- 1Department of Geography and Spatial Information Techniques, Ningbo University, Ningbo, China
- 2Advanced Institute of Natural Sciences, Beijing Normal University, Zhuhai, China
- 3National Key Lab of Regional and Urban Ecological Protection, Chinese Research Academy of Environmental Sciences, Beijing, China
- 4School of Life Sciences, Institute of Life Sciences and Green Development, Hebei University, Baoding, China
As an important regulator of ecosystem functions in river systems, microeukaryotes play an important role in energy and material conversion, yet little is known about the shift along a lentic-lotic continuum. In this study, the 18S rRNA genes sequencing was used to identify the microeukaryotic communities at 82 sites along a lentic-lotic continuum with the aim of understanding the impact of upstream inlet river on microeukaryotic communities in Baiyang Lake (BYD) and its downstream. Our results showed that the upstream inlet river affected the diversity and community composition of microeukaryotes in BYD and downstream rivers, and environmental variables greatly affected the composition of microeukaryotic community. The community composition in BYD had lower variabilities. Co-occurrence network analysis revealed that the network was non-random and clearly parsed into three modules, and different modules were relatively more abundant to a particular area. As keystone taxa, some nodes of the upstream microeukaryotic network played an important role in structuring network and maintaining the stability of the ecosystem. In BYD and downstream, the microeukaryotic network was highly fragmented, and the loss of keystone taxa would have an adverse impact on the integrity and function of the microeukaryotic community. Microeukaryotes had strong tendencies to co-occur, which may contribute to the stability and resilience of microeukaryotic communities. Overall, these findings extend the current understanding of the diversity and community composition of microeukaryotic along a lentic-lotic continuum.
Introduction
As an important regulator of ecosystem functions in river systems, microeukaryotes play an important role in energy and material conversion, and are used as biological indicators for water quality assessment (Zancarini et al., 2017; Cai et al., 2020). Eukaryotic plankton can quickly respond to the changes of water environment, because of its short life cycle and sensitive to the physicochemical changes of water body. The abundance, biomass, species diversity, and community composition of phytoplankton are severely affected by changes in water quality (Jeppesen et al., 2005; Xu et al., 2010; Wu et al., 2021). Several studies have shown that the structure and function of phytoplankton communities are directly affected by changes in water quality (Buchanan, 2020; Amorim and Moura, 2021). A recent study showed that when there is a significant environmental gradient in a polluted flowing water ecosystem, the structure of the microenvironmental phytoplankton community is largely determined by the species classification caused by local pollution, and the difference in community composition increases with the increase of the Euclidean distance of environmental variables (Xu et al., 2020). Eukaryotic plankton are key components in the trophic web structure, and play a vital role in the energy flow and nutrient cycle of the aquatic ecosystems (Boenigk and Arndt, 2002; Zubkov and Tarran, 2008). The impact of external disturbances (such as environmental factors, spatial processes and grazing pressure) on the phytoplankton community has always been of interest in ecological research (Guo et al., 2019, 2020; Wijewardene et al., 2021; Wu et al., 2022). However, there are few studies on how environmental conditions shape eukaryotic plankton communities (Caron and Hutchins, 2013).
Previous studies on phytoplankton communities were mostly based on morphological recognition, which were highly dependent on the identification experience of researchers. This method limits the research on phytoplankton diversity to a certain extent. The emergence of high-throughput sequencing makes it possible to quickly and accurately obtain the composition of the microbial community in samples (Amao et al., 2019). The high-throughput sequencing of environmental samples has the potential to detect rare, small and fragile species, and can be used to monitor and quantify biodiversity (Gao et al., 2020). Recently, the use of diatom to detect water quality and environmental changes in the freshwater system of the Han River in South Korea has been reported. Researchers have used 18S rRNA to analyze the molecular profiling of seasonal diatoms (Muhammad et al., 2021). Through high-throughput sequencing to explore the effects of nutrient and stoichiometry gradients on the microbial communities of Erhai Lake and its input rivers, the results showed that nutrients (phosphorus) were key drivers for the community structure dominated by Cyanobacteria (Liu et al., 2019). Overall, these studies provide a better understanding of the diversity and composition of microeukaryotic communities.
A lentic-lotic continuum links hydrology and ecology, and understanding the community shifts along a lentic-lotic continuum is conducive to our further understanding of hydro-ecological links to freshwater systems (Jones et al., 2017; Qu et al., 2018). However, there are still few studies on the shift of phytoplankton communities in the lentic-lotic continuum system. Along the lentic-lotic continuum, the composition and dissimilarities of phytoplankton are shaped more by local hydrological and physicochemical variables than species dispersal factors (Qu et al., 2018). But the study of shifts in the microeukaryotic community along a lentic-lotic continuum is still missing. The Baiyang Lake (BYD) is a flood slowing and detention area in the middle reaches of the Daqing River system in the Haihe River Basin, which is responsible for the flood regulation and storage of nine rivers. BYD and its upstream tributaries together constitute the southern branch of the Daqing River system in the Haihe River Basin. BYD has vast waters and rich resources of aquatic animals and plants. It is an important freshwater breeding base in northern China. Understanding how the upstream inlet river affects the microeukaryotic communities in BYD and downstream of the water quality gradient is essential for predicting the response of river ecosystems to global changes and human activities.
In this study, we examined how do microeukaryotic communities shift along the water quality gradient in a lentic-lotic continuum catchment (i.e., the BYD Basin) (Figure 1). We had three main research questions: (i) Does upstream inlet river affect the diversity of microeukaryotes in BYD and downstream rivers? (ii) Does upstream inlet river affect the community composition of microeukaryotes in BYD and downstream rivers? (iii) How does the upstream inlet river change the microeukaryotic communities of BYD and the downstream river? The change of environmental variables will directly affect the community structure of microeukaryotes. Thus, we hypothesized that: (H1) The alpha diversity in the upstream should be higher than that in BYD and downstream rivers along the water quality gradient; (H2) The upstream microeukaryotic community network should be the most complex; and (H3) Upstream inlet river could change the microeukaryotic communities of BYD and downstream rivers by changing environmental variables. In order to answer these questions and test these hypotheses, we analyzed the microeukaryotic communities in BYD and its upstream and downstream areas using high-throughput gene sequencing. By understanding the composition and changes of microeukaryotic communities in BYD and its upstream and downstream, this study attempts to fill the gaps in microeukaryotes changes along the water quality gradient in a lentic-lotic continuum catchment, and provides insights for the BYD Basin’s water environment management.
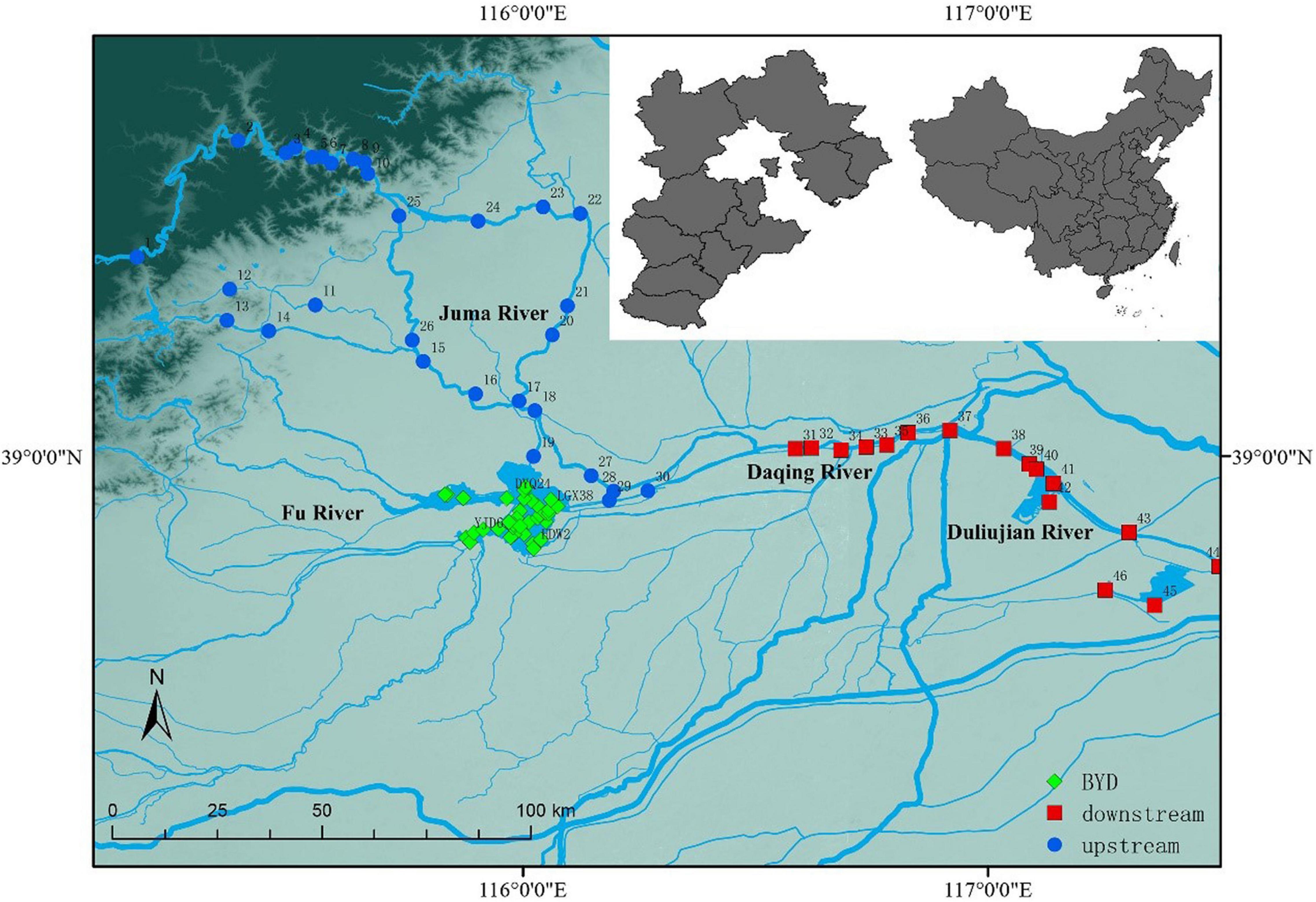
Figure 1. The distributions of 82 sampling sites located in the BYD Basin, northern China. The different shapes and colors in the figure represent different freshwater areas. Blue: upstream; green: BYD; red: downstream.
Materials and Methods
Study Area
This research was carried out in the BYD Basin (Figure 1). BYD (38°44′–38°59′ N, 115°45′–116°07′ E) is the largest lake in Hebei Province. It belongs to the Haihe River Basin and is the general term for the 143 interconnected large and small lakes at the junction of Baoding and Cangzhou. BYD is located in a warm temperate semi-humid continental monsoon climate zone. The four seasons are distinct and the precipitation is mostly concentrated in summer. The annual average temperature is 12.2°C, and the annual average precipitation is 529.7 mm (Tong et al., 2021). BYD has vast waters and rich resources of aquatic animals and plants. It is an important freshwater aquaculture base in northern China.
The main river entering BYD on the west side is the Fu River, and its water quality is poor (Brauns et al., 2016), leading to poor water quality on the west side and good water quality on the east side of BYD. The Juma River, another upstream river, is mainly distributed in mountainous areas, where the pollution mainly comes from tourism, and the water quality is relatively good. The Juma River with better water environment was selected for the upstream sampling. The samples in BYD were set up according to the gradient of water quality from west to east, covering the main lake of BYD. The Daqing River and Duliujian River are located in the downstream, both of which pass through urban areas. The physicochemical conditions of the river ecosystems have undergone great changes, and the water bodies have shown a certain degree of eutrophication and pollution (Chen et al., 2021).
Field Sampling and Chemical Analyses
The sample points covered the upstream, BYD, and downstream to reflect the differences in the characteristics of the overall microeukaryotes in the basin. A total of 82 sampling points were set up in the BYD Basin, including 30 samples in the upstream Juma River, 36 samples in BYD, and 16 samples in the downstream Daqing River and Duliujian River (Figure 1). At each sampling point, a YSI handheld meter (model 80; YSI, Yellow Springs, OH, United States) was used to measure water temperature (Temp), pH and conductivity (Cond). Latitude and longitude were measured using a Global Positioning System (GPS) unit (Triton 500, Magellan, Santa Clara, CA, United States). A total of 500 ml water, which was pre-filtered through a plankton net to remove larger multicellular organisms, was filtered with Whatman GFF filters using a vacuum pump. These filters were then wrapped in tin foil and stored at –80°C until DNA extraction and subsequent analyses. Water samples were acid-fixed and transported to the laboratory at 4°C for chemical analyses. Total nitrogen (TN) was analyzed using ion chromatography after persulfate oxidation. Total phosphorus (TP) was quantified using the ammonium molybdate method. Turbidity was measured using a portable turbidity meter HACH2100Q (HACH, Loveland, CO, United States). Chemical Oxygen Demand (COD) was determined using the rapid digestion spectrophotometry (HJ/T 399-2007).
DNA Extraction, PCR, Sequencing, and Sequence Processing
DNA was extracted using the E.Z.N.A. ® Soil DNA Kit according to the manufacturer’s instructions, and was quantified using QuantiFluor™-ST (Promega, Madison, WI, United States). The highly variable V4 region of the 18S rRNA were amplified using the forward primer containing sequence “GGCAAGTCTGGTGCCAG” and the reverse primer containing sequence “ACGGTATCTRATCRTCTTCG.” PCR reactions were performed in triplicate 20 μL mixture containing 4 μL of 5 × FastPfu Buffer, 2 μL of 2.5 mM dNTPs, 0.8 μL of each primer (5 μM), 0.4 μL of FastPfu Polymerase, and 10 ng of template DNA. DNA libraries were validated by Agilent 2100 Bioanalyzer (Agilent Technologies, Palo Alto, CA, United States), and quantified by using QuantiFluor™-ST (Promega, Madison, WI, United States). DNA libraries were multiplexed and loaded on an Illumina MiSeq instrument according to manufacturer’s instructions (Illumina, San Diego, CA, United States). Sequencing was performed using a 2 × 300 paired-end configuration; image analysis and base calling were conducted by the MiSeq Control Software embedded in the MiSeq instrument.
Raw sequences were demultiplexed and quality-filtered using QIIME (version 1.17). The operational taxonomic units (OTUs) were clustered with 97% similarity cutoff using UPARSE (version 7.1) and chimeric sequences were identified and removed using UCHIME. The taxonomy of each 18S rRNA gene sequence was analyzed by RDP Classifier against the silva database 138.
Data Analyses
Alpha diversity indexes, including Chao1, Observed OTUs, Shannon, and Phylogenetic Diversity, were calculated using QIIME (Caporaso et al., 2010). Beta diversity was calculated using Bray–Curtis distance and visualized by principal coordinate analysis (PCoA). The chord diagram was used to show the sum of relative abundances of dominant lineages (the lineages with a relative abundance >1%) of three freshwater areas. Three non-parametric statistical methods based on Adonis (Hartman et al., 2018), ANOSIM (Gao et al., 2019), and MRPP (Gao et al., 2019) were used to analyze the differences in the composition of microeukaryotic communities in the upstream, BYD and downstream using the vegan package (Oksanen et al., 2007). Distance-based redundancy analysis (db-RDA) was conducted using R package vegan 2.5-5 to assess the relationships between environmental variables and microeukaryotic community structure. The R package igraph was used to evaluate and visualize the co-occurrence interactions between OTUs (OTUs with an average relative abundance higher than 0.01% and exist in more than half of the samples) to reveal the complexity of microeukaryotic communities and the potential interrelationships between members of microeukaryotic communities. For the construction of co-occurrence networks, the Spearman correlations between all OTU pairs was calculated, and the P-values were adjusted for false discovery using FDR adjustment (Benjamini and Hochberg, 1995). We only considered strong (Spearman’s correlation coefficient r > 0.80 or r < −0.80) and significant (P < 0.05) correlations. Topological properties of the networks were calculated. The module structure of the networks was analyzed and the topological roles of the OTUs were assessed by calculating the connectivity of each OTU in the network, including within-module connectivity (Zi: within-module degree Z-score of node i) and among-module connectivity (Pi: participation coefficient of node i). The nodes with Zi ≥ 2.5 and/or Pi ≥ 0.62 were identified as keystone taxa (Deng et al., 2012).
Results
Physicochemical variables varied widely across the three areas (upstream, BYD and downstream) (Supplementary Table 1). For example, the turbidity ranged from 0.72 to 63 NTU at the time of sampling, the conductivity ranged from 264 to 10710 μs/cm, TN ranged from 0.63 to 5 mg/L, TP ranged from 0.01 to 0.75 mg/L, and COD ranged from 0.32 to 71.10 mg/L. The upstream had the lowest average turbidity (4.74 NTU), conductivity (517.32 μs/cm), and COD (14.63 mg/L) than BYD and downstream areas. The average pH (6.48), TN (0.37 mg/L), and TP (0.03 mg/L) of BYD were the lowest among the 3 areas. The average turbidity (19.68 NTU), conductivity (4014.69 μs/cm), TP (0.12 mg/L), COD (43.20 mg/L) were the highest in downstream than in other areas.
Alpha Diversity
Overall, 1,140,450 high-quality sequences were yielded through the sequencing of 18S rRNA genes and 1904 OTUs were detected at the 97% similarity level. Venn diagram showed that 451 OTUs were shared among the upstream, BYD, and downstream (Figure 2A). Downstream had the lowest alpha diversity indexes while the upstream had the highest value of Chao1 and Observed OTUs (Figure 2B). The results answered our first question (question i). Correlation analysis showed that alpha diversity indexes were negatively correlated with turbidity, temperature, conductivity, pH, COD, TP, TN, and positively correlated with the TN:TP ratio (Supplementary Figure 1).
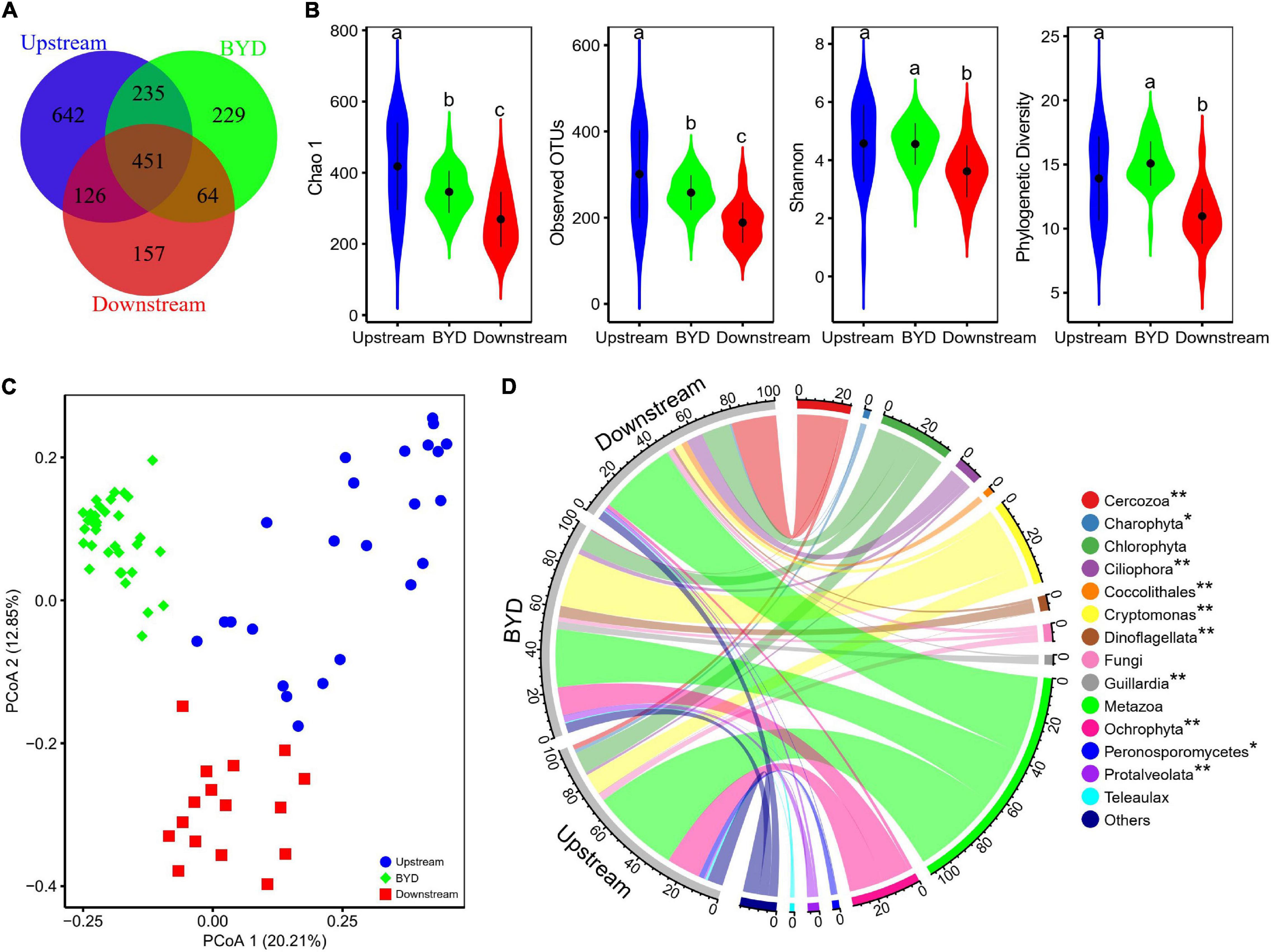
Figure 2. Basic differences of microeukaryotic communities along the lentic-lotic continuum in all three areas, including upstream, BYD, and downstream. (A) Venn diagram showing the unique and shared OTUs within and between three study areas. (B) Alpha diversity differences among three study areas. Different letters indicate the significant level at P < 0.05 determined by ANOVA. (C) Principal coordinates analysis (PCoA) based on Bray–Curtis distance in terms of the relative abundance of OTUs. (D) Chord diagram showing taxonomic composition and its differences of the microeukaryotic communities in three study areas. Only the lineages with an average relative abundance > 1% are shown. The significant differences were shown in “*” (p < 0.05) and “**” (p < 0.01).
Community Composition and Its Association to Environmental Factors
The results showed that the community composition of microeukaryotes in upstream, BYD, and downstream were differed significantly with each other (Figures 2C,D), which was further supported by the results of three non-parametric statistical approaches of Adonis, ANOSIM, and MRPP (Table 1). There were 14 lineages which had an average relative abundance above 1% in at least one area (Figure 2D). Ten of the lineages were significantly different among the three areas. Chlorophyta, Cryptomonas, and Metazoa were dominant (with an average relative richness > 5%) in all three areas. As shown by the mean Bray–Curtis distance between all pairs of samples, microeukaryotic communities in BYD had a significant lower mean Bray-Curtis distance value than that in upstream and downstream (Supplementary Figure 2), suggesting lower variabilities of the community composition (Figure 2C).
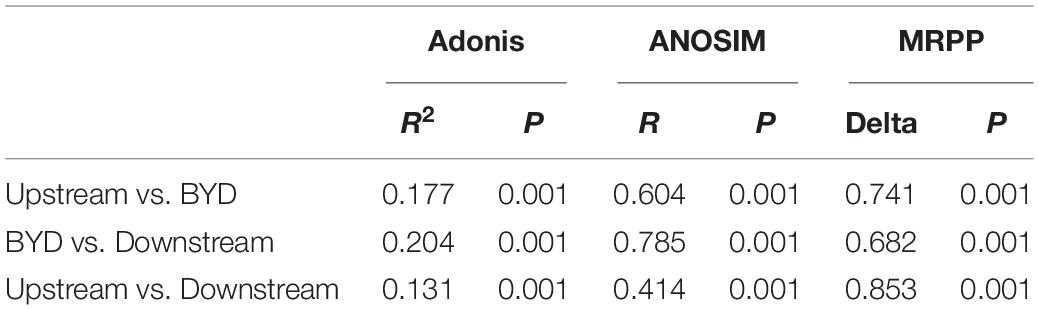
Table 1. Results of significant differences of microeukaryotic communities between the sample areas with three non-parametric statistical approaches.
Results of db-RDA showed that the shift of microeukaryotic communities in this Basin was significantly (P < 0.05) associated with all of the tested environmental factors (Figure 3A). Specifically, community shift in upstream was significantly (P < 0.05) associated to turbidity, temperature, conductivity, pH, COD, and the TN:TP ratio (Figure 3B). Community shift in BYD was significantly (P < 0.05) associated to conductivity, COD, TN, and TP (Figure 3C). Community shift in downstream area was significantly (P < 0.05) associated to conductivity and the TN:TP ratio (Figure 3D).
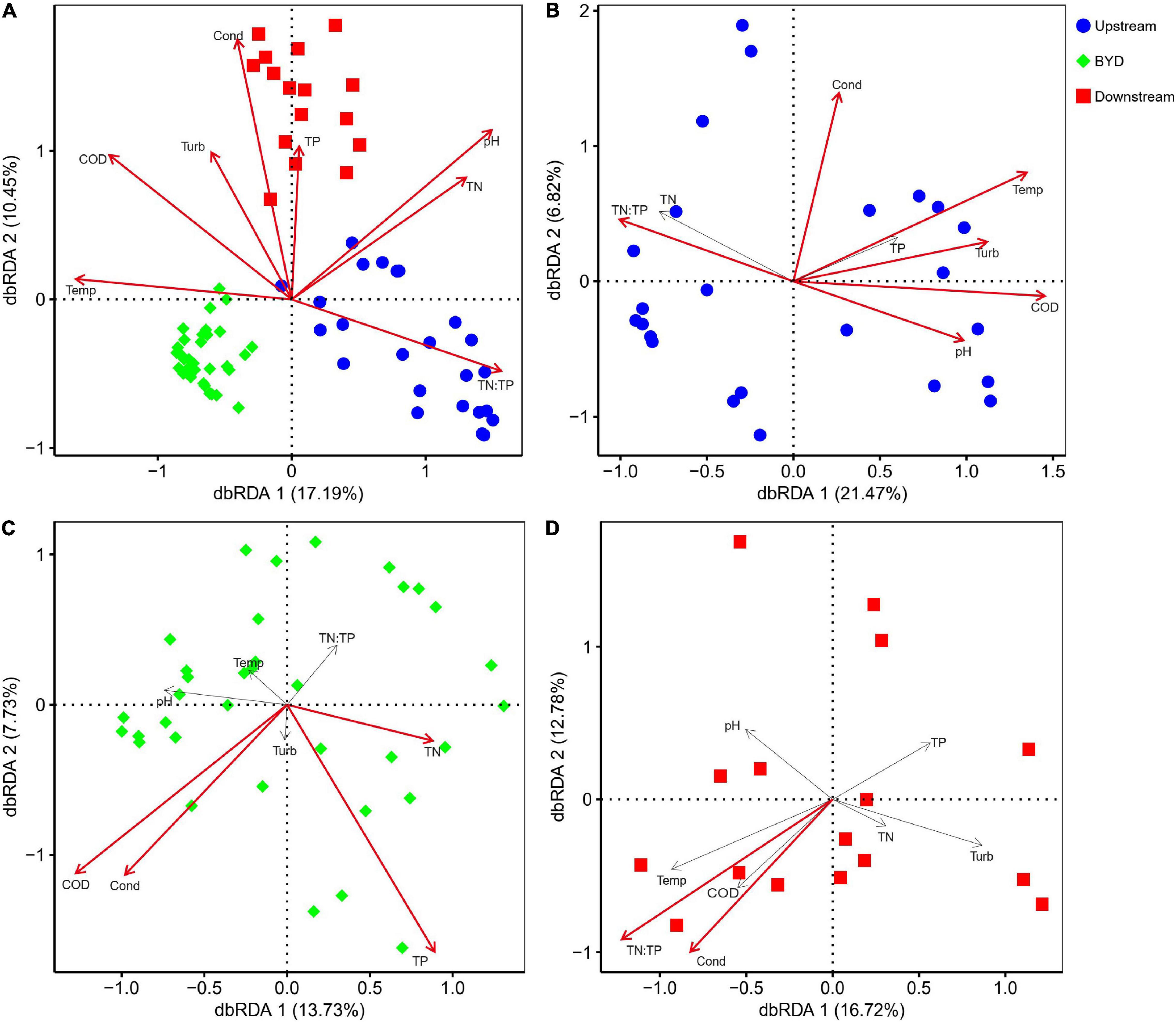
Figure 3. Distance-based redundancy analyses (db-RDA) reveal the relationships between environmental variables (arrows) and microeukaryotic communities (points) in (A) the all three areas, (B) upstream, (C) BYD, and (D) downstream. Significant variables (P < 0.05 by envfit function) are shown in red.
Co-occurrence Networks
For the microeukaryotic communities in the whole study area, the resulting network consisted of 442 nodes (OTUs) linked by 3591 edges (significant correlation relationships), with more than 98% of the edges were positive (Figure 4A). The average path length, clustering coefficient, centralization betweenness, and modularity were all greater than those of the respective random network (Supplementary Table 2), suggesting that the network was non-random and had “small-world” properties and a significant modular structure. The entire network was clearly parsed into three modules (Figure 4B). Module-A, -B, and -C accounted for the majority in upstream, BYD, downstream sites, respectively (Figure 5A). Ternary plot showed that most of the OTUs from the module-A, -B, and -C had higher relative abundances in upstream, BYD, and downstream sites, respectively, indicating that different modules were specific (relatively more abundant) to a particular area. Moreover, these three modules composed of OTUs which were primarily occupied by Metazoa in module-A, by Cryptomonas and Chlorophyta in module-B, and by Cercozoa and Ochrophyta in module-C (Figures 5B–D), suggesting that taxonomic relatedness played a key role in determining the modular structure. Based on the connectivity (within-module connectivity and between module connectivity), the co-occurrence network of microeukaryotic communities in the whole study area had three connectors and nine module hubs (Supplementary Figure 3). The connectors belonged to other modules, and the modules hubs came from module-A, -B, and -C.
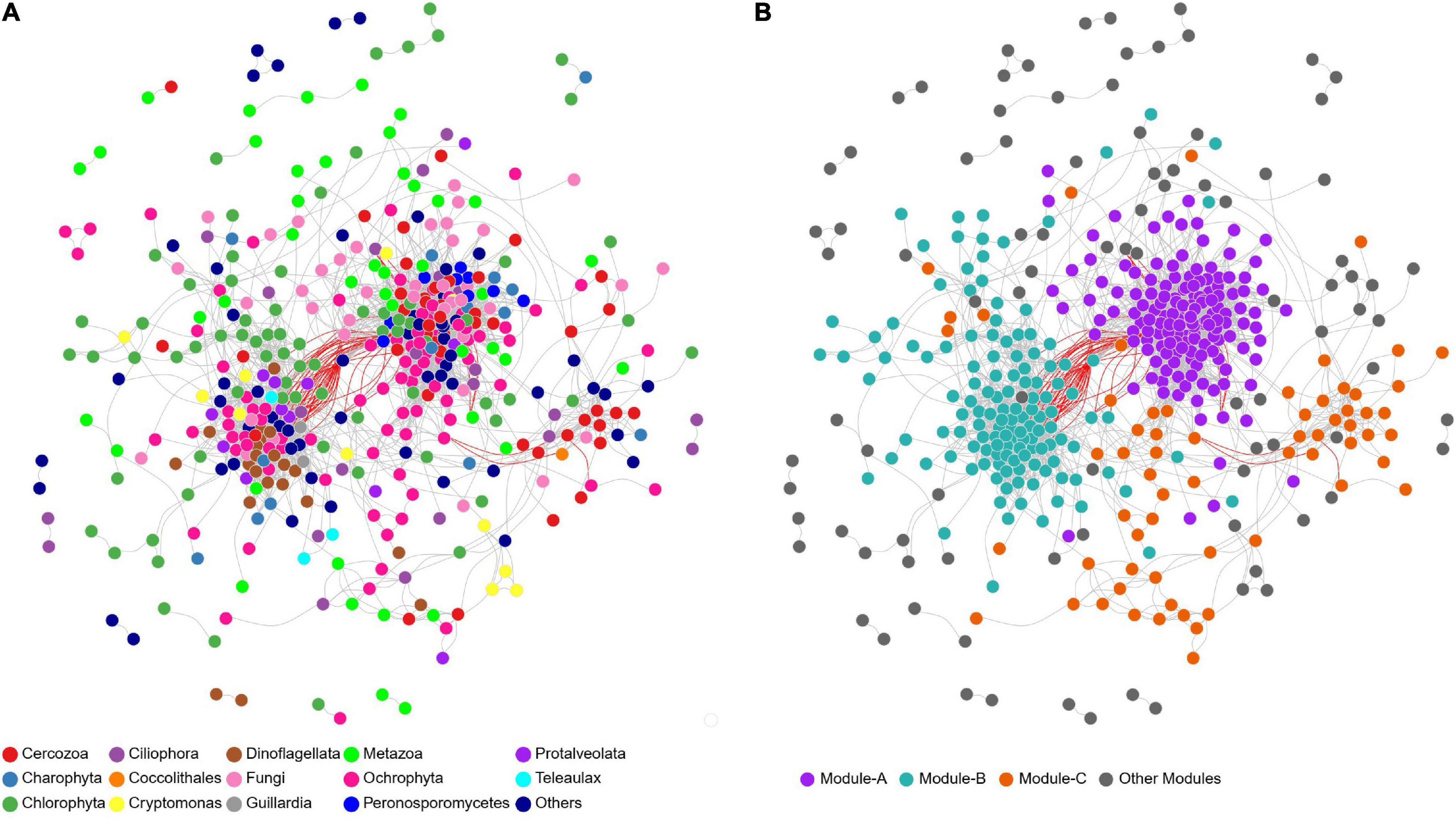
Figure 4. Co-occurrence network of microeukaryotic communities in the whole area colored by (A) major lineages and (B) major modules (modules have more than 50 nodes). Circle nodes represent OTUs. Edges represent Spearman correlations (only strong and significant relationships with Spearman’s r > 0.8 or r < –0.8 and P < 0.05 are shown). The gray and red lines indicate positive and negative correlations, respectively.
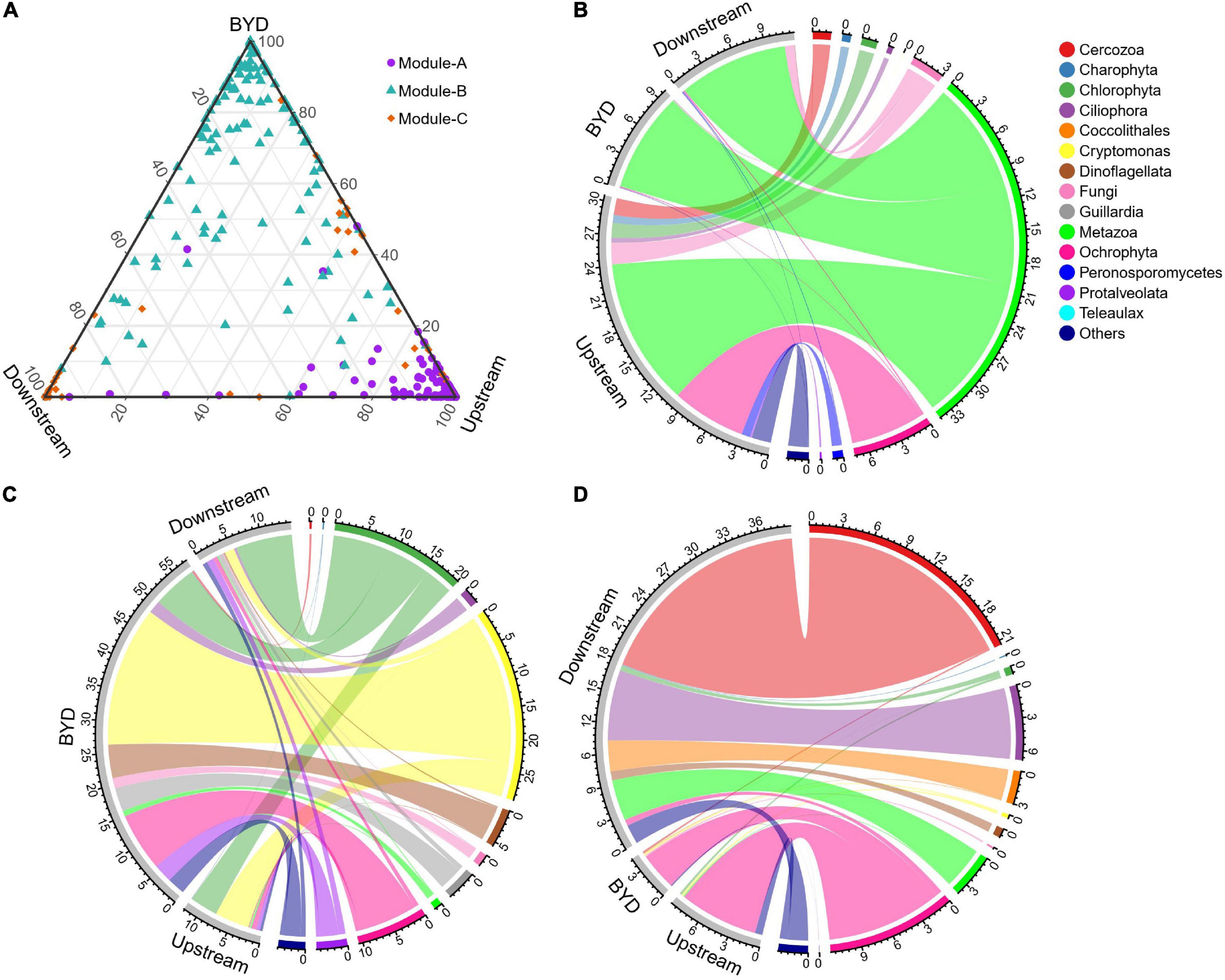
Figure 5. (A) Ternary plot of the OTUs in major modules of the co-occurrence network for the whole microeukaryotic communities of three area. Chord diagrams showing the taxonomic composition of (B) module-A, (C) module-B, and (D) module-C.
For each area specifically, the co-occurrence network of microeukaryotic communities consisted of 374 nodes and 4837 edges in upstream, 215 nodes and 558 edges in BYD, and 158 nodes and 290 edges in downstream, respectively (Figure 6). The microeukaryotic network in upstream had the highest average degree and clustering coefficient, and the lowest average pathlength and centralization betweenness, suggesting that microeukaryotic communities in upstream had the most complex network (Supplementary Table 2). This answered our second question (question ii). Moreover, according to the modularity, the network of upstream microeukaryotic communities had many keystone taxa playing as network hubs, module hubs, and connectors but do not have a well modular structure (Supplementary Table 2 and Figure 6A). On the contrary, the networks of BYD and downstream microeukaryotic communities had a highly modular structure with a few modular hubs and connectors (Supplementary Table 2 and Figures 6B,C).
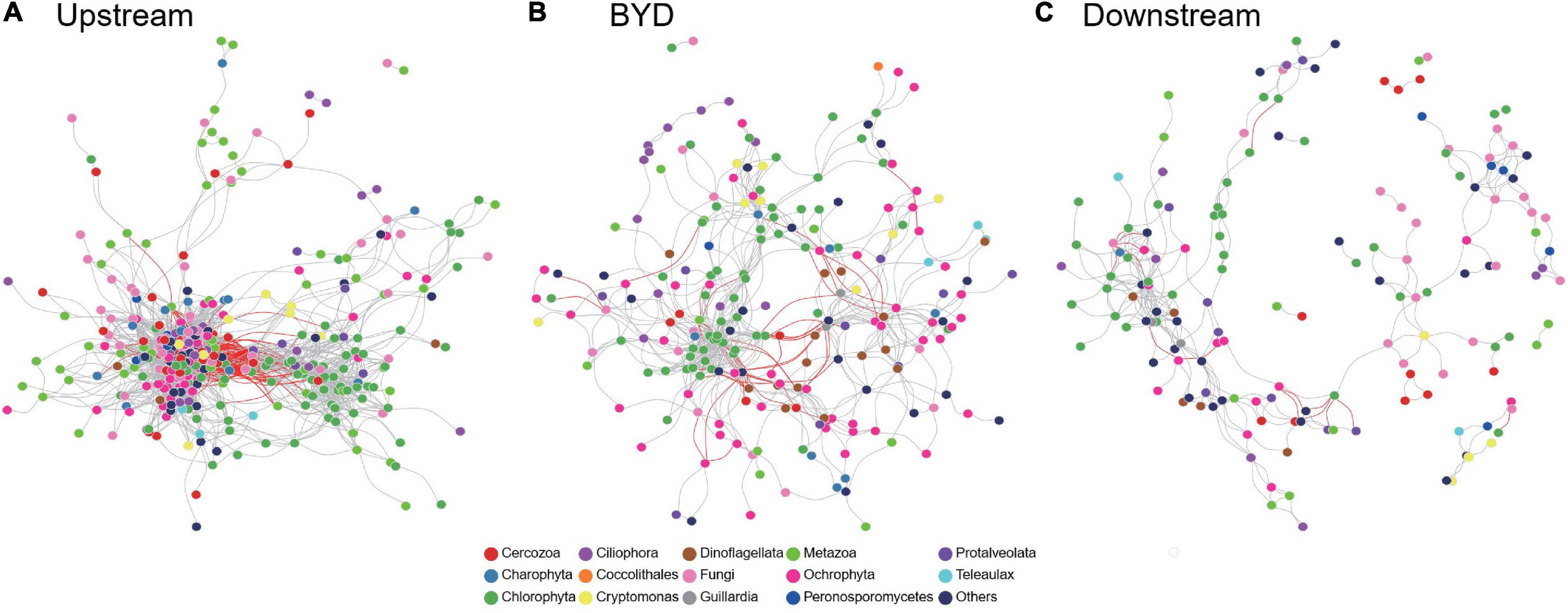
Figure 6. Co-occurrence network of microeukaryotic communities at (A) upstream, (B) BYD, and (C) downstream. Circle nodes represent OTUs. Edges represent Spearman correlations (only strong and significant relationships with Spearman’s r > 0.8 or r < –0.8 and P < 0.05 are shown). The gray and red lines indicate positive and negative correlations, respectively.
Discussion
Changes in Microeukaryotic Biodiversity
As hypothesized (H1), the downstream alpha diversity was significantly lower than that of the upstream, indicating that the diversity of microeukaryotes decreased significantly with the severity of water quality damage in the lentic-lotic continuum catchment. The decrease of downstream diversity may be due to the excessive interference of urban and agricultural activities on the water environment (higher TN and TP) (Teng et al., 2011; Hao et al., 2012; Hosen et al., 2017; Santos et al., 2017; Lopes et al., 2021). The variabilities of the community composition in BYD were significantly lower than that of upstream and downstream communities, reflecting significant changes in relative abundances of various taxa in upstream and downstream. Community composition is determined by environmental factors and spatial (distance) factors (Moran-Ordonez et al., 2015; Ptatscheck et al., 2020; Uritskiy et al., 2020). The upstream Juma River has pollution from tourism, while the downstream Daqing River has pollution influx along the way causing water quality degradation, which may affect the diversity of microeukaryotic communities in the lentic-lotic continuum catchment, resulting in high variations of alpha diversity in upstream and downstream samples. Because BYD is rich in fishery resources and human-made disturbances are serious, it may cause homogeneity between habitats, resulting in high similarity of microeukaryotic communities among samples in similar environments (Vilar et al., 2014; Parreira de Castro et al., 2018). In addition, the relative abundance of microeukaryotic communities in each sample site was not the same, which in turn reflected the high heterogeneity between different points in the lentic-lotic continuum catchment, indicating that the community composition of each sample site is unique to the ecosystem (Burgos-Caraballo et al., 2014). Environmental factors have a significant impact on the microeukaryotic community (Yuksek et al., 2006; Dunstan et al., 2012; Logares et al., 2020). Local environmental constraints such as environmental pollution caused by human activities can shape the local community structure of eukaryotes, which supports the species ordering hypothesis in river ecosystems (Lake, 2000; Huber et al., 2002). Since phytoplankton respond quickly and strongly to environmental disturbances, they have been used as an indicator of environmental changes and ecosystem status in freshwater ecosystems (Bianchi et al., 2003; Lepisto et al., 2004; Moustaka-Gouni et al., 2006; Li et al., 2015; Liu et al., 2015). Our results consistent with this view.
Changes in the Network Structure of Microeukaryotes
The co-occurrence network showed that the microeukaryotic community in the entire study area was non-random, with “small world” characteristics and a significant modular structure. The entire network was clearly parsed into three modules, which had different taxonomic composition. Several parameters representing the network topology were calculated. Compared with BYD and downstream, the upstream network had higher average degree and clustering coefficient in the lentic-lotic continuum catchment, while modularity and average path length showed the opposite trend (Supplementary Table 2). As hypothesized (H2), the upstream network showed higher transitivity than BYD and downstream networks (a measure of connectivity within a cluster). When the environment is disturbed by the outside world, it will quickly affect the entire network, causing the upstream network structure to be unstable, indicating that the network has a low ability to buffer environmental changes (Chen et al., 2021). These results indicated that the microeukaryotic OTUs were more connected upstream than BYD and downstream.
Compared with upstream networks, BYD and downstream networks had fewer OTUs. The inflow of microeukaryotes from the upstream can affect the structure of the microeukaryotic communities in BYD and downstream (Zhulidov et al., 2011). These inputs, to some extent, explained the overlap of the identified OTUs between the upstream, BYD and downstream (Figure 2A). Some of the microeukaryotes in BYD and the lower reaches may come from the upper reaches. However, the taxonomic composition of these communities was subsequently modified by local environments. In the lentic-lotic continuum catchment, microeukaryotes had strong tendencies to co-occur (93% positive edges in upstream, 92% positive edges in BYD, and 94% positive edges in downstream, Supplementary Table 2). The relatively high proportion of positive correlations in microeukaryotic networks indicated that in a given environment, these taxa were interdependent or complementary, and cooperative relationships are strong (Fernandez et al., 2015). Under the gradient of water quality changes along the lentic-lotic continuum, from upstream to BYD and downstream, the network gradually became smaller and there were fewer connections. This may be due to the greater amplitude or frequency of disturbance events in the middle and lower reaches. In BYD and downstream, environmental variables (such as temperature, hydrology) may be more variable and extreme than upstream. The microeukaryotic co-occurrence network structure responded to environmental disturbances, which may explain the difference in scale and structure of upstream, BYD and downstream networks along the lentic-lotic continuum (Yang et al., 2022). The composition and dissimilarities of phytoplankton were more affected by local hydrological and physicochemical variables along the lentic-lotic continuum (Qu et al., 2018).
Module structures can reveal more ecological properties of complex communities and shed light on the different groups of nodes performing different functions (Xiong et al., 2018). The way in which water quality affected the river’s eukaryote community not only represents the local environmental impact, but also represents the regional process. For instance, module-A was dominated by Metazona, which has a certain role in in promoting water purification. Module-B was dominated by Cryptomonas and Chlorophyta. Studies have shown that Chlorophyta can absorb nitrogen and phosphorus sources in the water body (Carrick and Lowe, 1989). Cryptomonas were also found to be favored by reductions of nutrient loading in shallow lakes (Jeppesen et al., 2007). This was consistent with the nutritional status of BYD. According to module structure, some nodes of the upstream microeukaryotic network were identified as keystone taxa indicating their important roles in structuring network and maintaining the stability of the ecosystem (Banerjee et al., 2018). There were only a few modular hubs and connectors in BYD and downstream (Supplementary Figure 3), indicating the high fragmentation of the network and the strong independence of modules. The loss of these keystone taxa will increase the fragmentation of the network, and adversely affect the integrity and function of the microeukaryotic community (Widder et al., 2014; Banerjee et al., 2018).
Environmental Factors Driving the Variation of Microeukaryotic Communities
Revealing the spatial distribution characteristics of microeukaryotic communities and their environmental driving factors is crucial to clarify the stability and function of the ecosystem (Ren et al., 2020). In the upstream, water from the Juma River, the Fu River and the South-to-North Water Diversion Project are collected. In addition to the Duliujian River, there are other rivers flowing downstream. The overlap ratio of the three freshwater areas is not high. This may explain the low similarity of microeukaryotic communities in the three freshwater areas. Our results also showed the main changes of microeukaryotic community composition along the lentic-lotic continuum. The upstream water body is flowing, BYD is a shallow lake, and the downstream is intercepted by dams. In the lentic-lotic continuum catchment, the fluidity of water body is not strong. Moreover, the downstream area has a high salinity because it is close to the estuary to the sea. There were many species found only downstream. In the lentic-lotic continuum, different water body states may have an impact on the microeukaryotic community (Fraisse et al., 2013; Dzhayani, 2020). As expected in H3, these changes in community composition can be attributed to changes in local environments (Hooper et al., 2005; Yuksek et al., 2006; Dunstan et al., 2012; Logares et al., 2020). The diversity of phytoplankton is mainly directly affected by river physicochemical and hydrodynamic variables (Padisak et al., 2006; Isabwe et al., 2018).
The changes of nutrient concentrations such as TN and TP are the main reasons driving the spatial changes of phytoplankton community structure (Parkes and Duggan, 2012; Wang et al., 2015; Xiong et al., 2016). The microtrophic community is mainly determined by the level of nutrients, and the increase of nutrients will change the community composition of phytoplankton, macroinvertebrates and fish in the water body (Chessman et al., 1999; Weijters et al., 2009; Zhang et al., 2021). The microeukaryotic community was mainly composed of Chlorophyta, Cryptomonas and Metazoa (with a relative richness >5%) in our study areas. The higher relative abundance of Chlorophyta may be partly due to the higher nitrogen and phosphorus values downstream. Studies have shown that within a certain range of nutrient salt concentration, filamentous Chlorophyta can absorb 90% of the available nitrogen sources and more than 80% of the phosphorus sources in the water body (Carrick and Lowe, 1989). This has a certain contribution to reduce the level of lake eutrophication, improving the self-purification capacity and transparency and restoring high aquatic vegetation communities. The water body at the downstream sampling point showed a certain degree of eutrophication, and the high abundance of Chlorophyta might have a beneficial effect on mitigating eutrophication in this area. Some studies have found that the existence of Cryptomonas are beneficial to the reduction of nutrient loading in shallow lakes (Jeppesen et al., 2007). Therefore, the presence of Chlorophyta and Cryptomonas suggested that the composition of microeukaryotic communities might be a response to the increased nutrient content in these rivers. Such species have ecological significance in the environment of eukaryotes. The change of temperature will indirectly affect the community composition of eukaryotes by affecting the physicochemical properties of the water body. The zooplankton microfauna are all chemoheterotrophic microorganisms, so the decrease in DO caused by warming can inhibit their diversity (Fortney et al., 2018). In BYD and downstream, the higher temperature may lead to the lower relative abundance of the Metazoa. At the same time, higher temperature will promote the increase of nitrate nitrogen, provide nitrogen source for phytoplankton, and may promote the increase of the abundance of Chlorophyta and Cryptomonas, thereby changing the community composition of eukaryotes. The increase in turbidity will affect the light penetration, resulting in the light tilt of phytoplankton growth (Shi et al., 2017). The lower abundance of Cryptomonas in the downstream may be due to the higher turbidity, TN and TP because, low light and high salinity conditions are harmful to the growth of these pigmented phyla in freshwater (Domingues et al., 2011). DO is the main driving force of nutrient and energy flow patterns in aquatic ecosystems (Diaz and Rosenberg, 2008). The DO of upstream and BYD was significantly higher than that of downstream, indicating that the water self-purification ability of upstream and BYD is better. There are many functional protozoa and micrometazoa in BYD and downstream, indicating that the water body has a high degree of self-purification (Li et al., 2019). The changes in the abundance of Metazoa may be a response to the degree of water quality degradation, which is consistent with the water quality in all three areas. COD marks the content of organic pollutants in the water (Margot et al., 2013). In short, the downstream water quality was worse, the water body eutrophication and organic pollution were more serious, which may have an impact on the microeukaryotic community composition of the downstream samples (Duan et al., 2009; Wang et al., 2011; Yang et al., 2012; Buchanan, 2020; Amorim and Moura, 2021).
Water diversion projects are playing an increasingly important role in improving the water quality of rivers. However, water transfers will also affect the concentration of nutrients and other physicochemical variables, which will have a certain impact on the water environment, thereby affecting the diversity and community composition of phytoplankton (Yin et al., 2011; Dai et al., 2020; Xu et al., 2021; Yang et al., 2021). At the same time, the short-period hydrological conditions brought by the water diversion project have also shaped the community composition of biofilms to a certain extent (Guo et al., 2020). In summary, the relative abundance of certain phyla, Chlorophyta and Cryptomonas were significantly different between upstream, BYD and downstream sample points, indicating that the variabilities of microeukaryotic communities are related to environmental factors. At present, there are many large-scale water diversion projects, especially in China, such as the South-to-North Water Diversion Project, BYD is also its water receiving lake. Large-scale water diversion projects are needed not only for meeting human demand for water, but also for maintaining the healthy development of the ecosystem. However, the construction of large-scale water diversion projects will undermine the concept of natural cascading reservoir continuum and increase the degree of homogeneity among water bodies (Barbosa et al., 1999; Nekola and White, 1999). From our results, the water quality and phytoplankton community of BYD in the lentic-lotic continuum system were all affected by the incoming water, so we suggest that river managers take some measures to reduce the impact of water diversion projects on water quality, such as dredging sediment, increasing water flow, and controlling sources of pollution.
Conclusion
Our results clearly demonstrated that the upstream incoming water had a certain impact on the diversity and community composition of microeukaryotes in BYD and downstream rivers by influencing the water environment. The lower variabilities of BYD’s community composition might be due to the homogeneity among habitats. Co-occurrence network analysis further revealed that microeukaryotes had a strong tendency to co-occur, which may help maintain community stability and ecological function. In addition, microeukaryotic networks was non-random and had “small-world” properties and a significant modular structure. Some nodes of the upstream microeukaryotic network, as keystone taxa, played an important role in structuring network and maintaining the stability of the ecosystem. In BYD and downstream, the microeukaryotic network was highly fragmented, and the loss of keystone taxa would be detrimental to the integrity and function of the microeukaryotic community. In summary, our results can provide a better understanding of the composition and diversity of microeukaryotes communities in the lentic-lotic continuum system, and provides insights for BYD’s water environment management. In addition, the study has a potential guiding significance for large-scale water conservancy diversion.
Data Availability Statement
The datasets presented in this study can be found in online repositories. The names of the repository/repositories and accession number(s) can be found below: https://www.ncbi.nlm.nih.gov/, SAMN26210067.
Author Contributions
YW: methodology, data curation, formal analysis, writing – original draft, writing – review and editing. ZR: methodology, data curation, and formal analysis. PH: conceptualization, methodology, writing – review and editing. JX: conceptualization and methodology. DL: methodology. CL: conceptualization and methodology. BL: writing – review and editing. NW: conceptualization, methodology, supervision, writing – review and editing. All authors contributed to the article and approved the submitted version.
Funding
This study was supported by the National Key R&D Program of China (2017YFC0506601) and the Biodiversity Survey and Assessment Project of the Ministry of Ecology and Environment, China (2019HJ2096001006).
Conflict of Interest
The authors declare that the research was conducted in the absence of any commercial or financial relationships that could be construed as a potential conflict of interest.
Publisher’s Note
All claims expressed in this article are solely those of the authors and do not necessarily represent those of their affiliated organizations, or those of the publisher, the editors and the reviewers. Any product that may be evaluated in this article, or claim that may be made by its manufacturer, is not guaranteed or endorsed by the publisher.
Supplementary Material
The Supplementary Material for this article can be found online at: https://www.frontiersin.org/articles/10.3389/fevo.2022.887787/full#supplementary-material
References
Amao, J. A., Barooah, M., and Omojasola, P. F. (2019). Comparative 16S rDNA metagenomics study of two samples of cassava peel heap from Nigeria and India. 3 Biotech 9:418. doi: 10.1007/s13205-019-1941-z
Amorim, C. A., and Moura, A. D. N. (2021). Ecological impacts of freshwater algal blooms on water quality, plankton biodiversity, structure, and ecosystem functioning. Sci. Total Environ. 758:140659. doi: 10.1016/j.scitotenv.2020.143605
Banerjee, S., Schlaeppi, K., and van der Heijden, M. G. A. (2018). Keystone taxa as drivers of microbiome structure and functioning. Nat. Rev. Microbiol. 16, 567–576. doi: 10.1038/s41579-018-0024-1
Barbosa, F. A. R., Padisák, J., Espíndola, E. L. G., Borics, G., and Rocha, O. (1999). “The cascading reservoir continuum concept (CRCC) and its application to the river Tietê-basin, São Paulo State, Brazil,” in Theoretical Reservoir Ecology and Its Applications, eds J. G. Tundisi and M. Straskraba (São Carlos: Brazilian Academy of Sciences), 425–437.
Benjamini, Y., and Hochberg, Y. (1995). Controlling The False Discovery Rate - A Practical And Powerful Approach To Multiple Testing. J. R. Stat. Soc. B Stat. Methodol. 57, 289–300. doi: 10.1111/j.2517-6161.1995.tb02031.x
Bianchi, F., Acri, F., Aubry, F. B., Berton, A., Boldrin, A., Camatti, E., et al. (2003). Can plankton communities be considered as bio-indicators of water quality in the Lagoon of Venice? Mar. Pollut. Bull. 46, 964–971. doi: 10.1016/S0025-326X(03)00111-5
Boenigk, J., and Arndt, H. (2002). Bacterivory by heterotrophic flagellates: community structure and feeding strategies. Antonie Van Leeuwenhoek 81, 465–480. doi: 10.1023/a:1020509305868
Brauns, B., Bjerg, P. L., Song, X., and Jakobsen, R. (2016). Field scale interaction and nutrient exchange between surface water and shallow groundwater in the Baiyang Lake region, North China Plain. J. Environ. Sci. 45, 60–75. doi: 10.1016/j.jes.2015.11.021
Buchanan, C. (2020). A Water Quality Binning Method to Infer Phytoplankton Community Structure and Function. Estuar. Coast. 43, 661–679. doi: 10.1007/s12237-020-00714-3
Burgos-Caraballo, S., Cantrell, S. A., and Ramirez, A. (2014). Diversity of benthic biofilms along a land use gradient in tropical headwater streams, Puerto Rico. Microb. Ecol. 68, 47–59. doi: 10.1007/s00248-014-0401-x
Cai, W., Li, Y., Zhang, W., Niu, L., Wang, L., and Cheng, H. (2020). Composition, Distribution, and Assembly Patterns of Eukaryotic Communities Under Vertical Geochemical Gradients in a Polluted Urban River. Water Air Soil Pollut. 231:335.
Caporaso, J. G., Kuczynski, J., Stombaugh, J., Bittinger, K., Bushman, F. D., Costello, E. K., et al. (2010). QIIME allows analysis of high-throughput community sequencing data. Nat. Methods 7, 335–336. doi: 10.1038/nmeth.f.303
Caron, D. A., and Hutchins, D. A. (2013). The effects of changing climate on microzooplankton grazing and community structure: drivers, predictions and knowledge gaps. J. Plankton Res. 35, 235–252. doi: 10.1093/plankt/fbs091
Carrick, H. J., and Lowe, R. L. (1989). Benthic algal response to N and P enrichment along a pH gradient. Hydrobiologia 179, 119–127. doi: 10.1007/bf00007599
Chen, Z.-J., Lin, L.-A., Li, Y.-J., Chen, Y., Zhang, H., Han, H., et al. (2021). Shifts in Rhizosphere Bacterial Community Structure, Co-occurrence Network, and Function of Miscanthus Following Cadmium Exposure. Huan jing ke xue 42, 3997–4004. doi: 10.13227/j.hjkx.202011198
Chessman, B., Growns, I., Currey, J., and Plunkett-Cole, N. (1999). Predicting diatom communities at the genus level for the rapid biological assessment of rivers. Freshw. Biol. 41, 317–331. doi: 10.1046/j.1365-2427.1999.00433.x
Dai, J., Wu, S., Wu, X., Lv, X., Sivakumar, B., Wang, F., et al. (2020). Impacts of a large river-to-lake water diversion project on lacustrine phytoplankton communities. J. Hydrol. 587:124938. doi: 10.1016/j.jhydrol.2020.124938
Deng, Y., Jiang, Y.-H., Yang, Y., He, Z., Luo, F., and Zhou, J. (2012). Molecular ecological network analyses. BMC Bioinformatics 13:113. doi: 10.1186/1471-2105-13-113
Diaz, R. J., and Rosenberg, R. (2008). Spreading dead zones and consequences for marine ecosystems. Science 321, 926–929. doi: 10.1126/science.1156401
Domingues, R. B., Anselmo, T. P., Barbosa, A. B., Sommer, U., and Galvao, H. M. (2011). Light as a driver of phytoplankton growth and production in the freshwater tidal zone of a turbid estuary. Estuar. Coast. Shelf Sci. 91, 526–535. doi: 10.1016/j.ecss.2010.12.008
Duan, H., Ma, R., Xu, X., Kong, F., Zhang, S., Kong, W., et al. (2009). Two-Decade Reconstruction of Algal Blooms in China’s Lake Taihu. Environ. Sci. Technol. 43, 3522–3528. doi: 10.1021/es8031852
Dunstan, P. K., Althaus, F., Williams, A., and Bax, N. J. (2012). Characterising and Predicting Benthic Biodiversity for Conservation Planning in Deepwater Environments. PLoS One 7:e36558. doi: 10.1371/journal.pone.0036558
Dzhayani, E. A. (2020). Phytoplankton of the Flowing and Flow-regulated Stretches of the Ural River in Different Seasons (ФИTOПЛaHкTOH ПpOTOчHO;ГO И зapеГУлиpOBaHHOГOyчacTкOBpГpaлBpaзHЬIe ceзOHЬI). Povolz. Ekol. Z. 1, 31–43.
Fernandez, M., Riveros, J. D., Campos, M., Mathee, K., and Narasimhan, G. (2015). Microbial “social networks”. BMC Genomics 16:S6. doi: 10.1186/1471-2164-16-S11-S6
Fortney, N. W., He, S., Converse, B. J., Boyd, E. S., and Roden, E. E. (2018). Investigating the Composition and Metabolic Potential of Microbial Communities in Chocolate Pots Hot Springs. Front. Microbiol. 9:2075. doi: 10.3389/fmicb.2018.02075
Fraisse, S., Bormans, M., and Lagadeuc, Y. (2013). Morphofunctional traits reflect differences in phytoplankton community between rivers of contrasting flow regime. Aquat. Ecol. 47, 315–327. doi: 10.1007/s10452-013-9446-z
Gao, G. F., Li, P. F., Zhong, J. X., Shen, Z. J., Chen, J., Li, Y. T., et al. (2019). Spartina alterniflora invasion alters soil bacterial communities and enhances soil N2O emissions by stimulating soil denitrification in mangrove wetland. Sci. Total Environ. 653, 231–240. doi: 10.1016/j.scitotenv.2018.10.277
Gao, Z., Xu, H., Zhang, P., Ji, D., Xia, L., Wang, X., et al. (2020). Variations in bacterial community structures during geothermal water recharge-induced bioclogging. J. Environ. Sci. Health A Tox. Hazard. Subst. Environ. Eng. 55, 629–637. doi: 10.1080/10934529.2020.1724744
Guo, K., Wu, N., Manolaki, P., Baattrup-Pedersen, A., and Riis, T. (2020). Short-period hydrological regimes override physico-chemical variables in shaping stream diatom traits, biomass and biofilm community functions. Sci. Total Environ. 743:140720. doi: 10.1016/j.scitotenv.2020.140720
Guo, K., Wu, N., Wang, C., Yang, D., He, Y., Luo, J., et al. (2019). Trait dependent roles of environmental factors, spatial processes and grazing pressure on lake phytoplankton metacommunity. Ecol. Indic. 103, 312–320. doi: 10.1016/j.ecolind.2019.04.028
Hao, J.-F., Liu, H.-Y., Hu, H.-B., An, J., and Zhang, X.-H. (2012). Responses of wetland water quality to influence the strengthness of urbanization in Nanjing, China. Huan jing ke xue 33, 2259–2264.
Hartman, K., van der Heijden, M. G. A., Wittwer, R. A., Banerjee, S., Walser, J.-C., and Schlaeppi, K. (2018). Cropping practices manipulate abundance patterns of root and soil microbiome members paving the way to smart farming. Microbiome 6:14.
Hooper, D. U., Chapin, F. S., Ewel, J. J., Hector, A., Inchausti, P., Lavorel, S., et al. (2005). Effects of biodiversity on ecosystem functioning: a consensus of current knowledge. Ecol. Monogr. 75, 3–35. doi: 10.1890/04-0922
Hosen, J. D., Febria, C. M., Crump, B. C., and Palmer, M. A. (2017). Watershed Urbanization Linked to Differences in Stream Bacterial Community Composition. Front. Microbiol. 8:1452. doi: 10.3389/fmicb.2017.01452
Huber, J. A., Butterfield, D. A., and Baross, J. A. (2002). Temporal changes in archaeal diversity and chemistry in a mid-ocean ridge subseafloor habitat. Appl. Environ. Microbiol. 68, 1585–1594. doi: 10.1128/AEM.68.4.1585-1594.2002
Isabwe, A., Yang, J. R., Wang, Y., Liu, L., Chen, H., and Yang, J. (2018). Community assembly processes underlying phytoplankton and bacterioplankton across a hydrologic change in a human-impacted river. Sci. Total Environ. 630, 658–667. doi: 10.1016/j.scitotenv.2018.02.210
Jeppesen, E., Sondergaard, M., Jensen, J. P., Havens, K. E., Anneville, O., Carvalho, L., et al. (2005). Lake responses to reduced nutrient loading - an analysis of contemporary long-term data from 35 case studies. Freshw. Biol. 50, 1747–1771. doi: 10.1111/j.1365-2427.2005.01415.x
Jeppesen, E., Sondergaard, M., Meerhoff, M., Lauridsen, T. L., and Jensen, J. P. (2007). Shallow lake restoration by nutrient loading reduction - some recent findings and challenges ahead. Hydrobiologia 584, 239–252. doi: 10.1007/978-1-4020-6399-2_22
Jones, A. E., Hodges, B. R., McClelland, J. W., Hardison, A. K., and Moffett, K. B. (2017). Residence-time-based classification of surface water systems. Water Resour. Res. 53, 5567–5584. doi: 10.1002/2016wr019928
Lake, P. S. (2000). Disturbance, patchiness, and diversity in streams. J. North Am. Benthol. Soc. 19, 573–592. doi: 10.2307/1468118
Lepisto, L., Holopainen, L. L., and Vuoristo, H. (2004). Type-specific and indicator taxa of phytoplankton as a quality criterion for assessing the ecological status of Finnish boreal lakes. Limnologica 34, 236–248. doi: 10.1016/s0075-9511(04)80048-3
Li, Q., Zhao, Y., Zhang, X., Wei, Y., Qiu, L., Wei, Z., et al. (2015). Spatial heterogeneity in a deep artificial lake plankton community revealed by PCR-DGGE fingerprinting. Chin. J. Oceanol. Limnol. 33, 624–635. doi: 10.1007/s00343-015-4184-9
Li, X., Huang, J., Filker, S., Stoeck, T., Bi, Y., Yu, Y., et al. (2019). Spatio-temporal patterns of zooplankton in a main-stem dam affected tributary: a case study in the Xiangxi River of the Three Gorges Reservoir, China. Sci. China Life Sci. 62, 1058–1069. doi: 10.1007/s11427-018-9523-0
Liu, L., Yang, J., Lv, H., Yu, X., Wilkinson, D. M., and Yang, J. (2015). Phytoplankton Communities Exhibit a Stronger Response to Environmental Changes than Bacterioplankton in Three Subtropical Reservoirs. Environ. Sci. Technol. 49, 10850–10858. doi: 10.1021/acs.est.5b02637
Liu, Y., Qu, X., Elser, J. J., Peng, W., Zhang, M., Ren, Z., et al. (2019). Impact of Nutrient and Stoichiometry Gradients on Microbial Assemblages in Erhai Lake and Its Input Streams. Water 11:1711. doi: 10.3390/w11081711
Logares, R. I, Deutschmann, M., Junger, P. C., Giner, C. R., Krabberod, A. K., Schmidt, T. S. B., et al. (2020). Disentangling the mechanisms shaping the surface ocean microbiota. Microbiome 8:55. doi: 10.1186/s40168-020-00827-8
Lopes, O. F., de Jesus, R. M., de Sousa, L. F., Rocha, F. A., da Silva, D. M. L., Amorim, A. F., et al. (2021). Comparison between water quality indices in watersheds of the Southern Bahia (Brazil) with different land use. Environ. Sci. Pollut. Res. 28, 12944–12959. doi: 10.1007/s11356-020-10941-7
Margot, J., Kienle, C., Magnet, A., Weil, M., Rossi, L., Alencastro, L. F. D., et al. (2013). Treatment of micropollutants in municipal wastewater: ozone or powdered activated carbon? Sci. Total Environ. 461–462, 480–498. doi: 10.1016/j.scitotenv.2013.05.034
Moran-Ordonez, A., Pavlova, A., Pinder, A. M., Sim, L., Sunnucks, P., Thompson, R. M., et al. (2015). Aquatic communities in arid landscapes: local conditions, dispersal traits and landscape configuration determine local biodiversity. Divers. Distrib. 21, 1230–1241. doi: 10.1111/ddi.12342
Moustaka-Gouni, M., Vardaka, E., Michaloudi, E., Kormas, K. A., Tryfon, E., Mihalatou, H., et al. (2006). Plankton food web structure in a eutrophic polymictic lake with a history of toxic cyanobacterial blooms. Limnol. Oceanogr. 51, 715–727. doi: 10.4319/lo.2006.51.1_part_2.0715
Muhammad, B. L., Lee, Y.-S., and Ki, J.-S. (2021). Molecular profiling of 18S rRNA reveals seasonal variation and diversity of diatoms community in the Han River, South Korea. J. Species Res. 10, 46–56.
Nekola, J. C., and White, P. S. (1999). The distance decay of similarity in biogeography and ecology. J. Biogeogr. 26, 867–878. doi: 10.1046/j.1365-2699.1999.00305.x
Oksanen, J., Kindt, R., Legendre, P. O., Hara, B., Stevens, M. H. H., Oksanen, M. J., et al. (2007). The vegan package. Community Ecol. Package 10, 631–637.
Padisak, J., Borics, G., Grigorszky, I., and Soroczki-Pinter, E. (2006). Use of phytoplankton assemblages for monitoring ecological status of lakes within the Water Framework Directive: the assemblage index. Hydrobiologia 553, 1–14. doi: 10.1007/s10750-005-1393-9
Parkes, S. M., and Duggan, I. C. (2012). Are zooplankton invasions in constructed waters facilitated by simple communities? Divers. Distrib. 18, 1199–1210. doi: 10.1111/j.1472-4642.2012.00913.x
Parreira de Castro, D. M., Doledec, S., and Callisto, M. (2018). Land cover disturbance homogenizes aquatic insect functional structure in neotropical savanna streams. Ecol. Indic. 84, 573–582. doi: 10.1016/j.ecolind.2017.09.030
Ptatscheck, C., Gansfort, B., Majdi, N., and Traunspurger, W. (2020). The influence of environmental and spatial factors on benthic invertebrate metacommunities differing in size and dispersal mode. Aquat. Ecol. 54, 447–461. doi: 10.1007/s10452-020-09752-2
Qu, Y., Wu, N., Guse, B., and Fohrer, N. (2018). Riverine phytoplankton shifting along a lentic-lotic continuum under hydrological, physiochemical conditions and species dispersal. Sci. Total Environ. 619–620, 1628–1636. doi: 10.1016/j.scitotenv.2017.10.139
Ren, Z., Niu, D., Ma, P., Wang, Y., Wang, Z., Fu, H., et al. (2020). Bacterial communities in stream biofilms in a degrading grassland watershed on the Qinghai-Tibet Plateau. Front. Microbiol. 11:1021. doi: 10.3389/fmicb.2020.01021
Santos, G. O., Hernandez, F. B. T., Ferraudo, A. S., Vanzela, L. S., and Santos, D. J. O. (2017). A study of the impact of land use and occupation on basin water quality through multivariate statistics. Engenharia Agricola 37, 453–462. doi: 10.1590/1809-4430-eng.agric.v37n3p453-462/2017
Shi, Z., Xu, J., Huang, X., Zhang, X., Jiang, Z., Ye, F., et al. (2017). Relationship between nutrients and plankton biomass in the turbidity maximum zone of the Pearl River Estuary. J. Environ. Sci. 57, 72–84. doi: 10.1016/j.jes.2016.11.013
Teng, Y., Yang, J., Zuo, R., and Wang, J. (2011). Impact of urbanization and industrialization upon surface water quality: a pilot study of Panzhihua mining town. J. Earth Sci. 22, 658–668. doi: 10.1007/s12583-011-0217-2
Tong, S., Wu-Qun, C., Qiu-Yu, B., Xiao, M., and Dong, L. (2021). Analysis on historical flood and countermeasures in prevention and control of flood in Daqing River Basin. Environ. Res. 196:110895. doi: 10.1016/j.envres.2021.110895
Uritskiy, G., Munn, A., Dailey, M., Gelsinger, D. R., Getsin, S., Davila, A., et al. (2020). Environmental Factors Driving Spatial Heterogeneity in Desert Halophile Microbial Communities. Front. Microbiol. 11:578669. doi: 10.3389/fmicb.2020.578669
Vilar, A. G., van Dam, H., van Loon, E. E., Vonk, J. A., van Der Geest, H. G., and Admiraal, W. (2014). Eutrophication decreases distance decay of similarity in diatom communities. Freshw. Biol. 59, 1522–1531. doi: 10.1111/fwb.12363
Wang, L., Cai, Q., Tan, L., and Kong, L. (2011). Phytoplankton development and ecological status during a cyanobacterial bloom in a tributary bay of the Three Gorges Reservoir, China. Sci. Total Environ. 409, 3820–3828. doi: 10.1016/j.scitotenv.2011.06.041
Wang, Y., Liu, L., Chen, H., and Yang, J. (2015). Spatiotemporal dynamics and determinants of planktonic bacterial and microeukaryotic communities in a Chinese subtropical river. Appl. Microbiol. Biotechnol. 99, 9255–9266. doi: 10.1007/s00253-015-6773-0
Weijters, M. J., Janse, J. H., Alkemade, R., and Verhoeven, J. T. A. (2009). Quantifying the effect of catchment land use and water nutrient concentrations on freshwater river and stream biodiversity. Aquat. Conserv. Mar. Freshw. Ecosyst. 19, 104–112. doi: 10.1016/j.scitotenv.2020.140950
Widder, S., Besemer, K., Singer, G. A., Ceola, S., Bertuzzo, E., Quince, C., et al. (2014). Fluvial network organization imprints on microbial co-occurrence networks. Proc. Natl. Acad. Sci. U. S. A. 111, 12799–12804. doi: 10.1073/pnas.1411723111
Wijewardene, L., Naicheng, W., Yueming, Q., Kun, G., Beata, M., Stefan, L., et al. (2021). Influences of pesticides, nutrients, and local environmental variables on phytoplankton communities in lentic small water bodies in a German lowland agricultural area. Sci. Total Environ. 780, 413–421. doi: 10.1016/j.scitotenv.2021.146481
Wu, N., Wang, Y., Wang, Y., Sun, X., Faber, C., and Fohrer, N. (2022). Environment regimes play an important role in structuring trait-and taxonomy-based temporal beta diversity of riverine diatoms. J. Ecol. doi: 10.5061/dryad.6q573n612
Wu, N., Zhou, S., Zhang, M., Peng, W., Guo, K., Qu, X., et al. (2021). Spatial and local environmental factors outweigh geo-climatic gradients in structuring taxonomically and trait-based b-diversity of benthic algae. J. Biogeogr. 48, 1842–1857. doi: 10.1111/jbi.14108
Xiong, W., Jousset, A., Guo, S., Karlsson, I., Zhao, Q., Wu, H., et al. (2018). Soil protist communities form a dynamic hub in the soil microbiome. ISME J. 12, 634–638. doi: 10.1038/ismej.2017.171
Xiong, W., Li, J., Chen, Y., Shan, B., Wang, W., and Zhan, A. (2016). Determinants of community structure of zooplankton in heavily polluted river ecosystems. Sci. Rep. 6:22043. doi: 10.1038/srep22043
Xu, H., Paerl, H. W., Qin, B., Zhu, G., and Gao, G. (2010). Nitrogen and phosphorus inputs control phytoplankton growth in eutrophic Lake Taihu, China. Limnol. Oceanogr. 55, 420–432. doi: 10.4319/lo.2010.55.1.0420
Xu, H., Zhang, S., Ma, G., Zhang, Y., Li, Y., and Pei, H. (2020). 18S rRNA gene sequencing reveals significant influence of anthropogenic effects on microeukaryote diversity and composition along a river-to-estuary gradient ecosystem. Sci. Total Environ. 705:135910. doi: 10.1016/j.scitotenv.2019.135910
Xu, Y., Liang, J., Lin, J., Lei, X., Ding, G., and Yuan, R. (2021). A study on the phytoplankton community structure in the Diaohe River section of the Middle Route of the South-to-North Water Diversion Project in winter. Water Supply 21, 959–970. doi: 10.2166/ws.2020.247
Yang, J., Yu, X., Liu, L., Zhang, W., and Guo, P. (2012). Algae community and trophic state of subtropical reservoirs in southeast Fujian, China. Environ. Sci. Pollut. Res. 19, 1432–1442. doi: 10.1007/s11356-011-0683-1
Yang, Y., Chen, H., Abdullah Al, M., Ndayishimiye, J. C., Yang, J. R., Isabwe, A., et al. (2022). Urbanization reduces resource use efficiency of phytoplankton community by altering the environment and decreasing biodiversity. J. Environ. Sci. 112, 140–151. doi: 10.1016/j.jes.2021.05.001
Yang, Y., Zhang, J., Yan, W., Zhang, Y., Wang, J., Wang, G., et al. (2021). Impact assessment of water diversion project on urban aquatic ecological environment. Ecol. Indic. 125:107496. doi: 10.1007/s11356-020-09182-5
Yin, D., Zheng, L., and Song, L. (2011). Spatio-temporal distribution of phytoplankton in the Danjiangkou Reservoir, a water source area for the Southto-North Water Diversion Project (Middle Route), China. Chin. J. Oceanol. Limnol. 29, 531–540. doi: 10.1007/s00343-011-0120-9
Yuksek, A., Okus, E. I, Yilmaz, N., Aslan-Yilmaz, A., and Tas, S. (2006). Changes in biodiversity of the extremely polluted Golden Horn Estuary following the improvements in water quality. Mar. Pollut. Bull. 52, 1209–1218. doi: 10.1016/j.marpolbul.2006.02.006
Zancarini, A., Echenique-Subiabre, I., Debroas, D., Taib, N., Quiblier, C., and Humbert, J. F. (2017). Deciphering biodiversity and interactions between bacteria and microeukaryotes within epilithic biofilms from the Loue River, France. Sci. Rep. 7:4344. doi: 10.1038/s41598-017-04016-w
Zhang, H., Zong, R., He, H., Liu, K., Yan, M., Miao, Y., et al. (2021). Biogeographic distribution patterns of algal community in different urban lakes in China: insights into the dynamics and co-existence. J. Environ. Sci. 100, 216–227. doi: 10.1016/j.jes.2020.07.024
Zhulidov, A. V., Kamari, J., Robarts, R. D., Pavlov, D. F., Rekolainen, S., Gurtovaya, T. Y., et al. (2011). Long-term dynamics of water-borne nitrogen, phosphorus and suspended solids in the lower Don River basin (Russian Federation). J. Water Clim. Chang. 2, 201–211. doi: 10.2166/wcc.2011.030
Keywords: high-throughput sequencing, community composition, microeukaryotes, co-occurrence network, phytoplankton
Citation: Wang Y, Ren Z, He P, Xu J, Li D, Liu C, Liu B and Wu N (2022) Microeukaryotic Community Shifting Along a Lentic-Lotic Continuum. Front. Ecol. Evol. 10:887787. doi: 10.3389/fevo.2022.887787
Received: 02 March 2022; Accepted: 25 March 2022;
Published: 02 May 2022.
Edited by:
Chao Wang, Pearl River Fisheries Research Institute, Chinese Academy of Fishery Sciences, ChinaReviewed by:
Kun Guo, East China Normal University, ChinaBeata Iwona Messyasz, Adam Mickiewicz University, Poland
Copyright © 2022 Wang, Ren, He, Xu, Li, Liu, Liu and Wu. This is an open-access article distributed under the terms of the Creative Commons Attribution License (CC BY). The use, distribution or reproduction in other forums is permitted, provided the original author(s) and the copyright owner(s) are credited and that the original publication in this journal is cited, in accordance with accepted academic practice. No use, distribution or reproduction is permitted which does not comply with these terms.
*Correspondence: Naicheng Wu, bmFpY2hlbmd3dTg4QGdtYWlsLmNvbQ==
†These authors have contributed equally to this work and share first authorship