- 1Department of Biological Sciences, Rutgers University – Newark, Newark, NJ, United States
- 2Life Sciences Section, Negaunee Integrative Research Center, Field Museum, Chicago, IL, United States
Over the past decade, museum genomics studies have focused on obtaining DNA of sufficient quality and quantity for sequencing from fluid-preserved natural history specimens, primarily to be used in systematic studies. While these studies have opened windows to evolutionary and biodiversity knowledge of many species worldwide, published works often focus on the success of these DNA sequencing efforts, which is undoubtedly less common than obtaining minimal or sometimes no DNA or unusable sequence data from specimens in natural history collections. Here, we attempt to obtain and sequence DNA extracts from 115 fresh and 41 degraded samples of homalopsid snakes, as well as from two degraded samples of a poorly known snake, Hydrablabes periops. Hydrablabes has been suggested to belong to at least two different families (Natricidae and Homalopsidae) and with no fresh tissues known to be available, intractable museum specimens currently provide the only opportunity to determine this snake’s taxonomic affinity. Although our aim was to generate a target-capture dataset for these samples, to be included in a broader phylogenetic study, results were less than ideal due to large amounts of missing data, especially using the same downstream methods as with standard, high-quality samples. However, rather than discount results entirely, we used mapping methods with references and pseudoreferences, along with phylogenetic analyses, to maximize any usable molecular data from our sequencing efforts, identify the taxonomic affinity of H. periops, and compare sequencing success between fresh and degraded tissue samples. This resulted in largely complete mitochondrial genomes for five specimens and hundreds to thousands of nuclear loci (ultra-conserved loci, anchored-hybrid enrichment loci, and a variety of loci frequently used in squamate phylogenetic studies) from fluid-preserved snakes, including a specimen of H. periops from the Field Museum of Natural History collection. We combined our H. periops data with previously published genomic and Sanger-sequenced datasets to confirm the familial designation of this taxon, reject previous taxonomic hypotheses, and make biogeographic inferences for Hydrablabes. A second H. periops specimen, despite being seemingly similar for initial raw sequencing results and after being put through the same protocols, resulted in little usable molecular data. We discuss the successes and failures of using different pipelines and methods to maximize the products from these data and provide expectations for others who are looking to use DNA sequencing efforts on specimens that likely have degraded DNA.
Life Science Identifier (Hydrablabes periops): urn:lsid:zoobank.org:pub:F2AA44 E2-D2EF-4747-972A-652C34C2C09D.
Introduction
Advances in DNA sequencing technologies have allowed for the rapid accumulation of genomic or subgenomic datasets with thousands of loci. These datasets have provided opportunities to determine genomic correlates of phenotypic traits (Card et al., 2019; Stuckert et al., 2021), understand the links between recombination landscapes and genetic diversity (Schield et al., 2020), and reconstruct evolutionary histories in megadiverse groups (Hime et al., 2021). Research on the latter topic in particular, focusing on the discipline of systematics, has included continuously growing datasets to discover undescribed diversity in poorly studied taxa (Weinell and Brown, 2018), time-calibrate the diversification of extant groups (Álvarez-Carretero et al., 2021), and infer historical biogeography in comparative frameworks to better understand patterns of biodiversity (de Bruyn et al., 2014). However, these research findings are only possible due to the now-common practice of explicitly preserving fresh tissues upon collection of study organisms for subsequent DNA/RNA analyses. There remain large gaps of knowledge for thousands of organisms only known from museum specimens in natural history collections, often collected before practices of tissue preservation and DNA extraction. For centuries, vertebrates have been fixed using formalin or ethanol (Simmons, 2014), degrading the DNA by shearing, cross-linking, and deamination/depurination (Zimmermann et al., 2008; Campos and Gilbert, 2012; Do and Dobrovic, 2015), typically leading to DNA quality insufficient for sequencing, especially for systematic studies. The current era of genomics has been met with several protocols to obtain useable DNA from these intractable museum specimens (e.g., Rohland et al., 2004; Hykin et al., 2015; Ruane and Austin, 2017; O’Connell et al., 2021; reviewed in Ruane, 2021). As a result, the taxonomic identity and phylogenetic placement of poorly known snakes (Allentoft et al., 2018; Deepak et al., 2018), lizards (Hykin et al., 2015; McGuire et al., 2018), frogs (Rancilhac et al., 2020), salamanders (Pyron et al., 2022), crustaceans (France and Kocher, 1996), spiders (Wood et al., 2018), and birds (McCormack et al., 2016) have been successful, and with some studies on birds (Linck et al., 2017; Tsai et al., 2019) and mammals (Roycroft et al., 2021) obtaining levels of informativeness adequate to determine biogeographic histories and extinction patterns. Studies involving ‘museum genomics’ research involve materials from traditional museums and cryogenic collections, as well as the respective supporting infrastructure (Card et al., 2021). In this study, we use the term ‘museum genomics’ to refer to the more focused goal of obtaining useable DNA from often intractable, preserved museum specimens, which has undoubtedly created new directions for what is possible with DNA from voucher specimens and allowed us to leverage these data for biodiversity knowledge and evolutionary inference. However, many attempts at these endeavors still result in less than optimal (and frequently unusable) results, and studies often only report the successes that are obtained, leaving expectations of data quality, processing, and manipulation as a black box in such efforts.
While often viewed as a single task, the success of acquiring DNA from preserved museum specimens and obtaining DNA raw reads of sufficient quality for systematic studies each present separate difficulties. Hot alkali treatments (Campos and Gilbert, 2012; Hykin et al., 2015), heavy use of proteinase-K (Ruane and Austin, 2017), and development of digestion buffers (Allentoft et al., 2015) have all been used with varying success to break formalin cross-links and retrieve DNA from fixed specimens. However, different tissues (e.g., skin, liver, muscle, and bone) may yield varying DNA concentrations upon extraction (Appleyard et al., 2021; Zacho et al., 2021), and the lysis of soft tissue using enzymes like proteinase-K may be unsuccessful depending on the age, storage conditions, and preservation history of the source tissue. Even if DNA is extracted from intractable specimens, decreases in number and uneven distribution of mapped reads to reference genomes (Hykin et al., 2015; Allentoft et al., 2018), short fragment lengths, and low numbers of loci (Ruane and Austin, 2017) are commonly reported. Reduced-quality DNA from museum specimens is expected, but issues when using bioinformatic pipelines, the efficacy of using different types of loci and approaches for locus acquisition, and expectations of phylogenetic placements are seldom discussed. Additionally, when bioinformatic-related problems arise using published software, not all researchers have the expertise to edit, troubleshoot, or modify the source code. Predicting the analytical difficulties from museum genomics studies and understanding how data from degraded DNA can be processed will allow for higher success rates in understanding the biological histories of taxa only known from natural history collections. With increased global extinction rates (Pimm et al., 2014; De Vos et al., 2015) due to anthropogenic-related causes, it is important to elucidate the systematics and biodiversity of poorly-studied, yet ecologically important, groups or taxa that are rare or even possibly extinct.
Snakes are an excellent system for studying evolutionary processes (Esquerré et al., 2020; Schield et al., 2020; Westeen et al., 2020; Burbrink et al., 2021), and the utilization of preserved museum specimens has expanded our knowledge on both extant and extinct diversity (Ruane and Austin, 2017; Allentoft et al., 2018; Zacho et al., 2021). Southeast Asia in particular includes a diverse assemblage of snakes with multiple endemic lineages, many concentrated in biodiversity hotspots, which have been affected by the region’s complex geological history (Hall, 2009; de Bruyn et al., 2014). Borneo, one of the largest islands in the world, harbors 160+ species of snakes, including multiple species that are only known from one to a few museum specimens and for which almost no natural history information is available (Stuebing et al., 2014; Das, 2018; Uetz et al., 2021). One such taxon is Hydrablabes, a genus consisting of two small-sized, aquatic snake species endemic to Borneo: Hydrablabes periops and Hydrablabes praefrontalis. Although the former species is more frequently encountered, Hydrablabes representation in natural history collections worldwide is lacking, with less than 10 and 0 specimens of each taxon in United States institutions, respectively. While these species are currently considered members of the family Natricidae, which contains hundreds of Old and New World aquatic species, they have also been hypothesized to belong to Homalopsidae (Murphy and Voris, 2014), a smaller family of mostly aquatic, mildly venomous snakes, also found across Southeast Asia. Much of Borneo’s herpetofauna and its respective natural history is still in the midst of being fully described and understood (Quah et al., 2019; Das and Wong, 2021; Fukuyama et al., 2021). Indeed, Southeast Asia’s undescribed diversity promises exciting discoveries, but it is equally worrisome that this diversity may disappear before ever being discovered (Sodhi et al., 2004; Strang and Rusli, 2021). Studying the systematics of rare snakes such as Hydrablabes can act as a first step in filling in the current knowledge gaps in the known biodiversity and evolutionary processes of Southeast Asia.
Here, we extract and sequence the DNA of homalopsid snakes from several natural history collections, and two specimens of H. periops from the Field Museum of Natural History (FMNH), as part of an ongoing study on homalopsids. We use a high-throughput target capture approach to sequence ultraconserved elements (UCEs; Faircloth et al., 2012), anchored hybrid enrichment loci (AHEs; Lemmon et al., 2012), and nuclear protein-coding genes (NPCGs) commonly used in squamate (lizards and snakes) phylogenetic studies via the SqCL v2 probe set from Singhal et al. (2017a,b). We use multiple pipelines and methods to isolate nuclear and mitochondrial loci to (i) maximize the utility of data obtained from museum specimens that would otherwise be considered ‘failed’ sequencing attempts, (ii) compare sequencing results between fresh and degraded tissue samples, (iii) place H. periops in a molecular phylogeny for the first time amongst all major extant snake lineages, and (iv) test competing taxonomic hypotheses for the familial designation of Hydrablabes (Natricidae vs. Homalopsidae). We focus on the failures/difficulties encountered pre- and post-sequencing, and make suggestions for future studies working with degraded DNA so as to increase expectations of error during project workflow and maximize the success of museum genomics for phylogenetic studies.
Materials and Methods
Sample Collection and Morphological Identification
For the homalopsids, we obtained 115 fresh liver/muscle samples and 41 degraded (39 liver/muscle; 2 bone) tissue samples from several natural history museums (Supplementary Table 1). Additionally, we extracted liver tissue from two specimens of H. periops from the herpetology collection of the Field Museum of Natural History (specimens FMNH 158616, 251051; Supplementary Table 1). These H. periops specimens were collected by the late Mr. William Hosmer in 1964 (FMNH 158616) and Curator Emeritus at the FMNH, the late Dato Dr. Robert F. Inger, respective collaborators from the Field Research Team of Sabah Parks, Malaysia and Datin Tan Fui Lian in 1993 (FMNH 251051). Although not generated and used comparatively in this study, we note that computed tomography (CT) scans of FMNH 251051 are available on the MorphoSource repository (ark: /87602/m4/415178). To confirm the taxonomic identity of the specimens of H. periops, we conducted morphological examinations and compared those to species accounts in the literature (Mocquard, 1890; Stuebing et al., 2014). We looked at the following characters: color pattern; total length (TtL); tail length (TL), measured from the cloaca to the tip of the tail; snout-vent-length (SVL), measured from the tip of the rostral scale to the vent; TL:TtL ratio; dorsal scale rows (DSR) at 10 scales behind the head (anterior), midbody (half of the total length), and 5 scales anterior to the cloaca (posterior); number of subcaudal scales; number supraocular, preocular, subocular, and postocular scales; number of supralabial and infralabial scales; temporal scale formula; and the state of the prefrontal scales (divided vs. complete); morphological data can be found in Supplementary Table 2. While we only attempted molecular work from two of the FMNH specimens, we also obtained morphological data of a third H. periops specimen (FMNH 146230) and report it here. Our sampling also includes molecular data, as part of an in-progress study (Bernstein et al., unpublished data), from 115 fresh and 41 degraded samples of homalopsid snakes; we also included 3 viperids (Bothrops moojeni and Bothrops pauloensis), a colubrid (Chironius exoletus), a dipsadid (Philodryas olfersii), and an elapid (Micrurus brasiliensis) from Singhal et al. (2017b) as outgroups (Supplementary Table 1). While all of the tissues in our study have been stored in natural history museums, we use the terms ‘museum specimens,’ ‘degraded,’ and ‘intractable’ interchangeably to refer specifically to historic, fixed specimens with degraded DNA. We reference the homalopsids, viperids, colubrid, and dipsadid to draw quantitative and qualitative comparisons between degraded and fresh samples when attempting to recover nuclear and mitochondrial DNA, test the hypothesis of H. periops being a member of Homalopsidae, and help establish expectations for museum genomics studies. However, we limit our phylogenetic results and corresponding discussion on the H. periops samples.
Museum Specimen DNA Extraction and Sequencing
Total genomic DNA (gDNA) was extracted using published protocols for target capture sequencing of museum specimens (Ruane and Austin, 2017). This method uses a heated alkali buffer solution and a modified protocol of Qiagen® DNeasy Blood and Tissue kits to increase the gDNA yield from intractable specimens. Briefly, 100–200 mg liver tissue was cut into 15–25 mg pieces and washed in distilled water for 6 h to remove excess ethanol. Tissue was then pulverized or cut up to a mashed consistency. We then added ∼25–50 mg of the tissue to a 2-mL microcentrifuge tube with 300 μL of preheated (98°C) ATL buffer, and incubated the samples at 98°C for 15 min. The tubes were then cooled on ice for 2 min. Finally, we added 40 μL of proteinase-K to the samples and digested them for 48–72 h at 65°C, vortexing samples periodically and adding more proteinase-K if undigested tissue was visible. We then followed the post-digestion steps from the Qiagen® DNeasy Blood and Tissue kits protocol, except with two 100-μL final AE elution steps, rather than a single 200-μL elution. Different extraction attempts on the same sample were combined to increase the total gDNA per sample. Two of the samples were extracted from bone (122 and 14 mg), and we followed published protocols for obtaining DNA from hard tissue (Allentoft et al., 2015, 2018), with the exception that we used Qiagen® DNeasy Blood and Tissue kits after the proteinase-K digestion step. All DNA extractions were performed in an area isolated from fresh DNA work, on surfaces that were sterilized with bleach, and with UV-sterilized equipment and filter pipette tips. We used a Qubit 3 fluorometer (high sensitivity; Thermo Fisher Scientific: Invitrogen) to quantify the DNA yield of all extractions. Genomic DNA was sent to Daicel Arbor Biosciences (Ann Arbor, MI, United States) and optimized for target capture using the SqCL v2 probe set (Singhal et al., 2017b) for UCEs, AHEs, and NPCGs. To increase the likelihood of recovering reads from each sample, we had a small percentage of the non-captured libraries spiked into the sequencing pool to increase the number of bycatch molecules, thus increasing the chance of obtaining mitochondrial DNA (mtDNA) from our museum samples. The final sequencing pool for the degraded samples was prepared by combining the enriched (85%) and unenriched (15%) pools. Samples were sequenced on the Illumina NovaSeq 6000 platform on partial S4 PE150 lanes. Raw fastq files for the two specimens of H. periops have been deposited in the National Center for Biotechnology Information (NCBI) Sequence Read Archive (SRA) under the BioProject Accession Number PRJNA796637. The raw fastq files for the fresh and museum homalopsid specimens and outgroups are deposited under the SRA BioProject Accession Number PRJNA792597, PRJNA667001, and PRJNA382381 (see Supplementary Table 1 for note on data availability for PRJNA792597).
Bioinformatics
We checked the quality of raw reads using FastQC (Andrews, 2010) and tested for contamination with FastQ Screen (Wingett and Andrews, 2018). To trim adapters and barcodes, we used illumiprocessor (Faircloth, 2011; Lohse et al., 2012; Del Fabbro et al., 2013) with default settings, and then assembled paired-end reads using SPAdes (Bankevich et al., 2012) in the Phyluce v1.7.1 (Faircloth, 2016) pipeline for processing UCEs. We also used Assembly By Short Sequences (ABySS; Simpson et al., 2009), separately, with k-mer values set to 30 and 60, to determine if more loci can be recovered. However, both assemblers achieved comparable run statistics, so we continued with using SPAdes, which averages over multiple k-mer values, as it is conveniently integrated into Phyluce. To test the hypothesis if H. periops is a homalopsid, we included H. periops into a dataset of homalopsid snakes and outgroup taxa (see Supplementary Table 1). We created DNA alignments for each locus following the workflow for Phyluce. Many of our museum samples had a high number of raw reads (>10–15 million); due to computational constraints, we used seqtk1 to subsample the raw data from these samples to 3.5 million reads from each pair (7 million total). To align our H. periops samples with homalopsid samples, alignments of homologous nucleotide sites for each locus were edge-trimmed with Gblocks, and data matrices were created for each locus that contained at least 75% of the taxa in the dataset.
Because Phyluce yielded poor final phylogenetic results for the museum specimens (see Section “Concatenated and Species Tree Analyses”), we also extracted individual loci from the cleaned and trimmed raw data using Geneious v11.1.5. We tested a few approaches to compare their success of recovering targeted loci. Some of these approaches involved the use of a pseudoreference genome consisting of SqCL concatenated loci (with 10 ambiguous bases [Ns] between each locus) from one of the fresh homalopsids or the Myanophis thanlyinensis (Homalopsidae) reference genome from Köhler et al. (2021), hereafter ‘pseudoreference’ and ‘reference genome,’ respectively. Our approaches include: (i) mapping the trimmed and cleaned raw reads to the pseudoreference, (ii) BLASTing (Basic Local Alignment Search Tool) the trimmed and cleaned raw reads to the pseudoreference, (iii) mapping the unaligned.loci file of each individual (containing fastas of all individual loci) from Phyluce to the pseudoreference, (iv) BLASTing the unaligned.loci file from Phyluce to the pseudoreference, and (v) mapping the trimmed and cleaned raw reads to the reference genome. BLAST databases containing the pseudoreference and references were created using the ‘Add sequence database’ function in Geneious (Figure 1). All mapping and BLAST methods were performed under default parameters. For BLAST, Megablast (5 iterations) was used so that only matches with high similarity were returned. Our goal was to find the most efficient way to retrieve loci for the museum samples, thus we considered methods that took >24 h per sample to take too long (this is especially important if done on computer clusters that have time constraints) and aborted the process. We test these approaches using two degraded samples as preliminary runs: (raw reads for Calamophis ruuddelangi RMNH.RENA 47517 and Gyiophis maculosa KU 92395 = ∼30 million reads each; unaligned.loci files for C. ruuddelangi and G. maculosa containing 1,527 and 2,016 loci, respectively), and then ran the rest of the samples using the approach that retrieved loci in the shortest amount of time and with the highest success. All approaches were run on a Digital Storm PC with a 64-bit operating system, x64-based processor, 16 cores, and 40 GB RAM allocated to Geneious for computation.
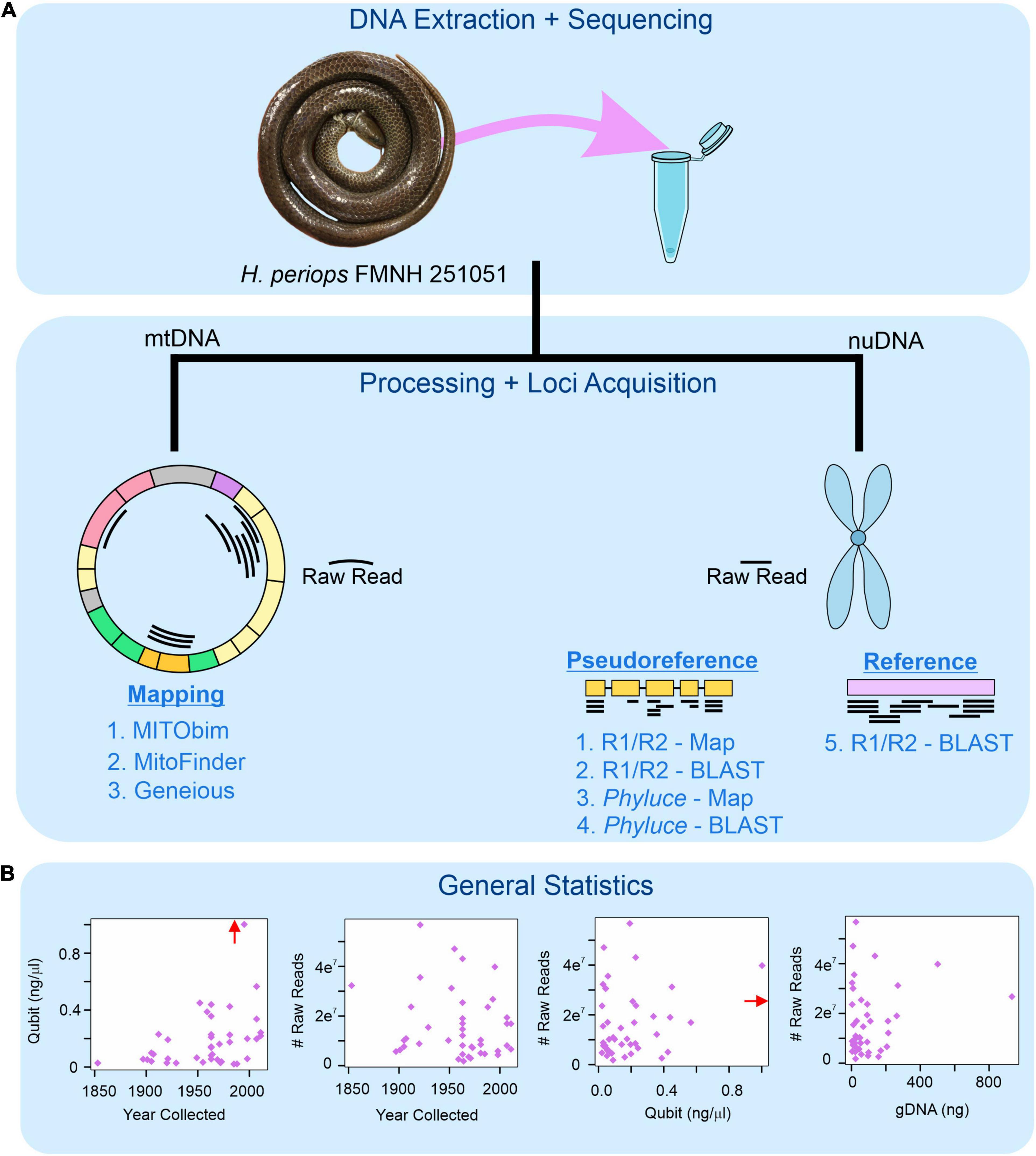
Figure 1. Workflow for museum genomics in this project. (A) Three approaches for mitochondrial mapping to a reference genome (left) and five for nuclear locus acquisition using mapping and BLAST (right). Phyluce = unaligned.fasta file from Phyluce pipeline; R1/R2 = *.R1.fastq.gz and *.R2.fastq.gz files for raw reads. (B) Read statistics for DNA yield (Qubit) and total number of raw reads obtained by age of specimen (year collected), DNA yield, and total genomic DNA used for sequencing (gDNA). Red arrow shows data point for Hydrablabes periops FMNH 251051 (Qubit = ∼6.0 ng/μl), arrow positioned along axis corresponding to respective X-axis and Y-axis values.
To increase the robustness of our phylogenetic results and determine if H. periops is a homalopsid, natricid, or a member of another family of snake, we used data from previously published studies to create additional DNA alignments based on our loci obtained (see Section “Read and Locus Acquisition”). We used the 50 longest UCEs of homalopsids (Bernstein et al., unpublished data) obtained from Geneious (see Section “Read and Locus Acquisition”) to create a UCE-only alignment with H. periops and homalopsid snakes (plus outgroups). We also created alignments using AHEs from almost all lizard and snake families using the data from Burbrink et al. (2019, 2020) and a multilocus dataset from several genera of natricids (and one outgroup colubrid) from Deepak et al. (2021a) and other studies (Alfaro and Arnold, 2001; de Queiroz et al., 2002; Nagy et al., 2005; Guo et al., 2012, 2019; McVay and Carstens, 2013; Pyron et al., 2013; Kindler et al., 2014; McVay et al., 2015; Alencar et al., 2016; Ren et al., 2019; Lalronunga et al., 2020; Deepak et al., 2021b) consisting of two mitochondrial genes (cytochrome b [cyt-b], NADH-ubiquinone oxidoreductase chain 4 [ND4]), and one nuclear gene [Brain Derived Neurotrophic Factor (BDNF)]. We used the AHEs from Burbrink et al. (2020) as a pseudoreference to identify the same loci from this and our study. Specimen metadata from other published or in-progress studies can be found in Supplementary Table 1.
To obtain mtDNA from museum specimens, we tried three methods: (i) mitochondrial baiting and iterative mapping using MITObim (Hahn et al., 2013), (ii) mitogenomic data extraction using MitoFinder (Allio et al., 2020), with metaSPAdes (Nurk et al., 2017) used as the assembler, and (iii) mapping raw reads to a mitochondrial reference genome in Geneious, all under default parameters. Both MITObim and MitoFinder require mitochondrial reference genomes to extract loci, thus we used a Hypsiscopus plumbea (Homalopsidae) mitochondrial genome (Genbank accession: DQ343650; Yan et al., 2008). We used this reference for our mapping approach in Geneious as well. We used the ‘Highest Quality’ consensus option in Geneious, which only creates consensus sequences out of mapped reads using high-quality chromatograms.
All loci obtained through Geneious were manually incorporated into DNA alignments from Phyluce by using the ‘Multiple Align’ tool in Geneious. We left these alignments untrimmed, which has been found to achieve the best phylogenetic results in studies using UCEs (Portik and Wiens, 2021). We used the lm function in the stats package in R (R Core Team, 2021) to graph linear model relationships of (i) number of raw reads obtained and (ii) number of nuclear loci recovered, with the age of specimen (years since collected), DNA yield, and total gDNA used for sequencing. We used the same methods to determine trends between the number of raw reads and base pairs (in bp and percentage) of museum specimen reads that mapped to the mtDNA reference genome. We note that our linear models are heavily influenced by FMNH 251051 due to its high DNA yield and sequencing success compared to other specimens (see Section “Results”). We leave this specimen out of our analyses due to it being an outlier, but as it represents an important piece of information in regards to museum genomics success, linear regressions with and without this specimen can be found in Supplementary Figures 1, 2.
Phylogenetic Analyses
To determine the phylogenetic placement of H. periops using loci obtained from the Phyluce pipeline, we reconstructed a phylogeny by concatenating the alignments with the ‘phyluce_align_concatenate_alignments’ command and then used this as input for IQ-TREE v1.6.12 (Nguyen et al., 2015) under a GTR+G substitution model and with 1,000 bootstrap replicates. Due to high levels of missing data from formalin specimens and failed phylogenetic reconstruction with the loci obtained from Phyluce (see Section “Read and Locus Acquisition”), we ran species tree analyses, taking gene-tree-species-tree discordance into account, selectively choosing AHEs and UCEs with minimal missing data (see below).
For the species tree analysis using the multilocus dataset (cyt-b, ND4, and BDNF), we used Bayesian inference in StarBEAST2 (Ogilvie et al., 2017) under a birth-death evolutionary model, partitioning each alignment using the best partitioning scheme determined by PartitionFinder2 (Lanfear et al., 2017). We ran the model for 50 million generations, inferred and marginalized site models for our analysis using the ‘bModelTest’ plugin (Bouckaert and Drummond, 2017), and used an uncorrelated lognormal clock rate to allow branch rate heterogeneity. We assessed the convergence of our runs in Tracer v1.7 (Rambaut et al., 2018), discarding 25% of the run as burn-in, and considered effective sample sizes (ESS) > 200 to indicate sufficient sampling of parameter space. In addition to the StarBEAST2 tree, we reconstructed gene trees and a concatenated tree for cyt-b, ND4, and BDNF in IQ-TREE, searching for the best nucleotide model for each dataset with ModelFinder (Kalyaanamoorthy et al., 2017). Branch support was assessed by 1,000 ultrafast (UF) bootstrap iterations and SH-aLRT tests (Guindon et al., 2010; Hoang et al., 2018); relationships with UF bootstraps and SH-aLRT tests ≥95 and ≥80, respectively, were considered to be well-supported.
Because we had more loci in our AHE (n = 33) and UCE (n = 50) alignments compared to the multilocus dataset, and Bayesian approaches can be computationally demanding, we used polynomial time species tree reconstruction in ASTRAL-III (Zhang et al., 2018) for divergence date estimation. ASTRAL-III uses individual gene trees as input, so we built genealogies with the UCEs and AHEs. Gene trees were created using IQ-TREE with the same parameters used for the multilocus dataset loci. The individual AHE and UCE trees were used as input for our species tree analysis in ASTRAL-III (Zhang et al., 2018), under default parameters. Relationships with Bayesian posterior probabilities (Bpp) ≥ 0.95 are considered strongly supported. As mentioned above, Bayesian methods can be a computationally difficult task with high numbers of loci, so we used treePL v1.0 (Smith and O’Meara, 2012) to estimate divergence times of our AHE dataset, which has the highest familial-level sampling. This software uses a semi-parametric penalized likelihood approach to estimate rates of gene evolution on branches of a concatenated input tree. We created a concatenated alignment of our AHEs and obtained a phylogeny using the parameters described above, with the exception that a GTR+G+I model was used. We used the ‘thorough’ and ‘prime’ commands to find the optimal parameters of our treePL analysis and to ensure the analysis ran until convergence. To identify the optimal smoothing parameter, which affects the rate variation penalty across the tree, we used the random subsample and replicate cross-validation (RSRCV) function. To calibrate the divergence times, we used squamate fossils that have been described and used in previous studies (Jones et al., 2013; Alencar et al., 2016; Zaher et al., 2018; Burbrink et al., 2020; see Supplementary Table 3). Although we date the entire tree, we focus on the Natricidae given our phylogenetic results, discussed below.
Results
Read and Locus Acquisition
Despite extremely low yields of DNA during extraction (see Supplementary Table 1), we were successful in sequencing many of the targeted museum specimens. Using Phyluce, we were able to recover 9–3,889 loci (median [M] = 403) from the museum (intractable) samples. However, these locus alignments were significantly smaller and contained fewer samples than loci obtained from fresh specimens, with DNA alignments from fresh samples and museum samples being 224–2,633 (average [] = 845.8) and 10–864 ( = 121.5), respectively. The alignments with degraded samples only contained an average of 6.7 (out of 43) formalin specimens across all alignments. Using alternative approaches, all mapping and BLASTing of raw reads to the pseudoreference and reference genomes took >24 h, and thus were terminated. When mapping the loci from these unaligned.loci files of C. ruuddelangi and G. maculosa, the analyses took, respectively, 17 and 10 seconds (s) to map 1,819 and 1,248 loci to the pseudoreference (remaining loci had no match). While these were faster, some loci that mapped to the pseudoreference spanned more than one gene, albeit rarely. When using the BLAST approach of the unaligned.loci files to the pseudoreference, run time took 60 s for C. ruuddelangi (2,016 recovered loci) and 45 s for G. maculosa, (1,800 recovered loci). Because BLASTing the Phyluce unaligned.loci file to the pseudoreference recovered more loci (and the DNA sequence of each locus is conveniently created in a separate file), we use this approach and considered it the most efficient. We note that despite two rounds of cleaning and trimming of adapter sequences, tens to hundreds of loci from museum specimens contained portions of adapters, which were trimmed off when we BLASTed sequences. No clear correlations were observed between DNA yield, specimen age, or raw reads obtained (Supplementary Figure 1).
BLASTing the unaligned.loci file to the pseudoreference retrieved a total of 25,126 UCEs, 1,657 AHEs, and 199 nuclear genes across all 43 museum specimens (Supplementary Table 4). Locus lengths (bp) ranged from 28–1,627 (/M = 218.84/152) for UCEs, 28–1,988 (/M = 156.37/94) for AHEs, and 34–1,225 (/M = 233.47/120.5) for nuclear genes. The two specimens of H. periops we sequenced did not yield similar numbers of loci: we obtained 5 loci (44–75 bp) from the older specimen FMNH 158616, and 3,530 loci (33–1,532 bp; /M = 292.5/218) from the more recently collected specimen FMNH 251051. This latter sample contained 275 loci that were ≥500 bp, and this specimen was used to determine Hydrablabes phylogenetic placement amongst other snake lineages. Taking all of our museum specimens into account, we recovered UCEs, AHEs, and NPCGs ≥ 250 bp for 25, 18, and 9 specimens, respectively (Figure 2A). We found positive relationships between DNA yield with the number of AHEs and NPCGs obtained, but not UCEs (Figure 2B). These patterns were also seen when comparing total gDNA used for sequencing with the number of loci obtained. Contrarily, there was no correlation between the number of loci obtained and the age of the specimen (Figure 2B). Graphs with lines, R2, and p-values from linear models are in Supplementary Figures 1, 2.
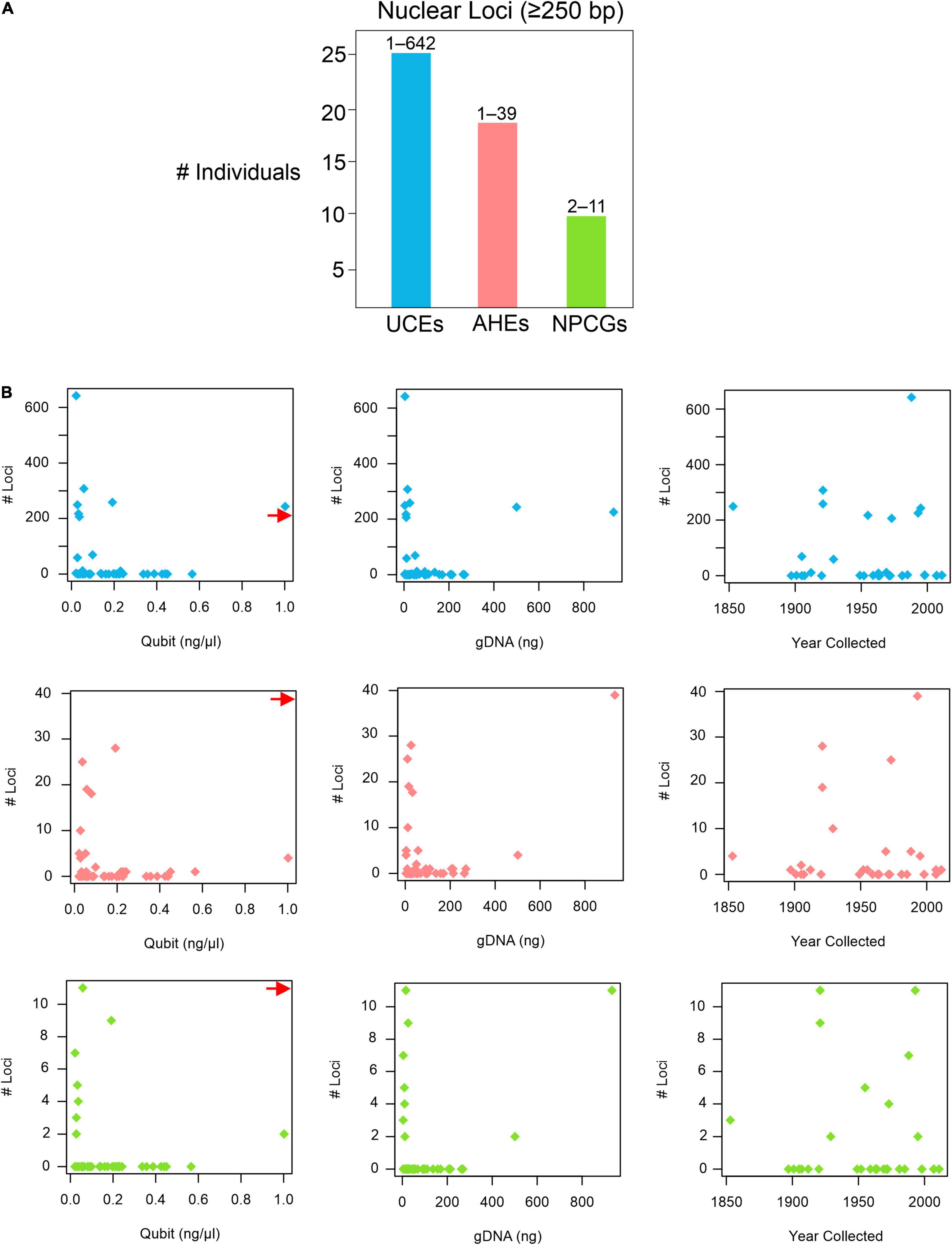
Figure 2. Nuclear loci recovered from pseudoreference BLASTing. (A) Number of individuals that yielded UCEs, AHEs, and NPCGs ≥ 250 bp. Numbers above bars represent the range of number of loci recovered amongst all individuals. (B) Graphs showing the relationships of the number of UCEs (top), AHEs (middle), and NPCGs (bottom) and specimen age (year collected), DNA yield (Qubit), and total genomic DNA used for sequencing (gDNA). Red arrow shows data point for H. periops FMNH 251051 (Qubit = ∼6.0 ng/μl), arrow positioned along axis corresponding to respective Y-axis values. Complete list of all nuclear loci and lengths for each specimen is in Supplementary Table 4.
All approaches for obtaining mitochondrial DNA from H. periops FMNH 158616 failed. However, attempts for FMNH 251051 were successful, depending on which approach was used to isolate mitochondrial bycatch. MITObim failed to extract any loci from the raw read data, while MitoFinder was successful in extracting 70 unique sequences of 15 mtDNA genes, across seven museum specimens (Supplementary Table 5). These sequences range from 162 to 1,785 bp (/M = 898.3/914). Our most successful attempts to obtain mtDNA was using Geneious. Our mapping method of raw reads to the mitochondrial genome of Hypsiscopus plumbea resulted in a near-complete mitochondrial genome of the more recently collected H. periops specimen, mapping ∼1.17 million reads (15,649 non-ambiguous [A, C, T, G] bp), obtaining whole or partial coverage of every gene, control region, and tRNA (except tRNA-Phe). Out of the 43 museum specimens sequenced in this study, 27 specimens had at least one read mapped to the H. plumbea mitochondrial reference genome, with >1,000 reads mapped for 9 of these specimens (Supplementary Table 6). We recovered a range of 0.72–98.95% (126–17,215 bp) of the mitochondrial genome (reference = 17,397 bp). For five specimens, we obtained near-complete mitochondrial genomes, with >85% of the genome sequenced with almost all protein-coding genes, tRNAs, control regions, and the replication origin (Figure 3A). We observed no relationship between age or total gDNA with the number of non-ambiguous bp mapped to the mtDNA reference, number of raw reads mapped to the mtDNA reference, or percent of mtDNA genome sequenced (Figure 3B and Supplementary Figure 1). This was also seen when comparing DNA yield to the number of raw reads mapped to the mtDNA reference. However, DNA yield had a positive relationship with the number of bp mapped to the reference and percent of mtDNA genome sequenced (Figure 3B). Graphs with lines, R2, and p-values from linear models are in Supplementary Figures 1, 2.
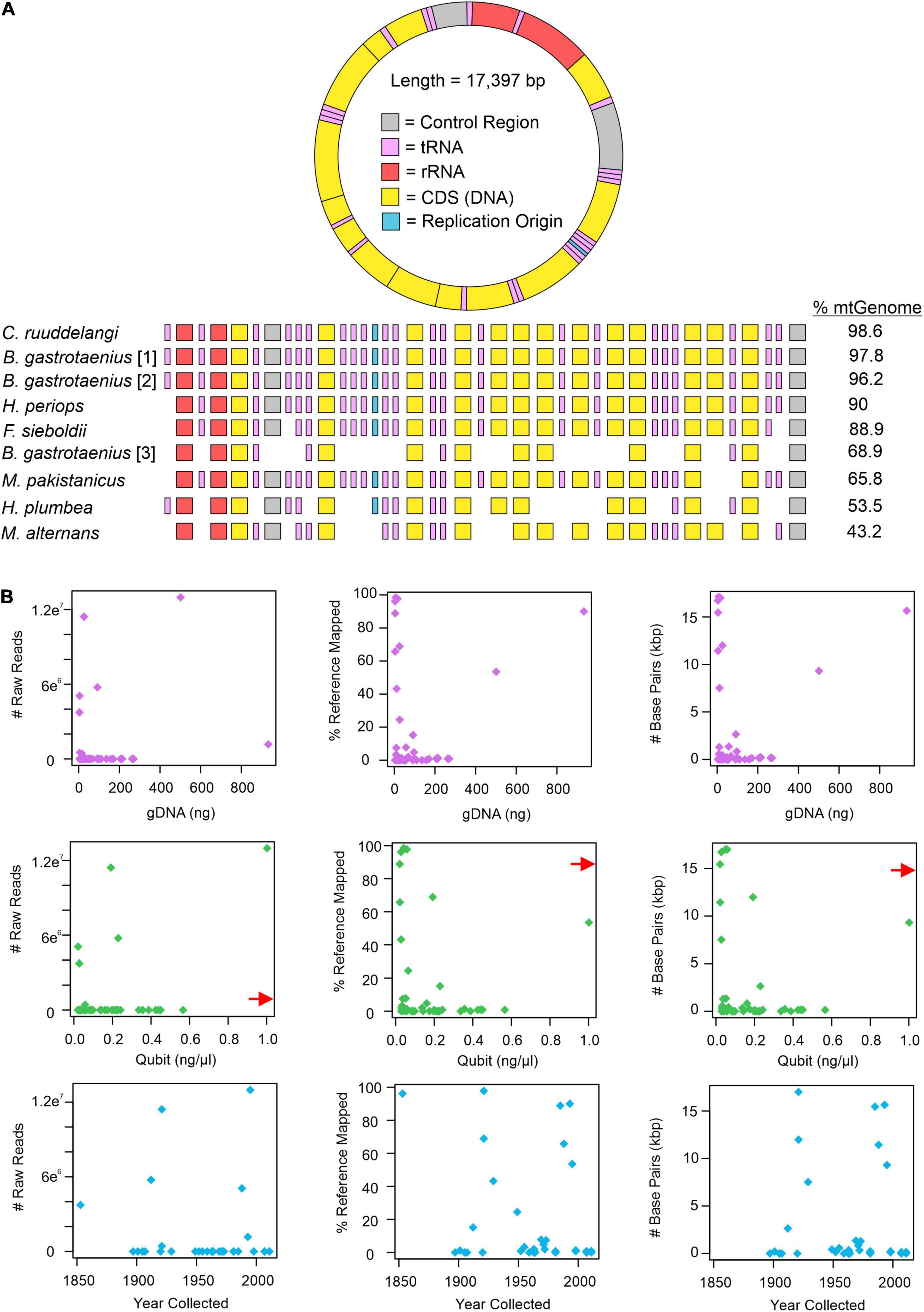
Figure 3. Mitochondrial mapping results from Geneious. (A) Mapped loci from specimens genera in order include Calamophis, Brachyorrhos, Hydrablabes, Ferania, Mintonophis, Hypsiscopus, and Miralia with ≥25% of the whole mitochondrial reference genome (mtGenome). Blocks indicate successfully recovered regions (minimum ≥ 20% coverage). Gene regions colored in order as they appear on the reference genome. (B) Graphs showing the number of mitochondrial raw reads mapped to the mtGenome, percent of the mtGenome obtained, and the total number of base pairs obtained relative to total genomic DNA used for sequencing (gDNA; purple dots), DNA yield (Qubit score; green dots), and sample age (year collected; blue dots). Red arrow shows data point for H. periops FMNH 251051 (Qubit = ∼6.0 ng/μl), arrow positioned along axis corresponding to respective Y-axis value. The complete list of mtDNA regions and respective coverage for each specimen is in Supplementary Table 6.
Concatenated and Species Tree Analyses
Our concatenated tree of homalopsids (fresh and degraded samples) + Hydrablabes (degraded samples) using all loci obtained from Phyluce (4,822 alignments concatenated to 2,346,038 bp) placed the two H. periops specimens within a group containing all museum sample homalopsids (Supplementary Figure 4). However, all of the museum samples randomly cluster (i.e., no sensible evolutionary relationships) together close to the outgroup taxa with long branches, and are placed outside of the fresh homalopsid specimens.
Our concatenated and genomic trees using the molecular data obtained from Geneious combined with published datasets supported the placement of H. periops in Natricidae. The multilocus dataset of natricids from Deepak et al. (2021a) recovered a monophyletic Natricidae, with Hydrablabes periops as the sister taxon to Trimerodytes praemaxillaris (Supplementary Figure 3). The single gene trees resulted in multiple positions for H. periops, including an unresolved placement (BDNF), as sister to T. praemaxillaris (cyt-b), and the most closely related lineage to the sister pair Smithophis atemporalis + Opisthotropis voquyi (ND4) (Supplementary Figure 3). The species tree constructed from StarBEAST2 reached convergence and most ESS values were >200, with the exception of a few bModelTest and shape parameters, and one gene subset likelihood; the posterior, likelihood, prior, and species coalescent parameters all had ESS > 200. The species tree shows H. periops in the same phylogenetic position as the ND4 gene tree. While there is low support for placement with respect to the generic relationships, H. periops is strongly supported as a natricid (Figure 4A) in this multilocus tree.
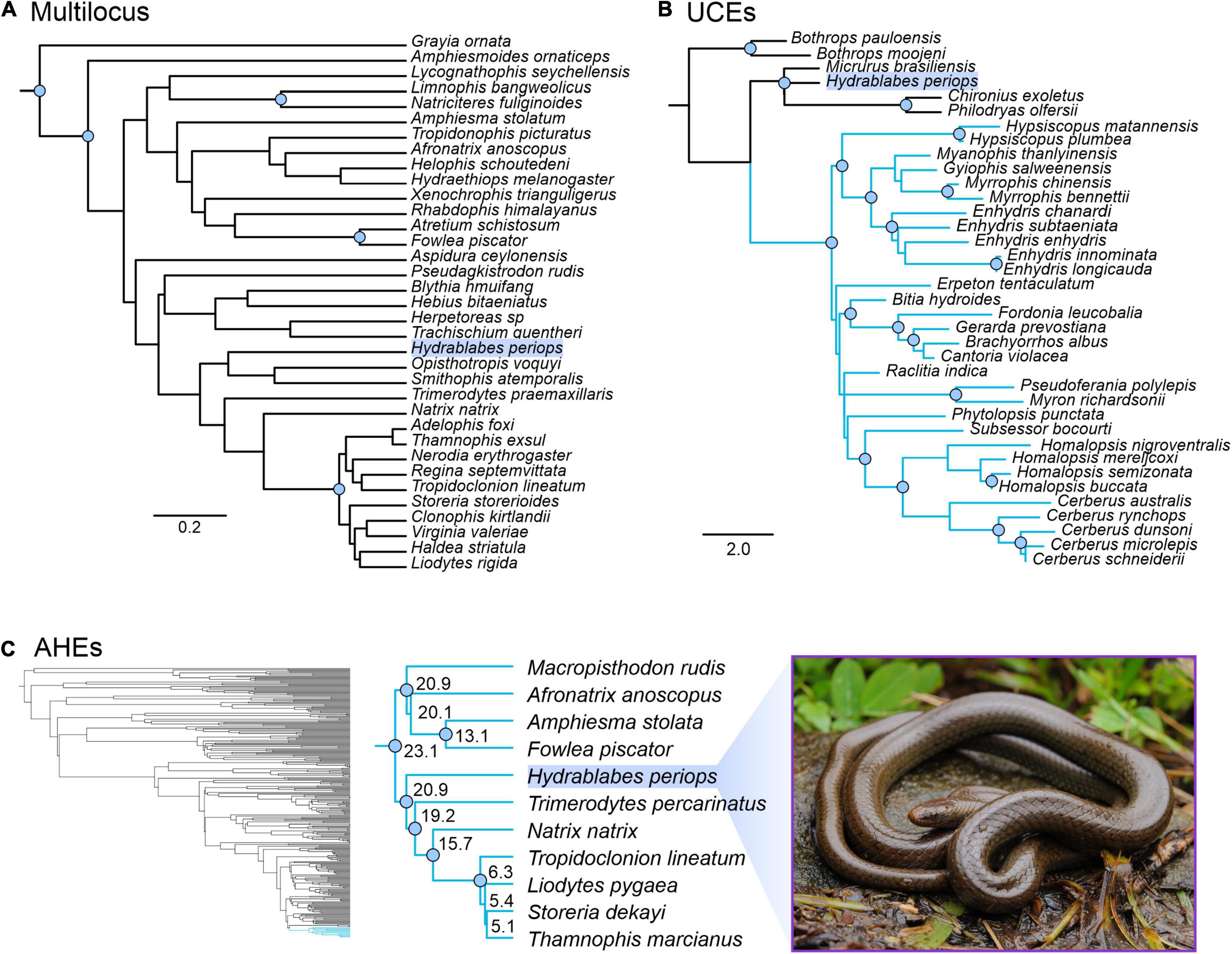
Figure 4. Phylogenetic placement of Hydrablabes periops using three datasets from other studies. (A) Species tree using one nuclear and two mitochondrial genes (outgroup = Grayia); scale bar in nucleotide substitutions per site. (B) Homalopsidae species tree using UCEs; blue clade = Homalopsidae, scale bar in coalescent units. (C) Squamate species tree using AHEs; blue clade = Natricidae, numbers on enlarged Natricidae clade represent divergence dates in millions of years. Node circles in all trees indicate strongly supported relationships (Bpp ≥ 0.95; not shown for Squamata AHE tree). Photo credit of live H. periops: Chien C. Lee.
The genomic trees using loci from published data also support that H. periops is in the family Natricidae, with its placement outside Homalopsidae. The UCE species tree strongly recovered Homalopsidae as a clade, and H. periops positioned with the outgroups Micrurus, Chironius, and Philodryas with strong support (Figure 4B). Specifically, H. periops is sister to Chironius (Colubridae) + Philodryas (Dipsadidae), although with poor support (Figure 4B). For the AHEs, we were able to obtain 33 loci that aligned to the AHEs of several snake families from Burbrink et al. (2020). Our species tree using 33 loci was broadly consistent with the full dataset from Burbrink et al. (2020), with most nodes strongly supported (Figure 4C). Higher-level relationships were identical between both trees, with the exception of the placement of Dibamia and Iguania, both of which have low support in our tree and in the species tree from Burbrink et al. (2020). Of the 37 snake families, our species tree shares the same relationships with ones seen in the full dataset tree, with the exception of the placements of Atractaspididae, Bolyeriidae, and Lamprophiidae, the latter poorly supported in both trees. Similar to the UCE tree, using AHEs recovered H. periops within Natricidae with strong support (Figure 4C); H. periops is sister to a clade containing Eurasian natricids (Trimerodytes percarinatus and Natrix natrix) and North American natricids (Tropidoclonion lineatum, Storeria dekayi, Thamnophis marcianus, Liodytes pygaea) (Figure 4C). Our AHE concatenated tree, used for divergence dating, is similar to the one obtained in Burbrink et al. (2020), with the exception that the ancestral Iguania lineage subtends Anguiformes (sister to Anguiformes in Burbrink et al., 2020). Our divergence dates of Natricidae are within 1–5 myr of those obtained from Burbrink et al. (2020) with the divergence of H. periops from its sister group ∼20.9 mya (Figure 4C).
Discussion
Museum Genomics Is Successful With a Range of Specimen Ages and DNA Yields
Our results emphasize that the outcomes of several phases of museum genomics projects (e.g., DNA extraction, DNA sequencing, and bioinformatics) may not be optimal, yet valuable results can still be obtained. Our DNA extraction attempts on museum specimens yielded poor concentrations of DNA (often <0.1 ng/μl; see Supplementary Table 1). However, we were still able to extract a large number of nuclear and mitochondrial loci for several specimens, and even near-complete mitochondrial genomes for 5 individuals. We observe comparable findings with respect to other studies in that DNA extractions on museum specimens yielded extremely low levels of quantifiable DNA, often resulting in Qubit readings of ‘Too Low’ or <0.01 ng/μl (rarely ≥1 ng/μl), which are similar to reported quantifications in museum genomics studies (Hykin et al., 2015; Ruane and Austin, 2017). Though some studies achieve a wide range of DNA yields (“Too Low”–11.5, Ruane and Austin, 2017; “Too Low”–92.4, Zacho et al., 2021) from specimens when using identical methods within the respective study, it is likely that this is the result of different preservation treatment and environmental factors from time of collection to DNA extraction [e.g., exposure to UV light, time span from the death of an animal to preservation, preservation methods (ethanol vs. formalin), etc.]. While such information may not be (and are often not) recorded, the year of collection is typically known, providing an estimate of the age of the specimen post-mortem. Our study obtained significantly more nuclear and mitochondrial loci from the 28-year old (1993) specimen compared to the 57-year old (1964) specimen, the latter of which we only obtained five nuclear genes (44–75 bp; Supplementary Table 4). However, we cannot draw concrete patterns in relation to sequencing success and age (or even DNA yield), as some specimens from 1963 yielded DNA concentrations of 0.3–0.8 ng/μl (compared to <0.1 for other specimens) with failed sequencing results, while others for which we obtained near-complete mitochondrial genomes and hundreds of nuclear loci had concentrations <0.1 or even ‘Too Low’ and were collected in 1853 and 1921. These latter specimens are 41–109 years older than the 1964 H. periops specimen with failed sequencing attempts. Other studies have also found positive correlations between specimen age and DNA sequencing success in reptiles (Hykin et al., 2015) and birds (McCormack et al., 2016), but this is not ubiquitous amongst museum genomic studies with the same study organisms (e.g., Linck et al., 2017; Ruane and Austin, 2017). It is worth noting that obtaining samples that have had less time in fixatives will be ideal for recovering and sequencing DNA, as has been seen in recent studies using AHEs from salamanders that are ∼50 years old (Pyron et al., 2022). We note that while many of our linear regressions do not support significant relationships between DNA yield, age of specimens, and particular locus types retrieved (Supplementary Figures 1, 2), future studies that include more specimens with an even sampling of specimen ages and tissue types may find better consistency with respect to specimen age and quality of results.
Museum Genomics Confirms Hydrablabes Is an Asian Natricid
The placement of H. periops supports the familial taxonomic status of Hydrablabes as a natricid, rejecting the hypothesis that this species is a homalopsid (Murphy and Voris, 2014). Divergence dates of natricids estimated here are slightly younger (Figure 4C) than those of Burbrink et al. (2020) (likely due to our reduced dataset and the inclusion of Hydrablabes), but are still broadly consistent with what is hypothesized about this family’s diversification. Hydrablabes is a genus that is endemic to Borneo, a continental island that has only recently separated from mainland Southeast Asia (Hall, 2009). Cenozoic Sundaland was composed of the islands of Borneo, Java, and Sumatra, connected to the mainland by a land bridge. At ∼400,000 years ago (kya), Pleistocene sea-level fluctuations caused cycles of emergence and submergence of this land bridge during respective glacial and interglacial periods, up until the Last Glacial Maximum ∼20 kya (Voris, 2000; Sarr et al., 2019; Husson et al., 2020). Given its distribution, the close relation of H. periops to other Asian natricids is not unexpected, and our multilocus and AHE datasets provide different, yet valuable, information regarding the evolution of Hydrablabes. The multilocus tree supports H. periops as most closely related to Asian natricids in South and Southeast Asia, but outside of the clade with Indochinese Trimerodytes, Eurasian Natrix natrix, and North American natricids. Similarly, the AHE tree supports H. periops as sister to a group containing these taxa. These topologies are congruent with that of Deepak et al. (2021a), whose study also supported an Asian origin of Natricidae. Results from Deepak et al. (2021a) show a Mainland Asia + Japan origin over 20 mya for the clade that Hydrablabes is sister to. Biogeographic scenarios make sense in relation to our findings, as Borneo was still connected to the mainland prior to 400 kya (Hall, 2009; Husson et al., 2020). Our age of the clade containing H. periops (∼20.9 mya) may indicate population dispersal into Borneo from the mainland, with subsequent extinction events outside Borneo. Alternatively, the lineage ancestral to this clade may have dispersed into Borneo, followed by an in situ speciation event. The divergence dates of H. periops and Trimerodytes occur at interesting points in Indochina’s geological record. Specifically, the rise of the Hengduan Mountains of the eastern Tibetan Plateau (Western China) are considered to have been a diversification driver of Trimerodytes ∼23.9 mya (Guo et al., 2020). While greater taxonomic sampling of Hydrablabes and other Borneo-endemics, as well as increased molecular sampling, will help to elucidate the evolutionary history of Hydrablabes amongst natricids, these initial results provide evolutionary and phylogeographic hypotheses for future testing.
Expectations and Suggestions for Museum Genomics
Museum genomics has advanced significantly in the last decade, undoubtably a product of newer, high-throughput sequencing technologies and the rapid accumulation of genome-scale datasets. A variety of biochemical protocols have been developed with varying degrees of success in different organismal systems. Some protocols (Campos and Gilbert, 2012; Hykin et al., 2015) rely on hot alkali treatments to increase the odds of extracting DNA by breaking formalin-induced crosslinking between DNA and protein. Other methods increase the amount of digestive enzymes (e.g., proteinase-K) and lengthen the digestion times to breakdown more total amount of tissue (Ruane and Austin, 2017; this study). Additional steps, such as ‘pre-digestion’ steps with buffers for removing surface contaminants, have also been used when working with bone (Allentoft et al., 2015). All of these biochemical differences in workflows will inevitably have differing success rates depending on tissue type and quality (discussed below), as well as the type of loci being targeted (mtDNA, UCEs, AHEs, NPCGs, introns, whole genomes, etc.). While we are starting to better understand the damaging effects of formalin- and ethanol-fixation on museum specimens that hampers their input into evolutionary studies (Card et al., 2019), there is still a paucity for expectations during various parts of the project workflow when dealing with intractable specimens. Below, we provide expectations and tips based on our experiences in dealing with museum specimen genomics, in hopes that other researchers can maximize the data obtained from seemingly failed sequencing attempts.
DNA Extraction
The success of extracting quantifiable DNA of sufficient quality is dependent on numerous variables, most of which are unknown (e.g., specimen storage conditions since collection, preservation technique, time span from death to preservation, etc.). If available, a variety of tissue types (liver, muscle, and bone) from multiple individuals of different ages, should be used. Some studies have found that higher DNA yields were extracted from soft tissues, such as liver and muscle, compared to hard bone tissue (Zacho et al., 2021). Though, bone tissue can yield surprisingly high amounts of DNA (Zacho et al., 2021) of sufficient quality for sequencing, as we found from our homalopsid specimens, potentially due to greater protection against chemicals inside dense tissue. Specifically, the two bone samples used here, with few nuclear loci, provided ∼66 and ∼89% of the mitochondrial genome. We also note that while bone digestion protocols (Allentoft et al., 2015, 2018) may be more tedious than using Qiagen or phenol-chloroform procedures, they should not be overlooked as a tissue source. Additionally, this may prevent destructive sampling of fluid specimens if skeletons are already available in natural history collections (especially in snakes, which have hundreds of ribs). We used minimal amounts of bone (122 and 14 mg) for the bone extractions here.
Our protocols used proteinase-K as a tissue digesting agent. We note that proteinase-K often failed to digest tissues completely or even partially (over 48–72 h). This was true even if tissue was pulverized, with or without the aid of liquid nitrogen, to increase surface area. Vortexing samples every 6 h could facilitate tissue lysis, as well as adding 25 μl of proteinase-K every 24 h. While relationships between proteinase-K concentration and DNA yield for museum samples are lacking, formalin-fixed, paraffin-embedded tissue sections have provided increased DNA amounts when subjected to more digestion enzyme (Frazer et al., 2020). The failure of tissues to digest completely may result in clogging of filter tubes in spin columns, thus using multiple tubes per tissue specimen is recommended during such scenarios. While museum genomics studies typically report low DNA yields during extraction, our results highlight that even quantifications of <0.1 ng/μl can lead to successful sequencing of nuclear and mitochondrial loci. Nonetheless, combining aliquots of DNA extractions from the same individuals will increase the total gDNA and increase the likelihood of sequencing success, especially with specimens that were preserved more recently. We found more mtDNA was caught as bycatch when more total gDNA was used for sequencing (Supplementary Figure 2). Though, linear regression results were influenced by the inclusion of FMNH 251051; without this specimen, total gDNA was positively correlated with the number of AHEs and UCEs, but not the amount of mtDNA obtained (Supplementary Figure 1). Our study included samples with 3.78–932.85 ng, with successful sequencing at the lowest and highest ends of this range (Supplementary Table 1).
Dataset Integration
One of the greatest potentials of museum genomics is to combine these fluid specimen data with already published datasets. While studies will differ in scientific disciplines, aims, and taxonomic groups, projects should preemptively focus on how potentially-obtained data from intractable specimens will or can be combined with available datasets (or future datasets for that matter). In this study, we use the SqCL v2 (Singhal et al., 2017b) probe set as it targets three different locus types: UCEs, AHEs, and NPCGs common to squamate studies. Here, we leveraged data from studies that used traditional nuclear and mitochondrial markers (Deepak et al., 2021b), AHEs (Burbrink et al., 2020), or UCEs (Bernstein et al., unpublished data), each providing information that ultimately allowed us to determine the taxonomic affinity and biogeographic hypotheses of H. periops. We note that we obtained significantly more UCEs than AHEs and NPCGs, but this may be due to the SqCL probe set targeting thousands more UCEs compared to the other nuclear loci. Additionally, spiking libraries with unenriched pools can increase the likelihood of mitochondrial bycatch. We found that mitochondrial protein coding genes (e.g., 16S, ATPase, COX1, and cyt-b) were more often recovered than tRNAs (Supplementary Table 6). Future studies that standardize the nuclear and mitochondrial loci targeted, as well as specimen sampling, might identify patterns that show specific types of loci are more likely to be sequenced than others. Regardless of the loci being targeted, we suggest the sampling of a conspecific or congener from fresh tissues to aid in downstream analyses (see below).
Bioinformatics and Locus Acquisition
The bioinformatics phase of museum genomics depends on the amount of DNA that was successfully sequenced, but pipelines may still run to completion, even with extremely short DNA fragments. We only use one pipeline here (Phyluce) and found that all steps ran to completion with no errors. However, a resulting concatenated phylogeny recovered all museum specimens in a clade near the outgroup, with extremely long branches and showing no reasonable evolutionary relationships (Supplementary Figure 4). While this is no reflection on the pipeline itself, a variety of assembly methods and parameters can be used to optimize results (e.g., ABySS with different k-values and SPAdes with k-value averaging). Averaging of k-values using SPAdes in Phyluce worked best for our data. We note that assembly took >72 h (a common computer cluster time limit if nodes are public), thus we had to subsample to 7 million reads from our paired-end raw read data when using computer nodes with 182 GB and 28 cores. This was often needed for samples that had >15 million raw reads (∼42% of our museum samples; Supplementary Table 1).
While we found that a mapping method using individual loci obtained from Phyluce was best for creating DNA alignments, we emphasize that loci should be visually checked for remaining adapter contamination. We found that even with two rounds of trimming and cleaning, partial or whole adapter sequences were still appended to one or both ends of many loci of only the formalin specimens. Sequencing a fresh sample to create a pseudoreference of the target loci (or using a reference genome if one is available) can allow for BLASTing of loci to the pseudoreference and eliminating remaining adapter contamination. Finally, we find that mapping to mitochondrial genomes in Geneious was the most efficient method for obtaining mtDNA loci, with results from Geneious obtaining better coverage of the mitochondrial genome across more specimens when compared to MitoFinder.
Terminology of Museum Genomics Specimens
In our study, we refer to our sampling as ‘museum’ or ‘intractable’ specimens. As biochemical protocols improve to increase the success rate of extracting and sequencing DNA from voucher specimens, the terminology we use to describe these processes may change as well. Currently, ‘museum,’ ‘intractable,’ ‘degraded,’ ‘fixed,’ ‘preserved,’ and ‘historic’ samples/specimens have all been used. However, this terminology may not always be accurate, and can be misleading or confusing. For example, the use of the word ‘specimen’ denotes the physical voucher animal, but this voucher is not always in a degraded or poor condition; contrarily, voucher specimens are often in great physical condition and it is the DNA and other molecular compounds that are damaged. Additionally, the term ‘museum samples’ does not distinguish between tissues of high quality (i.e., ‘fresh’ tissue) versus those that are degraded, as both tissue types likely came from natural history specimens. Furthermore, ‘preserved’ or ‘fixed’ samples may not include dried out samples that were not chemically treated, and the word ‘historic’ is ambiguous in relation to time. Though, the word ‘intractable’ may be informative in regards to sample/specimen quality; even as new protocols are developed, chemically-fixed samples will be more difficult (intractable) to sequence DNA from than freshly-extracted tissues prior to preservation. We also suggest, when known, stating the method of preservative (e.g., ‘formalin-fixed’ and ‘ethanol-fixed’ tissues) when referring to samples from liquid fixatives.
Conclusion
Museum genomics is rapidly advancing as new protocols are developed and resulting datasets are then used for a range of evolutionary studies (Guschanski et al., 2013; Mikheyev et al., 2017; Ruane and Austin, 2017; Allentoft et al., 2018; Deepak et al., 2018). Museum collections have been viewed as a window into the past of natural history, which is vital for understanding evolutionary processes, ecological dynamics, and global change (Suarez and Tsutsui, 2004; Bradley et al., 2014; Meineke et al., 2019). Morphological inspection of voucher specimens is indeed important for studying diversity and the processes that generate it, but may be hampered by poor specimen quality or changes in commonly-measured traits (Maayan et al., 2022). Opportunities provided by natural history collections have now expanded, with genetic and genomic sequencing of museum specimens facilitating species (re)discovery (Rasmussen et al., 2012) and determining past evolutionary dynamics (Mikheyev et al., 2017; Tsai et al., 2019; Roycroft et al., 2021). Our approach and methodology were successful for incorporating both nuclear and mitochondrial data for phylogenetics, the latter of which is often used in museum genomics studies due to its often-easier acquisition over nuclear loci. In this study, we maximized both nuclear and mitochondrial data from a seemingly-failed attempt at producing useful sequence data from a preserved specimen of H. periops, a poorly known snake endemic to an island biodiversity hotspot in Southeast Asia. The expectations of outcomes when conducting museum genomics projects are important for planning such studies, increasing the likelihood of success, and maximizing data use and interpretation of results. As more studies discuss both the successes, ‘failures,’ and difficulties when sequencing DNA from voucher specimens, the field of museum genomics will advance even further, as well as our knowledge of rare and even extinct species.
Data Availability Statement
The datasets presented in this study can be found in the supplementary material and in online repositories. The names of the repository/repositories and accession number(s) can be found below: https://www.ncbi.nlm.nih.gov/, PRJNA796637, https://www.ncbi.nlm.nih.gov/, PRJNA792597 and https://www.zoobank.org/, F2AA44E2-D2EF-4747-972A-652C34C2C09D. The specimen data can also be found in Supplementary Table 1. Any further queries should be directed to the corresponding author.
Ethics Statement
Ethical review and approval was not required for the animal study because this research was performed on tissues of specimens that were already deceased and on already-published data that is available on public repositories.
Author Contributions
JB contributed to the data collection, analyses, visualization, original writing of manuscript, and project conceptualization. SR contributed to the original writing of manuscript, project conceptualization, and funding for project. Both authors contributed to the article and approved the submitted version.
Funding
This work was supported by funds from Rutgers University – Newark provided to the Ruane Lab/SR, and by funds from the Field Museum of Natural History (including the Grainger Foundation and Negaunee Integrative Research Center) provided to SR.
Conflict of Interest
The authors declare that the research was conducted in the absence of any commercial or financial relationships that could be construed as a potential conflict of interest.
Publisher’s Note
All claims expressed in this article are solely those of the authors and do not necessarily represent those of their affiliated organizations, or those of the publisher, the editors and the reviewers. Any product that may be evaluated in this article, or claim that may be made by its manufacturer, is not guaranteed or endorsed by the publisher.
Acknowledgments
We acknowledge the following individuals and associated institutions for providing tissues used in DNA extraction and sequencing for this and the in-progress homalopsid study: Ned Gilmore (Academy of Natural Sciences of Philadelphia); Frank Burbrink, David Kizirian, and Lauren Vonnahme (American Museum of Natural History); Molly Hagemann (Bernice P. Bishop Museum); Rayna Bell, Lauren Scheinberg, and Erica Ely (California Academy of Sciences); Jennifer Sheridan (Carnegie Museum of Natural History); José Rosado (Museum of Comparative Zoology); David Blackburn and Coleman Sheehy III (Florida Museum of Natural History); Alan Resetar and Joshua Mata (Field Museum of Natural History); Rafe Brown and Jeffrey Weinell (KU Biodiversity Institute & Natural History Museum); Lee Grismer (La Sierra University Herpetology Collection); Christopher Austin (Louisiana State University Museum of Natural Science); Jadranka Rota and Maria Mostadius (Lund Museum of Zoology); Kevin de Queiroz and Addison Wynn (National Museum of Natural History); Gavin Dally (Museum and Art Gallery of the Northern Territory); Jimmy McGuire and Carol Spencer (Museum of Vertebrate Zoology); Esther Dondorp and Wendy van Bohemen (Naturalis Biodiversity Center); Bryan Stuart (North Carolina Museum of Natural Sciences); Patrick Couper and Andrew Amey (Queensland Museum); Bob Murphy (Royal Ontario Museum); Sally South (South Australian Museum); Bo Delling, Sven Kullander, and Andrea Hennyey (Swedish Museum of Natural History); Paul Doughty and Rebecca Bray (Western Australian Museum); Gregory Watkins-Colwell (Yale Peabody Museum of Natural History), and Chan Kin Onn (Lee Kong Chian Natural History Museum). We thank David Kizirian and Lauren Vonnahme for help with tissue importation from the South Australian Museum and Western Australian Museum, Justin Davis and Juan Flores (Rutgers University – Newark) for helpful conservations on formalinized tissues, and Huri Mucahit and Edward Bonder (Rutgers University – Newark) for the use of facilities related to DNA extractions. We acknowledge Jackson Roberts, Edward A. Myers, and Marcelo Gehara for assistance with bioinformatic pipelines and R coding. Finally, we thank W. Hosmer, the late Robert F. Inger, Tan Fui Lian, and the Sabah Parks field research team and colleagues for the collection of the original H. periops specimens during their field work in Borneo.
Supplementary Material
The Supplementary Material for this article can be found online at: https://www.frontiersin.org/articles/10.3389/fevo.2022.893088/full#supplementary-material
Supplementary Figure 1 | Linear regression models for nuclear and mitochondrial data, without the inclusion of Hydrablabes periops FMNH 251051. R2, F-, and p-values are given (α = 0.05). Trend lines shown in red.
Supplementary Figure 2 | Linear regression models for nuclear and mitochondrial data, with H. periops FMNH 251051 included in linear models. R2, F-, and p-values are given (α = 0.05). Trend lines shown in red.
Supplementary Figure 3 | Maximum likelihood trees of BDNF, ND4, cyt-b, and a concatenated tree of these loci, using sequence data from Deepak et al. (2021a). Values at nodes represent SH-aLRT (left) and UF bootstraps (right); scale bar in number of substitutions per site.
Supplementary Figure 4 | Phylogeny of Homalopsidae (fresh and degraded specimens) using a concatenated dataset of all loci obtained from the Phyluce pipeline. Degraded (Museum) specimens are shown in pink, with fresh (non-degraded specimens with high quality DNA) colored in black. Scale bar in number of substitutions per site.
Supplementary Table 1 | Specimen information, read data, and adapter and barcode sequences for all specimens used in this study. Raw read data, number of nuclear loci obtained from Geneious, and Qubit quantifications are given for museum samples only.
Supplementary Table 2 | Morphological data recorded for Hydrablabes periops specimens.
Supplementary Table 3 | Node calibrations used for treePL divergence dating for the AHE Squamata phylogeny. Fossil calibrations used, respective minimum and maximum fossil ages, and their uses in previous studies are obtained from Burbrink et al. (2020).
Supplementary Table 4 | Nuclear loci from the SqCL probe set that were extracted using BLAST in Geneious. Locus lengths are given in base pairs (bp).
Supplementary Table 5 | Successful MitoFinder results for museum specimens. Taxon, voucher ID, mitochondrial gene, and locus lengths (base pairs; bp) are given.
Supplementary Table 6 | Percentages and lengths (base pairs; bp) of mitochondrial reference genome and mitochondrial gene regions recovered for museum specimens using the mapping method in Geneious.
Footnotes
References
Alencar, L. R. V., Quental, T. B., Grazziotin, F. G., Alfaro, M. L., Martins, M., Venzon, M., et al. (2016). Diversification in vipers: phylogenetic relationships, time of divergence and shifts in speciation rates. Mol. Phylogenet. Evol. 105, 50–62. doi: 10.1016/j.ympev.2016.07.029
Alfaro, M. E., and Arnold, S. J. (2001). Molecular systematics and evolution of regina and the thamnophiine snakes. Mol. Phylogenet. Evol. 21, 408–423. doi: 10.1006/mpev.2001.1024
Allentoft, M. E., Rasmussen, A. R., and Kristensen, H. V. (2018). Centuries-Old DNA from an extinct population of aesculapian snake (zamenis longissimus) offers new phylogeographic insight. Diversity 10, 1–14. doi: 10.3390/d10010014
Allentoft, M. E., Sikora, M., Sjögren, K.-G., Rasmussen, S., Rasmussen, M., Stenderup, J., et al. (2015). Population genomics of bronze age eurasia. Nature 522, 167–172. doi: 10.1038/nature14507
Allio, R., Schomaker-Bastos, A., Romiguier, J., Prosdocimi, F., Nabholz, B., and Delsuc, F. (2020). MitoFinder: efficient automated large-scale extraction of mitogenomic data in target enrichment phylogenomics. Mol. Ecol. Resour. 20, 892–905. doi: 10.1111/1755-0998.13160
Álvarez-Carretero, S., Tamuri, A. U., Battini, M., Nascimento, F. F., Carlisle, E., Asher, R. J., et al. (2021). A species-level timeline of mammal evolution integrating phylogenomic data. Nature 2021, 1–8. doi: 10.1038/s41586-021-04341-1
Andrews, S. (2010). FastQC: A Quality Control Tool For High Throughput Sequence Data. Available online at: http://www.bioinformatics.babraham.ac.uk/projects/fastqc/ (accessed January 1, 2022)
Appleyard, S. A., Maher, S., Pogonoski, J. J., Bent, S. J., Chua, X.-Y., and McGrath, A. (2021). Assessing DNA for fish identifications from reference collections: the good, bad and ugly shed light on formalin fixation and sequencing approaches. J. Fish Biol. 98, 1421–1432. doi: 10.1111/jfb.14687
Bankevich, A., Nurk, S., Antipov, D., Gurevich, A. A., Dvorkin, M., Kulikov, A. S., et al. (2012). SPAdes: a new genome assembly algorithm and its applications to single-cell sequencing. J. Comput. Biol. 19, 455–477. doi: 10.1089/cmb.2012.0021
Bouckaert, R. R., and Drummond, A. J. (2017). bModelTest: bayesian phylogenetic site model averaging and model comparison. BMC Evol. Biol. 17:42. doi: 10.1186/s12862-017-0890-6
Bradley, R. D., Bradley, L. C., Garner, H. J., and Baker, R. J. (2014). Assessing the value of natural history collections and addressing issues regarding long-term growth and care. BioScience 64, 1150–1158. doi: 10.1093/biosci/biu166
Burbrink, F., Grazziotin, F., Pyron, R., Cundall, D., Donnellan, S., Irish, F., et al. (2019). Data from: interrogating genomic-scale data for squamata (lizards, snakes, and amphisbaenians) shows no support for key traditional morphological relationships. Dryad Digital Rep. 2019:sm6jb0. doi: 10.5061/dryad.sm6jb0p
Burbrink, F. T., Bernstein, J. M., Kuhn, A., Gehara, M., and Ruane, S. (2021). Ecological divergence and the history of gene flow in the nearctic milksnakes (Lampropeltis triangulum complex). Syst. Biol. 2021:syab093. doi: 10.1093/sysbio/syab093
Burbrink, F. T., Grazziotin, F. G., Pyron, R. A., Cundall, D., Donnellan, S., Irish, F., et al. (2020). Interrogating genomic-scale data for squamata (lizards, snakes, and amphisbaenians) shows no support for key traditional morphological relationships. Syst. Biol. 69, 502–520. doi: 10.1093/sysbio/syz062
Campos, P. F., and Gilbert, T. M. P. (2012). “DNA extraction from formalin-fixed material,” in Ancient DNA: Methods and Protocols Methods in Molecular Biology, eds B. Shapiro and M. Hofreiter (Totowa, NJ: Humana Press), 81–85. doi: 10.1007/978-1-61779-516-9_11
Card, D. C., Adams, R. H., Schield, D. R., Perry, B. W., Corbin, A. B., Pasquesi, G. I. M., et al. (2019). Genomic basis of convergent island phenotypes in boa constrictors. Genome Biol. Evol. 11, 3123–3143. doi: 10.1093/gbe/evz226
Card, D. C., Shapiro, B., Giribet, G., Moritz, C., and Edwards, S. V. (2021). Museum genomics. Annu. Rev. Genet. 55, 633–659. doi: 10.1146/annurev-genet-071719-020506
Das, I. (2018). A Naturalist’s Guide to the Snakes of Southeast Asia, 2nd Edn. Oxford, UK: John Beaufoy Publishing.
Das, I., and Wong, J. W. (2021). Predation on gonocephalus liogaster (agamidae) by ptyas carinata (colubridae) in sarawak. Borneo. Herpetol. Notes 13, 349–351.
de Bruyn, M., Stelbrink, B., Morley, R. J., Hall, R., Carvalho, G. R., Cannon, C. H., et al. (2014). Borneo and indochina are major evolutionary hotspots for southeast asian biodiversity. Syst. Biol. 63, 879–901. doi: 10.1093/sysbio/syu047
de Queiroz, A., Lawson, R., and Lemos-Espinal, J. A. (2002). Phylogenetic relationships of north American garter snakes (thamnophis) based on four mitochondrial genes: how much DNA sequence is enough? Mol. Phylogenet. Evol. 22, 315–329. doi: 10.1006/mpev.2001.1074
De Vos, J. M., Joppa, L. N., Gittleman, J. L., Stephens, P. R., and Pimm, S. L. (2015). Estimating the normal background rate of species extinction. Conserv. Biol. 29, 452–462. doi: 10.1111/cobi.12380
Deepak, V., Cooper, N., Poyarkov, N. A., Kraus, F., Burin, G., Das, A., et al. (2021a). Multilocus phylogeny, natural history traits and classification of natricine snakes (serpentes: natricinae). Zool. J. Linn. Soc. 2021:zlab099. doi: 10.1093/zoolinnean/zlab099
Deepak, V., Maddock, S. T., Williams, R., Nagy, Z. T., Conradie, W., Rocha, S., et al. (2021b). Molecular phylogenetics of sub-saharan African natricine snakes, and the biogeographic origins of the seychelles endemic lycognathophis seychellensis. Mol. Phylogenet. Evol. 161:107152. doi: 10.1016/j.ympev.2021.107152
Deepak, V., Ruane, S., and Gower, D. J. (2018). A new subfamily of fossorial colubroid snakes from the western ghats of peninsular India. J. Nat. Hist. 52, 2919–2934. doi: 10.1080/00222933.2018.1557756
Del Fabbro, C., Scalabrin, S., Morgante, M., and Giorgi, F. M. (2013). An extensive evaluation of read trimming effects on illumina NGS data analysis. PLoS One 8:e85024. doi: 10.1371/journal.pone.0085024
Do, H., and Dobrovic, A. (2015). Sequence artifacts in DNA from formalin-fixed tissues: causes and strategies for minimization. Clin. Chem. 61, 64–71. doi: 10.1373/clinchem.2014.223040
Esquerré, D., Donnellan, S., Brennan, I. G., Lemmon, A. R., Moriarty Lemmon, E., Zaher, H., et al. (2020). Phylogenomics, biogeography, and morphometrics reveal rapid phenotypic evolution in pythons after crossing wallace’s line. Syst. Biol. 69, 1039–1051. doi: 10.1093/sysbio/syaa024
Faircloth, B. C. (2011). Illumiprocessor - Software For Illumina Read Quality Filtering. Availble online at: https://github.com/faircloth-lab/illumiprocessor (accessed November 1, 2021).
Faircloth, B. C. (2016). PHYLUCE is a software package for the analysis of conserved genomic loci. Bioinformatics 32, 786–788.
Faircloth, B. C., McCormack, J. E., Crawford, N. G., Harvey, M. G., Brumfield, R. T., and Glenn, T. C. (2012). Ultraconserved elements anchor thousands of genetic markers spanning multiple evolutionary timescales. Syst. Biol. 61, 717–726. doi: 10.1093/sysbio/sys004
France, S. C., and Kocher, T. D. (1996). DNA sequencing of formalin-fixed crustaceans from archival research collections. Mol. Mar. Biol. Biotechnol. 5, 304–313.
Frazer, Z., Yoo, C., Sroya, M., Bellora, C., DeWitt, B. L., Sanchez, I., et al. (2020). Effect of different proteinase k digest protocols and deparaffinization methods on yield and integrity of DNA extracted from formalin-fixed, paraffin-embedded tissue. J. Histochem. Cytochem. 68, 171–184. doi: 10.1369/0022155420906234
Fukuyama, R., Fukuyama, I., Kurita, T., Kojima, Y., Hossman, M. Y., Noda, A., et al. (2021). New herpetofaunal records from gunung mulu national park and its surrounding areas in borneo. Herpetozoa 2021, 89–97.
Guindon, S., Dufayard, J.-F., Lefort, V., Anisimova, M., Hordijk, W., and Gascuel, O. (2010). New algorithms and methods to estimate maximum-likelihood phylogenies: assessing the performance of PhyML 3.0. Syst. Biol. 59, 307–321. doi: 10.1093/sysbio/syq010
Guo, P., Liu, Q., Xu, Y., Jiang, K., Hou, M., Ding, L., et al. (2012). Out of Asia: natricine snakes support the cenozoic beringian dispersal hypothesis. Mol. Phylogenet. Evol. 63, 825–833. doi: 10.1016/j.ympev.2012.02.021
Guo, P., Zhu, F., and Liu, Q. (2019). A new member of the genus sinonatrix (serpentes: colubridae) from western China. Zootaxa 2019:4623. doi: 10.11646/zootaxa.4623.3.5
Guo, P., Zhu, F., Liu, Q., Wang, P., Che, J., and Nguyen, T. Q. (2020). Out of the hengduan mountains: molecular phylogeny and historical biogeography of the asian water snake genus trimerodytes (squamata: colubridae). Mol. Phylogenet. Evol. 152:106927. doi: 10.1016/j.ympev.2020.106927
Guschanski, K., Krause, J., Sawyer, S., Valente, L. M., Bailey, S., Finstermeier, K., et al. (2013). Next-generation museomics disentangles one of the largest primate radiations. Syst. Biol. 62, 539–554. doi: 10.1093/sysbio/syt018
Hahn, C., Bachmann, L., and Chevreux, B. (2013). Reconstructing mitochondrial genomes directly from genomic next-generation sequencing reads—a baiting and iterative mapping approach. Nucleic Acids Res. 41:e129. doi: 10.1093/nar/gkt371
Hall, R. (2009). Southeast Asia’s changing palaeogeography. Blumea Biodivers Evol. Biogeogr. Plants 54, 148–161. doi: 10.3767/000651909X475941
Hime, P. M., Lemmon, A. R., Lemmon, E. C. M., Prendini, E., Brown, J. M., Thomson, R. C., et al. (2021). Phylogenomics reveals ancient gene tree discordance in the amphibian tree of life. Syst. Biol. 70, 49–66. doi: 10.1093/sysbio/syaa034
Hoang, D. T., Chernomor, O., von Haeseler, A., Minh, B. Q., and Vinh, L. S. (2018). UFBoot2: improving the ultrafast bootstrap approximation. Mol. Biol. Evol. 35, 518–522. doi: 10.1093/molbev/msx281
Husson, L., Boucher, F. C., Sarr, A.-C., Sepulchre, P., and Cahyarini, S. Y. (2020). Evidence of sundaland’s subsidence requires revisiting its biogeography. J. Biogeogr. 47, 843–853. doi: 10.1111/jbi.13762
Hykin, S. M., Bi, K., and McGuire, J. A. (2015). Fixing formalin: a method to recover genomic-scale DNA sequence data from formalin-fixed museum specimens using high-throughput sequencing. PLoS One 10:e0141579. doi: 10.1371/journal.pone.0141579
Jones, M. E., Anderson, C. L., Hipsley, C. A., Müller, J., Evans, S. E., and Schoch, R. R. (2013). Integration of molecules and new fossils supports a triassic origin for lepidosauria (lizards, snakes, and tuatara). BMC Evol. Biol. 13:208. doi: 10.1186/1471-2148-13-208
Kalyaanamoorthy, S., Minh, B. Q., Wong, T. K. F., von Haeseler, A., and Jermiin, L. S. (2017). ModelFinder: fast model selection for accurate phylogenetic estimates. Nat. Methods 14, 587–589. doi: 10.1038/nmeth.4285
Kindler, C., Bringsøe, H., and Fritz, U. (2014). Phylogeography of grass snakes (natrix natrix) all around the baltic sea: implications for the holocene colonization of fennoscandia. Amphib Reptil. 35, 413–424. doi: 10.1163/15685381-00002962
Köhler, G., Khaing, K. P. P., Than, N. L., Baranski, D., Schell, T., Greve, C., et al. (2021). A new genus and species of mud snake from myanmar (reptilia, squamata, homalopsidae). Zootaxa 4915, 301–325. doi: 10.11646/zootaxa.4915.3.1
Lalronunga, S., Lalrinchhana, C., Vanramliana, V., Das, A., Gower, D. J., and Deepak, V. (2020). A multilocus molecular perspective on the systematics of the poorly known northeast indian colubrid snakes blythia reticulata (blyth, 1854), b. hmuifang vogel, lalremsanga amp; vanlalhrima, 2017, and hebius xenura (wall, 1907). Zootaxa 2020:4768. doi: 10.11646/zootaxa.4768.2.2
Lanfear, R., Frandsen, P. B., Wright, A. M., Senfeld, T., and Calcott, B. (2017). PartitionFinder 2: new methods for selecting partitioned models of evolution for molecular and morphological phylogenetic analyses. Mol. Biol. Evol. 34, 772–773. doi: 10.1093/molbev/msw260
Lemmon, A. R., Emme, S. A., and Lemmon, E. M. (2012). Anchored hybrid enrichment for massively high-throughput phylogenomics. Syst. Biol. 61, 727–744. doi: 10.1093/sysbio/sys049
Linck, E. B., Hanna, Z. R., Sellas, A., and Dumbacher, J. P. (2017). Evaluating hybridization capture with RAD probes as a tool for museum genomics with historical bird specimens. Ecol. Evol. 7, 4755–4767. doi: 10.1002/ece3.3065
Lohse, M., Bolger, A. M., Nagel, A., Fernie, A. R., Lunn, J. E., Stitt, M., et al. (2012). RobiNA: a user-friendly, integrated software solution for RNA-Seq-based transcriptomics. Nucleic Acids Res. 40, W622–W627. doi: 10.1093/nar/gks540
Maayan, I., Reynolds, R. G., Goodman, R. M., Hime, P. M., Bickel, R., Luck, E. A., et al. (2022). Fixation and preservation contribute to distortion invertebrate museum specimens: a 10-year study with the lizard anolis sagrei. Biol. J. Linn. Soc. 2022:blac040.
McCormack, J. E., Tsai, W. L. E., and Faircloth, B. C. (2016). Sequence capture of ultraconserved elements from bird museum specimens. Mol. Ecol. Resour. 16, 1189–1203. doi: 10.1111/1755-0998.12466
McGuire, J. A., Cotoras, D. D., O’Connell, B., Lawalata, S. Z. S., Wang-Claypool, C. Y., Stubbs, A., et al. (2018). Squeezing water from a stone: high-throughput sequencing from a 145-year old holotype resolves (barely) a cryptic species problem in flying lizards. PeerJ 6:e4470. doi: 10.7717/peerj.4470
McVay, J. D., and Carstens, B. (2013). Testing monophyly without well-supported gene trees: evidence from multi-locus nuclear data conflicts with existing taxonomy in the snake tribe thamnophiini. Mol. Phylogenet. Evol. 68, 425–431. doi: 10.1016/j.ympev.2013.04.028
McVay, J. D., Flores-Villela, O., and Carstens, B. (2015). Diversification of north american natricine snakes. Biol. J. Linn. Soc. 116, 1–12. doi: 10.1111/bij.12558
Meineke, E. K., Davies, T. J., Daru, B. H., and Davis, C. C. (2019). Biological collections for understanding biodiversity in the anthropocene. Philos. Trans. R. Soc. B Biol. Sci. 374:20170386. doi: 10.1098/rstb.2017.0386
Mikheyev, A. S., Zwick, A., Magrath, M. J. L., Grau, M. L., Qiu, L., Su, Y. N., et al. (2017). Museum genomics confirms that the lord howe island stick insect survived extinction. Curr. Biol. 27, 3157–3161.e4. doi: 10.1016/j.cub.2017.08.058
Mocquard, F. (1890). Recherches sur la faune herpétologique des îles de bornèo et de palawan. Arch. Muséum Natl. Hist. Nat. Paris 3, 115–168.
Murphy, J. C., and Voris, H. K. (2014). A checklist and key to the homalopsid snakes (reptilia, squamata, serpentes), with the description of new genera. Fieldiana Life Earth Sci. 2014, 1–43. doi: 10.3158/2158-5520-14.8.1
Nagy, Z. T., Vidal, N., Vences, M., Branch, W. R., Pauwels, O. S. G., Wink, M., et al. (2005). “Molecular systematics of african colubroidea (squamata: serpentes),” in African Biodiversity, eds B. A. Huber, B. J. Sinclair, and K.-H. Lampe (Boston, MA: Springer), 221–228. doi: 10.1007/0-387-24320-8_20
Nguyen, L.-T., Schmidt, H. A., von Haeseler, A., and Minh, B. Q. (2015). IQ-TREE: a fast and effective stochastic algorithm for estimating maximum-likelihood phylogenies. Mol. Biol. Evol. 32, 268–274. doi: 10.1093/molbev/msu300
Nurk, S., Meleshko, D., Korobeynikov, A., and Pevzner, P. A. (2017). metaSPAdes: a new versatile metagenomic assembler. Genome Res. 27, 824–834. doi: 10.1101/gr.213959.116
O’Connell, K. A., Mulder, K. P., Wynn, A., Queiroz, K., and Bell, R. C. (2021). Genomic library preparation and hybridization capture of formalin-fixed tissues and allozyme supernatant for population genomics and considerations for combining capture- and RADseq-based single nucleotide polymorphism data sets. Mol. Ecol. Resour. 2021:13481. doi: 10.1111/1755-0998.13481
Ogilvie, H. A., Bouckaert, R. R., and Drummond, A. J. (2017). StarBEAST2 brings faster species tree inference and accurate estimates of substitution rates. Mol. Biol. Evol. 34, 2101–2114. doi: 10.1093/molbev/msx126
Pimm, S. L., Jenkins, C. N., Abell, R., Brooks, T. M., Gittleman, J. L., Joppa, L. N., et al. (2014). The biodiversity of species and their rates of extinction, distribution, and protection. Science 344:1246752. doi: 10.1126/science.1246752
Portik, D. M., and Wiens, J. J. (2021). Do alignment and trimming methods matter for phylogenomic (UCE) analyses? Syst. Biol. 70, 440–462. doi: 10.1093/sysbio/syaa064
Pyron, R. A., Beamer, D. A., Holzheuser, C. R., Lemmon, E. M., Lemmon, A. R., Wynn, A. H., et al. (2022). Contextualizing enigmatic extinctions using genomic DNA from fluid-preserved museum specimens of Desmognathus salamanders. Conserv. Genet. 23, 375–386. doi: 10.1007/s10592-021-01424-4
Pyron, R. A., Kandambi, H. K. D., Hendry, C. R., Pushpamal, V., Burbrink, F. T., and Somaweera, R. (2013). Genus-level phylogeny of snakes reveals the origins of species richness in Sri Lanka. Mol. Phylogenet. Evol. 66, 969–978. doi: 10.1016/j.ympev.2012.12.004
Quah, E. S. H., Grismer, L. L., Lim, K. K. P., Anuar, M. S. S., and Imbun, A. Y. (2019). A taxonomic reappraisal of the smooth slug snake asthenodipsas laevis (boie, 1827) (squamata: pareidae) in borneo with the description of two new species. Zootaxa 2019:4646. doi: 10.11646/zootaxa.4646.3.4
R Core Team (2021). R: A Language And Environment For Statistical Computing. Vienna, Austria: R Foundation for Statistical Computing.
Rambaut, A., Drummond, A. J., Xie, D., Baele, G., and Suchard, M. A. (2018). Posterior summarization in bayesian phylogenetics using Tracer 1.7. Syst. Biol. 67, 901–904. doi: 10.1093/sysbio/syy032
Rancilhac, L., Bruy, T., Scherz, M. D., Pereira, E. A., Preick, M., Straube, N., et al. (2020). Target-enriched DNA sequencing from historical type material enables a partial revision of the madagascar giant stream frogs (genus mantidactylus). J. Nat. Hist. 54, 87–118. doi: 10.1080/00222933.2020.1748243
Rasmussen, A. R., Elmberg, J., Sanders, K. L., and Gravlund, P. (2012). Rediscovery of the rare sea snake hydrophis parviceps smith 1935: identification and conservation status. Copeia 2012, 276–282. doi: 10.1643/CH-11-116
Ren, J., Wang, K., Guo, P., Wang, Y.-Y., Nguyen, T., and Li, J. (2019). On the generic taxonomy of opisthotropis balteata (cope, 1895) (squamata: colubridae: natricinae): taxonomic revision of two natricine genera. Asian Herpetol. Res. 10, 105–128. doi: 10.16373/j.cnki.ahr.180091
Rohland, N., Siedel, H., and Hofreiter, M. (2004). Nondestructive DNA extraction method for mitochondrial DNA analyses of museum specimens. BioTechniques 36, 814–821. doi: 10.2144/04365ST05
Roycroft, E., MacDonald, A. J., Moritz, C., Moussalli, A., Portela Miguez, R., and Rowe, K. C. (2021). Museum genomics reveals the rapid decline and extinction of Australian rodents since European settlement. Proc. Natl. Acad. Sci. U.S.A. 118:e2021390118. doi: 10.1073/pnas.2021390118
Ruane, S. (2021). New data from old specimens. Ichthyol. Herpetol. 109, 392–396. doi: 10.1643/t2019293
Ruane, S., and Austin, C. C. (2017). Phylogenomics using formalin-fixed and 100+ year-old intractable natural history specimens. Mol. Ecol. Resour. 17, 1003–1008. doi: 10.1111/1755-0998.12655
Sarr, A.-C., Husson, L., Sepulchre, P., Pastier, A.-M., Pedoja, K., Elliot, M., et al. (2019). Subsiding sundaland. Geology 47, 119–122. doi: 10.1130/G45629.1
Schield, D. R., Pasquesi, G. I. M., Perry, B. W., Adams, R. H., Nikolakis, Z. L., Westfall, A. K., et al. (2020). Snake recombination landscapes are concentrated in functional regions despite PRDM9. Mol. Biol. Evol. 37, 1272–1294. doi: 10.1093/molbev/msaa003
Simmons, J. E. (2014). Fluid Preservation: A Comprehensive Reference. Lanhan, MD: Rowman & Littlefield.
Simpson, J. T., Wong, K., Jackman, S. D., Schein, J. E., Jones, S. J. M., and Birol, I. (2009). ABySS: a parallel assembler for short read sequence data. Genome Res. 19, 1117–1123. doi: 10.1101/gr.089532.108
Singhal, S., Grundler, M., Colli, G., and Rabosky, D. L. (2017a). Data from: squamate conserved loci (SqCL): a unified set of conserved loci for phylogenomics and population genetics of squamate reptiles. Dryad Digital Repository 2017:r0q02. doi: 10.5061/dryad.r0q02
Singhal, S., Grundler, M., Colli, G., and Rabosky, D. L. (2017b). Squamate conserved loci (SqCL: a unified set of conserved loci for phylogenomics and population genetics of squamate reptiles. Mol. Ecol. Resour. 17, e12–e24. doi: 10.1111/1755-0998.12681
Smith, S. A., and O’Meara, B. C. (2012). treePL: divergence time estimation using penalized likelihood for large phylogenies. Bioinformatics 28, 2689–2690. doi: 10.1093/bioinformatics/bts492
Sodhi, N. S., Koh, L. P., Brook, B. W., and Ng, P. K. L. (2004). Southeast Asian biodiversity: an impending disaster. Trends Ecol. Evol. 19, 654–660. doi: 10.1016/j.tree.2004.09.006
Strang, K., and Rusli, N. (2021). “The challenges of conserving biodiversity: a spotlight on southeast asia,” in Wildlife Biodiversity Conservation: Multidisciplinary and Forensic Approaches, eds S. C. Underkoffler and H. R. Adams (Cham: Springer International Publishing), 47–66. doi: 10.1007/978-3-030-64682-0_3
Stuckert, A. M. M., Chouteau, M., McClure, M., LaPolice, T. M., Linderoth, T., Nielsen, R., et al. (2021). The genomics of mimicry: gene expression throughout development provides insights into convergent and divergent phenotypes in a müllerian mimicry system. Mol. Ecol. 30, 4039–4061. doi: 10.1111/mec.16024
Stuebing, R. B., Inger, R. F., and Lardner, B. (2014). A Field Guide to the Snakes of Borneo. Kota Kinabalu: Natural History Publications.
Suarez, A. V., and Tsutsui, N. D. (2004). The value of museum collections for research and society. BioScience 54, 66–74.
Tsai, W. L. E., Mota-Vargas, C., Rojas-Soto, O., Bhowmik, R., Liang, E. Y., Maley, J. M., et al. (2019). Museum genomics reveals the speciation history of dendrortyx wood-partridges in the mesoamerican highlands. Mol. Phylogenet. Evol. 136, 29–34. doi: 10.1016/j.ympev.2019.03.017
Uetz, P., Freed, P., Aguilar, R., and Hošek, J. (2021). The Reptile Database. Available online at: http://www.reptile-database.org (accessed February 1, 2022)
Voris, H. K. (2000). Maps of pleistocene sea levels in southeast asia: shorelines, river systems and time durations. J. Biogeogr. 27, 1153–1167. doi: 10.1046/j.1365-2699.2000.00489.x
Weinell, J. L., and Brown, R. M. (2018). Discovery of an old, archipelago-wide, endemic radiation of Philippine snakes. Mol. Phylogenet. Evol. 119, 144–150. doi: 10.1016/j.ympev.2017.11.004
Westeen, E. P., Durso, A. M., Grundler, M. C., Rabosky, D. L., and Davis Rabosky, A. R. (2020). What makes a fang? Phylogenetic and ecological controls on tooth evolution in rear-fanged snakes. BMC Evol. Biol. 20:80. doi: 10.1186/s12862-020-01645-0
Wingett, S. W., and Andrews, S. (2018). FastQ screen: a tool for multi-genome mapping and quality control. F1000Research 7:1338. doi: 10.12688/f1000research.15931.2
Wood, H. M., González, V. L., Lloyd, M., Coddington, J., and Scharff, N. (2018). Next-generation museum genomics: phylogenetic relationships among palpimanoid spiders using sequence capture techniques (araneae: palpimanoidea). Mol. Phylogenet. Evol. 127, 907–918. doi: 10.1016/j.ympev.2018.06.038
Yan, J., Li, H., and Zhou, K. (2008). Evolution of the mitochondrial genome in snakes: gene rearrangements and phylogenetic relationships. BMC Geno. 9:569. doi: 10.1186/1471-2164-9-569
Zacho, C. M., Bager, M. A., Margaryan, A., Gravlund, P., Galatius, A., Rasmussen, A. R., et al. (2021). Uncovering the genomic and metagenomic research potential in old ethanol-preserved snakes. PLoS One 16:e0256353. doi: 10.1371/journal.pone.0256353
Zaher, H., Yánez-Muñoz, M. H., Rodrigues, M. T., Graboski, R., Machado, F. A., Altamirano-Benavides, M., et al. (2018). Origin and hidden diversity within the poorly known galápagos snake radiation (serpentes: dipsadidae). Syst. Biodivers. 16, 614–642. doi: 10.1080/14772000.2018.1478910
Zhang, C., Rabiee, M., Sayyari, E., and Mirarab, S. (2018). ASTRAL-III: polynomial time species tree reconstruction from partially resolved gene trees. BMC Bioinform. 19:153. doi: 10.1186/s12859-018-2129-y
Keywords: formalin, Hydrablabes, museum genomics, Natricidae, natural history collections, phylogenomics, snakes, systematics
Citation: Bernstein JM and Ruane S (2022) Maximizing Molecular Data From Low-Quality Fluid-Preserved Specimens in Natural History Collections. Front. Ecol. Evol. 10:893088. doi: 10.3389/fevo.2022.893088
Received: 10 March 2022; Accepted: 30 May 2022;
Published: 30 June 2022.
Edited by:
Jimmy McGuire, University of California, Berkeley, United StatesReviewed by:
Alex Pyron, George Washington University, United StatesJairo Arroyave, National Autonomous University of Mexico, Mexico
Copyright © 2022 Bernstein and Ruane. This is an open-access article distributed under the terms of the Creative Commons Attribution License (CC BY). The use, distribution or reproduction in other forums is permitted, provided the original author(s) and the copyright owner(s) are credited and that the original publication in this journal is cited, in accordance with accepted academic practice. No use, distribution or reproduction is permitted which does not comply with these terms.
*Correspondence: Justin M. Bernstein, jmbernst223@gmail.com