- 1Modeling for Disease Control Improvement Lab, Department of Pathobiology, College of Veterinary Medicine, University of Illinois Urbana-Champaign, Urbana, IL, United States
- 2Applied Computational Ecology Lab, College of Natural Resources, University of Missouri, Columbia, MO, United States
Background: Globally, tick-borne disease is a pervasive and worsening problem that impacts human and domestic animal health, livelihoods, and numerous economies. Species distribution models are useful tools to help address these issues, but many different modeling approaches and environmental data sources exist.
Objective: We conducted a scoping review that examined all available research employing species distribution models to predict occurrence and map tick species to understand the diversity of model strategies, environmental predictors, tick data sources, frequency of climate projects of tick ranges, and types of model validation methods.
Design: Following the PRISMA-ScR checklist, we searched scientific databases for eligible articles, their references, and explored related publications through a graphical tool (www.connectedpapers.com). Two independent reviewers performed article selection and characterization using a priori criteria.
Results: We describe data collected from 107 peer-reviewed articles that met our inclusion criteria. The literature reflects that tick species distributions have been modeled predominantly in North America and Europe and have mostly modeled the habitat suitability for Ixodes ricinus (n = 23; 21.5%). A wide range of bioclimatic databases and other environmental correlates were utilized among models, but the WorldClim database and its bioclimatic variables 1–19 appeared in 60 (56%) papers. The most frequently chosen modeling approach was MaxEnt, which also appeared in 60 (56%) of papers. Despite the importance of ensemble modeling to reduce bias, only 23 papers (21.5%) employed more than one algorithm, and just six (5.6%) used an ensemble approach that incorporated at least five different modeling methods for comparison. Area under the curve/receiver operating characteristic was the most frequently reported model validation method, utilized in nearly all (98.9%) included studies. Only 21% of papers used future climate scenarios to predict tick range expansion or contraction. Regardless of the representative concentration pathway, six of seven genera were expected to both expand and retract depending on location, while Ornithodoros was predicted to only expand beyond its current range.
Conclusion: Species distribution modeling techniques are useful and widely employed tools for predicting tick habitat suitability and range movement. However, the vast array of methods, data sources, and validation strategies within the SDM literature support the need for standardized protocols for species distribution and ecological niche modeling for tick vectors.
Introduction
An urgency in understanding the geographic occurrence and epidemiology of emerging zoonotic pathogens has led to the continued interest in describing the climate and habitat suitability for ticks and tick-borne diseases (Tkadlec et al., 2018; MacDonald et al., 2019). Due to their impact on human and veterinary health, livelihoods, and numerous global economies (Jongejan and Uilenberg, 2004), identifying and prioritizing interventions for surveillance, prevention, and control of ticks is of the utmost importance (Signorini et al., 2019; Zanet et al., 2020). Globally, ticks and their associated pathogens cost billions of dollars annually in control measures, lost revenue due to livestock infestations and infection, and medical care (Jongejan and Uilenberg, 2004). Therefore, developing tools that identify suitable habitat for ticks can assist with creating strategies to slow the spread of both the ticks and tick-borne diseases, and further the understanding of environmental factors necessary for tick survival and reproduction (Wilson, 1996; Hahn et al., 2016). Knowledge of how climatic and habitat characteristics determine patterns of tick presence can be accomplished using species distribution models (SDM, Estrada-Peña et al., 2016).
Species distribution models, also referred to as ecological niche models and habitat suitability models [despite differences in depth of focus on defining fundamental species niches among these approaches (Peterson and Soberón, 2012)], represent a suite of statistical and machine-learning tools for predicting suitable species habitat ranges and niches based on correlated environmental conditions (Guisan and Zimmermann, 2000; Franklin, 2010; Peterson et al., 2011). These strategies range from deterministic (e.g., logistic regression) to stochastic (e.g., Bayesian regression trees) approaches, and utilize numerous model validation techniques. With the introduction of the BIOCLIM bioclimatic dataset in the mid-1980s, SDMs were applied to an increasing number of organisms over vast geographies (Booth, 2018). Improvements in modeling power and complexity occurred over the next several decades, but noted limitations still exist in both inherent sampling bias (Phillips et al., 2009; Eisen and Eisen, 2021; Mader et al., 2021) and autocorrelation (Veloz, 2009) of data sources, model assumptions (Stockwell and Peterson, 2002; Lobo et al., 2008; Peterson et al., 2008), and a lack of standardized reporting approaches to modeling parameters to ensure reproducibility (Feng et al., 2019; Wunderlich et al., 2019). Additionally, performance comparisons demonstrate that not all models are equally robust or appropriate depending on the species and its ecology (Stockwell and Peterson, 2002; Qiao et al., 2015; Wunderlich et al., 2019), therefore documenting the variety of SDMs used to predict tick distributions is critical to more thoroughly understanding the extent of their abilities and their limitations.
Predicting the global distributions of tick species often involves the use of climate projections to account for forecasted changes in abiotic conditions which subsequently affect tick survival. To effectively incorporate abiotic variables, many models use bioclimatic variables that summarize raster data derived from mean monthly temperature and precipitation to estimate climate ranges meaningful for biological species (1 Fick and Hijmans, 2017). Of the 19 bioclimatic variables available, only 15 are now considered to be suitable for habitat suitability modeling due to presence of spatial artifacts among estimates of mean temperature of wettest and driest quarter (BIO 8–9) and precipitation of warmest and driest quarter (BIO 18–19; Escobar et al., 2014). In concert with spatial data on vegetation composition and/or elevation data, SDMs can be incorporated into climate projections using either Intergovernmental Panel on Climate Change (IPCC) climate scenarios or Representative Concentration Pathways (R) with or without the use of Global Circulation Models (GCMs). Global Circulation Models are mathematical representations of the physical processes in the atmosphere, ocean, cryosphere and land surface, where with the many GCMs available, it is crucial to distinguish between the nuances in how climate values are estimated and how these results impact spatial variability in species distributions (Guevara et al., 2019). In contrast, climate and RCP emission scenarios (e.g., A2, A2A, B2A, RCP 4.5, RCP 6.0, RCP 8.5) represent predictions of climate outcomes based on greenhouse gas and aerosol concentrations and land use change (Jubb et al., 2013). For example, RCP 4.5 is the lowest-emission scenario where atmospheric carbon is 650 parts per million (ppm) and stabilizes after the year 2100, whereas RCP 8.5 is the highest-emission scenario with > 1,370 ppm atmospheric carbon (Arora et al., 2011; Jubb et al., 2013). Combining both GCMs and climate projection scenarios allow for a greater understanding of how tick species distributions can potentially shift because of suitable habitat availability.
Species distribution models are relatively new in their application to tick vectors, and a standardized approach for reproducibility does not exist for data inclusion, model ensembles, and validation methods (Feng et al., 2019). Additionally, the focus on projecting distributions based on future climate scenarios for different species presents multiple possibilities for tick control and mitigation. These reasons make a scoping review a practical and useful approach to systematically examine, evaluate, and identify any gaps within the literature on this topic. A previous scoping review demonstrated the gaps in SDM studies involving ticks within the Amblyomma genus in conjunction with rickettsial bacteria, a major global pathogen of human and veterinary concern (Lippi et al., 2021a). Similarly, a recent systematic review sought to examine the past 20 years of distribution modeling on ticks and tick-borne pathogens, with a focused meta-analysis on 20 SDM studies conducted in Africa (Zannou et al., 2021). Our study sought to understand the state of SDM research surrounding all tick vectors since the beginning of SDM algorithms because differences in habitat requirements across species may impact the types of algorithms, environmental correlates, and modeling approaches chosen.
We conducted a scoping review examining all available peer-reviewed literature that employed habitat suitability and species distribution models to predict occurrence of tick species for the purposes of documenting the diversity of model algorithms and strategies, abiotic and biotic environmental predictors, tick occurrence data sources, types of model validation methods, and frequency and source of climate projection data for forecasting of future tick occurrence.
Materials and Methods
Following the Preferred Reporting Items for Systematic Reviews and Meta-analyses (PRISMA) guidelines for scoping reviews (Tricco et al., 2018), we conducted literature searches through August 2021, using the databases Web of Science2 and PubMed3 since evidence suggests their keyword search tools together offer the best article update frequency and longitudinal coverage (Falagas et al., 2008). Our search criteria for all databases included ‘‘ticks’’ [AND] ‘‘species distribution model,’’ [OR] ‘‘habitat suitability model,’’ [OR] ‘‘ecological niche model.’’ We selected all resulting peer-reviewed articles published in English, or with English translations, with no publication date or study region exclusions. Secondary and tertiary literature searches for additional publications meeting these search criteria were conducted by reviewing references from the initial search results, and by entering the original search results into Connected Papers4, a graphical literature search tool that uses the concepts of co-citation and bibliographic coupling to compile relevant paper lists and graphics from Semantic Scholar Paper Corpus (Ammar et al., 2018). Duplicate papers were removed, and the final list was examined for eligibility by two independent reviewers.
We excluded records that were not primary research articles (e.g., reviews or synthesis articles), and did not include a statistical or machine learning modeling algorithm for evaluating tick distribution (e.g., articles that included a simple description of habitat types that a tick was found occupying). The remaining articles were assessed for relevant information including year of publication, where the study was published or conducted, study aims/purpose, tick species, modeling methodology, type of surveillance data used (active/passive), environmental or other correlative variables, and climate projections. Data were extracted and charted from the full-text versions of the articles. Currently, scoping review protocols are not eligible for registration in PROSPERO, so we instead deposited this protocol and relevant files in Open Science Framework5.
Results
Our literature search, after duplicate article removal and examination of references and related papers, revealed 138 peer-reviewed articles on species distribution models and tick vectors that were assessed for eligibility (Figure 1). Fifteen studies were removed from consideration because they lacked a statistical algorithm that evaluated tick occurrence based on abiotic or biotic environmental correlates. Six papers were excluded from analysis because they were reviews, not a primary literature article, or were gray literature (i.e., not peer-reviewed), and 10 papers were removed from analysis because they focused the ecological niche analysis primarily on a tick-borne pathogen or a non-tick animal. A total of 107 full-text articles were included in the final analysis (Figure 1).
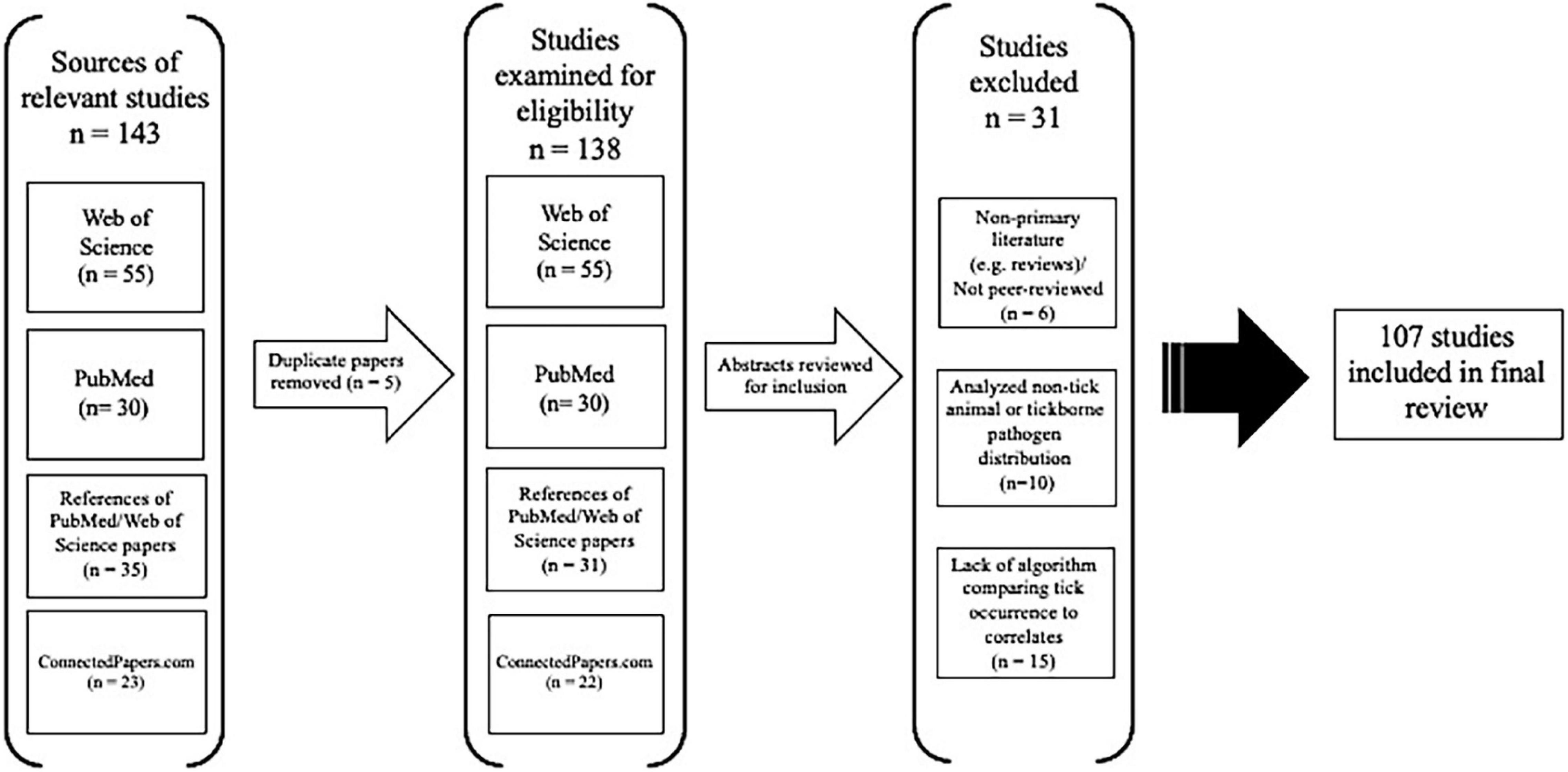
Figure 1. Flow chart of tick species distribution model (SDM) article inclusion and exclusion based on PRISMA criteria.
Species Geographic Representation Over Time
Within this collection of 107 papers, 95 tick species representing 12 genera (Table 1) were modeled across six continents between the years of 1998 to 2021 (Figure 2). Seventy-three percent of these studies were published within the past decade (Figure 2). The most frequently modeled species was Ixodes ricinus (23 papers), followed by Ixodes scapularis (16 papers), Amblyomma americanum (10 papers), Rhipicephalus microplus (10 papers), Dermacentor marginatus (nine papers), and Dermacentor variabilis (nine papers) (Table 1). Most tick distribution modeling studies represented species that are endemic to or invading the North American continent (51%; 55/107), followed by those species present in Europe (30%; 32/107), Africa (26%; 28/107), Asia (15%; 16/107), Australia (2.8%; 3/107), and lastly, two studies modeled Rhipicephalus species that have distributions spanning several continents (Figure 3).
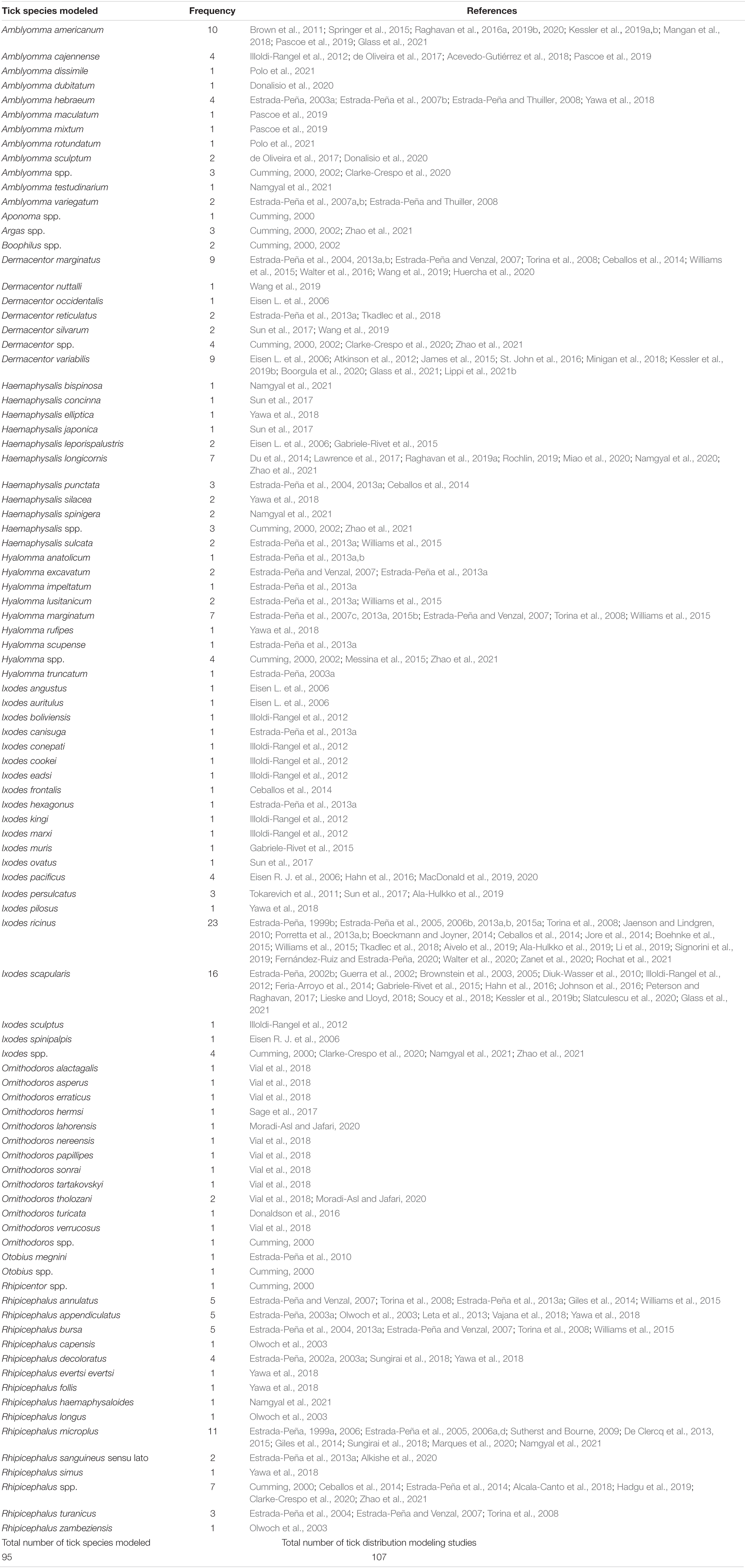
Table 1. Summary of tick species (n = 95) modeled across all included literature (n = 107), and frequency of each species’ appearance.
Tick Occurrence and Environmental Data Sources
Tick occurrence records were sourced from a variety of collections worldwide that included both active (n = 21) and passive (n = 16) surveillance methods, often both within a single paper (n = 106). The most frequently used source of tick location data was published literature records (n = 62), followed by field collections (n = 30) conducted by the authors (Figure 4). Four publicly accessible, internet-based databases of tick occurrence records were also observed in the literature, namely the Global Biodiversity Information Facility (GBIF) (n = 8), Walter Reed Biosystematics Unit (WBRU) (n = 7), VectorMap (n = 6), and Biodiversity Information Serving our Nation (BISON) (n = 1) (Figure 4).
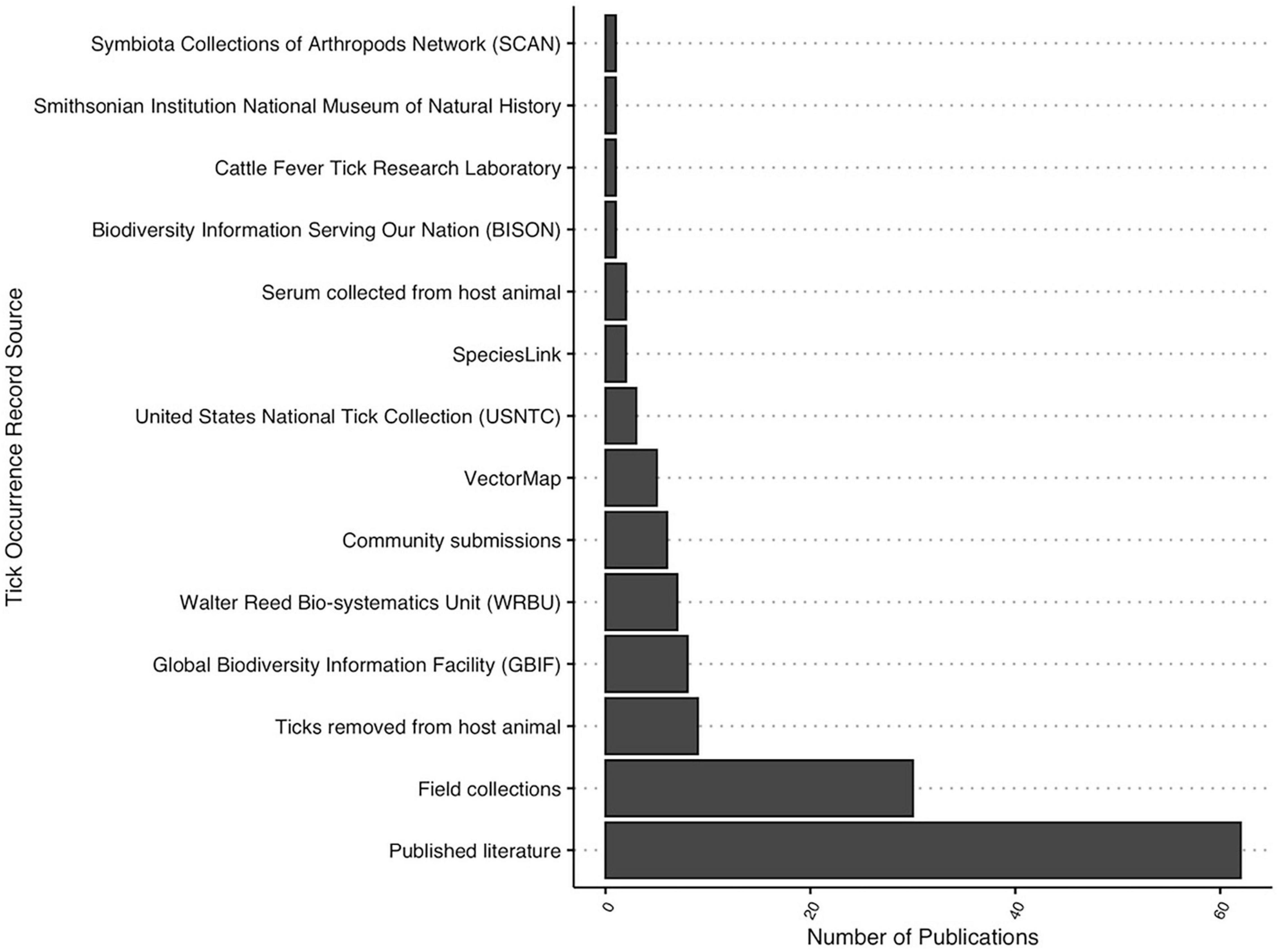
Figure 4. Sources (n = 14) of tick occurrence data reported within included distribution modeling papers.
There were 60 different databases and sources of environmental and other correlates used across the included literature (Supplementary Table 1). The most frequently used database for abiotic variables was WorldClim, included in just over half (56%) of papers. Variables representing tick host presence were rarely included and appeared in the form of host availability in only two papers (Li et al., 2019; Miao et al., 2020).
The finest scale resolution observed for environmental correlates was 15 m2 for elevation and land cover data extracted from the Southern Ontario Land Resource Information System (SOLRISv3.0) (Soucy et al., 2018; Slatculescu et al., 2020), while the coarsest scale observed was 600 km2 for rainfall, temperature, vegetation type, NDVI, and elevation from the Center for Resource and Environmental Studies (Cumming, 2002; Supplementary Table 1). Most papers examined tick distribution probabilities at a spatial resolution between 1 and 10 km2 (n = 65) (Supplementary Table 1). Analyses in 12 papers were conducted at a spatial resolution less than 1 km2, and tick species distribution modeling was performed in 18 papers at spatial resolutions greater than 10 km2 (Supplementary Table 1). Finally, 12 papers did not report the spatial resolution at which the analysis was performed.
Modeling and Validation Methods
Thirty-two different habitat distribution modeling methods were represented in this sample of publications. The most frequently chosen modeling approaches were maximum entropy, otherwise known as MaxEnt, which appeared in 56% of papers (n = 60), followed by generalized linear models (n = 24; 22.4%) (Figure 5 and Table 2). The remaining methods were used nine times or fewer, each accounting for less than 10% of the remaining papers (Figure 5 and Table 2). Only 23 papers (21.5%) employed more than one algorithm, and just six (5.6%) used an ensemble approach that incorporated at least five different modeling methods for comparison and weighted averaging across the interpolated surface (Table 2).
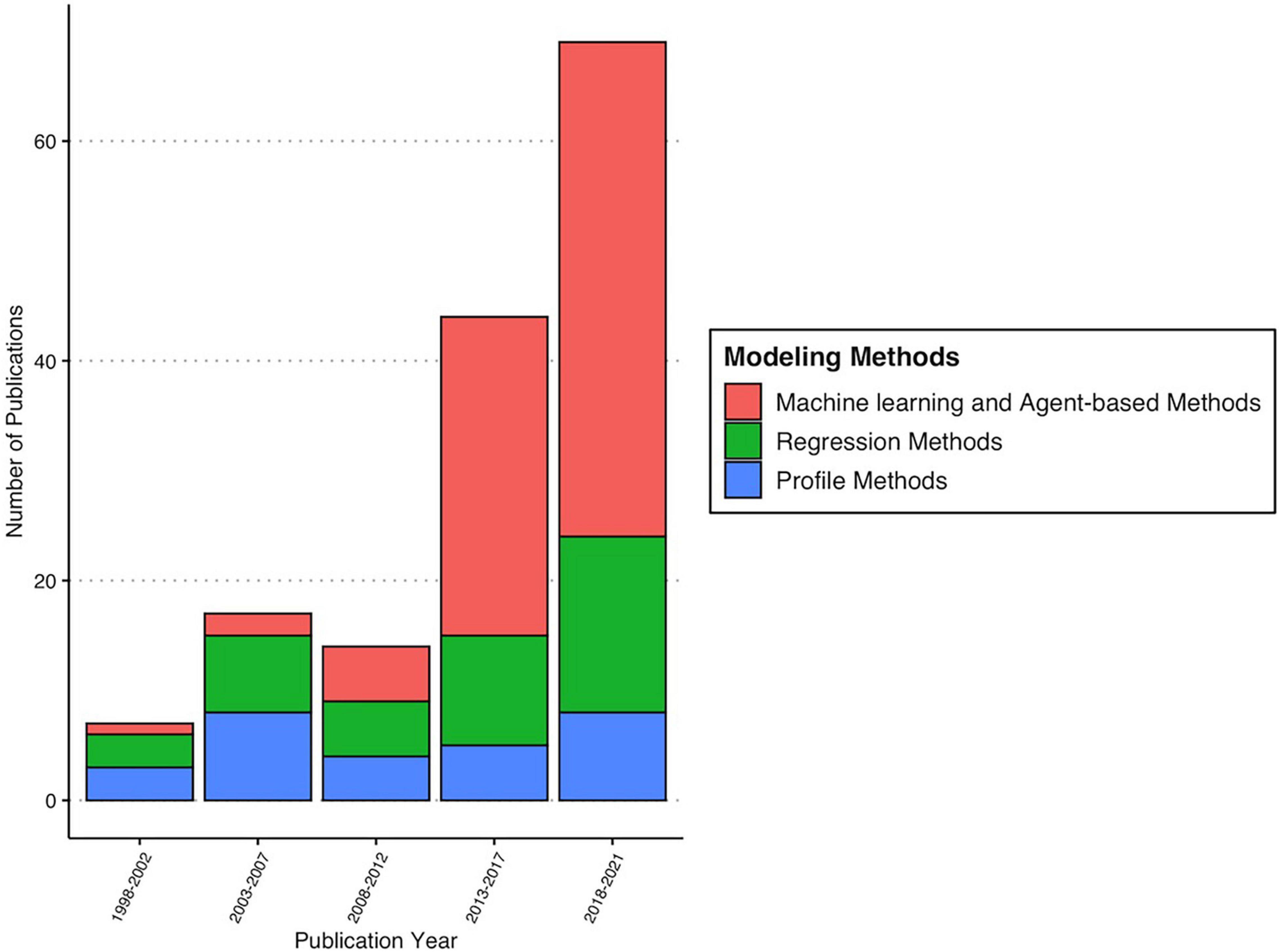
Figure 5. Tick distribution modeling methods* used in included literature over time. *Machine learning/agent-based methods include MaxEnt, genetic algorithm for rule-set prediction, agent-based modeling, boosted regression trees, random forest, and classification and regression trees. Profile methods include DOMAIN procedure, ecological niche factor analysis, bioclimatic envelope model, Cokriging, principal component analysis, ANCOVA, climate response model (CLIMEX), correspondence analysis, Gower metric, joint distribution modeling, linear discriminant analysis, mixture discriminant analysis, multi-criteria decision analysis, minimum convex polygon, occupancy models, point-to-point similarity metric, spatial clustering, and Fourier analysis. Regression methods include generalized linear models, generalized additive models, multivariate adaptive regression splines, mixed effect logistic regression, negative binomial regression, Poisson regression, zero-inflated Poisson regression, and zero-inflated negative binomial regression.
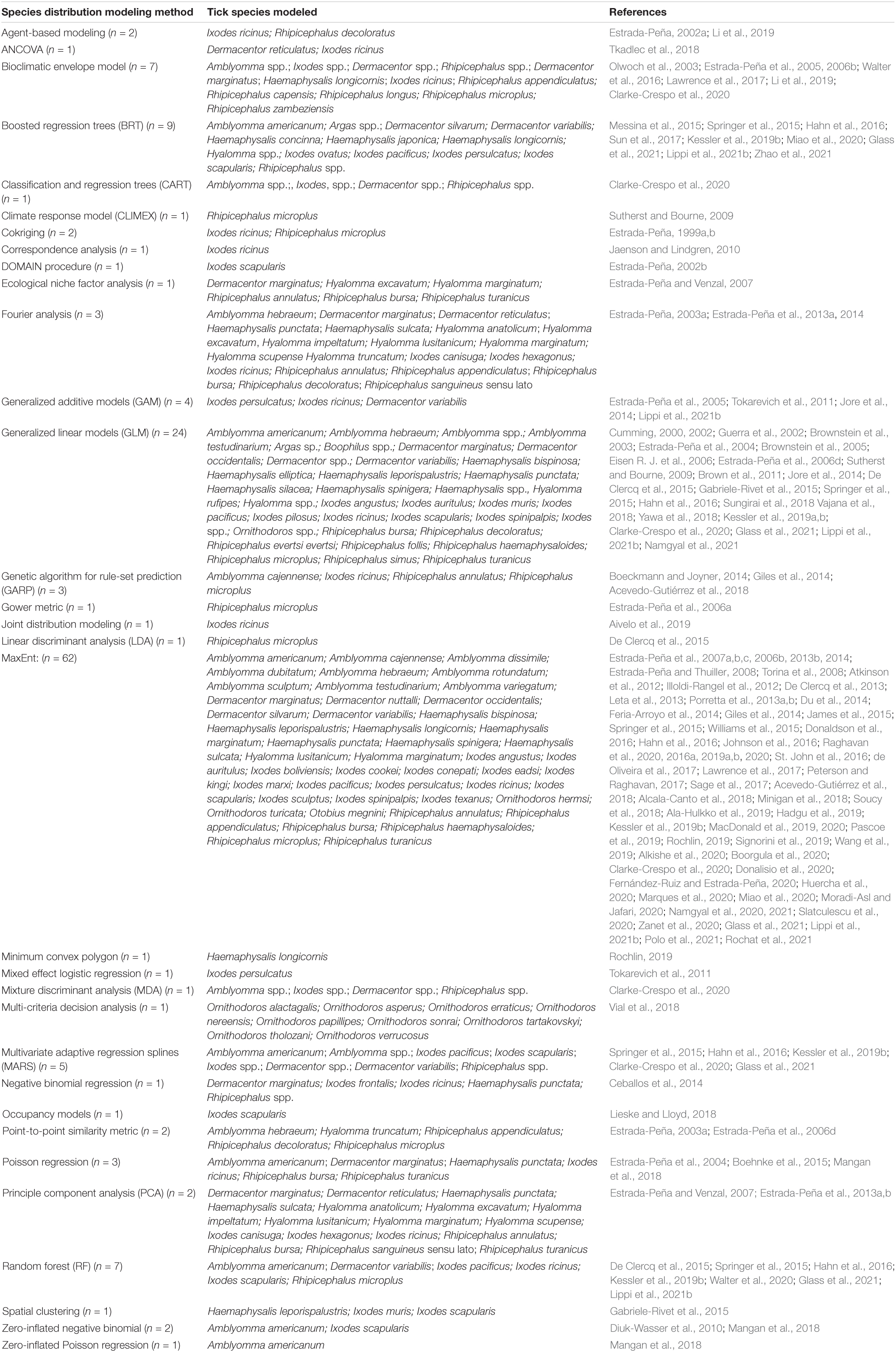
Table 2. Summary of species distribution modeling methods and associated tick species represented among studies that met scoping review inclusion criteria.
There were 22 different model validation methods reported across 99 articles (Figure 6 and Table 3). The most frequently employed evaluation strategies were receiver operating characteristic/area under the curve (ROC/AUC) (98 occurrences; 98.9% of articles), Akaike’s information criterion (41; 41.8%), k-fold cross validation (30; 30.6%), measures of sensitivity and specificity (30; 30.6%), one of several correlation coefficients (18; 18.2%), and kappa index of agreement (11; 11.1%) (Figure 6). The remaining validation methods were reported once or twice across all included literature. More than half of papers with validation methods reported (49; 60.6%) used two or more evaluation techniques for each model. Ten papers (roughly 9% of the total papers included in this review) either did not include a description of validation methods, or supplemental files with this information were not available.
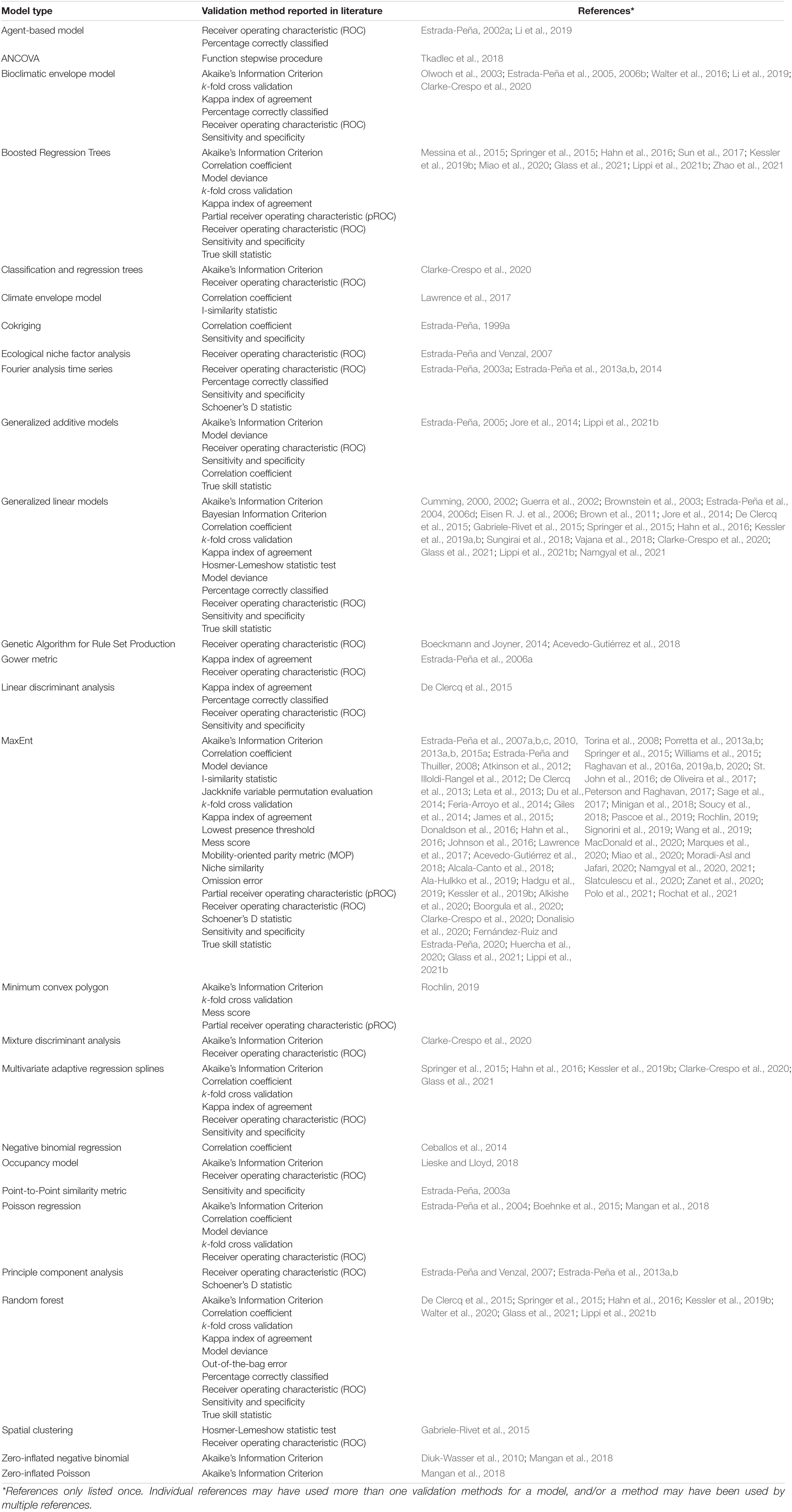
Table 3. Species distribution model validation methods reported across included literature according to respective modeling algorithms.
Future Climate Projections
There were 23 studies that included future climate projections, representing seven tick genera and 27 species (Table 4). Of these, only 65% of studies (15/23) used climate projections with the most used climate projection being MIROC (8; 53%), followed by CCSM (6; 40%) and NCAR (4; 26%). Note that multiple climate projection scenarios were used in a study, often as a comparison, with an average of approximately four climate projections used per study. In contrast, 86% of studies (20/23) used AB (5; 25%) or Representative Concentration Pathways (RCP, 15; 75%) emission scenarios. For RCP emissions scenarios, the most used scenario was 8.5 (14; 93%), followed closely by RCP 4.5 (13; 86%), while the most used Special Report on Emissions Scenarios (SRES) scenario was A2 (4; 80%). Eleven studies used environmental data such as NDVI, vegetation cover and elevation to parameterize their models and 14/23 used BIOCLIM data.
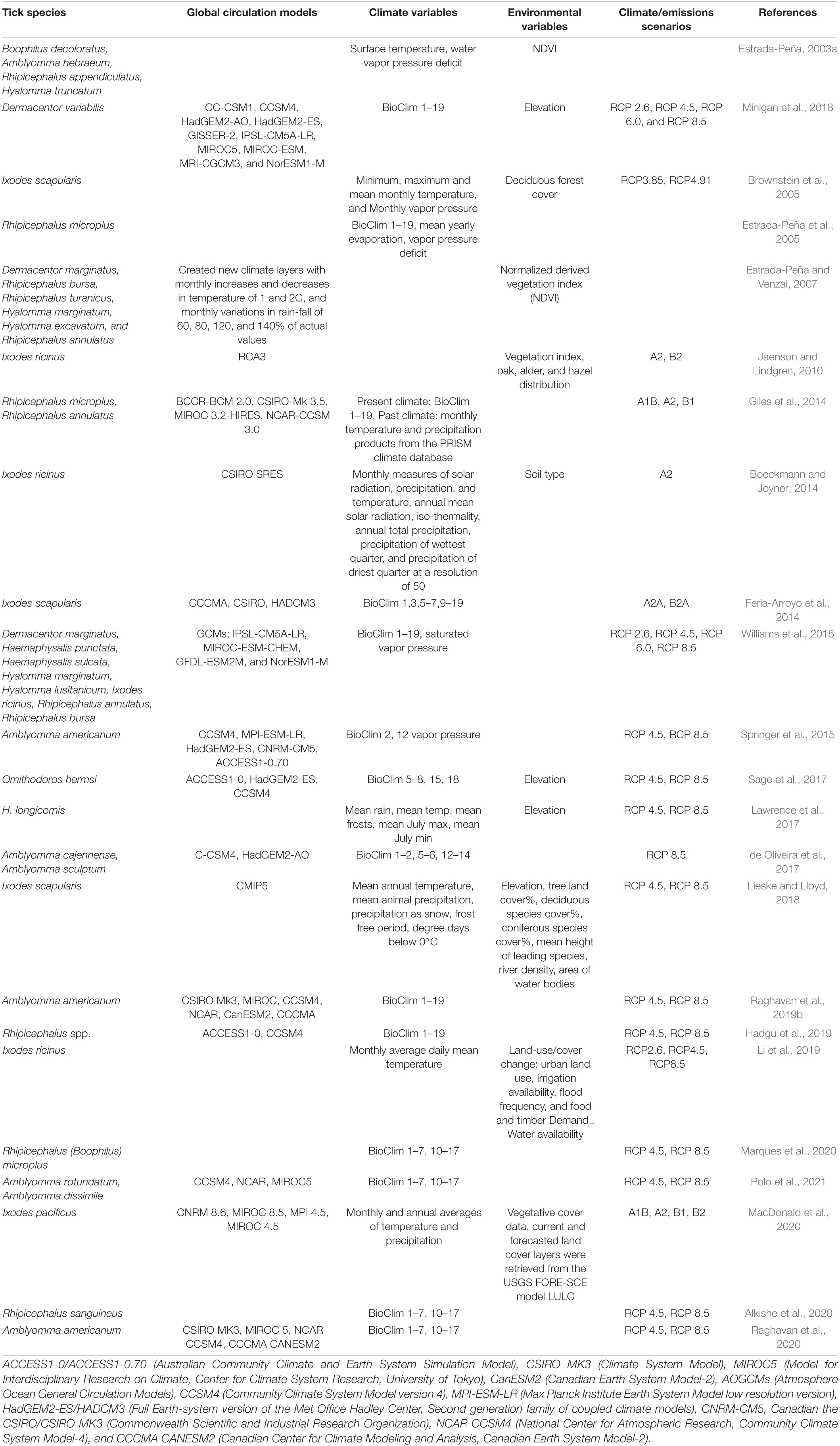
Table 4. Databases used for tick occurrence, climate projections, and environmental/host species variables.
For the seven tick genera, twenty studies used climate models to predict both global expansion and retraction of suitable habitat (Table 5). All genera (6/7) were expected to both expand and retract, except for Ornithodoros where only expansion was predicted. Of these studies, Rhipicephalus habitat suitability was predicted by seven studies (35%), with the range of Ixodes predicted by six studies (30%), followed by Amblyomma which was found in five studies (25%). In contrast, the habitat suitability, and future species distributions of Dermancentor and Ornithodoros were only forecasted by one study each.
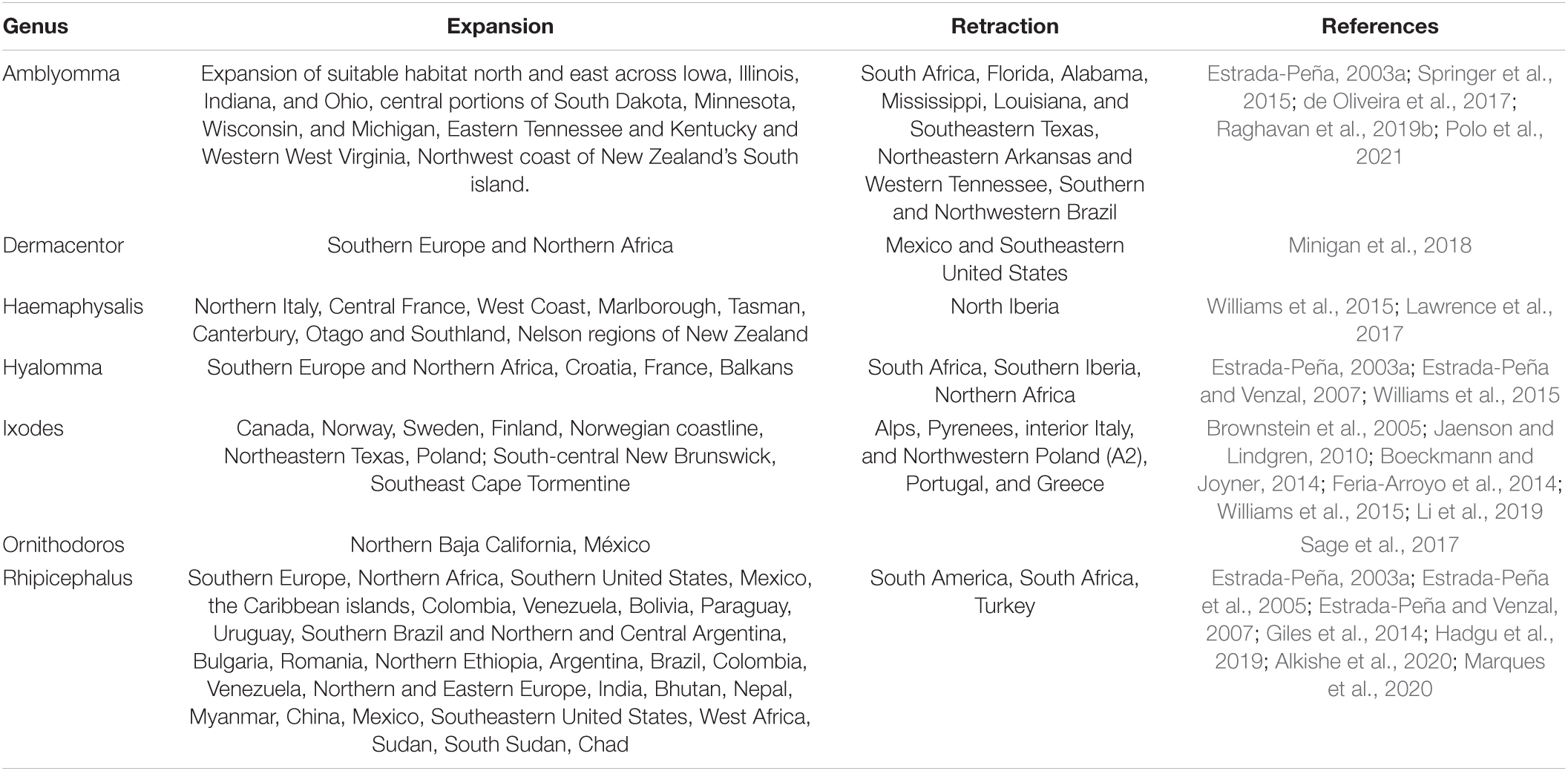
Table 5. Expected expansion and retraction of tick genera habitat based on future climate variables projections for various species.
Discussion
Our scoping review of tick vector species distribution modeling literature identified 107 papers that represent a wide array of tick occurrence record sources, environmental correlates, model validation strategies, and future climate-related scenarios for tick habitat suitability and distributions. However, we found that certain modeling strategies and data sources are more frequently used than others. MaxEnt, a machine-learning application, was the most used algorithm, appearing in 56% of papers, for predicting tick habitat suitability and distributions. This modeling approach is often favored because it only requires knowledge of species presence locations and generates pseudo-absences to complete the background locations to predict the distribution of a species (Phillips et al., 2006). It is reported to routinely perform better than other presence-only models (Elith et al., 2006; Merow et al., 2013) and is also highly accessible in both open-source R programming packages as well as its own software and is highly user-friendly (Phillips et al., 2006). A recent comparison of presence-absence model performance found little difference in the general performance (accuracy, discrimination, calibration, and precision) of most of these other model strategies, however, the authors caution that because poorly performing models can generate overfit predictions and overconfident estimations, it is important to work within sets of models first that are complementary within their assumptions before creating multi-model ensembles (Norberg et al., 2019). Overall, machine-learning approaches appear to be a popular choice for predicting tick distributions and habitat associations, though relatively few studies incorporated model ensembles despite the importance of controlling for individual model biases and ecological circumstances to reduce uncertainty (Marmion et al., 2009; Roura-Pascual et al., 2009; Qiao et al., 2015), or to simply highlighting model agreement and disagreement (Stohlgren et al., 2010).
Within this body of tick distribution modeling literature were dozens of different tick occurrence data sources that include both active and passive surveillance methods. Given the absence of standardized collection efforts and data recording and data availability across large spatial areas, many types and sources of occurrence records were combined without controlling for sampling biases. Tick occurrence data is inherently biased due to sampling regime and location uncertainty, and the inclusion of data sources must reflect this (Zizka et al., 2021). In addition, data collection methods vary widely across and within datasets, affecting both the completeness and the accuracy of reported data. Active surveillance for ticks can vary by collection method (dragging/flagging, small mammal trapping, CO2 trapping), by sampling intensity (time, distance/trap density), and by sampling based on habitat, (Rydzewski et al., 2011, 2012) leading to variation in the certainty of absence data (Lyons et al., 2021). This can result in models fitted to sampling effort and not true species distribution (Hendrickx et al., 2021). Passive surveillance varies by participation type and by the accuracy of data collected, from GPS location to self-reported, from tick submission to photo identification to self-report (Eisen and Eisen, 2021). A recent review of passive methods in mosquito surveillance found little coherence among programs, resulting in non-comparable data; similar issues likely exist in passive tick surveillance data (Sousa et al., 2022). Inclusion of these data sources without consideration of the potentially conflicting biases could lead to underestimation of model uncertainty (Kramer-Schadt et al., 2013). Particularly of importance with the most common modeling methods is certainty of location data. Positional uncertainty in data points reduces the prediction accuracy of the model (Naimi et al., 2014), but models may be built using historical databases and natural history collections with widely varying certainty due to changes in location methods (Gilliam et al., 2020), as well as misidentifications, changing taxonomical classifications, and unknown origin of the specimens (Graham et al., 2004). It should be noted, however, that these historical and museum-based records may include a substantial level of detail in the data collected, suggesting that they should not be ignored due to the potential complications in data collection; larger data sets can counteract the effect of positional uncertainty (Mitchell et al., 2017). The importance of positional uncertainty may also be mitigated by spatial autocorrelation among bioclimatic variables (Naimi et al., 2014), particularly if the range of the spatial autocorrelation is no more than three times the standard deviation of the positional error (Naimi et al., 2011). Positional uncertainty is also more important among species with narrow ranges than among widespread species (Soultan and Safi, 2017). For example, tick species (3-host) and life stages that parasitize hosts with small dispersal ranges (e.g., small rodents) require stricter thresholds and consideration of positional uncertainty error than those tick species (1-host) and life stages that rely on larger mammals that are capable of much broader dispersal. While difficult, it would be prudent for future tick SDMs to include metrics of host biology within model covariates. In addition, explanatory variables, such as landscape and climate, are frequently time-dependent; temporal alignment of observations and these time-dependent variables is essential to ensure model fitting based on circumstances at the time of observation and the climate niche (Estrada-Peña et al., 2013b). SDMs also differ in how they handle various sample sizes (Wisz et al., 2008) and proportions of true presence/absence data versus presence/pseudo-absences (Wisz and Guisan, 2009), therefore choosing the proper tools to handle these biases is crucial to reliable prediction outcomes. For example, MaxEnt (Phillips et al., 2017) is a prime option for handling data that contains known presence only, and would thus be a good choice for data structures that contain museum records. Sampling biases across a dataset can also be investigated prior to building models with software tools like “sampbias” which quantifies the biasing effect of human accessibility to data collection sites, and is available as a R package (Zizka et al., 2021). Similar to the variety of tick occurrence records were the numerous sources of bioclimatic data correlates. We reported 60 different databases for environmental parameters used across modeling papers, however, bioclimatic variables from WorldClim were the most frequently modeled. Since 1984, WorldClim has existed as a database of interpolated global climate data derived from weather stations using thin-plate splines, now including up to 35 different measures of temperature and precipitation/moisture (Hijmans et al., 2005). Variables 1–19, drawing from the years 1970–2000, are the most frequently used in modern SDM models (Booth, 2018), and a new high-resolution (1-km2) set of WorldClim monthly climate surfaces was released in 2017 (Fick and Hijmans, 2017). Many of these sources of environmental variables appear to be chosen due to ease of use and access, as well as due to the wide scale spatial coverage. For example, the WorldClim bioclimatic parameters are widely available via numerous spatial and targeted species distribution modeling R packages (e.g., SDM, krigR, envirem, etc.), and allow a user to apply them without having to upload and merge separate raster files (Naimi and Araújo, 2016; Title and Bemmels, 2018; Kusch and Davy, 2022). These data are also available in several resolutions and at a global scale, providing applicable climate resources regardless of the distribution locations chosen for model projections. Despite the common use and user-friendliness of these interpolated environmental variables to fit SDMs, it is important for modelers to recognize their limitations. Global bioclimatic datasets have not been validated on a smaller, local scale and could produce erroneous predictions in ecological niche modeling (Bedia et al., 2013). For this reason, it is important to also consider the use of satellite-derived remote sensing data for environmental parameters because they can provide more up-to-date and more precise measures of local climate and landscape (Amiri et al., 2020). Many of the bioclimatic variables also exhibit multicollinearity and spatial autocorrelation problems, and tests to exclude these biases from the models are inconsistently performed across the SDM literature (Araújo and Guisan, 2006; Estrada-Peña et al., 2013b).
We also tracked the types of species distribution model validation methods employed across the literature, with the understanding that there can be differences in how well validation applications can evaluate spatial models. The vast majority (98.9%) of papers used the receiver operating curve/area under the curve (ROC/AUC) metric to determine model fitness, and more than half of papers (60.6%) included two or more evaluation techniques. Nearly 10% of the body of literature reviewed did not report the validation method used to evaluate models, presenting challenges to the reproducibility of those modeling approaches. Similarly, the predominant use of WorldClim variables within this body of literature, there appears to be over-reliance on ROC/AUC to determine sensitivity and specificity of the model results (Lobo et al., 2008). Some researchers have cautioned that while ROC/AUC is helpful in that it is a threshold-independent measure and thereby more objective, it is a poor measure of model accuracy specifically in species distribution modeling. Lobo et al. (2008) report five issues with using ROC/AUC alone, including that ROC/AUC does not provide a spatial distribution of the model errors, and most critically, that the extent of the model can erroneously inflate the AUC score. Alternatives to basic ROC/AUC can include partial receiver operating curves (pROC), which avoids these criticisms and allows for differential weighting of omission and commission errors, as well as True Skill Statistic (TSS) (Lobo et al., 2008; Peterson et al., 2008). However, since many of the evaluation metrics can be biased by the proportions of occurrence presences to absences, strategies from other disciplines have also been suggested. Wunderlich et al. (2019) proposed the Odds Ratio Skill Score and the Symmetric Extremal Dependence Index (SEDI) to replace TSS in the evaluation of presence-background SDM methods, arguing that TSS can be biased depending on the number of true absences within a dataset. Overall, care must be taken in choosing evaluation methods that fit the type of species data and modeling algorithm.
The importance of model evaluation and validation methodology should not be understated as species distribution models are increasingly being used in the context of future climate-related projections to inform public policy on ticks and TBDs and improve priorities associated with One Health (Semenza et al., 2012). Approximately twenty percent of the studies included in this review included climate projection, of which fifty-six percent were published within the last 5 years. This showcases not only the increased interest in using SDM as a tool to understand the current distribution of tick species but also future possibilities to better control and mitigate TBDs. However, it is crucial that before integrating climate projections within SDM, there needs to be improved understanding of climate models and the inherent uncertainty within (Harris et al., 2014). Estrada-Peña (2003a) was the first to predict habitat suitability 15 years into the future for four tick species in South Africa using Fourier series analysis using decadal abiotic data from 1983 to 2000. More recently, researchers are using newer tools such as climate and emissions scenarios as well as Global Circulation Models (GCMs). When developing a SDM for projecting future species distributions, there are several considerations, including not only spatial resolutions and type of baseline data but also which environmental or climate variables to use. In addition, to avoid irrelevant or correlated climate variables there is need to account for phylogenetic data as species traits determine their distribution (Soberón, 2007; Morales-Castilla et al., 2017). Using an integrated approach that combines climate and phylogeny can clarify whether the underlying mechanism for differences in species richness or distribution shifts across space is more closely related to time and diversification rates (Kozak and Wiens, 2012; Wang et al., 2019).
The potential options allow for multiple combinations and corresponding divergence in climate estimates as the patterns of temperature and precipitation differ between GCMs (Guevara et al., 2019). To compensate for these differences, those studies in our review that used GCMs tend to use multiple models when evaluating future distributions. For example, Minigan et al. (2018), used ten GCMs when projecting the future distribution of Dermacentor variabilis in North America to incorporate uncertainty of possible future distributions and be able to showcase potential minimum and maximum range shifts (Harris et al., 2014). For those studies that used only one or two GCMs, the spatial extent of the projected area was limited, such as Lieske and Lloyd (2018) predicting I. scapularis in the province of New Brunswick or de Oliveira et al. (2017) predicting A. cajennense s.s. and A. sculptum in Brazil. Regardless of the number of GCMs incorporated within SDMs, it is important when drawing conclusions to consider that climate projections do not predict future climate, but instead provide possible futures under a given scenario (Rosentrater, 2010).
Emission and climate scenarios were included in most articles that included climate projections with less variation in which scenarios are included and compared. Most included a version of the Representative Concentration Pathways (RCPs) or the Intergovernmental Panel on Climate Change (IPCC) climate scenarios upon which the GCMs act to derive possible future climates. These climate and emission scenarios acted as possible futures with many including the recommended high and low emissions scenario to compare potential shifts in tick distributions under a changing climate. For example, at a simple level, Estrada-Peña (2003) found that increasing temperature by 2°C would potentially result in reduction of tick habitat in South Africa for A. hebraeum, B. decoloratus, H. truncatum, and R. appendiculatus. For more complex models using IPCC climate such as A2 (equivalent of RCP 8.5), a high emissions scenario that describes rapidly rising temperatures, there tends to be a loss of habitat range for I. ricinus in the southern range of its present distribution range in Poland and Italy yet expansion in Norway and Sweden (Boeckmann and Joyner, 2014). In contrast, in the southern hemisphere, in Brazil, for the RCP 8.5 emissions scenario, there is retraction of A. cajennense and A. sculptum in northern Brazil and expansion of suitable habitat in southern Brazil (de Oliveira et al., 2017). Alternative scenarios show similar expansion and retraction, however, the extent varies. For example, for low emission scenarios such as B1 (equivalent RCP 4.5) where there is a leveling of temperatures, there is increased loss of suitable habitat for Rhipicephalus sanguineus in South America as compared to the RCP 8.5 scenario (Alkishe et al., 2020). This suggests that moderate increases in temperature decreases habitat suitability without resulting in habitat changes elsewhere where tick species could find refuge. Overall, understanding how climate change could potentially influence tick distributions and range shifts will depend not only on the tick species, but also the region and climate model (Minigan et al., 2018).
We acknowledge several limitations to this review that also present opportunities for further research and investigation. Since we did not include gray literature or unpublished manuscripts, there are likely numerous applicable and appropriate analyses that are not represented in these data. The date cutoff for inclusion was August 2021, so there also are likely several relevant papers that have been released since that time that would have been included but are not. Within the assessment of validation methods, we did not include a question about training/testing dataset splitting, or in other words, how much of a dataset was used in prediction and forecasting. This is particularly important for ensuring reproducible projections of future climate-based tick habitat suitability. We also did not document whether researchers included location uncertainty thresholds to tick occurrence data. Some researchers recommend setting the limit of tick species observation position uncertainty to match that of the WorldClim data (i.e., 4,000 m) (Polo et al., 2021), and others (Pascoe et al., 2019) have set the limit to at a finer resolution (e.g., 1,000 m). Regardless, it is important for reproducibility of these analyses as well as accuracy of the model prediction for these thresholds to be set as well as reported in the literature. Researchers employing these modeling strategies for ticks or any species should consult checklists and guides on how to generate and report SDM studies in a reproducible manner (Araújo and Guisan, 2006; Austin and Van Niel, 2011; Feng et al., 2019).
Conclusion
Since the 1980s, species distribution modeling methods and data sources have greatly improved the ability to predict suitable tick habitat and likely distribution ranges. However, given the wide range of options that are used to estimate current and future tick habitat, it is critical to establish standardized methodologies for conducting, validating, and reporting species distribution model predictions. These parameters are necessary to create reproducible, comparable, and reliable guides to monitor tick vectors and their global risk to humans, domestic animals, and wildlife.
Author Contributions
RS and HK: conception. HK and SH: data collection and analysis. HK, SH, and RS: writing. RS: funding. SH: supervision. All authors contributed to the article and approved the submitted version.
Funding
This research was supported in part by a grant from the Department of Defense #TB180052.
Conflict of Interest
The authors declare that the research was conducted in the absence of any commercial or financial relationships that could be construed as a potential conflict of interest.
Publisher’s Note
All claims expressed in this article are solely those of the authors and do not necessarily represent those of their affiliated organizations, or those of the publisher, the editors and the reviewers. Any product that may be evaluated in this article, or claim that may be made by its manufacturer, is not guaranteed or endorsed by the publisher.
Acknowledgments
We are grateful for productive conversations, revisions, and suggestions from members of the Modeling for Disease Improvement Lab.
Supplementary Material
The Supplementary Material for this article can be found online at: https://www.frontiersin.org/articles/10.3389/fevo.2022.893016/full#supplementary-material
Footnotes
- ^ http://www.worldclim.org
- ^ https://clarivate.com/webofsciencegroup/solutions/web-of-science/
- ^ www.ncbi.nlm.nih.gov/pubmed
- ^ www.connectedpapers.com
- ^ http://www.osf.io/bd6qn
References
Acevedo-Gutiérrez, L. Y., Paternina, L. E., Londoño, A. F., Parra-Henao, G., and Rodas, J. D. (2018). Potential models of the geographic and climatic distribution of the Amblyomma cajennense complex (Acari: Ixodidae), a potential vector of Rickettsia rickettsii in Colombia. Biomedica 38, 534–544. doi: 10.7705/biomedica.v38i4.3916
Aivelo, T., Norberg, A., and Tschirren, B. (2019). Bacterial microbiota composition of Ixodes ricinus ticks: the role of environmental variation, tick characteristics and microbial interactions. PeerJ 7:e8217. doi: 10.7717/peerj.8217
Ala-Hulkko, T., Kotavaara, O., Alahuhta, J., Kesälä, M., and Hjort, J. (2019). Accessibility analysis in evaluating exposure risk to an ecosystem disservice. Appl. Geogr. 113:102098. doi: 10.1016/j.apgeog.2019.102098
Alcala-Canto, Y., Figueroa-Castillo, J. A., Ibarra-Velarde, F., Vera-Montenegro, Y., Cervantes-Valencia, M. E., Salem, A. Z. M., et al. (2018). Development of the first georeferenced map of Rhipicephalus (Boophilus) spp. in Mexico from 1970 to date and prediction of its spatial distribution. Geospat. Health 13, 110–117.
Alkishe, A., Cobos, M. E., Peterson, A. T., and Samy, A. M. (2020). Recognizing sources of uncertainty in disease vector ecological niche models: an example with the tick Rhipicephalus sanguineus sensu lato. Perspect. Ecol. Conserv. 18, 91–102. doi: 10.1016/j.pecon.2020.03.002
Amiri, M., Tarkesh, M., Jafari, R., and Jetschke, G. (2020). Bioclimatic variables from precipitation and temperature records vs. remote sensing-based bioclimatic variables: which side can perform better in species distribution modeling? Ecol. Inform. 57:101060. doi: 10.1016/j.ecoinf.2020.101060
Ammar, W., Groeneveld, D., Bhagavatula, C., Beltagy, I., Crawford, M., Downey, D., et al. (2018). “Construction of the literature graph in semantic scholar,” in Proceedings of the 2018 Conference of the North American Chapter of the Association for Computational Linguistics: Human Language Technologies, (Industry Papers), Vol. 3. (Stroudsburg, PA: Association for Computational Linguistics). doi: 10.18653/v1/n18-3011
Araújo, M. B., and Guisan, A. (2006). Five (or so) challenges for species distribution modelling. J. Biogeogr. 33, 1677–1688. doi: 10.1111/j.1365-2699.2006.01584.x
Arora, V. K., Scinocca, J. F., Boer, G. J., Christian, J. R., Denman, K. L., Flato, G. M., et al. (2011). Carbon emission limits required to satisfy future representative concentration pathways of greenhouse gases. Geophys. Res. Lett. 38:L05805. doi: 10.1029/2010gl046270
Atkinson, S. F., Sarkar, S., Aviña, A., Schuermann, J. A., and Williamson, P. (2012). Modelling spatial concordance between Rocky Mountain spotted fever disease incidence and habitat probability of its vector Dermacentor variabilis (American dog tick). Geospat. Health 7, 91–100. doi: 10.4081/gh.2012.108
Austin, M. P., and Van Niel, K. P. (2011). Improving species distribution models for climate change studies: variable selection and scale. J. Biogeogr. 38, 1–8. doi: 10.1111/j.1365-2699.2010.02416.x
Bedia, J., Herrera, S., and Gutiérrez, J. M. (2013). Dangers of using global bioclimatic datasets for ecological niche modeling. Limitations for future climate projections. Glob. Planet. Change 107, 1–12. doi: 10.1016/j.gloplacha.2013.04.005
Boeckmann, M., and Joyner, T. A. (2014). Old health risks in new places? An ecological niche model for I. ricinus tick distribution in Europe under a changing climate. Health Place 30, 70–77. doi: 10.1016/j.healthplace.2014.08.004
Boehnke, D., Brugger, K., Pfäffle, M., Sebastian, P., Norra, S., Petney, T., et al. (2015). Estimating Ixodes ricinus densities on the landscape scale. Int. J. Health Geogr. 14, 1–12. doi: 10.1186/s12942-015-0015-7
Boorgula, G. D. Y., Peterson, A. T., Foley, D. H., Ganta, R. R., and Raghavan, R. K. (2020). Assessing the current and future potential geographic distribution of the American dog tick, Dermacentor variabilis (Say) (Acari: Ixodidae) in North America. PLoS One 15:e0237191. doi: 10.1371/journal.pone.0237191
Booth, T. H. (2018). Why understanding the pioneering and continuing contributions of BIOCLIM to species distribution modelling is important. Aust. Ecol. 43, 852–860. doi: 10.1111/aec.12628
Brown, H. E., Yates, K. F., Dietrich, G., MacMillan, K., Graham, C. B., Reese, S. M., et al. (2011). An acarologic survey and Amblyomma americanum distribution map with implications for tularemia risk in Missouri. Am. J. Trop. Med. Hyg. 84, 411–419. doi: 10.4269/ajtmh.2011.10-0593
Brownstein, J. S., Holford, T. R., and Fish, D. (2003). A climate-based model predicts the spatial distribution of the Lyme disease vector Ixodes scapularis in the United States. Environ. Health Perspect. 111, 1152–1157. doi: 10.1289/ehp.6052
Brownstein, J. S., Holford, T. R., and Fish, D. (2005). Effect of climate change on Lyme disease risk in North America. Ecohealth 2, 38–46. doi: 10.1007/s10393-004-0139-x
Ceballos, L. A., Pintore, M. D., Tomassone, L., Pautasso, A., Bisanzio, D., Mignone, W., et al. (2014). Habitat and occurrence of ixodid ticks in the Liguria region, northwest Italy. Exp. Appl. Acarol. 64, 121–135. doi: 10.1007/s10493-014-9794-y
Clarke-Crespo, E., Moreno-Arzate, C. N., and López-González, C. A. (2020). Ecological niche models of four hard tick genera (Ixodidae) in Mexico. Animals 10:649. doi: 10.3390/ani10040649
Cumming, G. S. (2000). Using habitat models to map diversity: pan-African species richness of ticks (Acari: Ixodida). J. Biogeogr. 27, 425–440. doi: 10.1046/j.1365-2699.2000.00419.x
Cumming, G. S. (2002). Comparing climate and vegetation as limiting factors for species ranges of African ticks. Ecology 83, 255–268.
De Clercq, E. M., Estrada-Peña, A., Adehan, S., Madder, M., and Vanwambeke, S. O. (2013). An update on distribution models for Rhipicephalus microplus in West Africa. Geospat. Health 8, 301–308. doi: 10.4081/gh.2013.75
De Clercq, E. M., Leta, S., Estrada-Peña, A., Madder, M., Adehan, S., and Vanwambeke, S. O. (2015). Species distribution modelling for Rhipicephalus microplus (Acari: Ixodidae) in Benin, West Africa: comparing datasets and modelling algorithms. Prev. Vet. Med. 118, 8–21. doi: 10.1016/j.prevetmed.2014.10.015
de Oliveira, S. V., Romero-Alvarez, D., Martins, T. F., Santos, J. P. D., Labruna, M. B., Gazeta, G. S., et al. (2017). Amblyomma ticks and future climate: range contraction due to climate warming. Acta Trop. 176, 340–348. doi: 10.1016/j.actatropica.2017.07.033
Diuk-Wasser, M. A., Vourc’h, G., Cislo, P., Hoen, A. G., Melton, F., Hamer, S. A., et al. (2010). Field and climate-based model for predicting the density of host-seeking nymphal Ixodes scapularis, an important vector of tick-borne disease agents in the eastern United States. Glob. Ecol. Biogeogr. 19, 504–514. doi: 10.1111/j.1466-8238.2010.00526.x
Donaldson, T. G., Pèrez de León, A. A., Li, A. Y., Castro-Arellano, I., Wozniak, E., Boyle, W. K., et al. (2016). Correction: assessment of the geographic distribution of Ornithodoros turicata (Argasidae): climate variation and host diversity. PLoS Negl. Trop. Dis. 10:e0004538. doi: 10.1371/journal.pntd.0004538
Donalisio, M. R., Souza, C. E., Angerami, R. N., and Samy, A. M. (2020). Mapping Brazilian spotted fever: linking etiological agent, vectors, and hosts. Acta Trop. 207:105496. doi: 10.1016/j.actatropica.2020.105496
Du, Z., Wang, Z., Liu, Y., Wang, H., Xue, F., and Liu, Y. (2014). Ecological niche modeling for predicting the potential risk areas of severe fever with thrombocytopenia syndrome. Int. J. Infect. Dis. 26, 1–8. doi: 10.1016/j.ijid.2014.04.006
Eisen, L., and Eisen, R. J. (2021). Benefits and drawbacks of citizen science to complement traditional data gathering approaches for medically important hard ticks (Acari: Ixodidae) in the United States. J. Med. Entomol. 58, 1–9. doi: 10.1093/jme/tjaa165
Eisen, L., Eisen, R. J., and Lane, R. S. (2006). Geographical distribution patterns and habitat suitability models for presence of host-seeking ixodid ticks in dense woodlands of Mendocino County, California. J. Med. Entomol. 43, 415–427. doi: 10.1603/0022-2585(2006)043[0415:gdpahs]2.0.co;2
Eisen, R. J., Eisen, L., and Lane, R. S. (2006). Predicting density of Ixodes pacificus nymphs in dense woodlands in Mendocino County, California, based on geographic information systems and remote sensing versus field-derived data. Am. J. Trop. Med. Hyg. 74, 632–640.
Elith, J., Graham, C. H., Anderson, R. P., Dudík, M., Ferrier, S., Guisan, A., et al. (2006). Novel methods improve prediction of species’ distributions from occurrence data. Ecography 29, 129–151. doi: 10.1111/j.2006.0906-7590.04596.x
Escobar, L. E., Lira-Noriega, A., Medina-Vogel, G., and Townsend Peterson, A. (2014). Potential for spread of the white-nose fungus (Pseudogymnoascus destructans) in the Americas: use of Maxent and NicheA to assure strict model transference. Geospat. Health 9, 221–229. doi: 10.4081/gh.2014.19
Estrada-Peña, A. (1999a). Geostatistics and remote sensing using NOAA-AVHRR satellite imagery as predictive tools in tick distribution and habitat suitability estimations for Boophilus microplus (Acari: Ixodidae) in South America. Vet. Parasitol. 81, 73–82. doi: 10.1016/s0304-4017(98)00238-6
Estrada-Peña, A. (1999b). Geostatistics as predictive tools to estimate Ixodes ricinus (Acari: Ixodidae) habitat suitability in the western Palearctic from AVHRR satellite imagery. Exp. Appl. Acarol. 23, 337–349. doi: 10.1023/A:1006179318393
Estrada-Peña, A. (2002a). A simulation model for environmental population densities, survival rates and prevalence of Boophilus decoloratus (Acari: ixodidae) using remotely sensed environmental information. Vet. Parasitol. 104, 51–78. doi: 10.1016/s0304-4017(01)00607-0
Estrada-Peña, A. (2002b). Increasing habitat suitability in the United States for the tick that transmits Lyme disease: a remote sensing approach. Environ. Health Perspect. 110, 635–640. doi: 10.1289/ehp.110-1240908
Estrada-Peña, A. (2003a). Climate change decreases habitat suitability for some tick species (Acari: Ixodidae) in South Africa. Onderstepoort J. Vet. Res. 70, 79–93.
Estrada-Peña, A. (2003b). The relationships between habitat topology, critical scales of connectivity and tick abundance. Ixodes ricinusin a heterogeneous landscape in northern Spain. Ecography 26, 661–671. doi: 10.1034/j.1600-0587.2003.03530.x
Estrada-Peña, A. (2005). Effects of habitat suitability and landscape patterns on tick (Acarina) metapopulation processes. Landsc. Ecol. 20, 529–541. doi: 10.1007/s10980-004-3318-9
Estrada-Peña, A. (2006). “Prediction of habitat suitability for ticks,” in Century of Rickettsiology: Emerging, Reemerging Rickettsioses, Molecular Diagnostics, And Emerging Veterinary Rickettsioses Annals of the New York Academy of Sciences, eds K. E. Hechemy, J. A. Oteo, D. A. Raoult, D. J. Silverman, and J. R. Blanco (Logroño: Gobierno Rioja), 275–284. doi: 10.1196/annals.1374.052
Estrada-Peña, A., Acedo, C. S., Quílez, J., and Del Cacho, E. (2005). A retrospective study of climatic suitability for the tick Rhipicephalus (Boophilus) microplus in the Americas. Glob. Ecol. Biogeogr. 14, 565–573.
Estrada-Peña, A., Alexander, N., and Wint, G. R. W. (2016). Perspectives on modelling the distribution of ticks for large areas: so far so good? Parasit. Vectors 9:179. doi: 10.1186/s13071-016-1474-9
Estrada-Peña, A., and Thuiller, W. (2008). An assessment of the effect of data partitioning on the performance of modeling algorithms for habitat suitability for ticks. Med. Vet. Entomol. 22, 248–257. doi: 10.1111/j.1365-2915.2008.00745.x
Estrada-Peña, A., and Venzal, J. M. (2007). Climate niches of tick species in the Mediterranean region: modeling of occurrence data, distributional constraints, and impact of climate change. J. Med. Entomol. 44, 1130–1138. doi: 10.1603/0022-2585(2007)44[1130:cnotsi]2.0.co;2
Estrada-Peña, A., Corson, M., Venzal, J. M., Mangold, A. J., and Guglielmone, A. (2006a). Changes in climate and habitat suitability for the cattle tick Boophilus microplus in its southern Neotropical distribution range. J. Vector Ecol. 31, 158–167. doi: 10.3376/1081-1710(2006)31[158:cicahs]2.0.co;2
Estrada-Peña, A., Venzal, J. M., and Sánchez Acedo, C. (2006b). The tick Ixodes ricinus: distribution and climate preferences in the western Palaearctic. Med. Vet. Entomol. 20, 189–197. doi: 10.1111/j.1365-2915.2006.00622.x
Estrada-Peña, A., Bouattour, A., Camicas, J.-L., Guglielmone, A., Horak, I., Jongejan, F., et al. (2006c). The known distribution and ecological preferences of the tick subgenus Boophilus (Acari: Ixodidae) in Africa and Latin America. Exp. Appl. Acarol. 38, 219–235. doi: 10.1007/s10493-006-0003-5
Estrada-Peña, A. García, Z.Sánchez, H. F. (2006d). The distribution and ecological preferences of Boophilus microplus (Acari: Ixodidae) in Mexico. Exp. Appl. Acarol. 38, 307–316. doi: 10.1007/s10493-006-7251-2
Estrada-Peña, A., Estrada-Sánchez, A., and Estrada-Sánchez, D. (2015a). Methodological caveats in the environmental modelling and projections of climate niche for ticks, with examples for Ixodes ricinus (Ixodidae). Vet. Parasitol. 208, 14–25. doi: 10.1016/j.vetpar.2014.12.016
Estrada-Peña, A., de la Fuente, J., Latapia, T., and Ortega, C. (2015b). The impact of climate trends on a tick affecting public health: a retrospective modeling approach for Hyalomma marginatum (ixodidae). PLoS One 10:e0125760. doi: 10.1371/journal.pone.0125760
Estrada-Peña, A., Estrada-Sánchez, A., and de la Fuente, J. (2014). A global set of Fourier-transformed remotely sensed covariates for the description of abiotic niche in epidemiological studies of tick vector species. Parasit. Vectors 7:302. doi: 10.1186/1756-3305-7-302
Estrada-Peña, A., Farkas, R., Jaenson, T. G. T., Koenen, F., Madder, M., Pascucci, I., et al. (2013a). Association of environmental traits with the geographic ranges of ticks (Acari: Ixodidae) of medical and veterinary importance in the western Palearctic. A digital data set. Exp. Appl. Acarol. 59, 351–366. doi: 10.1007/s10493-012-9600-7
Estrada-Peña, A., Estrada-Sánchez, A., Estrada-Sánchez, D., and de la Fuente, J. (2013b). Assessing the effects of variables and background selection on the capture of the tick climate niche. Int. J. Health Geogr. 12:43. doi: 10.1186/1476-072X-12-43
Estrada-Peña, A., Pegram, R. G., Barré, N., and Venzal, J. M. (2007a). Using invaded range data to model the climate suitability for Amblyomma variegatum (Acari: Ixodidae) in the New World. Exp. Appl. Acarol. 41, 203–214. doi: 10.1007/s10493-007-9050-9
Estrada-Peña, A., Horak, I. G., and Petney, T. (2007b). Climate changes and suitability for the ticks Amblyomma hebraeum and Amblyomma variegatum (Ixodidae) in Zimbabwe (1974-1999). Vet. Parasitol. 151, 256–267. doi: 10.1016/j.vetpar.2007.11.014
Estrada-Peña, A., Zatansever, Z., Gargili, A., Aktas, M., Uzun, R., Ergonul, O., et al. (2007c). Modeling the spatial distribution of Crimean-Congo Hemorrhagic Fever outbreaks in Turkey. Vector Borne Zoonot. Dis. 7, 667–678. doi: 10.1089/vbz.2007.0134
Estrada-Peña, A., Nava, S., Horak, I. G., and Guglielmone, A. A. (2010). Using ground-derived data to assess the environmental niche of the spinose ear tick, Otobius megnini. Entomol. Exp. Appl. 137, 132–142. doi: 10.1111/j.1570-7458.2010.01050.x
Estrada-Peña, A., Quíez, J., and Sánchez Acedo, C. (2004). Species composition, distribution, and ecological preferences of the ticks of grazing sheep in north-central Spain. Med. Vet. Entomol. 18, 123–133. doi: 10.1111/j.0269-283X.2004.00486.x
Falagas, M. E., Pitsouni, E. I., Malietzis, G. A., and Pappas, G. (2008). Comparison of PubMed, Scopus, Web of Science, and Google Scholar: strengths and weaknesses. FASEB J. 22, 338–342. doi: 10.1096/fj.07-9492LSF
Feng, X., Park, D. S., Walker, C., Peterson, A. T., Merow, C., and Papeş, M. (2019). A checklist for maximizing reproducibility of ecological niche models. Nat. Ecol. Evol. 3, 1382–1395. doi: 10.1038/s41559-019-0972-5
Feria-Arroyo, T. P., Castro-Arellano, I., Gordillo-Perez, G., Cavazos, A. L., Vargas-Sandoval, M., Grover, A., et al. (2014). Implications of climate change on the distribution of the tick vector Ixodes scapularis and risk for Lyme disease in the Texas-Mexico transboundary region. Parasit. Vectors 7:199. doi: 10.1186/1756-3305-7-199
Fernández-Ruiz, N., and Estrada-Peña, A. (2020). Could climate trends disrupt the contact rates between Ixodes ricinus (Acari, Ixodidae) and the reservoirs of Borrelia burgdorferi s.l.? PLoS One 15:e0233771. doi: 10.1371/journal.pone.0233771
Fick, S. E., and Hijmans, R. J. (2017). WorldClim 2: new 1-km spatial resolution climate surfaces for global land areas: new climate surfaces for global land areas. Int. J. Climatol. 37, 4302–4315. doi: 10.1002/joc.5086
Franklin, J. (2010). Mapping Species Distributions: Spatial Inference and Prediction. Cambridge: Cambridge University Press.
Gabriele-Rivet, V., Arsenault, J., Badcock, J., Cheng, A., Edsall, J., Goltz, J., et al. (2015). Different ecological niches for ticks of public health significance in Canada. PLoS One 10:e0131282. doi: 10.1371/journal.pone.0131282
Giles, J. R., Peterson, A. T., Busch, J., Olafson, P. U., Scoles, G. A., Davey, R. B., et al. (2014). Invasive potential of cattle fever ticks in the southern United States. Parasit. Vectors 7:189. doi: 10.1186/1756-3305-7-189
Gilliam, B., Gronemeyer, P., Chakraborty, S., Winata, F., Lyons, L. A., Miller-Hunt, C., et al. (2020). Impact of unexplored data sources on the historical distribution of three vector tick species in Illinois. J. Med. Entomol. 57, 872–883. doi: 10.1093/jme/tjz235
Glass, G. E., Ganser, C., and Kessler, W. H. (2021). Validating species distribution models with standardized surveys for ixodid ticks in Mainland Florida. J. Med. Entomol. 58, 1345–1351. doi: 10.1093/jme/tjaa282
Graham, C. H., Ferrier, S., Huettman, F., Moritz, C., and Peterson, A. T. (2004). New developments in museum-based informatics and applications in biodiversity analysis. Trends Ecol. Evol. 19, 497–503. doi: 10.1016/j.tree.2004.07.006
Guerra, M., Walker, E., Jones, C., Paskewitz, S., Cortinas, M. R., Stancil, A., et al. (2002). Predicting the risk of Lyme disease: habitat suitability for Ixodes scapularis in the north central United States. Emerg. Infect. Dis. 8, 289–297. doi: 10.3201/eid0803.010166
Guevara, L., Morrone, J. J., and León-Paniagua, L. (2019). Spatial variability in species’ potential distributions during the Last Glacial Maximum under different global circulation models: relevance in evolutionary biology. J. Zool. Syst. Evol. Res. 57, 113–126. doi: 10.1111/jzs.12238
Guisan, A., and Zimmermann, N. E. (2000). Predictive habitat distribution models in ecology. Ecol. Model. 135, 147–186. doi: 10.1016/S0304-3800(00)00354-9
Hadgu, M., Menghistu, H. T., Girma, A., Abrha, H., and Hagos, H. (2019). Modeling the potential climate change- induced impacts on future genus Rhipicephalus (Acari: Ixodidae) tick distribution in semi-arid areas of Raya Azebo district, Northern Ethiopia. Hangug Hwangyeong Saengtae Haghoeji 43:43. doi: 10.1186/s41610-019-0137-0
Hahn, M. B., Jarnevich, C. S., Monaghan, A. J., and Eisen, R. J. (2016). Modeling the geographic distribution of Ixodes scapularis and Ixodes pacificus (Acari: Ixodidae) in the contiguous United States. J. Med. Entomol. 53, 1176–1191. doi: 10.1093/jme/tjw076
Harris, R. M. B., Grose, M. R., Lee, G., Bindoff, N. L., Porfirio, L. L., and Fox-Hughes, P. (2014). Climate projections for ecologists. Wiley Interdiscip. Rev. Clim. Change 5, 621–637. doi: 10.1002/wcc.291
Hendrickx, A., Marsboom, C., Rinaldi, L., Vineer, H. R., Morgoglione, M. E., Sotiraki, S., et al. (2021). Constraints of using historical data for modelling the spatial distribution of helminth parasites in ruminants. Parasite 28:46. doi: 10.1051/parasite/2021042
Hijmans, R. J., Cameron, S. E., Parra, J. L., Jones, P. G., and Jarvis, A. (2005). Very high resolution interpolated climate surfaces for global land areas. Int. J. Climatol. 25, 1965–1978. doi: 10.1002/joc.1276
Holman, I., Audsley, E., Berry, P., Brown, C., Bugmann, H., Clarke, L., et al. (2015). Specification for European Model Improvement and Development. IMPRESSIONS Deliverable D3B.1. Prepared under Contract from the European Commission by the Natural Environment Research Council Centre for Ecology and Hydrology.
Huercha, H., Song, R., Ma, Y., Hu, Z., Li, Y., Li, M., et al. (2020). MaxEnt modeling of Dermacentor marginatus (Acari: Ixodidae) distribution in Xinjiang, China. J. Med. Entomol. 57, 1659–1667. doi: 10.1093/jme/tjaa063
Hutchinson, M. F., Nix, H. A., MacMahon, J. P., and Ord, K. D. (1996). A Topographic and Climatic Database for Africa— Version 1.1. Canberra, ACT: Australian National University.
Illoldi-Rangel, P., Rivaldi, C.-L., Sissel, B., Trout Fryxell, R., Gordillo-Pérez, G., Rodríguez-Moreno, A., et al. (2012). Species distribution models and ecological suitability analysis for potential tick vectors of Lyme disease in Mexico. J. Trop. Med. 2012:959101. doi: 10.1155/2012/959101
Jaenson, T. G. T., and Lindgren, E. (2010). The range of Ixodes ricinus and the risk of contracting Lyme borreliosis will increase northwards when the vegetation period becomes longer. Ticks Tick Borne Dis. 2, 44–49. doi: 10.1016/j.ttbdis.2010.10.006
James, A. M., Burdett, C., McCool, M. J., Fox, A., and Riggs, P. (2015). The geographic distribution and ecological preferences of the American dog tick, Dermacentor variabilis (Say), in the U.S.A. Med. Vet. Entomol. 29, 178–188. doi: 10.1111/mve.12099
Johnson, T. L., Bjork, J. K. H., Neitzel, D. F., Dorr, F. M., Schiffman, E. K., and Eisen, R. J. (2016). Habitat suitability model for the distribution of Ixodes scapularis (Acari: Ixodidae) in Minnesota. J. Med. Entomol. 53, 598–606. doi: 10.1093/jme/tjw008
Jongejan, F., and Uilenberg, G. (2004). The global importance of ticks. Parasitology 129(Suppl.), S3–S14. doi: 10.1017/s0031182004005967
Jore, S., Vanwambeke, S. O., Viljugrein, H., Isaksen, K., Kristoffersen, A. B., Woldehiwet, Z., et al. (2014). Climate and environmental change drives Ixodes ricinus geographical expansion at the northern range margin. Parasit. Vectors 7:11. doi: 10.1186/1756-3305-7-11
Jubb, I., Canadell, P., and Dix, M. (2013). Representative Concentration Pathways (RCPs). Australian Government, Department of the Environment. Available online at: https://www.cawcr.gov.au/projects/Climatechange/wp-content/uploads/2016/11/ACCSP_RCP.pdf (accessed February 6, 2022).
Kessler, W. H., Blackburn, J. K., Sayler, K. A., and Glass, G. E. (2019a). Estimating the geographic distribution of host-seeking adult Amblyomma americanum (Acari: Ixodidae) in Florida. J. Med. Entomol. 56, 55–64. doi: 10.1093/jme/tjy147
Kessler, W. H., Ganser, C., and Glass, G. E. (2019b). Modeling the distribution of medically important tick species in Florida. Insects 10:190. doi: 10.3390/insects10070190
Kozak, K. H., and Wiens, J. J. (2012). Phylogeny, ecology, and the origins of climate–richness relationships. Ecology 93, S167–S181. doi: 10.1890/11-0542.1
Kramer-Schadt, S., Niedballa, J., Pilgrim, J. D., Schröder, B., Lindenborn, J., Reinfelder, V., et al. (2013). The importance of correcting for sampling bias in MaxEnt species distribution models. Divers. Distrib. 19, 1366–1379. doi: 10.1111/ddi.12096
Kusch, E., and Davy, R. (2022). KrigR—a tool for downloading and statistically downscaling climate reanalysis data. Environ. Res. Lett. 17:024005. doi: 10.1088/1748-9326/ac48b3
Lawrence, K. E., Summers, S. R., Heath, A. C. G., McFadden, A. M. J., Pulford, D. J., Tait, A. B., et al. (2017). Using a rule-based envelope model to predict the expansion of habitat suitability within New Zealand for the tick Haemaphysalis longicornis, with future projections based on two climate change scenarios. Vet. Parasitol. 243, 226–234. doi: 10.1016/j.vetpar.2017.07.001
Leta, S., De Clercq, E. M., and Madder, M. (2013). High-resolution predictive mapping for Rhipicephalus appendiculatus (Acari: Ixodidae) in the Horn of Africa. Exp. Appl. Acarol. 60, 531–542. doi: 10.1007/s10493-013-9670-1
Li, S., Gilbert, L., Vanwambeke, S. O., Yu, J., Purse, B. V., and Harrison, P. A. (2019). Lyme disease risks in Europe under multiple uncertain drivers of change. Environ. Health Perspect. 127:67010. doi: 10.1289/EHP4615
Lieske, D. J., and Lloyd, V. K. (2018). Combining public participatory surveillance and occupancy modelling to predict the distributional response of Ixodes scapularis to climate change. Ticks Tick Borne Dis. 9, 695–706. doi: 10.1016/j.ttbdis.2018.01.018
Lippi, C. A., Gaff, H. D., White, A. L., and Ryan, S. J. (2021a). Scoping review of distribution models for selected Amblyomma ticks and rickettsial group pathogens. PeerJ 9:e10596. doi: 10.7717/peerj.10596
Lippi, C. A., Gaff, H. D., White, A. L., St John, H. K., Richards, A. L., and Ryan, S. J. (2021b). Exploring the niche of Rickettsia montanensis (Rickettsiales: Rickettsiaceae) infection of the American dog tick (Acari: Ixodidae), using multiple species distribution model approaches. J. Med. Entomol. 58, 1083–1092. doi: 10.1093/jme/tjaa263
Lobo, J. M., Jiménez-Valverde, A., and Real, R. (2008). AUC: a misleading measure of the performance of predictive distribution models. Glob. Ecol. Biogeogr. 17, 145–151. doi: 10.1111/j.1466-8238.2007.00358.x
Lyons, L. A., Brand, M. E., Gronemeyer, P., Mateus-Pinilla, N., Ruiz, M. O., Stone, C. M., et al. (2021). Comparing contributions of passive and active tick collection methods to determine establishment of ticks of public health concern within Illinois. J. Med. Entomol. 58, 1849–1864. doi: 10.1093/jme/tjab031
MacDonald, A. J., McComb, S., O’Neill, C., Padgett, K. A., and Larsen, A. E. (2020). Projected climate and land use change alter western blacklegged tick phenology, seasonal host-seeking suitability and human encounter risk in California. Glob. Change Biol. 26, 5459–5474. doi: 10.1111/gcb.15269
MacDonald, A. J., O’Neill, C., Yoshimizu, M. H., Padgett, K. A., and Larsen, A. E. (2019). Tracking seasonal activity of the western blacklegged tick across California. J. Appl. Ecol. 56, 2562–2573. doi: 10.1111/1365-2664.13490
Mader, E. M., Ganser, C., Geiger, A., Harrington, L. C., Foley, J., Smith, R. L., et al. (2021). A survey of tick surveillance and control practices in the United States. J. Med. Entomol. 58, 1503–1512. doi: 10.1093/jme/tjaa094
Mangan, M. J., Foré, S. A., and Kim, H.-J. (2018). Ecological modeling over seven years to describe the number of host-seeking Amblyomma americanum in each life stage in northeast Missouri. J. Vector Ecol. 43, 271–284. doi: 10.1111/jvec.12311
Marmion, M., Parviainen, M., Luoto, M., Heikkinen, R. K., and Thuiller, W. (2009). Evaluation of consensus methods in predictive species distribution modelling. Divers. Distrib. 15, 59–69. doi: 10.1111/j.1472-4642.2008.00491.x
Marques, R., Krüger, R. F., Peterson, A. T., de Melo, L. F., Vicenzi, N., and Jiménez-García, D. (2020). Climate change implications for the distribution of the babesiosis and anaplasmosis tick vector, Rhipicephalus (Boophilus) microplus. Vet. Res. 51:81. doi: 10.1186/s13567-020-00802-z
Merow, C., Smith, M. J., and Silander, J. A. (2013). A practical guide to MaxEnt for modeling species’ distributions: what it does, and why inputs and settings matter. Ecography 36, 1058–1069. doi: 10.1111/j.1600-0587.2013.07872.x
Messina, J. P., Pigott, D. M., Golding, N., Duda, K. A., Brownstein, J. S., Weiss, D. J., et al. (2015). The global distribution of Crimean-Congo hemorrhagic fever. Trans. R. Soc. Trop. Med. Hyg. 109, 503–513. doi: 10.1093/trstmh/trv050
Miao, D., Dai, K., Zhao, G.-P., Li, X.-L., Shi, W.-Q., Zhang, J. S., et al. (2020). Mapping the global potential transmission hotspots for severe fever with thrombocytopenia syndrome by machine learning methods. Emerg. Microbes Infect. 9, 817–826. doi: 10.1080/22221751.2020.1748521
Minigan, J. N., Hager, H. A., Peregrine, A. S., and Newman, J. A. (2018). Current and potential future distribution of the American dog tick (Dermacentor variabilis, Say) in North America. Ticks Tick Borne Dis. 9, 354–362. doi: 10.1016/j.ttbdis.2017.11.012
Mitchell, P. J., Monk, J., and Laurenson, L. (2017). Sensitivity of fine-scale species distribution models to locational uncertainty in occurrence data across multiple sample sizes. Methods Ecol. Evol. 8, 12–21. doi: 10.1111/2041-210x.12645
Moradi-Asl, E., and Jafari, S. (2020). The habitat suitability model for the potential distribution of Ornithodoros tholozani (Laboulbène et Mégnin, 1882) and Ornithodoros lahorensis (Neumann, 1908) (Acari: Argasidae): the main vectors of tick-borne relapsing fever in Iran. Ann. Parasitol. 66, 357–363. doi: 10.17420/ap6603.274
Morales-Castilla, I., Davies, T. J., Pearse, W. D., and Peres-Neto, P. (2017). Combining phylogeny and co-occurrence to improve single species distribution models. Glob. Ecol. Biogeogr. 26, 740–752. doi: 10.1111/geb.12580
Naimi, B., and Araújo, M. B. (2016). sdm: a reproducible and extensible R platform for species distribution modelling. Ecography 39, 368–375. doi: 10.1111/ecog.01881
Naimi, B., Hamm, N. A. S., Groen, T. A., Skidmore, A. K., and Toxopeus, A. G. (2014). Where is positional uncertainty a problem for species distribution modelling? Ecography 37, 191–203. doi: 10.1111/j.1600-0587.2013.00205.x
Naimi, B., Skidmore, A. K., Groen, T. A., and Hamm, N. A. S. (2011). Spatial autocorrelation in predictors reduces the impact of positional uncertainty in occurrence data on species distribution modelling. J. Biogeogr. 38, 1497–1509. doi: 10.1111/j.1365-2699.2011.02523.x
Namgyal, J., Couloigner, I., Lysyk, T. J., Dergousoff, S. J., and Cork, S. C. (2020). Comparison of habitat suitability models for Haemaphysalis longicornis Neumann in North America to determine its potential geographic range. Int. J. Environ. Res. Public Health 17:8285. doi: 10.3390/ijerph17218285
Namgyal, J., Lysyk, T. J., Couloigner, I., Checkley, S., Gurung, R. B., Tenzin, T., et al. (2021). Identification, distribution, and habitat suitability models of ixodid tick species in cattle in Eastern Bhutan. Trop. Med. Infect. Dis. 6:27. doi: 10.3390/tropicalmed6010027
Norberg, A., Abrego, N., Blanchet, F. G., Adler, F. R., Anderson, B. J., Anttila, J., et al. (2019). A comprehensive evaluation of predictive performance of 33 species distribution models at species and community levels. Ecol. Monogr. 89:e01370. doi: 10.1002/ecm.1370
Olwoch, J. M., Rautenbach, C. J., de, W., Erasmus, B. F. N., Engelbrecht, F. A., and Van Jaarsveld, A. S. (2003). Simulating tick distributions over sub-Saharan Africa: the use of observed and simulated climate surfaces. J. Biogeogr. 30, 1221–1232. doi: 10.1046/j.1365-2699.2003.00913.x
Pascoe, E. L., Marcantonio, M., Caminade, C., and Foley, J. E. (2019). Modeling potential habitat for Amblyomma tick species in California. Insects 10:201. doi: 10.3390/insects10070201
Peterson, A. T., Papeş, M., and Soberón, J. (2008). Rethinking receiver operating characteristic analysis applications in ecological niche modeling. Ecol. Model. 213, 63–72. doi: 10.1016/j.ecolmodel.2007.11.008
Peterson, A. T., and Raghavan, R. K. (2017). The geographic distribution of Ixodes scapularis (Acari: Ixodidae) revisited: the importance of assumptions about error balance. J. Med. Entomol. 54, 1080–1084. doi: 10.1093/jme/tjx095
Peterson, A. T., and Soberón, J. (2012). Species distribution modeling and ecological niche modeling: getting the concepts right. Nat. Conserv. 10, 102–107. doi: 10.4322/natcon.2012.019
Peterson, A. T., Soberón, J., Pearson, R. G., Anderson, R. P., Martínez-Meyer, E., Nakamura, M., et al. (2011). Ecological Niches and Geographic Distributions (MPB-49). Princeton, NJ: Princeton University Press.
Phillips, S. B., Aneja, V. P., Kang, D., and Arya, S. P. (2006). Modelling and analysis of the atmospheric nitrogen deposition in North Carolina. Int. J. Glob. Environ. Issues 190, 231–259. doi: 10.1016/j.ecolmodel.2005.03.026
Phillips, S. J., Anderson, R. P., Dudík, M., Schapire, R. E., and Blair, M. E. (2017). Opening the black box: an open-source release of Maxent. Ecography 40, 887–893. doi: 10.1111/ecog.03049
Phillips, S. J., Dudík, M., Elith, J., Graham, C. H., Lehmann, A., Leathwick, J., et al. (2009). Sample selection bias and presence-only distribution models: implications for background and pseudo-absence data. Ecol. Appl. 19, 181–197. doi: 10.1890/07-2153.1
Polo, G., Luz, H. R., Regolin, A. L., Martins, T. F., Winck, G. R., da Silva, H. R., et al. (2021). Distribution modeling of Amblyomma rotundatum and Amblyomma dissimile in Brazil: estimates of environmental suitability. Parasitol. Res. 120, 797–806. doi: 10.1007/s00436-020-06924-9
Porretta, D., Mastrantonio, V., Amendolia, S., Gaiarsa, S., Epis, S., Genchi, C., et al. (2013a). Effects of global changes on the climatic niche of the tick Ixodes ricinus inferred by species distribution modelling. Parasit. Vectors 6:271. doi: 10.1186/1756-3305-6-271
Porretta, D., Mastrantonio, V., Mona, S., Epis, S., Montagna, M., Sassera, D., et al. (2013b). The integration of multiple independent data reveals an unusual response to Pleistocene climatic changes in the hard tick Ixodes ricinus. Mol. Ecol. 22, 1666–1682. doi: 10.1111/mec.12203
Qiao, H., Soberón, J., and Peterson, A. T. (2015). No silver bullets in correlative ecological niche modelling: insights from testing among many potential algorithms for niche estimation. Methods Ecol. Evol. 6, 1126–1136. doi: 10.1111/2041-210x.12397
Raghavan, R. K., Barker, S. C., Cobos, M. E., Barker, D., Teo, E. J. M., Foley, D. H., et al. (2019a). Potential spatial distribution of the newly introduced long-horned tick, Haemaphysalis longicornis in North America. Sci. Rep. 9:498. doi: 10.1038/s41598-018-37205-2
Raghavan, R. K., Peterson, A. T., Cobos, M. E., Ganta, R., and Foley, D. (2019b). Current and future distribution of the lone star tick, Amblyomma americanum (L.) (Acari: Ixodidae) in North America. PLoS One 14:e0209082. doi: 10.1371/journal.pone.0209082
Raghavan, R. K., Goodin, D. G., Hanzlicek, G. A., Zolnerowich, G., Dryden, M. W., Anderson, G. A., et al. (2016a). Maximum entropy-based ecological niche model and bio-climatic determinants of lone star tick (Amblyomma americanum) niche. Vector Borne Zoonot. Dis. 16, 205–211. doi: 10.1089/vbz.2015.1837
Raghavan, R. K., Goodin, D. G., Neises, D., Anderson, G. A., and Ganta, R. R. (2016b). Hierarchical Bayesian spatio-temporal analysis of climatic and socio-economic determinants of Rocky Mountain spotted fever. PLoS One 11:e0150180. doi: 10.1371/journal.pone.0150180
Raghavan, R. K., Heath, A. C. G., Lawrence, K. E., Ganta, R. R., Peterson, A. T., and Pomroy, W. E. (2020). Predicting the potential distribution of Amblyomma americanum (Acari: Ixodidae) infestation in New Zealand, using maximum entropy-based ecological niche modelling. Exp. Appl. Acarol. 80, 227–245. doi: 10.1007/s10493-019-00460-7
Rochat, E., Vuilleumier, S., Aeby, S., Greub, G., and Joost, S. (2021). Nested species distribution models of Chlamydiales in Ixodes ricinus (Tick) hosts in Switzerland. Appl. Environ. Microbiol. 87:e01237-20. doi: 10.1128/AEM.01237-20
Rochlin, I. (2019). Modeling the Asian longhorned tick (Acari: Ixodidae) suitable habitat in North America. J. Med. Entomol. 56, 384–391. doi: 10.1093/jme/tjy210
Rosentrater, L. D. (2010). Representing and using scenarios for responding to climate change. Wiley Interdiscip. Rev. Clim. Change 1, 253–259. doi: 10.1002/wcc.32
Roura-Pascual, N., Brotons, L., Peterson, A. T., and Thuiller, W. (2009). Consensual predictions of potential distributional areas for invasive species: a case study of Argentine ants in the Iberian Peninsula. Biol. Invasions 11, 1017–1031. doi: 10.1007/s10530-008-9313-3
Rydzewski, J., Mateus-Pinilla, N., Warner, R. E., Hamer, S., and Weng, H.-Y. (2011). Ixodes scapularis and Borrelia burgdorferi among diverse habitats within a natural area in east-central Illinois. Vector Borne Zoonot. Dis. 11, 1351–1358. doi: 10.1089/vbz.2010.0160
Rydzewski, J., Mateus-Pinilla, N., Warner, R. E., Nelson, J. A., and Velat, T. C. (2012). Ixodes scapularis (Acari: Ixodidae) distribution surveys in the Chicago metropolitan region. J. Med. Entomol. 49, 955–959. doi: 10.1603/me11233
Sage, K. M., Johnson, T. L., Teglas, M. B., Nieto, N. C., and Schwan, T. G. (2017). Ecological niche modeling and distribution of Ornithodoros hermsi associated with tick-borne relapsing fever in western North America. PLoS Negl. Trop. Dis. 11:e0006047. doi: 10.1371/journal.pntd.0006047
Semenza, J. C., Suk, J. E., Estevez, V., Ebi, K. L., and Lindgren, E. (2012). Mapping climate change vulnerabilities to infectious diseases in Europe. Environ. Health Perspect. 120, 385–392. doi: 10.1289/ehp.1103805
Signorini, M., Stensgaard, A.-S., Drigo, M., Simonato, G., Marcer, F., Montarsi, F., et al. (2019). Towards improved, cost-effective surveillance of Ixodes ricinus ticks and associated pathogens using species distribution modelling. Geospat. Health 14, 46–52. doi: 10.4081/gh.2019.745
Soberón, J. (2007). Grinnellian and Eltonian niches and geographic distributions of species. Ecol. Lett. 10, 1115–1123. doi: 10.1111/j.1461-0248.2007.01107.x
Soultan, A., and Safi, K. (2017). The interplay of various sources of noise on reliability of species distribution models hinges on ecological specialisation. PLoS One 12:e0187906. doi: 10.1371/journal.pone.0187906
Sousa, L. B., Craig, A., Chitkara, U., Fricker, S., Webb, C., Williams, C., et al. (2022). Methodological diversity in citizen science mosquito surveillance: a scoping review. Citiz. Sci. Theory Pract. 7:8. doi: 10.5334/cstp.469
Slatculescu, A. M., Clow, K. M., McKay, R., Talbot, B., Logan, J. J., Thickstun, C. R., et al. (2020). Species distribution models for the eastern blacklegged tick, Ixodes scapularis, and the Lyme disease pathogen, Borrelia burgdorferi, in Ontario, Canada. PLoS One 15:e0238126. doi: 10.1371/journal.pone.0238126
Soucy, J.-P. R., Slatculescu, A. M., Nyiraneza, C., Ogden, N. H., Leighton, P. A., Kerr, J. T., et al. (2018). High-resolution ecological niche modeling of Ixodes scapularis ticks based on passive surveillance data at the northern Frontier of Lyme disease emergence in North America. Vector Borne Zoonot. Dis. 18, 235–242. doi: 10.1089/vbz.2017.2234
Springer, Y. P., Jarnevich, C. S., Barnett, D. T., Monaghan, A. J., and Eisen, R. J. (2015). Modeling the present and future geographic distribution of the lone star tick, Amblyomma americanum (ixodida: Ixodidae), in the continental United States. Am. J. Trop. Med. Hyg. 93, 875–890. doi: 10.4269/ajtmh.15-0330
St. John, H. K., Adams, M. L., Masuoka, P. M., Flyer-Adams, J. G., Jiang, J., Rozmajzl, P. J., et al. (2016). Prevalence, distribution, and development of an ecological niche model of Dermacentor variabilis ticks positive for Rickettsia montanensis. Vector Borne Zoonot. Dis. 16, 253–263. doi: 10.1089/vbz.2015.1856
Stockwell, D. R. B., and Peterson, A. T. (2002). Effects of sample size on accuracy of species distribution models. Ecol. Model. 148, 1–13. doi: 10.1016/S0304-3800(01)00388-X
Stohlgren, T. J., Ma, P., Kumar, S., Rocca, M., Morisette, J. T., Jarnevich, C. S., et al. (2010). Ensemble habitat mapping of invasive plant species. Risk Anal. 30, 224–235. doi: 10.1111/j.1539-6924.2009.01343.x
Sun, R.-X., Lai, S.-J., Yang, Y., Li, X.-L., Liu, K., Yao, H.-W., et al. (2017). Mapping the distribution of tick-borne encephalitis in Mainland China. Ticks Tick Borne Dis. 8, 631–639. doi: 10.1016/j.ttbdis.2017.04.009
Sungirai, M., Moyo, D. Z., De Clercq, P., Madder, M., Vanwambeke, S. O., and De Clercq, E. M. (2018). Modelling the distribution of Rhipicephalus microplus and R. decoloratus in Zimbabwe. Vet. Parasitol. Reg. Stud. Rep. 14, 41–49. doi: 10.1016/j.vprsr.2018.08.006
Sutherst, R. W., and Bourne, A. S. (2009). Modelling non-equilibrium distributions of invasive species: a tale of two modelling paradigms. Biol. Invasions 11, 1231–1237. doi: 10.1007/s10530-008-9335-x
Title, P. O., and Bemmels, J. B. (2018). ENVIREM: an expanded set of bioclimatic and topographic variables increases flexibility and improves performance of ecological niche modeling. Ecography 41, 291–307. doi: 10.1111/ecog.02880
Tkadlec, E., Václavík, T., Kubelová, M., and Široký, P. (2018). Negative spatial covariation in abundance of two European ticks: diverging niche preferences or biotic interaction? Ecol. Entomol. 43, 804–812. doi: 10.1111/een.12668
Tokarevich, N. K., Tronin, A. A., Blinova, O. V., Buzinov, R. V., Boltenkov, V. P., Yurasova, E. D., et al. (2011). The impact of climate change on the expansion of Ixodes persulcatus habitat and the incidence of tick-borne encephalitis in the north of European Russia. Glob. Health Action 4:8448. doi: 10.3402/gha.v4i0.8448
Torina, A., Alongi, A., Naranjo, V., Estrada-Peña, A., Vicente, J., Scimeca, S., et al. (2008). Prevalence and genotypes of Anaplasma species and habitat suitability for ticks in a Mediterranean ecosystem. Appl. Environ. Microbiol. 74, 7578–7584. doi: 10.1128/AEM.01625-08
Tricco, A. C., Lillie, E., Zarin, W., O’Brien, K. K., Colquhoun, H., Levac, D., et al. (2018). PRISMA extension for scoping reviews (PRISMA-ScR): checklist and explanation. Ann. Intern. Med. 169, 467–473. doi: 10.7326/M18-0850
Veloz, S. D. (2009). Spatially autocorrelated sampling falsely inflates measures of accuracy for presence-only niche models. J. Biogeogr. 36, 2290–2299. doi: 10.1111/j.1365-2699.2009.02174.x
Vajana, E., Barbato, M., Colli, L., Milanesi, M., Rochat, E., Fabrizi, E., et al. (2018). Combining landscape genomics and ecological modelling to investigate local adaptation of indigenous Ugandan Cattle to East Coast Fever. Front. Genet. 9:385. doi: 10.3389/fgene.2018.00385
Vial, L., Ducheyne, E., Filatov, S., Gerilovych, A., McVey, D. S., Sindryakova, I., et al. (2018). Spatial multi-criteria decision analysis for modelling suitable habitats of Ornithodoros soft ticks in the Western Palearctic region. Vet. Parasitol. 249, 2–16. doi: 10.1016/j.vetpar.2017.10.022
Walter, M., Brugger, K., and Rubel, F. (2016). The ecological niche of Dermacentor marginatus in Germany. Parasitol. Res. 115, 2165–2174. doi: 10.1007/s00436-016-4958-9
Walter, M., Vogelgesang, J. R., Rubel, F., and Brugger, K. (2020). Tick-borne encephalitis virus and its European distribution in ticks and endothermic mammals. Microorganisms 8:1065. doi: 10.3390/microorganisms8071065
Wang, F., Wang, D., Guo, G., Hu, Y., Wei, J., and Liu, J. (2019). Species delimitation of the Dermacentor ticks based on phylogenetic clustering and niche modeling. PeerJ 7:e6911. doi: 10.7717/peerj.6911
Williams, H. W., Cross, D. E., Crump, H. L., Drost, C. J., and Thomas, C. J. (2015). Climate suitability for European ticks: assessing species distribution models against null models and projection under AR5 climate. Parasit. Vectors 8:440. doi: 10.1186/s13071-015-1046-4
Wilson, A. (1996). Appropriate strategies for the control or eradication of ticks and tick-borne diseases. Ann. N. Y. Acad. Sci. 791, 54–63. doi: 10.1111/j.1749-6632.1996.tb53511.x
Wisz, M. S., and Guisan, A. (2009). Do pseudo-absence selection strategies influence species distribution models and their predictions? An information-theoretic approach based on simulated data. BMC Ecol. 9:8. doi: 10.1186/1472-6785-9-8
Wisz, M. S., Hijmans, R. J., Li, J., Peterson, A. T., Graham, C. H., Guisan, A., et al. (2008). Effects of sample size on the performance of species distribution models. Divers. Distrib. 14, 763–773. doi: 10.1111/j.1472-4642.2008.00482.x
Wunderlich, R. F., Lin, Y.-P., Anthony, J., and Petway, J. R. (2019). Two alternative evaluation metrics to replace the true skill statistic in the assessment of species distribution models. Nat. Cconserv. 35, 97–116. doi: 10.3897/natureconservation.35.33918
Yawa, M., Nyangiwe, N., Muchenje, V., Kadzere, C. T., Mpendulo, T. C., and Marufu, M. C. (2018). Ecological preferences and seasonal dynamics of ticks (Acari: Ixodidae) on and off bovine hosts in the Eastern Cape Province, South Africa. Exp. Appl. Acarol. 74, 317–328. doi: 10.1007/s10493-018-0234-2
Zanet, S., Ferroglio, E., Battisti, E., and Tizzani, P. (2020). Ecological niche modelling of Babesia spp. infection in wildlife experimentally evaluated in northern Italy with reference to questing Ixodes ricinus ticks. Geospat. Health 15, 60–70. doi: 10.4081/gh.2020.843
Zannou, O. M., Ouedraogo, A. S., Biguezoton, A. S., Abatih, E., Coral-Almeida, M., Farougou, S., et al. (2021). Models for studying the distribution of ticks and tick-borne diseases in animals: a systematic review and a meta-analysis with a focus on Africa. Pathogens 10:893. doi: 10.3390/pathogens10070893
Zhao, G.-P., Wang, Y.-X., Fan, Z.-W., Ji, Y., Liu, M.-J., Zhang, W.-H., et al. (2021). Mapping ticks and tick-borne pathogens in China. Nat. Commun. 12:1075. doi: 10.1038/s41467-021-21375-1
Keywords: ticks, species distribution models, ecological niche models, habitat suitability models, climate change
Citation: Kopsco HL, Smith RL and Halsey SJ (2022) A Scoping Review of Species Distribution Modeling Methods for Tick Vectors. Front. Ecol. Evol. 10:893016. doi: 10.3389/fevo.2022.893016
Received: 09 March 2022; Accepted: 27 April 2022;
Published: 15 June 2022.
Edited by:
Sandrine Charles, Université de Lyon, FranceReviewed by:
Deon Bakkes, Agricultural Research Council of South Africa, South AfricaDivina Medina Amalin, De La Salle University, Philippines
Copyright © 2022 Kopsco, Smith and Halsey. This is an open-access article distributed under the terms of the Creative Commons Attribution License (CC BY). The use, distribution or reproduction in other forums is permitted, provided the original author(s) and the copyright owner(s) are credited and that the original publication in this journal is cited, in accordance with accepted academic practice. No use, distribution or reproduction is permitted which does not comply with these terms.
*Correspondence: Heather L. Kopsco, aGtvcHNjb0BpbGxpbm9pcy5lZHU=
†These authors have contributed equally to this work