors (Frey and Stutzer, 2002). Since these factors are of the individual level and household level, respectively, the control variables in this study are mainly personal features and family features of the heads of households, including the education level, health condition, age, communication tools, household size, working conditions, fixed assets, fund demand, and food expenses. Specific variable selection and descriptive statistics are listed in Table 1.
Comparative Analysis of the Characteristics of Farmers and Their Families in Samples
The t-test results of dependent variables and control variables before matching are listed in Table 2. When other economic conditions of respondents are not controlled, the subjective well-being gap between farmers affected by natural hazards and the rest of the farmers was –0.133, and the mean farmer household income difference was –0.291, both of which pass the significance test at a 1% level. This reveals that the subjective well-being and income of farmers affected by natural hazards were lower than those of other farmers. Additionally, the statistical results also demonstrated that individuals with a lower education degree, poorer physical health, and worse communication tools were affected more by natural hazards. Farmer households with more family members, fewer fixed assets, and those that require capital support for production and management are more easily affected by natural hazards. Through a comparison of mean values, we found that there are significant differences in individual and family features between farmers affected by natural hazards and those unaffected. However, the goal of our study is to understand the specific influences of natural hazards on the subjective well-being and income of farmers. As a result, it is necessary to further prove the aforementioned influences by using the precise metering method.
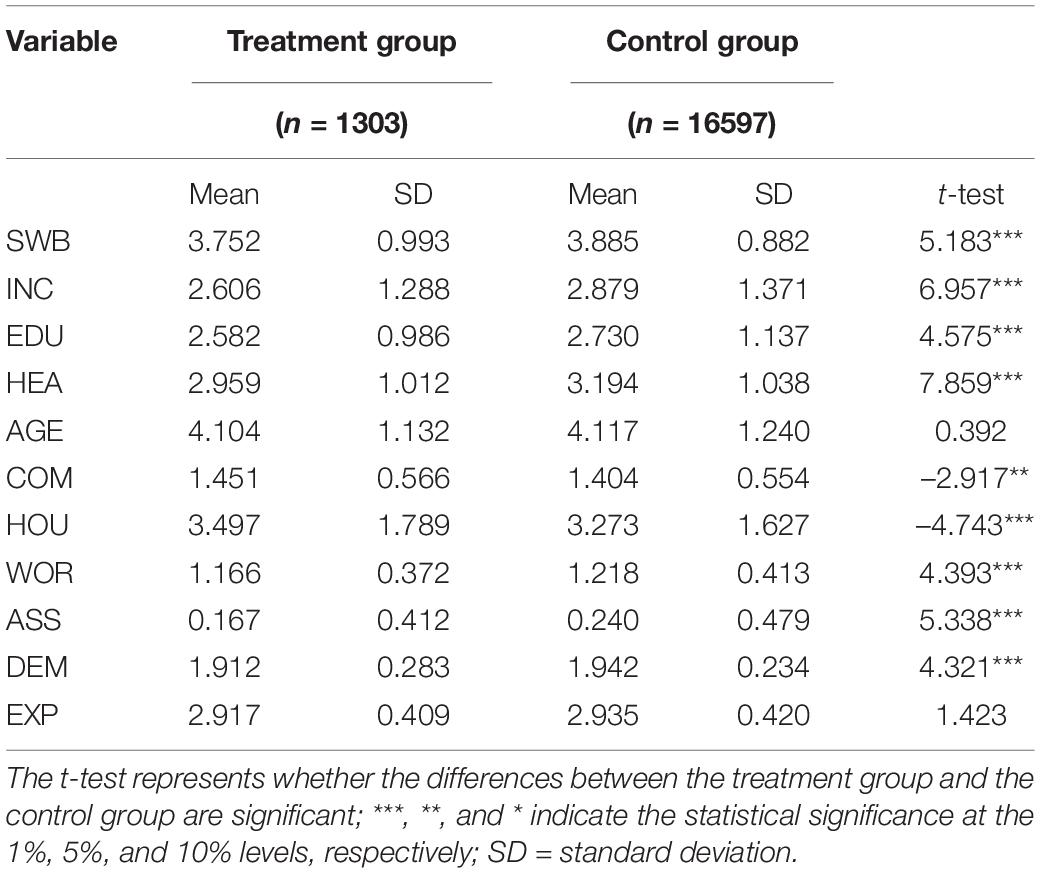
Table 2. Differences between farmers who have been affected by natural hazards and those unaffected.
Methods
To prove whether there are significant relationships between natural hazards and subjective well-being and income, differences in the subjective well-being and income caused by other factors need to be eliminated first. PSM is a method that can overcome the selection bias problem and make the treatment effect evaluation more effective. This is a concept from quasi-natural experimentation, and is a nonlinear estimation method proposed by Rosenbaum and Rubin (1982), mainly used for post-event balance treatment of covariate elements in the experimental group and for balance treatment of covariate elements, similar to randomization. The specific step involves conducting propensity value matching by using the “propensity score, PS” as the distance function and eliminating the influences of variable “confusion” through phenomenon causality, which is gained by controlling the PS. Hence, the causal efficiency of the explanatory variables on the outcome variables can be ensured.
In the present study, farmers affected by natural hazards were determined as the treatment group (HAZ = 1), and farmers unaffected by natural hazards were used as the control group (HAZ = 0). By matching members with similar individual features between the treatment group and the control group, the differences in the subjective well-being and household income between the two groups were further analyzed. A total of nine factors of individual features and family features of the heads of households were chosen as the control variables. Nearest neighbor matching (k = 4), kernel matching, radius matching (r = 0.01), and local linear regression matching were used. The matching was completed by using the “psmatch2” command in STATA/MP 16. The specific algorithm used is as follows:
(1) The conditional probability fitting values of the influences of natural hazards on farmers were calculated using the Logit model, also known as the PS. The probability model was:
where, D is the treatment variable. When D = 1, farmers have been affected by natural hazards; if D = 0, farmers have not been affected by natural hazards. Xi represents the observable individual and family features (control variables) of farmer households.
(2) The treatment group and the control group were matched according to their PSs. Since different matching methods applied different matching values and weights, the matching results could differ. Therefore, four matching methods, including nearest neighbor matching (k = 4), radius matching (r = 0.01), kernel matching, and local linear regression matching were applied. In this section, nearest neighbor matching (k = 4) was chosen. The calculation method can be formalized as
where, Pi and Pj are PS values of the ith “treatment group” and jth “control group,” respectively. C(P) refers to the neighborhood relationship between i and j.
(3) The influences of natural hazards on the subjective well-being and income of farmers were calculated. This is the mean treatment effect (average treatment effect, ATT):
where, Y is the outcome variable, indicating subjective well-being and income in this study. Other variables and parameters, such as i and j, have the same meanings as above.
Results
Regional Differences in the Subjective Well-Being and Income of Farmers
Regional differences in the subjective well-being and household income of farmers are quite prominent. To ascertain more spatial distribution characteristics of subjective well-being and income, we will analyze the distribution pattern of the subjective well-being and income in six administrative regions based on the mean value (Figure 1). The mean subjective well-being of farmers is shown in Figure 1A, at is 3.875, which lies between Moderate and Subjective well-being. The subjective well-being in NE, NC, and EC is higher than the national mean level. Specifically, the subjective well-being score of NC is the highest, reaching 3.97. Furthermore, there are three regions below the national level, among which CS (SWB = 3.81) is the lowest and SW (SWB = 3.86) is relatively the highest.
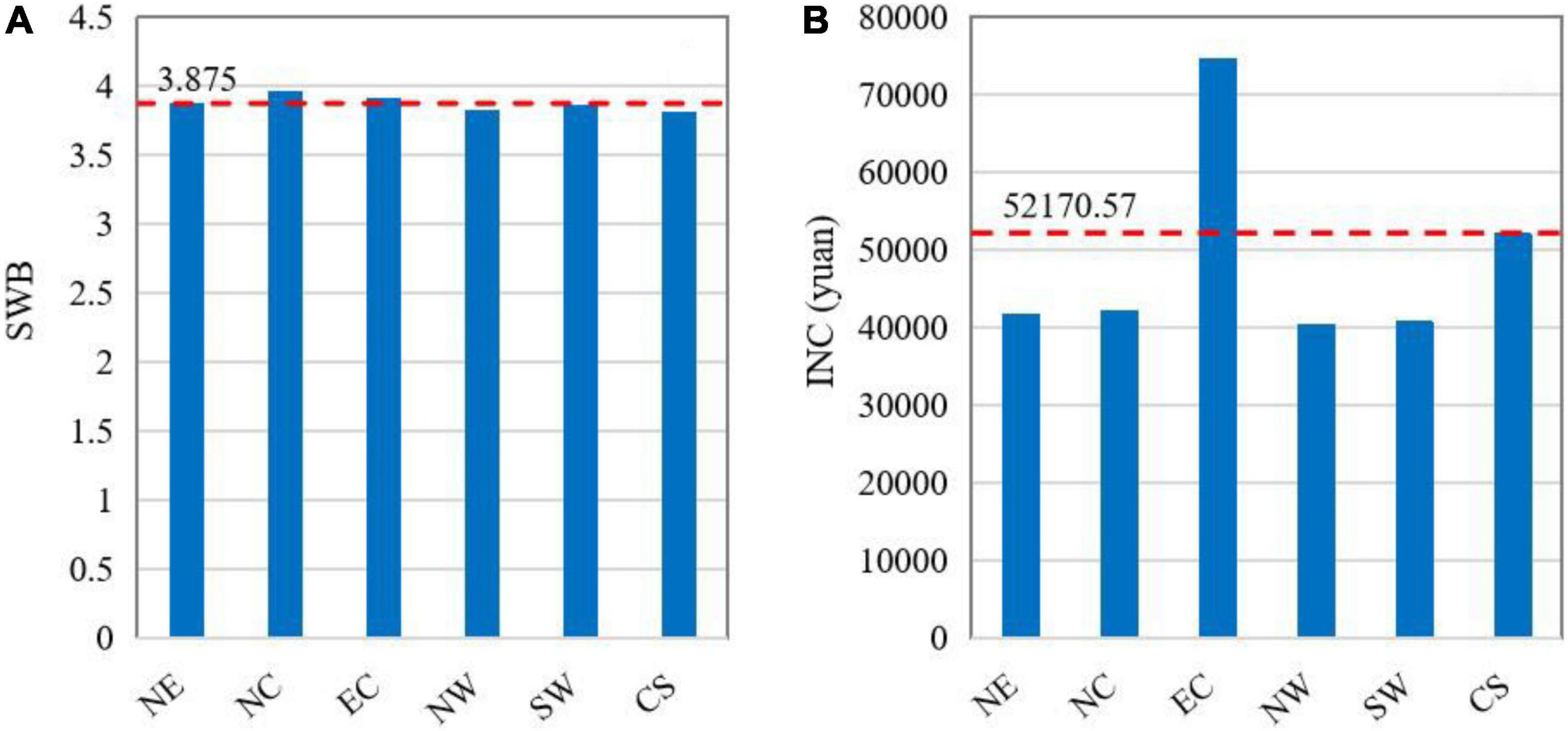
Figure 1. Regional differences in farmers’ subjective well-being and income: (A) Subjective well-being; (B) Income (yuan).
This distribution can be attributed to three factors, including economic factors, environmental factors and political factors. The economy of NC is relatively developed, and the income of rural residents is high and from a wide range of sources. More importantly, this region is close to the political center of China (Beijing), and its social recognition is high. Therefore, subjective well-being is higher than other regions. However, the CS is an area with rapid economic development and large-scale factories, so as to attract a large number of migrant workers came to the region. And result in a high population density in the region, which in turn leads to negative impacts such as deterioration of the living environment and traffic congestion (Luo et al., 2016). In addition, the income and quality of life of local farmers did not improve, so their subjective well-being has decreased.
The regional distribution of farmer household income is shown in Figure 1B. Among the six administrative regions, EC has the highest average household income—at 74556.73 yuan—and it is the only region where farmer household income is higher than the national average (INC = 52,170.57 yuan). Generally speaking, farmer household income is consistent with the regional economic development level, i.e., EC is the most developed region in China. EC has not only good natural conditions and infrastructure, but also has a higher average wave level than other regions. Hence, farmer household income in EC is far higher than that in other regions and the national average. Additionally, the farmer household income levels significantly differ among NE, NC, NW, and SW, at 41647.77 yuan, 42111.01 yuan, 40277.17 yuan, and 40572.95 yuan, respectively.
Effects of Natural Hazards on the Subjective Well-Being and Income of Farmers
Estimation of Propensity Score
To match the treatment group and the control group, the probability of farmers being influenced by natural hazards was estimated by using the Logit model, which was taken as the PS value. The estimation results of PS are shown in Table 3. It can be seen from the regression results of the Logit model that the education background (EDU = –0.082), physical condition (HEA = –0.225), age (AGE = –0.059), working conditions (WOR = –0.468), fixed assets (ASS = –0.375), and capital demands (DEM = –0.252) can affect the influence of natural hazards on farmers and their families significantly. In other words, these factors all can lower the probability of farmers being affected by natural hazards. In contrast, communication tools (COM = 0.133), family size (HOU = 0.097), and food expenses (EXP = 0.147) could increase the probability of farmers and their families being affected by natural hazards. Generally speaking, older farmers, with a lower education background and poorer physical condition, are more easily affected by these hazards. This is primarily because this group of individuals has little knowledge regarding hazard prevention and alleviation. Thus, they cannot avoid hazards well and their post-hazard recovery is relatively weak. Additionally, families that have more family members, lower fixed assets, and poor working conditions are more easily affected by natural hazards.
Matching Effect Analysis
The effectiveness of the PSM method depends on two prerequisites. One is the balance test and the other is the common support test. Since test results of different matching methods are mostly consistent, only the robustness test results of nearest neighbor matching (K = 4) are shown in this section.
The balance test requires that the treatment group and control group have no systematic differences in terms of the matching variables after the completion of matching. Rosenbaum (1985) tested the balance before and after matching by using the standardized bias. In other words, whether the balance passes the test is mainly determined by the covariate deviation changes and t-statistical significance level changes before and after matching (Caliendo and Scheel-Kopeinig, 2008). It can be seen from Table 4 that the standardized bias of all covariates is smaller than 5% after matching, and the t-test statistics of most control variables before matching are significant. Moreover, all P values of the t-test statistics for all control variables after matching are higher than 0.2, indicating that control variables are insignificant after matching. Therefore, matching significantly reduced the difference in the distribution of explanatory variables between the treatment group and the control group, and the overall matching quality was good.
The kernel density diagram (Figure 2) shows the density distribution fitting conditions of the P-score (calculated using Equation 1) before and after matching between the treatment group and the control group. In this study, the common support domain was tested by plotting the kernel density. If the common support domain is too narrow, samples beyond the common support domain cannot be matched effectively, leading to sample loss. Otherwise, the matching effect is relatively good if the common support domain increases after matching. The kernel density diagrams of subjective well-being and income are shown in Figures 2A,B, respectively. It is clear that the PS probability density distributions of two groups after matching differ significantly. In other words, there is significant coverage between the two groups, and the PS probability distribution of the two groups has gradually become consistent. This reveals that the chosen matching variables and matching method in this study are reasonable and can lower differences in the explanatory variable distribution between two groups to a certain extent, facilitating decreases in the biased error of sample selection.
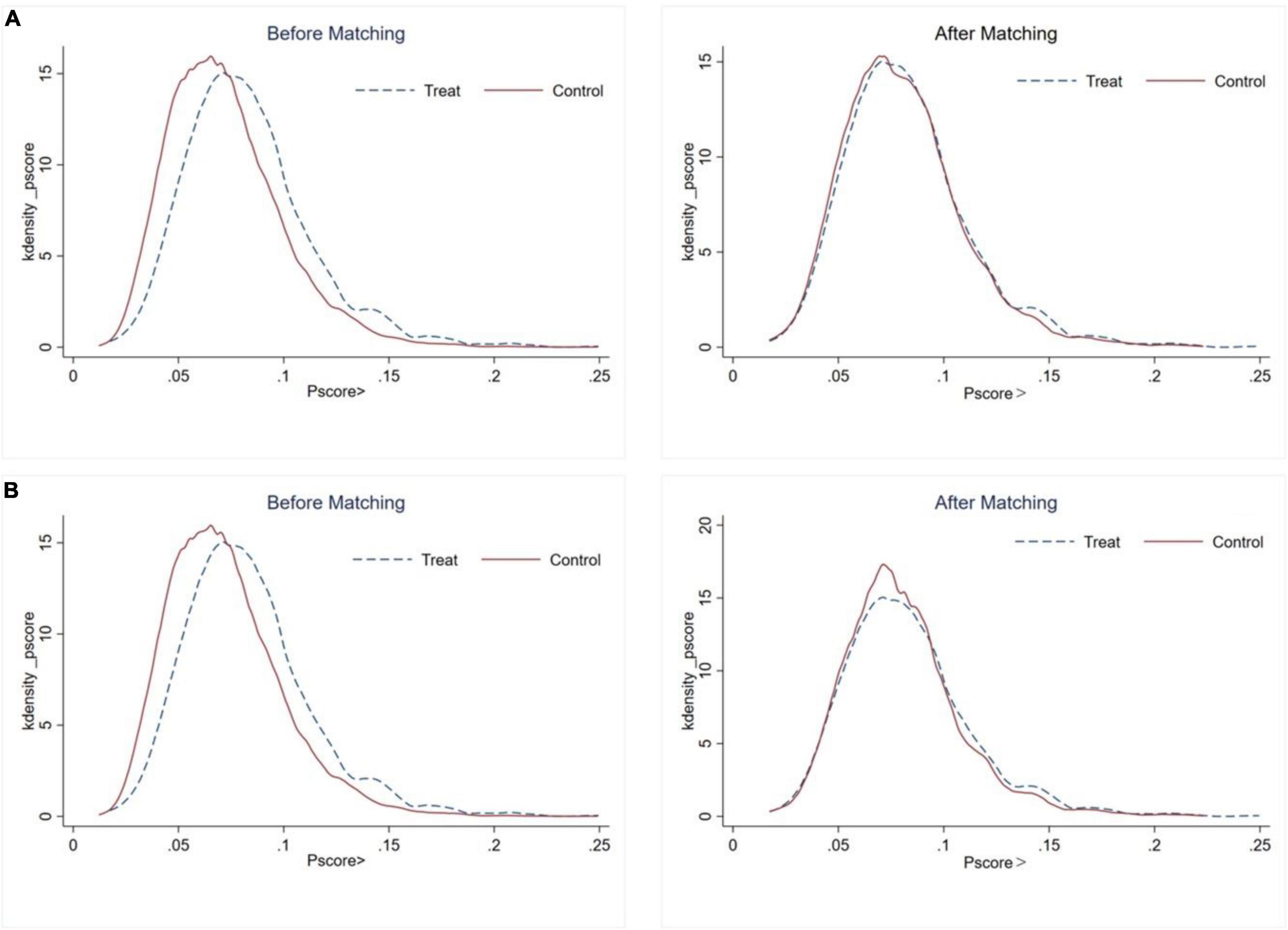
Figure 2. Kernel density distribution of natural hazards before and after matching: (A) Subjective well-being; (B) Income. The solid line represents rural residents who have received the indicated intervention. —The dashed line represents rural residents who have not received the indicated intervention.
Average Treatment Effect
Estimation based on multiple matching methods can increase the robustness and reliability of the results. Therefore, different matching methods were used in this section to estimate the ATT of natural hazards on the subjective well-being and income of farmers, including nearest neighbor matching (k = 4), radius matching (r = 0.01), kernel matching, and local linear regression matching. The ATT results of the treatment group after matching using different matching methods are shown in Table 5.
Although the calculated results of different matching methods differ slightly, their general trends are consistent. Firstly, the influences of natural hazards on the subjective well-being of farmers were analyzed. It can be seen from Table 5 that the mean ATT = –0.1040, indicating that natural hazards have significantly negative effects on the subjective well-being of farmers. In other words, under the same conditions, the subjective well-being of the treatment group is 0.1040 lower than that of the control group. Hence, H1 is verified. Similarly, the household income of the treatment group is lower than that of the control group. This means that natural hazards can decrease the household income (Mean ATT = –0.1715) of farmers significantly. Therefore, H2 is verified. Therefore, we found, through PSM, that natural hazards have a significant negative relationship with farmers’ subjective well-being and income. It is important to note that the influences of natural hazards on subjective well-being are smaller than those on income (0.104 < 0.1714). This is mainly because, in comparison to income, the influences of natural hazards on subjective well-being are not direct. Additionally, there are many influencing factors on the subjective well-being, which can relieve the influences of hazards to some extent.
Discussion
In 2021, the number of people affected and economic losses caused by natural hazards in China decreased by 31 million and 36.13 billion yuan, respectively, compared to the previous year (data source: Ministry of Emergency Management). However, China is a country that suffers from the most severe hazards. As a result, it is necessary to obtain a more systematic and comprehensive understanding of the natural hazards to alleviate their effects. This study found that the distributions of the subjective well-being and income of farmers differ significantly among different regions. However, natural hazards have significantly negative influences on subjective well-being and income. This is consistent with the research results of Mertens et al. (2016) and Lohmann et al. (2019). Nevertheless, some scholars have also pointed out that the influences of natural hazards on income decreased significantly in the short-term (Mu and Chen, 2016); however, rural income may exceed the level before hazards in the long-term. This is because farmers recombine their productive asset inventories, and infrastructure in affected areas is rebuilt and sometimes improved, reducing transaction costs (Gignoux and Menendez, 2014). Additionally, post-hazard rebuilding can provide more jobs, and these regions can obtain economic support. Therefore, a farmer’s income will be recovered and can even increase.
Natural hazards affect people in a variety of ways. People who become homeless, lose income, lose jobs, or get injured owing to natural hazards could have low subjective well-being because their living conditions have changed greatly. Moreover, natural hazards could influence subjective well-being indirectly through people’s perceptions of hazard risks. Cui and Han (2019) found—through an empirical study—that risk perception of earthquakes includes perceiving the probability of earthquakes in the future, which influences an individual’s subjective well-being. Further, natural hazards can affect farmers’ income through direct and indirect channels. Direct influences include decreased incomes as a response to decreases in the agricultural output due to natural hazards, while indirect influences include income reduction caused by hindered transportation of agricultural produce owing to damaged infrastructure (e.g., highways). Hence, it is believed that natural hazards can influence the subjective well-being of individuals and household income through direct and indirect channels.
The relationship between income and subjective well-being is an important research topic in the field of economics. Early studies can be dated back to Easterlin (1974), who found that subjective well-being may not continue to increase with increases in income. Subsequent researchers called this phenomenon the “Easterlin paradox”. However, some studies found that economic or income growth would promote continuous improvements in subjective well-being (Diener et al., 2012). Generally speaking, such positive influences exhibited a decreasing trend. It is important to note that some researchers have found that there is a negative correlation between subjective well-being and per capita GDP (Deaton and Stone, 2013). The empirical results of this study indicate that income has a certain positive impact on subjective well-being, but the degree of this impact varies with time, the region, and the individual. It can be seen from Figures 1A,B that the regional average income and subjective well-being do not have the same trend. This reflects that the region with the highest average income may not have the highest average subjective well-being. For example, CS has a relatively higher average income, but its average subjective well-being is the lowest of all six administrative regions, at only 3.81. Therefore, income is a factor that influences subjective well-being, but does not play a decisive role in it.
Furthermore, although subjective well-being is highly related to income, income is not the only factor that influences subjective well-being; it is also influenced by individual characteristics, social relations, the external environment, policy systems, etc. Moreover, natural hazards influence subjective well-being indirectly, but directly influence income. As a result, natural hazards influence subjective well-being less than income. This might be because many factors influence subjective well-being, including income, which relieves some influences of hazards on subjective well-being; in contrast, the influences of natural hazards on income are more direct.
Natural hazards can influence the subjective well-being and household income of farmers significantly. The two factors are part of the individual level and household level, respectively. Therefore, the government has to consider farmer victims at different levels during hazard rescue and when formulating post-hazard recovery policies. They must provide employment and psychological counseling to individuals, house repair and rebuilding, material assistance at the household level, etc. Moreover, the government should increase hazard prevention measures, including safety education and general engineering measures. Some studies have found that preventive measures not only strengthen the hazard risk perception of farmers, but also are conducive to preventing decreases in subjective well-being caused by anxiety regarding future natural hazards (Berlemann, 2016). In addition, hazard prevention measures can reduce the loss of life and property.
Conclusion
Natural hazards can have significant influences on the social economy owing to the uncertainty, uncontrollability, and difficulties associated with their prediction. Based on micro-data, this study got rid of small-scale research and focused on macro scale to quantify the impact of natural hazards on rural residents’ subjective well-being and family income from individual and household levels. Moreover, the method of this study is also separated from the traditional regression analysis, and PSM method is used to reduce the result bias caused by sample selection, so as to get more accurate quantitative results.
The results demonstrate that (1) the average subjective well-being of farmers in China is 3.875. Farmers in NC have the highest subjective well-being and farmers in CS have the lowest subjective well-being. (2) The household income of farmers in China exhibit obvious regional differences. It is generally high in the southeast and low in the northwest. (3) Natural hazards can lower the subjective well-being (Mean ATT = –0.1040) and income (Mean ATT = –0.1715) of farmers significantly, and natural hazards influence subjective well-being less than income.
Almost all countries have experienced the influences of various types of natural hazards, such as climate change. Rural areas are facing greater threats due to their exposure and vulnerability. This study also has some limitations. On the one hand, CHFS is not obtained through stratified sampling, and there may be regional imbalance in the sample data; on the other hand, the large difference in the number of control group and treatment group in the sample may not be conducive to matching (the matching effect is found to meet the requirements through testing). However, this study reveals the relationship of natural hazards with the subjective well-being and income of farmers in rural areas in China. This not only helps fill this research gap in China, but also provides a theoretical reference to deepen our understanding of natural hazards. In addition, the study of SWB can also provide more direct suggestions for the government’s policy design to improve residents’ happiness and sense of gain. We know that income will affect people’s subjective well-being, and to improve the well-being of rural residents, we should start from the aspects of land cultivation and industry. The government should actively explore local characteristics, drive the development of relevant industries, increase employment and income, and constantly strengthen ecological restoration and disaster prevention. More importantly, during hazard rescue, the government shall not only pay attention to the practical situation, but also consider issues from the individual and family perspectives, aiming to help farmers return to their normal lives and stabilize the regional development.
Data Availability Statement
The original contributions presented in the study are included in the article/supplementary material, further inquiries can be directed to the corresponding author.
Author Contributions
The author confirms being the sole contributor of this work and has approved it for publication.
Funding
This work was supported by Postdoctoral Programme of Environmental Science and Engineering, College of Architecture and Environment, Sichuan University.
Conflict of Interest
The author declares that the research was conducted in the absence of any commercial or financial relationships that could be construed as a potential conflict of interest.
Publisher’s Note
All claims expressed in this article are solely those of the authors and do not necessarily represent those of their affiliated organizations, or those of the publisher, the editors and the reviewers. Any product that may be evaluated in this article, or claim that may be made by its manufacturer, is not guaranteed or endorsed by the publisher.
Footnotes
References
Ambrey, C., Fleming, C., and Manning, M. (2017). The social cost of the Black Saturday bushfires. J. Soc. Issues 52, 298–312. doi: 10.1002/ajs4.21
Arouri, M., Nguyen, C., and Youssef, A. B. (2015). Natural disasters, household welfare, and resilience: evidence from rural vietnam. World Dev. 70, 59–77. doi: 10.1016/j.worlddev.2014.12.017
Belasen, A. R., and Polachek, S. W. (2008). How hurricanes affect employment and wages in local labor markets. SSRN Elect. J. 2008:212. doi: 10.2139/ssrn.1136212
Berlemann, M. (2016). Does hurricane risk affect individual well-being? Empirical evidence on the indirect effects of natural disasters. Ecol. Econ. 124, 99–113. doi: 10.1016/j.ecolecon.2016.01.020
Berlemann, M., and Eurich, M. (2021). Natural hazard risk and life satisfaction – Empirical evidence for hurricanes. Ecol. Econ. 190:107194. doi: 10.1016/j.ecolecon.2021.107194
Butler, J., Wildermuth, G. A., Thiede, B. C., and Brown, D. L. (2020). Population change and income inequality in rural america. Popul. Res. Policy Rev. 39, 1–23. doi: 10.1007/s11113-020-09606-7
Caliendo, M., and Scheel-Kopeinig, S. (2008). Some practical guidance for the implementation of propensity score matching. J. Econ. Surv. 22, 31–72. doi: 10.1111/j.1467-6419.2007.00527.x
Carroll, N., Frijters, P., and Shields, M. A. (2009). Quantifying the costs of drought: new evidence from life satisfaction data. J. Pub. Econ. 22, 445–461. doi: 10.1007/s00148-007-0174-3
Cavallo, E., and Noy, I. (2011). Natural disasters and the economy — a survey. Environ. Resour. Econ. 5, 63–102. doi: 10.1561/101.00000039
Chen, F., Chen, S., Zhang, X., Chen, J., Wang, X., Gowan, E. J., et al. (2020). Asian dust-storm activity dominated by Chinese dynasty changes since 2000 BP. Nat. Commun. 11:992. doi: 10.1038/s41467-020-14765-4
Clark, A. (2018). Four decades of the economics of happiness: where next? Revi. Incom. Weal. 64:12369. doi: 10.1111/roiw.12369
Clark, G., Moser, S., Ratick, S., Dow, K., Meyer, W., Emani, S., et al. (1998). Assessing the vulnerability of coastal communities to extreme storms: the case of revere, MA., USA. Mitig. Adapt. Strateg. Glob. Chang. 3, 59–82. doi: 10.1023/A:1009609710795
Coffman, M., and Noy, I. (2012). Hurricane Iniki: measuring the long-term economic impact of a natural disaster using synthetic control. Environ. Dev. 17, 187–205. doi: 10.1017/S1355770X11000350
Cui, K., and Han, Z. (2019). Association between disaster experience and quality of life: the mediating role of disaster risk perception. Qual. Life Res. 28, 509–513. doi: 10.1007/s11136-018-2011-4
Cuñado, J., and de Gracia, F. P. (2013). Environment and happiness: new evidence for spain. Soc. Indic. Res. 112, 549–567. doi: 10.1007/s11205-012-0038-4
Deaton, A., and Stone, A. (2013). Two happiness puzzles. Am. Econ. Rev. 103, 591–597. doi: 10.1257/aer.103.3.591
Dell’Anno, R., and Amendola, A. (2015). Social exclusion and economic growth: an empirical investigation in european economies. Rev. Incom. Weal. 61:96. doi: 10.1111/roiw.12096
Diener, E., Lucas, R., and Scollon, C. (2013). Beyond the hedonic treadmill: revising the adaptation theory of well-being. Am. Psychol. 61, 305–314. doi: 10.1037/0003-066X.61.4.305
Diener, E., Suh, E., Lucas, R., and Smith, H. (1999). Subjective well-being: three decades of progress. Psychol. Bull. 125, 276–302. doi: 10.1037/0033-2909.125.2.276
Diener, E., Tay, L., and Oishi, S. (2012). Rising income and the subjective well-being of nations. J. Pers. Soc. Psychol. 104, 67–76. doi: 10.1037/a0030487
Easterlin, R. A. (1974). “Does Economic Growth Improve the Human Lot? Some Empirical Evidence,” in Nat. Hous. Econ. Growth, eds P. A. David and M. W. Reder (Cambridge: Academic Press), 89–125. doi: 10.1016/b978-0-12-205050-3.50008-7
Eren, K. A., and Aşıcı, A. A. (2017). The determinants of happiness in turkey: evidence from city-level data. J. Happ. Stud. 18, 647–669. doi: 10.1007/s10902-016-9746-9
Frey, B., and Stutzer, A. (2002). What can economists learn from happiness research? J. Econ. Lit. 40:1320. doi: 10.1257/002205102320161320
Gignoux, J., and Menendez, M. (2014). Benefit in the wake of disaster: long-run effects of earthquakes on welfare in rural Indonesia. J. Dev. Econ. 118, 26–44. doi: 10.1016/j.jdeveco.2015.08.004
Goebel, J., Krekel, C., Tiefenbach, T., and Ziebarth, N. (2013). Natural Disaster, Policy Action, and Mental Well-Being: The Case of Fukushima. Berlin: Deutsches Institut für Wirtschaftsforschung. doi: 10.2139/ssrn.2345608
Hamama-Raz, Y., Palgi, Y., Leshem, E., Ben-Ezra, M., and Lavenda, O. (2017). Typhoon survivors’ subjective wellbeing-A different view of responses to natural disaster. PLoS One 12:327. doi: 10.1371/journal.pone.0184327
Helliwell, J. F. (2003). How’s life? Combining individual and national variables to explain subjective well-being. Ecol. Modell. 20, 331–360. doi: 10.1016/S0264-9993(02)00057-3
Helliwell, J. F. (2006). Well-being, social capital and public policy: what’s new?. Econ. J. 116, C34–C45. doi: 10.1111/j.1468-0297.2006.01074.x
Kan, I., Kimhi, A., and Lerman, Z. (2006). Farm output, non-farm income and commercialization in rural Georgia. J. Agric. Econ. 3, 276–286. doi: 10.1111/agec.12327
Kind, J., Wouter Botzen, W. J., and Aerts, J. C. J. H. (2017). Accounting for risk aversion, income distribution and social welfare in cost-benefit analysis for flood risk management. Wiley Interdiscip. Rev.-Clim. Chang. 8:e446. doi: 10.1002/wcc.446
Kumar, A. (2019). Subjective income expectations and risks in rural india. J. Dev. Area. 53:35. doi: 10.1353/jda.2019.0035
Kunreuther, H. (1997). Mitigating disaster losses through insurance. Insur. Math. Econ. 19, 262–262. doi: 10.1016/S0167-6687(97)83509-3
Liu, Y., Zhang, Q., Liu, Q., Yan, Y., Hei, W., Yu, D., et al. (2020). Different household livelihood strategies and influencing factors in the inner mongolian grassland. Sustainability 12, 1–15. doi: 10.3390/su12030839
Lohmann, P., Pondorfer, A., and Rehdanz, K. (2019). Natural hazards and well-being in a small-scale island society. Ecol. Econ. 159, 344–353. doi: 10.1016/j.ecolecon.2018.12.023
Luo, H., Yang, H., Xu, X., Yun, L., Chen, R., Chen, Y., et al. (2016). Relationship between occupational stress and job burnout among rural-to-urban migrant workers in Dongguan, China: a cross-sectional study. BMJ Open 61:e012597. doi: 10.1136/bmjopen-2016-012597
Mertens, K., Jacobs, L., Maes, J., Kabaseke, C., Maertens, M., Poesen, J., et al. (2016). The direct impact of landslides on household income in tropical regions: a case study from the Rwenzori Mountains in Uganda. Sci. Total Environ. 550, 1032–1043. doi: 10.1016/j.scitotenv.2016.01.171
Mori, N., Takemi, T., Tachikawa, Y., Tatano, H., Shimura, T., Tanaka, T., et al. (2021). Recent nationwide climate change impact assessments of natural hazards in Japan and East Asia. Weather Clim. Extrem. 32:100309. doi: 10.1016/j.wace.2021.100309
Mottaleb, K. A., Mohanty, S., Hoang, H. T. K., and Rejesus, R. M. (2013). The effects of natural disasters on farm household income and expenditures: a study on rice farmers in Bangladesh. Agric. Syst. 121, 43–52. doi: 10.1016/j.agsy.2013.06.003
Mu, J., and Chen, Y. (2016). Impacts of large natural disasters on regional income. Nat. Haz. 83:723. doi: 10.1007/s11069-016-2372-3
Murlidharan, T., and Shah, H. (2022). Economic consequences of catastrophes triggered by natural hazards. Stanford: Stanford University.
Oishi, S., Kesebir, S., and Diener, E. (2011). Income inequality and happiness. Psychol. Sci. 22, 1095–1100. doi: 10.1177/0956797611417262
Osberghaus, D., and Kühling, J. (2016). Direct and indirect effects of weather experiences on life satisfaction – which role for climate change expectations? J. Environ.Plan. Manag. 59, 2198–2230. doi: 10.1080/09640568.2016.1139490
Palanca-Tan, R. (2020). Social capital and vulnerability to extreme climate in a semi-urban fishing community in laguna de bay, philippines. J. Environ. Manage. 23, 89–101. doi: 10.47125/jesam/2020_2/10
Rafael, M., Tella, D., Robert, J., and MacCulloch, A. (2003). The macroeconomics of happiness. Revi. Econ. Stat. 85, 809–827. doi: 10.1162/003465303772815745
Rentfrow, P. J., Mellander, C., and Florida, R. (2009). Happy states of america: a state-level analysis of psychological, economic, and social well-being. J. Res. Pers. 43, 1073–1082. doi: 10.1016/j.jrp.2009.08.005
Rosenbaum, P. R. (1985). Constructing a control group using multivariate matched sampling methods that incorporate the propensity score. Am. Stat. 39, 33–38. doi: 10.1080/00031305.1985.10479383
Rosenbaum, P. R., and Rubin, D. B. (1982). Assessing Sensitivity to an Unobserved Binary Covariate in a Nonrandomized Experiment with Binary Outcome. Fort Belvoir: Defense Technical Information Center. 1–20.
Sapkota, J. (2018). Human well-being after 2015 Nepal earthquake: micro-evidence from one of the hardest hit rural villages. Int. J. Sustain. Dev. 21:825. doi: 10.1504/IJSD.2018.100825
Stevenson, B., and Wolfers, J. (2008). Economic growth and subjective well-being: reassessing the easterlin paradox. Brook. Pap. Econ. Act. 2008, 1–87. doi: 10.2139/ssrn.1121237
Strobl, E. (2011). The economic growth impact of hurricanes: evidence from U.S. Coast. Count. Revi. Econ. Stat. 93, 575–589. doi: 10.1162/REST_a_00082
Tsou, M.-W., and Liu, J.-T. (2001). Happiness and domain satisfaction in taiwan. J. Happ. Stud. 2, 269–288. doi: 10.1023/A:1011816429264
Wang, X., Gao, L., Zhang, H., Zhao, C., Shen, Y., and Shinfuku, N. (2000). Post-earthquake quality of life and psychological well-being: Longitudinal evaluation in a rural community sample in northern China. Psychiat. Clin. Neuros. 54, 427–433. doi: 10.1046/j.1440-1819.2000.00732.x
Ward, P., and Shively, G. (2015). Migration and land rental as responses to income shocks in rural china. Pac. Econ. Rev. 20, 511–543. doi: 10.1111/1468-0106.12072
Wei, B. Y., Gui-Wu, S. U., Wen-Hua, Q. I., Sun, L., Yang, W. U., Jin, Y. F., et al. (2017). The livelihood vulnerability of rural household in the earthquake-stricken area of ning’er,yunnan province. Seismol. Geol. 39, 158–171.
Westmore, B. (2018). Do government transfers reduce poverty in China? Micro evidence from five regions. China Econ. Rev. 51, 59–69. doi: 10.1016/j.chieco.2018.05.009
Wu, H., Ding, S., and Wan, G. (2015). Income inequality and rural poverty in China: focusing on the role of government transfer payments. China Agric. Econ. Rev. 7, 65–85. doi: 10.1108/CAER-01-2014-0005
Zhang, F., Zhang, C., and Hudson, J. (2018). Housing conditions and life satisfaction in urban China. Cities 81, 35–44. doi: 10.1016/j.cities.2018.03.012
Keywords: natural hazards, household income, subjective well-being, propensity score matching, China
Citation: Huang L (2022) Effect of Natural Hazards on the Income and Sense of Subjective Well-Being of Rural Residents: Evidence From Rural China. Front. Ecol. Evol. 10:898557. doi: 10.3389/fevo.2022.898557
Received: 17 March 2022; Accepted: 04 April 2022;
Published: 27 April 2022.
Edited by:
Shaoquan Liu, Institute of Mountain Hazards and Environment (CAS), ChinaReviewed by:
Yongsheng Wang, Institute of Geographic Sciences and Natural Resources Research (CAS), ChinaJing Tan, Southwestern University of Finance and Economics, China
Copyright © 2022 Huang. This is an open-access article distributed under the terms of the Creative Commons Attribution License (CC BY). The use, distribution or reproduction in other forums is permitted, provided the original author(s) and the copyright owner(s) are credited and that the original publication in this journal is cited, in accordance with accepted academic practice. No use, distribution or reproduction is permitted which does not comply with these terms.
*Correspondence: Lei Huang, aHVhbmdsZWkxQGNkdXQuZWR1LmNu