- 1School of Management, Xi’an University of Architecture and Technology, Xi’an, China
- 2Key Laboratory of Regional Sustainable Development Modeling, Institute of Geographic Sciences and Natural Resources Research, Chinese Academy of Sciences (CAS), Beijing, China
- 3School of Public Administration, Xi’an University of Architecture and Technology, Xi’an, China
- 4State Key Laboratory of Green Building in Western China, Xi’an University of Architecture and Technology, Xi’an, China
- 5College of Civil Engineering, Xi’an University of Architecture and Technology, Xi’an, China
China’s energy consumption and its growth trend determine the domestic energy production and supply pattern. The understanding of energy consumption and its changes will help to enhance urban resilience. Based on the improved input-output model and hypothesis extraction model, this paper aims at constructing the energy-industry connection model, analyzing the energy transfer implied in the economic activities of various industrial sectors, and examining the energy transfer effect between the supply-side (SS) and demand-side industry sectors of the Shaanxi Province. The results showed that, in 2017, the energy industrial sector was the most energy consumption industry in Shaanxi Province. The industrial energy sector belonged to the net energy output industrial sector, and the energy products were transferred to other industrial sectors. This paper can provide a scientific basis for the energy SS reform, adjusting the industrial layout of regions in the Yellow River Basin.
Introduction
Since the industrial revolution, greenhouse gas emissions have directly caused climate change and affected our daily lives (He et al., 2014a; Li et al., 2019a; Rahman et al., 2020; Wang F. et al., 2021; Yang et al., 2021b; Bherwani et al., 2022). The 2016 Paris Climate Change Agreement advised countries to take action to reduce greenhouse gas emissions and enhance their response to climate change (Seidler et al., 2020; Iraganaboina and Eluru, 2021). Achieving global temperature rise control and sustainable development goals requires rapid and far-reaching reforms in urban areas including land, energy, industry and building (He et al., 2014b; Li et al., 2019b; As et al., 2020; Estrella Guillen et al., 2021; Häring et al., 2021). In 2020, the United Nations developed the 17 Sustainable Development Goals (SDGs), but significant human infectious diseases represented by COVID-19 made it more challenging to achieve the goals (Christoforidis and Katrakilidis, 2021; Kousar and Shabbir, 2021; Shaikh, 2021). Due to the differences in development stages and development capabilities of countries and regions, the implementation progress of SDGs is not ideal (Gao et al., 2021). We need to speed up the SDG progress since the SDGs are not at the right pace (He et al., 2022). China’s energy consumption and its growth trend did not only determine the changes in the domestic energy production and supply pattern but also had a profound impact on the global energy production and supply (Xiao et al., 2019; Chen et al., 2020; Li J. et al., 2021; Wang R et al., 2021). In 2020, China has proposed a development strategy for CO2 emissions striving to peak before 2030 and striving to achieve carbon neutrality before 2060 (Xu et al., 2021). In the face of a new crisis arising in current and future human activities and climate change, a correct understanding of energy consumption and its growth changes helps to enhance urban resilience (Mensah et al., 2021; Qayyum et al., 2021; Salem, 2021).
The energy consumption of Shaanxi Province in the Yellow River Basin is still mainly fossil energy, especially coal consumption accounts for more than 80% of the overall primary energy consumption in 2016 (Cao et al., 2016; Yu et al., 2020). The imbalance of energy consumption structure objectively leads to low energy utilization efficiency and environmental pollution in Shaanxi Province. Since 2000, the overall urbanization rate of Shaanxi Province has increased from 24.0 to 56.8% (2017), the population density from 173 to 188 per km2, the total resident population reached 38,640 (2018), and the annual gross regional product (GDP) has increased from 180.4 billion RMB to 2,443.8 billion RMB (2018) (Han and Cao, 2021). As the “One Belt and One Road” strategic location and one of the first national low-carbon pilot provinces, an in-depth exploration of energy consumption in Shaanxi Province will be conducive to improving energy utilization efficiency and realizing the sustainable development of energy resources (Mohammed et al., 2021).
According to the specific division of labor within the national economy, industrial sectors are the general term for enterprises and institutions specializing in similar economic activities (Li and Wu, 2022). The current relevant studies have explored the interaction between energy consumption and economic variables among industrial sectors (Cao et al., 2016; Bherwani et al., 2022). However, research on the complete measurement of energy demand from the perspectives of demand and supply is still insufficient. Measuring energy demand from the viewpoint of demand and supply has become a vital issue in this field. Therefore, based on the improved input-output model and hypothesis extraction model, this paper aims at constructing the energy-industry connection model, analyzing the energy transfer implied in the economic activities of various industrial sectors, and examining the energy transfer effect between the supply side (SS) and demand-side (DS) industry sectors of Shaanxi Province.
The remainder of this paper is structured as follows: Section 2 introduces the literature review Section 3 introduces the research idea, research methods, and data sources. Section 4 takes Shaanxi Province as an example and analyzes characteristics of energy demand, implied net energy transfer among industries and energy linkages between industry sectors. Section 5 discusses the applicability of the method and proposes policy suggestions to improve energy efficiency in this article. Section 6 provides the conclusion of this paper and suggests policy recommendations.
Literature Review
Industrial Connection Effect Based on Hypothetical Extraction Model
Input-output table, which can systematically reflect the input-output relationship among various sectors of the national economy, can provide a basis for the study of industrial structure, especially for the determination and verification of national economic plans and the development of various quantitative analyses (Xu et al., 2021). As a part of the national economic accounting system, input-output accounting reflects the economic flow of various sectors of the national economy in the form of an input-output table (Li and Wu, 2022). The hypothetical extraction method (HEM), based on the input-output table, assumes separating an industrial sector from the economic system to compare the changes in the total economic output before and after and to analyze the impact of the industrial sector on the economic system as a whole (Iqbal et al., 2021). The earliest research used the improved HEM for departmental water correlation impact effect calculation to measure the water correlation characteristics among industrial sectors (Duarte et al., 2002; Dyarto and Setyawan, 2021). The existing research mainly adopts a HEM to incorporate water resources, energy, carbon emissions, land use, and other elements into the accounting of economic activities and clarifies the correlation effect of various resources or environmental factors in various industrial sectors (Chen and Chen, 2013; Deng and Zhang, 2016; Chuai et al., 2020; Yang et al., 2021a). For example, Deng et al. (2016) expanded the HEM to the multiregional input-output model, and they studied the correlation effect of Chinese added value trade with other countries and regions and the international correlation effect of implied carbon trade, respectively. Based on the improved hypothetical extraction model, Chen and Xu (2017) explored the direct energy demand and implied energy demand for other sectors from the perspective of the SS and DS, thus analyzing the correlation effect of suggested energy consumption among various industries.
Energy Consumption Based on the Input-Output Model
The relevant research on input-output methods for energy problems is developed mainly from two spatial and temporal dimensions (Hao et al., 2021). Research on spatial dimensions focuses on energy problems at different spatial scales, including direct energy and implied energy accounting at different spatial scales, including international level, national level, regional level, energy transfer quantity calculation, energy efficiency calculation, etc. (Isik et al., 2021; Koondhar et al., 2021; Zafar et al., 2021). As for energy consumption calculation of import and export commodities, there are generally three models, namely, single-regional input-output model, bilateral-regional input-output model, and multiregional input-output model, among which the multiregional input-output model is widely used (Mfb et al., 2021) because it can meet the needs of multiregional calculation (Mfb et al., 2021). For example, Han and Wang (2019) used the multiregional input-output model to measure the added value trade scale and the net value of China and the United States from 1995 to 2009. It used the energy consumption and carbon emission data in the environmental account to calculate the overall level of implied energy. It implied carbon emissions and its industrial structure. Research on the time scale mainly uses the input-output tables of different years to clarify the changing in energy consumption trends. For example, Cao et al. (2016) based the direct energy consumption coefficient and complete energy consumption coefficient in Shaanxi Province in 2002 and input-output tables in 2002, 2007, and 2012. They studied the economic energy efficiency in different years.
As the research deepened, more scholars focused on the energy consumption problem in the industrial dimension (Khan et al., 2021; Wang F. et al., 2021). The final use of the industrial sector and the final energy consumption caused by the intermediate use of the industrial sector are increasingly gaining attention from scholars (Zakari et al., 2021). Wang and Gao (2020) measured the energy consumption of the industrial sector from the perspective of implied energy. They found that there were significant differences in the implied energy intensity between the industrial sector, and 73.85% of the intermediate indicated energy is consumed by production in other industrial sectors downstream of the industrial chain. Yang et al. (2021c) analyzed the water and energy footprints of Heilongjiang based on the value-based input and output table, adding departmental water consumption and energy usage. The study found that the water industrial sector is also a high-energy consumption industrial sector. The top nine industrial sectors of the water resources of the national economic industrial sector of Heilongjiang Province and the total output are the same.
When establishing a model, there are generally two ways, namely, the method based on a value-based input-output equation and the method based on a hybrid input-output model. The value-type energy input-output model is more widely used in the existing literature (Ocal and Aslan, 2013; Qazi and Abushammala, 2021). The value-oriented input-output model is mainly used to analyze the implied energy volume of international trade and inter-regional trade, the energy consumption between different industrial sectors, the impact of household consumption on energy use, etc. (Hatice, 2021). Hybrid input-output models are also mainly used for implied energy calculation in residential consumption, influencing factor analysis of green productivity, energy consumption intensity, or energy consumption (Hatice, 2021; Iheonu et al., 2021). Zhang (2018) started with the energy balance equation and analyzed the essential characteristics and differences of the two models by obtaining the applicable scenarios. The results show that the hybrid energy input-output model can still guarantee the energy balance equation under the new final demand impact. Although the value energy input-output model uses the energy balance equation, it cannot be guaranteed under the new final demand impact. The above studies have explored the interaction between energy consumption and economic variables. The research on the complete measurement of energy demand from the perspectives of demand and supply is still insufficient. Therefore, this paper focuses on constructing the energy-industry connection model, analyzing the energy transfer implied in the economic activities of various industrial sectors, and examining the energy transfer effect between the SS and DS industry sectors.
Study Materials
Study Context
In recent years, the Yellow River Basin has achieved rapid economic development and significant economic strength, which play an essential role in supporting China’s future economic development (Demirtas, 2021; Li C. et al., 2021; Luo et al., 2021). The Yellow River basin is an important ecological function area in China and an ecological barrier in North China. Therefore, the ecological protection of the Yellow River basin is of great significance to ecological security in China (Li et al., 2020; Wu et al., 2020; Yang et al., 2021d). Energy conservation and emission reduction are essential links in realizing high-quality development, which is influenced by the energy structure and industrial structure of various provinces. Provinces in the Yellow River basin face great pressure on emission reduction, and the economic development of all provinces brings great pressure to the ecological environment. For example, the Yellow River basin ecological barrier is damaged, and carbon emissions continue to rise (Chen et al., 2020; Zhang et al., 2021). It is of great significance to the ecological protection and high-quality development of the Yellow River Basin to have a scientific understanding of the energy consumption status and characteristics of the typical areas of the Yellow River Basin. Therefore, Shaanxi Province is selected as the distinct study area of the Yellow River Basin (Figure 1).
Methods
Taking each industrial sector of the economic system of Shaanxi Province as the research object, this paper separates the energy sector, relies on the multiyear input-output table, analyzes the energy transfer between the sectoral economic activities implied in the economic activities of each sector, and compares the correlation effect of energy utilization.
Hypothetical Extraction Method
First, accounting for the energy input matrix of various industrial sectors qi, it is the direct energy utilization coefficient line vector:
where Ei refers to the total sector energy consumption; xi refers to the total output of the corresponding sector; i refers to the industry sector; -i refers to excluding the remaining industrial sector outside the i.
where refers to the total output matrix for the industrial sector; A refers to a direct consumption coefficient matrix; Ai,j refers to the amount of sector i products consumed by j manufacturing units; refers to the Leontief inverse matrix (Bherwani et al., 2022); Δi,j refers to the division j production unit final product complete demand for i division products; refers to the final demand matrix of each industrial sector, including total government consumption, total household consumption, capital, and exports.
Based on this research idea, the four connection effects of the energy connection of the industrial sectors are as follows:
(1) Internal effect (IE). The IE refers to the amount of energy consumed by the industry sector that does not connect with the outside world in the production process. The energy consumption of the means of production itself is provided within the industry sector.
(2) Mixed effect (ME). The ME is the energy consumed by part of the industry sector purchased by other industrial sectors as put into production and the final consumer goods purchased by the industry sector.
(3) Net backward linkage (NBL). NBL means that the industry sector reflects the net energy input brought about to obtain the final demand by using intermediate products and services provided by other sectors.
(4) Net forward linkage (NFL). NFL is the energy consumption of the industry sector products purchased by other sectors. It will not return to produce products, reflecting the net energy output of that sector.
SS and DS Models
From the perspective of energy, the supply system mainly includes two aspects. First, the industrial energy sector itself directly supplies energy to the industrial sector, and second, the direct consumption or indirect consumption of energy in the process of economic activities of various industries. The former needs to be studied independently from the energy supply industrial sector, extraction, and processing of energy. In addition to the direct supply allocation of other industrial sectors, it also inevitably involves the further consumption of energy and the development of the economy and society in nonfossil energy ways such as power and heat. The latter needs to judge the implied trade energy transfer between industries. The implied indirect energy consumption of trade between producers in the economic sector is analyzed, the SS energy consumption and the DS energy consumption are analyzed, the energy input industrial sector and the energy output industrial sector are selected, and the internal connection between the economic system from the direct energy consumption and the indirect energy consumption is explored. Net energy transfer calculation of industrial sectors is as follows: calculate the energy transfer flow in the industrial production sector, clarify the dependence of the industrial sector and other industrial sectors, and identify the key industrial sectors in energy utilization (Cao et al., 2016).
where NTst refers to the net transfer of energy to the t sector for the s sector. If the value of NTst is positive, it indicates that s has net output to t and s is net output; The value of NTst is negative, which indicates that net energy input is from the s sector to the t sector and s is net input sector.
Energy connection between industrial sectors: through the research of energy connection between industrial sectors, we can explore the industrial sector energy demand from the supply and demand parts and scientifically analyze and define the carbon emission responsibility DS energy consumption of the industrial sector, also known as vertically integrated consumption. It is the sum of direct energy and indirect energy demands to meet the needs for productions and services in the industry sector, including IE, ME, NBL, and NFL of the industry sector. The SS consumption, also known as direct consumption, includes not only energy consumption in the production process that meets the final consumption of the industry sector but also energy consumption implied in goods purchased satisfying production and consumption activities. It is the sum of NFL, IE, and ME.
Data Collection
The input-output table can systematically reflect the input-output relationship between various sectors of the national economy, providing a basis for the study of industrial structure, especially for the determination and verification of national economic plans and various quantitative analyses. As an important part of the national economic accounting system, input-output accounting reflects the economic flow of various industrial sectors of the national economy in the form of the input-output table. The input-output table can be divided into regional tables (individual regions) and interregional tables (multiple regions). Since the domestic input-output table has been compiled once in the past 5 years, this paper only studies the transfer of energy demand industrial sectors among various industries in Shaanxi Province in 2007, 2012, and 2017 and then analyzes the energy correlation between industrial economic sectors from the perspective of SS and DS. Among them, the final demand of various industrial sectors, intermediate input, and total output is from Shaanxi Province in 2007, 2012, and 2017. With 2002 as the base period, various comprehensive energy utilization data came from Shaanxi Statistical Yearbook in 2008, 2013, and 2018. Due to some differences in the industry sector classification of the input-output table and statistical Yearbook, we need to form a unified industry classification. This paper divides industrial sectors into the energy sector and nonenergy industrial sector (China Input-Output Association, 2007). Industrial sector indicators will be unified according to caliber, classified into seven categories as follows: agriculture industry (AI), nonenergy industry (NEI), energy industry (EI), building industry (BI), transportation, warehousing, and postal industry (TWPI), wholesale and retail accommodation, catering industry (WRACI), and other service industry (OSI) (Chen and Xu, 2017; Table 1).
Results
Energy Demand Characteristics in Shaanxi Province
By accounting for the energy consumption of the industrial economic sector of Shaanxi Province (Table 2 and Figure 2), in 2017, the largest energy consumption industry in Shaanxi Province, the comprehensive energy consumption was 4,161.05 thousand tce, accounting for approximately 45% of the total consumption of the whole industry. Second, the nonenergy sector accounts for approximately 30% of the total consumption of the total industry. The construction industry is the industry with the most minor energy consumption in Shaanxi Province, with a comprehensive energy consumption of 175.76 million tce accounting for approximately 2% of the total consumption of the whole industry, followed by the agricultural industrial sector, accounting for approximately 3% of the total consumption of the entire industry. By analyzing the consumption of 10,000-yuan output value, the energy intensity of transportation, storage, and postal industry is 0.87tce/10,000 yuan, which is higher than the average energy consumption intensity of all industries by 0.33 tce/10,000 yuan, and the energy intensity of the energy sector is 0.61 tce/10,000 yuan. The energy intensity of wholesale and retail accommodation and catering, other service industries, nonenergy industrial sectors, and agricultural sectors is slightly lower than the average level. In comparison, the energy intensity of the construction industry is lower than 0.10 tce/10,000 yuan. Therefore, the high the energy intensity of transportation, warehousing, postal industry is, the high the industrial energy sectors are, directly leading to the increase in energy consumption in Shaanxi Province.
Based on the analysis of energy consumption in 2007, 2012, and 2017 (Figure 3), in the past 10 years, energy consumption in Shaanxi Province continued to grow, with energy intensity decreasing from 2.72 tce per 20,000 yuan to 0.33 tce per 10,000 yuan in 2017 by 88%, among which the energy sector has the most significant decline, reached 95%, the improvement of energy efficiency of the energy industry sector, thus promoting the energy intensity of the decline in Shaanxi Province in the past 10 years. Unlike the reduction of energy intensity in other industries, the energy intensity of other service industries is gradually increasing. Energy intensity increased from 0.20 tce per 10,000 in 2007 to 0.23 tce per 10,000 in 2017, indicating that the change rate of energy demand in other service industries increased the output value, while other service industries are in small energy consumption industrial sectors (approximately 5% of total energy consumption). The energy utilization intensity is small, reflecting the intensity of energy conservation and emission reduction in Shaanxi Province, and the energy use efficiency is increasing year by year.
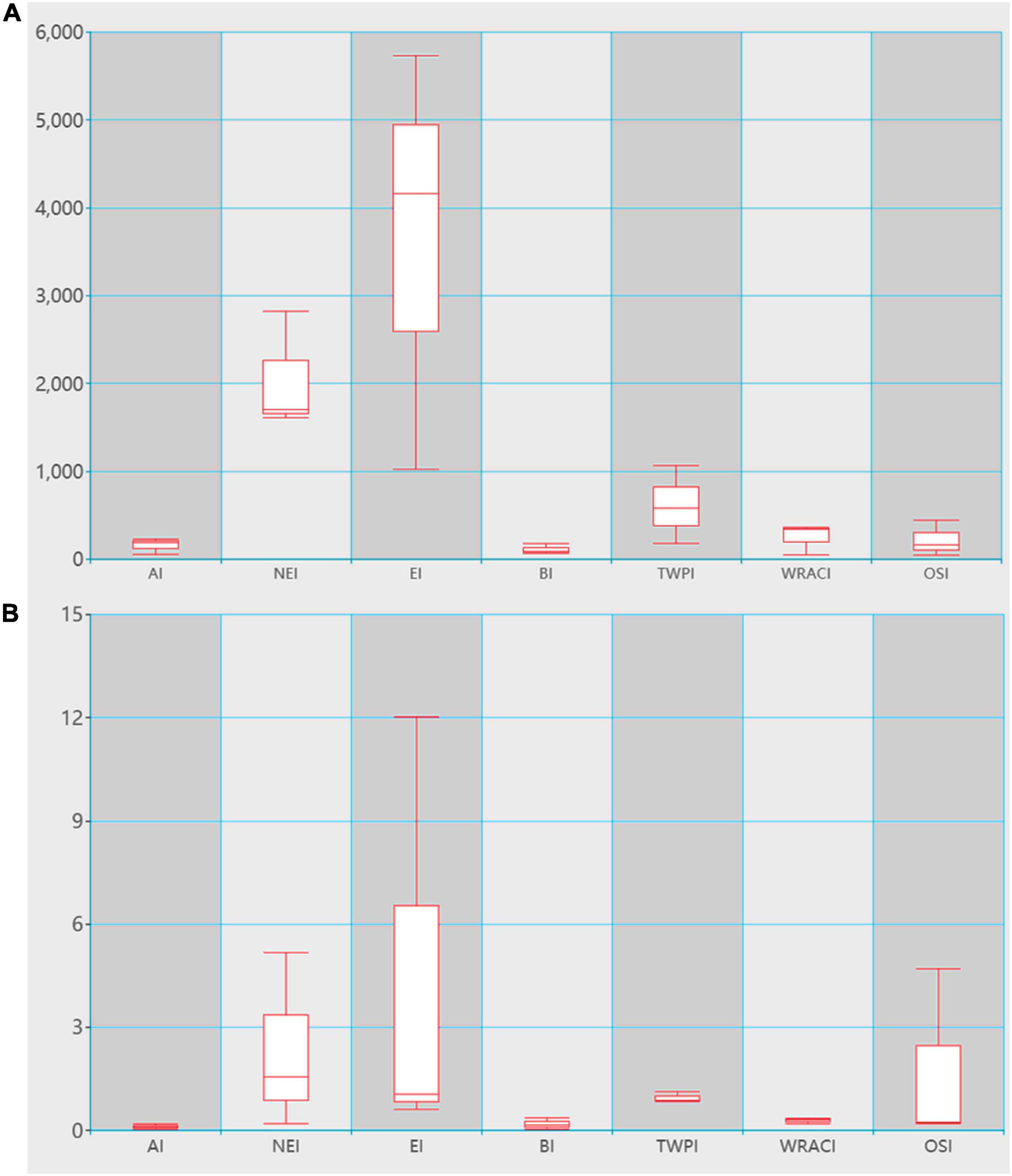
Figure 3. (A) Comprehensive energy consumption of various industries in Shaanxi Province in 2007, 2012, and 2017; (B) energy consumption of 10,000 yuan in Shaanxi industries in 2007, 2012, and 2017.
Implicit Net Energy Transfer Among Industries in Shaanxi Province
According to Eq (14), taking data in 2017 as an example, it can indicate the quantity and specific direction of the implied net transfer of energy in Shaanxi Province among various industries (Figure 4). The energy sector is the net energy output sector with the most net transfer to the nonenergy industry sector, 35.751 million tce, followed by the net transfer to construction, 14.583 million tce. The construction industry is the energy net input industrial sector. In addition to the energy sector, the nonenergy sector transfer more implied energy transfer from the nonenergy industry sector to the transportation storage and postal industrial sector, 2.164 million tce.
Energy Connection of Industrial Sector in Shaanxi Province
According to Eq (10)–(16), IE, ME, NBL, and NFL in 2012 were calculated (Figure 5). Consumer energy in the agricultural sector IE is 129,600 tce. As for the raw material supply industrial sector, ME consumption energy accounts for 80% of the total DS energy consumption. It indicates that the agricultural sector is transferred to other industrial sectors, and the implied energy is 36,745.700 tce. Most of them transferred to other industrial sectors did not return. The nonenergy sector IE, as the direct energy supply sector, ranks first, with 26,973 million tce, accounting for approximately 10% of DS energy consumption. It indicates that most of the energy needed by the nonenergy industry sector to meet their final demand comes from product exchanges within the industry. The energy industry sector has the most NFL in all industries, ranking first at 66.94499 million tce, and the energy industry sector at 1,243,543 million tce, representing approximately 73% of DS energy consumption. It indicates that more energy implied by the energy industry is transferred to other sectors, and it has the most NBL of all industries. The construction industry, as the net energy input sector, has 82% of the total DS energy consumption for the exchange of 171,002 million tce, having an output of 72,900 tce, transferred to other sectors. In the transportation warehousing and postal industry, energy NFL accounts for the most minor proportion of SS energy consumption, at 8%. The transportation warehousing and postal industry have less implied energy transfer to the remaining industries. In wholesale and retail accommodation catering and agricultural sector, NBL is small compared with NFL, showing certain equilibrium. Net implied energy transfer in other services (net transfer energy volume minus net transferred energy volume) is −3.4554 million tce, which is the net input energy sector.
Discussion
The HEM, based on the input-output table, assumes that an industrial sector is separated from the economic system, compares the changes in the total output of the economic system, and analyzes the impact of the industrial sector on the whole economic system (Chen and Xu, 2017). However, the HEM cannot analyze IE, ME, NBL, and NFL. Based on the two methods of constructing the input-output model, the hybrid energy input-output model can still guarantee the establishment of the energy balance equation under the new final demand impact (Cao et al., 2016; Bherwani et al., 2022). Although the value-based energy input-output model uses the energy balance equation in the initial construction process, it cannot guarantee that the energy balance equation is established under the new final demand impact (Xu et al., 2021).
This paper analyzes the energy consumption inside and between industrial sectors from the economic system, explores the direct energy demand of other industries from the SS and DS, and analyzes the correlation effect of implied energy consumption (Figure 6). The method is applicable to identify the key industrial sectors in energy utilization and the implied energy flow law. It can be extended to other regions, clarifying the key energy consumption industrial sectors in the economic system. To speed up the SDG progress (He et al., 2022), it is not only to improve the supply efficiency of energy supply in the energy sector but also to solve the existing structural imbalance in the energy industry from the root cause. Besides, it is essential to address the characteristics of energy consumption, energy use efficiency, and social and economic conditions in various industries. It is also necessary to improve the energy utilization efficiency of various industries, especially the industrial industry, and coordinate the energy demand on the SS and the DS according to the characteristics of energy consumption, energy use efficiency, and social and economic conditions in various industries. Based on the above understandings, energy supply and economic, social, and environmental development should be planned in a coordinated manner.
Based on the above findings of this paper, we can formulate policy suggestions to improve energy efficiency from the aspects of industrial structure adjustment and technology improvement policy formulation. As a low energy consumption sector, the reduction of the full energy consumption intensity of the agricultural industry is mainly due to the improvement of energy production technology. Therefore, energy utilization could be improved by adjusting the energy consumption structure and the improvement of energy utilization technology. As a high-energy consumption department, the reduction of energy consumption intensity is mainly due to energy production technology. Energy efficiency could be improved by adjusting the energy consumption structure and energy utilization technology. In transportation, warehousing, and post-industry as a high-energy consumption department, the reduction of its full energy consumption intensity is mainly due to the net output structure effect and energy technology effect. While adjusting the energy consumption structure and improving energy utilization technology, we can pay attention to adjusting the input and output structures of its products, reduce the output scale of high energy consumption and low value-added products, and output more products with high value-added. As a low-energy sector, the reduction of total energy consumption intensity in the wholesale, retail, accommodation, and catering industries can be attributed to many reasons, such as the production technology effect, final demand structure effect, and energy technology effect, among which the consumption structure effect contributes to the most. As a low-energy sector, the reduction of full energy consumption intensity of other service industries mainly comes from the effect of energy technology and final demand structure. It can improve energy utilization efficiency from the aspects of introducing new energy technology, guiding rational public consumption and rational investment.
Conclusion
Based on the improved HEM, taking the industrial sectors of Shaanxi Province as the research object, relying on the multi-annual input-output table, this paper analyzes the energy transfer implied among industrial sectoral economic activities, compares the energy utilization correlation effect, and provides a scientific basis for the energy SS reform and adjusting the industrial layout of Shaanxi Province.
In 2017, the energy industrial sector was the most energy consumption industry in Shaanxi Province. The construction industry was the most minor energy consumption industry in Shaanxi Province, with high energy intensity in the transportation, warehousing, postal industry, and energy industrial sectors. The energy sector belongs to the net energy net output sector, followed by the net transfer of the energy sector to the construction industry is the net energy input sector. In addition to the energy sector and the nonenergy sector, there are more implied energy transfers from the nonenergy industry sector to the transportation, storage, and postal sector. The agricultural sector moved to other sectors, and recycled products implied more energy. Most of the energy needed by the nonenergy industry sector to meet their ultimate needs comes from product exchanges within the industry. The transfer of energy industry sectors to other sectors and recycling products implies more energy. Construction and other services belong to the net energy input sector. Transportation warehousing and postal industries have less indicated energy transfer to the remaining industries and return. Wholesale and retail accommodation, catering, and agricultural industrial sectors show a certain balance.
Data Availability Statement
The original contributions presented in this study are included in the article/supplementary material, further inquiries can be directed to the corresponding author/s.
Author Contributions
JX and XL contributed to the conception of this study. DS and CL performed the experiment. JX and CL contributed significantly to the analysis and manuscript preparation. JX and CL performed the data analyses and wrote the manuscript. XL and ZW helped perform the analysis with constructive discussions. All authors contributed to the article and approved the submitted version.
Funding
This study has been supported by the Shaanxi Province Soft Science Research Project (2020KRM197), Social Science Fund of Shaanxi Province (2020R051), Xi’an Social Science Planning Fund (22JX72), Shaanxi Province Soft Science Research Project (2021KRM093), Independent Research and Development project of State Key Laboratory of Green Building in Western China (LSZZ202215), and Joint Project of Major Theoretical and Practical Problems in the Social Sciences of Shaanxi Province (Grant no. 2022ND0429).
Conflict of Interest
The authors declare that the research was conducted in the absence of any commercial or financial relationships that could be construed as a potential conflict of interest.
The reviewer YZ declared a shared affiliation with the several authors JX, XL, CL, and ZW to the handling editor at the time of review.
Publisher’s Note
All claims expressed in this article are solely those of the authors and do not necessarily represent those of their affiliated organizations, or those of the publisher, the editors and the reviewers. Any product that may be evaluated in this article, or claim that may be made by its manufacturer, is not guaranteed or endorsed by the publisher.
References
As, A., Jmm, B., Bh, B., and Kba, B. (2020). Estimating residential building energy consumption using overhead imagery. Appl. Energy 280:116018. doi: 10.1016/j.apenergy.2020.116018
Bherwani, H., Nair, M., Niwalkar, A., Balachandran, D., and Kumar, R. (2022). Application of Circular Economy Framework for Reducing the Impacts of Climate Change: a Case Study from India on the Application of Carbon and Materials Footprint Nexus. Energy Nexus 5:100047. doi: 10.1016/j.nexus.2022.100047
Cao, D., Liu, J., and Zhang, S. (2016). Study on Energy Efficiency of Shaanxi Province Based on Input-output Technology. J. Xidian University 26, 36–44.
Chen, B., and Chen, S. (2013). Life cycle assessment of coupling household biogas production to agricultural industry: a case study of biogas-linked persimmon cultivation and processing system. Energy Policy 62, 707–716. doi: 10.1016/j.enpol.2013.07.084
Chen, C., Pinar, M., and Stengos, T. (2020). Renewable energy consumption and economic growth nexus: evidence from a threshold model. Ener. Policy 139:111295.
Chen, L., and Xu, L. (2017). Energy consumption of Guangdong based on the industry linkage analysis. China Environ. Sci. 37, 3972–3980.
China Input-Output Association. (2007). The Inter-Industrial Linkages of Energy Sectors in China-Study on the Improved Structural Coefficients by Using2002Input-Output Table of China. Stat. Res. 24, 3–6.
Christoforidis, T., and Katrakilidis, C. (2021). The dynamic role of institutional quality, renewable and non-renewable energy on the ecological footprint of OECD countries: do institutions and renewables function as leverage points for environmental sustainability? Environ. Sci. Pollut. Res. 28, 28038–28057. doi: 10.1007/s11356-021-13877-8
Chuai, X., Lu, Q., Huang, X., Gao, R., and Zhao, R. (2020). China’s construction industry-linked economy-resources-environment flow in international trade. J. Clean. Prod. 278:123990. doi: 10.1016/j.jclepro.2020.123990
Demirtas, M. (2021). Evaluation of energy use and carbon dioxide emissions from the consumption of fossil fuels and agricultural chemicals for paste tomato cultivation in the Bursa region of Turkey. Environ. Sci. Pollut. Res. 28, 27132–27146. doi: 10.1007/s11356-021-12593-7
Deng, G., and Zhang, Z. (2016). A Study on the International Relating Effect of China’s Embodied Carbon Trade: based on Generalized Hypothetical Extraction Method. Mod. Finance Econ. J. Tianjin University Finance Econ. 36, 102–113.
Deng, G., Zhang, Z., and Ren, S. (2016). Effect of the Value-added Trade of China in the Global Value Chains:Based on the Generalized Hypothetical Extraction Method. Stat. Inf. Forum 31, 46–51.
Duarte, R., Sanchez-Choliz, J., and Bielsa, J. (2002). Water use in the Spanish economy: an input-output approach. Ecol. Econ. 43, 71–85. doi: 10.1016/S0921-8009(02)00183-0
Dyarto, R., and Setyawan, D. (2021). Understanding the political challenges of introducing a carbon tax in Indonesia. Int. J. Environ. Sci. Technol. 18, 1479–1488. doi: 10.1007/s13762-020-02925-4
Estrella Guillen, E., Samuelson, H. W., and Vohringer, C. (2021). The impact of cultural assumptions on simulated energy, comfort, and investment returns of design decisions in two desert climates. Build. Simul. 14, 931–944. doi: 10.1007/s12273-020-0718-y
Gao, X., Zhang, X., and He, Z. (2021). Emergence of the urban goal and its practice: background and progress of the SDG11. Chin. Popul. Resour. Environ. 2021, 144–154.
Han, X., and Cao, T. (2021). Study on ecological environment quality evaluation of the energy consumption pollution treatment in industrial parks. Environ. Sci. Pollut. Res. 28, 28038–28057. doi: 10.1007/s11356-020-10147-x
Han, Z., and Wang, G. (2019). Estimation of embodied energy and carbon emissions in Sino-U.S.trade based on Multi-Region Input-Output Model. Clim. Chang. Res. 015, 416–426.
Hao, W., Rasul, F., Bhatti, Z., Hassan, M. S., Ahmed, I., and Asghar, N. (2021). A technological innovation and economic progress enhancement: an assessment of sustainable economic and environmental management. Environ. Sci. Pollut. Res. 28, 28585–28597. doi: 10.1007/s11356-021-12559-9
Häring, I., Fehling-Kaschek, M., Miller, N., Faist, K., Ganter, S., and Srivastava, K. (2021). A performance-based tabular approach for joint systematic improvement of risk control and resilience applied to telecommunication grid, gas network, and ultrasound localization system. Environ. Syst. Decis. 41, 286–329. doi: 10.1007/s10669-021-09811-5
Hatice, G. (2021). Renewable energy consumption policy in Turkey: an energy extended input-output analysis. Renew. Energy 175, 783–796. doi: 10.1016/j.renene.2021.05.025
He, B., Wang, J., Zhu, J., and Qi, J. (2022). Beating the urban heat: situation, background, impacts and the way forward in China. Renew. Sustain. Energy Rev. 161:112350. doi: 10.1016/j.rser.2022.112350
He, B. J., Yang, L., and Ye, M. (2014a). Building energy efficiency in China rural areas: situation, drawbacks, challenges, corresponding measures and policies. Sustain. Cities Soc. 11, 7–15. doi: 10.1016/j.scs.2013.11.005
He, B. J., Yang, L., Ye, M., Mou, B., and Zhou, Y. (2014b). Overview of rural building energy efficiency in China. Energy Policy 69, 385–396. doi: 10.1016/j.enpol.2014.03.018
Iheonu, C. O., Anyanwu, O. C., Odo, O. K., and Nathaniel, S. P. (2021). Does economic growth, international trade, and urbanization uphold environmental sustainability in sub-Saharan Africa? Insights from quantile and causality procedures. Environ. Sci. Pollut. Res. 28, 28222–28233. doi: 10.1007/s11356-021-12539-z
Iqbal, N., Sakhani, M. A., Khan, A. R., Atiq-Ur-Rehman, Ajmal, Z., and Khan, M. Z. (2021). Socioeconomic impacts of domestic biogas plants on rural households to strengthen energy security. Environ. Sci. Pollut. Res. 28, 27446–27456. doi: 10.1007/s11356-021-12633-2
Iraganaboina, N. C., and Eluru, N. (2021). An examination of factors affecting residential energy consumption using a multiple discrete continuous approach. Energy Build. 240:110934. doi: 10.1016/j.enbuild.2021.110934
Isik, C., Ongan, S., Ozdemir, D., Ahmad, M., and Ongan, A. (2021). The increases and decreases of the environment kuznets curve (ekc) for 8 OECD countries. Environ. Sci. Pollut. Res. 28, 28535–28543. doi: 10.1007/s11356-021-12637-y
Khan, I., Hou, F., Zakari, A., and Tawiah, V. (2021). The dynamic links among energy transitions, energy consumption, and sustainable economic growth: a novel framework for IEA countries. Energy 222:119935. doi: 10.1016/j.energy.2021.119935
Koondhar, M. A., Udemba, E. N., Cheng, Y., Khan, Z. A., and Kong, R. (2021). Asymmetric causality among carbon emission from agriculture, energy consumption, fertilizer, and cereal food production -a nonlinear analysis for Pakistan. Sustain. Energy Technol. Assess. 45:101099. doi: 10.1016/j.seta.2021.101099
Kousar, S., and Shabbir, A. (2021). Analysis of environmental degradation mechanism in the nexus among energy consumption and poverty in Pakistan. Environ. Sci. Pollut. Res. 28, 27528–27541. doi: 10.1007/s11356-020-12140-w
Li, C., Gao, X., He, B.-J., Wu, J., and Wu, K. (2019a). Coupling Coordination Relationships between Urban-industrial Land Use Efficiency and Accessibility of Highway Networks: evidence from Beijing-Tianjin-Hebei Urban Agglomeration, China. Sustainability 11:1446. doi: 10.3390/su11051446
Li, C., Gao, X., Wu, J., and Wu, K. (2019b). Demand prediction and regulation zoning of urban-industrial land: evidence from Beijing-Tianjin-Hebei Urban Agglomeration, China. Environ. Monitor. Assess. 191:412. doi: 10.1007/s10661-019-7547-4
Li, C., Hu, H., Deng, L., Liu, Y., and Wang, Z. (2021). Structural decoupling the sectoral growth from complete energy consumption in china. Energy Strategy Rev. 34:100634. doi: 10.1016/j.esr.2021.100634
Li, C., and Wu, K. (2022). An input–output analysis of transportation equipment manufacturing industrial transfer: evidence from Beijing-Tianjin-Hebei region, China. Growth Change 53, 91–111. doi: 10.1111/grow.12571
Li, G., Jiang, C., Zhang, Y., and Jiang, G. (2020). Whether land greening in different geomorphic units are beneficial to water yield in the yellow river basin? Ecol. Indic. 120:106926. doi: 10.1016/j.ecolind.2020.106926
Li, J., Sun, W., Li, M., and Meng, L. (2021). Coupling coordination degree of production, living and ecological spaces and its influencing factors in the yellow river basin. J. Clean. Prod. 298:126803. doi: 10.1016/j.jclepro.2021.126803
Luo, X., Yang, J., Sun, W., and He, B. (2021). Suitability of human settlements in mountainous areas from the perspective of ventilation: a case study of the main urban area of Chongqing. J. Clean. Prod. 310:127467. doi: 10.1016/j.jclepro.2021.127467
Mensah, H., Ahadzie, D. K., Takyi, S. A., and Amponsah, O. (2021). Climate change resilience: lessons from local climate-smart agricultural practices in Ghana. Energy Ecol. Environ. 6, 271–284. doi: 10.1007/s40974-020-00181-3
Mfb, A., Mab, A., Msb, C., Us, D., and Xuan, V. (2021). Unveiling the heterogeneous impacts of environmental taxes on energy consumption and energy intensity: empirical evidence from OECD countries. Energy 226:120366. doi: 10.1016/j.energy.2021.120366
Mohammed, N. A. A., Xianhui, G., and Shah, S. A. A. (2021). Non-oil economic transition for economic and environmental sustainability in Saudi Arabia: a multi-factor analysis under fuzzy environment. Environ. Sci. Pollut. Res. 28, 56219–56233. doi: 10.1007/s11356-021-14304-8
Ocal, O., and Aslan, A. (2013). Renewable energy consumption–economic growth nexus in turkey. Renew. Sustain. Energy Rev. 28, 494–499. doi: 10.1016/j.rser.2013.08.036
Qayyum, M., Yu, Y., and Li, S. (2021). The impact of economic complexity on embodied carbon emission in trade: new empirical evidence from cross-country panel data. Environ. Sci. Pollut. Res. 28, 54015–54029. doi: 10.1007/s11356-021-14414-3
Qazi, W. A., and Abushammala, M. F. M. (2021). The analysis of electricity production and greenhouse-gas emission reduction from municipal solid waste sector in Oman. Int. J. Environ. Sci. Technol. 18, 1395–1406. doi: 10.1007/s13762-020-02871-1
Rahman, M. M., Hosan, S., Karmaker, S. C., Chapman, A. J., and Saha, B. B. (2020). The effect of remittance on energy consumption: panel cointegration and dynamic causality analysis for south asian countries. Energy 220:119684. doi: 10.1016/j.energy.2020.119684
Salem, H. S. (2021). Evaluation of the stone and marble industry in palestine: environmental, geological, health, socioeconomic, cultural, and legal perspectives, in view of sustainable development. Environ. Sci. Pollut. Res. 28, 28058–28080. doi: 10.1007/s11356-021-12526-4
Seidler, J., Strugatchi, J., Grtner, T., and Waldvogel, S. R. (2020). Does electrifying organic synthesis pay off? The energy efficiency of electro-organic conversions. MRS Energy Sustain. 7:42. doi: 10.1557/mre.2020.42
Shaikh, I. (2021). Impact of covid-19 pandemic on the energy markets. Econ. Change Restruct. 55, 433–484. doi: 10.1007/s10644-021-09320-0
Wang, F., and Gao, C. (2020). Measurement, Decomposition, and Cross-Border Transfer of Embodied Energy of China’s Industrial Sectors: based on the CRIO Model. Inquiry Econ. Issues 458, 5–15.
Wang, F., Xie, J., Wu, S., Li, J., and Zhang, L. (2021). Life cycle energy consumption by roads and associated interpretative analysis of sustainable policies. Renew. Sustain. Energy Rev. 141:110823. doi: 10.1016/j.rser.2021.110823
Wang, R., Lu, S., Feng, W., and Xu, B. (2021). Tradeoff between heating energy demand in winter and indoor overheating risk in summer constrained by building standards. Build. Simul. 14, 987–1003. doi: 10.1007/s12273-020-0719-x
Wu, J., Abban, O. J., Boadi, A. D., Haris, M., and Addo, A. A. (2020). Exploring the relationships among CO2 emissions, urbanization, economic growth, economic structure, energy consumption, and trade along the BRI based on income classification. Energy Ecol. Environ. 6, 213–231. doi: 10.1007/s40974-020-00176-0
Xiao, H., Ma, Z., Zhang, P., and Liu, M. (2019). Study of the impact of energy consumption structure on carbon emission intensity in China from the perspective of spatial effects. Nat. Hazards 99, 1365–1380. doi: 10.1007/s11356-021-16619-y
Xu, X., Zhang, N., Zhao, D., and Liu, C. (2021). The effect of trade openness on the relationship between agricultural technology inputs and carbon emissions: evidence from a panel threshold model. Environ. Sci. Pollut. Res. 28, 9991–10004. doi: 10.1007/s11356-020-11255-4
Yang, J., Wang, Y., Xue, B., Li, Y., Xiao, X., Xia, J., et al. (2021b). Contribution of urban ventilation to the thermal environment and urban energy demand: different climate background perspectives. Sci. Total Environ. 795:148791. doi: 10.1016/j.scitotenv.2021.148791
Yang, J., Ren, J., Sun, D., Xiao, X., and Li, X. (2021a). Understanding land surface temperature impact factors based on local climate zones. Sustain. Cities Soc. 69:102818. doi: 10.1016/j.scs.2021.102818
Yang, J., Yang, Y., Sun, D., Jin, C., and Xiao, X. (2021c). Influence of urban morphological characteristics on thermal environment. Sustain. Cities Soc. 72:103045. doi: 10.1016/j.scs.2021.103045
Yang, Y., Qiu, Y., Wang, X., Liu, H., Zhang, J., Li, H., et al. (2021d). Analysis of Water Resources and Energy Consumption Path in Heilongjiang Province. Water Power 47, 18–23.
Yu, C. A., Mz, A., Jl, B., Qian, Z. C., and Wm, D. (2020). Evaluation of ecological city and analysis of obstacle factors under the background of high-quality development: taking cities in the yellow river basin as examples. Ecol. Indic. 118:106771. doi: 10.1016/j.ecolind.2020.106771
Zafar, M. W., Sinha, A., Ahmed, Z., Qin, Q., and Zaidi, S. (2021). Effects of biomass energy consumption on environmental quality: the role of education and technology in asia-pacific economic cooperation countries. Renew. Sustain. Energy Rev. 142:110868. doi: 10.1016/j.rser.2021.110868
Zakari, A., Toplak, J., Ibtissem, M., Dagar, V., and Khan, M. K. (2021). Impact of nigeria’s industrial sector on level of inefficiency for energy consumption: fisher ideal index decomposition analysis. Heliyon 7:e06952. doi: 10.1016/j.heliyon.2021.e06952
Zhang, H. (2018). Choosing energy input-output models: hybrid type or monetary type. Econ. Theory Bus. Manag. 334, 104–114.
Keywords: supply side, demand-side, energy linkage, hypothetical extraction method, China
Citation: Xia J, Liu X, Sun D, Li C and Wang Z (2022) Energy Consumption Connection of Industrial Sector Based on Industrial Link Theory: A Case Study of China. Front. Ecol. Evol. 10:897574. doi: 10.3389/fevo.2022.897574
Received: 16 March 2022; Accepted: 23 May 2022;
Published: 06 July 2022.
Edited by:
Xinli Ke, Huazhong Agricultural University, ChinaReviewed by:
Ying Zhu, Xi’an University of Architecture and Technology, ChinaBao-Jie He, Chongqing University, China
Bing Xue, Institute of Applied Ecology (CAS), China
Copyright © 2022 Xia, Liu, Sun, Li and Wang. This is an open-access article distributed under the terms of the Creative Commons Attribution License (CC BY). The use, distribution or reproduction in other forums is permitted, provided the original author(s) and the copyright owner(s) are credited and that the original publication in this journal is cited, in accordance with accepted academic practice. No use, distribution or reproduction is permitted which does not comply with these terms.
*Correspondence: Dongqi Sun, c3VuZHFAaWdzbnJyLmFjLmNu; Chenxi Li, eHl3Z2xjeEAxNjMuY29t